How it works
Transform your enterprise with the scalable mindsets, skills, & behavior change that drive performance.
Explore how BetterUp connects to your core business systems.
We pair AI with the latest in human-centered coaching to drive powerful, lasting learning and behavior change.
Build leaders that accelerate team performance and engagement.
Unlock performance potential at scale with AI-powered curated growth journeys.
Build resilience, well-being and agility to drive performance across your entire enterprise.
Transform your business, starting with your sales leaders.
Unlock business impact from the top with executive coaching.
Foster a culture of inclusion and belonging.
Accelerate the performance and potential of your agencies and employees.
See how innovative organizations use BetterUp to build a thriving workforce.
Discover how BetterUp measurably impacts key business outcomes for organizations like yours.
Daring Leadership Institute: a groundbreaking partnership that amplifies Brené Brown's empirically based, courage-building curriculum with BetterUp’s human transformation platform.

- What is coaching?
Learn how 1:1 coaching works, who its for, and if it's right for you.
Accelerate your personal and professional growth with the expert guidance of a BetterUp Coach.
Types of Coaching
Navigate career transitions, accelerate your professional growth, and achieve your career goals with expert coaching.
Enhance your communication skills for better personal and professional relationships, with tailored coaching that focuses on your needs.
Find balance, resilience, and well-being in all areas of your life with holistic coaching designed to empower you.
Discover your perfect match : Take our 5-minute assessment and let us pair you with one of our top Coaches tailored just for you.
Find your coach
-1.png)
Research, expert insights, and resources to develop courageous leaders within your organization.
Best practices, research, and tools to fuel individual and business growth.
View on-demand BetterUp events and learn about upcoming live discussions.
The latest insights and ideas for building a high-performing workplace.
- BetterUp Briefing
The online magazine that helps you understand tomorrow's workforce trends, today.
Innovative research featured in peer-reviewed journals, press, and more.
Founded in 2022 to deepen the understanding of the intersection of well-being, purpose, and performance
We're on a mission to help everyone live with clarity, purpose, and passion.
Join us and create impactful change.
Read the buzz about BetterUp.
Meet the leadership that's passionate about empowering your workforce.

For Business
For Individuals

31 examples of problem solving performance review phrases

Jump to section
You're doing great
You should think of improving
Tips to improve
Use these practical examples of phrases, sample comments, and templates for your performance review , 360-degree feedback survey, or manager appraisal.
The following examples not only relate to problem-solving but also conflict management , effective solutions, selecting the best alternatives, decision making , problem identification, analyzing effectively, and generally becoming an effective problem-solving strategist. Start using effective performance review questions to help better guide your workforce's development.
Problem solving appraisal comments: you're doing great
- You always maintain an effective dialogue with clients when they have technical problems. Being clear and articulate makes sure our customers' faults are attended to promptly.
- You constantly make sure to look beyond the obvious you never stop at the first answer. You’re really good at exploring alternatives. Well done!
- Keeping the supervisors and managers informed of status changes and requests is important. You’re really good at communicating the changes to the projects at all times. Keep it up!
- You stay cool and collected even when things aren’t going according to plan or up in the air. This is a great trait to possess. Well done!
- You’re excellent at giving an honest and logical analysis. Keep it up! Effectively diagnosing complex problems and reaching sustainable solutions is one of your strong points.
- Your ability to ability to make complex systems into simple ones is truly a unique skill to possess. Well done!
- You often identify practical solutions to every roadblock. You’re a real asset to the team! Great job.
- You always listen actively and attentively to make sure you understand what the exact problem is and you come up with solutions in an effective manner.
- You have an amazing ability to clearly explain options and solutions effectively and efficiently. Well done!
- When driving projects, you can shift to other areas comfortably and easily. making sure the project runs smoothly. Great job!

Problem solving performance review phrases: you should think of improving
- You always seem too overwhelmed when faced with multiple problems. Try to think of ways to make problems more manageable so that they can be solved in a timely and effective manner.
- Avoiding conflicts constantly with people is not a good idea as you will only build up personal frustration and nothing will be done to remedy the situation. Try to face people when there are problems and rectify problems when they occur.
- Don’t allow demanding customers to rattle your cage too much. If they become too demanding, take a step back, regulate your emotions , and try to make use of online support tools to help you rectify problems these tools can help a lot!
- It’s necessary that you learn from your past mistakes . You cannot keep making the same mistakes , as this is not beneficial to the company.
- You tend to ask the same questions over and over again. Try to listen more attentively or take notes when colleagues are answering!
- Providing multiple solutions in an indirect and creative approach will allow you to be more effective at problem-solving . if you struggle with this typically through viewing the problem in a new and unusual light.
- You fail to provide staff with the appropriate amount of structure and direction. They must know the direction you wish them to go in to achieve their goals .
- You need to be able to recognize repetitive trends to solve problems promptly.
- You tend to have problems troubleshooting even the most basic of questions. As a problem solver and customer support person, it’s imperative that you can answer these questions easily.
- Read through your training manual and make sure you fully understand it before attempting questions again.

Performance review tips to improve problem solving
- Try to complain less about problems and come up with solutions to the problems more often. Complaining is not beneficial to progression and innovation.
- As a problem solver, it’s important to be able to handle multiple priorities under short deadlines.
- You need to be able to effectively distinguish between the cause and the symptoms of problems to solve them in an efficient and timely manner.
- Try to anticipate problems in advance before they become major roadblocks down the road.
- Try to view obstacles as opportunities to learn and thrive at the challenge of solving the problem.
- Remember to prioritize problems according to their degree of urgency. It's important that you spend the majority of your time on urgent tasks over menial ones.
- When putting plans into place, stick to them and make sure they are completed.
- When solving problems, try to allocate appropriate levels of resources when undertaking new projects. It is important to become as efficient and as effective as possible.
- Try to learn to pace yourself when solving problems to avoid burnout . You’re a great asset to the team and we cannot afford to lose at this point.
- Meeting regularly with your staff to review results is vital to the problem-solving process.
- Staff that has regular check-ins understand what it is that is required of them, what they are currently achieving, and areas they may need to improve. Try to hold one-on-one meetings every week.
Understand Yourself Better:
Big 5 Personality Test
Madeline Miles
Madeline is a writer, communicator, and storyteller who is passionate about using words to help drive positive change. She holds a bachelor's in English Creative Writing and Communication Studies and lives in Denver, Colorado. In her spare time, she's usually somewhere outside (preferably in the mountains) — and enjoys poetry and fiction.
25 performance review questions (and how to use them)
How a performance review template improves the feedback process, 10 performance review tips to drastically move the needle, 5 tactics for managing managers effectively — and why it matters, 53 performance review examples to boost growth, agile performance management: how to improve an agile team, 37 innovation and creativity appraisal comments, how to manage poor performance in 5 steps, how stanford executive education embraces vulnerability as a form of resilience, 10 problem-solving strategies to turn challenges on their head, teamwork skills self-appraisal comments: 40 example phrases, your complete guide to self-assessments (with examples), 30 communication feedback examples, 30 customer service review examples to develop your team, 15 tips for your end-of-year reviews, 8 creative solutions to your most challenging problems, stay connected with betterup, get our newsletter, event invites, plus product insights and research..
3100 E 5th Street, Suite 350 Austin, TX 78702
- Platform Overview
- Integrations
- Powered by AI
- BetterUp Lead™
- BetterUp Manage™
- BetterUp Care®
- Sales Performance
- Diversity & Inclusion
- Case Studies
- Why BetterUp?
- About Coaching
- Find your Coach
- Career Coaching
- Communication Coaching
- Personal Coaching
- News and Press
- Leadership Team
- Become a BetterUp Coach
- BetterUp Labs
- Center for Purpose & Performance
- Leadership Training
- Business Coaching
- Contact Support
- Contact Sales
- Privacy Policy
- Acceptable Use Policy
- Trust & Security
- Cookie Preferences
- Book a Demo
Struggling to overcome challenges in your life? We all face problems, big and small, on a regular basis.
So how do you tackle them effectively? What are some key problem-solving strategies and skills that can guide you?
Effective problem-solving requires breaking issues down logically, generating solutions creatively, weighing choices critically, and adapting plans flexibly based on outcomes. Useful strategies range from leveraging past solutions that have worked to visualizing problems through diagrams. Core skills include analytical abilities, innovative thinking, and collaboration.
Want to improve your problem-solving skills? Keep reading to find out 17 effective problem-solving strategies, key skills, common obstacles to watch for, and tips on improving your overall problem-solving skills.
Key Takeaways:
- Effective problem-solving requires breaking down issues logically, generating multiple solutions creatively, weighing choices critically, and adapting plans based on outcomes.
- Useful problem-solving strategies range from leveraging past solutions to brainstorming with groups to visualizing problems through diagrams and models.
- Core skills include analytical abilities, innovative thinking, decision-making, and team collaboration to solve problems.
- Common obstacles include fear of failure, information gaps, fixed mindsets, confirmation bias, and groupthink.
- Boosting problem-solving skills involves learning from experts, actively practicing, soliciting feedback, and analyzing others’ success.
- Onethread’s project management capabilities align with effective problem-solving tenets – facilitating structured solutions, tracking progress, and capturing lessons learned.
What Is Problem-Solving?
Problem-solving is the process of understanding an issue, situation, or challenge that needs to be addressed and then systematically working through possible solutions to arrive at the best outcome.
It involves critical thinking, analysis, logic, creativity, research, planning, reflection, and patience in order to overcome obstacles and find effective answers to complex questions or problems.
The ultimate goal is to implement the chosen solution successfully.
What Are Problem-Solving Strategies?
Problem-solving strategies are like frameworks or methodologies that help us solve tricky puzzles or problems we face in the workplace, at home, or with friends.
Imagine you have a big jigsaw puzzle. One strategy might be to start with the corner pieces. Another could be looking for pieces with the same colors.
Just like in puzzles, in real life, we use different plans or steps to find solutions to problems. These strategies help us think clearly, make good choices, and find the best answers without getting too stressed or giving up.
Why Is It Important To Know Different Problem-Solving Strategies?
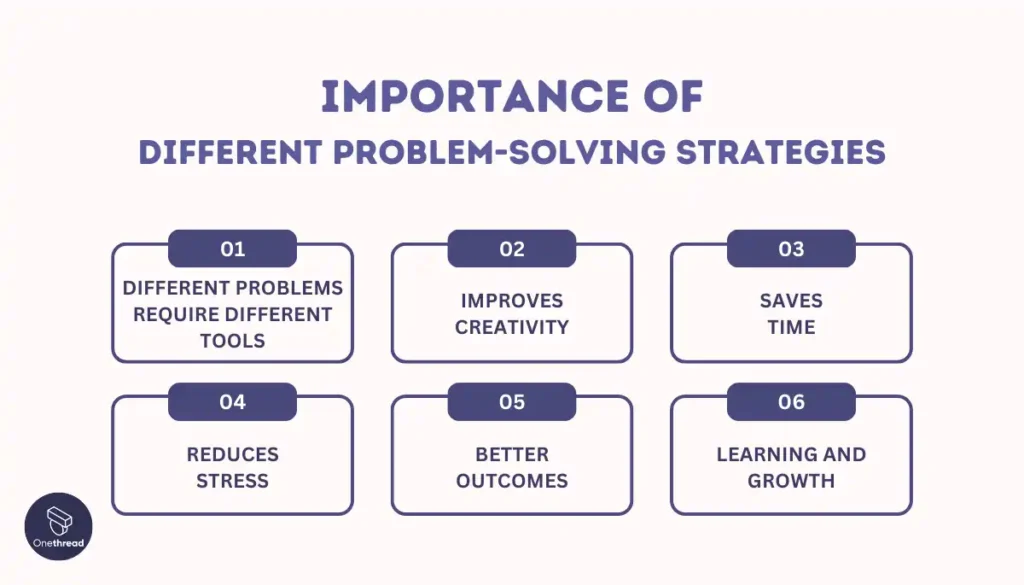
Knowing different problem-solving strategies is important because different types of problems often require different approaches to solve them effectively. Having a variety of strategies to choose from allows you to select the best method for the specific problem you are trying to solve.
This improves your ability to analyze issues thoroughly, develop solutions creatively, and tackle problems from multiple angles. Knowing multiple strategies also aids in overcoming roadblocks if your initial approach is not working.
Here are some reasons why you need to know different problem-solving strategies:
- Different Problems Require Different Tools: Just like you can’t use a hammer to fix everything, some problems need specific strategies to solve them.
- Improves Creativity: Knowing various strategies helps you think outside the box and come up with creative solutions.
- Saves Time: With the right strategy, you can solve problems faster instead of trying things that don’t work.
- Reduces Stress: When you know how to tackle a problem, it feels less scary and you feel more confident.
- Better Outcomes: Using the right strategy can lead to better solutions, making things work out better in the end.
- Learning and Growth: Each time you solve a problem, you learn something new, which makes you smarter and better at solving future problems.
Knowing different ways to solve problems helps you tackle anything that comes your way, making life a bit easier and more fun!
17 Effective Problem-Solving Strategies
Effective problem-solving strategies include breaking the problem into smaller parts, brainstorming multiple solutions, evaluating the pros and cons of each, and choosing the most viable option.
Critical thinking and creativity are essential in developing innovative solutions. Collaboration with others can also provide diverse perspectives and ideas.
By applying these strategies, you can tackle complex issues more effectively.
Now, consider a challenge you’re dealing with. Which strategy could help you find a solution? Here we will discuss key problem strategies in detail.
1. Use a Past Solution That Worked
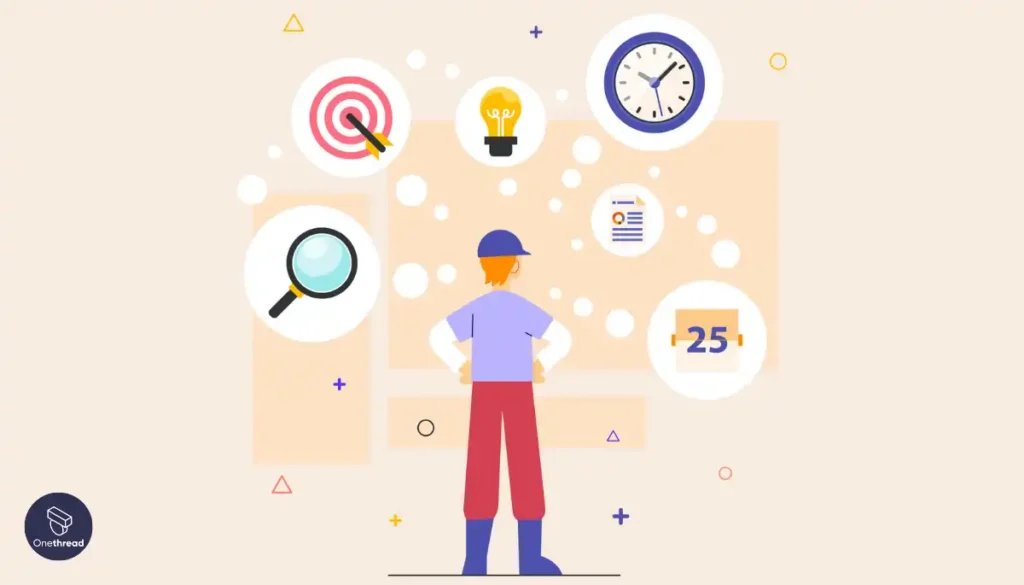
This strategy involves looking back at previous similar problems you have faced and the solutions that were effective in solving them.
It is useful when you are facing a problem that is very similar to something you have already solved. The main benefit is that you don’t have to come up with a brand new solution – you already know the method that worked before will likely work again.
However, the limitation is that the current problem may have some unique aspects or differences that mean your old solution is not fully applicable.
The ideal process is to thoroughly analyze the new challenge, identify the key similarities and differences versus the past case, adapt the old solution as needed to align with the current context, and then pilot it carefully before full implementation.
An example is using the same negotiation tactics from purchasing your previous home when putting in an offer on a new house. Key terms would be adjusted but overall it can save significant time versus developing a brand new strategy.
2. Brainstorm Solutions
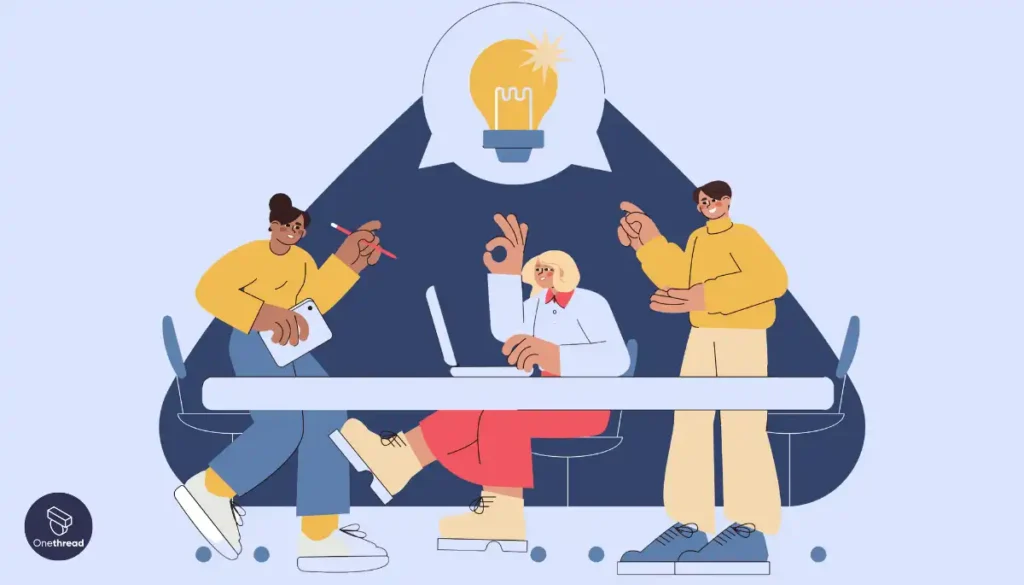
This involves gathering a group of people together to generate as many potential solutions to a problem as possible.
It is effective when you need creative ideas to solve a complex or challenging issue. By getting input from multiple people with diverse perspectives, you increase the likelihood of finding an innovative solution.
The main limitation is that brainstorming sessions can sometimes turn into unproductive gripe sessions or discussions rather than focusing on productive ideation —so they need to be properly facilitated.
The key to an effective brainstorming session is setting some basic ground rules upfront and having an experienced facilitator guide the discussion. Rules often include encouraging wild ideas, avoiding criticism of ideas during the ideation phase, and building on others’ ideas.
For instance, a struggling startup might hold a session where ideas for turnaround plans are generated and then formalized with financials and metrics.
3. Work Backward from the Solution
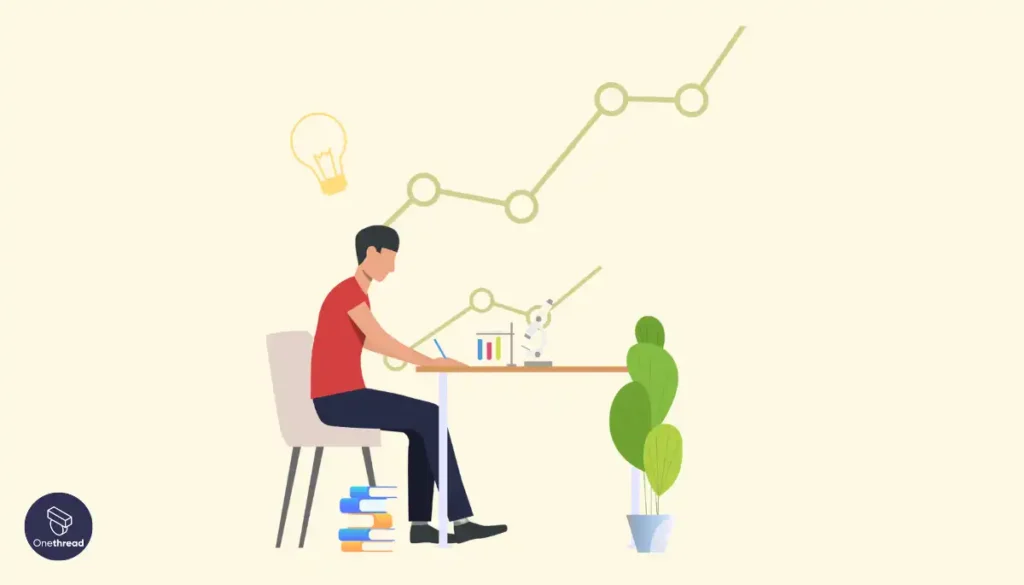
This technique involves envisioning that the problem has already been solved and then working step-by-step backward toward the current state.
This strategy is particularly helpful for long-term, multi-step problems. By starting from the imagined solution and identifying all the steps required to reach it, you can systematically determine the actions needed. It lets you tackle a big hairy problem through smaller, reversible steps.
A limitation is that this approach may not be possible if you cannot accurately envision the solution state to start with.
The approach helps drive logical systematic thinking for complex problem-solving, but should still be combined with creative brainstorming of alternative scenarios and solutions.
An example is planning for an event – you would imagine the successful event occurring, then determine the tasks needed the week before, two weeks before, etc. all the way back to the present.
4. Use the Kipling Method
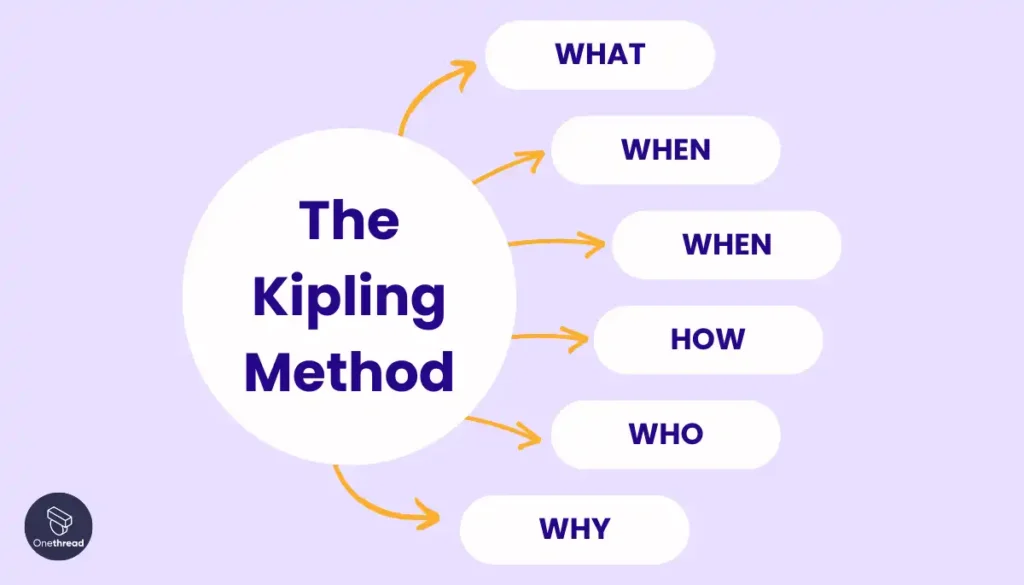
This method, named after author Rudyard Kipling, provides a framework for thoroughly analyzing a problem before jumping into solutions.
It consists of answering six fundamental questions: What, Where, When, How, Who, and Why about the challenge. Clearly defining these core elements of the problem sets the stage for generating targeted solutions.
The Kipling method enables a deep understanding of problem parameters and root causes before solution identification. By jumping to brainstorm solutions too early, critical information can be missed or the problem is loosely defined, reducing solution quality.
Answering the six fundamental questions illuminates all angles of the issue. This takes time but pays dividends in generating optimal solutions later tuned precisely to the true underlying problem.
The limitation is that meticulously working through numerous questions before addressing solutions can slow progress.
The best approach blends structured problem decomposition techniques like the Kipling method with spurring innovative solution ideation from a diverse team.
An example is using this technique after a technical process failure – the team would systematically detail What failed, Where/When did it fail, How it failed (sequence of events), Who was involved, and Why it likely failed before exploring preventative solutions.
5. Try Different Solutions Until One Works (Trial and Error)
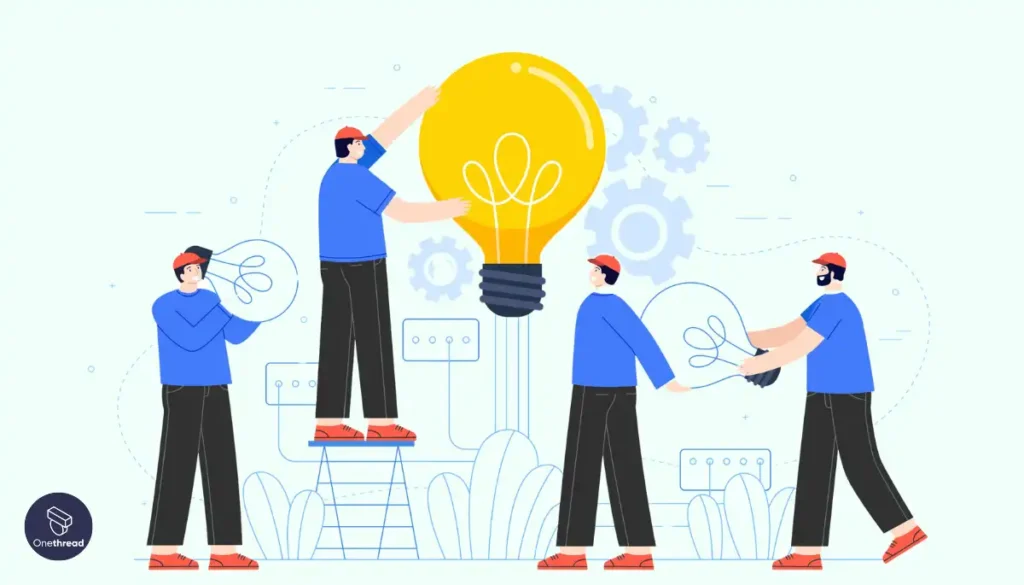
This technique involves attempting various potential solutions sequentially until finding one that successfully solves the problem.
Trial and error works best when facing a concrete, bounded challenge with clear solution criteria and a small number of discrete options to try. By methodically testing solutions, you can determine the faulty component.
A limitation is that it can be time-intensive if the working solution set is large.
The key is limiting the variable set first. For technical problems, this boundary is inherent and each element can be iteratively tested. But for business issues, artificial constraints may be required – setting decision rules upfront to reduce options before testing.
Furthermore, hypothesis-driven experimentation is far superior to blind trial and error – have logic for why Option A may outperform Option B.
Examples include fixing printer jams by testing different paper tray and cable configurations or resolving website errors by tweaking CSS/HTML line-by-line until the code functions properly.
6. Use Proven Formulas or Frameworks (Heuristics)
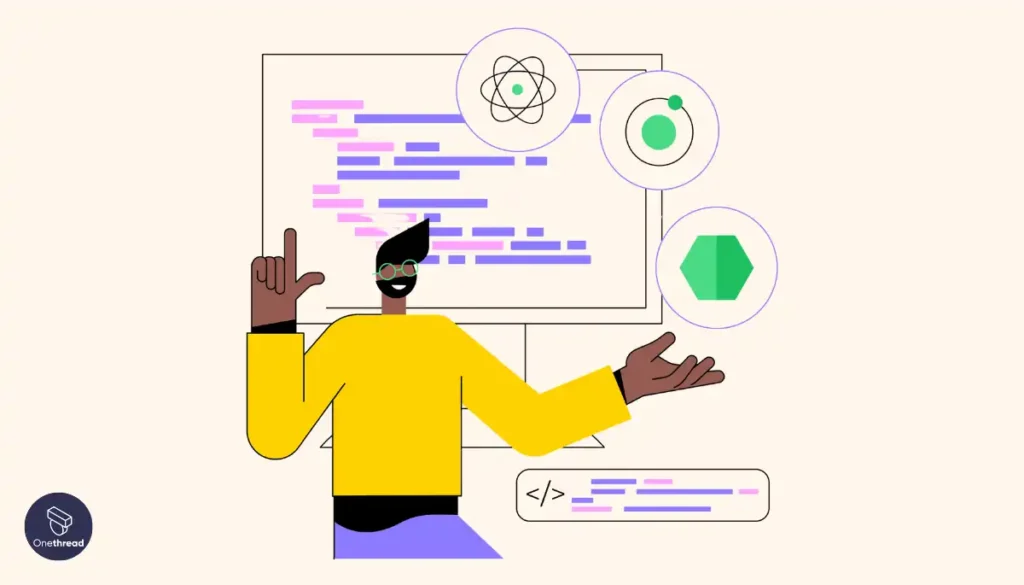
Heuristics refers to applying existing problem-solving formulas or frameworks rather than addressing issues completely from scratch.
This allows leveraging established best practices rather than reinventing the wheel each time.
It is effective when facing recurrent, common challenges where proven structured approaches exist.
However, heuristics may force-fit solutions to non-standard problems.
For example, a cost-benefit analysis can be used instead of custom weighting schemes to analyze potential process improvements.
Onethread allows teams to define, save, and replicate configurable project templates so proven workflows can be reliably applied across problems with some consistency rather than fully custom one-off approaches each time.
Try One thread
Experience One thread full potential, with all its features unlocked. Sign up now to start your 14-day free trial!
7. Trust Your Instincts (Insight Problem-Solving)
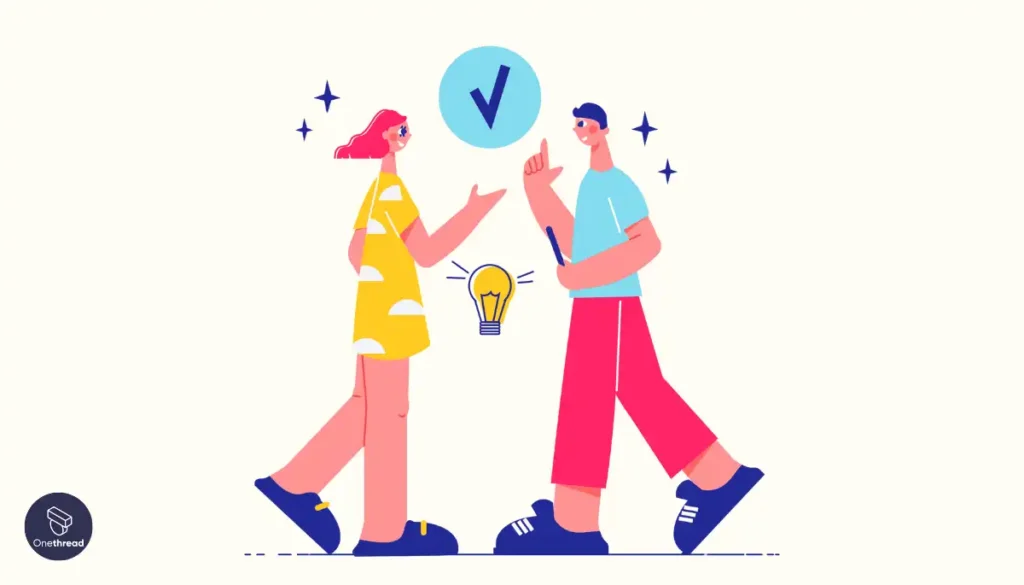
Insight is a problem-solving technique that involves waiting patiently for an unexpected “aha moment” when the solution pops into your mind.
It works well for personal challenges that require intuitive realizations over calculated logic. The unconscious mind makes connections leading to flashes of insight when relaxing or doing mundane tasks unrelated to the actual problem.
Benefits include out-of-the-box creative solutions. However, the limitations are that insights can’t be forced and may never come at all if too complex. Critical analysis is still required after initial insights.
A real-life example would be a writer struggling with how to end a novel. Despite extensive brainstorming, they feel stuck. Eventually while gardening one day, a perfect unexpected plot twist sparks an ideal conclusion. However, once written they still carefully review if the ending flows logically from the rest of the story.
8. Reverse Engineer the Problem
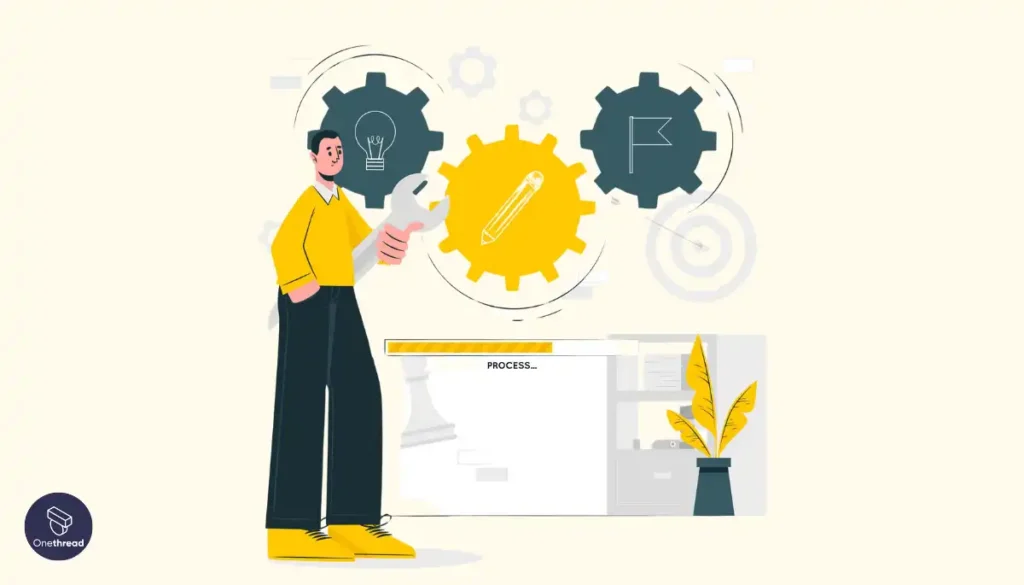
This approach involves deconstructing a problem in reverse sequential order from the current undesirable outcome back to the initial root causes.
By mapping the chain of events backward, you can identify the origin of where things went wrong and establish the critical junctures for solving it moving ahead. Reverse engineering provides diagnostic clarity on multi-step problems.
However, the limitation is that it focuses heavily on autopsying the past versus innovating improved future solutions.
An example is tracing back from a server outage, through the cascade of infrastructure failures that led to it finally terminating at the initial script error that triggered the crisis. This root cause would then inform the preventative measure.
9. Break Down Obstacles Between Current and Goal State (Means-End Analysis)
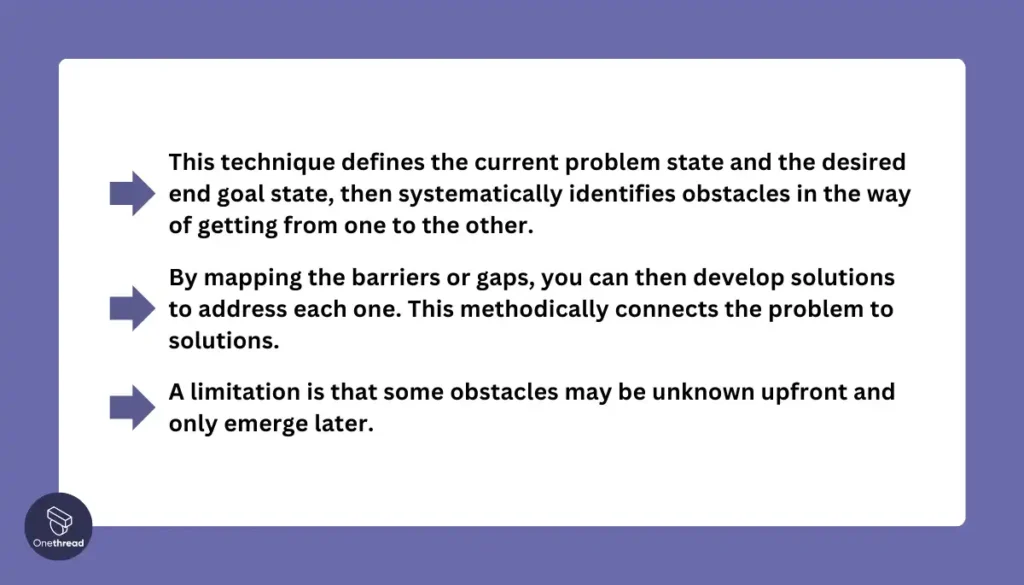
This technique defines the current problem state and the desired end goal state, then systematically identifies obstacles in the way of getting from one to the other.
By mapping the barriers or gaps, you can then develop solutions to address each one. This methodically connects the problem to solutions.
A limitation is that some obstacles may be unknown upfront and only emerge later.
For example, you can list down all the steps required for a new product launch – current state through production, marketing, sales, distribution, etc. to full launch (goal state) – to highlight where resource constraints or other blocks exist so they can be addressed.
Onethread allows dividing big-picture projects into discrete, manageable phases, milestones, and tasks to simplify execution just as problems can be decomposed into more achievable components. Features like dependency mapping further reinforce interconnections.
Using Onethread’s issues and subtasks feature, messy problems can be decomposed into manageable chunks.
10. Ask “Why” Five Times to Identify the Root Cause (The 5 Whys)
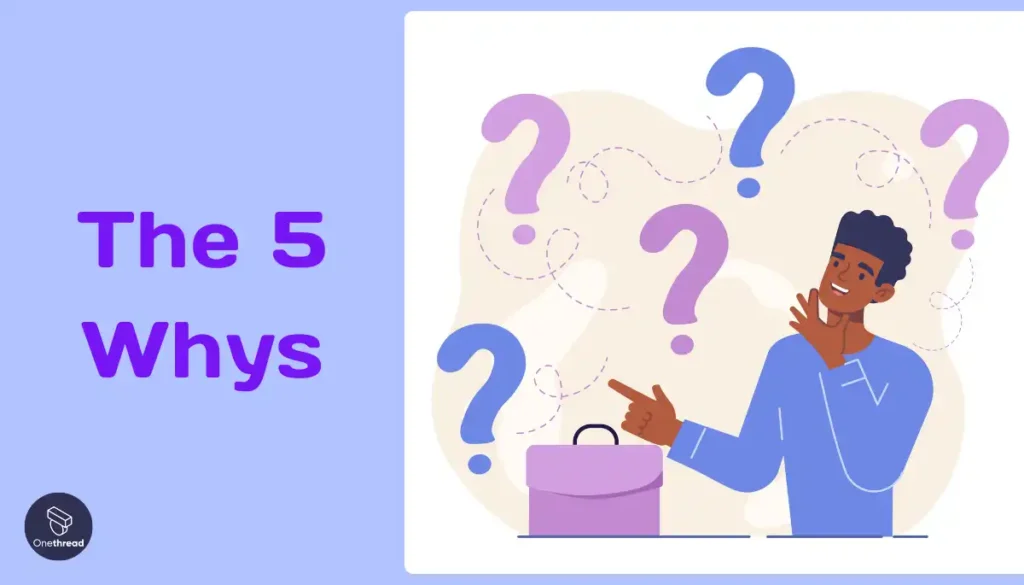
This technique involves asking “Why did this problem occur?” and then responding with an answer that is again met with asking “Why?” This process repeats five times until the root cause is revealed.
Continually asking why digs deeper from surface symptoms to underlying systemic issues.
It is effective for getting to the source of problems originating from human error or process breakdowns.
However, some complex issues may have multiple tangled root causes not solvable through this approach alone.
An example is a retail store experiencing a sudden decline in customers. Successively asking why five times may trace an initial drop to parking challenges, stemming from a city construction project – the true starting point to address.
11. Evaluate Strengths, Weaknesses, Opportunities, and Threats (SWOT Analysis)
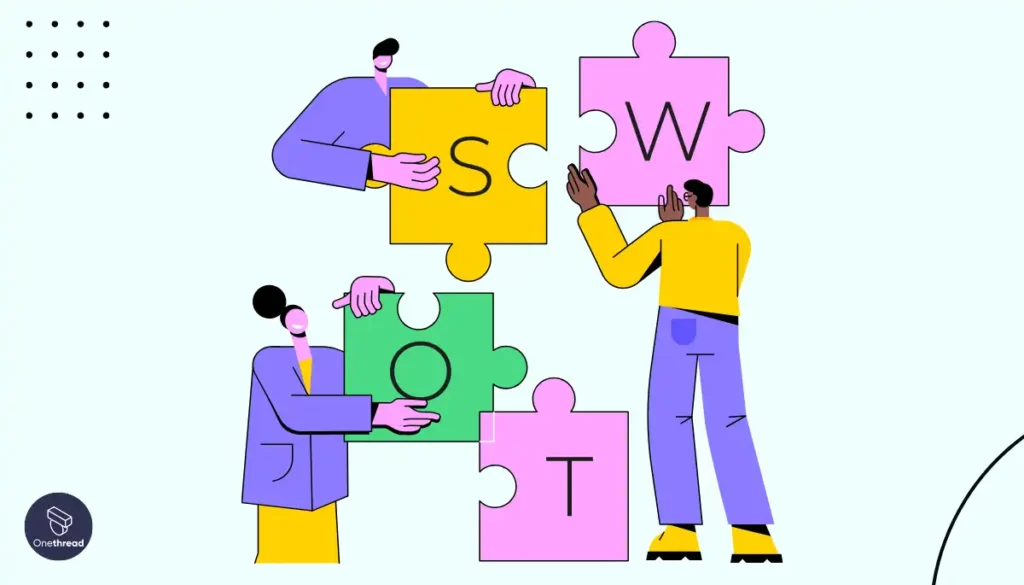
This involves analyzing a problem or proposed solution by categorizing internal and external factors into a 2×2 matrix: Strengths, Weaknesses as the internal rows; Opportunities and Threats as the external columns.
Systematically identifying these elements provides balanced insight to evaluate options and risks. It is impactful when evaluating alternative solutions or developing strategy amid complexity or uncertainty.
The key benefit of SWOT analysis is enabling multi-dimensional thinking when rationally evaluating options. Rather than getting anchored on just the upsides or the existing way of operating, it urges a systematic assessment through four different lenses:
- Internal Strengths: Our core competencies/advantages able to deliver success
- Internal Weaknesses: Gaps/vulnerabilities we need to manage
- External Opportunities: Ways we can differentiate/drive additional value
- External Threats: Risks we must navigate or mitigate
Multiperspective analysis provides the needed holistic view of the balanced risk vs. reward equation for strategic decision making amid uncertainty.
However, SWOT can feel restrictive if not tailored and evolved for different issue types.
Teams should view SWOT analysis as a starting point, augmenting it further for distinct scenarios.
An example is performing a SWOT analysis on whether a small business should expand into a new market – evaluating internal capabilities to execute vs. risks in the external competitive and demand environment to inform the growth decision with eyes wide open.
12. Compare Current vs Expected Performance (Gap Analysis)
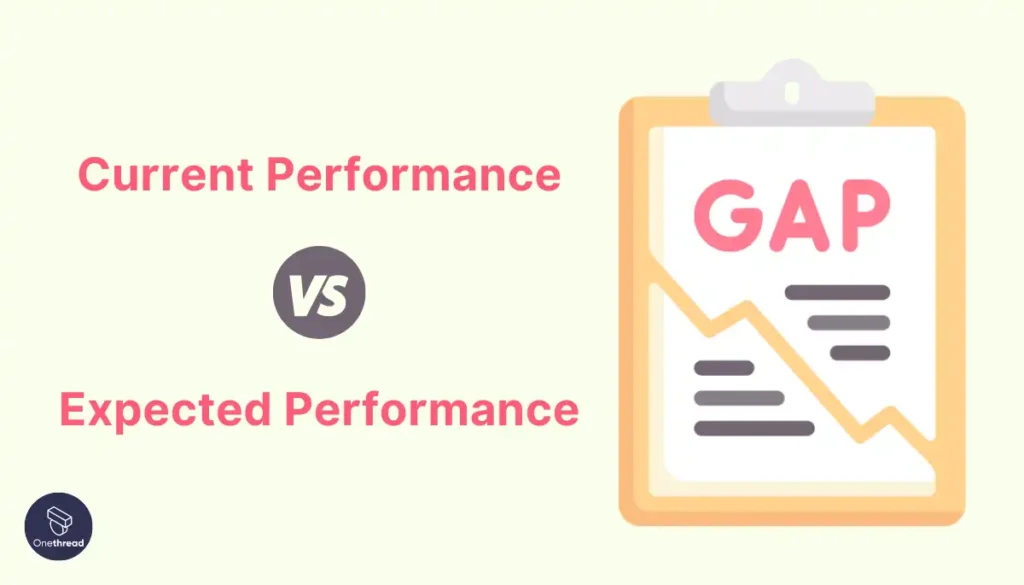
This technique involves comparing the current state of performance, output, or results to the desired or expected levels to highlight shortfalls.
By quantifying the gaps, you can identify problem areas and prioritize address solutions.
Gap analysis is based on the simple principle – “you can’t improve what you don’t measure.” It enables facts-driven problem diagnosis by highlighting delta to goals, not just vague dissatisfaction that something seems wrong. And measurement immediately suggests improvement opportunities – address the biggest gaps first.
This data orientation also supports ROI analysis on fixing issues – the return from closing larger gaps outweighs narrowly targeting smaller performance deficiencies.
However, the approach is only effective if robust standards and metrics exist as the benchmark to evaluate against. Organizations should invest upfront in establishing performance frameworks.
Furthermore, while numbers are invaluable, the human context behind problems should not be ignored – quantitative versus qualitative gap assessment is optimally blended.
For example, if usage declines are noted during software gap analysis, this could be used as a signal to improve user experience through design.
13. Observe Processes from the Frontline (Gemba Walk)
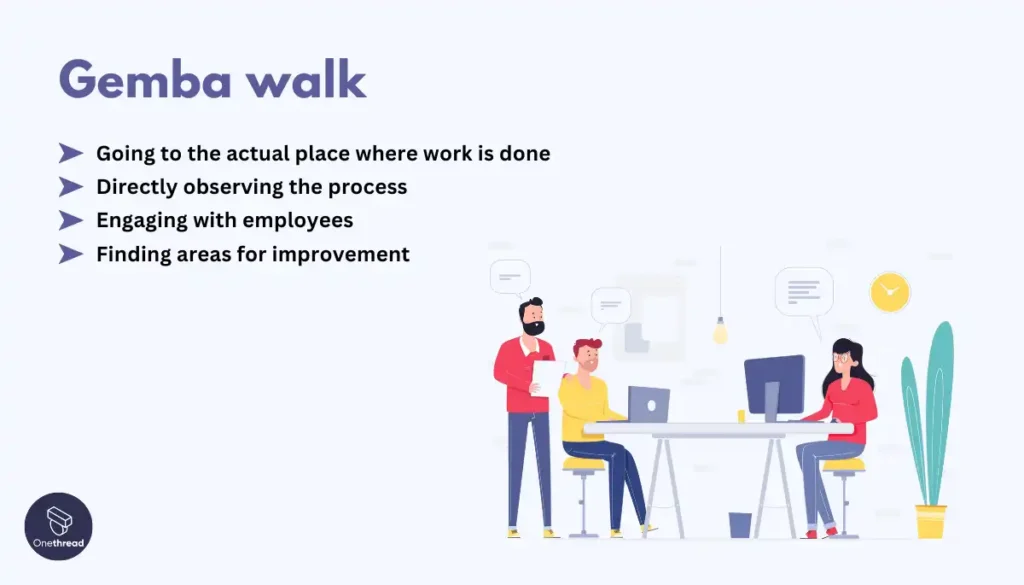
A Gemba walk involves going to the actual place where work is done, directly observing the process, engaging with employees, and finding areas for improvement.
By experiencing firsthand rather than solely reviewing abstract reports, practical problems and ideas emerge.
The limitation is Gemba walks provide anecdotes not statistically significant data. It complements but does not replace comprehensive performance measurement.
An example is a factory manager inspecting the production line to spot jam areas based on direct reality rather than relying on throughput dashboards alone back in her office. Frontline insights prove invaluable.
14. Analyze Competitive Forces (Porter’s Five Forces)
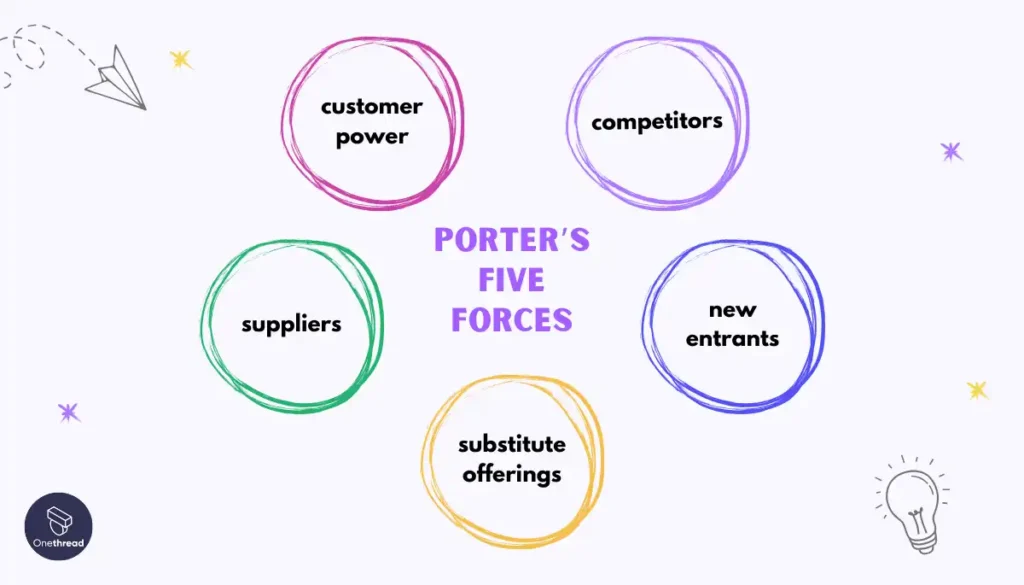
This involves assessing the marketplace around a problem or business situation via five key factors: competitors, new entrants, substitute offerings, suppliers, and customer power.
Evaluating these forces illuminates risks and opportunities for strategy development and issue resolution. It is effective for understanding dynamic external threats and opportunities when operating in a contested space.
However, over-indexing on only external factors can overlook the internal capabilities needed to execute solutions.
A startup CEO, for example, may analyze market entry barriers, whitespace opportunities, and disruption risks across these five forces to shape new product rollout strategies and marketing approaches.
15. Think from Different Perspectives (Six Thinking Hats)
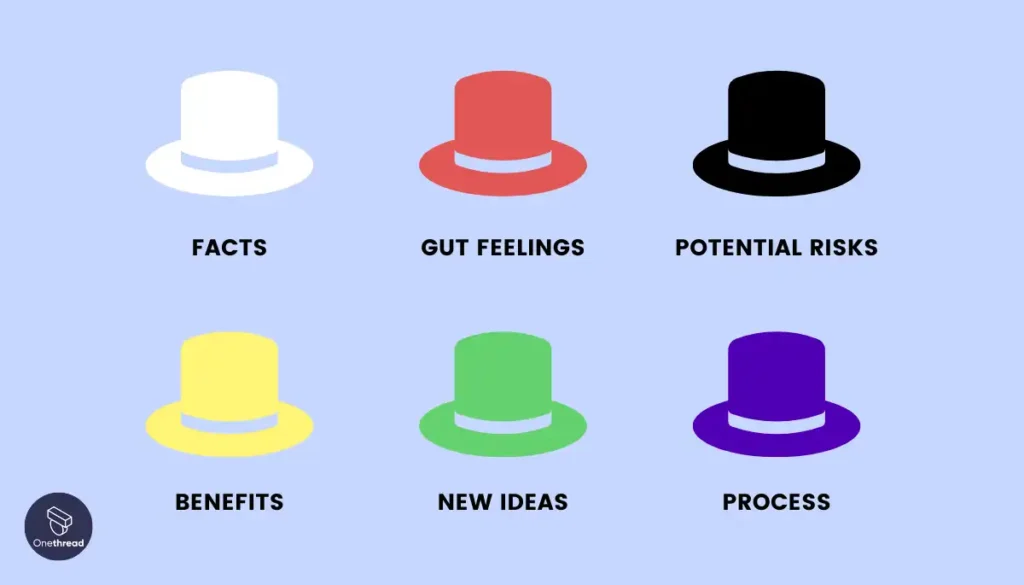
The Six Thinking Hats is a technique developed by Edward de Bono that encourages people to think about a problem from six different perspectives, each represented by a colored “thinking hat.”
The key benefit of this strategy is that it pushes team members to move outside their usual thinking style and consider new angles. This brings more diverse ideas and solutions to the table.
It works best for complex problems that require innovative solutions and when a team is stuck in an unproductive debate. The structured framework keeps the conversation flowing in a positive direction.
Limitations are that it requires training on the method itself and may feel unnatural at first. Team dynamics can also influence success – some members may dominate certain “hats” while others remain quiet.
A real-life example is a software company debating whether to build a new feature. The white hat focuses on facts, red on gut feelings, black on potential risks, yellow on benefits, green on new ideas, and blue on process. This exposes more balanced perspectives before deciding.
Onethread centralizes diverse stakeholder communication onto one platform, ensuring all voices are incorporated when evaluating project tradeoffs, just as problem-solving should consider multifaceted solutions.
16. Visualize the Problem (Draw it Out)
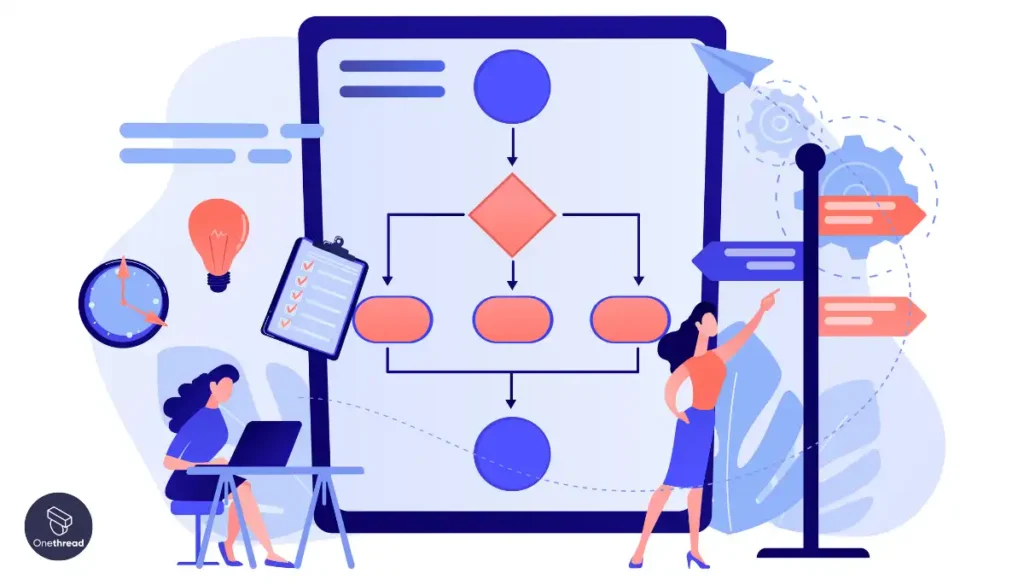
Drawing out a problem involves creating visual representations like diagrams, flowcharts, and maps to work through challenging issues.
This strategy is helpful when dealing with complex situations with lots of interconnected components. The visuals simplify the complexity so you can thoroughly understand the problem and all its nuances.
Key benefits are that it allows more stakeholders to get on the same page regarding root causes and it sparks new creative solutions as connections are made visually.
However, simple problems with few variables don’t require extensive diagrams. Additionally, some challenges are so multidimensional that fully capturing every aspect is difficult.
A real-life example would be mapping out all the possible causes leading to decreased client satisfaction at a law firm. An intricate fishbone diagram with branches for issues like service delivery, technology, facilities, culture, and vendor partnerships allows the team to trace problems back to their origins and brainstorm targeted fixes.
17. Follow a Step-by-Step Procedure (Algorithms)
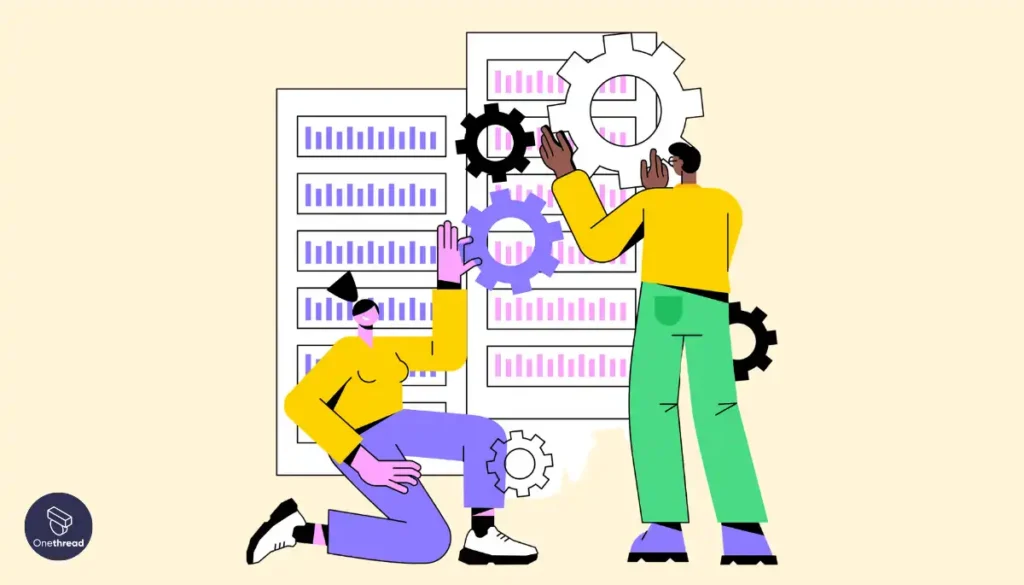
An algorithm is a predefined step-by-step process that is guaranteed to produce the correct solution if implemented properly.
Using algorithms is effective when facing problems that have clear, binary right and wrong answers. Algorithms work for mathematical calculations, computer code, manufacturing assembly lines, and scientific experiments.
Key benefits are consistency, accuracy, and efficiency. However, they require extensive upfront development and only apply to scenarios with strict parameters. Additionally, human error can lead to mistakes.
For example, crew members of fast food chains like McDonald’s follow specific algorithms for food prep – from grill times to ingredient amounts in sandwiches, to order fulfillment procedures. This ensures uniform quality and service across all locations. However, if a step is missed, errors occur.
The Problem-Solving Process
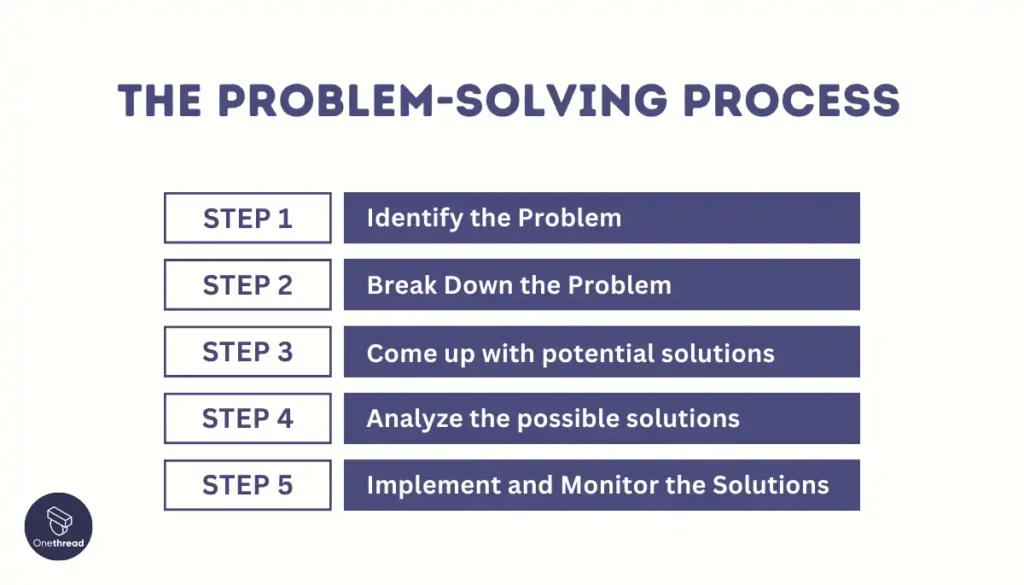
The problem-solving process typically includes defining the issue, analyzing details, creating solutions, weighing choices, acting, and reviewing results.
In the above, we have discussed several problem-solving strategies. For every problem-solving strategy, you have to follow these processes. Here’s a detailed step-by-step process of effective problem-solving:
Step 1: Identify the Problem
The problem-solving process starts with identifying the problem. This step involves understanding the issue’s nature, its scope, and its impact. Once the problem is clearly defined, it sets the foundation for finding effective solutions.
Identifying the problem is crucial. It means figuring out exactly what needs fixing. This involves looking at the situation closely, understanding what’s wrong, and knowing how it affects things. It’s about asking the right questions to get a clear picture of the issue.
This step is important because it guides the rest of the problem-solving process. Without a clear understanding of the problem, finding a solution is much harder. It’s like diagnosing an illness before treating it. Once the problem is identified accurately, you can move on to exploring possible solutions and deciding on the best course of action.
Step 2: Break Down the Problem
Breaking down the problem is a key step in the problem-solving process. It involves dividing the main issue into smaller, more manageable parts. This makes it easier to understand and tackle each component one by one.
After identifying the problem, the next step is to break it down. This means splitting the big issue into smaller pieces. It’s like solving a puzzle by handling one piece at a time.
By doing this, you can focus on each part without feeling overwhelmed. It also helps in identifying the root causes of the problem. Breaking down the problem allows for a clearer analysis and makes finding solutions more straightforward.
Each smaller problem can be addressed individually, leading to an effective resolution of the overall issue. This approach not only simplifies complex problems but also aids in developing a systematic plan to solve them.
Step 3: Come up with potential solutions
Coming up with potential solutions is the third step in the problem-solving process. It involves brainstorming various options to address the problem, considering creativity and feasibility to find the best approach.
After breaking down the problem, it’s time to think of ways to solve it. This stage is about brainstorming different solutions. You look at the smaller issues you’ve identified and start thinking of ways to fix them. This is where creativity comes in.
You want to come up with as many ideas as possible, no matter how out-of-the-box they seem. It’s important to consider all options and evaluate their pros and cons. This process allows you to gather a range of possible solutions.
Later, you can narrow these down to the most practical and effective ones. This step is crucial because it sets the stage for deciding on the best solution to implement. It’s about being open-minded and innovative to tackle the problem effectively.
Step 4: Analyze the possible solutions
Analyzing the possible solutions is the fourth step in the problem-solving process. It involves evaluating each proposed solution’s advantages and disadvantages to determine the most effective and feasible option.
After coming up with potential solutions, the next step is to analyze them. This means looking closely at each idea to see how well it solves the problem. You weigh the pros and cons of every solution.
Consider factors like cost, time, resources, and potential outcomes. This analysis helps in understanding the implications of each option. It’s about being critical and objective, ensuring that the chosen solution is not only effective but also practical.
This step is vital because it guides you towards making an informed decision. It involves comparing the solutions against each other and selecting the one that best addresses the problem.
By thoroughly analyzing the options, you can move forward with confidence, knowing you’ve chosen the best path to solve the issue.
Step 5: Implement and Monitor the Solutions
Implementing and monitoring the solutions is the final step in the problem-solving process. It involves putting the chosen solution into action and observing its effectiveness, making adjustments as necessary.
Once you’ve selected the best solution, it’s time to put it into practice. This step is about action. You implement the chosen solution and then keep an eye on how it works. Monitoring is crucial because it tells you if the solution is solving the problem as expected.
If things don’t go as planned, you may need to make some changes. This could mean tweaking the current solution or trying a different one. The goal is to ensure the problem is fully resolved.
This step is critical because it involves real-world application. It’s not just about planning; it’s about doing and adjusting based on results. By effectively implementing and monitoring the solutions, you can achieve the desired outcome and solve the problem successfully.
Why This Process is Important
Following a defined process to solve problems is important because it provides a systematic, structured approach instead of a haphazard one. Having clear steps guides logical thinking, analysis, and decision-making to increase effectiveness. Key reasons it helps are:
- Clear Direction: This process gives you a clear path to follow, which can make solving problems less overwhelming.
- Better Solutions: Thoughtful analysis of root causes, iterative testing of solutions, and learning orientation lead to addressing the heart of issues rather than just symptoms.
- Saves Time and Energy: Instead of guessing or trying random things, this process helps you find a solution more efficiently.
- Improves Skills: The more you use this process, the better you get at solving problems. It’s like practicing a sport. The more you practice, the better you play.
- Maximizes collaboration: Involving various stakeholders in the process enables broader inputs. Their communication and coordination are streamlined through organized brainstorming and evaluation.
- Provides consistency: Standard methodology across problems enables building institutional problem-solving capabilities over time. Patterns emerge on effective techniques to apply to different situations.
The problem-solving process is a powerful tool that can help us tackle any challenge we face. By following these steps, we can find solutions that work and learn important skills along the way.
Key Skills for Efficient Problem Solving
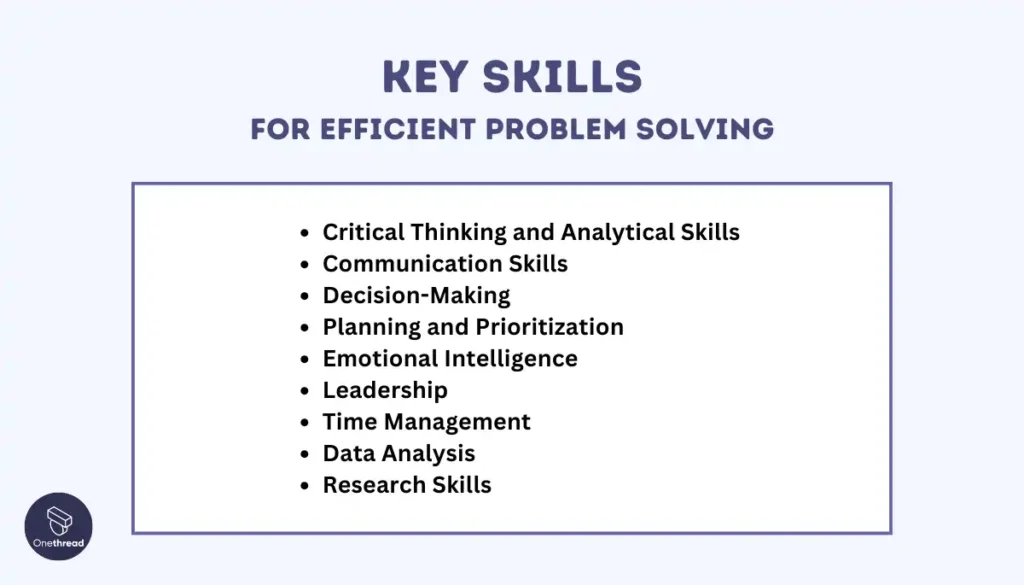
Efficient problem-solving requires breaking down issues logically, evaluating options, and implementing practical solutions.
Key skills include critical thinking to understand root causes, creativity to brainstorm innovative ideas, communication abilities to collaborate with others, and decision-making to select the best way forward. Staying adaptable, reflecting on outcomes, and applying lessons learned are also essential.
With practice, these capacities will lead to increased personal and team effectiveness in systematically addressing any problem.
Let’s explore the powers you need to become a problem-solving hero!
Critical Thinking and Analytical Skills
Critical thinking and analytical skills are vital for efficient problem-solving as they enable individuals to objectively evaluate information, identify key issues, and generate effective solutions.
These skills facilitate a deeper understanding of problems, leading to logical, well-reasoned decisions. By systematically breaking down complex issues and considering various perspectives, individuals can develop more innovative and practical solutions, enhancing their problem-solving effectiveness.
Communication Skills
Effective communication skills are essential for efficient problem-solving as they facilitate clear sharing of information, ensuring all team members understand the problem and proposed solutions.
These skills enable individuals to articulate issues, listen actively, and collaborate effectively, fostering a productive environment where diverse ideas can be exchanged and refined. By enhancing mutual understanding, communication skills contribute significantly to identifying and implementing the most viable solutions.
Decision-Making
Strong decision-making skills are crucial for efficient problem-solving, as they enable individuals to choose the best course of action from multiple alternatives.
These skills involve evaluating the potential outcomes of different solutions, considering the risks and benefits, and making informed choices. Effective decision-making leads to the implementation of solutions that are likely to resolve problems effectively, ensuring resources are used efficiently and goals are achieved.
Planning and Prioritization
Planning and prioritization are key for efficient problem-solving, ensuring resources are allocated effectively to address the most critical issues first. This approach helps in organizing tasks according to their urgency and impact, streamlining efforts towards achieving the desired outcome efficiently.
Emotional Intelligence
Emotional intelligence enhances problem-solving by allowing individuals to manage emotions, understand others, and navigate social complexities. It fosters a positive, collaborative environment, essential for generating creative solutions and making informed, empathetic decisions.
Leadership skills drive efficient problem-solving by inspiring and guiding teams toward common goals. Effective leaders motivate their teams, foster innovation, and navigate challenges, ensuring collective efforts are focused and productive in addressing problems.
Time Management
Time management is crucial in problem-solving, enabling individuals to allocate appropriate time to each task. By efficiently managing time, one can ensure that critical problems are addressed promptly without neglecting other responsibilities.
Data Analysis
Data analysis skills are essential for problem-solving, as they enable individuals to sift through data, identify trends, and extract actionable insights. This analytical approach supports evidence-based decision-making, leading to more accurate and effective solutions.
Research Skills
Research skills are vital for efficient problem-solving, allowing individuals to gather relevant information, explore various solutions, and understand the problem’s context. This thorough exploration aids in developing well-informed, innovative solutions.
Becoming a great problem solver takes practice, but with these skills, you’re on your way to becoming a problem-solving hero.
How to Improve Your Problem-Solving Skills?
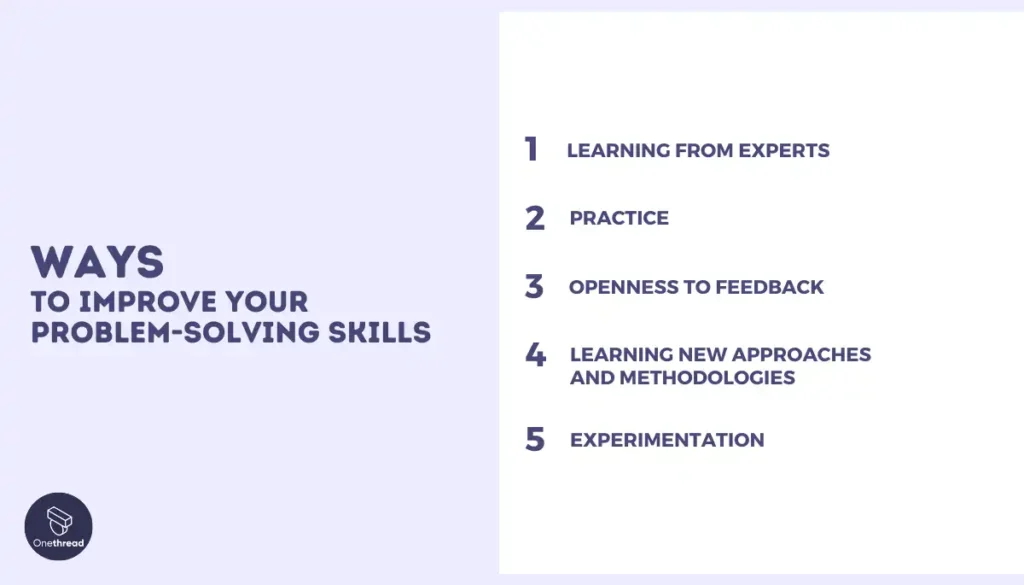
Improving your problem-solving skills can make you a master at overcoming challenges. Learn from experts, practice regularly, welcome feedback, try new methods, experiment, and study others’ success to become better.
Learning from Experts
Improving problem-solving skills by learning from experts involves seeking mentorship, attending workshops, and studying case studies. Experts provide insights and techniques that refine your approach, enhancing your ability to tackle complex problems effectively.
To enhance your problem-solving skills, learning from experts can be incredibly beneficial. Engaging with mentors, participating in specialized workshops, and analyzing case studies from seasoned professionals can offer valuable perspectives and strategies.
Experts share their experiences, mistakes, and successes, providing practical knowledge that can be applied to your own problem-solving process. This exposure not only broadens your understanding but also introduces you to diverse methods and approaches, enabling you to tackle challenges more efficiently and creatively.
Improving problem-solving skills through practice involves tackling a variety of challenges regularly. This hands-on approach helps in refining techniques and strategies, making you more adept at identifying and solving problems efficiently.
One of the most effective ways to enhance your problem-solving skills is through consistent practice. By engaging with different types of problems on a regular basis, you develop a deeper understanding of various strategies and how they can be applied.
This hands-on experience allows you to experiment with different approaches, learn from mistakes, and build confidence in your ability to tackle challenges.
Regular practice not only sharpens your analytical and critical thinking skills but also encourages adaptability and innovation, key components of effective problem-solving.
Openness to Feedback
Being open to feedback is like unlocking a secret level in a game. It helps you boost your problem-solving skills. Improving problem-solving skills through openness to feedback involves actively seeking and constructively responding to critiques.
This receptivity enables you to refine your strategies and approaches based on insights from others, leading to more effective solutions.
Learning New Approaches and Methodologies
Learning new approaches and methodologies is like adding new tools to your toolbox. It makes you a smarter problem-solver. Enhancing problem-solving skills by learning new approaches and methodologies involves staying updated with the latest trends and techniques in your field.
This continuous learning expands your toolkit, enabling innovative solutions and a fresh perspective on challenges.
Experimentation
Experimentation is like being a scientist of your own problems. It’s a powerful way to improve your problem-solving skills. Boosting problem-solving skills through experimentation means trying out different solutions to see what works best. This trial-and-error approach fosters creativity and can lead to unique solutions that wouldn’t have been considered otherwise.
Analyzing Competitors’ Success
Analyzing competitors’ success is like being a detective. It’s a smart way to boost your problem-solving skills. Improving problem-solving skills by analyzing competitors’ success involves studying their strategies and outcomes. Understanding what worked for them can provide valuable insights and inspire effective solutions for your own challenges.
Challenges in Problem-Solving
Facing obstacles when solving problems is common. Recognizing these barriers, like fear of failure or lack of information, helps us find ways around them for better solutions.
Fear of Failure
Fear of failure is like a big, scary monster that stops us from solving problems. It’s a challenge many face. Because being afraid of making mistakes can make us too scared to try new solutions.
How can we overcome this? First, understand that it’s okay to fail. Failure is not the opposite of success; it’s part of learning. Every time we fail, we discover one more way not to solve a problem, getting us closer to the right solution. Treat each attempt like an experiment. It’s not about failing; it’s about testing and learning.
Lack of Information
Lack of information is like trying to solve a puzzle with missing pieces. It’s a big challenge in problem-solving. Because without all the necessary details, finding a solution is much harder.
How can we fix this? Start by gathering as much information as you can. Ask questions, do research, or talk to experts. Think of yourself as a detective looking for clues. The more information you collect, the clearer the picture becomes. Then, use what you’ve learned to think of solutions.
Fixed Mindset
A fixed mindset is like being stuck in quicksand; it makes solving problems harder. It means thinking you can’t improve or learn new ways to solve issues.
How can we change this? First, believe that you can grow and learn from challenges. Think of your brain as a muscle that gets stronger every time you use it. When you face a problem, instead of saying “I can’t do this,” try thinking, “I can’t do this yet.” Look for lessons in every challenge and celebrate small wins.
Everyone starts somewhere, and mistakes are just steps on the path to getting better. By shifting to a growth mindset, you’ll see problems as opportunities to grow. Keep trying, keep learning, and your problem-solving skills will soar!
Jumping to Conclusions
Jumping to conclusions is like trying to finish a race before it starts. It’s a challenge in problem-solving. That means making a decision too quickly without looking at all the facts.
How can we avoid this? First, take a deep breath and slow down. Think about the problem like a puzzle. You need to see all the pieces before you know where they go. Ask questions, gather information, and consider different possibilities. Don’t choose the first solution that comes to mind. Instead, compare a few options.
Feeling Overwhelmed
Feeling overwhelmed is like being buried under a mountain of puzzles. It’s a big challenge in problem-solving. When we’re overwhelmed, everything seems too hard to handle.
How can we deal with this? Start by taking a step back. Breathe deeply and focus on one thing at a time. Break the big problem into smaller pieces, like sorting puzzle pieces by color. Tackle each small piece one by one. It’s also okay to ask for help. Sometimes, talking to someone else can give you a new perspective.
Confirmation Bias
Confirmation bias is like wearing glasses that only let you see what you want to see. It’s a challenge in problem-solving. Because it makes us focus only on information that agrees with what we already believe, ignoring anything that doesn’t.
How can we overcome this? First, be aware that you might be doing it. It’s like checking if your glasses are on right. Then, purposely look for information that challenges your views. It’s like trying on a different pair of glasses to see a new perspective. Ask questions and listen to answers, even if they don’t fit what you thought before.
Groupthink is like everyone in a group deciding to wear the same outfit without asking why. It’s a challenge in problem-solving. It means making decisions just because everyone else agrees, without really thinking it through.
How can we avoid this? First, encourage everyone in the group to share their ideas, even if they’re different. It’s like inviting everyone to show their unique style of clothes.
Listen to all opinions and discuss them. It’s okay to disagree; it helps us think of better solutions. Also, sometimes, ask someone outside the group for their thoughts. They might see something everyone in the group missed.
Overcoming obstacles in problem-solving requires patience, openness, and a willingness to learn from mistakes. By recognizing these barriers, we can develop strategies to navigate around them, leading to more effective and creative solutions.
What are the most common problem-solving techniques?
The most common techniques include brainstorming, the 5 Whys, mind mapping, SWOT analysis, and using algorithms or heuristics. Each approach has its strengths, suitable for different types of problems.
What’s the best problem-solving strategy for every situation?
There’s no one-size-fits-all strategy. The best approach depends on the problem’s complexity, available resources, and time constraints. Combining multiple techniques often yields the best results.
How can I improve my problem-solving skills?
Improve your problem-solving skills by practicing regularly, learning from experts, staying open to feedback, and continuously updating your knowledge on new approaches and methodologies.
Are there any tools or resources to help with problem-solving?
Yes, tools like mind mapping software, online courses on critical thinking, and books on problem-solving techniques can be very helpful. Joining forums or groups focused on problem-solving can also provide support and insights.
What are some common mistakes people make when solving problems?
Common mistakes include jumping to conclusions without fully understanding the problem, ignoring valuable feedback, sticking to familiar solutions without considering alternatives, and not breaking down complex problems into manageable parts.
Final Words
Mastering problem-solving strategies equips us with the tools to tackle challenges across all areas of life. By understanding and applying these techniques, embracing a growth mindset, and learning from both successes and obstacles, we can transform problems into opportunities for growth. Continuously improving these skills ensures we’re prepared to face and solve future challenges more effectively.
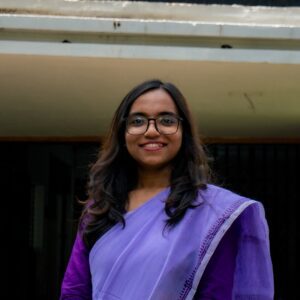
Let's Get Started with Onethread
Onethread empowers you to plan, organise, and track projects with ease, ensuring you meet deadlines, allocate resources efficiently, and keep progress transparent.
By subscribing you agree to our Privacy Policy .
Giving modern marketing teams superpowers with short links that stand out.
- Live Product Demo
© Copyright 2023 Onethread, Inc
HYPOTHESIS AND THEORY article
Impact of cognitive abilities and prior knowledge on complex problem solving performance – empirical results and a plea for ecologically valid microworlds.
- 1 Institute of Psychology, Otto-von-Guericke University Magdeburg, Magdeburg, Germany
- 2 Hector Research Institute of Education Sciences and Psychology, University of Tübingen, Tübingen, Germany
The original aim of complex problem solving (CPS) research was to bring the cognitive demands of complex real-life problems into the lab in order to investigate problem solving behavior and performance under controlled conditions. Up until now, the validity of psychometric intelligence constructs has been scrutinized with regard to its importance for CPS performance. At the same time, different CPS measurement approaches competing for the title of the best way to assess CPS have been developed. In the first part of the paper, we investigate the predictability of CPS performance on the basis of the Berlin Intelligence Structure Model and Cattell’s investment theory as well as an elaborated knowledge taxonomy. In the first study, 137 students managed a simulated shirt factory ( Tailorshop ; i.e., a complex real life-oriented system) twice, while in the second study, 152 students completed a forestry scenario ( FSYS ; i.e., a complex artificial world system). The results indicate that reasoning – specifically numerical reasoning (Studies 1 and 2) and figural reasoning (Study 2) – are the only relevant predictors among the intelligence constructs. We discuss the results with reference to the Brunswik symmetry principle. Path models suggest that reasoning and prior knowledge influence problem solving performance in the Tailorshop scenario mainly indirectly. In addition, different types of system-specific knowledge independently contribute to predicting CPS performance. The results of Study 2 indicate that working memory capacity, assessed as an additional predictor, has no incremental validity beyond reasoning. We conclude that (1) cognitive abilities and prior knowledge are substantial predictors of CPS performance, and (2) in contrast to former and recent interpretations, there is insufficient evidence to consider CPS a unique ability construct. In the second part of the paper, we discuss our results in light of recent CPS research, which predominantly utilizes the minimally complex systems (MCS) measurement approach. We suggest ecologically valid microworlds as an indispensable tool for future CPS research and applications.
Introduction
People are frequently confronted with problems in their daily lives that can be characterized as complex in many aspects. A subset of these problems can be described as interactions between a person and a dynamic system of interconnected variables. By manipulating some of these variables, the person can try to move the system from its present state to a goal state or keep certain critical variables within tolerable ranges. Problems of this kind can be simulated using computer models (aka microworlds), offering an opportunity to observe human behavior in realistic problem environments under controlled conditions.
The study of human interaction with complex computer-simulated problem scenarios has become an increasingly popular field of research in numerous areas of psychology over the past four decades. For example, computer models have been built to simulate the job of a small-town mayor ( Dörner et al., 1983 ), a production plant operator ( Bainbridge, 1974 ; Morris and Rouse, 1985 ), a business manager ( Putz-Osterloh, 1981 ; Wolfe and Roberts, 1986 ), a coal-fired power plant operator ( Wallach, 1997 ), and a water distribution system operator ( Gonzalez et al., 2003 ). Real-time simulations have put users in the role of the head of a firefighting crew ( Brehmer, 1986 ; Rigas et al., 2002 ) or an air traffic controller ( Ackerman and Kanfer, 1993 ). In experimental psychology, research on complex problem solving (CPS) has sought to formally describe simulations (e.g., Buchner and Funke, 1993 ; Funke, 1993 ), the effects of system features on task difficulty (e.g., Funke, 1985 ; Gonzalez and Dutt, 2011 ), the role of emotions (e.g., Spering et al., 2005 ; Barth and Funke, 2010 ), and the effects of practice and training programs (e.g., Kluge, 2008b ; Kretzschmar and Süß, 2015 ; Goode and Beckmann, 2016 ; Engelhart et al., 2017 ; see also Funke, 1995 , 1998 ). Differential and cognitive psychology research has investigated the psychometrical features of CPS assessments (e.g., Rigas et al., 2002 ), the utility of computational models for explaining CPS performance (e.g., Dutt and Gonzalez, 2015 ), the relationship between CPS performance and cognitive abilities (e.g., Wittmann and Süß, 1999 ), and its ability to predict real-life success criteria (e.g., Kersting, 2001 ). For detailed summaries of different areas of CPS research, see Frensch and Funke (1995) and Funke (2006) .
Meanwhile, many researchers have moved away from complex real life-oriented systems (CRS) to complex artificial world systems (CAS) in order to increase the psychometric quality of measures and to control for the effects of preexisting knowledge (e.g., Funke, 1992 ; Wagener, 2001 ; Kröner et al., 2005 ). This development ultimately culminated in the minimally complex systems (MCS) approach ( Greiff et al., 2012 ), also known as the multiple complex systems approach (e.g., Greiff et al., 2015a ). This approach has recently become prominent in educational psychology (e.g., Greiff et al., 2013b ; Sonnleitner et al., 2013 ; Kretzschmar et al., 2014 ; OECD, 2014 ; Csapó and Molnár, 2017 ). In addition, this shift has led to the question of what are and are not complex problems, with some researchers questioning the relevance of MCS as a tool for CPS research and the validity of the conclusions drawn from them (e.g., Funke, 2014 ; Dörner and Funke, 2017 ; Funke et al., 2017 ; Kretzschmar, 2017 ).
Originally, simulated dynamic task environments were used to reproduce the cognitive demands associated with real-life problems in the laboratory ( Dörner et al., 1983 ; Dörner, 1986 ). These environments have several features: (1) Complexity: Many aspects of a situation must be taken into account at the same time. (2) Interconnectivity: The different aspects of a situation are not independent of one another and therefore cannot be controlled separately. (3) Intransparency: Only some of the relevant information is made available to the problem solver. (4) Dynamics: Changes in the system occur without intervention from the agent. (5) Polytely: The problem solver must sometimes pursue multiple and even contradictory goals simultaneously. (6) Vagueness: Goals are only vaguely formulated and must be defined more precisely by the problem solver. Whereas older microworlds featured all of these characteristics to a considerable extent, more recent approaches such as MCS have substituted complexity and ecological validity (i.e., the simulation’s validity as a realistic problem-solving environment allowing psychological statements to be made about the real world; see Fahrenberg, 2017 ) for highly reliable assessment instruments by simulating tiny artificial world relationships (e.g., Greiff et al., 2012 ; Sonnleitner et al., 2012 ).
The present paper is divided into two parts. In the first part, we deal with one of the oldest but still an ongoing issue in the area of CPS research: the cognitive prerequisites of CPS performance. In two different studies, we used microworlds (CRS and CAS) to empirically investigate the impact of cognitive abilities (i.e., intelligence and working memory capacity) and prior knowledge on CPS performance. In doing so, we considered the impact of the Brunswik symmetry principle, which effects the empirical correlations between hierarchical constructs (e.g., Wittmann, 1988 ). Integrating our results with previous CPS research, we review the basis and empirical evidence for ‘complex problem solving ability’ as a distinct cognitive construct. In the second part of the paper, we discuss our approach and results in light of recent problem solving research, which predominantly utilizes the MCS approach. Finally, we conclude with some recommendations for future research on CPS and suggest ecologically valid microworlds as tools for research and applications.
Part I: Empirical Investigation of the Cognitive Prerequisites of Complex Problem Solving Performance
Intelligence and complex problem solving.
At the beginning of complex problem solving (CPS) research, CPS pioneers raised sharp criticisms of the validity of psychometric intelligence tests ( Putz-Osterloh, 1981 ; Dörner et al., 1983 ; Dörner and Kreuzig, 1983 ). These measures, derisively referred to as “test intelligence,” are argued to be bad predictors of performance on partially intransparent, ill-defined complex problems. In contrast to simulated scenarios, intelligence test tasks are less complex, static, transparent, and well-defined problems that do not resemble most real-life demands in any relevant way. Zero correlations between intelligence measures and CPS performance were interpreted as evidence of the discriminant validity of CPS assessments, leading to the development of a new ability construct labeled complex problem solving ability or operative intelligence ( Dörner, 1986 ). However, no evidence of the convergent validity of CPS assessments or empirical evidence for their predictive validity with regard to relevant external criteria or even incremental validity beyond psychometric intelligence tests have been presented.
By now, numerous studies have investigated the relationship between control performance on computer-simulated complex systems and intelligence. Whereas Kluwe et al. (1991) found no evidence of a relationship in an older review, more recent studies have found correlations that are substantial but still modest enough to argue in favor of a distinct CPS construct (e.g., Wüstenberg et al., 2012 ; Greiff et al., 2013b ; Sonnleitner et al., 2013 ). In a more recent meta-analysis, Stadler et al. (2015) calculated the overall average effect size between general intelligence (g) and CPS performance to be r = 0.43 (excluding outliers, r = 0.40), with a 95% confidence interval ranging from 0.37 to 0.49. The mean correlation between CPS performance and reasoning was r = 0.47 (95% CI: 0.40 to 0.54). The relationship with g was stronger for MCS ( r = 0.58) than CRSs ( r = 0.34) 1 . From our point of view, this difference results from the higher reliability of MCS but also a difference in cognitive demands. MCS are tiny artificial world simulations in which domain-specific prior knowledge is irrelevant. Complex real life-oriented tasks, however, activate preexisting knowledge about the simulated domain. This knowledge facilitates problem solving; in some cases, the problems are so complex that they cannot be solved at all without prior knowledge (e.g., Hesse, 1982 ).
The main issues with many complex real life-oriented studies that investigated the relation between intelligence and CPS performance concern the ecological validity of the simulations and the psychometric quality of the problem-solving performance criteria. This often leads to much larger confidence intervals in their correlations with intelligence compared to minimal complex tasks ( Stadler et al., 2015 ). When the goals of a simulation are multiple and vaguely defined, the validity of any objective criterion is questionable since it might not correspond to the problem solver’s subjective goal. However, people are unlikely to face a single, well-defined goal in real-life problems, limiting the ecological validity of such systems – despite the fact that a well-defined goal is a necessary precondition for assessing problem solving success in a standardized way, which is necessary in order to compare subjects’ performance. Moreover, single problem solving trials produce only “single act criteria” ( Fishbein and Ajzen, 1974 ), criticized as “one-item-testing” (e.g., Wüstenberg et al., 2012 ), the reliability of which is severely limited. Performance scores must be aggregated via repeated measurements to increase the proportion of reliable variance that can be predicted (e.g., Wittmann and Süß, 1999 ; Rigas et al., 2002 ). The MCS has implemented these steps, resulting in strong reliability estimates (e.g., Greiff et al., 2012 ; Sonnleitner et al., 2012 ).
Another crucial issue with regard to the relation between intelligence and CPS performance is the operationalization of intelligence. Numerous prior studies have used a measure of general intelligence ( g ) to predict problem solving success. Since g is a compound of several more specific abilities, g scores comprise variance in abilities relevant to complex problem solving as well as variance in irrelevant abilities. According to Wittmann’s (1988) multivariate reliability theory and the Brunswik symmetry principle (see also Wittmann and Süß, 1999 ), this results in an asymmetric relationship between predictor and criterion, attenuating their correlation. More specific subconstructs of intelligence might be more symmetrical predictors because they exclude irrelevant variance. In our view, controlling complex systems requires a great deal of reasoning ability (e.g., Süß, 1996 ; Wittmann and Süß, 1999 ; Kröner et al., 2005 ; Sonnleitner et al., 2013 ; Kretzschmar et al., 2016 , 2017 ). Inductive reasoning is required to detect systematic patterns within the ever-changing system states and develop viable hypotheses about the system’s causal structure. Deductive reasoning is necessary to infer expectations about future developments from knowledge of causal connections and deduce more specific goals from higher-order goals. Abilities such as perceptual speed (except in real-time simulations), memory, and verbal fluency, meanwhile, should be less relevant for success in complex problem solving. In this sense, it is an open question in CPS research whether WMC, as a more basic ability construct (e.g., Süß et al., 2002 ; Oberauer et al., 2008 ), is a more symmetrical predictor of CPS performance than reasoning (for an overview of previous findings, see Zech et al., 2017 ).
In summary, a substantial correlation between intelligence and CPS performance measured with real life-oriented microworlds can be expected if (1) sufficient reliability of the CPS measures is ensured (e.g., aggregation via repeated measures), and (2) the best symmetrical intelligence construct is used (e.g., reasoning instead of general intelligence or perceptual speed).
Knowledge and Complex Problem Solving
In addition to the debate about intelligence’s contribution to complex problem solving, many researchers have pointed out the significance of knowledge for the successful control of complex systems (e.g., Bainbridge, 1974 ; Dörner et al., 1983 ; Chi et al., 1988 ; Goode and Beckmann, 2010 ; Beckmann and Goode, 2014 ). Expert knowledge is sometimes claimed to be the only important predictor of real-life problem solving success ( Ceci and Liker, 1986 ), while others point out that both intelligence and knowledge contribute substantially to predicting job performance ( Schmidt, 1992 ), which certainly includes complex problem solving.
Scenarios that accurately simulate real-world relationships provide an opportunity to draw on preexisting knowledge about the part of reality being simulated. That being said, a simulation never is exactly equivalent to what the problem solver has experienced before. Experts in a domain can make use of their knowledge to operate a simulation within that domain, but they are not automatically experts in the simulated scenario. The application of domain knowledge to the simulation requires a considerable amount of transfer. Following Cattell’s investment theory ( Cattell, 1987 ), we assume that intelligence, and particularly reasoning, plays an important role in mediating this transfer. Therefore both, intellectual abilities, particularly reasoning and prior knowledge of the simulated domain, should be powerful predictors of complex problem solving success, although the effect of intelligence has been found to be mainly indirect, mediated through knowledge ( Schmidt et al., 1986 ; Schmidt, 1992 ).
The knowledge relevant for successfully controlling a complex system can be differentiated conceptually on two dimensions. First, knowledge about the system can be distinguished from knowledge about appropriate actions. System knowledge is knowledge about the features and structure of a system, such as what variables it consists of, how these variables are related, and what kind of behaviors the system tends to exhibit. Action-related knowledge is knowledge about what to do in order to pursue a given goal. In contrast to system knowledge, action knowledge is always bound to a specific goal. Studies by Vollmeyer et al. (1996) provided evidence for the distinction between system knowledge and action knowledge: Participants who acquired knowledge about a system during an exploration phase with or without a given goal performed equally well on a subsequent test trial with the same goal. However, the group which had not been given a specific goal during the exploration phase outperformed the group with the specific goal on a test with a new goal. Presumably, the specific goal group had learned mainly action knowledge, whereas the other group had acquired more system knowledge, which was then transferable to new goals.
A second distinction, independent of the first, exists between declarative and procedural knowledge. Declarative knowledge is knowledge that a person can represent symbolically in some way – verbally, graphically or otherwise. Declarative knowledge can be expressed as accurate answers to questions. Procedural knowledge, on the other hand, can be expressed only through accurate performance. The distinction between declarative and procedural knowledge is based on the conceptual difference between “knowing that” and “knowing how” ( Ryle, 1949 ).
While system knowledge and action knowledge differ in content, declarative and procedural knowledge are different forms of knowledge. Therefore, the two dimensions can be conceived of as orthogonal. System knowledge and action knowledge can both be declarative: A person can talk about which variables are causally related to which other variables, but also about what to do in order to keep the system stable. Similarly, both system knowledge and action knowledge can also be procedural: Knowing how to stabilize a system without being able to express it is procedural action knowledge. Being able to mentally simulate a system or diagnose what variable is causing a disturbance without being able to give a full verbal account of the reasons is indicative of procedural system knowledge. Several studies have found that people do not improve their problem-solving performance in controlling or repairing complex systems after receiving instructions in the form of declarative system knowledge (e.g., Morris and Rouse, 1985 ; Kluge, 2008b ; but see Goode and Beckmann, 2010 ), and declarative knowledge sometimes is not correlated with problem solving performance (e.g., Berry and Dienes, 1993 ). Therefore, we must consider the possibility that procedural knowledge is part of the relevant knowledge base that guides a person’s actions within complex dynamic environments.
In summary, prior domain knowledge must be considered as an additional substantial predictor of CPS performance. However, differentiating between different types of knowledge is necessary in order to explain CPS performance. In addition, different semantic embeddings (i.e., CRS vs. CAS) have different demands with regard to preexisting knowledge.
The Present Study
The first goal of the two studies presented in this paper was to test the hypothesized criterion validity of reasoning in predicting problem solving performance in complex dynamic tasks. In addition, considering the Brunswik symmetry principle ( Wittmann, 1988 ), we explored the predictive validity of additional more specific or more general intelligence constructs. Our investigation was based on the Berlin Intelligence Structure Model (BIS), a hierarchical and faceted model of intelligence ( Jäger, 1982 , 1984 ; for a detailed description in English, see Süß and Beauducel, 2015 ). The BIS differentiates intellectual abilities along two facets. The operation facet comprises four abilities: Reasoning (R) includes inductive, deductive and spatial reasoning and is equivalent to fluid intelligence (Gf). Creativity (C) refers to the ability to fluently produce many different ideas. Memory (M) refers to the ability to recall lists and configurations of items a few minutes after having learned them (episodic memory), whereas speed (S) refers to the ability to perform simple tasks quickly and accurately (perceptual speed). The second facet is postulated to include three content-related abilities: verbal (V), numerical (N) and figural-spatial (F) intelligence. Cross-classifying the four operational and three content abilities results in 12 lower-order cells. In addition, general intelligence is conceptualized as an overarching factor (Figure 1 ). For summaries of the validity and scope of the BIS, see the handbook for the BIS Test ( Jäger et al., 1997 ) as well as Süß and Beauducel (2005 , 2015 ).
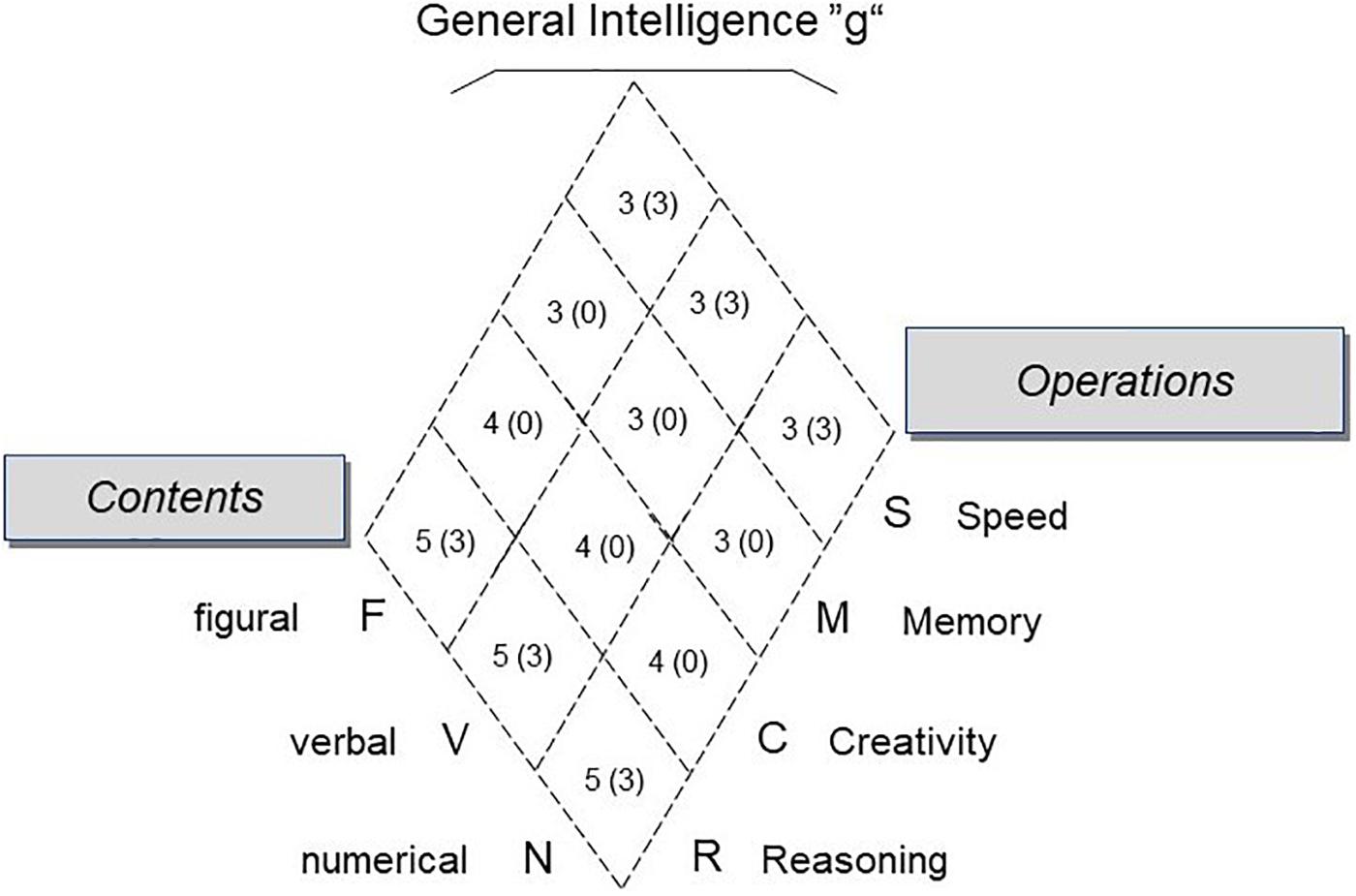
FIGURE 1. The Berlin Intelligence Structure Model (BIS), and the number of tasks for each cell applied in Study 1 (in brackets, Study 2). In the BIS, four operation ability constructs are crossed with three content constructs, yielding twelve cells. On a higher level of aggregation, general intelligence integrates the primary factors for each facet.
In the second study, we included WMC as an additional predictor. Working memory is considered the most important cognitive resource for complex information processing, which includes reasoning (e.g., Kyllonen and Christal, 1990 ; Süß et al., 2002 ; Conway et al., 2003 ), language comprehension (e.g., King and Just, 1991 ), and math performance (e.g., Swanson and Kim, 2007 ). Consequently, previous research has found a significant relation between WMC and CPS (e.g., Wittmann and Süß, 1999 ; Bühner et al., 2008 ; Schweizer et al., 2013 ; Greiff et al., 2016 ). However, whether the more basic construct (i.e., WMC) is a stronger symmetrical predictor of CPS than reasoning from the perspective of the Brunswik symmetry principle ( Wittmann, 1988 ) is not clear (for an overview, see Zech et al., 2017 ). For example, Wittmann and Süß, 1999 demonstrated that WMC has incremental validity in predicting CPS performance beyond intelligence. Bühner et al. (2008) could not confirm this result, but their study relied upon narrow operationalizations.
The second goal of the two studies presented in this paper was to investigate the relation between knowledge and complex problem solving performance. We attempted to measure knowledge about complex systems in several categories. We focused on declarative knowledge in the form of both system knowledge and action knowledge because assessing declarative knowledge is straightforward. We also attempted to measure procedural knowledge, despite the fact that no evidence has ever been put forward that responses to complex problem-solving tests exclusively reflect procedural knowledge and not declarative knowledge. Based on Cattell’s investment theory ( Cattell, 1987 ), we assumed that knowledge represents invested intelligence and examined whether the predictive effect of intelligence on CPS performance is completely mediated by prior knowledge.
We applied a CRS (i.e., a microworld with a realistic semantic embedding) in the first study, whereas we used a CAS (i.e., a microworld with an artificial semantic embedding) in the second study. Hence, the importance of preexisting knowledge with regard to CPS performance should differ between the two studies.
In the first study, we used a complex real life-oriented simulation to examine the criterion validity of intelligence, particularly reasoning, and prior knowledge for control performance in a simulated shirt factory ( Tailorshop ). As we used a very comprehensive assessment of intelligence and knowledge, we were also interested in exploring the predictive validity of additional, more specific constructs in order to investigate the influence of the Brunswik symmetry principle ( Wittmann, 1988 ) on the relation between intelligence, knowledge and CPS performance.
Participants
One hundred and thirty-seven students from 13 high schools in Berlin took part in the experimental study in 1990 ( Süß et al., 1991 ). They had all participated in a similar study 1 year before in which they had taken prior versions of the BIS Test and the knowledge tests and had explored the Tailorshop system ( Süß et al., 1993a , b ). Their mean age was 17.6 years ( SD = 0.67), and 40.9% were female. The participants were fully informed about the study and the voluntary nature of their participation, and anonymity was guaranteed. Written informed consent was obtained from school principals and the state school board. Subjects who withdrew from the study were required to attend other school lessons. Both Berlin studies were published in German only; a full report including the longitudinal results can be found in Süß (1996) . In this paper, we report the results of the second Berlin study (here labeled Study 1) to make the results available for international readers and to discuss the two studies in the light of recent developments in CPS research.
Problem solving
An extended version of the Tailorshop system ( Funke, 1983 ; Danner et al., 2011 ), originally designed by D. Dörner and first used in a published study by Putz-Osterloh (1981) , was applied as a CRS ( Süß and Faulhaber, 1990 ). Additional minor modifications were made in the system to resolve issues with the validity of the problem-solving score that had become apparent in the study conducted 1 year before ( Süß et al., 1993a , b ). Tailorshop is a computer simulation of a shirt factory. The system has 27 variables: 10 are exogenous variables that can be manipulated directly, and 17 are endogenous variables computed by the simulation. Figure 2 provides a screenshot of the system, and Figure 3 an overview of the variables and their interconnections.
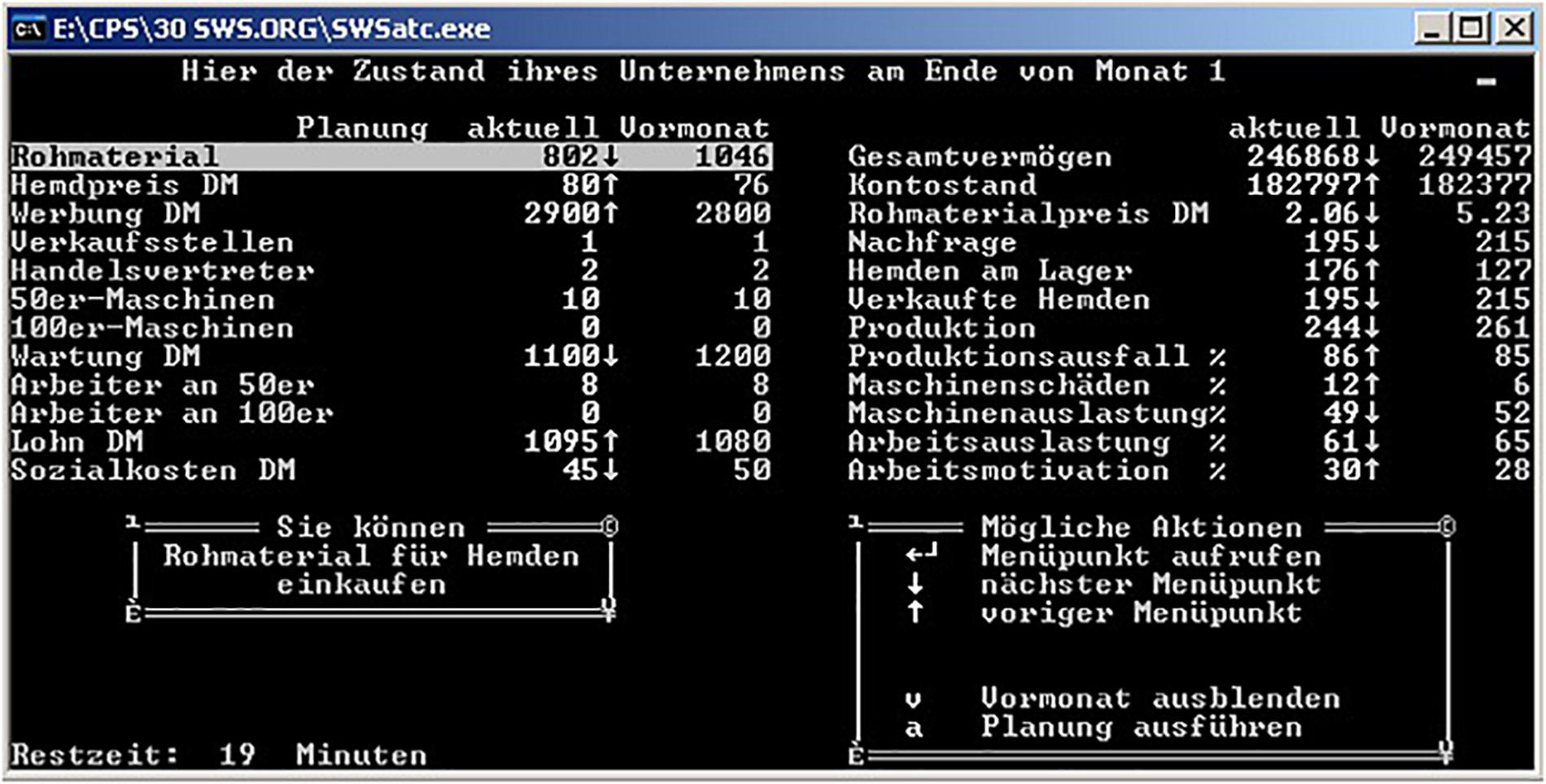
FIGURE 2. Screenshot of the exploration phase of the Tailorshop system as applied in Study 1.
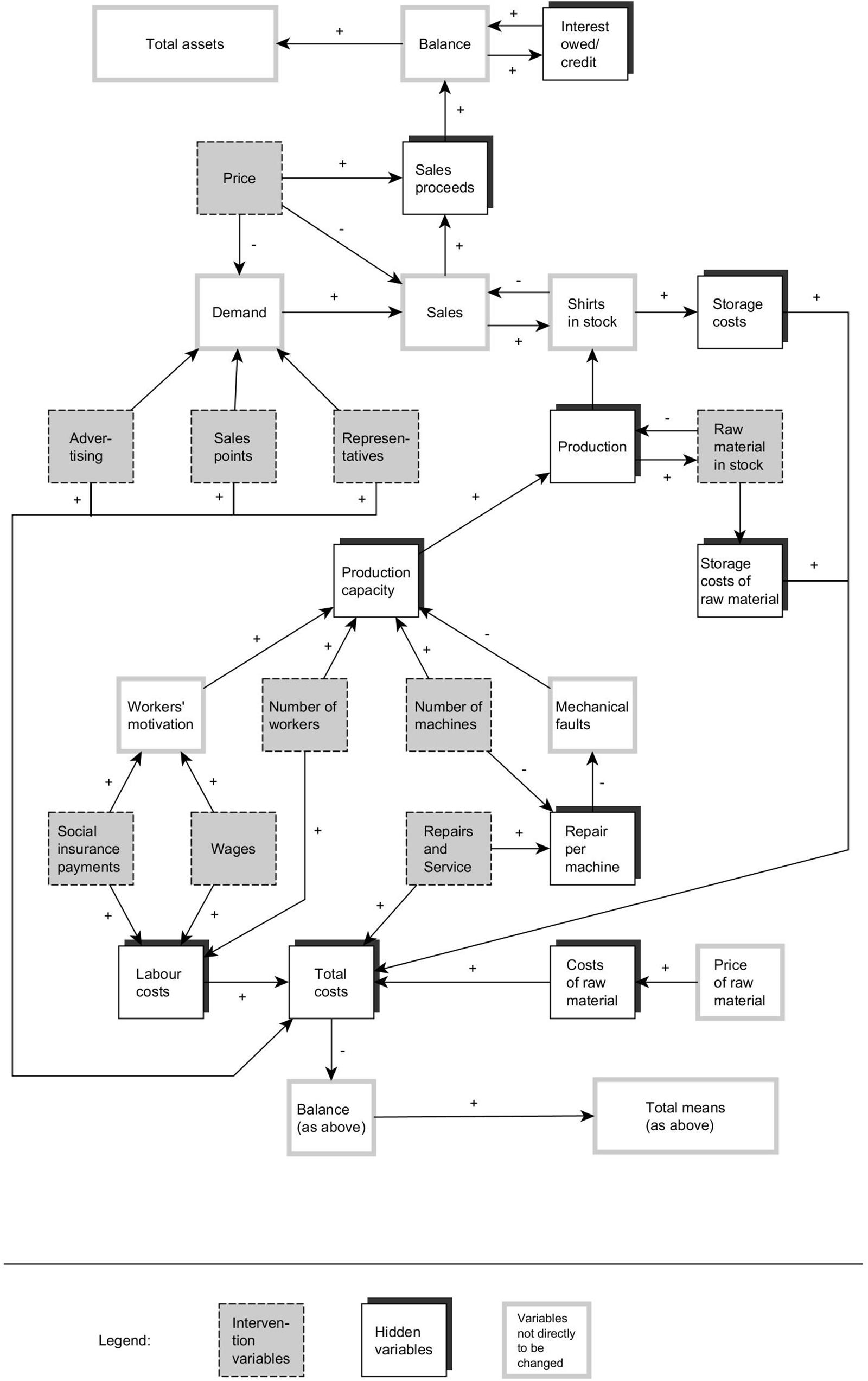
FIGURE 3. The causal structure of the Tailorshop system.
The system was run on a personal computer. All variables were presented in a single menu, and the values of exogenous variables could be selected via a pull-down menu. After planning all decisions, the operator ran the simulation for one virtual month. A complete trial consisted of twelve simulation cycles corresponding to 1 year of management. To obtain two independent indicators of problem solving success, participants worked on two versions of Tailorshop with different starting values corresponding to different shirt factories and different economic conditions. Problem solving performance was measured by participants’ total assets after 12 simulated months. Since the distribution of raw scores deviated considerably from a normal distribution, we transformed them into rank scores and aggregated participants’ ranks from the two simulation runs into one total score.
Intelligence test
To assess intellectual abilities, we used a prior version of the BIS Test ( Jäger et al., 1997 ; for a full English description see Süß and Beauducel, 2015 ; for prior test versions see Süß, 1996 ). This test consists of three to five different tasks for each of the 12 cells in the matrix structure of the BIS. Each task assigned to a cell in the model is used to measure one operation ability as well as one content ability. The four operation abilities are thus measured with scales consisting of 9–15 tasks each and balanced over the three content categories. Analogously, content abilities are measured with scales consisting of 15 tasks across the four different operation abilities. Thus, the same variables are used in different ways for different scales. The scales for one facet are built by aggregating variables that are distributed in a balanced way over the other facet. This suppresses unwanted variance, i.e., the variance associated with factors from the other facet ( Wittmann, 1988 ). However, the scores for operation abilities and content abilities are not statistically independent. An indicator of general intelligence is built by aggregating either the operation scores or content scores.
Knowledge tests
Preexisting general economics knowledge was assessed with an age-normed economics test ( Deutsche Gesellschaft für Personalwesen [DGP], 1986 , with a few questions added from the economics test from Krumm and Seidel, 1970 ) 2 . The questionnaire consisted of 25 multiple-choice items on the meaning of technical terms from the domain of economics.
A new test was developed to assess system-specific knowledge about Tailorshop ( Kersting and Süß, 1995 ). This test had two parts, one for system knowledge and one for action knowledge.
System knowledge refers to knowledge about features of individual variables (e.g., development over time, degree of connectedness with other variables) and about relationships between variables in a system. The system knowledge part of the test was developed in accordance with test construction principles for optimizing content validity ( Klauer, 1984 ; Haynes et al., 1995 ). It consisted of three scales:
(1) Multiple choice questions about the connections between two variables. One out of six statements in the following form had to be selected as correct:
(a) An increase in variable X increases variable Y.
(b) An increase in variable X decreases variable Y.
(c) An increase in variable Y increases variable X.
(d) An increase in variable Y decreases variable X.
(e) Variable X and variable Y interact, that is, they both depend on one another.
(f) (a) through (e) are false.
There were 20 questions of this type.
(2) Questions about hypotheses concerning single variables: Participants had to evaluate statements about the regular behavior of individual system variables, e.g., “The price of shirts rises and falls by chance” (which is false) or “Production depends – among other factors – on my workers’ motivation, which in turn depends on the level of wages” (which is true). The scale consisted of 25 independent items.
(3) Arrow test for connections among multiple variables: Sets of four variables were represented by labeled boxes in a diamond-shaped arrangement. Participants had to draw arrows connecting the variables that had a direct causal connection in the system, and designate the direction of correlation with a plus or minus sign (as in Figure 3 ). Each of the six possible pairings in a set was counted as an independent item that was marked as either correct or incorrect, yielding a total of 42 items.
Action knowledge refers to knowledge about appropriate actions in a certain situation, given a certain goal. It was assessed in this study via two subtests. The test of declarative action knowledge presented “rules of thumb” for successfully managing the Tailorshop simulation, which had to be evaluated as correct or incorrect. Half of the 12 rules were correct, i.e., they were helpful in obtaining high total assets within 12 months, while the other half were incorrect.
In the second subtest, participants were given a system state in the form of a screen display. They were given the goal of maximizing or minimizing a certain system variable, for example, minimizing the number of shirts in the store. They had to select which one out of six alternative decision patterns would be best-suited to reaching this goal in the next simulation cycle. This subtest consisted of six items with different system states, goals, and decision options. In contrast to the declarative questions, this task did not require participants to explicit declare rules for action. Instead, the rules governing their decision-making remained implicit, providing a good opportunity to capture task relevant procedural knowledge. Thus, we will refer to this subscale as procedural action knowledge.
Sum scores were built for each subtest and a total score was calculated by aggregating the subtest scores, weighted equally.
Each type of question was introduced by the experimenter with one or two examples. There was no time limit, but participants were instructed not to spend too much time on any single question.
The students took tests on 2 days for 5–6 h each. On the first day, they worked on the BIS Test and the general economics test as well as some further questionnaires. Testing was done in groups of 20–30 in school classrooms. On the second day, participants were first introduced to the Tailorshop system via detailed instructions, including two standardized practice cycles guided by the experimenter. Afterward, the students in the sample were randomly divided into three groups, and two groups were given additional opportunities to acquire system-specific knowledge. 3 Next, system-specific knowledge was assessed (time T1) by instructing participants to build hypotheses about Tailorshop on basis of their (superficial) experience with the system. Participants then tried to manage the Tailorshop twice for 12 simulated months. Finally, system-specific knowledge was tested again (time T2). The knowledge test took about 80 min the first time and about 60 min the second time. Each problem solving trial lasted about 50 min. The participants took these tests in smaller groups at the university’s computer lab.
We will first present the results of separate analyses of the relationship between problem solving performance and different groups of predictors. Then, we integrate all the variables into a path model. Ten participants had missing data for the economics knowledge test. Thus, we applied the full information maximum likelihood (FIML) procedure to account for the missing data. See Table 1 for descriptive statistics and the full correlation matrix.
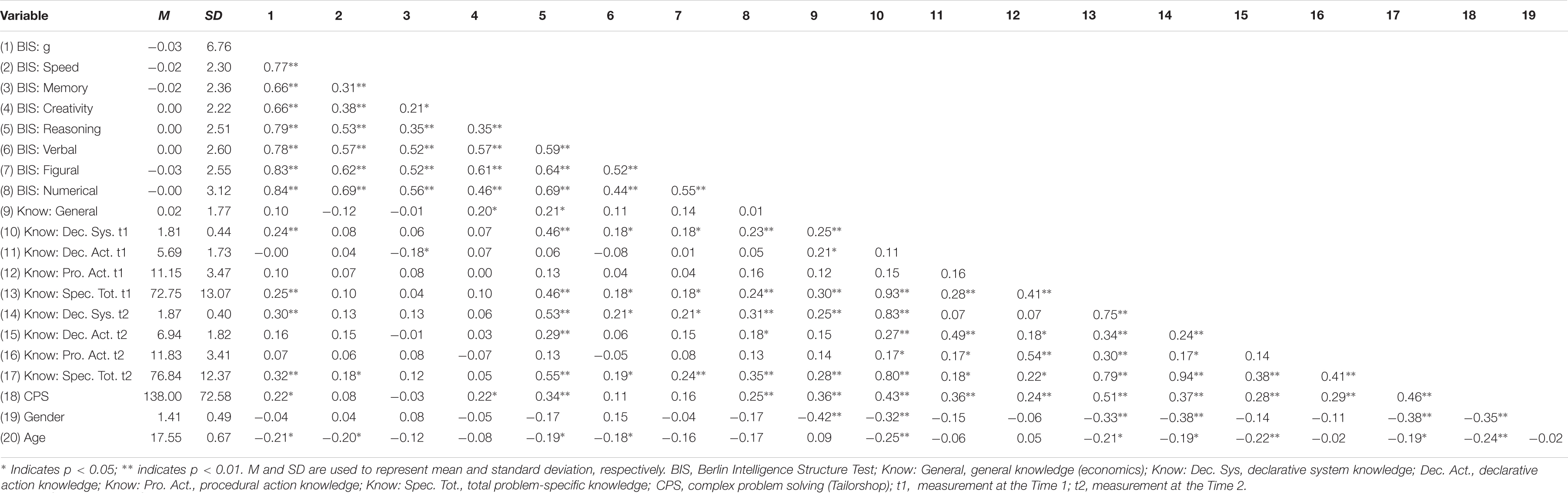
TABLE 1. Study 1: Means, standard deviations, and correlations.
Complex Problem Solving and Intelligence
The parallel-test reliability of problem solving performance was r = 0.67 ( p < 0.01). This indicates that the criterion measures had satisfactory reliability and justifies their aggregation into a single score. Two multivariate regressions were computed with the aggregated performance criterion, first with the four operation scales and then with the three content scales of the BIS as predictors. The results are summarized in Table 2 (upper half, correlations in brackets).

TABLE 2. Multiple regression of problem solving performance on the operation, content, and total scales of the BIS.
Among the operation scales, reasoning ( r = 0.34, p < 0.01) was as expected significantly correlated with problem-solving success, furthermore, creativity ( r = 0.22, p = 0.01) as well. In the regression model, however, only reasoning had a significant beta weight (β = 0.43, p < 0.01). Among the content scales, only numerical intelligence had a significant beta weight (β = 0.22, p = 0.03). The proportion of variance accounted for by the operation scales was much higher than that accounted for by the content scales, despite the fact that the two groups of predictors consisted of the same items that had merely been aggregated in different ways. Building an overall aggregate for all BIS scales (BIS-g) only accounted for five percent of the criterion variance ( r = 0.22, p = 0.01) 4 , compared to 15 percent with the four operation scales. In line with the Brunswik symmetry principle ( Wittmann, 1988 ; Wittmann and Süß, 1999 ), this comparison shows the benefit of differentiating intellectual abilities into multiple components using a multi-faceted model. Taking the cell level of the BIS 5 into account, numerical reasoning was the best and thus likely the most symmetrical predictor of Tailorshop performance ( r = 0.36, p < 0.01). 6 While the correlation between the numerical reasoning cell and the criterion was nearly the same as the correlation for reasoning, numerical reasoning was the better predictor given the substantially lower reliability of the cell score for numerical reasoning (Cronbach’s α = 0.77) compared to reasoning (1-year stability, r = 0.90, p < 0.01). Corrected for unreliability, the true correlation was r = 0.43. In summary, aggregating repeated measures increases the reliability and thus also the validity of the CPS performance score. However, the correlations are lower than for minimally complex tasks even on the most symmetrical level ( r = 0.58), as reported in Stadler et al.’s (2015) meta-analysis.
Complex Problem Solving and Knowledge
Four scales representing prior knowledge (time T1) were used as predictors of problem solving success in the regression analysis. These were the general economics test and the three categories of knowledge represented in the system-specific knowledge test: declarative system knowledge (measured with three subtests), declarative action knowledge (measured with the rules of thumb), and procedural action knowledge (measured using the system-states task). General economics knowledge (β = 0.21, p < 0.01; r zero-order = 0.36, p < 0.01), declarative system knowledge (β = 0.33, p < 0.01; r zero-order = 0.43, p < 0.01), and declarative action knowledge (β = 0.26, p < 0.01; r zero-order = 0.36, p < 0.01) were significantly associated with problem solving performance, whereas procedural action knowledge was not (β = 0.13, p = 0.07; r zero-order = 0.24, p < 0.01). The latter might be in part due to the low reliability of the test, which consisted of only six items. Together, general and system-specific knowledge accounted for 34 percent of the variance in CPS performance.
A significant increase in domain-specific knowledge from pre- to post-test was observed for every subscale. The strongest effect was for declarative action knowledge ( t = 8.16, p < 0.01, d = 0.70), with smaller effects observed for declarative system knowledge ( t = 2.86, p < 0.01, d = 0.25) and procedural action knowledge ( t = 2.33, p < 0.05, d = 0.20). Pre-post correlations were 0.83 ( p < 0.01) for declarative system knowledge, 0.49 ( p < 0.01) for declarative action knowledge, and 0.54 ( p < 0.01) for procedural action knowledge.
An Integrative Path Model
In a second step, we tested our theoretical model via path analysis. Reasoning and general economics knowledge were assumed to be correlated exogenous variables influencing the generation of hypotheses and the acquisition of system-specific knowledge during instruction and exploration, and thus also the amount of system-specific (prior) knowledge measured at time T1. We also assumed direct paths from reasoning, general economics knowledge and system-specific prior knowledge (T1) to control performance, and tested whether reasoning, domain-specific prior knowledge (T1) and problem-solving performance influence system-specific knowledge measured after controlling the system (T2). The resulting model is presented in Figure 4 .
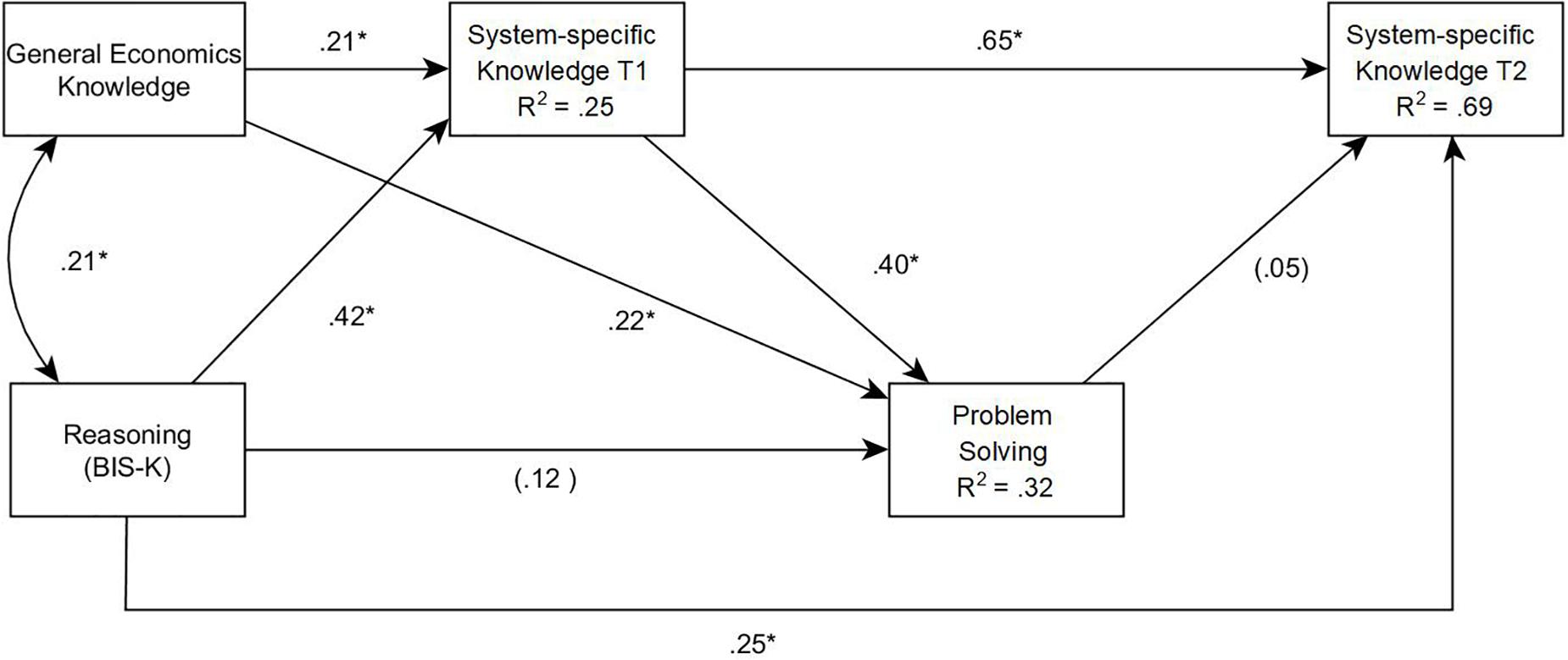
FIGURE 4. Study 1: Path model for problem solving performance in Tailorshop with knowledge and reasoning as predictors. χ 2 (1) = 0.347, p = 0.556, Comparative Fit Index (CFI) = 1.000. Values with ∗ are significant at the 5% level.
The path model reflects and extends the results above. System-specific prior knowledge (T1) was significantly influenced by the two correlated exogenous variables, indicating the importance of general domain knowledge, and especially of reasoning, for generating and testing hypotheses in the Tailorshop simulation. System-specific prior knowledge (T1) was influenced by learning processes during the instructions and, for a part of the sample, during system exploration. A total of 25.4% of the variance was explained by the two exogenous variables. General economics knowledge (β = 0.22, p < 0.01) and system-specific prior knowledge (T1; β = 0.40, p < 0.01) also had direct effects on control performance. Reasoning ability, meanwhile, had no direct effect (β = 0.12, p = 0.12), but a strong indirect effect on problem solving performance as mediated by prior knowledge. The total amount of explained variance in problem solving performance was 32%. Finally, system-specific knowledge after controlling the system (T2) primarily depended on system-specific prior knowledge (T1; β = 0.65, p < 0.01) as well as reasoning (β = 0.25, p < 0.01). Remarkably, while control performance and acquired system knowledge (T2) were substantially correlated ( r = 0.46, p < 0.01), the direct path from control performance to acquired system-specific knowledge (T2) was not significant (β = 0.05, p = 0.35). Overall, 68.6% of the variance was explained.
Both intelligence and prior knowledge were shown to be important predictors of performance controlling a complex system. Some qualifications, however, must be made to this conclusion. First, it is not general intelligence that has predictive power for problem solving success in Tailorshop; instead and as expected, it is the primary factor reasoning, and more specifically numerical reasoning. This underscores the importance of finding the right level of symmetry between predictor and criterion in order to estimate their true relationship ( Wittmann, 1988 ). Second, the correlation between reasoning and problem solving performance was mediated through prior knowledge; reasoning had no direct influence on problem solving performance. This finding is in line with the results of the meta-analysis by Schmidt et al. (1986 ; Schmidt, 1992 ), which showed that the relationship between intelligence and job performance is nearly completely mediated by task-related knowledge. This may indicate that persons with higher reasoning ability have used their ability to accumulate more domain knowledge in the past. The strong relationship between reasoning and general economics knowledge supports this account. An alternative explanation is that high reasoning ability helps people transfer their general domain knowledge to the specific situation, i.e., by deriving good hypotheses about the unknown system from their general theoretical knowledge about the corresponding domain. System-specific knowledge measured after controlling the system (T2) depends primarily on prior knowledge and reasoning. Therefore, controlling a complex system can be described as a knowledge acquisition process, providing evidence for Cattel’s investment theory ( Cattell, 1987 ). Assuming that the system has ecologically validity, this finding also indicates that system-specific knowledge measured after controlling a complex system is a powerful predictor of external criteria.
The study was limited to the computer-simulated system Tailorshop , a microworld mainly developed by psychologists. The scenario is realistic in that it captures many psychologically relevant features of complex real-life problems, but its ecological validity as a model for a real business environment is limited. For example, real company executives spend more than 80% of their time communicating orally (e.g., Mintzberg, 1973 ; Kotter, 1982 ), a demand which was not implemented in the simulation (see Süß, 1996 ).
A final but important qualification to the study’s results concerns reasoning in the context of knowledge. System-specific knowledge was consistently the best single predictor of problem solving success in Tailorshop , while general domain knowledge in economics significantly predicted additional variance. System-specific knowledge was made up of two independent predictors, declarative system knowledge and declarative action knowledge. Our study found no evidence of the dissociation between verbalized knowledge and control performance repeatedly reported by Broadbent and colleagues ( Broadbent et al., 1986 ; Berry and Broadbent, 1988 ; see Berry and Dienes, 1993 ). Tailorshop is a more complex and realistic system than those used by Broadbent and colleagues. Both factors might have strongly motivated people to make use of their preexisting knowledge, i.e., to formulate explicit hypotheses for controlling the system rather than following a trial-and-error approach that would result in the acquisition of implicit knowledge.
The aim of the second study was to replicate and extend the findings presented so far. Study 2 differed from Study 1 in two important ways. First, we used the artificial world simulation FSYS ( Wagener, 2001 ), which simulated a forestry company. Although FSYS has a rich semantic embedding and all the characteristics of complex problems, FSYS was developed with the aim of reducing the impact of previous knowledge of the simulated domain (i.e., general forestry knowledge) on problem solving performance. Therefore, FSYS can be classified as a CAS. Second, we included WMC as a further predictor. WMC is a more basic construct than reasoning and whether it is a better (i.e., more symmetrical) predictor of CPS performance than reasoning is an open question (see Zech et al., 2017 ). Thus, we were interested in whether one of the two constructs had incremental validity in predicting CPS performance beyond the other construct.
One hundred fifty-nine students from the University of Magdeburg participated in the second study, which was originally conducted to evaluate a complex problem solving training (for details, see Kretzschmar and Süß, 2015 ), in 2010/2011. 7 In the present analyses, we used the full sample but excluded all non-native German speakers ( n = 7) due to the high language requirements of the intelligence test. The mean age was 23.99 years ( SD = 4.43), and 50% were female. Participants received course credit for their participation or took part in a book raffle. Participants were informed about the content of the study, the voluntary nature of participation and their ability to withdraw at any point, and that anonymity was guaranteed. All subjects provided informed consent.
We used version 2.0 of the microworld FSYS ( Wagener, 2001 ). FSYS was developed on the basis of Dörner et al.’s (1983) theoretical framework for complex problem solving ( Dörner, 1986 ). It is a microworld with 85 variables connected via linear, exponential, or logistic relations. The goal was to manage five independent forests in order to increase the company’s value (i.e., planting and felling trees, fertilizing, pest control, etc.). Participants were first given an introduction to the program and had an opportunity to explore the system. They then managed the forest company for 50 simulated months. We used the company’s total capital (i.e., an aggregated score of the five independent forests) at the end of the simulation as the performance indicator (SKAPKOR; see Wagener, 2001 ). Although FSYS simulates a forestry enterprise, the impact of prior knowledge was reduced by using abstract names for tree species, pests, fertilizer etc., and providing essential information about the artificial foresting world via an integrated information system. Previous studies have shown that FSYS has incremental predictive validity beyond general intelligence with regard to occupational ( Wagener and Wittmann, 2002 ) and educational ( Stadler et al., 2016 ) performance indicators. Figure 5 provides a screenshot of FSYS .
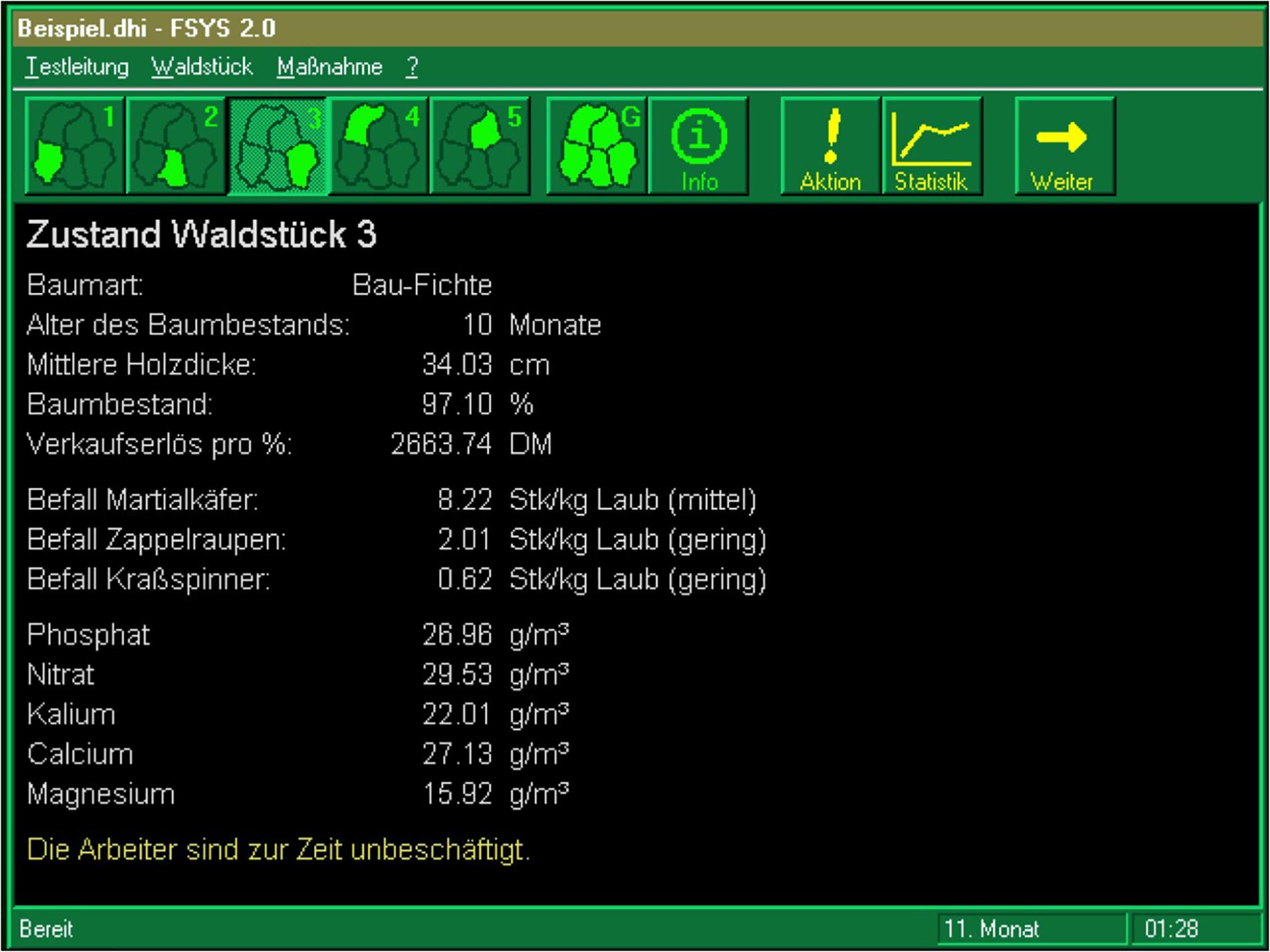
FIGURE 5. Screenshot of the exploration phase of FSYS system as applied in Study 2.
Intelligence
A short version of the BIS Test was used to assess intellectual abilities ( Jäger et al., 1997 ). We specifically focused on reasoning and perceptual speed. Nine tasks were applied for each operation, balanced over the three content areas (i.e., figural, verbal, numerical; see Figure 1 ). These 18 tasks were administered according to the test manual. As in Study 1, the tasks were aggregated in order to build scales for each operation (i.e., reasoning, perceptual speed) or content (i.e., figural intelligence, verbal intelligence, numerical intelligence). An indicator for general intelligence was built by aggregating the 18 tasks in a balanced way, as described in the test handbook. Please note that the reliability of the two operative scales was lower than in Study 1; the construct validity of the three content scales and the measure of general intelligence were also reduced because no memory or creativity tasks were used. This limits the interpretability of the BIS content scales and the comparability of the results of the two studies.
Working memory
Working memory capacity was assessed with three tasks from the computerized test battery by Oberauer et al. (2003) . The numerical memory updating (adaptive) and reading span (non-adaptive) tasks measured the simultaneous storage and processing functions of working memory, whereas the dot span task (also named spatial coordination ; adaptive) primarily measured the coordination function. Moreover, each content category (i.e., figural, verbal, numerical) was represented by one task. A global score for WMC was calculated by aggregating the three equally weighted total task scores.
A questionnaire to assess general forestry knowledge as a measure of preexisting domain knowledge was developed for the purpose of this study 8 . It covered forestry knowledge in the subdomains of tree species, soils, nutrients, damage to a forest, and silviculture. An example question was: “Which tree is not a conifer?” The 22 multiple-choice items were scored dichotomously. Four items were excluded due to poor psychometric properties (i.e., a low item-total correlation). The remaining 18 items were aggregated to form a global sum score.
To assess system-specific knowledge about FSYS , we used Wagener’s (2001) knowledge test about the microworld. The 11 multiple-choice items addressed system and action knowledge across all relevant areas of FSYS . For example: “A forest is infested by vermin XY. Which procedure would you apply?” In order to limit the number of questions, we did not differentiate between different types of knowledge. Therefore, we used a sum score as a global indicator of system-specific knowledge.
Participants took part in two sessions each lasting about 2.5 h. All testing was done in groups of up to 20 persons at the university computer lab. The first session comprised tests of intelligence and WMC. In the second session, participants completed tests of general forestry knowledge, complex problem solving, and system-specific knowledge. In contrast to Study 1, system-specific knowledge was assessed only once, after participants had worked with the CPS scenario (similar to Wagener, 2001 ). As the study was originally designed as an experimental training study (see Kretzschmar and Süß, 2015 ), the procedure differed slightly between the two experimental groups. About half of the participants completed the second session the day after the first session. The other half participated in a CPS training in between and completed the second session about 1 week after the first session.
We will first present results for individual groups of predictors of CPS performance before integrating the results into a combined path model. Due to the original study design (i.e., exclusion criteria for the training, dropout from the first session to the second), up to 24% of the data for the knowledge tests and the CPS scenario were missing. We used the full information maximum likelihood (FIML) procedure to account for missing data. The smallest sample size in the analyses of individual groups of predictors was 116. The data are publicly available via the Open Science Framework 9 . See Table 3 for descriptive statistics and the full correlation matrix.
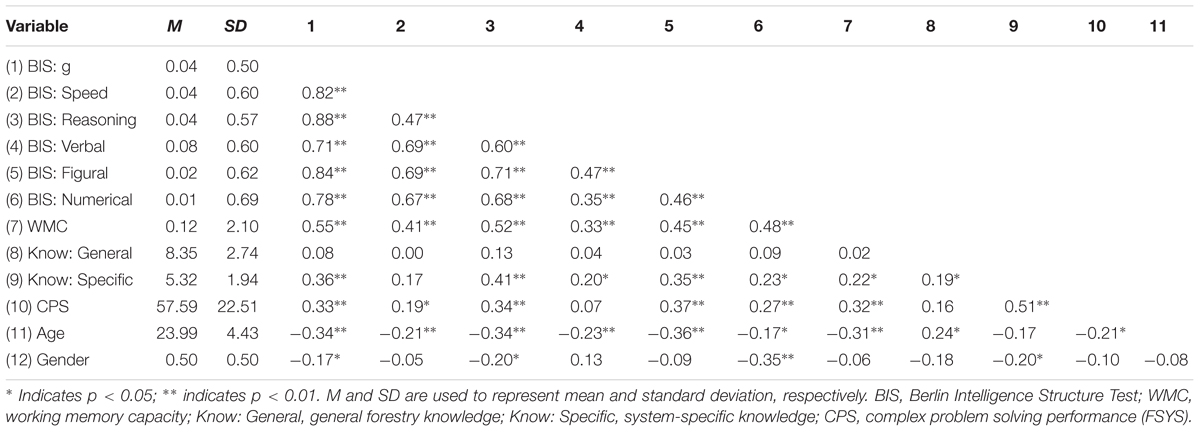
TABLE 3. Study 2: Means, standard deviations, and correlations.
Complex Problem Solving, Intelligence, and Working Memory
The results of two multivariate regressions of FSYS performance scores on the BIS operative and content scales, respectively, are summarized in Table 2 (lower half, correlations in brackets). The results for operation abilities are similar to those from the first study, with reasoning the only significant predictor (β = 0.33, p < 0.01). However, figural intelligence was the only statistically significant predictor among the content scales (β = 0.38, p < 0.01). This seems plausible given that FSYS displays important information graphically rather than numerically (e.g., diagrams showing the forestry company’s development). However, a large amount of information is also presented numerically, meaning that numerical reasoning should exert an influence as well. Taking the cell level of the BIS into consideration: Numerical reasoning (Cronbach’s α = 0.66) became similarly strongly associated with FSYS control performance ( r = 0.37, p < 0.01; corrected for unreliability r = 0.46) as figural reasoning (Cronbach’s α = 0.72; r = 0.36, p < 0.01; corrected for unreliability r = 0.42). Verbal reasoning (Cronbach’s α = 0.51) remained unassociated with FSYS performance ( r = 0.02, p = 0.82). In contrast to Study 1, the content scales accounted for a slightly larger share of the variance in FSYS (16%) than the operation scales (10%). General intelligence (BIS-g) had a.33 ( p < 0.01) correlation with problem solving performance.
Next, we compared the impact of reasoning and WMC as predictors of success in FSYS . Both predictors exhibited an almost equal and statistically significant zero-order correlation ( r BIS-R. FSYS = 0.34, p < 0.01; r WMC. FSYS = 0.32, p < 0.01). In hierarchical regressions, each explained a similar but non-significant amount of incremental variance over and above the other predictor (Δ R 2 BIS.K = 0.02; Δ R 2 WMC = 0.02). The total explained variance was 12.2% (adjusted). In summary, working memory did not increase the statistical significance of the multiple correlation when entered as a second predictor.
General forestry knowledge was not significantly correlated with FSYS performance ( r = 0.16, p = 0.09). Thus, the (non-)impact of prior domain knowledge in FSYS was similar as in previous studies ( r = 0.13; Wagener, 2001 ), emphasizing how the impact of prior knowledge depends on the specific type of microworld (i.e., CRS in Study 1 vs. CAS in Study 2). The correlation between system-specific knowledge (measured after working on FSYS ) and FSYS performance was r = 0.51 ( p < 0.01).
In line with our assumptions about the relations among the predictor and criterion variables and building upon the results of the first study, we constructed a path model to integrate our findings. Perceptual speed from the BIS Test was excluded from the analyses because it was not significantly associated with any endogenous variable when controlling for reasoning. Prior general forestry knowledge was also omitted from the path model for the same reason.
In the first model (Figure 6 , Model A), working memory had a direct influence on reasoning but not on FSYS control performance and system-specific knowledge. In this model [χ 2 (2) = 4.538, p = 0.10, CFI = 0.977, SRMR = 0.038], control performance (β = 0.34, p < 0.01) and acquired system-specific knowledge about the microworld FSYS (β = 0.26, p < 0.01) were significantly influenced by reasoning. The total amount of explained variance for control performance and system-specific knowledge were 11% and 32%, respectively.
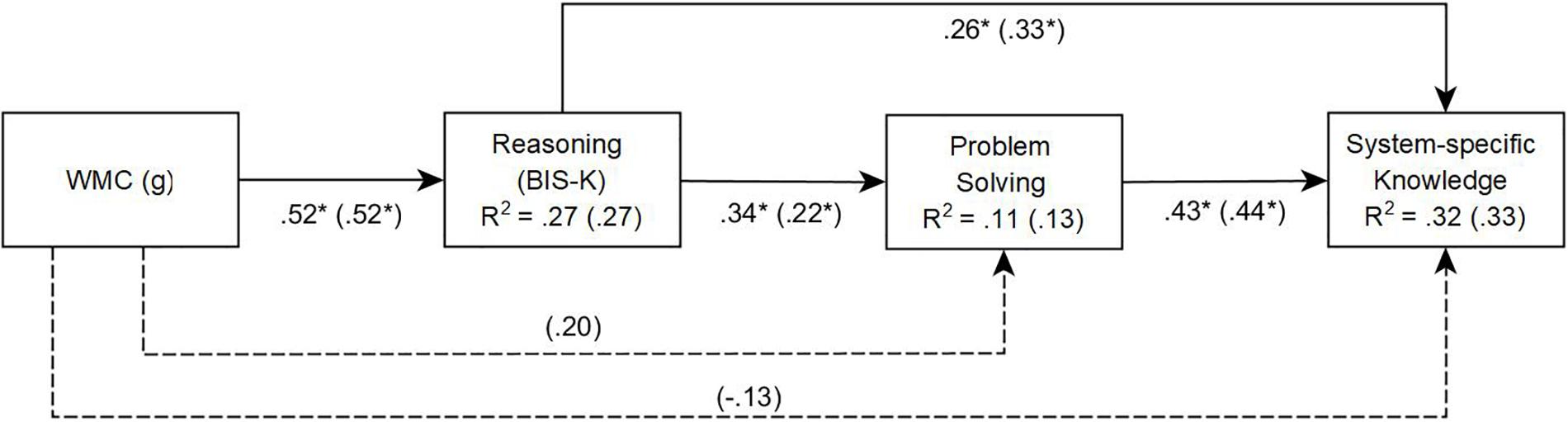
FIGURE 6. Study 2: Path Model A for problem solving performance and system-specific knowledge in FSYS , predicted by reasoning and working memory capacity (WMC). Fit for model A (without dashed lines): χ 2 (2) = 4.538, p = 0.10, Comparative Fit Index (CFI) = 0.977. Path model B (saturated) with dashed lines and values in brackets. Values with ∗ are significant at the 5% level.
In a second (fully saturated) model (Figure 6 , Model B: dashed lines and coefficients in brackets), direct paths from working memory to FSYS control performance and system-specific knowledge were added. In this model, working memory had a small but non-significant direct effect on control performance (β = 0.20, p = 0.09), i.e., the effect of working memory is primarily based on its shared variance with reasoning. Furthermore, WMC functioned as a suppressor when it came to predicting system-specific knowledge. In other words, despite the positive zero order correlation between the two variables (see above), the direct path from WMC to system-specific knowledge was negative (β = -0.13, p = 0.19), while the impact of reasoning on system-specific knowledge slightly increased (β = 0.33, p < 0.01). On the other hand, the path from working memory to system-specific knowledge was statistically non-significant, and the explained variance in system-specific knowledge did not significantly increase [Δ R 2 = 0.012, F (1,148) = 2.663, p = 0.46].
The general findings of Study 1 with regard to the impact of intelligence on CPS performance could be replicated in Study 2. However, as Study 2 was conducted with a different microworld with different cognitive demands (e.g., less relevance of prior knowledge), the results differed somewhat compared to those of Study 1.
With regard to intelligence, reasoning was again the strongest and sole predictor of CPS performance. Because general intelligence (g) was operationalized substantially more narrowly than in Study 1, the results for reasoning and g were comparable. These findings highlight the effect of the specific operationalization of intelligence selected. If intelligence is broadly operationalized, as proposed in the BIS (see Study 1), the general intelligence factor is not equivalent to reasoning (aka fluid intelligence; see also Carroll, 1993 ; McGrew, 2005 ; Horn, 2008 ) and different results for g and for reasoning in predicting CPS performance can be expected (see e.g., Süß, 1996 ). With regard to the content facet, FSYS shared the most variance with figural intelligence. However, the cell level of the BIS provided a more fine-grained picture: figural reasoning was just as highly correlated with FSYS performance as numerical reasoning. Although Study 1 and Study 2 must be compared with caution (i.e., due to different operationalizations of the BIS scales, see Figure 1 , and limited BIS reliability on the cell level), it is clear that different CPS tests demand different cognitive abilities. At the same time, these findings highlight the importance of the Brunswik symmetry principle ( Wittmann, 1988 ; Wittmann and Süß, 1999 ). A mismatch between predictor and criterion (e.g., figural reasoning and Tailorshop performance in Study 1; or numerical intelligence and FSYS performance in Study 2) substantially reduces the observed correlation (for another empirical demonstration in the context of CPS, see Kretzschmar et al., 2017 ). Ensuring that the operationalizations of the constructs are correctly matched provides an unbiased picture of the association across studies ( Zech et al., 2017 ).
Working memory capacity was strongly related to reasoning and largely accounted for the same portion of variance in problem solving success as reasoning; it did not explain substantial variance over and above reasoning. These results complement the mixed pattern of previous findings, in which working memory explained CPS variance above and beyond intelligence ( Wittmann and Süß, 1999 ), was the only predictor of CPS variance when simultaneously considering figural reasoning ( Bühner et al., 2008 ), but did not explain CPS variance above and beyond reasoning ( Greiff et al., 2016 ). In our view, there is little unique criterion variance to explain because the predictors are highly correlated. Even small differences in operationalization or random fluctuations can make one or the other predictor dominate (for a different view, see Zech et al., 2017 ).
Preexisting knowledge (i.e., general forestry knowledge) did not contribute to problem solving success. This finding highlights the importance of the CPS measurement approach selected. Whereas Tailorshop was developed as a complex real life-oriented simulation in which prior domain knowledge plays a substantial role, FSYS was developed with the aim of reducing the influence of prior knowledge ( Wagener, 2001 ). Therefore, in addition to the distinction between microworlds and MCS, the differential impact of prior knowledge in terms of semantic embedding has to be considered when examining the validity of CPS (e.g., the effects might differ for CRS vs. CAS, as in the present study). It should be noted that in Stadler et al.’s (2015) meta-analysis, a study featuring FSYS (in which prior knowledge has no impact) and a study involving a virtual chemistry laboratory (in which prior knowledge has an effect; see Scherer and Tiemann, 2014 ) were both classified as single complex system studies. As a substantial portion of the variance in CPS performance in semantically embedded microworlds can be attributed to prior knowledge, the question arises as to whether a more fine-grained classification of the CPS measures in Stadler et al.’s (2015) meta-analysis would have resulted in different findings. In summary, the heterogeneity of different CPS measurements makes it difficult to compare studies or conduct meta-analyses (some would say impossible, see Kluwe et al., 1991 ).
General Discussion
The presented studies had two main goals. First, we wanted to investigate the predictive validity of differentiated cognitive constructs for control performance in complex systems. Second, we were interested in how preexisting general knowledge and system-specific prior knowledge contribute to successful system control.
Both studies clearly demonstrate that intelligence plays an important role in control performance in complex systems. This is in contrast to former claims in early CPS research that problem solving success in complex, dynamic, partially intransparent systems is not at all correlated with intelligence test scores (e.g., Kluwe et al., 1991 ). Our results point to several explanations for prior failures to find positive correlations. First, previous studies used only a single problem solving trial, meaning that the performance criterion presumably was not satisfactorily reliable. Second, several previous studies did not differentiate between different aspects of intelligence, but used a measure of general intelligence. In our studies, however, general intelligence (g) as conceptualized in the BIS and operationalized with the BIS Test was not a good predictor of control performance. Instead and as was expected, the second-order construct of reasoning, and more specifically numerical reasoning, had the strongest relationship with success in the complex real-world oriented system ( Tailorshop ), while figural and numerical reasoning had the strongest relationship with success in the complex artificial world problem ( FSYS ). However, whether g and reasoning are distinguishable from each other ( Carroll, 1993 ), and thus also whether the two differ in predicting CPS performance, depends on the level of generality, i.e., the broadness of the operationalization of g.
Our results are in line with the first Berlin study ( Süß et al., 1993a , b ) and several other studies using the Tailorshop system and other CRSs focusing on ecological validity (e.g., Wittmann and Süß, 1999 ; Kersting, 2001 ; Leutner, 2002 ; Rigas et al., 2002 ; Ryan, 2006 ; Danner et al., 2011 ), and were confirmed in Stadler et al.’s (2015) meta-analysis.
Is There Evidence for a New Construct ‘Complex Problem Solving Ability’?
The two presented studies, however, are limited to one microworld each, and do not answer broader questions regarding generalizability. In particular, the convergent validity of microworlds was not addressed, but this question is essential for postulating complex problem solving ability as a new ability construct.
The following criteria must be considered in justifying a new ability construct (cf., Süß, 1996 , 1999 ): (1) temporal stability, (2) a high degree of generality (i.e., the construct can be operationalized across different tasks, showing convergent validity), (3) partial autonomy in the nomological network of established constructs (i.e., the shared performance variance in different tasks cannot be explained by well-established constructs), and (4) evidence for incremental criterion validity compared to established constructs. In this section, we briefly review the empirical results regarding the existence of a unique CPS construct. We focus on CPS research utilizing CRS (i.e., microworlds with semantic embeddings) 10 .
The 1-year stability of CRS performance in the Berlin study (see Süß, 1996 ) was r = 0.49, which is substantial, but much lower than that for the intelligence constructs. The temporal stability of the BIS scales ranged from 0.65 for creativity to 0.90 for reasoning. In addition, the time-stable performance variance was explained completely by intelligence and prior knowledge ( Süß, 1996 ). To the best of our knowledge, no results on temporal stability for other CRS and temporal stability for aggregated scores based on different CRS are currently available.
Wittmann et al., (1996 ; Wittmann and Süß, 1999 ; Wittmann and Hattrup, 2004 ) investigated the convergent validity of CRS. Wittmann et al. (1996) applied three different CRS ( PowerPlant , Tailorshop , and Learn !), the BIS Test and domain-specific knowledge tests for each system to a sample of university students. The correlations of the CRS were significant but rather small (0.22–0.38), indicating low convergent validity 11 . However, because the reliability of each CRS was substantially higher than their intercorrelations, substantial system-specific variance has to be assumed. Performance on each of the three systems was predicted by reasoning and domain-specific prior knowledge to a substantial degree. In a structural equation model with a nested-factor BIS model ( Schmid and Leiman, 1957 ; Gustafsson and Balke, 1993 ) as predictor, the CPS g-factor with two performance indicators for each of the three systems (i.e., the CPS ability construct) was predicted by general intelligence (β = 0.54), creativity (0.25) and reasoning (0.76), whereas perceptual speed and memory did not contribute to prediction ( Süß, 2001 ) 12 . In this model, reasoning, though orthogonal to general intelligence, was the strongest predictor of the complex problem solving ability factor. Almost all of the variance could be explained by the BIS, putting the autonomy of the CPS construct into question.
In sum, there is no evidence for a new ability construct based on CRSs. This, however, does not mean that this kind of research cannot provide important new insights into CPS processes (see Süß, 1999 ), and that CPS performance cannot predict real-life performance beyond psychometric intelligence measures to a certain extent (e.g., Kersting, 2001 ; Danner et al., 2011 ).
Kersting (2001) predicted police officers’ job performance over 20 months on the basis of intelligence (short scales for reasoning and general intelligence from the BIS Test), CPS performance (two simulations, including Tailorshop ), and acquired system-specific knowledge (measured after controlling the system). In a commonality analysis ( Kerlinger and Pedhazur, 1973 ), 24.9% of job performance variance was explained. The strongest specific predictor was intelligence (7.3%; reasoning and general intelligence at about the same level); CPS performance and system-specific knowledge explained 3.9 and 3.0% of the overall criterion, respectively. The largest share of the variance was confounded variance between intelligence and system-specific knowledge (24.9%). In comparison to our first study, both intelligence scales had reduced predictive validity due to lower reliabilities. However, this study shows that exploring and controlling CRS must be considered a learning process. Acquired system knowledge represents invested intelligence (i.e., crystallized intelligence) and was a small but additional predictor of real-life performance beyond intelligence. This provides that ecological-valid complex systems can additionally predict external criteria, and are useful learning and training tools for acquiring domain-specific knowledge.
Part II: Review and Critique of the Minimally Complex System (MCS) Approach
The research presented and discussed in the first part of the paper focuses on CRSs. From the beginning, CRS research was criticized for numerous reasons, including the lack of a formal description of the system, the lack of an optimal solution as an evaluation criterion for subjects’ behavior and performance, the uncontrolled influence of prior knowledge, low or unknown reliability of the scores, and low or even non-existent convergent validity and predictive validity with respect to relevant external criteria (for summaries, see e.g., Funke, 1995 ; Süß, 1996 ; Kluge, 2008a ). Therefore, the MCS approach ( Greiff et al., 2012 ) was developed to overcome the limitations of former microworlds. The MCS approach is remarkably prominent in recent CPS research, which may be a consequence of the higher reliability and validity such systems are assumed to have in comparison to CRS (e.g., Greiff et al., 2015b ). Consequently, some might argue that research on CPS performance based on CRS, as presented in the first part of the paper, is less reliable and informative. However, whether the MCS approach is really a superior alternative to studying problem solving in complex situations remains up for debate.
The MCS approach updates and further develops ideas that have been present since the beginning of CPS research. Funke (1993) suggested artificial dynamic systems as a research tool based on systems of linear equations. Buchner and Funke (1993) proposed the theory of finite state automata as a tool for developing CPS tasks. Applying this, Kröner (2001 ; Kröner et al., 2005 ), for example, implemented MultiFlux , which simulates a fictitious machine, within the finite-state framework. This idea was further developed into MCS, e.g., Genetics lab ( Sonnleitner et al., 2012 ) and MicroDYN ( Greiff et al., 2012 ). Generally, about 9–12 artificial world tasks, tiny systems with up to three exogeneous and three endogenous variables each, are applied in three phases: (1) free system exploration, (2) knowledge acquisition (i.e., assessment of acquired system knowledge), and (3) knowledge application (i.e., assessment of action knowledge). The required testing time is less than 5 min for each minimal system. Each system provides three scores, one for each of the above-mentioned phases, which are then used to form three corresponding knowledge scales. According to our knowledge taxonomy, Phase 2 measures declarative system knowledge (i.e., relations between variables), while Phase 3 measures procedural action knowledge (i.e., system interventions in order to achieve a given goal). The items in these two subtests are similar to the items in the arrows task and the system-states task of the Tailorshop knowledge test. Whereas each item in the MCS scales refers to a different minimal system, all items in the Tailorshop knowledge test refer to the same system. Nevertheless, the MCS tasks are very similar to each other and implement only a small number of CPS characteristics, giving the subtests high internal consistencies. Specifically, all minimal systems can be fully explored with the simple strategy “vary one thing at a time” (VOTAT; e.g., Vollmeyer et al., 1996 ) or the closely related strategy “vary one or none at a time” ( Beckmann and Goode, 2014 ; for additional distinctions see Lotz et al., 2017 ). No special training is necessary to learn these strategies. Instead, they can be learned by instruction or examples of correct and incorrect applications. On the other hand, these strategies are clearly not sufficient for exploring CRS, i.e., systems with many exogeneous variables, indirect and side effects, delayed effects, and eigendynamics, especially if the time for the task is limited or in real-time simulations (e.g., Bremer’s fire-fighter; Rigas et al., 2002 ). For the latter, the quality of one’s hypotheses, which is based on domain knowledge, is a necessary prerequisite for successfully exploring the system. In summary, the features of MCS measurements outlined here, along with further criticisms of this approach (e.g., Funke, 2014 ; Scherer, 2015 ; Schoppek and Fischer, 2015 ; Dörner and Funke, 2017 ; Funke et al., 2017 ; Kretzschmar, 2017 ), substantially narrow the validity of the MCS approach as an indicator of CPS.
On the other hand, the relevance of the MCS approach is shown by many studies that have modeled the internal structure of MCS tasks (e.g., Greiff et al., 2012 ; Sonnleitner et al., 2012 ), provided evidence that performance variance cannot be sufficiently explained by reasoning (e.g., Wüstenberg et al., 2012 ; Sonnleitner et al., 2013 ; Kretzschmar et al., 2016 ), found strong convergent validity as well as a lower correlation with a CRS (i.e., Tailorshop ; Greiff et al., 2015b ; for a different view, see Kretzschmar, 2017 ), and demonstrated incremental validity in predicting school grades beyond reasoning (e.g., Greiff et al., 2013b ; Sonnleitner et al., 2013 ; for different results, see Kretzschmar et al., 2016 ; Lotz et al., 2016 ) and beyond a CRS task ( Greiff et al., 2015b ). MCS have been proposed as a tool for assessing 21st Century skills ( Greiff et al., 2014 ) and were applied in the international large-scale study PISA to assess general problem-solving skills ( OECD, 2014 ). They have further been proposed as training tools and evaluation instruments for these skills (e.g., Greiff et al., 2013a ; Herde et al., 2016 ). This begs the question: how strong is the empirical evidence? Are these far-reaching conclusions and recommendations justified?
Studies provide support for the psychometric quality, especially the reliability, of the MCS approach, although scale building and some statistics have been criticized ( Funke et al., 2017 ; Kretzschmar, 2017 ). Only one study so far has attempted to compare MCS and CRS. In it, Greiff et al. (2015b) argued that MCS had a higher validity than Tailorshop in predicting school grades. The knowledge scales assessed after exploring the system were used as predictors for the MCS. However, system-specific knowledge for Tailorshop after controlling the system was not assessed ( Kretzschmar, 2017 ). Instead, control performance was used as a predictor of school grades. Control performance, however, is not a valid measure of acquired knowledge, as demonstrated in our first study. For this, additional tests are needed after controlling the system, conducted in both studies in this paper.
Minimally complex systems research also only sparingly addresses questions of construct validity related to the measures and the conclusions (i.e., generalizability; see Kretzschmar, 2015 ). This concerns the operationalization of CPS characteristics (i.e., the construct validity of the MCS), which was addressed in more detail above. However, limitations also exist concerning the choice of the additional instruments applied in validation studies. The construct validity of many instruments is considerably limited, causing results to be overgeneralized (cf., Shadish et al., 2002 ). For example, operationalizing reasoning (i.e., fluid intelligence) with a single task (e.g., the Raven matrices; Wüstenberg et al., 2012 ; Greiff and Fischer, 2013 ) is not sufficient. Construct validity is also restricted if only one task is used to measure WMC (e.g., Bühner et al., 2008 ; Schweizer et al., 2013 ). Since Spearman’s (1904) , work we know that task-specific variance can be reduced only through heterogeneous operationalizations of the intended constructs. The two studies reported in this paper show how strongly the relationship between intelligence and CPS performance varies depending on the generality level of the intelligence construct (see also Kretzschmar et al., 2017 ). The symmetry problem was demonstrated here for the BIS, but is also evident with regard to other hierarchical intelligence models, e.g., the Three Stratum theory ( Carroll, 1993 , 2005 ), the extended Gf-Gc theory ( Horn and Blankson, 2005 ; Horn, 2008 ), and the Cattell-Horn-Carroll theory (CHC theory; McGrew, 2005 , 2009 ). Süß and Beauducel (2011) , therefore, classified every task of the most frequently used tests into the BIS, the three stratum theory, and the CHC theory to give a framework for this problem.
According to the BIS ( Jäger, 1982 ), every intelligence task depends on at least two abilities (an operative and a content ability), i.e., every task relates to two different constructs. By extension, the interpretation in terms of only one ability is of limited validity due to unintended but reliable task-specific variance. It is either necessary to have several tasks for every construct and theory-based aggregation ( Jäger, 1982 , 1984 ) to reduce unintended variance, or the interpretation must be limited to a more specific conclusion (e.g., to numerical reasoning in our first study). The two studies presented here and many others show that these kinds of problems substantially influence the validity of conclusions in intelligence and problem solving research as well as in many other fields ( Shadish et al., 2002 ).
In summary, the MCS approach provides solutions to psychometrics problems in CPS research, especially the reliability problem, but its validity as an indicator of CPS performance is substantially restricted. In our view, MCS are an interesting new class of problem-solving tasks, but provide few insights into complex real-world problem solving. Modifications of the MCS approach toward increased complexity (e.g., MicroFIN; Neubert et al., 2015 ; Kretzschmar et al., 2017 ) are a promising step in the right direction.
Conclusion and Outlook
The primary aim of CPS research with CRSs (e.g., Lohhausen ; Dörner et al., 1983 ) is ecological validity, i.e., “the validity of the empirical results as psychological statements for the real world” ( Fahrenberg, 2017 ). In the past, many systems were “ ad hoc ” constructions by psychologists that had not been sufficiently validated, but this need not be the case. What is needed is interdisciplinary research in the form of collaboration with experts in the simulated domains. For example, Dörner collaborated with a business expert to develop Tailorshop. Powerplant was developed by Wallach (1997) together with engineers from a coal-fired power plant near Saarbrücken (Germany). LEARN! , a complex management simulator with more than 2000 connected variables, was originally developed by an economics research group at the University of Mannheim (Germany) as a tool for testing economic theories ( Milling, 1996 ; Größler et al., 2000 ; Maier and Größler, 2000 ). In the version applied by Wittmann et al. (1996) , participants have to manage a high-technology company competing with three others simulated by the computer. ATC ( Air Traffic Controller Test; Ackerman and Kanfer, 1993 ) and TRACON ( Terminal Radar Air Control ; Ackerman, 1992 ) are simplified versions of vocational training simulators for professional air traffic controllers. The Situational Awareness Real Time Assessment Tool ( SARA-T ) was developed to measure the situational awareness of air traffic controllers working in the NLR ATM Research Simulator ( NARSIM ; ten Have, 1993 ), a system also used in expert studies ( Kraemer and Süß, 2015 ; Kraemer, 2018 ). Finally, technological developments (e.g., video clips, virtual worlds; Funke, 1998 ) have enabled the development of complex systems that are much more similar to real-world demands than ever before, an opportunity that should be capitalized upon in psychological research (see Dörner and Funke, 2017 ).
In this line of research, the ecological validity of the simulated real-world relationships is essential and must be ensured. In addition, domain-specific prior knowledge is necessary to generate hypotheses for system exploration and system control. Valid measures of the amount, type, and structure of domain-specific prior knowledge, the knowledge acquisition processes, and the acquired knowledge are necessary for understanding and measuring CPS behavior and performance. In light of all this, this line of research can help us to understand how people face the challenge of dealing with complexity and uncertainty, identify causes of failure, and detect successful strategies for reducing complexity during problem solving (e.g., Dörner, 1996 ; Dörner and Funke, 2017 ), a laborious and time-consuming but important field of research in complex decision making (cf., Gigerenzer and Gaissmaier, 2011 ). The research strategy of restricting complex problem solving tasks to MCS, however, leads into a cul-de-sac.
Ethics Statement
The studies were carried out in accordance with the ethical guidelines of the German Association of Psychology with informed consent from all subjects. Considering the time when the studies were conducted and the fact that the materials and procedures were not invasive, the studies were not approved by an ethical committee.
Author Contributions
H-MS conceptualized the manuscript and conducted the first study. AK conducted the second study. H-MS and AK analyzed the data and drafted the manuscript in collaboration.
The first study was supported by a grant from the Free University of Berlin’s Commission for Research Promotion (FNK) to the first author and A. O. Jäger. We further acknowledge support by the Deutsche Forschungsgemeinschaft and the University of Tübingen’s Open Access Publishing Fund. In addition, this research project was supported by the Postdoc Academy of the Hector Research Institute of Education Sciences and Psychology, Tübingen, funded by the Baden-Württemberg Ministry of Science, Education and the Arts.
Conflict of Interest Statement
The authors declare that the research was conducted in the absence of any commercial or financial relationships that could be construed as a potential conflict of interest.
Acknowledgments
We thank Klaus Oberauer for his helpful comments on the manuscript.
- ^ The correlation between complex real life-oriented systems and reasoning was not reported, nor was the effect of outliers on relationships other than that between CPS and g.
- ^ Participants only took the economics test in the first Berlin study, i.e., these data were assessed 1 year before all others reported here.
- ^ The first group could explore the system for 30 min on their own (exploration group), while the second group could study the system’s causal model for 30 min following standardized instructions (instructions group). The third group had no opportunity to acquire additional system-specific knowledge (control group). In this paper, we use the results for the full sample without considering the experimental variations. Experimental results and group-specific results are reported in Süß (1996).
- ^ The correlation with CPS was slightly higher ( r = 0.27) for a conventional g-score based on the factor scores of the first unrotated factor ( Jensen and Wang, 1994 ), i.e., 7.3% of CPS variance was explained.
- ^ According to the BIS, numerical reasoning is not a more specific ability but a performance based on reasoning and numerical intelligence ( Jäger, 1982 ).
- ^ The correlation of CPS performance with figural reasoning was 0.26, and 0.24 with verbal reasoning.
- ^ A subsample was used in Kretzschmar and Süß (2015) to evaluate a CPS training. However, none of the relations between CPS and the variables used in the present study have been previously examined (for details, see the data transparency table at https://osf.io/n2jvy ). Therefore, all analyses and findings presented here are novel.
- ^ We would like to thank Clemens Leutner for professional advice in developing the questionnaire.
- ^ https://osf.io/n2jvy
- ^ For a review focusing on CPS research applying the minimally complex systems (MCS) approach, see Kretzschmar and Süß (2015) .
- ^ In the study of Ryan (2006) with 298 University students the intercorrelations of three scenarios, Furniture Factory (FF), Taylorshop (T) and FSYS (F), were also rather small but significant ( r FF,T = 0.30, r FF,F = 0.27, r T,F = 0.10; Stankov, 2017 ).
- ^ The structural equation model by Süß (2001) is copied in Wittmann and Hattrup (2004) as Figure 6 . This model was built in two steps: First, BIS and CPS-g were modeled separately. Specific CPS factors for the three systems were not modeled because only two indicators were available for each system. Instead, the errors of the two indicators in each system were allowed to correlated as system-specific variance. Second, the five BIS factors (g and the four operative abilities) were used to predict CPS-g. Fit statistics for the final model are not valid because the loadings of both measurement models were optimized in the first step.
Ackerman, P. L. (1992). Predicting individual differences in complex skill acquisition: dynamics of ability determinants. J. Appl. Psychol. 77, 598–614. doi: 10.1037/0021-9010.77.5.598
PubMed Abstract | CrossRef Full Text | Google Scholar
Ackerman, P. L., and Kanfer, R. (1993). Integrating laboratory and field study for improving selection: development of a battery for predicting air traffic controller success. J. Appl. Psychol. 78, 413–432. doi: 10.1037/0021-9010.78.3.413
CrossRef Full Text | Google Scholar
Bainbridge, L. (1974). “Analysis of verbal protocols from a process control task,” in The Human Operator in Process Control , eds E. Edwards and F. P. Lees (London: Taylor & Francis), 146–158.
Google Scholar
Barth, C. M., and Funke, J. (2010). Negative affective environments improve complex solving performance. Cogn. Emot. 24, 1259–1268. doi: 10.1080/02699930903223766
Beckmann, J. F., and Goode, N. (2014). The benefit of being naïve and knowing it: the unfavourable impact of perceived context familiarity on learning in complex problem solving tasks. Instr. Sci. 42, 271–290. doi: 10.1007/s11251-013-9280-7
Berry, D. C., and Broadbent, D. E. (1988). Interactive tasks and the implicit-explicit distinction. Br. J. Psychol. 79, 251–272. doi: 10.1111/j.2044-8295.1988.tb02286.x
Berry, D. C., and Dienes, Z. (1993). Implicit Learning. Theoretical and Empirical Issues. Hillsdale, MI: LEA.
Brehmer, B. (1986). “In one word: not from experience,” in Judgment and Decision Making , eds H. R. Arkes and K. R. Hammond (Cambridge: Cambridge University Press), 705–720.
Broadbent, D. E., Fitzgerald, P., and Broadbent, M. H. P. (1986). Implicit and explicit knowledge in the control of complex systems. Br. J. Psychol. 77, 33–50. doi: 10.1111/j.2044-8295.1986.tb01979.x
Buchner, A., and Funke, J. (1993). Finite state automata: dynamic task environments in problem solving research. Q. J. Exp. Psychol. 46A, 83–118. doi: 10.1080/14640749308401068
Bühner, M., Kröner, S., and Ziegler, M. (2008). Working memory, visual–spatial-intelligence and their relationship to problem-solving. Intelligence 36, 672–680. doi: 10.1016/j.intell.2008.03.008
Carroll, J. B. (1993). Human Cognitive Abilities. A Survey of Factor-Analytic Studies. New York, NY: Cambridge University Press. doi: 10.1017/CBO9780511571312
Carroll, J. B. (2005). “The three-stratum theory of cognitive abilities,” in Contemporary Intellectual Assessment: Theories, Test, and Issues , 2nd Edn, eds D. P. Flanagan and P. L. Harrison (New York, NY: Guilford Press), 69–76.
Cattell, R. B. (1987). Intelligence: Its Structure, Growth, and Action. Amsterdam: Elsevier.
Ceci, S. J., and Liker, J. K. (1986). A day at the races: a study of IQ, expertise, and cognitive complexity. J. Exp. Psychol. Gen. 115, 255–266. doi: 10.1037/0096-3445.115.3.255
Chi, M. T. H., Glaser, R., and Farr, M. J. (1988). The Nature of Expertise. Hillsdale, NJ: Erlbaum.
Conway, A. R., Kane, M. J., and Engle, R. W. (2003). Working memory capacity and its relation to general intelligence. Trends Cogn. Sci. 7, 547–552. doi: 10.1016/j.tics.2003.10.005
Csapó, B., and Molnár, G. (2017). Potential for assessing dynamic problem-solving at the beginning of higher education studies. Front. Psychol. 8:2022. doi: 10.3389/fpsyg.2017.02022
Danner, D., Hagemann, D., Holt, D. V., Hager, M., Schankin, A., Wüstenberg, S., et al. (2011). Measuring performance in dynamic decision making: reliability and validity of the Tailorshop simulation. J. Individ. Dif. 32, 225–233. doi: 10.1027/1614-0001/a000055
Deutsche Gesellschaft für Personalwesen [DGP] (1986). Differentieller Kenntnistest (DKT). Subtest Wirtschaft [Differential Test of Knowledge: Subtest Economics]. Hannover: DGP.
Dörner, D. (1986). Diagnostik der operativen Intelligenz [Diagnostics of operative intelligence]. Diagnostica 32, 290–308.
Dörner, D. (1996). The Logic of Failure: Recognizing and Avoiding Error in Complex Situations. New York, NY: Basic Books.
Dörner, D., and Funke, J. (2017). Complex problem solving: what it is and what it is not. Front. Psychol. 8:1153. doi: 10.3389/fpsyg.2017.01153
Dörner, D., and Kreuzig, H. W. (1983). Problemlösefähigkeit und Intelligenz [Problem solving ability and intelligence]. Psychol. Rundsch. 34, 185–192.
Dörner, D., Kreuzig, H. W., Reither, F., and Stäudel, T. (1983). Lohhausen. Vom Umgang mit Unbestimmtheit und Komplexität [Lohhausen. About Dealing with Uncertainty and Complexity]. Bern: Huber.
Dutt, V., and Gonzalez, C. (2015). Accounting for outcome and process measures in dynamic decision-making tasks through model calibration. J. Dyn. Decis. Mak. 1, 1–10. doi: 10.11588/jddm.2015.1.17663
Engelhart, M., Funke, J., and Sager, S. (2017). A web-based feedback study on optimization-based training and analysis of human decision making. J. Dyn. Decis. Mak. 3, 1–23. doi: 10.11588/jddm.2017.1.34608
Fahrenberg, J. (2017). “Ökologische Validität [ecological validity],” in Dorsch - Lexikon der Psychologie , ed. H. Wirz (Bern: Huber), 1202.
Fishbein, M., and Ajzen, I. (1974). Attitudes towards objects as predictors of single and multiple behavioral criteria. Psychol. Rev. 81, 59–74. doi: 10.1037/h0035872
Frensch, P. A., and Funke, J. (1995). “Definitions, traditions, and a general framework for understanding complex problem solving,” in Complex Problem Solving. The European Perspective , eds P. A. Frensch and J. Funke (Hillsdale, NJ: Lawrence Erlbaum Associates), 3–25.
Funke, J. (1983). Einige Bemerkungen zu Problemen der Problemlöseforschung oder: Ist Testintelligenz doch ein Prädiktor? [Issues in problem solving research: is test intelligence a predictor after all?]. Diagnostica 29, 283–302.
Funke, J. (1985). Steuerung dynamischer Systeme durch Aufbau und Anwendung subjektiver Kausalmodelle [Control of dynamic systems by building up and using subjective causal models]. Z. Psychol. 193, 435–457.
Funke, J. (1992). Wissen über dynamische Systeme: Erwerb, Repräsentation und Anwendung [Knowledge About Dynamic Systems: Acquisition, Representation, and use]. Berlin: Springer. doi: 10.1007/978-3-64x2-77346-4
CrossRef Full Text
Funke, J. (1993). “Microworlds based on linear equation systems: a new approach to complex problem solving and experimental results,” in The Cognitive Psychology of Knowledge , eds G. Strube and K.-F. Wender (Amsterdam: Elsevier), 313–330.
Funke, J. (1998). Computer-based testing and training with scenarios from complex problem solving research: advantages and disadvantages. Int. J. Sel. Assess. 6, 90–96. doi: 10.1111/1468-2389.00077
Funke, J. (2006). “Komplexes Problemlösen,” in Denken und Problemlösen (Enzyklopädie der Psychologie, Serie II Kognition, Bd. 8 , ed. J. Funke (Göttingen: Hogrefe), 375–445.
Funke, J. (2014). Analysis of minimal complex systems and complex problem solving require different forms of causal cognition. Front. Psychol. 5:739. doi: 10.3389/fpsyg.2014.00739
Funke, J., Fischer, A., and Holt, V. D. (2017). When less is less: solving multiple simple problems is not complex problem solving—a comment on Greiff et al. (2015). J. Intell. 5:5. doi: 10.3390/jintelligence5010005
Funke, U. (1995). “Using complex problem solving tasks in personnel selection and training,” in Complex Problem Solving. The European Perspective , eds P. A. Frensch and J. Funke (Hillsdale NJ: Erlbaum), 219–240.
Gigerenzer, G., and Gaissmaier, W. (2011). Heuristic decision making. Annu. Rev. Psychol. 62, 451–482. doi: 10.1146/annurev-psych-120709-145346
Gonzalez, C., and Dutt, V. (2011). A generic dynamic control task for behavioral research and education. Comput. Hum. Behav. 27, 1904–1914. doi: 10.1016/j.chb.2011.04.015
Gonzalez, C., Lerch, J. F., and Lebiere, C. (2003). Instance-based learning in dynamic decision making. Cogn. Sci. 27, 591–635. doi: 10.1016/S0364-0213(03)00031-4
Goode, N., and Beckmann, J. F. (2010). You need to know: there is a causal relationship between structural knowledge and control performance in complex problem solving tasks. Intelligence 38, 345–352. doi: 10.1016/j.intell.2010.01.001
Goode, N., and Beckmann, J. F. (2016). With a little help …: on the role of guidance in the acquisition and utilisation of knowledge in the control of complex, dynamic systems. J. Dyn. Decis. Mak. 2:4. doi: 10.11588/jddm.2016.1.33346
Greiff, S., and Fischer, A. (2013). Der Nutzen einer komplexen Problemlösekompetenz: Theoretische Überlegungen und empirische Befunde [The value of complex problem solving competency: theoretical considerations and empirical results]. Z. Pädagog. Psychol. 27, 27–39. doi: 10.1024/1010-0652/a000086
Greiff, S., Fischer, A., Stadler, M., and Wüstenberg, S. (2015a). Assessing complex problem-solving skills with multiple complex systems. Think. Reason. 21, k356–382. doi: 10.1080/13546783.2014.989263
Greiff, S., Kretzschmar, A., Müller, J. C., Spinath, B., and Martin, R. (2014). The computer-based assessment of complex problem solving and how it is influenced by students’ information and communication technology literacy. J. Educ. Psychol. 106, 666–680. doi: 10.1037/a0035426
Greiff, S., Krkovic, K., and Hautamäki, J. (2016). The prediction of problem-solving assessed via microworlds a study on the relative relevance of fluid reasoning and working memory. Eur. J. Psychol. Assess. 32, 298–306. doi: 10.1027/1015-5759/a000263
Greiff, S., Stadler, M., Sonnleitner, P., Wolff, C., and Martin, R. (2015b). Sometimes less is more: comparing the validity of complex problem solving measures. Intelligence 50, 100–113. doi: 10.1016/j.intell.2015.02.007
Greiff, S., Wüstenberg, S., and Funke, J. (2012). Dynamic problem solving: a new assessment perspective. Appl. Psychol. Meas. 36, 189–213. doi: 10.1177/0146621612439620
Greiff, S., Wüstenberg, S., Holt, D. V., Goldhammer, F., and Funke, J. (2013a). Computer-based assessment of Complex Problem Solving: concept, implementation, and application. Educ. Technol. Res. Dev. 61, 407–421. doi: 10.1007/s11423-013-9301-x
Greiff, S., Wüstenberg, S., Molnar, G., Fischer, A., Funke, J., and Csapo, B. (2013b). Complex problem solving in educational contexts—something beyond g: concept, assessment, measurement invariance, and construct validity. J. Educ. Psychol. 105, 364–379. doi: 10.1037/a0031856
Größler, A., Maier, F. H., and Milling, P. M. (2000). Enhancing learning capabilities by providing transparency in business simulators. Simul. Gaming 31, 257–278. doi: 10.1177/104687810003100209
Gustafsson, J.-E., and Balke, G. (1993). General and specific abilities as predictors of school achievement. Multivariate Behav. Res. 28, 407–434. doi: 10.1207/s15327906mbr2804_2
Haynes, S. N., Richard, D. C. S., and Kubany, E. S. (1995). Content validity in psychological assessment: a functional approach to concepts and methods. Psychol. Assess. 7, 238–247. doi: 10.1037/1040-3590.7.3.238
Herde, C. N., Wüstenberg, S., and Greiff, S. (2016). Assessment of complex problem solving: what we know and what we don’t know. Appl. Meas. Educ. 29, 265–277. doi: 10.1080/08957347.2016.1209208
Hesse, F. W. (1982). Effekte des semantischen Kontextes auf die Bearbeitung komplexer Probleme [Effect of semantic context on the solution of complex problems]. Z. Exp. Angew. Psychol. 29, 62–91.
Horn, J. L. (2008). “Spearman, g, expertise, and the nature of human cognitive capability,” in Extending Intelligence: Enhancement and New Constructs , eds P. C. Kyllonen, R. D. Roberts, and L. Stankov (New York, NY: Lawrence Erlbaum Associates), 185–230.
Horn, J. L., and Blankson, N. (2005). “Foundations for better understanding of cognitive abilities,” in Contemporary Intellectual Assessment: Theories, Tests, and Issues , 2nd Edn, eds D. P. Flanagan and P. I. Harrison (New York, NY: Guilford Press), 41–68.
Jäger, A. O. (1982). Mehrmodale Klassifikation von Intelligenzleistungen. Experimentell kontrollierte Weiterentwicklung eines deskriptiven Intelligenzstrukturmodells [Multimodal classification of intellectual performance. Experimental development of a descriptive intelligence structure model]. Diagnostica 28, 195–226.
Jäger, A. O. (1984). Intelligenzstrukturforschung: Konkurrierende Modelle, neue Entwicklungen, Perspektiven [Intelligence structure research: competing models, new developments, perspectives]. Psychol. Rundsch. 35, 21–35.
Jäger, A. O., Süß, H.-M., and Beauducel, A. (1997). Test für das Berliner Intelligenzstrukturmodell. BIS-Test. Form 4 [Test for the Berlin Intelligence Structure Model]. Göttingen: Hogrefe.
Jensen, A. R., and Wang, L.-J. (1994). What is a good g? Intelligence 18, 231–258. doi: 10.1016/0160-2896(94)90029-9
Kerlinger, F. N., and Pedhazur, E. J. (1973). Multiple Regression in Behavioral Research. New York, NY: Holt, Rinehart and Winston.
Kersting, M. (2001). Zur Konstrukt- und Kriteriumsvalidität von Problemlöseszenarien anhand der Vorhersage von Vorgesetztenurteilen über die berufliche Bewährung [On the construct and criterion validity of problem-solving scenarios based on the prediction of supervisor assessment of job performance]. Diagnostica 47, 67–76. doi: 10.1026//0012-1924.47.2.67
Kersting, M., and Süß, H.-M. (1995). Kontentvalide Wissensdiagnostik und Problemlösen: Zur Entwicklung, testtheoretischen Begründung und empirischen Bewährung eines problemspezifischen Diagnoseverfahrens [Content-valid diagnosis of knowledge and problem-solving: development, test theory justification, and empirical validation of a new problem-specific test]. Z. Pädagog. Psychol. 9, 83–93.
King, J., and Just, M. A. (1991). Individual differences in syntactic processing: the role of working memory. J. Mem. Lang. 30, 580–602. doi: 10.1016/0749-596X(91)90027-H
Klauer, K. J. (1984). Kontentvalidität. [Content validity]. Diagnostica 30, 1–23.
Kluge, A. (2008b). What you train is what you get? Task requirements and training methods in complex problem-solving. Comput. Hum. Behav. 24, 284–308. doi: 10.1016/j.chb.2007.01.013
Kluge, A. (2008a). Performance assessments with microworlds and their difficulty. Appl. Psychol. Meas. 32, 156–180. doi: 10.1177/0146621607300015
Kluwe, R. H., Misiak, C., and Haider, H. (1991). “The control of complex systems and performance in intelligence tests,” in Intelligence: Reconceptualization and Measurement , ed. H. Rowe (Hillsdale: Lawrence Erlbaum Associates).
Kotter, J. P. (1982). What effective general managers really do. Harv. Bus. Rev. 60, 156–167.
Kraemer, J. (2018). Die Lücke im Entscheidungsprozess. Die Bedeutsamkeit von Situationsbewusstsein und Optionsgenerierung für die Leistung von Fluglotsen [The Gap in Decision Making. The Significance of Situation Awareness and Option Generation for Air Traffic Controller Performance]. Köln: Deutsches Zentrum fr Luft- und Raumfahrt e. V.
Kraemer, J., and Süß, H.-M. (2015). Real time validation of online situation awareness questionnaires in simulated approach air traffic control. Procedia Manuf. 3, 3152–3159. doi: 10.1016/j.promfg.2015.07.864
Kretzschmar, A. (2015). Konstruktvalidität des komplexen Problemlösens Unter Besonderer Berücksichtigung Moderner Diagnostischer Ansätze [Construct Validity of Complex Problem Solving With Particular Focus on Modern Assessment Approaches]. Doctoral dissertation, University of Luxembourg, Luxembourg.
Kretzschmar, A. (2017). Sometimes less is not enough: a commentary on Greiff et al. (2015). J. Intell. 5:4. doi: 10.3390/jintelligence5010004
Kretzschmar, A., Hacatrjana, L., and Rascevska, M. (2017). Re-evaluating the psychometric properties of MicroFIN: a multidimensional measurement of complex problem solving or a unidimensional reasoning test? Psychol. Test Assess. Model. 59, 157–182.
Kretzschmar, A., Neubert, J. C., and Greiff, S. (2014). Komplexes Problemlösen, schulfachliche Kompetenzen und ihre Relation zu Schulnoten [Complex problem solving, school competencies and their relation to school grades]. Z. Pädagog. Psychol. 28, 205–215. doi: 10.1024/1010-0652/a000137
Kretzschmar, A., Neubert, J. C., Wüstenberg, S., and Greiff, S. (2016). Construct validity of complex problem solving: a comprehensive view on different facets of intelligence and school grades. Intelligence 54, 55–69. doi: 10.1016/j.intell.2015.11.004
Kretzschmar, A., and Süß, H.-M. (2015). A study on the training of complex problem solving competence. J. Dyn. Decis. Mak. 1, 1–14. doi: 10.11588/jddm.2015.1.15455
Kröner, S. (2001). Intelligenzdiagnostik per Computersimulation [Intelligence assessment via computer simulation]. Münster: Waxmann.
Kröner, S., Plass, J. L., and Leutner, D. (2005). Intelligence assessment with computer simulations. Intelligence 33, 347–368. doi: 10.1016/j.intell.2005.03.002
Krumm, V., and Seidel, G. (1970). Wirtschaftslehretest [Economics Test]. Weinheim: Beltz.
Kyllonen, P. C., and Christal, R. E. (1990). Reasoning ability is (little more than) working-memory capacity?! Intelligence 14, 389–433. doi: 10.1016/S0160-2896(05)80012-1
Leutner, D. (2002). The fuzzy relationship of intelligence and problem solving in computer simulations. Comput. Hum. Behav. 18, 685–697. doi: 10.1016/s0747-5632(02)00024-9
Lotz, C., Scherer, R., Greiff, S., and Sparfeldt, J. R. (2017). Intelligence in action – Effective strategic behaviors while solving complex problems. Intelligence 64, 98–112. doi: 10.1016/j.intell.2017.08.002
Lotz, C., Sparfeldt, J. R., and Greiff, S. (2016). Complex problem solving in educational contexts – Still something beyond a “good g”? Intelligence 59, 127–138. doi: 10.1016/j.intell.2016.09.001
Maier, F. H., and Größler, A. (2000). What are we talking about? - A taxonomy of computer simulations to support learning. Syst. Dyn. Rev. 16, 135–148. doi: 10.1002/1099-1727(200022)16:2<135::AID-SDR193>3.0.CO;2-P
McGrew, K. S. (2005). “The Cattell-Horn-Carroll theory of cognitive abilities,” in Contemporary Intellectual Assessment: Theories, Test, and Issues , 2nd Edn, eds D. P. Flanagan and P. L. Harrison (New York, NY: Guilford Press), 136–181.
McGrew, K. S. (2009). CHC theory and the human cognitive abilities project: standing on the shoulders of the giants of psychometric intelligence research. Intelligence 37, 1–10. doi: 10.1016/j.intell.2008.08.004
Milling, P. M. (1996). Modeling innovation processes for decision support and management simulation. Syst. Dyn. Rev. 12, 211–234. doi: 10.1002/(SICI)1099-1727(199623)12:3<211::AID-SDR105>3.0.CO;2-8
Mintzberg, H. (1973). The Nature of Managerial Work. New York, NY: Harper & Row.
Morris, N. M., and Rouse, W. B. (1985). The effects of type of knowledge upon human problem solving in a process control task. IEEE Trans. Syst. Man Cybern. 15, 698–707. doi: 10.1109/TSMC.1985.6313453
Neubert, J. C., Kretzschmar, A., Wüstenberg, S., and Greiff, S. (2015). Extending the assessment of complex problem solving to finite state automata: embracing heterogeneity. Eur. J. Psychol. Assess. 31, 181–194. doi: 10.1027/1015-5759/a000224
Oberauer, K., Süß, H.-M., Wilhelm, O., and Wittmann, W. W. (2003). The multiple faces of working memory: storage, processing, supervision, and coordination. Intelligence 31, 167–193. doi: 10.1016/S0160-2896(02)00115-0
Oberauer, K., Süß, H.-M., Wilhelm, O., and Wittmann, W. W. (2008). Which working memory functions predict intelligence? Intelligence 36, 641–652. doi: 10.1016/j.intell.2008.01.007
OECD (2014). Pisa 2012 Results: Creative Problem Solving: Students’ Skills in Tackling Real-Life Problems (Volume V). Paris: OECD Publishing. doi: 10.1787/9789264208070-en
Putz-Osterloh, W. (1981). Über die Beziehung zwischen Testintelligenz und Problemlöseerfolg [On the relationship between test intelligence and problem solving success]. Z. Psychol. 189, 79–100.
Rigas, G., Carling, E., and Brehmer, B. (2002). Reliability and validity of performance measures in microworlds. Intelligence 30, 463–480. doi: 10.1016/S0160-2896(02)00121-6
Ryan, K. J. (2006). The Relationship Between Complex Problem Solving and Intelligence: An Analysis of Three Computer Simulated Scenarios. Doctoral dissertation, University of Sydney, Sydney.
Ryle, G. (1949). The Concept of Mind. London: Hutchinson.
Scherer, R. (2015). Is it time for a new measurement approach? A closer look at the assessment of cognitive adaptability in complex problem solving. Front. Psychol. 6:1664. doi: 10.3389/fpsyg.2015.01664
Scherer, R., and Tiemann, R. (2014). Measuring students’ progressions in scientific problem solving: a psychometric approach. Procedia Soc. Behav. Sci. 112, 87–96. doi: 10.1016/j.sbspro.2014.01.1142
Schmid, J., and Leiman, J. M. (1957). The development of hierarchical factor solutions. Psychometrika 22, 53–61. doi: 10.1007/BF02289209
Schmidt, F. L. (1992). What do data really mean? Research findings, meta-analysis, and cumulative knowledge in psychology. Am. Psychol. 47, 1173–1181. doi: 10.1037/0003-066X.47.10.1173
Schmidt, F. L., Hunter, J. E., and Outerbridge, A. N. (1986). Impact of job experience and ability on job knowledge, work sample performance, and supervisory ratings of job performance. J. Appl. Psychol. 71, 432–439. doi: 10.1037/0021-9010.71.3.432
Schoppek, W., and Fischer, A. (2015). Complex problem solving-single ability or complex phenomenon? Front. Psychol. 6:1669. doi: 10.3389/fpsyg.2015.01669
Schweizer, F., Wüstenberg, S., and Greiff, S. (2013). Validity of the MicroDYN approach: complex problem solving predicts school grades beyond working memory capacity. Learn. Individ. Dif. 24, 42–52. doi: 10.1016/j.lindif.2012.12.011
Shadish, W. R., Cook, T. D., and Campbell, D. T. (2002). Experimental and Quasi-Experimental Designs for Generalized Causal Inference. Boston, MA: Houghton-Mifflin.
Sonnleitner, P., Brunner, M., Greiff, S., Funke, J., Keller, U., Martin, R., et al. (2012). The genetics lab: acceptance and psychometric characteristics of a computer-based microworld assessing complex problem solving. Psychol. Test Assess. Model. 54, 54–72. doi: 10.1037/e578442014-045
Sonnleitner, P., Keller, U., Martin, R., and Brunner, M. (2013). Students’ complex problem-solving abilities: their structure and relations to reasoning ability and educational success. Intelligence 41, 289–305. doi: 10.1016/j.intell.2013.05.002
Spearman, C. (1904). “General intelligence”, objectively determined and measured. Am. J. Psychol. 15, 201–293. doi: 10.2307/1412107
Spering, M., Wagener, D., and Funke, J. (2005). The role of emotions in complex problem-solving. Cogn. Emot. 19, 1252–1261. doi: 10.1080/02699930500304886
Stadler, M. J., Becker, N., Gödker, M., Leutner, D., and Greiff, S. (2015). Complex problem solving and intelligence: a meta-analysis. Intelligence 53, 92–101. doi: 10.1016/j.intell.2015.09.005
Stadler, M. J., Becker, N., Greiff, S., and Spinath, F. M. (2016). The complex route to success: complex problem-solving skills in the prediction of university success. High. Educ. Res. Dev. 35, 365–379. doi: 10.1080/07294360.2015.1087387
Stankov, L. (2017). Overemphasized “g”. J. Intell. 5:33. doi: 10.3390/jintelligence5040033
Süß, H.-M. (1996). Intelligenz, Wissen und Problemlösen. Kognitive Voraussetzungen für erfolgreiches Handeln bei computersimulierten Problemen [Intelligence, Knowledge, and Problem Solving: Cognitive Prerequisites of Successful Performance in Computer-Simulated Problems]. Lehr- und Forschungstexte Psychologie. Göttingen: Hogrefe.
Süß, H.-M. (1999). Intelligenz und komplexes Problemlösen: Perspektiven für eine Kooperation zwischen differentiell-psychometrischer und kognitionspsychologischer Forschung [Intelligence and complex problem solving: perspectives on the cooperation between differential-psychometric and cognitive research methods]. Psychol. Rundsch. 50, 220–228. doi: 10.1026//0033-3042.50.4.220
Süß, H.-M. (2001). “The predictive validity of reasoning and g in complex problem solving,” in Paper Presented at the ISSID 2001 Conference , Edinburgh.
Süß, H.-M., and Beauducel, A. (2005). “Faceted models of intelligence,” in Understanding and Measuring Intelligence , eds O. Wilhelm and R. Engle (Thousand Oaks, CA: Sage), 313–332.
Süß, H.-M., and Beauducel, A. (2011). “Intelligenztests und ihre Bezüge zu Intelligenztheorien. [Intelligence tests and their relationships to theories of intelligence],” in Leistungs-, Intelligenz- und Verhaltensdiagnostik (Enzyklopädie der Psychologie, Serie Psychologische Diagnostik, Bd. 3 , eds L. F. Hornke, M. Amelang, and M. Kersting (Göttingen: Hogrefe), 97–234.
Süß, H.-M., and Beauducel, A. (2015). Modeling the construct validity of the Berlin intelligence structure model. Estud. Psicol. 32, 13–25. doi: 10.1590/0103-166X2015000100002
Süß, H.-M., and Faulhaber, J. (1990). Berliner Version der Schneiderwerkstatt. PC-Simulationsprogramm [Berlin Version of the Tailorshop]. Berlin: Freie Univer-sität Berlin, Fachbereich Erziehungs- und Unterrichtswissenschaften, Institut für Psychologie.
Süß, H.-M., Kersting, M., and Oberauer, K. (1991). Intelligenz und Wissen als Prädiktoren für Leistungen bei computersimulierten komplexen Problemen [Intelligence and knowledge as predictors of performance in solving complex computer-simulated problems]. Diagnostica 37, 334–352.
Süß, H.-M., Kersting, M., and Oberauer, K. (1993a). Zur Vorhersage von Steuerungsleistungen an computersimulierten Systemen durch Wissen und Intelligenz [On the predictability of control performance on computer-simulated systems by knowledge and intelligence]. Z. Differ. Diagnostische Psychol. 14, 189–203.
Süß, H.-M., Oberauer, K., and Kersting, M. (1993b). Intellektuelle Fähigkeiten und die Steuerung komplexer Systeme [Intelligence and control performance on computer-simulated systems]. Spr. Kognition 12, 83–97.
Süß, H.-M., Oberauer, K., Wittmann, W. W., Wilhelm, O., and Schulze, R. (2002). Working-memory capacity explains reasoning ability - And a little bit more. Intelligence 30, 261–288. doi: 10.1016/S0160-2896(01)00100-3
Swanson, L., and Kim, K. (2007). Working memory, short-term memory, and naming speed as predictors of children’s mathematical performance. Intelligence 35, 151–168. doi: 10.1016/j.intell.2006.07.001
ten Have, J. M. (1993). The development of the NLR ATC Research Simulator (Narsim): design philosophy and potential for ATM research. Simul. Pract. Theory 1, 31–39. doi: 10.1016/0928-4869(93)90009-F
Vollmeyer, R., Burns, B. D., and Holyoak, K. J. (1996). The impact of goal specificity on strategy use and the acquisition of problem structure. Cogn. Sci. 20, 75–100. doi: 10.1207/s15516709cog2001_3
Wagener, D. (2001). Psychologische Diagnostik mit komplexen Szenarios - Taxonomie, Entwicklung, Evaluation [Psychological Assessment with Complex Scenarios - Taxonomy, Development, Evaluation]. Lengerich: Pabst Science Publishers.
Wagener, D., and Wittmann, W. W. (2002). Personalarbeit mit dem komplexen Szenario FSYS [Human resource management using the complex scenario FSYS ]. Z. Personalpsychologie 1, 80–93. doi: 10.1026//1617-6391.1.2.80
Wallach, D. (1997). Kognitionswissenschaftliche Analysen komplexer Problemlöseprozesse [Cognitive Science Analyses of Complex Problem Solving Processes]. Wiesbaden: Westdeutscher Verlag.
Wittmann, W. W. (1988). “Multivariate reliability theory. Principles of symmetry and successful validation strategies,” in Handbook of Multivariate Experimental Psychology , eds R. B. Cattell and J. R. Nesselroade (New York, NY: Plenum), 505–560. doi: 10.1007/978-1-4613-0893-5_16
Wittmann, W. W., and Hattrup, K. (2004). The relationship between performance in dynamic systems and intelligence. Syst. Res. Behav. Sci. 21, 393–409. doi: 10.1002/sres.653
Wittmann, W. W., and Süß, H.-M. (1999). “Investigating the paths between working memory, intelligence, knowledge, and complex problem-solving performances via Brunswik symmetry,” in Learning and Individual Differences: Process, Trait and Content Determinants , eds P. L. Ackerman, P. C. Kyllonen, and R. D. Roberts (Washington, DC: APA), 77–104.
Wittmann, W. W., Süß, H.-M., and Oberauer, K. (1996). Determinanten komplexen Problemlösens [Determinants of Complex Problem Solving]. Research Report No. 9. Mannheim: Universität Mannheim.
Wolfe, J., and Roberts, C. R. (1986). The external validity of a business management game: a five-year longitudinal study. Simul. Games 17, 45–59. doi: 10.1177/0037550086171004
Wüstenberg, S., Greiff, S., and Funke, J. (2012). Complex problem solving - More than reasoning? Intelligence 40, 1–14. doi: 10.1016/j.intell.2011.11.003
Zech, A., Bühner, M., Kröner, S., Heene, M., and Hilbert, S. (2017). The impact of symmetry: explaining contradictory results concerning working memory, reasoning, and complex problem solving. J. Intell. 5:22. doi: 10.3390/jintelligence5020022
Keywords : complex problem solving, microworlds, minimally complex systems, intelligence, investment theory, knowledge assessment, working memory, Brunswik symmetry
Citation: Süß H-M and Kretzschmar A (2018) Impact of Cognitive Abilities and Prior Knowledge on Complex Problem Solving Performance – Empirical Results and a Plea for Ecologically Valid Microworlds. Front. Psychol. 9:626. doi: 10.3389/fpsyg.2018.00626
Received: 06 October 2017; Accepted: 13 April 2018; Published: 08 May 2018.
Reviewed by:
Copyright © 2018 Süß and Kretzschmar. This is an open-access article distributed under the terms of the Creative Commons Attribution License (CC BY) . The use, distribution or reproduction in other forums is permitted, provided the original author(s) and the copyright owner are credited and that the original publication in this journal is cited, in accordance with accepted academic practice. No use, distribution or reproduction is permitted which does not comply with these terms.
*Correspondence: Heinz-Martin Süß, [email protected]
Disclaimer: All claims expressed in this article are solely those of the authors and do not necessarily represent those of their affiliated organizations, or those of the publisher, the editors and the reviewers. Any product that may be evaluated in this article or claim that may be made by its manufacturer is not guaranteed or endorsed by the publisher.

26 Expert-Backed Problem Solving Examples – Interview Answers
Published: February 13, 2023
Interview Questions and Answers
Actionable advice from real experts:

Biron Clark
Former Recruiter

Contributor
Dr. Kyle Elliott
Career Coach

Hayley Jukes
Editor-in-Chief

Biron Clark , Former Recruiter
Kyle Elliott , Career Coach

Hayley Jukes , Editor
As a recruiter , I know employers like to hire people who can solve problems and work well under pressure.
A job rarely goes 100% according to plan, so hiring managers are more likely to hire you if you seem like you can handle unexpected challenges while staying calm and logical.
But how do they measure this?
Hiring managers will ask you interview questions about your problem-solving skills, and they might also look for examples of problem-solving on your resume and cover letter.
In this article, I’m going to share a list of problem-solving examples and sample interview answers to questions like, “Give an example of a time you used logic to solve a problem?” and “Describe a time when you had to solve a problem without managerial input. How did you handle it, and what was the result?”
- Problem-solving involves identifying, prioritizing, analyzing, and solving problems using a variety of skills like critical thinking, creativity, decision making, and communication.
- Describe the Situation, Task, Action, and Result ( STAR method ) when discussing your problem-solving experiences.
- Tailor your interview answer with the specific skills and qualifications outlined in the job description.
- Provide numerical data or metrics to demonstrate the tangible impact of your problem-solving efforts.
What are Problem Solving Skills?
Problem-solving is the ability to identify a problem, prioritize based on gravity and urgency, analyze the root cause, gather relevant information, develop and evaluate viable solutions, decide on the most effective and logical solution, and plan and execute implementation.
Problem-solving encompasses other skills that can be showcased in an interview response and your resume. Problem-solving skills examples include:
- Critical thinking
- Analytical skills
- Decision making
- Research skills
- Technical skills
- Communication skills
- Adaptability and flexibility
Why is Problem Solving Important in the Workplace?
Problem-solving is essential in the workplace because it directly impacts productivity and efficiency. Whenever you encounter a problem, tackling it head-on prevents minor issues from escalating into bigger ones that could disrupt the entire workflow.
Beyond maintaining smooth operations, your ability to solve problems fosters innovation. It encourages you to think creatively, finding better ways to achieve goals, which keeps the business competitive and pushes the boundaries of what you can achieve.
Effective problem-solving also contributes to a healthier work environment; it reduces stress by providing clear strategies for overcoming obstacles and builds confidence within teams.
Examples of Problem-Solving in the Workplace
- Correcting a mistake at work, whether it was made by you or someone else
- Overcoming a delay at work through problem solving and communication
- Resolving an issue with a difficult or upset customer
- Overcoming issues related to a limited budget, and still delivering good work through the use of creative problem solving
- Overcoming a scheduling/staffing shortage in the department to still deliver excellent work
- Troubleshooting and resolving technical issues
- Handling and resolving a conflict with a coworker
- Solving any problems related to money, customer billing, accounting and bookkeeping, etc.
- Taking initiative when another team member overlooked or missed something important
- Taking initiative to meet with your superior to discuss a problem before it became potentially worse
- Solving a safety issue at work or reporting the issue to those who could solve it
- Using problem solving abilities to reduce/eliminate a company expense
- Finding a way to make the company more profitable through new service or product offerings, new pricing ideas, promotion and sale ideas, etc.
- Changing how a process, team, or task is organized to make it more efficient
- Using creative thinking to come up with a solution that the company hasn’t used before
- Performing research to collect data and information to find a new solution to a problem
- Boosting a company or team’s performance by improving some aspect of communication among employees
- Finding a new piece of data that can guide a company’s decisions or strategy better in a certain area
Problem-Solving Examples for Recent Grads/Entry-Level Job Seekers
- Coordinating work between team members in a class project
- Reassigning a missing team member’s work to other group members in a class project
- Adjusting your workflow on a project to accommodate a tight deadline
- Speaking to your professor to get help when you were struggling or unsure about a project
- Asking classmates, peers, or professors for help in an area of struggle
- Talking to your academic advisor to brainstorm solutions to a problem you were facing
- Researching solutions to an academic problem online, via Google or other methods
- Using problem solving and creative thinking to obtain an internship or other work opportunity during school after struggling at first
How To Answer “Tell Us About a Problem You Solved”
When you answer interview questions about problem-solving scenarios, or if you decide to demonstrate your problem-solving skills in a cover letter (which is a good idea any time the job description mentions problem-solving as a necessary skill), I recommend using the STAR method.
STAR stands for:
It’s a simple way of walking the listener or reader through the story in a way that will make sense to them.
Start by briefly describing the general situation and the task at hand. After this, describe the course of action you chose and why. Ideally, show that you evaluated all the information you could given the time you had, and made a decision based on logic and fact. Finally, describe the positive result you achieved.
Note: Our sample answers below are structured following the STAR formula. Be sure to check them out!
EXPERT ADVICE

Dr. Kyle Elliott , MPA, CHES Tech & Interview Career Coach caffeinatedkyle.com
How can I communicate complex problem-solving experiences clearly and succinctly?
Before answering any interview question, it’s important to understand why the interviewer is asking the question in the first place.
When it comes to questions about your complex problem-solving experiences, for example, the interviewer likely wants to know about your leadership acumen, collaboration abilities, and communication skills, not the problem itself.
Therefore, your answer should be focused on highlighting how you excelled in each of these areas, not diving into the weeds of the problem itself, which is a common mistake less-experienced interviewees often make.
Tailoring Your Answer Based on the Skills Mentioned in the Job Description
As a recruiter, one of the top tips I can give you when responding to the prompt “Tell us about a problem you solved,” is to tailor your answer to the specific skills and qualifications outlined in the job description.
Once you’ve pinpointed the skills and key competencies the employer is seeking, craft your response to highlight experiences where you successfully utilized or developed those particular abilities.
For instance, if the job requires strong leadership skills, focus on a problem-solving scenario where you took charge and effectively guided a team toward resolution.
By aligning your answer with the desired skills outlined in the job description, you demonstrate your suitability for the role and show the employer that you understand their needs.
Amanda Augustine expands on this by saying:
“Showcase the specific skills you used to solve the problem. Did it require critical thinking, analytical abilities, or strong collaboration? Highlight the relevant skills the employer is seeking.”
Interview Answers to “Tell Me About a Time You Solved a Problem”
Now, let’s look at some sample interview answers to, “Give me an example of a time you used logic to solve a problem,” or “Tell me about a time you solved a problem,” since you’re likely to hear different versions of this interview question in all sorts of industries.
The example interview responses are structured using the STAR method and are categorized into the top 5 key problem-solving skills recruiters look for in a candidate.
1. Analytical Thinking

Situation: In my previous role as a data analyst , our team encountered a significant drop in website traffic.
Task: I was tasked with identifying the root cause of the decrease.
Action: I conducted a thorough analysis of website metrics, including traffic sources, user demographics, and page performance. Through my analysis, I discovered a technical issue with our website’s loading speed, causing users to bounce.
Result: By optimizing server response time, compressing images, and minimizing redirects, we saw a 20% increase in traffic within two weeks.
2. Critical Thinking

Situation: During a project deadline crunch, our team encountered a major technical issue that threatened to derail our progress.
Task: My task was to assess the situation and devise a solution quickly.
Action: I immediately convened a meeting with the team to brainstorm potential solutions. Instead of panicking, I encouraged everyone to think outside the box and consider unconventional approaches. We analyzed the problem from different angles and weighed the pros and cons of each solution.
Result: By devising a workaround solution, we were able to meet the project deadline, avoiding potential delays that could have cost the company $100,000 in penalties for missing contractual obligations.
3. Decision Making

Situation: As a project manager , I was faced with a dilemma when two key team members had conflicting opinions on the project direction.
Task: My task was to make a decisive choice that would align with the project goals and maintain team cohesion.
Action: I scheduled a meeting with both team members to understand their perspectives in detail. I listened actively, asked probing questions, and encouraged open dialogue. After carefully weighing the pros and cons of each approach, I made a decision that incorporated elements from both viewpoints.
Result: The decision I made not only resolved the immediate conflict but also led to a stronger sense of collaboration within the team. By valuing input from all team members and making a well-informed decision, we were able to achieve our project objectives efficiently.
4. Communication (Teamwork)

Situation: During a cross-functional project, miscommunication between departments was causing delays and misunderstandings.
Task: My task was to improve communication channels and foster better teamwork among team members.
Action: I initiated regular cross-departmental meetings to ensure that everyone was on the same page regarding project goals and timelines. I also implemented a centralized communication platform where team members could share updates, ask questions, and collaborate more effectively.
Result: Streamlining workflows and improving communication channels led to a 30% reduction in project completion time, saving the company $25,000 in operational costs.
5. Persistence
Situation: During a challenging sales quarter, I encountered numerous rejections and setbacks while trying to close a major client deal.
Task: My task was to persistently pursue the client and overcome obstacles to secure the deal.
Action: I maintained regular communication with the client, addressing their concerns and demonstrating the value proposition of our product. Despite facing multiple rejections, I remained persistent and resilient, adjusting my approach based on feedback and market dynamics.
Result: After months of perseverance, I successfully closed the deal with the client. By closing the major client deal, I exceeded quarterly sales targets by 25%, resulting in a revenue increase of $250,000 for the company.
Tips to Improve Your Problem-Solving Skills
Throughout your career, being able to showcase and effectively communicate your problem-solving skills gives you more leverage in achieving better jobs and earning more money .
So to improve your problem-solving skills, I recommend always analyzing a problem and situation before acting.
When discussing problem-solving with employers, you never want to sound like you rush or make impulsive decisions. They want to see fact-based or data-based decisions when you solve problems.
Don’t just say you’re good at solving problems. Show it with specifics. How much did you boost efficiency? Did you save the company money? Adding numbers can really make your achievements stand out.
To get better at solving problems, analyze the outcomes of past solutions you came up with. You can recognize what works and what doesn’t.
Think about how you can improve researching and analyzing a situation, how you can get better at communicating, and deciding on the right people in the organization to talk to and “pull in” to help you if needed, etc.
Finally, practice staying calm even in stressful situations. Take a few minutes to walk outside if needed. Step away from your phone and computer to clear your head. A work problem is rarely so urgent that you cannot take five minutes to think (with the possible exception of safety problems), and you’ll get better outcomes if you solve problems by acting logically instead of rushing to react in a panic.
You can use all of the ideas above to describe your problem-solving skills when asked interview questions about the topic. If you say that you do the things above, employers will be impressed when they assess your problem-solving ability.
More Interview Resources
- 3 Answers to “How Do You Handle Stress?”
- How to Answer “How Do You Handle Conflict?” (Interview Question)
- Sample Answers to “Tell Me About a Time You Failed”

About the Author
Biron Clark is a former executive recruiter who has worked individually with hundreds of job seekers, reviewed thousands of resumes and LinkedIn profiles, and recruited for top venture-backed startups and Fortune 500 companies. He has been advising job seekers since 2012 to think differently in their job search and land high-paying, competitive positions. Follow on Twitter and LinkedIn .
Read more articles by Biron Clark
About the Contributor
Kyle Elliott , career coach and mental health advocate, transforms his side hustle into a notable practice, aiding Silicon Valley professionals in maximizing potential. Follow Kyle on LinkedIn .

About the Editor
Hayley Jukes is the Editor-in-Chief at CareerSidekick with five years of experience creating engaging articles, books, and transcripts for diverse platforms and audiences.
Continue Reading
12 Expert-Approved Responses to ‘What Makes You Unique?’ in Job Interviews
15 most common pharmacist interview questions and answers, 15 most common paralegal interview questions and answers, top 30+ funny interview questions and answers, 60 hardest interview questions and answers, 100+ best ice breaker questions to ask candidates, top 20 situational interview questions (& sample answers), 15 most common physical therapist interview questions and answers.

- SUGGESTED TOPICS
- The Magazine
- Newsletters
- Managing Yourself
- Managing Teams
- Work-life Balance
- The Big Idea
- Data & Visuals
- Reading Lists
- Case Selections
- HBR Learning
- Topic Feeds
- Account Settings
- Email Preferences
Teams Solve Problems Faster When They’re More Cognitively Diverse
- Alison Reynolds
- David Lewis

Find people who disagree with you and cherish them.
Looking at the executive teams we work with as consultants and those we teach in the classroom, increased diversity of gender, ethnicity, and age is apparent. Over recent decades the rightful endeavor to achieve a more representative workforce has had an impact. Of course, there is a ways to go, but progress has been made.
- AR Alison Reynolds is a member of faculty at the UK’s Ashridge Business School where she works with executive groups in the field of leadership development, strategy execution and organization development. She has previously worked in the public sector and management consulting, and is an advisor to a number of small businesses and charities.
- DL David Lewis is Director of London Business School’s Senior Executive Programme and teaches on strategy execution and leading in uncertainty. He is a consultant and works with global corporations, advising and coaching board teams. He is co-founder of a research company focusing on developing tools to enhance individual, team and organization performance through better interaction.
Partner Center

How To Solve Complex Problems
In today’s increasingly complex world, we are constantly faced with ill-defined problems that don’t have a clear solution. From poverty and climate change to crime and addiction, complex situations surround us. Unlike simple problems with a pre-defined or “right” answer, complex problems share several basic characteristics that make them hard to solve. While these problems can be frustrating and overwhelming, they also offer an opportunity for growth and creativity. Complex problem-solving skills are the key to addressing these tough issues.
In this article, I will discuss simple versus complex problems, define complex problem solving, and describe why it is so important in complex dynamic environments. I will also explain how to develop problem-solving skills and share some tips for effectively solving complex problems.
How is simple problem-solving different from complex problem-solving?
Solving problems is about getting from a currently undesirable state to an intended goal state. In other words, about bridging the gap between “what is” and “what ought to be”. However, the challenge of reaching a solution varies based on the kind of problem that is being solved. There are generally three different kinds of problems you should consider.
Simple problems have one problem solution. The goal is to find that answer as quickly and efficiently as possible. Puzzles are classic examples of simple problem solving. The objective is to find the one correct solution out of many possibilities.
Problems are different from puzzles in that they don’t have a known problem solution. As such, many people may agree that there is an issue to be solved, but they may not agree on the intended goal state or how to get there. In this type of problem, people spend a lot of time debating the best solution and the optimal way to achieve it.
Messes are collections of interrelated problems where many stakeholders may not even agree on what the issue is. Unlike problems where there is agreement about what the problem is, in messes, there isn’t agreement amongst stakeholders. In other words, even “what is” can’t be taken for granted. Most complex social problems are messes, made up of interrelated social issues with ill-defined boundaries and goals.
Problems and messes can be complicated or complex
Puzzles are simple, but problems and messes exist on a continuum between complicated and complex. Complicated problems are technical in nature. There may be many involved variables, but the relationships are linear. As a result, complicated problems have step-by-step, systematic solutions. Repairing an engine or building a rocket may be difficult because of the many parts involved, but it is a technical problem we call complicated.
On the other hand, solving a complex problem is entirely different. Unlike complicated problems that may have many variables with linear relationships, a complex problem is characterized by connectivity patterns that are harder to understand and predict.
Characteristics of complex problems and messes
So what else makes a problem complex? Here are seven additional characteristics (from Funke and Hester and Adams ).
- Lack of information. There is often a lack of data or information about the problem itself. In some cases, variables are unknown or cannot be measured.
- Many goals. A complex problem has a mix of conflicting objectives. In some sense, every stakeholder involved with the problem may have their own goals. However, with limited resources, not all goals can be simultaneously satisfied.
- Unpredictable feedback loops. In part due to many variables connected by a range of different relationships, a change in one variable is likely to have effects on other variables in the system. However, because we do not know all of the variables it will affect, small changes can have disproportionate system-wide effects. These unexpected events that have big, unpredictable effects are sometimes called Black Swans.
- Dynamic. A complex problem changes over time and there is a significant impact based on when you act. In other words, because the problem and its parts and relationships are constantly changing, an action taken today won’t have the same effects as the same action taken tomorrow.
- Time-delayed. It takes a while for cause and effect to be realized. Thus it is very hard to know if any given intervention is working.
- Unknown unknowns. Building off the previous point about a lack of information, in a complex problem you may not even know what you don’t know. In other words, there may be very important variables that you are not even aware of.
- Affected by (error-prone) humans. Simply put, human behavior tends to be illogical and unpredictable. When humans are involved in a problem, avoiding error may be impossible.
What is complex problem-solving?
“Complex problem solving” is the term for how to address a complex problem or messes that have the characteristics listed above.
Since a complex problem is a different phenomenon than a simple or complicated problem, solving them requires a different approach. Methods designed for simple problems, like systematic organization, deductive logic, and linear thinking don’t work well on their own for a complex problem.
And yet, despite its importance, there isn’t complete agreement about what exactly it is.
How is complex problem solving defined by experts?
Let’s look at what scientists, researchers, and system thinkers have come up with in terms of a definition for solving a complex problem.
As a series of observations and informed decisions
For many employers, the focus is on making smart decisions. These must weigh the future effects to the company of any given solution. According to Indeed.com , it is defined as “a series of observations and informed decisions used to find and implement a solution to a problem. Beyond finding and implementing a solution, complex problem solving also involves considering future changes to circumstance, resources, and capabilities that may affect the trajectory of the process and success of the solution. Complex problem solving also involves considering the impact of the solution on the surrounding environment and individuals.”
As using information to review options and develop solutions
For others, it is more of a systematic way to consider a range of options. According to O*NET , the definition focuses on “identifying complex problems and reviewing related information to develop and evaluate options and implement solutions.”
As a self-regulated psychological process
Others emphasize the broad range of skills and emotions needed for change. In addition, they endorse an inspired kind of pragmatism. For example, Dietrich Dorner and Joachim Funke define it as “a collection of self-regulated psychological processes and activities necessary in dynamic environments to achieve ill-defined goals that cannot be reached by routine actions. Creative combinations of knowledge and a broad set of strategies are needed. Solutions are often more bricolage than perfect or optimal. The problem-solving process combines cognitive, emotional, and motivational aspects, particularly in high-stakes situations. Complex problems usually involve knowledge-rich requirements and collaboration among different persons.”
As a novel way of thinking and reasoning
Finally, some emphasize the multidisciplinary nature of knowledge and processes needed to tackle a complex problem. Patrick Hester and Kevin MacG. Adams have stated that “no single discipline can solve truly complex problems. Problems of real interest, those vexing ones that keep you up at night, require a discipline-agnostic approach…Simply they require us to think systemically about our problem…a novel way of thinking and reasoning about complex problems that encourages increased understanding and deliberate intervention.”
A synthesis definition
By pulling the main themes of these definitions together, we can get a sense of what complex problem-solvers must do:
Gain a better understanding of the phenomena of a complex problem or mess. Use a discipline-agnostic approach in order to develop deliberate interventions. Take into consideration future impacts on the surrounding environment.
Why is complex problem solving important?
Many efforts aimed at complex social problems like reducing homelessness and improving public health – despite good intentions giving more effort than ever before – are destined to fail because their approach is based on simple problem-solving. And some efforts might even unwittingly be contributing to the problems they’re trying to solve.
Einstein said that “We can’t solve problems by using the same kind of thinking we used when we created them.” I think he could have easily been alluding to the need for more complex problem solvers who think differently. So what skills are required to do this?
What are complex problem-solving skills?
The skills required to solve a complex problem aren’t from one domain, nor are they an easily-packaged bundle. Rather, I like to think of them as a balancing act between a series of seemingly opposite approaches but synthesized. This brings a sort of cognitive dissonance into the process, which is itself informative.
It brings F. Scott Fitzgerald’s maxim to mind:
“The test of a first-rate intelligence is the ability to hold two opposing ideas in mind at the same time and still retain the ability to function. One should, for example, be able to see that things are hopeless yet be determined to make them otherwise.”
To see the problem situation clearly, for example, but also with a sense of optimism and possibility.
Here are the top three dialectics to keep in mind:
Thinking and reasoning
Reasoning is the ability to make logical deductions based on evidence and counterevidence. On the other hand, thinking is more about imagining an unknown reality based on thoughts about the whole picture and how the parts could fit together. By thinking clearly, one can have a sense of possibility that prepares the mind to deduce the right action in the unique moment at hand.
As Dorner and Funke explain: “Not every situation requires the same action, and we may want to act this way or another to reach this or that goal. This appears logical, but it is a logic based on constantly shifting grounds: We cannot know whether necessary conditions are met, sometimes the assumptions we have made later turn out to be incorrect, and sometimes we have to revise our assumptions or make completely new ones. It is necessary to constantly switch between our sense of possibility and our sense of reality, that is, to switch between thinking and reasoning. It is an arduous process, and some people handle it well, while others do not.”
Analysis and reductionism combined with synthesis and holism
It’s important to be able to use scientific processes to break down a complex problem into its parts and analyze them. But at the same time, a complex problem is more than the sum of its parts. In most cases, the relationships between the parts are more important than the parts themselves. Therefore, decomposing problems with rigor isn’t enough. What’s needed, once problems are reduced and understood, is a way of understanding the relationships between various components as well as putting the pieces back together. However, synthesis and holism on their own without deductive analysis can often miss details and relationships that matter.
What makes this balancing act more difficult is that certain professions tend to be trained in and prefer one domain over the other. Scientists prefer analysis and reductionism whereas most social scientists and practitioners default to synthesis and holism. Unfortunately, this divide of preferences results in people working in their silos at the expense of multi-disciplinary approaches that together can better “see” complexity.
Situational awareness and self-awareness
Dual awareness is the ability to pay attention to two experiences simultaneously. In the case of complex problems, context really matters. In other words, problem-solving exists in an ecosystem of environmental factors that are not incidental. Personal and cultural preferences play a part as do current events unfolding over time. But as a problem solver, knowing the environment is only part of the equation.
The other crucial part is the internal psychological process unique to every individual who also interacts with the problem and the environment. Problem solvers inevitably come into contact with others who may disagree with them, or be advancing seemingly counterproductive solutions, and these interactions result in emotions and motivations. Without self-awareness, we can become attached to our own subjective opinions, fall in love with “our” solutions, and generally be driven by the desire to be seen as problem solvers at the expense of actually solving the problem.
By balancing these three dialectics, practitioners can better deal with uncertainty as well as stay motivated despite setbacks. Self-regulation among these seemingly opposite approaches also reminds one to stay open-minded.
How do you develop complex problem-solving skills?
There is no one answer to this question, as the best way to develop them will vary depending on your strengths and weaknesses. However, there are a few general things that you can do to improve your ability to solve problems.
Ground yourself in theory and knowledge
First, it is important to learn about systems thinking and complexity theories. These frameworks will help you understand how complex systems work, and how different parts of a system interact with each other. This conceptual understanding will allow you to identify potential solutions to problems more quickly and effectively.
Practice switching between approaches
Second, practice switching between the dialectics mentioned above. For example, in your next meeting try to spend roughly half your time thinking and half your time reasoning. The important part is trying to get habituated to regularly switching lenses. It may seem disjointed at first, but after a while, it becomes second nature to simultaneously see how the parts interact and the big picture.

Focus on the specific problem phenomena
Third, it may sound obvious, but people often don’t spend very much time studying the problem itself and how it functions. In some sense, becoming a good problem-solver involves becoming a problem scientist. Your time should be spent regularly investigating the phenomena of “what is” rather than “what ought to be”. A holistic understanding of the problem is the required prerequisite to coming up with good solutions.
Stay curious
Finally, after we have worked on a problem for a while, we tend to think we know everything about it, including how to solve it. Even if we’re working on a problem, which may change dynamically from day to day, we start treating it more like a puzzle with a definite solution. When that happens, we can lose our motivation to continue learning about the problem. This is very risky because it closes the door to learning from others, regardless of whether we completely agree with them or not.
As Neils Bohr said, “Two different perspectives or models about a system will reveal truths regarding the system that are neither entirely independent nor entirely compatible.”
By staying curious, we can retain our ability to learn on a daily basis.
Tips for how to solve complex problems
Focus on processes over results.
It’s easy to get lost in utopian thinking. Many people spend so much time on “what ought to be” that they forget that problem solving is about the gap between “what is” and “what ought to be”. It is said that “life is a journey, not a destination.” The same is true for complex problem-solving. To do it well, a problem solver must focus on enjoying the process of gaining a holistic understanding of the problem.
Adaptive and iterative methods and tools
A variety of adaptive and iterative methods have been developed to address complexity. They share a laser focus on gaining holistic understanding with tools that best match the phenomena of complexity. They are also non-ideological, trans-disciplinary, and flexible. In most cases, your journey through a set of steps won’t be linear. Rather, as you think and reason, analyze and synthesize, you’ll jump around to get a holistic picture.
In my online course , we generally follow a seven-step method:
- Get clear sight with a complex problem-solving frame
- Establish a secure base of operation
- Gain a deep understanding of the problem
- Create an interactive model of the problem
- Develop an impact strategy
- Create an action plan and implement
- Embed systemic solutions
Of course, each of these steps involves testing to see what works and consistently evaluating our process and progress.
Resolution is about systematically managing a problem over time
One last thing to keep in mind. Most social problems are not just solved one day, never to return. In reality, most complex problems are managed, not solved. For all practical purposes, what this means is that “the solution” is a way of systematically dealing with the problem over time. Some find this disappointing, but it’s actually a pragmatic pointer to think about resolution – a way move problems in the right direction – rather than final solutions.
Problem solvers regularly train and practice
If you need help developing your complex problem-solving skills, I have an online class where you can learn everything you need to know.
Sign up today and learn how to be successful at making a difference in the world!

What is Problem Solving? (Steps, Techniques, Examples)
By Status.net Editorial Team on May 7, 2023 — 5 minutes to read
What Is Problem Solving?
Definition and importance.
Problem solving is the process of finding solutions to obstacles or challenges you encounter in your life or work. It is a crucial skill that allows you to tackle complex situations, adapt to changes, and overcome difficulties with ease. Mastering this ability will contribute to both your personal and professional growth, leading to more successful outcomes and better decision-making.
Problem-Solving Steps
The problem-solving process typically includes the following steps:
- Identify the issue : Recognize the problem that needs to be solved.
- Analyze the situation : Examine the issue in depth, gather all relevant information, and consider any limitations or constraints that may be present.
- Generate potential solutions : Brainstorm a list of possible solutions to the issue, without immediately judging or evaluating them.
- Evaluate options : Weigh the pros and cons of each potential solution, considering factors such as feasibility, effectiveness, and potential risks.
- Select the best solution : Choose the option that best addresses the problem and aligns with your objectives.
- Implement the solution : Put the selected solution into action and monitor the results to ensure it resolves the issue.
- Review and learn : Reflect on the problem-solving process, identify any improvements or adjustments that can be made, and apply these learnings to future situations.
Defining the Problem
To start tackling a problem, first, identify and understand it. Analyzing the issue thoroughly helps to clarify its scope and nature. Ask questions to gather information and consider the problem from various angles. Some strategies to define the problem include:
- Brainstorming with others
- Asking the 5 Ws and 1 H (Who, What, When, Where, Why, and How)
- Analyzing cause and effect
- Creating a problem statement
Generating Solutions
Once the problem is clearly understood, brainstorm possible solutions. Think creatively and keep an open mind, as well as considering lessons from past experiences. Consider:
- Creating a list of potential ideas to solve the problem
- Grouping and categorizing similar solutions
- Prioritizing potential solutions based on feasibility, cost, and resources required
- Involving others to share diverse opinions and inputs
Evaluating and Selecting Solutions
Evaluate each potential solution, weighing its pros and cons. To facilitate decision-making, use techniques such as:
- SWOT analysis (Strengths, Weaknesses, Opportunities, Threats)
- Decision-making matrices
- Pros and cons lists
- Risk assessments
After evaluating, choose the most suitable solution based on effectiveness, cost, and time constraints.
Implementing and Monitoring the Solution
Implement the chosen solution and monitor its progress. Key actions include:
- Communicating the solution to relevant parties
- Setting timelines and milestones
- Assigning tasks and responsibilities
- Monitoring the solution and making adjustments as necessary
- Evaluating the effectiveness of the solution after implementation
Utilize feedback from stakeholders and consider potential improvements. Remember that problem-solving is an ongoing process that can always be refined and enhanced.
Problem-Solving Techniques
During each step, you may find it helpful to utilize various problem-solving techniques, such as:
- Brainstorming : A free-flowing, open-minded session where ideas are generated and listed without judgment, to encourage creativity and innovative thinking.
- Root cause analysis : A method that explores the underlying causes of a problem to find the most effective solution rather than addressing superficial symptoms.
- SWOT analysis : A tool used to evaluate the strengths, weaknesses, opportunities, and threats related to a problem or decision, providing a comprehensive view of the situation.
- Mind mapping : A visual technique that uses diagrams to organize and connect ideas, helping to identify patterns, relationships, and possible solutions.
Brainstorming
When facing a problem, start by conducting a brainstorming session. Gather your team and encourage an open discussion where everyone contributes ideas, no matter how outlandish they may seem. This helps you:
- Generate a diverse range of solutions
- Encourage all team members to participate
- Foster creative thinking
When brainstorming, remember to:
- Reserve judgment until the session is over
- Encourage wild ideas
- Combine and improve upon ideas
Root Cause Analysis
For effective problem-solving, identifying the root cause of the issue at hand is crucial. Try these methods:
- 5 Whys : Ask “why” five times to get to the underlying cause.
- Fishbone Diagram : Create a diagram representing the problem and break it down into categories of potential causes.
- Pareto Analysis : Determine the few most significant causes underlying the majority of problems.
SWOT Analysis
SWOT analysis helps you examine the Strengths, Weaknesses, Opportunities, and Threats related to your problem. To perform a SWOT analysis:
- List your problem’s strengths, such as relevant resources or strong partnerships.
- Identify its weaknesses, such as knowledge gaps or limited resources.
- Explore opportunities, like trends or new technologies, that could help solve the problem.
- Recognize potential threats, like competition or regulatory barriers.
SWOT analysis aids in understanding the internal and external factors affecting the problem, which can help guide your solution.
Mind Mapping
A mind map is a visual representation of your problem and potential solutions. It enables you to organize information in a structured and intuitive manner. To create a mind map:
- Write the problem in the center of a blank page.
- Draw branches from the central problem to related sub-problems or contributing factors.
- Add more branches to represent potential solutions or further ideas.
Mind mapping allows you to visually see connections between ideas and promotes creativity in problem-solving.
Examples of Problem Solving in Various Contexts
In the business world, you might encounter problems related to finances, operations, or communication. Applying problem-solving skills in these situations could look like:
- Identifying areas of improvement in your company’s financial performance and implementing cost-saving measures
- Resolving internal conflicts among team members by listening and understanding different perspectives, then proposing and negotiating solutions
- Streamlining a process for better productivity by removing redundancies, automating tasks, or re-allocating resources
In educational contexts, problem-solving can be seen in various aspects, such as:
- Addressing a gap in students’ understanding by employing diverse teaching methods to cater to different learning styles
- Developing a strategy for successful time management to balance academic responsibilities and extracurricular activities
- Seeking resources and support to provide equal opportunities for learners with special needs or disabilities
Everyday life is full of challenges that require problem-solving skills. Some examples include:
- Overcoming a personal obstacle, such as improving your fitness level, by establishing achievable goals, measuring progress, and adjusting your approach accordingly
- Navigating a new environment or city by researching your surroundings, asking for directions, or using technology like GPS to guide you
- Dealing with a sudden change, like a change in your work schedule, by assessing the situation, identifying potential impacts, and adapting your plans to accommodate the change.
- How to Resolve Employee Conflict at Work [Steps, Tips, Examples]
- How to Write Inspiring Core Values? 5 Steps with Examples
- 30 Employee Feedback Examples (Positive & Negative)
40 problem-solving techniques and processes
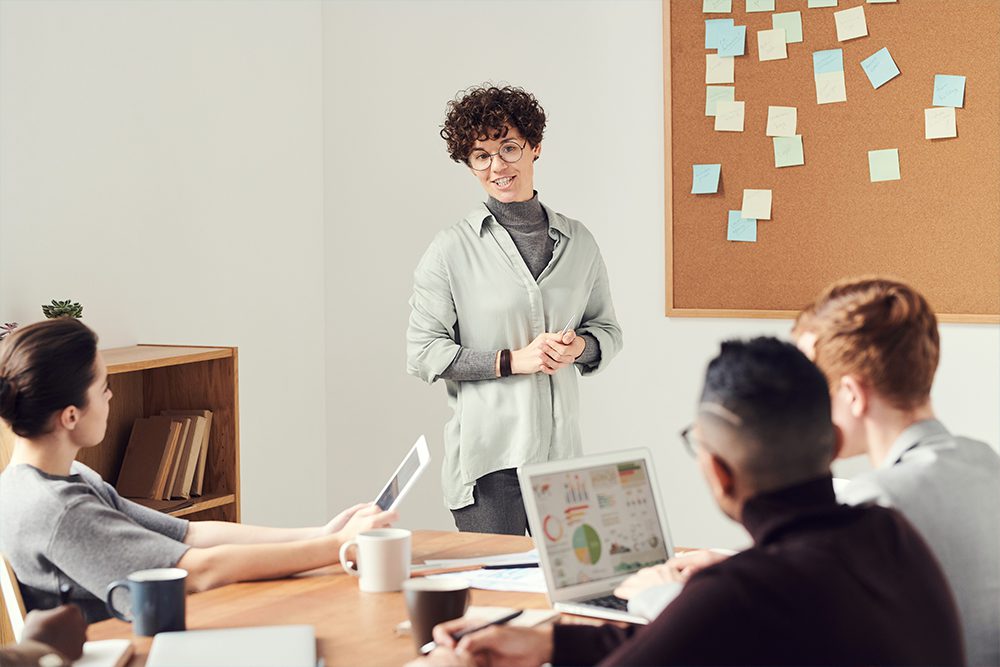
All teams and organizations encounter challenges. Approaching those challenges without a structured problem solving process can end up making things worse.
Proven problem solving techniques such as those outlined below can guide your group through a process of identifying problems and challenges , ideating on possible solutions , and then evaluating and implementing the most suitable .
In this post, you'll find problem-solving tools you can use to develop effective solutions. You'll also find some tips for facilitating the problem solving process and solving complex problems.
Design your next session with SessionLab
Join the 150,000+ facilitators using SessionLab.
Recommended Articles
A step-by-step guide to planning a workshop, 54 great online tools for workshops and meetings, how to create an unforgettable training session in 8 simple steps.
- 18 Free Facilitation Resources We Think You’ll Love
What is problem solving?
Problem solving is a process of finding and implementing a solution to a challenge or obstacle. In most contexts, this means going through a problem solving process that begins with identifying the issue, exploring its root causes, ideating and refining possible solutions before implementing and measuring the impact of that solution.
For simple or small problems, it can be tempting to skip straight to implementing what you believe is the right solution. The danger with this approach is that without exploring the true causes of the issue, it might just occur again or your chosen solution may cause other issues.
Particularly in the world of work, good problem solving means using data to back up each step of the process, bringing in new perspectives and effectively measuring the impact of your solution.
Effective problem solving can help ensure that your team or organization is well positioned to overcome challenges, be resilient to change and create innovation. In my experience, problem solving is a combination of skillset, mindset and process, and it’s especially vital for leaders to cultivate this skill.
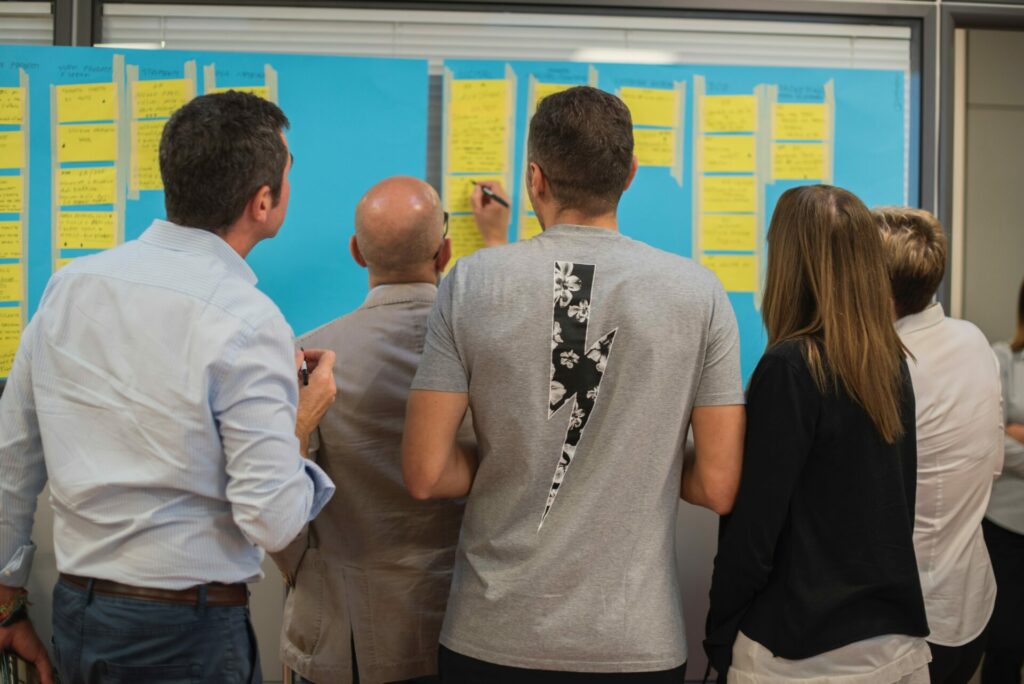
What is the seven step problem solving process?
A problem solving process is a step-by-step framework from going from discovering a problem all the way through to implementing a solution.
With practice, this framework can become intuitive, and innovative companies tend to have a consistent and ongoing ability to discover and tackle challenges when they come up.
You might see everything from a four step problem solving process through to seven steps. While all these processes cover roughly the same ground, I’ve found a seven step problem solving process is helpful for making all key steps legible.
We’ll outline that process here and then follow with techniques you can use to explore and work on that step of the problem solving process with a group.
The seven-step problem solving process is:
1. Problem identification
The first stage of any problem solving process is to identify the problem(s) you need to solve. This often looks like using group discussions and activities to help a group surface and effectively articulate the challenges they’re facing and wish to resolve.
Be sure to align with your team on the exact definition and nature of the problem you’re solving. An effective process is one where everyone is pulling in the same direction – ensure clarity and alignment now to help avoid misunderstandings later.
2. Problem analysis and refinement
The process of problem analysis means ensuring that the problem you are seeking to solve is the right problem . Choosing the right problem to solve means you are on the right path to creating the right solution.
At this stage, you may look deeper at the problem you identified to try and discover the root cause at the level of people or process. You may also spend some time sourcing data, consulting relevant parties and creating and refining a problem statement.
Problem refinement means adjusting scope or focus of the problem you will be aiming to solve based on what comes up during your analysis. As you analyze data sources, you might discover that the root cause means you need to adjust your problem statement. Alternatively, you might find that your original problem statement is too big to be meaningful approached within your current project.
Remember that the goal of any problem refinement is to help set the stage for effective solution development and deployment. Set the right focus and get buy-in from your team here and you’ll be well positioned to move forward with confidence.
3. Solution generation
Once your group has nailed down the particulars of the problem you wish to solve, you want to encourage a free flow of ideas connecting to solving that problem. This can take the form of problem solving games that encourage creative thinking or techniquess designed to produce working prototypes of possible solutions.
The key to ensuring the success of this stage of the problem solving process is to encourage quick, creative thinking and create an open space where all ideas are considered. The best solutions can often come from unlikely places and by using problem solving techniques that celebrate invention, you might come up with solution gold.
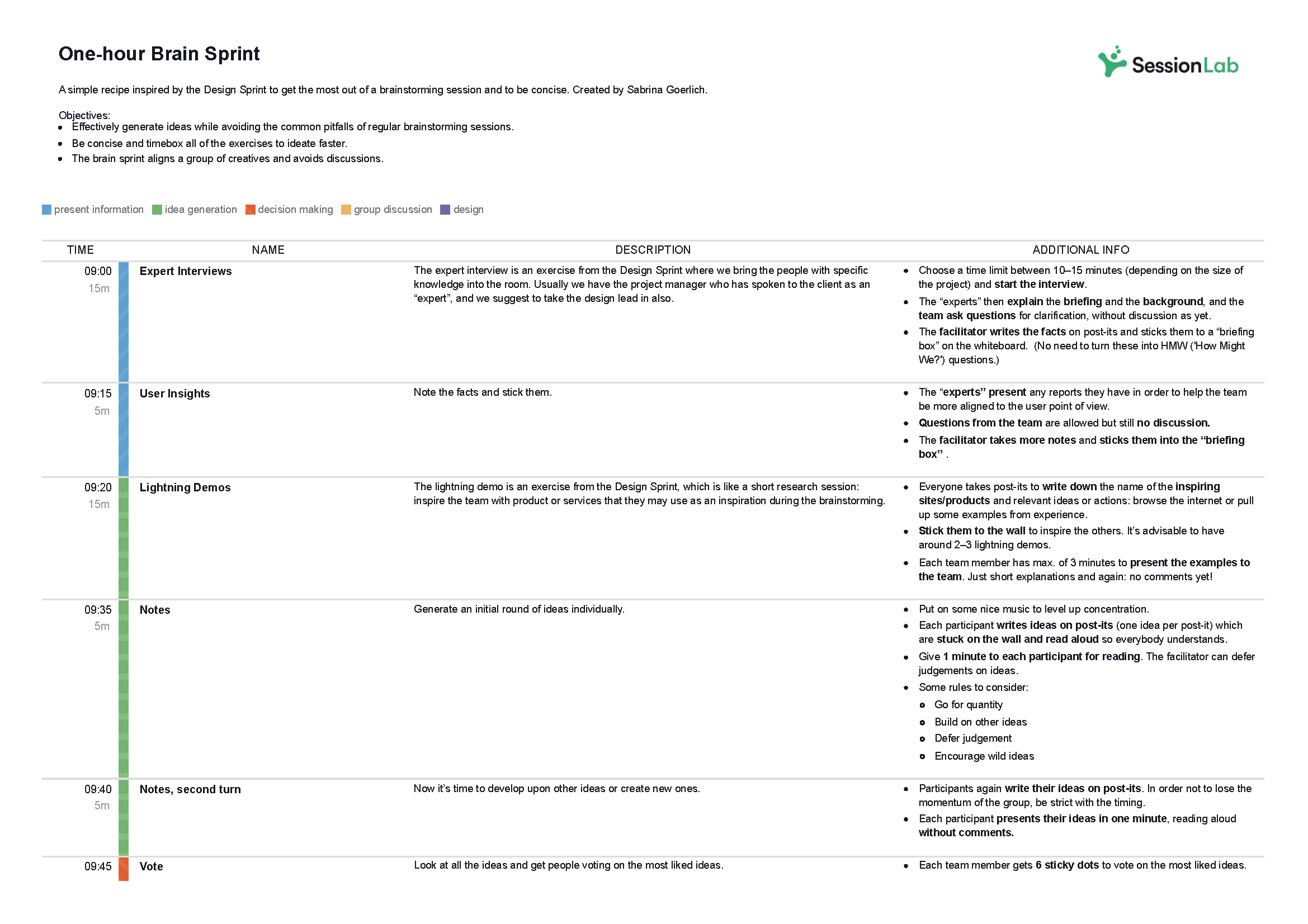
4. Solution development
No solution is perfect right out of the gate. It’s important to discuss and develop the solutions your group has come up with over the course of following the previous problem solving steps in order to arrive at the best possible solution. Problem solving games used in this stage involve lots of critical thinking, measuring potential effort and impact, and looking at possible solutions analytically.
During this stage, you will often ask your team to iterate and improve upon your front-running solutions and develop them further. Remember that problem solving strategies always benefit from a multitude of voices and opinions, and not to let ego get involved when it comes to choosing which solutions to develop and take further.
Finding the best solution is the goal of all problem solving workshops and here is the place to ensure that your solution is well thought out, sufficiently robust and fit for purpose.
5. Decision making and planning
Nearly there! Once you’ve got a set of possible, you’ll need to make a decision on which to implement. This can be a consensus-based group decision or it might be for a leader or major stakeholder to decide. You’ll find a set of effective decision making methods below.
Once your group has reached consensus and selected a solution, there are some additional actions that also need to be decided upon. You’ll want to work on allocating ownership of the project, figure out who will do what, how the success of the solution will be measured and decide the next course of action.
Set clear accountabilities, actions, timeframes, and follow-ups for your chosen solution. Make these decisions and set clear next-steps in the problem solving workshop so that everyone is aligned and you can move forward effectively as a group.
Ensuring that you plan for the roll-out of a solution is one of the most important problem solving steps. Without adequate planning or oversight, it can prove impossible to measure success or iterate further if the problem was not solved.
6. Solution implementation
This is what we were waiting for! All problem solving processes have the end goal of implementing an effective and impactful solution that your group has confidence in.
Project management and communication skills are key here – your solution may need to adjust when out in the wild or you might discover new challenges along the way. For some solutions, you might also implement a test with a small group and monitor results before rolling it out to an entire company.
You should have a clear owner for your solution who will oversee the plans you made together and help ensure they’re put into place. This person will often coordinate the implementation team and set-up processes to measure the efficacy of your solution too.
7. Solution evaluation
So you and your team developed a great solution to a problem and have a gut feeling it’s been solved. Work done, right? Wrong. All problem solving strategies benefit from evaluation, consideration, and feedback.
You might find that the solution does not work for everyone, might create new problems, or is potentially so successful that you will want to roll it out to larger teams or as part of other initiatives.
None of that is possible without taking the time to evaluate the success of the solution you developed in your problem solving model and adjust if necessary.
Remember that the problem solving process is often iterative and it can be common to not solve complex issues on the first try. Even when this is the case, you and your team will have generated learning that will be important for future problem solving workshops or in other parts of the organization.
It’s also worth underlining how important record keeping is throughout the problem solving process. If a solution didn’t work, you need to have the data and records to see why that was the case. If you go back to the drawing board, notes from the previous workshop can help save time.
What does an effective problem solving process look like?
Every effective problem solving process begins with an agenda . In our experience, a well-structured problem solving workshop is one of the best methods for successfully guiding a group from exploring a problem to implementing a solution.
The format of a workshop ensures that you can get buy-in from your group, encourage free-thinking and solution exploration before making a decision on what to implement following the session.
This Design Sprint 2.0 template is an effective problem solving process from top agency AJ&Smart. It’s a great format for the entire problem solving process, with four-days of workshops designed to surface issues, explore solutions and even test a solution.
Check it for an example of how you might structure and run a problem solving process and feel free to copy and adjust it your needs!
For a shorter process you can run in a single afternoon, this remote problem solving agenda will guide you effectively in just a couple of hours.
Whatever the length of your workshop, by using SessionLab, it’s easy to go from an idea to a complete agenda . Start by dragging and dropping your core problem solving activities into place . Add timings, breaks and necessary materials before sharing your agenda with your colleagues.
The resulting agenda will be your guide to an effective and productive problem solving session that will also help you stay organized on the day!
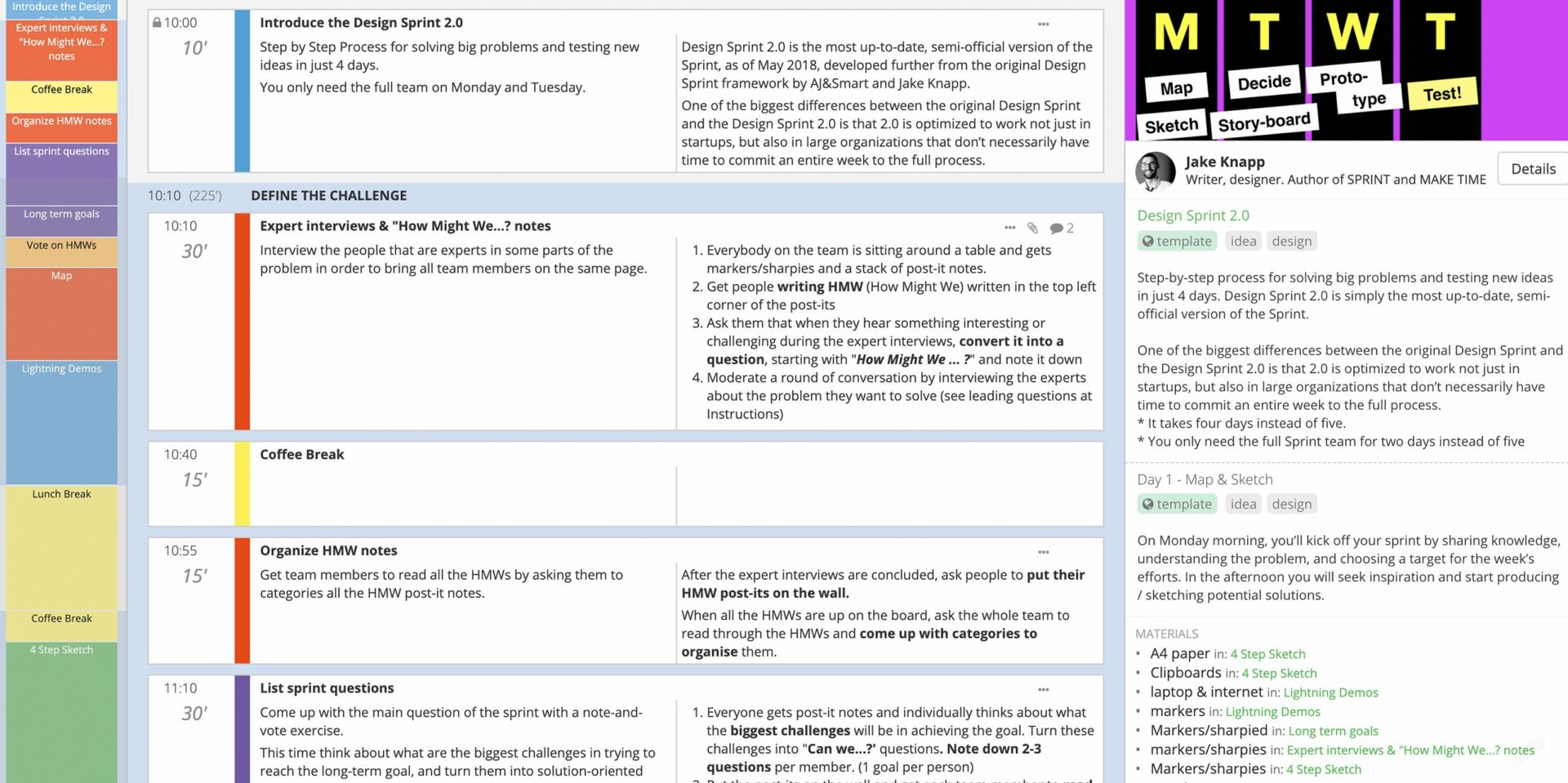
Complete problem-solving methods
In this section, we’ll look at in-depth problem-solving methods that provide a complete end-to-end process for developing effective solutions. These will help guide your team from the discovery and definition of a problem through to delivering the right solution.
If you’re looking for an all-encompassing method or problem-solving model, these processes are a great place to start. They’ll ask your team to challenge preconceived ideas and adopt a mindset for solving problems more effectively.
Six Thinking Hats
Individual approaches to solving a problem can be very different based on what team or role an individual holds. It can be easy for existing biases or perspectives to find their way into the mix, or for internal politics to direct a conversation.
Six Thinking Hats is a classic method for identifying the problems that need to be solved and enables your team to consider them from different angles, whether that is by focusing on facts and data, creative solutions, or by considering why a particular solution might not work.
Like all problem-solving frameworks, Six Thinking Hats is effective at helping teams remove roadblocks from a conversation or discussion and come to terms with all the aspects necessary to solve complex problems.
The Six Thinking Hats #creative thinking #meeting facilitation #problem solving #issue resolution #idea generation #conflict resolution The Six Thinking Hats are used by individuals and groups to separate out conflicting styles of thinking. They enable and encourage a group of people to think constructively together in exploring and implementing change, rather than using argument to fight over who is right and who is wrong.
Lightning Decision Jam
Featured courtesy of Jonathan Courtney of AJ&Smart Berlin, Lightning Decision Jam is one of those strategies that should be in every facilitation toolbox. Exploring problems and finding solutions is often creative in nature, though as with any creative process, there is the potential to lose focus and get lost.
Unstructured discussions might get you there in the end, but it’s much more effective to use a method that creates a clear process and team focus.
In Lightning Decision Jam, participants are invited to begin by writing challenges, concerns, or mistakes on post-its without discussing them before then being invited by the moderator to present them to the group.
From there, the team vote on which problems to solve and are guided through steps that will allow them to reframe those problems, create solutions and then decide what to execute on.
By deciding the problems that need to be solved as a team before moving on, this group process is great for ensuring the whole team is aligned and can take ownership over the next stages.
Lightning Decision Jam (LDJ) #action #decision making #problem solving #issue analysis #innovation #design #remote-friendly It doesn’t matter where you work and what your job role is, if you work with other people together as a team, you will always encounter the same challenges: Unclear goals and miscommunication that cause busy work and overtime Unstructured meetings that leave attendants tired, confused and without clear outcomes. Frustration builds up because internal challenges to productivity are not addressed Sudden changes in priorities lead to a loss of focus and momentum Muddled compromise takes the place of clear decision- making, leaving everybody to come up with their own interpretation. In short, a lack of structure leads to a waste of time and effort, projects that drag on for too long and frustrated, burnt out teams. AJ&Smart has worked with some of the most innovative, productive companies in the world. What sets their teams apart from others is not better tools, bigger talent or more beautiful offices. The secret sauce to becoming a more productive, more creative and happier team is simple: Replace all open discussion or brainstorming with a structured process that leads to more ideas, clearer decisions and better outcomes. When a good process provides guardrails and a clear path to follow, it becomes easier to come up with ideas, make decisions and solve problems. This is why AJ&Smart created Lightning Decision Jam (LDJ). It’s a simple and short, but powerful group exercise that can be run either in-person, in the same room, or remotely with distributed teams.
Problem Definition Process
While problems can be complex, the problem-solving methods you use to identify and solve those problems can often be simple in design.
By taking the time to truly identify and define a problem before asking the group to reframe the challenge as an opportunity, this method is a great way to enable change.
Begin by identifying a focus question and exploring the ways in which it manifests before splitting into five teams who will each consider the problem using a different method: escape, reversal, exaggeration, distortion or wishful. Teams develop a problem objective and create ideas in line with their method before then feeding them back to the group.
This method is great for enabling in-depth discussions while also creating space for finding creative solutions too!
Problem Definition #problem solving #idea generation #creativity #online #remote-friendly A problem solving technique to define a problem, challenge or opportunity and to generate ideas.
The 5 Whys
Sometimes, a group needs to go further with their strategies and analyze the root cause at the heart of organizational issues. An RCA or root cause analysis is the process of identifying what is at the heart of business problems or recurring challenges.
The 5 Whys is a simple and effective method of helping a group go find the root cause of any problem or challenge and conduct analysis that will deliver results.
By beginning with the creation of a problem statement and going through five stages to refine it, The 5 Whys provides everything you need to truly discover the cause of an issue.
The 5 Whys #hyperisland #innovation This simple and powerful method is useful for getting to the core of a problem or challenge. As the title suggests, the group defines a problems, then asks the question “why” five times, often using the resulting explanation as a starting point for creative problem solving.
World Cafe is a simple but powerful facilitation technique to help bigger groups to focus their energy and attention on solving complex problems.
World Cafe enables this approach by creating a relaxed atmosphere where participants are able to self-organize and explore topics relevant and important to them which are themed around a central problem-solving purpose. Create the right atmosphere by modeling your space after a cafe and after guiding the group through the method, let them take the lead!
Making problem-solving a part of your organization’s culture in the long term can be a difficult undertaking. More approachable formats like World Cafe can be especially effective in bringing people unfamiliar with workshops into the fold.
World Cafe #hyperisland #innovation #issue analysis World Café is a simple yet powerful method, originated by Juanita Brown, for enabling meaningful conversations driven completely by participants and the topics that are relevant and important to them. Facilitators create a cafe-style space and provide simple guidelines. Participants then self-organize and explore a set of relevant topics or questions for conversation.
Discovery & Action Dialogue (DAD)
One of the best approaches is to create a safe space for a group to share and discover practices and behaviors that can help them find their own solutions.
With DAD, you can help a group choose which problems they wish to solve and which approaches they will take to do so. It’s great at helping remove resistance to change and can help get buy-in at every level too!
This process of enabling frontline ownership is great in ensuring follow-through and is one of the methods you will want in your toolbox as a facilitator.
Discovery & Action Dialogue (DAD) #idea generation #liberating structures #action #issue analysis #remote-friendly DADs make it easy for a group or community to discover practices and behaviors that enable some individuals (without access to special resources and facing the same constraints) to find better solutions than their peers to common problems. These are called positive deviant (PD) behaviors and practices. DADs make it possible for people in the group, unit, or community to discover by themselves these PD practices. DADs also create favorable conditions for stimulating participants’ creativity in spaces where they can feel safe to invent new and more effective practices. Resistance to change evaporates as participants are unleashed to choose freely which practices they will adopt or try and which problems they will tackle. DADs make it possible to achieve frontline ownership of solutions.
Design Sprint 2.0
Want to see how a team can solve big problems and move forward with prototyping and testing solutions in a few days? The Design Sprint 2.0 template from Jake Knapp, author of Sprint, is a complete agenda for a with proven results.
Developing the right agenda can involve difficult but necessary planning. Ensuring all the correct steps are followed can also be stressful or time-consuming depending on your level of experience.
Use this complete 4-day workshop template if you are finding there is no obvious solution to your challenge and want to focus your team around a specific problem that might require a shortcut to launching a minimum viable product or waiting for the organization-wide implementation of a solution.
Open space technology
Open space technology- developed by Harrison Owen – creates a space where large groups are invited to take ownership of their problem solving and lead individual sessions. Open space technology is a great format when you have a great deal of expertise and insight in the room and want to allow for different takes and approaches on a particular theme or problem you need to be solved.
Start by bringing your participants together to align around a central theme and focus their efforts. Explain the ground rules to help guide the problem-solving process and then invite members to identify any issue connecting to the central theme that they are interested in and are prepared to take responsibility for.
Once participants have decided on their approach to the core theme, they write their issue on a piece of paper, announce it to the group, pick a session time and place, and post the paper on the wall. As the wall fills up with sessions, the group is then invited to join the sessions that interest them the most and which they can contribute to, then you’re ready to begin!
Everyone joins the problem-solving group they’ve signed up to, record the discussion and if appropriate, findings can then be shared with the rest of the group afterward.
Open Space Technology #action plan #idea generation #problem solving #issue analysis #large group #online #remote-friendly Open Space is a methodology for large groups to create their agenda discerning important topics for discussion, suitable for conferences, community gatherings and whole system facilitation
Techniques to identify and analyze problems
Using a problem-solving method to help a team identify and analyze a problem can be a quick and effective addition to any workshop or meeting.
While further actions are always necessary, you can generate momentum and alignment easily, and these activities are a great place to get started.
We’ve put together this list of techniques to help you and your team with problem identification, analysis, and discussion that sets the foundation for developing effective solutions.
Let’s take a look!
Fishbone Analysis
Organizational or team challenges are rarely simple, and it’s important to remember that one problem can be an indication of something that goes deeper and may require further consideration to be solved.
Fishbone Analysis helps groups to dig deeper and understand the origins of a problem. It’s a great example of a root cause analysis method that is simple for everyone on a team to get their head around.
Participants in this activity are asked to annotate a diagram of a fish, first adding the problem or issue to be worked on at the head of a fish before then brainstorming the root causes of the problem and adding them as bones on the fish.
Using abstractions such as a diagram of a fish can really help a team break out of their regular thinking and develop a creative approach.
Fishbone Analysis #problem solving ##root cause analysis #decision making #online facilitation A process to help identify and understand the origins of problems, issues or observations.
Problem Tree
Encouraging visual thinking can be an essential part of many strategies. By simply reframing and clarifying problems, a group can move towards developing a problem solving model that works for them.
In Problem Tree, groups are asked to first brainstorm a list of problems – these can be design problems, team problems or larger business problems – and then organize them into a hierarchy. The hierarchy could be from most important to least important or abstract to practical, though the key thing with problem solving games that involve this aspect is that your group has some way of managing and sorting all the issues that are raised.
Once you have a list of problems that need to be solved and have organized them accordingly, you’re then well-positioned for the next problem solving steps.
Problem tree #define intentions #create #design #issue analysis A problem tree is a tool to clarify the hierarchy of problems addressed by the team within a design project; it represents high level problems or related sublevel problems.
SWOT Analysis
Chances are you’ve heard of the SWOT Analysis before. This problem-solving method focuses on identifying strengths, weaknesses, opportunities, and threats is a tried and tested method for both individuals and teams.
Start by creating a desired end state or outcome and bare this in mind – any process solving model is made more effective by knowing what you are moving towards. Create a quadrant made up of the four categories of a SWOT analysis and ask participants to generate ideas based on each of those quadrants.
Once you have those ideas assembled in their quadrants, cluster them together based on their affinity with other ideas. These clusters are then used to facilitate group conversations and move things forward.
SWOT analysis #gamestorming #problem solving #action #meeting facilitation The SWOT Analysis is a long-standing technique of looking at what we have, with respect to the desired end state, as well as what we could improve on. It gives us an opportunity to gauge approaching opportunities and dangers, and assess the seriousness of the conditions that affect our future. When we understand those conditions, we can influence what comes next.
Agreement-Certainty Matrix
Not every problem-solving approach is right for every challenge, and deciding on the right method for the challenge at hand is a key part of being an effective team.
The Agreement Certainty matrix helps teams align on the nature of the challenges facing them. By sorting problems from simple to chaotic, your team can understand what methods are suitable for each problem and what they can do to ensure effective results.
If you are already using Liberating Structures techniques as part of your problem-solving strategy, the Agreement-Certainty Matrix can be an invaluable addition to your process. We’ve found it particularly if you are having issues with recurring problems in your organization and want to go deeper in understanding the root cause.
Agreement-Certainty Matrix #issue analysis #liberating structures #problem solving You can help individuals or groups avoid the frequent mistake of trying to solve a problem with methods that are not adapted to the nature of their challenge. The combination of two questions makes it possible to easily sort challenges into four categories: simple, complicated, complex , and chaotic . A problem is simple when it can be solved reliably with practices that are easy to duplicate. It is complicated when experts are required to devise a sophisticated solution that will yield the desired results predictably. A problem is complex when there are several valid ways to proceed but outcomes are not predictable in detail. Chaotic is when the context is too turbulent to identify a path forward. A loose analogy may be used to describe these differences: simple is like following a recipe, complicated like sending a rocket to the moon, complex like raising a child, and chaotic is like the game “Pin the Tail on the Donkey.” The Liberating Structures Matching Matrix in Chapter 5 can be used as the first step to clarify the nature of a challenge and avoid the mismatches between problems and solutions that are frequently at the root of chronic, recurring problems.
Organizing and charting a team’s progress can be important in ensuring its success. SQUID (Sequential Question and Insight Diagram) is a great model that allows a team to effectively switch between giving questions and answers and develop the skills they need to stay on track throughout the process.
Begin with two different colored sticky notes – one for questions and one for answers – and with your central topic (the head of the squid) on the board. Ask the group to first come up with a series of questions connected to their best guess of how to approach the topic. Ask the group to come up with answers to those questions, fix them to the board and connect them with a line. After some discussion, go back to question mode by responding to the generated answers or other points on the board.
It’s rewarding to see a diagram grow throughout the exercise, and a completed SQUID can provide a visual resource for future effort and as an example for other teams.
SQUID #gamestorming #project planning #issue analysis #problem solving When exploring an information space, it’s important for a group to know where they are at any given time. By using SQUID, a group charts out the territory as they go and can navigate accordingly. SQUID stands for Sequential Question and Insight Diagram.
To continue with our nautical theme, Speed Boat is a short and sweet activity that can help a team quickly identify what employees, clients or service users might have a problem with and analyze what might be standing in the way of achieving a solution.
Methods that allow for a group to make observations, have insights and obtain those eureka moments quickly are invaluable when trying to solve complex problems.
In Speed Boat, the approach is to first consider what anchors and challenges might be holding an organization (or boat) back. Bonus points if you are able to identify any sharks in the water and develop ideas that can also deal with competitors!
Speed Boat #gamestorming #problem solving #action Speedboat is a short and sweet way to identify what your employees or clients don’t like about your product/service or what’s standing in the way of a desired goal.
The Journalistic Six
Some of the most effective ways of solving problems is by encouraging teams to be more inclusive and diverse in their thinking.
Based on the six key questions journalism students are taught to answer in articles and news stories, The Journalistic Six helps create teams to see the whole picture. By using who, what, when, where, why, and how to facilitate the conversation and encourage creative thinking, your team can make sure that the problem identification and problem analysis stages of the are covered exhaustively and thoughtfully. Reporter’s notebook and dictaphone optional.
The Journalistic Six – Who What When Where Why How #idea generation #issue analysis #problem solving #online #creative thinking #remote-friendly A questioning method for generating, explaining, investigating ideas.
Individual and group perspectives are incredibly important, but what happens if people are set in their minds and need a change of perspective in order to approach a problem more effectively?
Flip It is a method we love because it is both simple to understand and run, and allows groups to understand how their perspectives and biases are formed.
Participants in Flip It are first invited to consider concerns, issues, or problems from a perspective of fear and write them on a flip chart. Then, the group is asked to consider those same issues from a perspective of hope and flip their understanding.
No problem and solution is free from existing bias and by changing perspectives with Flip It, you can then develop a problem solving model quickly and effectively.
Flip It! #gamestorming #problem solving #action Often, a change in a problem or situation comes simply from a change in our perspectives. Flip It! is a quick game designed to show players that perspectives are made, not born.
LEGO Challenge
Now for an activity that is a little out of the (toy) box. LEGO Serious Play is a facilitation methodology that can be used to improve creative thinking and problem-solving skills.
The LEGO Challenge includes giving each member of the team an assignment that is hidden from the rest of the group while they create a structure without speaking.
What the LEGO challenge brings to the table is a fun working example of working with stakeholders who might not be on the same page to solve problems. Also, it’s LEGO! Who doesn’t love LEGO!
LEGO Challenge #hyperisland #team A team-building activity in which groups must work together to build a structure out of LEGO, but each individual has a secret “assignment” which makes the collaborative process more challenging. It emphasizes group communication, leadership dynamics, conflict, cooperation, patience and problem solving strategy.
What, So What, Now What?
If not carefully managed, the problem identification and problem analysis stages of the problem-solving process can actually create more problems and misunderstandings.
The What, So What, Now What? problem-solving activity is designed to help collect insights and move forward while also eliminating the possibility of disagreement when it comes to identifying, clarifying, and analyzing organizational or work problems.
Facilitation is all about bringing groups together so that might work on a shared goal and the best problem-solving strategies ensure that teams are aligned in purpose, if not initially in opinion or insight.
Throughout the three steps of this game, you give everyone on a team to reflect on a problem by asking what happened, why it is important, and what actions should then be taken.
This can be a great activity for bringing our individual perceptions about a problem or challenge and contextualizing it in a larger group setting. This is one of the most important problem-solving skills you can bring to your organization.
W³ – What, So What, Now What? #issue analysis #innovation #liberating structures You can help groups reflect on a shared experience in a way that builds understanding and spurs coordinated action while avoiding unproductive conflict. It is possible for every voice to be heard while simultaneously sifting for insights and shaping new direction. Progressing in stages makes this practical—from collecting facts about What Happened to making sense of these facts with So What and finally to what actions logically follow with Now What . The shared progression eliminates most of the misunderstandings that otherwise fuel disagreements about what to do. Voila!
Journalists
Problem analysis can be one of the most important and decisive stages of all problem-solving tools. Sometimes, a team can become bogged down in the details and are unable to move forward.
Journalists is an activity that can avoid a group from getting stuck in the problem identification or problem analysis stages of the process.
In Journalists, the group is invited to draft the front page of a fictional newspaper and figure out what stories deserve to be on the cover and what headlines those stories will have. By reframing how your problems and challenges are approached, you can help a team move productively through the process and be better prepared for the steps to follow.
Journalists #vision #big picture #issue analysis #remote-friendly This is an exercise to use when the group gets stuck in details and struggles to see the big picture. Also good for defining a vision.
Problem-solving techniques for brainstorming solutions
Now you have the context and background of the problem you are trying to solving, now comes the time to start ideating and thinking about how you’ll solve the issue.
Here, you’ll want to encourage creative, free thinking and speed. Get as many ideas out as possible and explore different perspectives so you have the raw material for the next step.
Looking at a problem from a new angle can be one of the most effective ways of creating an effective solution. TRIZ is a problem-solving tool that asks the group to consider what they must not do in order to solve a challenge.
By reversing the discussion, new topics and taboo subjects often emerge, allowing the group to think more deeply and create ideas that confront the status quo in a safe and meaningful way. If you’re working on a problem that you’ve tried to solve before, TRIZ is a great problem-solving method to help your team get unblocked.
Making Space with TRIZ #issue analysis #liberating structures #issue resolution You can clear space for innovation by helping a group let go of what it knows (but rarely admits) limits its success and by inviting creative destruction. TRIZ makes it possible to challenge sacred cows safely and encourages heretical thinking. The question “What must we stop doing to make progress on our deepest purpose?” induces seriously fun yet very courageous conversations. Since laughter often erupts, issues that are otherwise taboo get a chance to be aired and confronted. With creative destruction come opportunities for renewal as local action and innovation rush in to fill the vacuum. Whoosh!
Mindspin
Brainstorming is part of the bread and butter of the problem-solving process and all problem-solving strategies benefit from getting ideas out and challenging a team to generate solutions quickly.
With Mindspin, participants are encouraged not only to generate ideas but to do so under time constraints and by slamming down cards and passing them on. By doing multiple rounds, your team can begin with a free generation of possible solutions before moving on to developing those solutions and encouraging further ideation.
This is one of our favorite problem-solving activities and can be great for keeping the energy up throughout the workshop. Remember the importance of helping people become engaged in the process – energizing problem-solving techniques like Mindspin can help ensure your team stays engaged and happy, even when the problems they’re coming together to solve are complex.
MindSpin #teampedia #idea generation #problem solving #action A fast and loud method to enhance brainstorming within a team. Since this activity has more than round ideas that are repetitive can be ruled out leaving more creative and innovative answers to the challenge.
The Creativity Dice
One of the most useful problem solving skills you can teach your team is of approaching challenges with creativity, flexibility, and openness. Games like The Creativity Dice allow teams to overcome the potential hurdle of too much linear thinking and approach the process with a sense of fun and speed.
In The Creativity Dice, participants are organized around a topic and roll a dice to determine what they will work on for a period of 3 minutes at a time. They might roll a 3 and work on investigating factual information on the chosen topic. They might roll a 1 and work on identifying the specific goals, standards, or criteria for the session.
Encouraging rapid work and iteration while asking participants to be flexible are great skills to cultivate. Having a stage for idea incubation in this game is also important. Moments of pause can help ensure the ideas that are put forward are the most suitable.
The Creativity Dice #creativity #problem solving #thiagi #issue analysis Too much linear thinking is hazardous to creative problem solving. To be creative, you should approach the problem (or the opportunity) from different points of view. You should leave a thought hanging in mid-air and move to another. This skipping around prevents premature closure and lets your brain incubate one line of thought while you consciously pursue another.
Idea and Concept Development
Brainstorming without structure can quickly become chaotic or frustrating. In a problem-solving context, having an ideation framework to follow can help ensure your team is both creative and disciplined.
In this method, you’ll find an idea generation process that encourages your group to brainstorm effectively before developing their ideas and begin clustering them together. By using concepts such as Yes and…, more is more and postponing judgement, you can create the ideal conditions for brainstorming with ease.
Idea & Concept Development #hyperisland #innovation #idea generation Ideation and Concept Development is a process for groups to work creatively and collaboratively to generate creative ideas. It’s a general approach that can be adapted and customized to suit many different scenarios. It includes basic principles for idea generation and several steps for groups to work with. It also includes steps for idea selection and development.
Problem-solving techniques for developing and refining solutions
The success of any problem-solving process can be measured by the solutions it produces. After you’ve defined the issue, explored existing ideas, and ideated, it’s time to develop and refine your ideas in order to bring them closer to a solution that actually solves the problem.
Use these problem-solving techniques when you want to help your team think through their ideas and refine them as part of your problem solving process.
Improved Solutions
After a team has successfully identified a problem and come up with a few solutions, it can be tempting to call the work of the problem-solving process complete. That said, the first solution is not necessarily the best, and by including a further review and reflection activity into your problem-solving model, you can ensure your group reaches the best possible result.
One of a number of problem-solving games from Thiagi Group, Improved Solutions helps you go the extra mile and develop suggested solutions with close consideration and peer review. By supporting the discussion of several problems at once and by shifting team roles throughout, this problem-solving technique is a dynamic way of finding the best solution.
Improved Solutions #creativity #thiagi #problem solving #action #team You can improve any solution by objectively reviewing its strengths and weaknesses and making suitable adjustments. In this creativity framegame, you improve the solutions to several problems. To maintain objective detachment, you deal with a different problem during each of six rounds and assume different roles (problem owner, consultant, basher, booster, enhancer, and evaluator) during each round. At the conclusion of the activity, each player ends up with two solutions to her problem.
Four Step Sketch
Creative thinking and visual ideation does not need to be confined to the opening stages of your problem-solving strategies. Exercises that include sketching and prototyping on paper can be effective at the solution finding and development stage of the process, and can be great for keeping a team engaged.
By going from simple notes to a crazy 8s round that involves rapidly sketching 8 variations on their ideas before then producing a final solution sketch, the group is able to iterate quickly and visually. Problem-solving techniques like Four-Step Sketch are great if you have a group of different thinkers and want to change things up from a more textual or discussion-based approach.
Four-Step Sketch #design sprint #innovation #idea generation #remote-friendly The four-step sketch is an exercise that helps people to create well-formed concepts through a structured process that includes: Review key information Start design work on paper, Consider multiple variations , Create a detailed solution . This exercise is preceded by a set of other activities allowing the group to clarify the challenge they want to solve. See how the Four Step Sketch exercise fits into a Design Sprint
Ensuring that everyone in a group is able to contribute to a discussion is vital during any problem solving process. Not only does this ensure all bases are covered, but its then easier to get buy-in and accountability when people have been able to contribute to the process.
1-2-4-All is a tried and tested facilitation technique where participants are asked to first brainstorm on a topic on their own. Next, they discuss and share ideas in a pair before moving into a small group. Those groups are then asked to present the best idea from their discussion to the rest of the team.
This method can be used in many different contexts effectively, though I find it particularly shines in the idea development stage of the process. Giving each participant time to concretize their ideas and develop them in progressively larger groups can create a great space for both innovation and psychological safety.
1-2-4-All #idea generation #liberating structures #issue analysis With this facilitation technique you can immediately include everyone regardless of how large the group is. You can generate better ideas and more of them faster than ever before. You can tap the know-how and imagination that is distributed widely in places not known in advance. Open, generative conversation unfolds. Ideas and solutions are sifted in rapid fashion. Most importantly, participants own the ideas, so follow-up and implementation is simplified. No buy-in strategies needed! Simple and elegant!
15% Solutions
Some problems are simpler than others and with the right problem-solving activities, you can empower people to take immediate actions that can help create organizational change.
Part of the liberating structures toolkit, 15% solutions is a problem-solving technique that focuses on finding and implementing solutions quickly. A process of iterating and making small changes quickly can help generate momentum and an appetite for solving complex problems.
Problem-solving strategies can live and die on whether people are onboard. Getting some quick wins is a great way of getting people behind the process.
It can be extremely empowering for a team to realize that problem-solving techniques can be deployed quickly and easily and delineate between things they can positively impact and those things they cannot change.
15% Solutions #action #liberating structures #remote-friendly You can reveal the actions, however small, that everyone can do immediately. At a minimum, these will create momentum, and that may make a BIG difference. 15% Solutions show that there is no reason to wait around, feel powerless, or fearful. They help people pick it up a level. They get individuals and the group to focus on what is within their discretion instead of what they cannot change. With a very simple question, you can flip the conversation to what can be done and find solutions to big problems that are often distributed widely in places not known in advance. Shifting a few grains of sand may trigger a landslide and change the whole landscape.
Problem-solving techniques for making decisions and planning
After your group is happy with the possible solutions you’ve developed, now comes the time to choose which to implement. There’s more than one way to make a decision and the best option is often dependant on the needs and set-up of your group.
Sometimes, it’s the case that you’ll want to vote as a group on what is likely to be the most impactful solution. Other times, it might be down to a decision maker or major stakeholder to make the final decision. Whatever your process, here’s some techniques you can use to help you make a decision during your problem solving process.
How-Now-Wow Matrix
The problem-solving process is often creative, as complex problems usually require a change of thinking and creative response in order to find the best solutions. While it’s common for the first stages to encourage creative thinking, groups can often gravitate to familiar solutions when it comes to the end of the process.
When selecting solutions, you don’t want to lose your creative energy! The How-Now-Wow Matrix from Gamestorming is a great problem-solving activity that enables a group to stay creative and think out of the box when it comes to selecting the right solution for a given problem.
Problem-solving techniques that encourage creative thinking and the ideation and selection of new solutions can be the most effective in organisational change. Give the How-Now-Wow Matrix a go, and not just for how pleasant it is to say out loud.
How-Now-Wow Matrix #gamestorming #idea generation #remote-friendly When people want to develop new ideas, they most often think out of the box in the brainstorming or divergent phase. However, when it comes to convergence, people often end up picking ideas that are most familiar to them. This is called a ‘creative paradox’ or a ‘creadox’. The How-Now-Wow matrix is an idea selection tool that breaks the creadox by forcing people to weigh each idea on 2 parameters.
Impact and Effort Matrix
All problem-solving techniques hope to not only find solutions to a given problem or challenge but to find the best solution. When it comes to finding a solution, groups are invited to put on their decision-making hats and really think about how a proposed idea would work in practice.
The Impact and Effort Matrix is one of the problem-solving techniques that fall into this camp, empowering participants to first generate ideas and then categorize them into a 2×2 matrix based on impact and effort.
Activities that invite critical thinking while remaining simple are invaluable. Use the Impact and Effort Matrix to move from ideation and towards evaluating potential solutions before then committing to them.
Impact and Effort Matrix #gamestorming #decision making #action #remote-friendly In this decision-making exercise, possible actions are mapped based on two factors: effort required to implement and potential impact. Categorizing ideas along these lines is a useful technique in decision making, as it obliges contributors to balance and evaluate suggested actions before committing to them.
If you’ve followed each of the problem-solving steps with your group successfully, you should move towards the end of your process with heaps of possible solutions developed with a specific problem in mind. But how do you help a group go from ideation to putting a solution into action?
Dotmocracy – or Dot Voting -is a tried and tested method of helping a team in the problem-solving process make decisions and put actions in place with a degree of oversight and consensus.
One of the problem-solving techniques that should be in every facilitator’s toolbox, Dot Voting is fast and effective and can help identify the most popular and best solutions and help bring a group to a decision effectively.
Dotmocracy #action #decision making #group prioritization #hyperisland #remote-friendly Dotmocracy is a simple method for group prioritization or decision-making. It is not an activity on its own, but a method to use in processes where prioritization or decision-making is the aim. The method supports a group to quickly see which options are most popular or relevant. The options or ideas are written on post-its and stuck up on a wall for the whole group to see. Each person votes for the options they think are the strongest, and that information is used to inform a decision.
Straddling the gap between decision making and planning, MoSCoW is a simple and effective method that allows a group team to easily prioritize a set of possible options.
Use this method in a problem solving process by collecting and summarizing all your possible solutions and then categorize them into 4 sections: “Must have”, “Should have”, “Could have”, or “Would like but won‘t get”.
This method is particularly useful when its less about choosing one possible solution and more about prioritorizing which to do first and which may not fit in the scope of your project. In my experience, complex challenges often require multiple small fixes, and this method can be a great way to move from a pile of things you’d all like to do to a structured plan.
MoSCoW #define intentions #create #design #action #remote-friendly MoSCoW is a method that allows the team to prioritize the different features that they will work on. Features are then categorized into “Must have”, “Should have”, “Could have”, or “Would like but won‘t get”. To be used at the beginning of a timeslot (for example during Sprint planning) and when planning is needed.
When it comes to managing the rollout of a solution, clarity and accountability are key factors in ensuring the success of the project. The RAACI chart is a simple but effective model for setting roles and responsibilities as part of a planning session.
Start by listing each person involved in the project and put them into the following groups in order to make it clear who is responsible for what during the rollout of your solution.
- Responsibility (Which person and/or team will be taking action?)
- Authority (At what “point” must the responsible person check in before going further?)
- Accountability (Who must the responsible person check in with?)
- Consultation (Who must be consulted by the responsible person before decisions are made?)
- Information (Who must be informed of decisions, once made?)
Ensure this information is easily accessible and use it to inform who does what and who is looped into discussions and kept up to date.
RAACI #roles and responsibility #teamwork #project management Clarifying roles and responsibilities, levels of autonomy/latitude in decision making, and levels of engagement among diverse stakeholders.
Problem-solving warm-up activities
All facilitators know that warm-ups and icebreakers are useful for any workshop or group process. Problem-solving workshops are no different.
Use these problem-solving techniques to warm up a group and prepare them for the rest of the process. Activating your group by tapping into some of the top problem-solving skills can be one of the best ways to see great outcomes from your session.
Check-in / Check-out
Solid processes are planned from beginning to end, and the best facilitators know that setting the tone and establishing a safe, open environment can be integral to a successful problem-solving process. Check-in / Check-out is a great way to begin and/or bookend a problem-solving workshop. Checking in to a session emphasizes that everyone will be seen, heard, and expected to contribute.
If you are running a series of meetings, setting a consistent pattern of checking in and checking out can really help your team get into a groove. We recommend this opening-closing activity for small to medium-sized groups though it can work with large groups if they’re disciplined!
Check-in / Check-out #team #opening #closing #hyperisland #remote-friendly Either checking-in or checking-out is a simple way for a team to open or close a process, symbolically and in a collaborative way. Checking-in/out invites each member in a group to be present, seen and heard, and to express a reflection or a feeling. Checking-in emphasizes presence, focus and group commitment; checking-out emphasizes reflection and symbolic closure.
Doodling Together
Thinking creatively and not being afraid to make suggestions are important problem-solving skills for any group or team, and warming up by encouraging these behaviors is a great way to start.
Doodling Together is one of our favorite creative ice breaker games – it’s quick, effective, and fun and can make all following problem-solving steps easier by encouraging a group to collaborate visually. By passing cards and adding additional items as they go, the workshop group gets into a groove of co-creation and idea development that is crucial to finding solutions to problems.
Doodling Together #collaboration #creativity #teamwork #fun #team #visual methods #energiser #icebreaker #remote-friendly Create wild, weird and often funny postcards together & establish a group’s creative confidence.
Show and Tell
You might remember some version of Show and Tell from being a kid in school and it’s a great problem-solving activity to kick off a session.
Asking participants to prepare a little something before a workshop by bringing an object for show and tell can help them warm up before the session has even begun! Games that include a physical object can also help encourage early engagement before moving onto more big-picture thinking.
By asking your participants to tell stories about why they chose to bring a particular item to the group, you can help teams see things from new perspectives and see both differences and similarities in the way they approach a topic. Great groundwork for approaching a problem-solving process as a team!
Show and Tell #gamestorming #action #opening #meeting facilitation Show and Tell taps into the power of metaphors to reveal players’ underlying assumptions and associations around a topic The aim of the game is to get a deeper understanding of stakeholders’ perspectives on anything—a new project, an organizational restructuring, a shift in the company’s vision or team dynamic.
Constellations
Who doesn’t love stars? Constellations is a great warm-up activity for any workshop as it gets people up off their feet, energized, and ready to engage in new ways with established topics. It’s also great for showing existing beliefs, biases, and patterns that can come into play as part of your session.
Using warm-up games that help build trust and connection while also allowing for non-verbal responses can be great for easing people into the problem-solving process and encouraging engagement from everyone in the group. Constellations is great in large spaces that allow for movement and is definitely a practical exercise to allow the group to see patterns that are otherwise invisible.
Constellations #trust #connection #opening #coaching #patterns #system Individuals express their response to a statement or idea by standing closer or further from a central object. Used with teams to reveal system, hidden patterns, perspectives.
Draw a Tree
Problem-solving games that help raise group awareness through a central, unifying metaphor can be effective ways to warm-up a group in any problem-solving model.
Draw a Tree is a simple warm-up activity you can use in any group and which can provide a quick jolt of energy. Start by asking your participants to draw a tree in just 45 seconds – they can choose whether it will be abstract or realistic.
Once the timer is up, ask the group how many people included the roots of the tree and use this as a means to discuss how we can ignore important parts of any system simply because they are not visible.
All problem-solving strategies are made more effective by thinking of problems critically and by exposing things that may not normally come to light. Warm-up games like Draw a Tree are great in that they quickly demonstrate some key problem-solving skills in an accessible and effective way.
Draw a Tree #thiagi #opening #perspectives #remote-friendly With this game you can raise awarness about being more mindful, and aware of the environment we live in.
Closing activities for a problem-solving process
Each step of the problem-solving workshop benefits from an intelligent deployment of activities, games, and techniques. Bringing your session to an effective close helps ensure that solutions are followed through on and that you also celebrate what has been achieved.
Here are some problem-solving activities you can use to effectively close a workshop or meeting and ensure the great work you’ve done can continue afterward.
One Breath Feedback
Maintaining attention and focus during the closing stages of a problem-solving workshop can be tricky and so being concise when giving feedback can be important. It’s easy to incur “death by feedback” should some team members go on for too long sharing their perspectives in a quick feedback round.
One Breath Feedback is a great closing activity for workshops. You give everyone an opportunity to provide feedback on what they’ve done but only in the space of a single breath. This keeps feedback short and to the point and means that everyone is encouraged to provide the most important piece of feedback to them.
One breath feedback #closing #feedback #action This is a feedback round in just one breath that excels in maintaining attention: each participants is able to speak during just one breath … for most people that’s around 20 to 25 seconds … unless of course you’ve been a deep sea diver in which case you’ll be able to do it for longer.
Who What When Matrix
Matrices feature as part of many effective problem-solving strategies and with good reason. They are easily recognizable, simple to use, and generate results.
The Who What When Matrix is a great tool to use when closing your problem-solving session by attributing a who, what and when to the actions and solutions you have decided upon. The resulting matrix is a simple, easy-to-follow way of ensuring your team can move forward.
Great solutions can’t be enacted without action and ownership. Your problem-solving process should include a stage for allocating tasks to individuals or teams and creating a realistic timeframe for those solutions to be implemented or checked out. Use this method to keep the solution implementation process clear and simple for all involved.
Who/What/When Matrix #gamestorming #action #project planning With Who/What/When matrix, you can connect people with clear actions they have defined and have committed to.
Response cards
Group discussion can comprise the bulk of most problem-solving activities and by the end of the process, you might find that your team is talked out!
Providing a means for your team to give feedback with short written notes can ensure everyone is head and can contribute without the need to stand up and talk. Depending on the needs of the group, giving an alternative can help ensure everyone can contribute to your problem-solving model in the way that makes the most sense for them.
Response Cards is a great way to close a workshop if you are looking for a gentle warm-down and want to get some swift discussion around some of the feedback that is raised.
Response Cards #debriefing #closing #structured sharing #questions and answers #thiagi #action It can be hard to involve everyone during a closing of a session. Some might stay in the background or get unheard because of louder participants. However, with the use of Response Cards, everyone will be involved in providing feedback or clarify questions at the end of a session.
Tips for effective problem solving
Problem-solving activities are only one part of the puzzle. While a great method can help unlock your team’s ability to solve problems, without a thoughtful approach and strong facilitation the solutions may not be fit for purpose.
Let’s take a look at some problem-solving tips you can apply to any process to help it be a success!
Clearly define the problem
Jumping straight to solutions can be tempting, though without first clearly articulating a problem, the solution might not be the right one. Many of the problem-solving activities below include sections where the problem is explored and clearly defined before moving on.
This is a vital part of the problem-solving process and taking the time to fully define an issue can save time and effort later. A clear definition helps identify irrelevant information and it also ensures that your team sets off on the right track.
Don’t jump to conclusions
It’s easy for groups to exhibit cognitive bias or have preconceived ideas about both problems and potential solutions. Be sure to back up any problem statements or potential solutions with facts, research, and adequate forethought.
The best techniques ask participants to be methodical and challenge preconceived notions. Make sure you give the group enough time and space to collect relevant information and consider the problem in a new way. By approaching the process with a clear, rational mindset, you’ll often find that better solutions are more forthcoming.
Try different approaches
Problems come in all shapes and sizes and so too should the methods you use to solve them. If you find that one approach isn’t yielding results and your team isn’t finding different solutions, try mixing it up. You’ll be surprised at how using a new creative activity can unblock your team and generate great solutions.
Don’t take it personally
Depending on the nature of your team or organizational problems, it’s easy for conversations to get heated. While it’s good for participants to be engaged in the discussions, ensure that emotions don’t run too high and that blame isn’t thrown around while finding solutions.
You’re all in it together, and even if your team or area is seeing problems, that isn’t necessarily a disparagement of you personally. Using facilitation skills to manage group dynamics is one effective method of helping conversations be more constructive.
Get the right people in the room
Your problem-solving method is often only as effective as the group using it. Getting the right people on the job and managing the number of people present is important too!
If the group is too small, you may not get enough different perspectives to effectively solve a problem. If the group is too large, you can go round and round during the ideation stages.
Creating the right group makeup is also important in ensuring you have the necessary expertise and skillset to both identify and follow up on potential solutions. Carefully consider who to include at each stage to help ensure your problem-solving method is followed and positioned for success.
Create psychologically safe spaces for discussion
Identifying a problem accurately also requires that all members of a group are able to contribute their views in an open and safe manner.
It can be tough for people to stand up and contribute if the problems or challenges are emotive or personal in nature. Try and create a psychologically safe space for these kinds of discussions and where possible, create regular opportunities for challenges to be brought up organically.
Document everything
The best solutions can take refinement, iteration, and reflection to come out. Get into a habit of documenting your process in order to keep all the learnings from the session and to allow ideas to mature and develop. Many of the methods below involve the creation of documents or shared resources. Be sure to keep and share these so everyone can benefit from the work done!
Bring a facilitator
Facilitation is all about making group processes easier. With a subject as potentially emotive and important as problem-solving, having an impartial third party in the form of a facilitator can make all the difference in finding great solutions and keeping the process moving. Consider bringing a facilitator to your problem-solving session to get better results and generate meaningful solutions!
Develop your problem-solving skills
It takes time and practice to be an effective problem solver. While some roles or participants might more naturally gravitate towards problem-solving, it can take development and planning to help everyone create better solutions.
You might develop a training program, run a problem-solving workshop or simply ask your team to practice using the techniques below. Check out our post on problem-solving skills to see how you and your group can develop the right mental process and be more resilient to issues too!
Design a great agenda
Workshops are a great format for solving problems. With the right approach, you can focus a group and help them find the solutions to their own problems. But designing a process can be time-consuming and finding the right activities can be difficult.
Check out our workshop planning guide to level-up your agenda design and start running more effective workshops. Need inspiration? Check out templates designed by expert facilitators to help you kickstart your process!
Save time and effort creating an effective problem solving process
A structured problem solving process is a surefire way of solving tough problems, discovering creative solutions and driving organizational change. But how can you design for successful outcomes?
With SessionLab, it’s easy to design engaging workshops that deliver results. Drag, drop and reorder blocks to build your agenda. When you make changes or update your agenda, your session timing adjusts automatically , saving you time on manual adjustments.
Collaborating with stakeholders or clients? Share your agenda with a single click and collaborate in real-time. No more sending documents back and forth over email.
Explore how to use SessionLab to design effective problem solving workshops or watch this five minute video to see the planner in action!
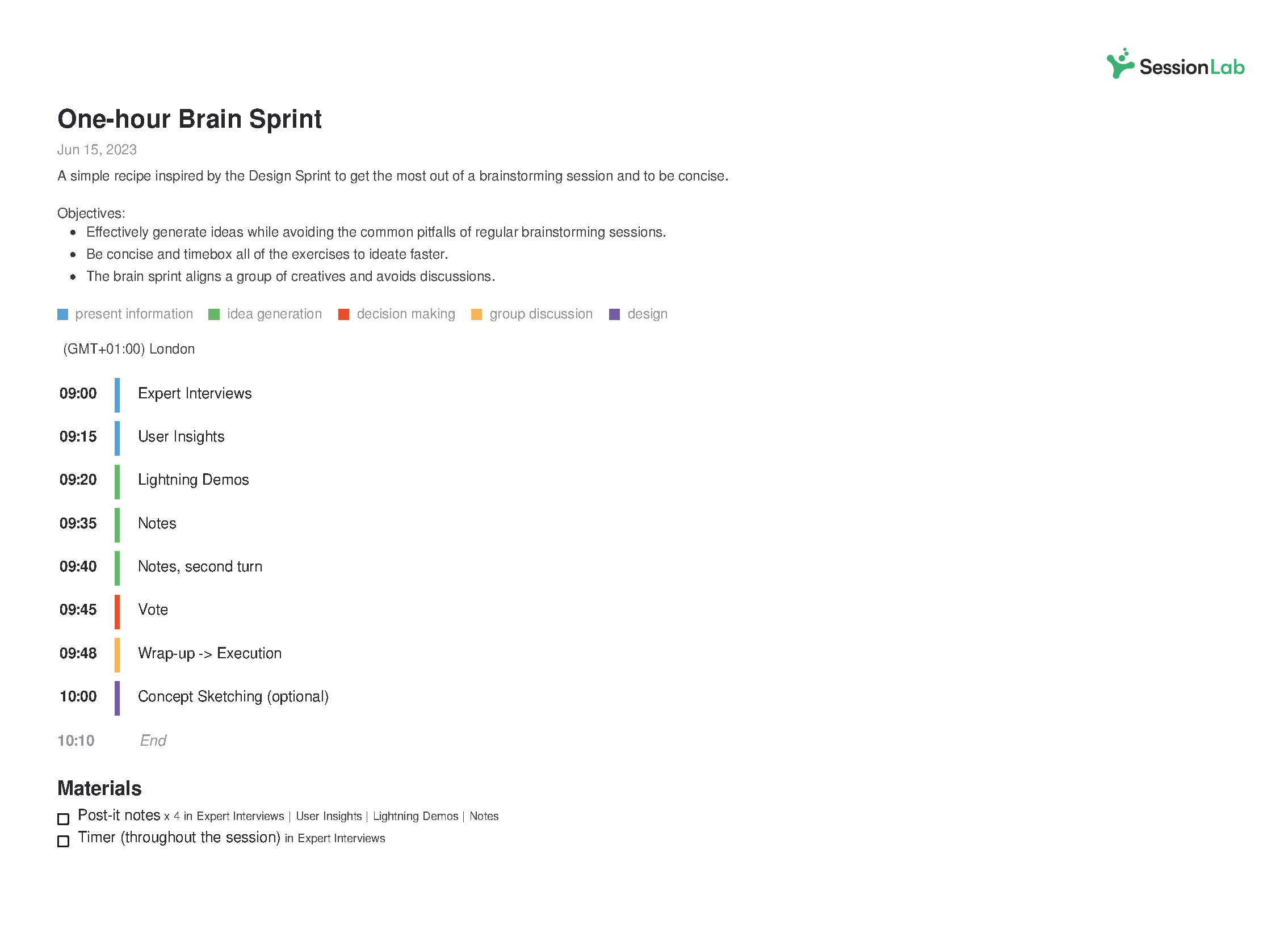
Over to you
The problem-solving process can often be as complicated and multifaceted as the problems they are set-up to solve. With the right problem-solving techniques and a mix of exercises designed to guide discussion and generate purposeful ideas, we hope we’ve given you the tools to find the best solutions as simply and easily as possible.
Is there a problem-solving technique that you are missing here? Do you have a favorite activity or method you use when facilitating? Let us know in the comments below, we’d love to hear from you!
James Smart is Head of Content at SessionLab. He’s also a creative facilitator who has run workshops and designed courses for establishments like the National Centre for Writing, UK. He especially enjoys working with young people and empowering others in their creative practice.
thank you very much for these excellent techniques
Certainly wonderful article, very detailed. Shared!
Your list of techniques for problem solving can be helpfully extended by adding TRIZ to the list of techniques. TRIZ has 40 problem solving techniques derived from methods inventros and patent holders used to get new patents. About 10-12 are general approaches. many organization sponsor classes in TRIZ that are used to solve business problems or general organiztational problems. You can take a look at TRIZ and dwonload a free internet booklet to see if you feel it shound be included per your selection process.
Leave a Comment Cancel reply
Your email address will not be published. Required fields are marked *
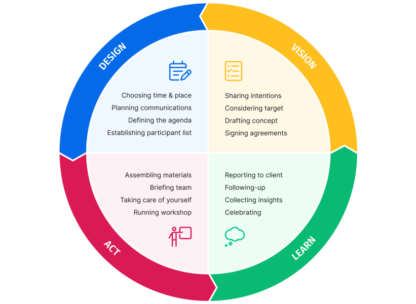
Going from a mere idea to a workshop that delivers results for your clients can feel like a daunting task. In this piece, we will shine a light on all the work behind the scenes and help you learn how to plan a workshop from start to finish. On a good day, facilitation can feel like effortless magic, but that is mostly the result of backstage work, foresight, and a lot of careful planning. Read on to learn a step-by-step approach to breaking the process of planning a workshop into small, manageable chunks. The flow starts with the first meeting with a client to define the purposes of a workshop.…
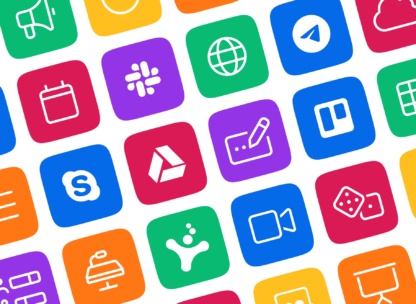
Effective online tools are a necessity for smooth and engaging virtual workshops and meetings. But how do you choose the right ones? Do you sometimes feel that the good old pen and paper or MS Office toolkit and email leaves you struggling to stay on top of managing and delivering your workshop? Fortunately, there are plenty of great workshop tools to make your life easier when you need to facilitate a meeting and lead workshops. In this post, we’ll share our favorite online tools you can use to make your life easier and run better workshops and meetings. In fact, there are plenty of free online workshop tools and meeting…
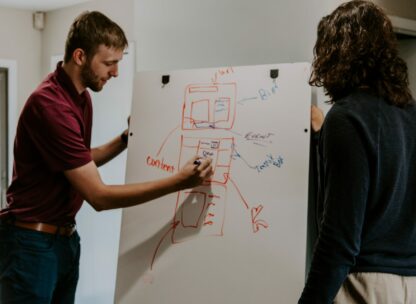
How does learning work? A clever 9-year-old once told me: “I know I am learning something new when I am surprised.” The science of adult learning tells us that, in order to learn new skills (which, unsurprisingly, is harder for adults to do than kids) grown-ups need to first get into a specific headspace. In a business, this approach is often employed in a training session where employees learn new skills or work on professional development. But how do you ensure your training is effective? In this guide, we'll explore how to create an effective training session plan and run engaging training sessions. As team leader, project manager, or consultant,…
Design your next workshop with SessionLab
Join the 150,000 facilitators using SessionLab
Sign up for free
- Product overview
- All features
- Latest feature release
- App integrations
CAPABILITIES
- project icon Project management
- Project views
- Custom fields
- Status updates
- goal icon Goals and reporting
- Reporting dashboards
- workflow icon Workflows and automation
- portfolio icon Resource management
- Capacity planning
- Time tracking
- my-task icon Admin and security
- Admin console
- asana-intelligence icon Asana AI
- list icon Personal
- premium icon Starter
- briefcase icon Advanced
- Goal management
- Organizational planning
- Campaign management
- Creative production
- Content calendars
- Marketing strategic planning
- Resource planning
- Project intake
- Product launches
- Employee onboarding
- View all uses arrow-right icon
- Project plans
- Team goals & objectives
- Team continuity
- Meeting agenda
- View all templates arrow-right icon
- Work management resources Discover best practices, watch webinars, get insights
- Customer stories See how the world's best organizations drive work innovation with Asana
- Help Center Get lots of tips, tricks, and advice to get the most from Asana
- Asana Academy Sign up for interactive courses and webinars to learn Asana
- Developers Learn more about building apps on the Asana platform
- Community programs Connect with and learn from Asana customers around the world
- Events Find out about upcoming events near you
- Partners Learn more about our partner programs
- Asana for nonprofits Get more information on our nonprofit discount program, and apply.
Featured Reads
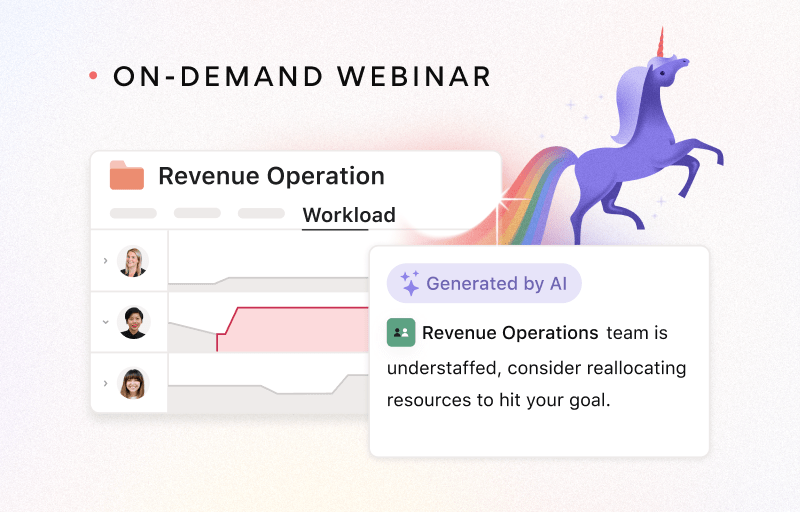
- Collaboration |
- Turn your team into skilled problem sol ...
Turn your team into skilled problem solvers with these problem-solving strategies
Picture this, you're handling your daily tasks at work and your boss calls you in and says, "We have a problem."
Unfortunately, we don't live in a world in which problems are instantly resolved with the snap of our fingers. Knowing how to effectively solve problems is an important professional skill to hone. If you have a problem that needs to be solved, what is the right process to use to ensure you get the most effective solution?
In this article we'll break down the problem-solving process and how you can find the most effective solutions for complex problems.
What is problem solving?
Problem solving is the process of finding a resolution for a specific issue or conflict. There are many possible solutions for solving a problem, which is why it's important to go through a problem-solving process to find the best solution. You could use a flathead screwdriver to unscrew a Phillips head screw, but there is a better tool for the situation. Utilizing common problem-solving techniques helps you find the best solution to fit the needs of the specific situation, much like using the right tools.
Decision-making tools for agile businesses
In this ebook, learn how to equip employees to make better decisions—so your business can pivot, adapt, and tackle challenges more effectively than your competition.
4 steps to better problem solving
While it might be tempting to dive into a problem head first, take the time to move step by step. Here’s how you can effectively break down the problem-solving process with your team:
1. Identify the problem that needs to be solved
One of the easiest ways to identify a problem is to ask questions. A good place to start is to ask journalistic questions, like:
Who : Who is involved with this problem? Who caused the problem? Who is most affected by this issue?
What: What is happening? What is the extent of the issue? What does this problem prevent from moving forward?
Where: Where did this problem take place? Does this problem affect anything else in the immediate area?
When: When did this problem happen? When does this problem take effect? Is this an urgent issue that needs to be solved within a certain timeframe?
Why: Why is it happening? Why does it impact workflows?
How: How did this problem occur? How is it affecting workflows and team members from being productive?
Asking journalistic questions can help you define a strong problem statement so you can highlight the current situation objectively, and create a plan around that situation.
Here’s an example of how a design team uses journalistic questions to identify their problem:
Overarching problem: Design requests are being missed
Who: Design team, digital marketing team, web development team
What: Design requests are forgotten, lost, or being created ad hoc.
Where: Email requests, design request spreadsheet
When: Missed requests on January 20th, January 31st, February 4th, February 6th
How : Email request was lost in inbox and the intake spreadsheet was not updated correctly. The digital marketing team had to delay launching ads for a few days while design requests were bottlenecked. Designers had to work extra hours to ensure all requests were completed.
In this example, there are many different aspects of this problem that can be solved. Using journalistic questions can help you identify different issues and who you should involve in the process.
2. Brainstorm multiple solutions
If at all possible, bring in a facilitator who doesn't have a major stake in the solution. Bringing an individual who has little-to-no stake in the matter can help keep your team on track and encourage good problem-solving skills.
Here are a few brainstorming techniques to encourage creative thinking:
Brainstorm alone before hand: Before you come together as a group, provide some context to your team on what exactly the issue is that you're brainstorming. This will give time for you and your teammates to have some ideas ready by the time you meet.
Say yes to everything (at first): When you first start brainstorming, don't say no to any ideas just yet—try to get as many ideas down as possible. Having as many ideas as possible ensures that you’ll get a variety of solutions. Save the trimming for the next step of the strategy.
Talk to team members one-on-one: Some people may be less comfortable sharing their ideas in a group setting. Discuss the issue with team members individually and encourage them to share their opinions without restrictions—you might find some more detailed insights than originally anticipated.
Break out of your routine: If you're used to brainstorming in a conference room or over Zoom calls, do something a little different! Take your brainstorming meeting to a coffee shop or have your Zoom call while you're taking a walk. Getting out of your routine can force your brain out of its usual rut and increase critical thinking.
3. Define the solution
After you brainstorm with team members to get their unique perspectives on a scenario, it's time to look at the different strategies and decide which option is the best solution for the problem at hand. When defining the solution, consider these main two questions: What is the desired outcome of this solution and who stands to benefit from this solution?
Set a deadline for when this decision needs to be made and update stakeholders accordingly. Sometimes there's too many people who need to make a decision. Use your best judgement based on the limitations provided to do great things fast.
4. Implement the solution
To implement your solution, start by working with the individuals who are as closest to the problem. This can help those most affected by the problem get unblocked. Then move farther out to those who are less affected, and so on and so forth. Some solutions are simple enough that you don’t need to work through multiple teams.
After you prioritize implementation with the right teams, assign out the ongoing work that needs to be completed by the rest of the team. This can prevent people from becoming overburdened during the implementation plan . Once your solution is in place, schedule check-ins to see how the solution is working and course-correct if necessary.
Implement common problem-solving strategies
There are a few ways to go about identifying problems (and solutions). Here are some strategies you can try, as well as common ways to apply them:
Trial and error
Trial and error problem solving doesn't usually require a whole team of people to solve. To use trial and error problem solving, identify the cause of the problem, and then rapidly test possible solutions to see if anything changes.
This problem-solving method is often used in tech support teams through troubleshooting.
The 5 whys problem-solving method helps get to the root cause of an issue. You start by asking once, “Why did this issue happen?” After answering the first why, ask again, “Why did that happen?” You'll do this five times until you can attribute the problem to a root cause.
This technique can help you dig in and find the human error that caused something to go wrong. More importantly, it also helps you and your team develop an actionable plan so that you can prevent the issue from happening again.
Here’s an example:
Problem: The email marketing campaign was accidentally sent to the wrong audience.
“Why did this happen?” Because the audience name was not updated in our email platform.
“Why were the audience names not changed?” Because the audience segment was not renamed after editing.
“Why was the audience segment not renamed?” Because everybody has an individual way of creating an audience segment.
“Why does everybody have an individual way of creating an audience segment?” Because there is no standardized process for creating audience segments.
“Why is there no standardized process for creating audience segments?” Because the team hasn't decided on a way to standardize the process as the team introduced new members.
In this example, we can see a few areas that could be optimized to prevent this mistake from happening again. When working through these questions, make sure that everyone who was involved in the situation is present so that you can co-create next steps to avoid the same problem.
A SWOT analysis
A SWOT analysis can help you highlight the strengths and weaknesses of a specific solution. SWOT stands for:
Strength: Why is this specific solution a good fit for this problem?
Weaknesses: What are the weak points of this solution? Is there anything that you can do to strengthen those weaknesses?
Opportunities: What other benefits could arise from implementing this solution?
Threats: Is there anything about this decision that can detrimentally impact your team?
As you identify specific solutions, you can highlight the different strengths, weaknesses, opportunities, and threats of each solution.
This particular problem-solving strategy is good to use when you're narrowing down the answers and need to compare and contrast the differences between different solutions.
Even more successful problem solving
After you’ve worked through a tough problem, don't forget to celebrate how far you've come. Not only is this important for your team of problem solvers to see their work in action, but this can also help you become a more efficient, effective , and flexible team. The more problems you tackle together, the more you’ll achieve.
Looking for a tool to help solve problems on your team? Track project implementation with a work management tool like Asana .
Related resources
10 tips to improve nonverbal communication
Scaling clinical trial management software with PM solutions
How to build your critical thinking skills in 7 steps (with examples)
4 ways to establish roles and responsibilities for team success
Problem Solving: 15 Examples for Setting Performance Goals
Problem Solving: Use these examples for setting employee performance goals. Help your employees master this skill with 5 fresh ideas that drive change.
Problem Solving is the skill of defining a problem to determine its cause, identify it, prioritize and select alternative solutions to implement in solving the problems and reviving relationships.
Problem Solving: Set Goals for your Employees. Here are some examples:
- To be accommodative of other people's ideas and views and to be willing to take them on board.
- Research well enough to gather factual information before setting out to solve a problem.
- Look at things in different perspectives and angles and to develop alternative options.
- Be willing enough to collaborate with other when it comes to problem-solving issues.
- Learn to articulate or communicate in a proper manner that can be well understood by people.
- Get first to understand what the problem really is before starting to solve it.
- Show great confidence and poise when making decisions and not afraid to make mistakes and learn from them.
- Keep a cool head when dealing with more pressing and exhausting issues.
- Try to ask the right questions that will act as a guide to coming up with proper solutions.
- Be more flexible to change and adapt to new tact and ways of finding new solutions.
Problem Solving: Improve and master this core skill with these ideas
- Identify the problem. determine the nature of the problem, break it down and come up with a useful set of actions to address the challenges that are related to it.
- Concentrate on the solution, not the problem. Looking for solutions will not happen if you focus on the problem all the time. Concentrating on finding the answer is a move that brings about new opportunities and ideas that can be lucrative.
- Write down as many solutions as possible. Listing down various solutions is important to help you keep an open mind and boost creative thinking that can trigger potential solutions. This list will also act as your reminder.
- Think laterally. Thinking laterally means changing the approach and looking at things differently as well as making different choices.
- Use positive language that creates possibilities. Using words that are active to speak to others, and yourself build a mind that thinks creatively encouraging new ideas and solutions to be set.
These articles may interest you
Recent articles.
- Skills needed to be a traffic engineer
- Skills needed to be a senior project estimator
- Outstanding Employee Performance Feedback: Inventory & Operations Auditor
- New Hire Offer Letter
- Presentation Skills: 15 Examples for Setting Performance Goals
- Poor Employee Performance Feedback: Comptroller/Controller
- 7 Steps For Developing A Performance Appraisal System
- Employee Performance Goals Sample: Chief Executive Officer
- Skills needed to be a benefits analyst
- Skills needed to be an it audit senior manager
- Employee Separation Definition, Formula And Examples
- Good Employee Performance Feedback: Invoice Control Clerk
- Good Employee Performance Feedback: Senior Cost Analyst
- Top 28 Employee Appreciation Day Activities
- Poor Employee Performance Feedback: Facilities Technician

An official website of the United States government
The .gov means it’s official. Federal government websites often end in .gov or .mil. Before sharing sensitive information, make sure you’re on a federal government site.
The site is secure. The https:// ensures that you are connecting to the official website and that any information you provide is encrypted and transmitted securely.
- Publications
- Account settings
Preview improvements coming to the PMC website in October 2024. Learn More or Try it out now .
- Advanced Search
- Journal List
- Front Psychol
Complex Problem Solving: What It Is and What It Is Not
Dietrich dörner.
1 Department of Psychology, University of Bamberg, Bamberg, Germany
Joachim Funke
2 Department of Psychology, Heidelberg University, Heidelberg, Germany
Computer-simulated scenarios have been part of psychological research on problem solving for more than 40 years. The shift in emphasis from simple toy problems to complex, more real-life oriented problems has been accompanied by discussions about the best ways to assess the process of solving complex problems. Psychometric issues such as reliable assessments and addressing correlations with other instruments have been in the foreground of these discussions and have left the content validity of complex problem solving in the background. In this paper, we return the focus to content issues and address the important features that define complex problems.
Succeeding in the 21st century requires many competencies, including creativity, life-long learning, and collaboration skills (e.g., National Research Council, 2011 ; Griffin and Care, 2015 ), to name only a few. One competence that seems to be of central importance is the ability to solve complex problems ( Mainzer, 2009 ). Mainzer quotes the Nobel prize winner Simon (1957) who wrote as early as 1957:
The capacity of the human mind for formulating and solving complex problems is very small compared with the size of the problem whose solution is required for objectively rational behavior in the real world or even for a reasonable approximation to such objective rationality. (p. 198)
The shift from well-defined to ill-defined problems came about as a result of a disillusion with the “general problem solver” ( Newell et al., 1959 ): The general problem solver was a computer software intended to solve all kind of problems that can be expressed through well-formed formulas. However, it soon became clear that this procedure was in fact a “special problem solver” that could only solve well-defined problems in a closed space. But real-world problems feature open boundaries and have no well-determined solution. In fact, the world is full of wicked problems and clumsy solutions ( Verweij and Thompson, 2006 ). As a result, solving well-defined problems and solving ill-defined problems requires different cognitive processes ( Schraw et al., 1995 ; but see Funke, 2010 ).
Well-defined problems have a clear set of means for reaching a precisely described goal state. For example: in a match-stick arithmetic problem, a person receives a false arithmetic expression constructed out of matchsticks (e.g., IV = III + III). According to the instructions, moving one of the matchsticks will make the equations true. Here, both the problem (find the appropriate stick to move) and the goal state (true arithmetic expression; solution is: VI = III + III) are defined clearly.
Ill-defined problems have no clear problem definition, their goal state is not defined clearly, and the means of moving towards the (diffusely described) goal state are not clear. For example: The goal state for solving the political conflict in the near-east conflict between Israel and Palestine is not clearly defined (living in peaceful harmony with each other?) and even if the conflict parties would agree on a two-state solution, this goal again leaves many issues unresolved. This type of problem is called a “complex problem” and is of central importance to this paper. All psychological processes that occur within individual persons and deal with the handling of such ill-defined complex problems will be subsumed under the umbrella term “complex problem solving” (CPS).
Systematic research on CPS started in the 1970s with observations of the behavior of participants who were confronted with computer simulated microworlds. For example, in one of those microworlds participants assumed the role of executives who were tasked to manage a company over a certain period of time (see Brehmer and Dörner, 1993 , for a discussion of this methodology). Today, CPS is an established concept and has even influenced large-scale assessments such as PISA (“Programme for International Student Assessment”), organized by the Organization for Economic Cooperation and Development ( OECD, 2014 ). According to the World Economic Forum, CPS is one of the most important competencies required in the future ( World Economic Forum, 2015 ). Numerous articles on the subject have been published in recent years, documenting the increasing research activity relating to this field. In the following collection of papers we list only those published in 2010 and later: theoretical papers ( Blech and Funke, 2010 ; Funke, 2010 ; Knauff and Wolf, 2010 ; Leutner et al., 2012 ; Selten et al., 2012 ; Wüstenberg et al., 2012 ; Greiff et al., 2013b ; Fischer and Neubert, 2015 ; Schoppek and Fischer, 2015 ), papers about measurement issues ( Danner et al., 2011a ; Greiff et al., 2012 , 2015a ; Alison et al., 2013 ; Gobert et al., 2015 ; Greiff and Fischer, 2013 ; Herde et al., 2016 ; Stadler et al., 2016 ), papers about applications ( Fischer and Neubert, 2015 ; Ederer et al., 2016 ; Tremblay et al., 2017 ), papers about differential effects ( Barth and Funke, 2010 ; Danner et al., 2011b ; Beckmann and Goode, 2014 ; Greiff and Neubert, 2014 ; Scherer et al., 2015 ; Meißner et al., 2016 ; Wüstenberg et al., 2016 ), one paper about developmental effects ( Frischkorn et al., 2014 ), one paper with a neuroscience background ( Osman, 2012 ) 1 , papers about cultural differences ( Güss and Dörner, 2011 ; Sonnleitner et al., 2014 ; Güss et al., 2015 ), papers about validity issues ( Goode and Beckmann, 2010 ; Greiff et al., 2013c ; Schweizer et al., 2013 ; Mainert et al., 2015 ; Funke et al., 2017 ; Greiff et al., 2017 , 2015b ; Kretzschmar et al., 2016 ; Kretzschmar, 2017 ), review papers and meta-analyses ( Osman, 2010 ; Stadler et al., 2015 ), and finally books ( Qudrat-Ullah, 2015 ; Csapó and Funke, 2017b ) and book chapters ( Funke, 2012 ; Hotaling et al., 2015 ; Funke and Greiff, 2017 ; Greiff and Funke, 2017 ; Csapó and Funke, 2017a ; Fischer et al., 2017 ; Molnàr et al., 2017 ; Tobinski and Fritz, 2017 ; Viehrig et al., 2017 ). In addition, a new “Journal of Dynamic Decision Making” (JDDM) has been launched ( Fischer et al., 2015 , 2016 ) to give the field an open-access outlet for research and discussion.
This paper aims to clarify aspects of validity: what should be meant by the term CPS and what not? This clarification seems necessary because misunderstandings in recent publications provide – from our point of view – a potentially misleading picture of the construct. We start this article with a historical review before attempting to systematize different positions. We conclude with a working definition.
Historical Review
The concept behind CPS goes back to the German phrase “komplexes Problemlösen” (CPS; the term “komplexes Problemlösen” was used as a book title by Funke, 1986 ). The concept was introduced in Germany by Dörner and colleagues in the mid-1970s (see Dörner et al., 1975 ; Dörner, 1975 ) for the first time. The German phrase was later translated to CPS in the titles of two edited volumes by Sternberg and Frensch (1991) and Frensch and Funke (1995a) that collected papers from different research traditions. Even though it looks as though the term was coined in the 1970s, Edwards (1962) used the term “dynamic decision making” to describe decisions that come in a sequence. He compared static with dynamic decision making, writing:
- simple In dynamic situations, a new complication not found in the static situations arises. The environment in which the decision is set may be changing, either as a function of the sequence of decisions, or independently of them, or both. It is this possibility of an environment which changes while you collect information about it which makes the task of dynamic decision theory so difficult and so much fun. (p. 60)
The ability to solve complex problems is typically measured via dynamic systems that contain several interrelated variables that participants need to alter. Early work (see, e.g., Dörner, 1980 ) used a simulation scenario called “Lohhausen” that contained more than 2000 variables that represented the activities of a small town: Participants had to take over the role of a mayor for a simulated period of 10 years. The simulation condensed these ten years to ten hours in real time. Later, researchers used smaller dynamic systems as scenarios either based on linear equations (see, e.g., Funke, 1993 ) or on finite state automata (see, e.g., Buchner and Funke, 1993 ). In these contexts, CPS consisted of the identification and control of dynamic task environments that were previously unknown to the participants. Different task environments came along with different degrees of fidelity ( Gray, 2002 ).
According to Funke (2012) , the typical attributes of complex systems are (a) complexity of the problem situation which is usually represented by the sheer number of involved variables; (b) connectivity and mutual dependencies between involved variables; (c) dynamics of the situation, which reflects the role of time and developments within a system; (d) intransparency (in part or full) about the involved variables and their current values; and (e) polytely (greek term for “many goals”), representing goal conflicts on different levels of analysis. This mixture of features is similar to what is called VUCA (volatility, uncertainty, complexity, ambiguity) in modern approaches to management (e.g., Mack et al., 2016 ).
In his evaluation of the CPS movement, Sternberg (1995) compared (young) European approaches to CPS with (older) American research on expertise. His analysis of the differences between the European and American traditions shows advantages but also potential drawbacks for each side. He states (p. 301): “I believe that although there are problems with the European approach, it deals with some fundamental questions that American research scarcely addresses.” So, even though the echo of the European approach did not enjoy strong resonance in the US at that time, it was valued by scholars like Sternberg and others. Before attending to validity issues, we will first present a short review of different streams.
Different Approaches to CPS
In the short history of CPS research, different approaches can be identified ( Buchner, 1995 ; Fischer et al., 2017 ). To systematize, we differentiate between the following five lines of research:
- simple (a) The search for individual differences comprises studies identifying interindividual differences that affect the ability to solve complex problems. This line of research is reflected, for example, in the early work by Dörner et al. (1983) and their “Lohhausen” study. Here, naïve student participants took over the role of the mayor of a small simulated town named Lohhausen for a simulation period of ten years. According to the results of the authors, it is not intelligence (as measured by conventional IQ tests) that predicts performance, but it is the ability to stay calm in the face of a challenging situation and the ability to switch easily between an analytic mode of processing and a more holistic one.
- simple (b) The search for cognitive processes deals with the processes behind understanding complex dynamic systems. Representative of this line of research is, for example, Berry and Broadbent’s (1984) work on implicit and explicit learning processes when people interact with a dynamic system called “Sugar Production”. They found that those who perform best in controlling a dynamic system can do so implicitly, without explicit knowledge of details regarding the systems’ relations.
- simple (c) The search for system factors seeks to identify the aspects of dynamic systems that determine the difficulty of complex problems and make some problems harder than others. Representative of this line of research is, for example, work by Funke (1985) , who systematically varied the number of causal effects within a dynamic system or the presence/absence of eigendynamics. He found, for example, that solution quality decreases as the number of systems relations increases.
- simple (d) The psychometric approach develops measurement instruments that can be used as an alternative to classical IQ tests, as something that goes “beyond IQ”. The MicroDYN approach ( Wüstenberg et al., 2012 ) is representative for this line of research that presents an alternative to reasoning tests (like Raven matrices). These authors demonstrated that a small improvement in predicting school grade point average beyond reasoning is possible with MicroDYN tests.
- simple (e) The experimental approach explores CPS under different experimental conditions. This approach uses CPS assessment instruments to test hypotheses derived from psychological theories and is sometimes used in research about cognitive processes (see above). Exemplary for this line of research is the work by Rohe et al. (2016) , who test the usefulness of “motto goals” in the context of complex problems compared to more traditional learning and performance goals. Motto goals differ from pure performance goals by activating positive affect and should lead to better goal attainment especially in complex situations (the mentioned study found no effect).
To be clear: these five approaches are not mutually exclusive and do overlap. But the differentiation helps to identify different research communities and different traditions. These communities had different opinions about scaling complexity.
The Race for Complexity: Use of More and More Complex Systems
In the early years of CPS research, microworlds started with systems containing about 20 variables (“Tailorshop”), soon reached 60 variables (“Moro”), and culminated in systems with about 2000 variables (“Lohhausen”). This race for complexity ended with the introduction of the concept of “minimal complex systems” (MCS; Greiff and Funke, 2009 ; Funke and Greiff, 2017 ), which ushered in a search for the lower bound of complexity instead of the higher bound, which could not be defined as easily. The idea behind this concept was that whereas the upper limits of complexity are unbound, the lower limits might be identifiable. Imagine starting with a simple system containing two variables with a simple linear connection between them; then, step by step, increase the number of variables and/or the type of connections. One soon reaches a point where the system can no longer be considered simple and has become a “complex system”. This point represents a minimal complex system. Despite some research having been conducted in this direction, the point of transition from simple to complex has not been identified clearly as of yet.
Some years later, the original “minimal complex systems” approach ( Greiff and Funke, 2009 ) shifted to the “multiple complex systems” approach ( Greiff et al., 2013a ). This shift is more than a slight change in wording: it is important because it taps into the issue of validity directly. Minimal complex systems have been introduced in the context of challenges from large-scale assessments like PISA 2012 that measure new aspects of problem solving, namely interactive problems besides static problem solving ( Greiff and Funke, 2017 ). PISA 2012 required test developers to remain within testing time constraints (given by the school class schedule). Also, test developers needed a large item pool for the construction of a broad class of problem solving items. It was clear from the beginning that MCS deal with simple dynamic situations that require controlled interaction: the exploration and control of simple ticket machines, simple mobile phones, or simple MP3 players (all of these example domains were developed within PISA 2012) – rather than really complex situations like managerial or political decision making.
As a consequence of this subtle but important shift in interpreting the letters MCS, the definition of CPS became a subject of debate recently ( Funke, 2014a ; Greiff and Martin, 2014 ; Funke et al., 2017 ). In the words of Funke (2014b , p. 495):
- simple It is funny that problems that nowadays come under the term ‘CPS’, are less complex (in terms of the previously described attributes of complex situations) than at the beginning of this new research tradition. The emphasis on psychometric qualities has led to a loss of variety. Systems thinking requires more than analyzing models with two or three linear equations – nonlinearity, cyclicity, rebound effects, etc. are inherent features of complex problems and should show up at least in some of the problems used for research and assessment purposes. Minimal complex systems run the danger of becoming minimal valid systems.
Searching for minimal complex systems is not the same as gaining insight into the way how humans deal with complexity and uncertainty. For psychometric purposes, it is appropriate to reduce complexity to a minimum; for understanding problem solving under conditions of overload, intransparency, and dynamics, it is necessary to realize those attributes with reasonable strength. This aspect is illustrated in the next section.
Importance of the Validity Issue
The most important reason for discussing the question of what complex problem solving is and what it is not stems from its phenomenology: if we lose sight of our phenomena, we are no longer doing good psychology. The relevant phenomena in the context of complex problems encompass many important aspects. In this section, we discuss four phenomena that are specific to complex problems. We consider these phenomena as critical for theory development and for the construction of assessment instruments (i.e., microworlds). These phenomena require theories for explaining them and they require assessment instruments eliciting them in a reliable way.
The first phenomenon is the emergency reaction of the intellectual system ( Dörner, 1980 ): When dealing with complex systems, actors tend to (a) reduce their intellectual level by decreasing self-reflections, by decreasing their intentions, by stereotyping, and by reducing their realization of intentions, (b) they show a tendency for fast action with increased readiness for risk, with increased violations of rules, and with increased tendency to escape the situation, and (c) they degenerate their hypotheses formation by construction of more global hypotheses and reduced tests of hypotheses, by increasing entrenchment, and by decontextualizing their goals. This phenomenon illustrates the strong connection between cognition, emotion, and motivation that has been emphasized by Dörner (see, e.g., Dörner and Güss, 2013 ) from the beginning of his research tradition; the emergency reaction reveals a shift in the mode of information processing under the pressure of complexity.
The second phenomenon comprises cross-cultural differences with respect to strategy use ( Strohschneider and Güss, 1999 ; Güss and Wiley, 2007 ; Güss et al., 2015 ). Results from complex task environments illustrate the strong influence of context and background knowledge to an extent that cannot be found for knowledge-poor problems. For example, in a comparison between Brazilian and German participants, it turned out that Brazilians accept the given problem descriptions and are more optimistic about the results of their efforts, whereas Germans tend to inquire more about the background of the problems and take a more active approach but are less optimistic (according to Strohschneider and Güss, 1998 , p. 695).
The third phenomenon relates to failures that occur during the planning and acting stages ( Jansson, 1994 ; Ramnarayan et al., 1997 ), illustrating that rational procedures seem to be unlikely to be used in complex situations. The potential for failures ( Dörner, 1996 ) rises with the complexity of the problem. Jansson (1994) presents seven major areas for failures with complex situations: acting directly on current feedback; insufficient systematization; insufficient control of hypotheses and strategies; lack of self-reflection; selective information gathering; selective decision making; and thematic vagabonding.
The fourth phenomenon describes (a lack of) training and transfer effects ( Kretzschmar and Süß, 2015 ), which again illustrates the context dependency of strategies and knowledge (i.e., there is no strategy that is so universal that it can be used in many different problem situations). In their own experiment, the authors could show training effects only for knowledge acquisition, not for knowledge application. Only with specific feedback, performance in complex environments can be increased ( Engelhart et al., 2017 ).
These four phenomena illustrate why the type of complexity (or degree of simplicity) used in research really matters. Furthermore, they demonstrate effects that are specific for complex problems, but not for toy problems. These phenomena direct the attention to the important question: does the stimulus material used (i.e., the computer-simulated microworld) tap and elicit the manifold of phenomena described above?
Dealing with partly unknown complex systems requires courage, wisdom, knowledge, grit, and creativity. In creativity research, “little c” and “BIG C” are used to differentiate between everyday creativity and eminent creativity ( Beghetto and Kaufman, 2007 ; Kaufman and Beghetto, 2009 ). Everyday creativity is important for solving everyday problems (e.g., finding a clever fix for a broken spoke on my bicycle), eminent creativity changes the world (e.g., inventing solar cells for energy production). Maybe problem solving research should use a similar differentiation between “little p” and “BIG P” to mark toy problems on the one side and big societal challenges on the other. The question then remains: what can we learn about BIG P by studying little p? What phenomena are present in both types, and what phenomena are unique to each of the two extremes?
Discussing research on CPS requires reflecting on the field’s research methods. Even if the experimental approach has been successful for testing hypotheses (for an overview of older work, see Funke, 1995 ), other methods might provide additional and novel insights. Complex phenomena require complex approaches to understand them. The complex nature of complex systems imposes limitations on psychological experiments: The more complex the environments, the more difficult is it to keep conditions under experimental control. And if experiments have to be run in labs one should bring enough complexity into the lab to establish the phenomena mentioned, at least in part.
There are interesting options to be explored (again): think-aloud protocols , which have been discredited for many years ( Nisbett and Wilson, 1977 ) and yet are a valuable source for theory testing ( Ericsson and Simon, 1983 ); introspection ( Jäkel and Schreiber, 2013 ), which seems to be banned from psychological methods but nevertheless offers insights into thought processes; the use of life-streaming ( Wendt, 2017 ), a medium in which streamers generate a video stream of think-aloud data in computer-gaming; political decision-making ( Dhami et al., 2015 ) that demonstrates error-proneness in groups; historical case studies ( Dörner and Güss, 2011 ) that give insights into the thinking styles of political leaders; the use of the critical incident technique ( Reuschenbach, 2008 ) to construct complex scenarios; and simulations with different degrees of fidelity ( Gray, 2002 ).
The methods tool box is full of instruments that have to be explored more carefully before any individual instrument receives a ban or research narrows its focus to only one paradigm for data collection. Brehmer and Dörner (1993) discussed the tensions between “research in the laboratory and research in the field”, optimistically concluding “that the new methodology of computer-simulated microworlds will provide us with the means to bridge the gap between the laboratory and the field” (p. 183). The idea behind this optimism was that computer-simulated scenarios would bring more complexity from the outside world into the controlled lab environment. But this is not true for all simulated scenarios. In his paper on simulated environments, Gray (2002) differentiated computer-simulated environments with respect to three dimensions: (1) tractability (“the more training subjects require before they can use a simulated task environment, the less tractable it is”, p. 211), correspondence (“High correspondence simulated task environments simulate many aspects of one task environment. Low correspondence simulated task environments simulate one aspect of many task environments”, p. 214), and engagement (“A simulated task environment is engaging to the degree to which it involves and occupies the participants; that is, the degree to which they agree to take it seriously”, p. 217). But the mere fact that a task is called a “computer-simulated task environment” does not mean anything specific in terms of these three dimensions. This is one of several reasons why we should differentiate between those studies that do not address the core features of CPS and those that do.
What is not CPS?
Even though a growing number of references claiming to deal with complex problems exist (e.g., Greiff and Wüstenberg, 2015 ; Greiff et al., 2016 ), it would be better to label the requirements within these tasks “dynamic problem solving,” as it has been done adequately in earlier work ( Greiff et al., 2012 ). The dynamics behind on-off-switches ( Thimbleby, 2007 ) are remarkable but not really complex. Small nonlinear systems that exhibit stunningly complex and unstable behavior do exist – but they are not used in psychometric assessments of so-called CPS. There are other small systems (like MicroDYN scenarios: Greiff and Wüstenberg, 2014 ) that exhibit simple forms of system behavior that are completely predictable and stable. This type of simple systems is used frequently. It is even offered commercially as a complex problem-solving test called COMPRO ( Greiff and Wüstenberg, 2015 ) for business applications. But a closer look reveals that the label is not used correctly; within COMPRO, the used linear equations are far from being complex and the system can be handled properly by using only one strategy (see for more details Funke et al., 2017 ).
Why do simple linear systems not fall within CPS? At the surface, nonlinear and linear systems might appear similar because both only include 3–5 variables. But the difference is in terms of systems behavior as well as strategies and learning. If the behavior is simple (as in linear systems where more input is related to more output and vice versa), the system can be easily understood (participants in the MicroDYN world have 3 minutes to explore a complex system). If the behavior is complex (as in systems that contain strange attractors or negative feedback loops), things become more complicated and much more observation is needed to identify the hidden structure of the unknown system ( Berry and Broadbent, 1984 ; Hundertmark et al., 2015 ).
Another issue is learning. If tasks can be solved using a single (and not so complicated) strategy, steep learning curves are to be expected. The shift from problem solving to learned routine behavior occurs rapidly, as was demonstrated by Luchins (1942) . In his water jar experiments, participants quickly acquired a specific strategy (a mental set) for solving certain measurement problems that they later continued applying to problems that would have allowed for easier approaches. In the case of complex systems, learning can occur only on very general, abstract levels because it is difficult for human observers to make specific predictions. Routines dealing with complex systems are quite different from routines relating to linear systems.
What should not be studied under the label of CPS are pure learning effects, multiple-cue probability learning, or tasks that can be solved using a single strategy. This last issue is a problem for MicroDYN tasks that rely strongly on the VOTAT strategy (“vary one thing at a time”; see Tschirgi, 1980 ). In real-life, it is hard to imagine a business manager trying to solve her or his problems by means of VOTAT.
What is CPS?
In the early days of CPS research, planet Earth’s dynamics and complexities gained attention through such books as “The limits to growth” ( Meadows et al., 1972 ) and “Beyond the limits” ( Meadows et al., 1992 ). In the current decade, for example, the World Economic Forum (2016) attempts to identify the complexities and risks of our modern world. In order to understand the meaning of complexity and uncertainty, taking a look at the worlds’ most pressing issues is helpful. Searching for strategies to cope with these problems is a difficult task: surely there is no place for the simple principle of “vary-one-thing-at-a-time” (VOTAT) when it comes to global problems. The VOTAT strategy is helpful in the context of simple problems ( Wüstenberg et al., 2014 ); therefore, whether or not VOTAT is helpful in a given problem situation helps us distinguish simple from complex problems.
Because there exist no clear-cut strategies for complex problems, typical failures occur when dealing with uncertainty ( Dörner, 1996 ; Güss et al., 2015 ). Ramnarayan et al. (1997) put together a list of generic errors (e.g., not developing adequate action plans; lack of background control; learning from experience blocked by stereotype knowledge; reactive instead of proactive action) that are typical of knowledge-rich complex systems but cannot be found in simple problems.
Complex problem solving is not a one-dimensional, low-level construct. On the contrary, CPS is a multi-dimensional bundle of competencies existing at a high level of abstraction, similar to intelligence (but going beyond IQ). As Funke et al. (2018) state: “Assessment of transversal (in educational contexts: cross-curricular) competencies cannot be done with one or two types of assessment. The plurality of skills and competencies requires a plurality of assessment instruments.”
There are at least three different aspects of complex systems that are part of our understanding of a complex system: (1) a complex system can be described at different levels of abstraction; (2) a complex system develops over time, has a history, a current state, and a (potentially unpredictable) future; (3) a complex system is knowledge-rich and activates a large semantic network, together with a broad list of potential strategies (domain-specific as well as domain-general).
Complex problem solving is not only a cognitive process but is also an emotional one ( Spering et al., 2005 ; Barth and Funke, 2010 ) and strongly dependent on motivation (low-stakes versus high-stakes testing; see Hermes and Stelling, 2016 ).
Furthermore, CPS is a dynamic process unfolding over time, with different phases and with more differentiation than simply knowledge acquisition and knowledge application. Ideally, the process should entail identifying problems (see Dillon, 1982 ; Lee and Cho, 2007 ), even if in experimental settings, problems are provided to participants a priori . The more complex and open a given situation, the more options can be generated (T. S. Schweizer et al., 2016 ). In closed problems, these processes do not occur in the same way.
In analogy to the difference between formative (process-oriented) and summative (result-oriented) assessment ( Wiliam and Black, 1996 ; Bennett, 2011 ), CPS should not be reduced to the mere outcome of a solution process. The process leading up to the solution, including detours and errors made along the way, might provide a more differentiated impression of a person’s problem-solving abilities and competencies than the final result of such a process. This is one of the reasons why CPS environments are not, in fact, complex intelligence tests: research on CPS is not only about the outcome of the decision process, but it is also about the problem-solving process itself.
Complex problem solving is part of our daily life: finding the right person to share one’s life with, choosing a career that not only makes money, but that also makes us happy. Of course, CPS is not restricted to personal problems – life on Earth gives us many hard nuts to crack: climate change, population growth, the threat of war, the use and distribution of natural resources. In sum, many societal challenges can be seen as complex problems. To reduce that complexity to a one-hour lab activity on a random Friday afternoon puts it out of context and does not address CPS issues.
Theories about CPS should specify which populations they apply to. Across populations, one thing to consider is prior knowledge. CPS research with experts (e.g., Dew et al., 2009 ) is quite different from problem solving research using tasks that intentionally do not require any specific prior knowledge (see, e.g., Beckmann and Goode, 2014 ).
More than 20 years ago, Frensch and Funke (1995b) defined CPS as follows:
- simple CPS occurs to overcome barriers between a given state and a desired goal state by means of behavioral and/or cognitive, multi-step activities. The given state, goal state, and barriers between given state and goal state are complex, change dynamically during problem solving, and are intransparent. The exact properties of the given state, goal state, and barriers are unknown to the solver at the outset. CPS implies the efficient interaction between a solver and the situational requirements of the task, and involves a solver’s cognitive, emotional, personal, and social abilities and knowledge. (p. 18)
The above definition is rather formal and does not account for content or relations between the simulation and the real world. In a sense, we need a new definition of CPS that addresses these issues. Based on our previous arguments, we propose the following working definition:
- simple Complex problem solving is a collection of self-regulated psychological processes and activities necessary in dynamic environments to achieve ill-defined goals that cannot be reached by routine actions. Creative combinations of knowledge and a broad set of strategies are needed. Solutions are often more bricolage than perfect or optimal. The problem-solving process combines cognitive, emotional, and motivational aspects, particularly in high-stakes situations. Complex problems usually involve knowledge-rich requirements and collaboration among different persons.
The main differences to the older definition lie in the emphasis on (a) the self-regulation of processes, (b) creativity (as opposed to routine behavior), (c) the bricolage type of solution, and (d) the role of high-stakes challenges. Our new definition incorporates some aspects that have been discussed in this review but were not reflected in the 1995 definition, which focused on attributes of complex problems like dynamics or intransparency.
This leads us to the final reflection about the role of CPS for dealing with uncertainty and complexity in real life. We will distinguish thinking from reasoning and introduce the sense of possibility as an important aspect of validity.
CPS as Combining Reasoning and Thinking in an Uncertain Reality
Leading up to the Battle of Borodino in Leo Tolstoy’s novel “War and Peace”, Prince Andrei Bolkonsky explains the concept of war to his friend Pierre. Pierre expects war to resemble a game of chess: You position the troops and attempt to defeat your opponent by moving them in different directions.
“Far from it!”, Andrei responds. “In chess, you know the knight and his moves, you know the pawn and his combat strength. While in war, a battalion is sometimes stronger than a division and sometimes weaker than a company; it all depends on circumstances that can never be known. In war, you do not know the position of your enemy; some things you might be able to observe, some things you have to divine (but that depends on your ability to do so!) and many things cannot even be guessed at. In chess, you can see all of your opponent’s possible moves. In war, that is impossible. If you decide to attack, you cannot know whether the necessary conditions are met for you to succeed. Many a time, you cannot even know whether your troops will follow your orders…”
In essence, war is characterized by a high degree of uncertainty. A good commander (or politician) can add to that what he or she sees, tentatively fill in the blanks – and not just by means of logical deduction but also by intelligently bridging missing links. A bad commander extrapolates from what he sees and thus arrives at improper conclusions.
Many languages differentiate between two modes of mentalizing; for instance, the English language distinguishes between ‘thinking’ and ‘reasoning’. Reasoning denotes acute and exact mentalizing involving logical deductions. Such deductions are usually based on evidence and counterevidence. Thinking, however, is what is required to write novels. It is the construction of an initially unknown reality. But it is not a pipe dream, an unfounded process of fabrication. Rather, thinking asks us to imagine reality (“Wirklichkeitsfantasie”). In other words, a novelist has to possess a “sense of possibility” (“Möglichkeitssinn”, Robert Musil; in German, sense of possibility is often used synonymously with imagination even though imagination is not the same as sense of possibility, for imagination also encapsulates the impossible). This sense of possibility entails knowing the whole (or several wholes) or being able to construe an unknown whole that could accommodate a known part. The whole has to align with sociological and geographical givens, with the mentality of certain peoples or groups, and with the laws of physics and chemistry. Otherwise, the entire venture is ill-founded. A sense of possibility does not aim for the moon but imagines something that might be possible but has not been considered possible or even potentially possible so far.
Thinking is a means to eliminate uncertainty. This process requires both of the modes of thinking we have discussed thus far. Economic, political, or ecological decisions require us to first consider the situation at hand. Though certain situational aspects can be known, but many cannot. In fact, von Clausewitz (1832) posits that only about 25% of the necessary information is available when a military decision needs to be made. Even then, there is no way to guarantee that whatever information is available is also correct: Even if a piece of information was completely accurate yesterday, it might no longer apply today.
Once our sense of possibility has helped grasping a situation, problem solvers need to call on their reasoning skills. Not every situation requires the same action, and we may want to act this way or another to reach this or that goal. This appears logical, but it is a logic based on constantly shifting grounds: We cannot know whether necessary conditions are met, sometimes the assumptions we have made later turn out to be incorrect, and sometimes we have to revise our assumptions or make completely new ones. It is necessary to constantly switch between our sense of possibility and our sense of reality, that is, to switch between thinking and reasoning. It is an arduous process, and some people handle it well, while others do not.
If we are to believe Tuchman’s (1984) book, “The March of Folly”, most politicians and commanders are fools. According to Tuchman, not much has changed in the 3300 years that have elapsed since the misguided Trojans decided to welcome the left-behind wooden horse into their city that would end up dismantling Troy’s defensive walls. The Trojans, too, had been warned, but decided not to heed the warning. Although Laocoön had revealed the horse’s true nature to them by attacking it with a spear, making the weapons inside the horse ring, the Trojans refused to see the forest for the trees. They did not want to listen, they wanted the war to be over, and this desire ended up shaping their perception.
The objective of psychology is to predict and explain human actions and behavior as accurately as possible. However, thinking cannot be investigated by limiting its study to neatly confined fractions of reality such as the realms of propositional logic, chess, Go tasks, the Tower of Hanoi, and so forth. Within these systems, there is little need for a sense of possibility. But a sense of possibility – the ability to divine and construe an unknown reality – is at least as important as logical reasoning skills. Not researching the sense of possibility limits the validity of psychological research. All economic and political decision making draws upon this sense of possibility. By not exploring it, psychological research dedicated to the study of thinking cannot further the understanding of politicians’ competence and the reasons that underlie political mistakes. Christopher Clark identifies European diplomats’, politicians’, and commanders’ inability to form an accurate representation of reality as a reason for the outbreak of World War I. According to Clark’s (2012) book, “The Sleepwalkers”, the politicians of the time lived in their own make-believe world, wrongfully assuming that it was the same world everyone else inhabited. If CPS research wants to make significant contributions to the world, it has to acknowledge complexity and uncertainty as important aspects of it.
For more than 40 years, CPS has been a new subject of psychological research. During this time period, the initial emphasis on analyzing how humans deal with complex, dynamic, and uncertain situations has been lost. What is subsumed under the heading of CPS in modern research has lost the original complexities of real-life problems. From our point of view, the challenges of the 21st century require a return to the origins of this research tradition. We would encourage researchers in the field of problem solving to come back to the original ideas. There is enough complexity and uncertainty in the world to be studied. Improving our understanding of how humans deal with these global and pressing problems would be a worthwhile enterprise.
Author Contributions
JF drafted a first version of the manuscript, DD added further text and commented on the draft. JF finalized the manuscript.
Authors Note
After more than 40 years of controversial discussions between both authors, this is the first joint paper. We are happy to have done this now! We have found common ground!
Conflict of Interest Statement
The authors declare that the research was conducted in the absence of any commercial or financial relationships that could be construed as a potential conflict of interest.
Acknowledgments
The authors thank the Deutsche Forschungsgemeinschaft (DFG) for the continuous support of their research over many years. Thanks to Daniel Holt for his comments on validity issues, thanks to Julia Nolte who helped us by translating German text excerpts into readable English and helped us, together with Keri Hartman, to improve our style and grammar – thanks for that! We also thank the two reviewers for their helpful critical comments on earlier versions of this manuscript. Finally, we acknowledge financial support by Deutsche Forschungsgemeinschaft and Ruprecht-Karls-Universität Heidelberg within their funding programme Open Access Publishing .
1 The fMRI-paper from Anderson (2012) uses the term “complex problem solving” for tasks that do not fall in our understanding of CPS and is therefore excluded from this list.
- Alison L., van den Heuvel C., Waring S., Power N., Long A., O’Hara T., et al. (2013). Immersive simulated learning environments for researching critical incidents: a knowledge synthesis of the literature and experiences of studying high-risk strategic decision making. J. Cogn. Eng. Deci. Mak. 7 255–272. 10.1177/1555343412468113 [ CrossRef ] [ Google Scholar ]
- Anderson J. R. (2012). Tracking problem solving by multivariate pattern analysis and hidden markov model algorithms. Neuropsychologia 50 487–498. 10.1016/j.neuropsychologia.2011.07.025 [ PMC free article ] [ PubMed ] [ CrossRef ] [ Google Scholar ]
- Barth C. M., Funke J. (2010). Negative affective environments improve complex solving performance. Cogn. Emot. 24 1259–1268. 10.1080/02699930903223766 [ CrossRef ] [ Google Scholar ]
- Beckmann J. F., Goode N. (2014). The benefit of being naïve and knowing it: the unfavourable impact of perceived context familiarity on learning in complex problem solving tasks. Instruct. Sci. 42 271–290. 10.1007/s11251-013-9280-7 [ CrossRef ] [ Google Scholar ]
- Beghetto R. A., Kaufman J. C. (2007). Toward a broader conception of creativity: a case for “mini-c” creativity. Psychol. Aesthetics Creat. Arts 1 73–79. 10.1037/1931-3896.1.2.73 [ CrossRef ] [ Google Scholar ]
- Bennett R. E. (2011). Formative assessment: a critical review. Assess. Educ. Princ. Policy Pract. 18 5–25. 10.1080/0969594X.2010.513678 [ CrossRef ] [ Google Scholar ]
- Berry D. C., Broadbent D. E. (1984). On the relationship between task performance and associated verbalizable knowledge. Q. J. Exp. Psychol. 36 209–231. 10.1080/14640748408402156 [ CrossRef ] [ Google Scholar ]
- Blech C., Funke J. (2010). You cannot have your cake and eat it, too: how induced goal conflicts affect complex problem solving. Open Psychol. J. 3 42–53. 10.2174/1874350101003010042 [ CrossRef ] [ Google Scholar ]
- Brehmer B., Dörner D. (1993). Experiments with computer-simulated microworlds: escaping both the narrow straits of the laboratory and the deep blue sea of the field study. Comput. Hum. Behav. 9 171–184. 10.1016/0747-5632(93)90005-D [ CrossRef ] [ Google Scholar ]
- Buchner A. (1995). “Basic topics and approaches to the study of complex problem solving,” in Complex Problem Solving: The European Perspective , eds Frensch P. A., Funke J. (Hillsdale, NJ: Erlbaum; ), 27–63. [ Google Scholar ]
- Buchner A., Funke J. (1993). Finite state automata: dynamic task environments in problem solving research. Q. J. Exp. Psychol. 46A , 83–118. 10.1080/14640749308401068 [ CrossRef ] [ Google Scholar ]
- Clark C. (2012). The Sleepwalkers: How Europe Went to War in 1914 . London: Allen Lane. [ Google Scholar ]
- Csapó B., Funke J. (2017a). “The development and assessment of problem solving in 21st-century schools,” in The Nature of Problem Solving: Using Research to Inspire 21st Century Learning , eds Csapó B., Funke J. (Paris: OECD Publishing; ), 19–31. [ Google Scholar ]
- Csapó B., Funke J. (eds) (2017b). The Nature of Problem Solving. Using Research to Inspire 21st Century Learning. Paris: OECD Publishing. [ Google Scholar ]
- Danner D., Hagemann D., Holt D. V., Hager M., Schankin A., Wüstenberg S., et al. (2011a). Measuring performance in dynamic decision making. Reliability and validity of the Tailorshop simulation. J. Ind. Differ. 32 225–233. 10.1027/1614-0001/a000055 [ CrossRef ] [ Google Scholar ]
- Danner D., Hagemann D., Schankin A., Hager M., Funke J. (2011b). Beyond IQ: a latent state-trait analysis of general intelligence, dynamic decision making, and implicit learning. Intelligence 39 323–334. 10.1016/j.intell.2011.06.004 [ CrossRef ] [ Google Scholar ]
- Dew N., Read S., Sarasvathy S. D., Wiltbank R. (2009). Effectual versus predictive logics in entrepreneurial decision-making: differences between experts and novices. J. Bus. Ventur. 24 287–309. 10.1016/j.jbusvent.2008.02.002 [ CrossRef ] [ Google Scholar ]
- Dhami M. K., Mandel D. R., Mellers B. A., Tetlock P. E. (2015). Improving intelligence analysis with decision science. Perspect. Psychol. Sci. 10 753–757. 10.1177/1745691615598511 [ PubMed ] [ CrossRef ] [ Google Scholar ]
- Dillon J. T. (1982). Problem finding and solving. J. Creat. Behav. 16 97–111. 10.1002/j.2162-6057.1982.tb00326.x [ CrossRef ] [ Google Scholar ]
- Dörner D. (1975). Wie Menschen eine Welt verbessern wollten [How people wanted to improve a world]. Bild Der Wissenschaft 12 48–53. [ Google Scholar ]
- Dörner D. (1980). On the difficulties people have in dealing with complexity. Simulat. Gam. 11 87–106. 10.1177/104687818001100108 [ CrossRef ] [ Google Scholar ]
- Dörner D. (1996). The Logic of Failure: Recognizing and Avoiding Error in Complex Situations. New York, NY: Basic Books. [ Google Scholar ]
- Dörner D., Drewes U., Reither F. (1975). “Über das Problemlösen in sehr komplexen Realitätsbereichen,” in Bericht über den 29. Kongreß der DGfPs in Salzburg 1974 Band 1 , ed. Tack W. H. (Göttingen: Hogrefe; ), 339–340. [ Google Scholar ]
- Dörner D., Güss C. D. (2011). A psychological analysis of Adolf Hitler’s decision making as commander in chief: summa confidentia et nimius metus. Rev. Gen. Psychol. 15 37–49. 10.1037/a0022375 [ CrossRef ] [ Google Scholar ]
- Dörner D., Güss C. D. (2013). PSI: a computational architecture of cognition, motivation, and emotion. Rev. Gen. Psychol. 17 297–317. 10.1037/a0032947 [ CrossRef ] [ Google Scholar ]
- Dörner D., Kreuzig H. W., Reither F., Stäudel T. (1983). Lohhausen. Vom Umgang mit Unbestimmtheit und Komplexität. Bern: Huber. [ Google Scholar ]
- Ederer P., Patt A., Greiff S. (2016). Complex problem-solving skills and innovativeness – evidence from occupational testing and regional data. Eur. J. Educ. 51 244–256. 10.1111/ejed.12176 [ CrossRef ] [ Google Scholar ]
- Edwards W. (1962). Dynamic decision theory and probabiIistic information processing. Hum. Factors 4 59–73. 10.1177/001872086200400201 [ CrossRef ] [ Google Scholar ]
- Engelhart M., Funke J., Sager S. (2017). A web-based feedback study on optimization-based training and analysis of human decision making. J. Dynamic Dec. Mak. 3 1–23. [ Google Scholar ]
- Ericsson K. A., Simon H. A. (1983). Protocol Analysis: Verbal Reports As Data. Cambridge, MA: Bradford. [ Google Scholar ]
- Fischer A., Greiff S., Funke J. (2017). “The history of complex problem solving,” in The Nature of Problem Solving: Using Research to Inspire 21st Century Learning , eds Csapó B., Funke J. (Paris: OECD Publishing; ), 107–121. [ Google Scholar ]
- Fischer A., Holt D. V., Funke J. (2015). Promoting the growing field of dynamic decision making. J. Dynamic Decis. Mak. 1 1–3. 10.11588/jddm.2015.1.23807 [ CrossRef ] [ Google Scholar ]
- Fischer A., Holt D. V., Funke J. (2016). The first year of the “journal of dynamic decision making.” J. Dynamic Decis. Mak. 2 1–2. 10.11588/jddm.2016.1.28995 [ CrossRef ] [ Google Scholar ]
- Fischer A., Neubert J. C. (2015). The multiple faces of complex problems: a model of problem solving competency and its implications for training and assessment. J. Dynamic Decis. Mak. 1 1–14. 10.11588/jddm.2015.1.23945 [ CrossRef ] [ Google Scholar ]
- Frensch P. A., Funke J. (eds) (1995a). Complex Problem Solving: The European Perspective. Hillsdale, NJ: Erlbaum. [ Google Scholar ]
- Frensch P. A., Funke J. (1995b). “Definitions, traditions, and a general framework for understanding complex problem solving,” in Complex Problem Solving: The European Perspective , eds Frensch P. A., Funke J. (Hillsdale, NJ: Lawrence Erlbaum; ), 3–25. [ Google Scholar ]
- Frischkorn G. T., Greiff S., Wüstenberg S. (2014). The development of complex problem solving in adolescence: a latent growth curve analysis. J. Educ. Psychol. 106 1004–1020. 10.1037/a0037114 [ CrossRef ] [ Google Scholar ]
- Funke J. (1985). Steuerung dynamischer Systeme durch Aufbau und Anwendung subjektiver Kausalmodelle. Z. Psychol. 193 435–457. [ Google Scholar ]
- Funke J. (1986). Komplexes Problemlösen - Bestandsaufnahme und Perspektiven [Complex Problem Solving: Survey and Perspectives]. Heidelberg: Springer. [ Google Scholar ]
- Funke J. (1993). “Microworlds based on linear equation systems: a new approach to complex problem solving and experimental results,” in The Cognitive Psychology of Knowledge , eds Strube G., Wender K.-F. (Amsterdam: Elsevier Science Publishers; ), 313–330. [ Google Scholar ]
- Funke J. (1995). “Experimental research on complex problem solving,” in Complex Problem Solving: The European Perspective , eds Frensch P. A., Funke J. (Hillsdale, NJ: Erlbaum; ), 243–268. [ Google Scholar ]
- Funke J. (2010). Complex problem solving: a case for complex cognition? Cogn. Process. 11 133–142. 10.1007/s10339-009-0345-0 [ PubMed ] [ CrossRef ] [ Google Scholar ]
- Funke J. (2012). “Complex problem solving,” in Encyclopedia of the Sciences of Learning Vol. 38 ed. Seel N. M. (Heidelberg: Springer; ), 682–685. [ Google Scholar ]
- Funke J. (2014a). Analysis of minimal complex systems and complex problem solving require different forms of causal cognition. Front. Psychol. 5 : 739 10.3389/fpsyg.2014.00739 [ PMC free article ] [ PubMed ] [ CrossRef ] [ Google Scholar ]
- Funke J. (2014b). “Problem solving: what are the important questions?,” in Proceedings of the 36th Annual Conference of the Cognitive Science Society , eds Bello P., Guarini M., McShane M., Scassellati B. (Austin, TX: Cognitive Science Society; ), 493–498. [ Google Scholar ]
- Funke J., Fischer A., Holt D. V. (2017). When less is less: solving multiple simple problems is not complex problem solving—A comment on Greiff et al. (2015). J. Intell. 5 : 5 10.3390/jintelligence5010005 [ PMC free article ] [ PubMed ] [ CrossRef ] [ Google Scholar ]
- Funke J., Fischer A., Holt D. V. (2018). “Competencies for complexity: problem solving in the 21st century,” in Assessment and Teaching of 21st Century Skills , eds Care E., Griffin P., Wilson M. (Dordrecht: Springer; ), 3. [ Google Scholar ]
- Funke J., Greiff S. (2017). “Dynamic problem solving: multiple-item testing based on minimally complex systems,” in Competence Assessment in Education. Research, Models and Instruments , eds Leutner D., Fleischer J., Grünkorn J., Klieme E. (Heidelberg: Springer; ), 427–443. [ Google Scholar ]
- Gobert J. D., Kim Y. J., Pedro M. A. S., Kennedy M., Betts C. G. (2015). Using educational data mining to assess students’ skills at designing and conducting experiments within a complex systems microworld. Think. Skills Creat. 18 81–90. 10.1016/j.tsc.2015.04.008 [ CrossRef ] [ Google Scholar ]
- Goode N., Beckmann J. F. (2010). You need to know: there is a causal relationship between structural knowledge and control performance in complex problem solving tasks. Intelligence 38 345–352. 10.1016/j.intell.2010.01.001 [ CrossRef ] [ Google Scholar ]
- Gray W. D. (2002). Simulated task environments: the role of high-fidelity simulations, scaled worlds, synthetic environments, and laboratory tasks in basic and applied cognitive research. Cogn. Sci. Q. 2 205–227. [ Google Scholar ]
- Greiff S., Fischer A. (2013). Measuring complex problem solving: an educational application of psychological theories. J. Educ. Res. 5 38–58. [ Google Scholar ]
- Greiff S., Fischer A., Stadler M., Wüstenberg S. (2015a). Assessing complex problem-solving skills with multiple complex systems. Think. Reason. 21 356–382. 10.1080/13546783.2014.989263 [ CrossRef ] [ Google Scholar ]
- Greiff S., Stadler M., Sonnleitner P., Wolff C., Martin R. (2015b). Sometimes less is more: comparing the validity of complex problem solving measures. Intelligence 50 100–113. 10.1016/j.intell.2015.02.007 [ CrossRef ] [ Google Scholar ]
- Greiff S., Fischer A., Wüstenberg S., Sonnleitner P., Brunner M., Martin R. (2013a). A multitrait–multimethod study of assessment instruments for complex problem solving. Intelligence 41 579–596. 10.1016/j.intell.2013.07.012 [ CrossRef ] [ Google Scholar ]
- Greiff S., Holt D. V., Funke J. (2013b). Perspectives on problem solving in educational assessment: analytical, interactive, and collaborative problem solving. J. Problem Solv. 5 71–91. 10.7771/1932-6246.1153 [ CrossRef ] [ Google Scholar ]
- Greiff S., Wüstenberg S., Molnár G., Fischer A., Funke J., Csapó B. (2013c). Complex problem solving in educational contexts—something beyond g: concept, assessment, measurement invariance, and construct validity. J. Educ. Psychol. 105 364–379. 10.1037/a0031856 [ CrossRef ] [ Google Scholar ]
- Greiff S., Funke J. (2009). “Measuring complex problem solving: the MicroDYN approach,” in The Transition to Computer-Based Assessment. New Approaches to Skills Assessment and Implications for Large-Scale Testing , eds Scheuermann F., Björnsson J. (Luxembourg: Office for Official Publications of the European Communities; ), 157–163. [ Google Scholar ]
- Greiff S., Funke J. (2017). “Interactive problem solving: exploring the potential of minimal complex systems,” in The Nature of Problem Solving: Using Research to Inspire 21st Century Learning , eds Csapó B., Funke J. (Paris: OECD Publishing; ), 93–105. [ Google Scholar ]
- Greiff S., Martin R. (2014). What you see is what you (don’t) get: a comment on Funke’s (2014) opinion paper. Front. Psychol. 5 : 1120 10.3389/fpsyg.2014.01120 [ PMC free article ] [ PubMed ] [ CrossRef ] [ Google Scholar ]
- Greiff S., Neubert J. C. (2014). On the relation of complex problem solving, personality, fluid intelligence, and academic achievement. Learn. Ind. Diff. 36 37–48. 10.1016/j.lindif.2014.08.003 [ CrossRef ] [ Google Scholar ]
- Greiff S., Niepel C., Scherer R., Martin R. (2016). Understanding students’ performance in a computer-based assessment of complex problem solving: an analysis of behavioral data from computer-generated log files. Comput. Hum. Behav. 61 36–46. 10.1016/j.chb.2016.02.095 [ CrossRef ] [ Google Scholar ]
- Greiff S., Stadler M., Sonnleitner P., Wolff C., Martin R. (2017). Sometimes more is too much: a rejoinder to the commentaries on Greif et al. (2015). J. Intell. 5 : 6 10.3390/jintelligence5010006 [ PMC free article ] [ PubMed ] [ CrossRef ] [ Google Scholar ]
- Greiff S., Wüstenberg S. (2014). Assessment with microworlds using MicroDYN: measurement invariance and latent mean comparisons. Eur. J. Psychol. Assess. 1 1–11. 10.1027/1015-5759/a000194 [ CrossRef ] [ Google Scholar ]
- Greiff S., Wüstenberg S. (2015). Komplexer Problemlösetest COMPRO [Complex Problem-Solving Test COMPRO]. Mödling: Schuhfried. [ Google Scholar ]
- Greiff S., Wüstenberg S., Funke J. (2012). Dynamic problem solving: a new assessment perspective. Appl. Psychol. Measure. 36 189–213. 10.1177/0146621612439620 [ CrossRef ] [ Google Scholar ]
- Griffin P., Care E. (2015). “The ATC21S method,” in Assessment and Taching of 21st Century Skills , eds Griffin P., Care E. (Dordrecht, NL: Springer; ), 3–33. [ Google Scholar ]
- Güss C. D., Dörner D. (2011). Cultural differences in dynamic decision-making strategies in a non-linear, time-delayed task. Cogn. Syst. Res. 12 365–376. 10.1016/j.cogsys.2010.12.003 [ CrossRef ] [ Google Scholar ]
- Güss C. D., Tuason M. T., Orduña L. V. (2015). Strategies, tactics, and errors in dynamic decision making in an Asian sample. J. Dynamic Deci. Mak. 1 1–14. 10.11588/jddm.2015.1.13131 [ CrossRef ] [ Google Scholar ]
- Güss C. D., Wiley B. (2007). Metacognition of problem-solving strategies in Brazil, India, and the United States. J. Cogn. Cult. 7 1–25. 10.1163/156853707X171793 [ CrossRef ] [ Google Scholar ]
- Herde C. N., Wüstenberg S., Greiff S. (2016). Assessment of complex problem solving: what we know and what we don’t know. Appl. Meas. Educ. 29 265–277. 10.1080/08957347.2016.1209208 [ CrossRef ] [ Google Scholar ]
- Hermes M., Stelling D. (2016). Context matters, but how much? Latent state – trait analysis of cognitive ability assessments. Int. J. Sel. Assess. 24 285–295. 10.1111/ijsa.12147 [ CrossRef ] [ Google Scholar ]
- Hotaling J. M., Fakhari P., Busemeyer J. R. (2015). “Dynamic decision making,” in International Encyclopedia of the Social & Behavioral Sciences , 2nd Edn, eds Smelser N. J., Batles P. B. (New York, NY: Elsevier; ), 709–714. [ Google Scholar ]
- Hundertmark J., Holt D. V., Fischer A., Said N., Fischer H. (2015). System structure and cognitive ability as predictors of performance in dynamic system control tasks. J. Dynamic Deci. Mak. 1 1–10. 10.11588/jddm.2015.1.26416 [ CrossRef ] [ Google Scholar ]
- Jäkel F., Schreiber C. (2013). Introspection in problem solving. J. Problem Solv. 6 20–33. 10.7771/1932-6246.1131 [ CrossRef ] [ Google Scholar ]
- Jansson A. (1994). Pathologies in dynamic decision making: consequences or precursors of failure? Sprache Kogn. 13 160–173. [ Google Scholar ]
- Kaufman J. C., Beghetto R. A. (2009). Beyond big and little: the four c model of creativity. Rev. Gen. Psychol. 13 1–12. 10.1037/a0013688 [ CrossRef ] [ Google Scholar ]
- Knauff M., Wolf A. G. (2010). Complex cognition: the science of human reasoning, problem-solving, and decision-making. Cogn. Process. 11 99–102. 10.1007/s10339-010-0362-z [ PubMed ] [ CrossRef ] [ Google Scholar ]
- Kretzschmar A. (2017). Sometimes less is not enough: a commentary on Greiff et al. (2015). J. Intell. 5 : 4 10.3390/jintelligence5010004 [ PMC free article ] [ PubMed ] [ CrossRef ] [ Google Scholar ]
- Kretzschmar A., Neubert J. C., Wüstenberg S., Greiff S. (2016). Construct validity of complex problem solving: a comprehensive view on different facets of intelligence and school grades. Intelligence 54 55–69. 10.1016/j.intell.2015.11.004 [ CrossRef ] [ Google Scholar ]
- Kretzschmar A., Süß H.-M. (2015). A study on the training of complex problem solving competence. J. Dynamic Deci. Mak. 1 1–14. 10.11588/jddm.2015.1.15455 [ CrossRef ] [ Google Scholar ]
- Lee H., Cho Y. (2007). Factors affecting problem finding depending on degree of structure of problem situation. J. Educ. Res. 101 113–123. 10.3200/JOER.101.2.113-125 [ CrossRef ] [ Google Scholar ]
- Leutner D., Fleischer J., Wirth J., Greiff S., Funke J. (2012). Analytische und dynamische Problemlösekompetenz im Lichte internationaler Schulleistungsvergleichsstudien: Untersuchungen zur Dimensionalität. Psychol. Rundschau 63 34–42. 10.1026/0033-3042/a000108 [ CrossRef ] [ Google Scholar ]
- Luchins A. S. (1942). Mechanization in problem solving: the effect of einstellung. Psychol. Monogr. 54 1–95. 10.1037/h0093502 [ CrossRef ] [ Google Scholar ]
- Mack O., Khare A., Krämer A., Burgartz T. (eds) (2016). Managing in a VUCA world. Heidelberg: Springer. [ Google Scholar ]
- Mainert J., Kretzschmar A., Neubert J. C., Greiff S. (2015). Linking complex problem solving and general mental ability to career advancement: does a transversal skill reveal incremental predictive validity? Int. J. Lifelong Educ. 34 393–411. 10.1080/02601370.2015.1060024 [ CrossRef ] [ Google Scholar ]
- Mainzer K. (2009). Challenges of complexity in the 21st century. An interdisciplinary introduction. Eur. Rev. 17 219–236. 10.1017/S1062798709000714 [ CrossRef ] [ Google Scholar ]
- Meadows D. H., Meadows D. L., Randers J. (1992). Beyond the Limits. Vermont, VA: Chelsea Green Publishing. [ Google Scholar ]
- Meadows D. H., Meadows D. L., Randers J., Behrens W. W. (1972). The Limits to Growth. New York, NY: Universe Books. [ Google Scholar ]
- Meißner A., Greiff S., Frischkorn G. T., Steinmayr R. (2016). Predicting complex problem solving and school grades with working memory and ability self-concept. Learn. Ind. Differ. 49 323–331. 10.1016/j.lindif.2016.04.006 [ CrossRef ] [ Google Scholar ]
- Molnàr G., Greiff S., Wüstenberg S., Fischer A. (2017). “Empirical study of computer-based assessment of domain-general complex problem-solving skills,” in The Nature of Problem Solving: Using research to Inspire 21st Century Learning , eds Csapó B., Funke J. (Paris: OECD Publishing; ), 125–141. [ Google Scholar ]
- National Research Council (2011). Assessing 21st Century Skills: Summary of a Workshop. Washington, DC: The National Academies Press. [ PubMed ] [ Google Scholar ]
- Newell A., Shaw J. C., Simon H. A. (1959). A general problem-solving program for a computer. Comput. Automat. 8 10–16. [ Google Scholar ]
- Nisbett R. E., Wilson T. D. (1977). Telling more than we can know: verbal reports on mental processes. Psychol. Rev. 84 231–259. 10.1037/0033-295X.84.3.231 [ CrossRef ] [ Google Scholar ]
- OECD (2014). “PISA 2012 results,” in Creative Problem Solving: Students’ Skills in Tackling Real-Life problems , Vol. 5 (Paris: OECD Publishing; ). [ Google Scholar ]
- Osman M. (2010). Controlling uncertainty: a review of human behavior in complex dynamic environments. Psychol. Bull. 136 65–86. 10.1037/a0017815 [ PubMed ] [ CrossRef ] [ Google Scholar ]
- Osman M. (2012). The role of reward in dynamic decision making. Front. Neurosci. 6 : 35 10.3389/fnins.2012.00035 [ PMC free article ] [ PubMed ] [ CrossRef ] [ Google Scholar ]
- Qudrat-Ullah H. (2015). Better Decision Making in Complex, Dynamic Tasks. Training with Human-Facilitated Interactive Learning Environments. Heidelberg: Springer. [ Google Scholar ]
- Ramnarayan S., Strohschneider S., Schaub H. (1997). Trappings of expertise and the pursuit of failure. Simulat. Gam. 28 28–43. 10.1177/1046878197281004 [ CrossRef ] [ Google Scholar ]
- Reuschenbach B. (2008). Planen und Problemlösen im Komplexen Handlungsfeld Pflege. Berlin: Logos. [ Google Scholar ]
- Rohe M., Funke J., Storch M., Weber J. (2016). Can motto goals outperform learning and performance goals? Influence of goal setting on performance, intrinsic motivation, processing style, and affect in a complex problem solving task. J. Dynamic Deci. Mak. 2 1–15. 10.11588/jddm.2016.1.28510 [ CrossRef ] [ Google Scholar ]
- Scherer R., Greiff S., Hautamäki J. (2015). Exploring the relation between time on task and ability in complex problem solving. Intelligence 48 37–50. 10.1016/j.intell.2014.10.003 [ CrossRef ] [ Google Scholar ]
- Schoppek W., Fischer A. (2015). Complex problem solving – single ability or complex phenomenon? Front. Psychol. 6 : 1669 10.3389/fpsyg.2015.01669 [ PMC free article ] [ PubMed ] [ CrossRef ] [ Google Scholar ]
- Schraw G., Dunkle M., Bendixen L. D. (1995). Cognitive processes in well-defined and ill-defined problem solving. Appl. Cogn. Psychol. 9 523–538. 10.1002/acp.2350090605 [ CrossRef ] [ Google Scholar ]
- Schweizer F., Wüstenberg S., Greiff S. (2013). Validity of the MicroDYN approach: complex problem solving predicts school grades beyond working memory capacity. Learn. Ind. Differ. 24 42–52. 10.1016/j.lindif.2012.12.011 [ CrossRef ] [ Google Scholar ]
- Schweizer T. S., Schmalenberger K. M., Eisenlohr-Moul T. A., Mojzisch A., Kaiser S., Funke J. (2016). Cognitive and affective aspects of creative option generation in everyday life situations. Front. Psychol. 7 : 1132 10.3389/fpsyg.2016.01132 [ PMC free article ] [ PubMed ] [ CrossRef ] [ Google Scholar ]
- Selten R., Pittnauer S., Hohnisch M. (2012). Dealing with dynamic decision problems when knowledge of the environment is limited: an approach based on goal systems. J. Behav. Deci. Mak. 25 443–457. 10.1002/bdm.738 [ CrossRef ] [ Google Scholar ]
- Simon H. A. (1957). Administrative Behavior: A Study of Decision-Making Processes in Administrative Organizations , 2nd Edn New York, NY: Macmillan. [ Google Scholar ]
- Sonnleitner P., Brunner M., Keller U., Martin R. (2014). Differential relations between facets of complex problem solving and students’ immigration background. J. Educ. Psychol. 106 681–695. 10.1037/a0035506 [ CrossRef ] [ Google Scholar ]
- Spering M., Wagener D., Funke J. (2005). The role of emotions in complex problem solving. Cogn. Emot. 19 1252–1261. 10.1080/02699930500304886 [ CrossRef ] [ Google Scholar ]
- Stadler M., Becker N., Gödker M., Leutner D., Greiff S. (2015). Complex problem solving and intelligence: a meta-analysis. Intelligence 53 92–101. 10.1016/j.intell.2015.09.005 [ CrossRef ] [ Google Scholar ]
- Stadler M., Niepel C., Greiff S. (2016). Easily too difficult: estimating item difficulty in computer simulated microworlds. Comput. Hum. Behav. 65 100–106. 10.1016/j.chb.2016.08.025 [ CrossRef ] [ Google Scholar ]
- Sternberg R. J. (1995). “Expertise in complex problem solving: a comparison of alternative conceptions,” in Complex Problem Solving: The European Perspective , eds Frensch P. A., Funke J. (Hillsdale, NJ: Erlbaum; ), 295–321. [ Google Scholar ]
- Sternberg R. J., Frensch P. A. (1991). Complex Problem Solving: Principles and Mechanisms. (eds) Sternberg R. J., Frensch P. A. Hillsdale, NJ: Erlbaum. [ Google Scholar ]
- Strohschneider S., Güss C. D. (1998). Planning and problem solving: differences between brazilian and german students. J. Cross-Cult. Psychol. 29 695–716. 10.1177/0022022198296002 [ CrossRef ] [ Google Scholar ]
- Strohschneider S., Güss C. D. (1999). The fate of the Moros: a cross-cultural exploration of strategies in complex and dynamic decision making. Int. J. Psychol. 34 235–252. 10.1080/002075999399873 [ CrossRef ] [ Google Scholar ]
- Thimbleby H. (2007). Press On. Principles of Interaction. Cambridge, MA: MIT Press. [ Google Scholar ]
- Tobinski D. A., Fritz A. (2017). “EcoSphere: a new paradigm for problem solving in complex systems,” in The Nature of Problem Solving: Using Research to Inspire 21st Century Learning , eds Csapó B., Funke J. (Paris: OECD Publishing; ), 211–222. [ Google Scholar ]
- Tremblay S., Gagnon J.-F., Lafond D., Hodgetts H. M., Doiron M., Jeuniaux P. P. J. M. H. (2017). A cognitive prosthesis for complex decision-making. Appl. Ergon. 58 349–360. 10.1016/j.apergo.2016.07.009 [ PubMed ] [ CrossRef ] [ Google Scholar ]
- Tschirgi J. E. (1980). Sensible reasoning: a hypothesis about hypotheses. Child Dev. 51 1–10. 10.2307/1129583 [ CrossRef ] [ Google Scholar ]
- Tuchman B. W. (1984). The March of Folly. From Troy to Vietnam. New York, NY: Ballantine Books. [ Google Scholar ]
- Verweij M., Thompson M. (eds) (2006). Clumsy Solutions for A Complex World. Governance, Politics and Plural Perceptions. New York, NY: Palgrave Macmillan; 10.1057/9780230624887 [ CrossRef ] [ Google Scholar ]
- Viehrig K., Siegmund A., Funke J., Wüstenberg S., Greiff S. (2017). “The heidelberg inventory of geographic system competency model,” in Competence Assessment in Education. Research, Models and Instruments , eds Leutner D., Fleischer J., Grünkorn J., Klieme E. (Heidelberg: Springer; ), 31–53. [ Google Scholar ]
- von Clausewitz C. (1832). Vom Kriege [On war]. Berlin: Dämmler. [ Google Scholar ]
- Wendt A. N. (2017). The empirical potential of live streaming beyond cognitive psychology. J. Dynamic Deci. Mak. 3 1–9. 10.11588/jddm.2017.1.33724 [ CrossRef ] [ Google Scholar ]
- Wiliam D., Black P. (1996). Meanings and consequences: a basis for distinguishing formative and summative functions of assessment? Br. Educ. Res. J. 22 537–548. 10.1080/0141192960220502 [ CrossRef ] [ Google Scholar ]
- World Economic Forum (2015). New Vsion for Education Unlocking the Potential of Technology. Geneva: World Economic Forum. [ Google Scholar ]
- World Economic Forum (2016). Global Risks 2016: Insight Report , 11th Edn Geneva: World Economic Forum. [ Google Scholar ]
- Wüstenberg S., Greiff S., Funke J. (2012). Complex problem solving — more than reasoning? Intelligence 40 1–14. 10.1016/j.intell.2011.11.003 [ CrossRef ] [ Google Scholar ]
- Wüstenberg S., Greiff S., Vainikainen M.-P., Murphy K. (2016). Individual differences in students’ complex problem solving skills: how they evolve and what they imply. J. Educ. Psychol. 108 1028–1044. 10.1037/edu0000101 [ CrossRef ] [ Google Scholar ]
- Wüstenberg S., Stadler M., Hautamäki J., Greiff S. (2014). The role of strategy knowledge for the application of strategies in complex problem solving tasks. Technol. Knowl. Learn. 19 127–146. 10.1007/s10758-014-9222-8 [ CrossRef ] [ Google Scholar ]
How to master the seven-step problem-solving process
In this episode of the McKinsey Podcast , Simon London speaks with Charles Conn, CEO of venture-capital firm Oxford Sciences Innovation, and McKinsey senior partner Hugo Sarrazin about the complexities of different problem-solving strategies.
Podcast transcript
Simon London: Hello, and welcome to this episode of the McKinsey Podcast , with me, Simon London. What’s the number-one skill you need to succeed professionally? Salesmanship, perhaps? Or a facility with statistics? Or maybe the ability to communicate crisply and clearly? Many would argue that at the very top of the list comes problem solving: that is, the ability to think through and come up with an optimal course of action to address any complex challenge—in business, in public policy, or indeed in life.
Looked at this way, it’s no surprise that McKinsey takes problem solving very seriously, testing for it during the recruiting process and then honing it, in McKinsey consultants, through immersion in a structured seven-step method. To discuss the art of problem solving, I sat down in California with McKinsey senior partner Hugo Sarrazin and also with Charles Conn. Charles is a former McKinsey partner, entrepreneur, executive, and coauthor of the book Bulletproof Problem Solving: The One Skill That Changes Everything [John Wiley & Sons, 2018].
Charles and Hugo, welcome to the podcast. Thank you for being here.
Hugo Sarrazin: Our pleasure.
Charles Conn: It’s terrific to be here.
Simon London: Problem solving is a really interesting piece of terminology. It could mean so many different things. I have a son who’s a teenage climber. They talk about solving problems. Climbing is problem solving. Charles, when you talk about problem solving, what are you talking about?
Charles Conn: For me, problem solving is the answer to the question “What should I do?” It’s interesting when there’s uncertainty and complexity, and when it’s meaningful because there are consequences. Your son’s climbing is a perfect example. There are consequences, and it’s complicated, and there’s uncertainty—can he make that grab? I think we can apply that same frame almost at any level. You can think about questions like “What town would I like to live in?” or “Should I put solar panels on my roof?”
You might think that’s a funny thing to apply problem solving to, but in my mind it’s not fundamentally different from business problem solving, which answers the question “What should my strategy be?” Or problem solving at the policy level: “How do we combat climate change?” “Should I support the local school bond?” I think these are all part and parcel of the same type of question, “What should I do?”
I’m a big fan of structured problem solving. By following steps, we can more clearly understand what problem it is we’re solving, what are the components of the problem that we’re solving, which components are the most important ones for us to pay attention to, which analytic techniques we should apply to those, and how we can synthesize what we’ve learned back into a compelling story. That’s all it is, at its heart.
I think sometimes when people think about seven steps, they assume that there’s a rigidity to this. That’s not it at all. It’s actually to give you the scope for creativity, which often doesn’t exist when your problem solving is muddled.
Simon London: You were just talking about the seven-step process. That’s what’s written down in the book, but it’s a very McKinsey process as well. Without getting too deep into the weeds, let’s go through the steps, one by one. You were just talking about problem definition as being a particularly important thing to get right first. That’s the first step. Hugo, tell us about that.
Hugo Sarrazin: It is surprising how often people jump past this step and make a bunch of assumptions. The most powerful thing is to step back and ask the basic questions—“What are we trying to solve? What are the constraints that exist? What are the dependencies?” Let’s make those explicit and really push the thinking and defining. At McKinsey, we spend an enormous amount of time in writing that little statement, and the statement, if you’re a logic purist, is great. You debate. “Is it an ‘or’? Is it an ‘and’? What’s the action verb?” Because all these specific words help you get to the heart of what matters.
Want to subscribe to The McKinsey Podcast ?
Simon London: So this is a concise problem statement.
Hugo Sarrazin: Yeah. It’s not like “Can we grow in Japan?” That’s interesting, but it is “What, specifically, are we trying to uncover in the growth of a product in Japan? Or a segment in Japan? Or a channel in Japan?” When you spend an enormous amount of time, in the first meeting of the different stakeholders, debating this and having different people put forward what they think the problem definition is, you realize that people have completely different views of why they’re here. That, to me, is the most important step.
Charles Conn: I would agree with that. For me, the problem context is critical. When we understand “What are the forces acting upon your decision maker? How quickly is the answer needed? With what precision is the answer needed? Are there areas that are off limits or areas where we would particularly like to find our solution? Is the decision maker open to exploring other areas?” then you not only become more efficient, and move toward what we call the critical path in problem solving, but you also make it so much more likely that you’re not going to waste your time or your decision maker’s time.
How often do especially bright young people run off with half of the idea about what the problem is and start collecting data and start building models—only to discover that they’ve really gone off half-cocked.
Hugo Sarrazin: Yeah.
Charles Conn: And in the wrong direction.
Simon London: OK. So step one—and there is a real art and a structure to it—is define the problem. Step two, Charles?
Charles Conn: My favorite step is step two, which is to use logic trees to disaggregate the problem. Every problem we’re solving has some complexity and some uncertainty in it. The only way that we can really get our team working on the problem is to take the problem apart into logical pieces.
What we find, of course, is that the way to disaggregate the problem often gives you an insight into the answer to the problem quite quickly. I love to do two or three different cuts at it, each one giving a bit of a different insight into what might be going wrong. By doing sensible disaggregations, using logic trees, we can figure out which parts of the problem we should be looking at, and we can assign those different parts to team members.
Simon London: What’s a good example of a logic tree on a sort of ratable problem?
Charles Conn: Maybe the easiest one is the classic profit tree. Almost in every business that I would take a look at, I would start with a profit or return-on-assets tree. In its simplest form, you have the components of revenue, which are price and quantity, and the components of cost, which are cost and quantity. Each of those can be broken out. Cost can be broken into variable cost and fixed cost. The components of price can be broken into what your pricing scheme is. That simple tree often provides insight into what’s going on in a business or what the difference is between that business and the competitors.
If we add the leg, which is “What’s the asset base or investment element?”—so profit divided by assets—then we can ask the question “Is the business using its investments sensibly?” whether that’s in stores or in manufacturing or in transportation assets. I hope we can see just how simple this is, even though we’re describing it in words.
When I went to work with Gordon Moore at the Moore Foundation, the problem that he asked us to look at was “How can we save Pacific salmon?” Now, that sounds like an impossible question, but it was amenable to precisely the same type of disaggregation and allowed us to organize what became a 15-year effort to improve the likelihood of good outcomes for Pacific salmon.
Simon London: Now, is there a danger that your logic tree can be impossibly large? This, I think, brings us onto the third step in the process, which is that you have to prioritize.
Charles Conn: Absolutely. The third step, which we also emphasize, along with good problem definition, is rigorous prioritization—we ask the questions “How important is this lever or this branch of the tree in the overall outcome that we seek to achieve? How much can I move that lever?” Obviously, we try and focus our efforts on ones that have a big impact on the problem and the ones that we have the ability to change. With salmon, ocean conditions turned out to be a big lever, but not one that we could adjust. We focused our attention on fish habitats and fish-harvesting practices, which were big levers that we could affect.
People spend a lot of time arguing about branches that are either not important or that none of us can change. We see it in the public square. When we deal with questions at the policy level—“Should you support the death penalty?” “How do we affect climate change?” “How can we uncover the causes and address homelessness?”—it’s even more important that we’re focusing on levers that are big and movable.
Would you like to learn more about our Strategy & Corporate Finance Practice ?
Simon London: Let’s move swiftly on to step four. You’ve defined your problem, you disaggregate it, you prioritize where you want to analyze—what you want to really look at hard. Then you got to the work plan. Now, what does that mean in practice?
Hugo Sarrazin: Depending on what you’ve prioritized, there are many things you could do. It could be breaking the work among the team members so that people have a clear piece of the work to do. It could be defining the specific analyses that need to get done and executed, and being clear on time lines. There’s always a level-one answer, there’s a level-two answer, there’s a level-three answer. Without being too flippant, I can solve any problem during a good dinner with wine. It won’t have a whole lot of backing.
Simon London: Not going to have a lot of depth to it.
Hugo Sarrazin: No, but it may be useful as a starting point. If the stakes are not that high, that could be OK. If it’s really high stakes, you may need level three and have the whole model validated in three different ways. You need to find a work plan that reflects the level of precision, the time frame you have, and the stakeholders you need to bring along in the exercise.
Charles Conn: I love the way you’ve described that, because, again, some people think of problem solving as a linear thing, but of course what’s critical is that it’s iterative. As you say, you can solve the problem in one day or even one hour.
Charles Conn: We encourage our teams everywhere to do that. We call it the one-day answer or the one-hour answer. In work planning, we’re always iterating. Every time you see a 50-page work plan that stretches out to three months, you know it’s wrong. It will be outmoded very quickly by that learning process that you described. Iterative problem solving is a critical part of this. Sometimes, people think work planning sounds dull, but it isn’t. It’s how we know what’s expected of us and when we need to deliver it and how we’re progressing toward the answer. It’s also the place where we can deal with biases. Bias is a feature of every human decision-making process. If we design our team interactions intelligently, we can avoid the worst sort of biases.
Simon London: Here we’re talking about cognitive biases primarily, right? It’s not that I’m biased against you because of your accent or something. These are the cognitive biases that behavioral sciences have shown we all carry around, things like anchoring, overoptimism—these kinds of things.
Both: Yeah.
Charles Conn: Availability bias is the one that I’m always alert to. You think you’ve seen the problem before, and therefore what’s available is your previous conception of it—and we have to be most careful about that. In any human setting, we also have to be careful about biases that are based on hierarchies, sometimes called sunflower bias. I’m sure, Hugo, with your teams, you make sure that the youngest team members speak first. Not the oldest team members, because it’s easy for people to look at who’s senior and alter their own creative approaches.
Hugo Sarrazin: It’s helpful, at that moment—if someone is asserting a point of view—to ask the question “This was true in what context?” You’re trying to apply something that worked in one context to a different one. That can be deadly if the context has changed, and that’s why organizations struggle to change. You promote all these people because they did something that worked well in the past, and then there’s a disruption in the industry, and they keep doing what got them promoted even though the context has changed.
Simon London: Right. Right.
Hugo Sarrazin: So it’s the same thing in problem solving.
Charles Conn: And it’s why diversity in our teams is so important. It’s one of the best things about the world that we’re in now. We’re likely to have people from different socioeconomic, ethnic, and national backgrounds, each of whom sees problems from a slightly different perspective. It is therefore much more likely that the team will uncover a truly creative and clever approach to problem solving.
Simon London: Let’s move on to step five. You’ve done your work plan. Now you’ve actually got to do the analysis. The thing that strikes me here is that the range of tools that we have at our disposal now, of course, is just huge, particularly with advances in computation, advanced analytics. There’s so many things that you can apply here. Just talk about the analysis stage. How do you pick the right tools?
Charles Conn: For me, the most important thing is that we start with simple heuristics and explanatory statistics before we go off and use the big-gun tools. We need to understand the shape and scope of our problem before we start applying these massive and complex analytical approaches.
Simon London: Would you agree with that?
Hugo Sarrazin: I agree. I think there are so many wonderful heuristics. You need to start there before you go deep into the modeling exercise. There’s an interesting dynamic that’s happening, though. In some cases, for some types of problems, it is even better to set yourself up to maximize your learning. Your problem-solving methodology is test and learn, test and learn, test and learn, and iterate. That is a heuristic in itself, the A/B testing that is used in many parts of the world. So that’s a problem-solving methodology. It’s nothing different. It just uses technology and feedback loops in a fast way. The other one is exploratory data analysis. When you’re dealing with a large-scale problem, and there’s so much data, I can get to the heuristics that Charles was talking about through very clever visualization of data.
You test with your data. You need to set up an environment to do so, but don’t get caught up in neural-network modeling immediately. You’re testing, you’re checking—“Is the data right? Is it sound? Does it make sense?”—before you launch too far.
Simon London: You do hear these ideas—that if you have a big enough data set and enough algorithms, they’re going to find things that you just wouldn’t have spotted, find solutions that maybe you wouldn’t have thought of. Does machine learning sort of revolutionize the problem-solving process? Or are these actually just other tools in the toolbox for structured problem solving?
Charles Conn: It can be revolutionary. There are some areas in which the pattern recognition of large data sets and good algorithms can help us see things that we otherwise couldn’t see. But I do think it’s terribly important we don’t think that this particular technique is a substitute for superb problem solving, starting with good problem definition. Many people use machine learning without understanding algorithms that themselves can have biases built into them. Just as 20 years ago, when we were doing statistical analysis, we knew that we needed good model definition, we still need a good understanding of our algorithms and really good problem definition before we launch off into big data sets and unknown algorithms.
Simon London: Step six. You’ve done your analysis.
Charles Conn: I take six and seven together, and this is the place where young problem solvers often make a mistake. They’ve got their analysis, and they assume that’s the answer, and of course it isn’t the answer. The ability to synthesize the pieces that came out of the analysis and begin to weave those into a story that helps people answer the question “What should I do?” This is back to where we started. If we can’t synthesize, and we can’t tell a story, then our decision maker can’t find the answer to “What should I do?”
Simon London: But, again, these final steps are about motivating people to action, right?
Charles Conn: Yeah.
Simon London: I am slightly torn about the nomenclature of problem solving because it’s on paper, right? Until you motivate people to action, you actually haven’t solved anything.
Charles Conn: I love this question because I think decision-making theory, without a bias to action, is a waste of time. Everything in how I approach this is to help people take action that makes the world better.
Simon London: Hence, these are absolutely critical steps. If you don’t do this well, you’ve just got a bunch of analysis.
Charles Conn: We end up in exactly the same place where we started, which is people speaking across each other, past each other in the public square, rather than actually working together, shoulder to shoulder, to crack these important problems.
Simon London: In the real world, we have a lot of uncertainty—arguably, increasing uncertainty. How do good problem solvers deal with that?
Hugo Sarrazin: At every step of the process. In the problem definition, when you’re defining the context, you need to understand those sources of uncertainty and whether they’re important or not important. It becomes important in the definition of the tree.
You need to think carefully about the branches of the tree that are more certain and less certain as you define them. They don’t have equal weight just because they’ve got equal space on the page. Then, when you’re prioritizing, your prioritization approach may put more emphasis on things that have low probability but huge impact—or, vice versa, may put a lot of priority on things that are very likely and, hopefully, have a reasonable impact. You can introduce that along the way. When you come back to the synthesis, you just need to be nuanced about what you’re understanding, the likelihood.
Often, people lack humility in the way they make their recommendations: “This is the answer.” They’re very precise, and I think we would all be well-served to say, “This is a likely answer under the following sets of conditions” and then make the level of uncertainty clearer, if that is appropriate. It doesn’t mean you’re always in the gray zone; it doesn’t mean you don’t have a point of view. It just means that you can be explicit about the certainty of your answer when you make that recommendation.
Simon London: So it sounds like there is an underlying principle: “Acknowledge and embrace the uncertainty. Don’t pretend that it isn’t there. Be very clear about what the uncertainties are up front, and then build that into every step of the process.”
Hugo Sarrazin: Every step of the process.
Simon London: Yeah. We have just walked through a particular structured methodology for problem solving. But, of course, this is not the only structured methodology for problem solving. One that is also very well-known is design thinking, which comes at things very differently. So, Hugo, I know you have worked with a lot of designers. Just give us a very quick summary. Design thinking—what is it, and how does it relate?
Hugo Sarrazin: It starts with an incredible amount of empathy for the user and uses that to define the problem. It does pause and go out in the wild and spend an enormous amount of time seeing how people interact with objects, seeing the experience they’re getting, seeing the pain points or joy—and uses that to infer and define the problem.
Simon London: Problem definition, but out in the world.
Hugo Sarrazin: With an enormous amount of empathy. There’s a huge emphasis on empathy. Traditional, more classic problem solving is you define the problem based on an understanding of the situation. This one almost presupposes that we don’t know the problem until we go see it. The second thing is you need to come up with multiple scenarios or answers or ideas or concepts, and there’s a lot of divergent thinking initially. That’s slightly different, versus the prioritization, but not for long. Eventually, you need to kind of say, “OK, I’m going to converge again.” Then you go and you bring things back to the customer and get feedback and iterate. Then you rinse and repeat, rinse and repeat. There’s a lot of tactile building, along the way, of prototypes and things like that. It’s very iterative.
Simon London: So, Charles, are these complements or are these alternatives?
Charles Conn: I think they’re entirely complementary, and I think Hugo’s description is perfect. When we do problem definition well in classic problem solving, we are demonstrating the kind of empathy, at the very beginning of our problem, that design thinking asks us to approach. When we ideate—and that’s very similar to the disaggregation, prioritization, and work-planning steps—we do precisely the same thing, and often we use contrasting teams, so that we do have divergent thinking. The best teams allow divergent thinking to bump them off whatever their initial biases in problem solving are. For me, design thinking gives us a constant reminder of creativity, empathy, and the tactile nature of problem solving, but it’s absolutely complementary, not alternative.
Simon London: I think, in a world of cross-functional teams, an interesting question is do people with design-thinking backgrounds really work well together with classical problem solvers? How do you make that chemistry happen?
Hugo Sarrazin: Yeah, it is not easy when people have spent an enormous amount of time seeped in design thinking or user-centric design, whichever word you want to use. If the person who’s applying classic problem-solving methodology is very rigid and mechanical in the way they’re doing it, there could be an enormous amount of tension. If there’s not clarity in the role and not clarity in the process, I think having the two together can be, sometimes, problematic.
The second thing that happens often is that the artifacts the two methodologies try to gravitate toward can be different. Classic problem solving often gravitates toward a model; design thinking migrates toward a prototype. Rather than writing a big deck with all my supporting evidence, they’ll bring an example, a thing, and that feels different. Then you spend your time differently to achieve those two end products, so that’s another source of friction.
Now, I still think it can be an incredibly powerful thing to have the two—if there are the right people with the right mind-set, if there is a team that is explicit about the roles, if we’re clear about the kind of outcomes we are attempting to bring forward. There’s an enormous amount of collaborativeness and respect.
Simon London: But they have to respect each other’s methodology and be prepared to flex, maybe, a little bit, in how this process is going to work.
Hugo Sarrazin: Absolutely.
Simon London: The other area where, it strikes me, there could be a little bit of a different sort of friction is this whole concept of the day-one answer, which is what we were just talking about in classical problem solving. Now, you know that this is probably not going to be your final answer, but that’s how you begin to structure the problem. Whereas I would imagine your design thinkers—no, they’re going off to do their ethnographic research and get out into the field, potentially for a long time, before they come back with at least an initial hypothesis.

Want better strategies? Become a bulletproof problem solver
Hugo Sarrazin: That is a great callout, and that’s another difference. Designers typically will like to soak into the situation and avoid converging too quickly. There’s optionality and exploring different options. There’s a strong belief that keeps the solution space wide enough that you can come up with more radical ideas. If there’s a large design team or many designers on the team, and you come on Friday and say, “What’s our week-one answer?” they’re going to struggle. They’re not going to be comfortable, naturally, to give that answer. It doesn’t mean they don’t have an answer; it’s just not where they are in their thinking process.
Simon London: I think we are, sadly, out of time for today. But Charles and Hugo, thank you so much.
Charles Conn: It was a pleasure to be here, Simon.
Hugo Sarrazin: It was a pleasure. Thank you.
Simon London: And thanks, as always, to you, our listeners, for tuning into this episode of the McKinsey Podcast . If you want to learn more about problem solving, you can find the book, Bulletproof Problem Solving: The One Skill That Changes Everything , online or order it through your local bookstore. To learn more about McKinsey, you can of course find us at McKinsey.com.
Charles Conn is CEO of Oxford Sciences Innovation and an alumnus of McKinsey’s Sydney office. Hugo Sarrazin is a senior partner in the Silicon Valley office, where Simon London, a member of McKinsey Publishing, is also based.
Explore a career with us
Related articles.
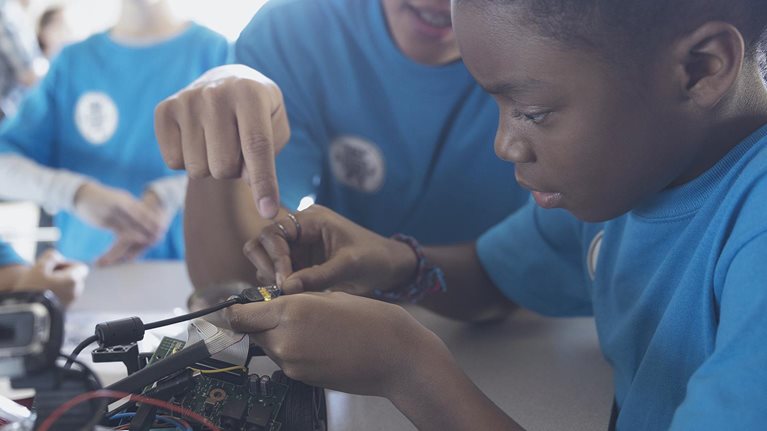
Strategy to beat the odds
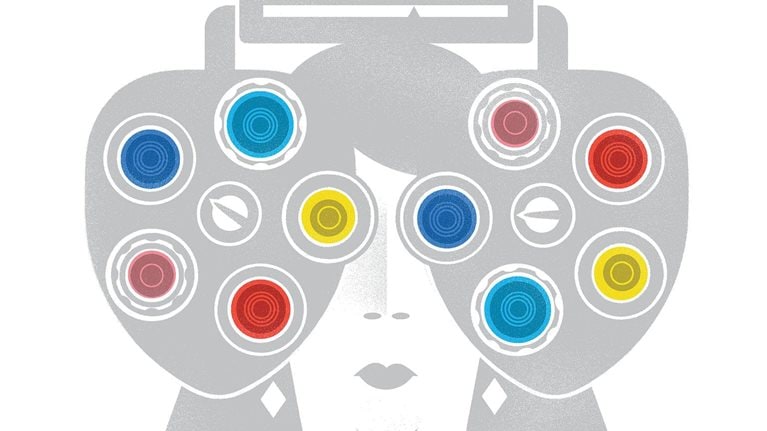
Five routes to more innovative problem solving

COMMENTS
Problem solving is an important skill in any work environment: it includes the ability to identify, understand, and develop solutions to complex issues while maintaining a focus on the end goal. Evaluating this skill in employees during performance reviews can be highly beneficial for both the employee and the organization. Questions that can help you...
Use these practical examples of phrases, sample comments, and templates for your performance review, 360-degree feedback survey, or manager appraisal.. The following examples not only relate to problem-solving but also conflict management, effective solutions, selecting the best alternatives, decision making, problem identification, analyzing effectively, and generally becoming an effective ...
A study from the World Economic Forum lists complex problem-solving as the top skill needed for the future. Still, it also highlights the importance of coordinating with others, emotional intelligence, and critical thinking. These aren't just add-ons but integral parts of the complex problem-solving process.
There is a slight increase in problem-solving performance with increased familiarity with the microworlds (Lotz et al., 2017) that could alter the tasks' difficulties as observed in the long version. In other words, the reduced opportunities to familiarize with the tasks might increase item difficulties for complex microworlds or microworlds ...
The approach helps drive logical systematic thinking for complex problem-solving, but should still be combined with creative brainstorming of alternative scenarios and solutions. ... Compare Current vs Expected Performance (Gap Analysis) This technique involves comparing the current state of performance, output, or results to the desired or ...
Phrases Examples: Fails to demonstrate basic problem-solving skills. Lacks the ability to analyze data and make informed decisions. Consistently overlooks important details, leading to poor choices. Fails to demonstrate basic analytical skills. Largely unable to accurately interpret data or discern patterns.
Ethics: 25 Performance Review Phrases Examples. He tends to apply a narrow and rushed decision-making approach. He avoid conflicts at work in relation to the decision making process. He struggles to work out a solution to any difficult problem. He is uncomfortable when faced with any awkward problem.
Complex problem solving is a collection of self-regulated psychological processes and activities necessary in dynamic environments to achieve ill-defined goals that cannot be reached by routine actions. ... You need to know: there is a causal relationship between structural knowledge and control performance in complex problem solving tasks ...
The second goal of the two studies presented in this paper was to investigate the relation between knowledge and complex problem solving performance. We attempted to measure knowledge about complex systems in several categories. We focused on declarative knowledge in the form of both system knowledge and action knowledge because assessing ...
The example interview responses are structured using the STAR method and are categorized into the top 5 key problem-solving skills recruiters look for in a candidate. 1. Analytical Thinking. Situation: In my previous role as a data analyst, our team encountered a significant drop in website traffic.
Complex problem solving is a series of observations and informed decisions used to find and implement a solution to a problem. Beyond finding and implementing a solution, complex problem solving also involves considering future changes to circumstance, resources and capabilities that may affect the trajectory of the process and success of the ...
In this paper, we describe an approach to understanding the data from complex performances based on evidence-centered design (Mislevy, Almond, & Lukas, in press), a methodology for devising assessments and for using the evidence observed in complex student performances to make inferences about proficiency. We use as an illustration the NAEP ...
He is co-founder of a research company focusing on developing tools to enhance individual, team and organization performance through better interaction. Post Share
Solutions are often more bricolage than perfect or optimal. The problem-solving process combines cognitive, emotional, and motivational aspects, particularly in high-stakes situations. Complex problems usually involve knowledge-rich requirements and collaboration among different persons.".
Definition and Importance. Problem solving is the process of finding solutions to obstacles or challenges you encounter in your life or work. It is a crucial skill that allows you to tackle complex situations, adapt to changes, and overcome difficulties with ease. Mastering this ability will contribute to both your personal and professional ...
Computer-based assessments of complex problem solving performance often take place in group settings like classrooms and computer laboratories. Such computer-based procedures provide an excellent opportunity to examine setting effects that might occur while participants are tested in a non-group session online at a time and place of their own ...
Share it on social media and in your performance review. Specialization - 4 course series. SOLVING COMPLEX PROBLEMS will teach you revolutionary new problem-solving skills. Involving lectures from over 50 experts from all faculties at Macquarie University, we look at solving complex problems in a way that has never been done before.
7. Solution evaluation. 1. Problem identification. The first stage of any problem solving process is to identify the problem (s) you need to solve. This often looks like using group discussions and activities to help a group surface and effectively articulate the challenges they're facing and wish to resolve.
4 steps to better problem solving. While it might be tempting to dive into a problem head first, take the time to move step by step. Here's how you can effectively break down the problem-solving process with your team: 1. Identify the problem that needs to be solved. One of the easiest ways to identify a problem is to ask questions.
Problem Solving: Use these examples for setting employee performance goals. Help your employees master this skill with 5 fresh ideas that drive change. Problem Solving is the skill of defining a problem to determine its cause, identify it, prioritize and select alternative solutions to implement in solving the problems and reviving relationships.
Go to: Computer-simulated scenarios have been part of psychological research on problem solving for more than 40 years. The shift in emphasis from simple toy problems to complex, more real-life oriented problems has been accompanied by discussions about the best ways to assess the process of solving complex problems.
In this episode of the McKinsey Podcast, Simon London speaks with Charles Conn, CEO of venture-capital firm Oxford Sciences Innovation, and McKinsey senior partner Hugo Sarrazin about the complexities of different problem-solving strategies.. Podcast transcript. Simon London: Hello, and welcome to this episode of the McKinsey Podcast, with me, Simon London.