We're sorry but you will need to enable Javascript to access all of the features of this site.

Stanford Online
Your guide to genetics and genomics.

The study of heredity has advanced considerably in recent years. Scientists’ understanding of the human genome has influenced advanced genetic testing methods that have moved physicians’ ability to diagnose and treat diseases forward.
As the collective knowledge of the human genome continues to become more sophisticated, genomics and genetics will play an increasingly important role in disease research.
Understanding genomics and genetics
Genetics and genomics are at the cutting edge of our understanding of human nature. While advancements in gene research have made the two branches of molecular biology virtually indistinguishable from one another, it’s still worth considering their differences to understand their usage and application.
Genetics refers to the scientific study of heredity — or, the study of how genes are passed from parents to offspring. The gene is the basic building block of heredity. It contains hundreds of sequences of DNA with instructions for creating proteins that govern various functions around the body.
Let’s take a look at the expression of green eyes at the genetic level to illustrate the point. The gene OCA2 — located in chromosome 15 — plays an important role in the pigmentation of eye color. OCA2 produces a protein known as P protein, which is involved in the production and storage of melanin. Melanin is the pigment that forms the basis for the coloration of the eyes.
A genetic variation in OCA2 will affect the amount of P protein that is produced, determining how much melanin is present — when there is more melanin, the individual will have darker eye color (like brown), while less melanin will lead to lighter eye colors (like green). It’s important to note that several other genes are also involved in eye color, and a single gene rarely acts completely on its own.
Genomics is a broader term that refers to the study of an individual’s entire genetic makeup — or genome — including the composition of each one of their genes as well as the external influences (including diet and environment) that influence the way those genes are expressed. The human genome is estimated to contain roughly 20,000 to 25,000 individual genes, according to the National Human Genome Research Institute.
Traditionally, genomics was considered distinct from genetics in that the latter focused on the individual proteins produced by a single set of genes, whereas researchers studying the former would instead take a broader view to genetics, examining the way multiple genes interact with one another to produce specific traits and characteristics.
While genetics and genomics are technically distinct, the advancement of gene research in recent years has increasingly led geneticists to use the terms interchangeably. Researchers are discovering that it’s virtually impossible to study a single gene without also studying other genes, environmental factors and other factors that influence the expression of a specific set of genes, making these two branches of molecular biology virtually synonymous.
Applying genetic and genomic testing to disease research
Genetics and genomics play a critical role in the study of human disease. Just as genes pass information about traits and characteristics from parent to offspring, they also inform an individual’s susceptibility to certain diseases and conditions.
For example, cystic fibrosis, Huntington’s disease and phenylketonuria are all inherited diseases that can be passed from parent to offspring, according to the National Human Genome Research Institute. It’s also believed that many lifestyle diseases — including obesity, heart disease, diabetes and certain types of cancers — have a genetic component.
For that reason, physicians have developed a series of genetic tests that examine an individual’s genome to help determine their genetic susceptibility to various diseases, both those with and without known cures.
According to Medline Plus, the most common types of genetic tests include:
- Molecular tests evaluate a specific gene (or set of genes) to identify possible changes in the chromosome to make a disease or cancer diagnosis. Molecular tests are helpful to physicians when trying to confirm a diagnosis with limited or conflicting information, like when an individual’s symptoms could point to numerous possible conditions.
- Chromosomal tests assess the entire chromosomal structure to pinpoint changes in the larger body that could point to a genetic disease. Certain conditions are the result of large-scale changes in an entire chromosome, including deletion or duplication of whole portions of the chromosome.
- Gene expression tests examine the activity of genes in certain cells and organs. Even when an individual contains a specific gene or set of genes, it’s not guaranteed that those genes will receive expression (i.e., an individual can have a genetic predisposition to diabetes but never actually get diabetes).
- Biochemical tests focus on measuring the amount and number of specific proteins found in the blood. Biochemical tests help determine whether certain genes are overproducing (or underproducing) various types of protein, which could make an individual susceptible to disease.
The use of genetic testing can help physicians identify the possibility of disease long before an individual actually becomes sick, helping patients take preventive wellness measures far in advance.
The future of genomics and genetics: What to expect
The study of genomics and genetics is rapidly advancing, meaning it will likely play an increasingly important role in human disease research and prevention. Some of the emerging trends in genomics and genetics include:
Direct-to-consumer genetic testing
As the technology underlying genetic testing becomes more sophisticated, some private companies are making direct-to-consumer (DTC) genetic tests more readily available. This gives patients easy access to genetic tests without having to go through a healthcare organization.
There is an inherent risk associated with DTC genetic tests. The U.S. Food and Drug Administration (FDA) notes that not all over-the-counter genetic tests can back their claims with empirical evidence, meaning consumers might be receiving results that are imprecise (or wholly incorrect). That could cause them to make ill-informed decisions about their health.
Gene therapy
The advancement of genetic testing has given clinicians a comprehensive depth of information regarding the genetic makeup of patients. Now, that information is being used to inform genetic therapeutic remedies aimed at preventing inherited diseases and conditions.
Gene therapy is a type of medical treatment that enables scientists to replace defective genes, introduce healthy genes or deactivate genes, all of which can help prevent or mitigate certain genetic conditions.
Gene therapy is now being used to treat a number of complex diseases that have perplexed clinicians for generations. In 2017, the FDA approved the first gene therapy in the United States, this one targeting leukemia, according to Science magazine. This particular gene therapy involved introducing a new protein to a patient’s T cells to encourage them to target harmful leukemia cancer cells.
Stay ahead of advancements in genetics and genomics with Stanford Online
Stanford Online’s Genetics and Genomics Programs aims to equip learners with the knowledge and skills they need to keep up with the recent trends in genetics and genomics research, helping them stay ahead in a rapidly advancing field of study.
Learn more about the Fundamentals of Genetics and Genomics and Advanced Topics in Genetics and Genomics Programs and sign up today.
- Engineering
- Artificial Intelligence
- Computer Science & Security
- Business & Management
- Energy & Sustainability
- Data Science
- Medicine & Health
- Explore All
- Technical Support
- Master’s Application FAQs
- Master’s Student FAQs
- Master's Tuition & Fees
- Grades & Policies
- Graduate Application FAQs
- Graduate Student FAQs
- Graduate Tuition & Fees
- Community Standards Review Process
- Academic Calendar
- Exams & Homework FAQs
- Enrollment FAQs
- Tuition, Fees, & Payments
- Custom & Executive Programs
- Free Online Courses
- Free Content Library
- School of Engineering
- Graduate School of Education
- Stanford Doerr School of Sustainability
- School of Humanities & Sciences
- Stanford Human Centered Artificial Intelligence (HAI)
- Graduate School of Business
- Stanford Law School
- School of Medicine
- Learning Collaborations
- Stanford Credentials
- What is a digital credential?
- Grades and Units Information
- Our Community
- Get Course Updates

An official website of the United States government
Here’s how you know
Official websites use .gov A .gov website belongs to an official government organization in the United States.
Secure .gov websites use HTTPS A lock ( Lock Locked padlock icon ) or https:// means you’ve safely connected to the .gov website. Share sensitive information only on official, secure websites.
New NIH study reveals shared genetic markers underlying substance use disorders
Breakthrough findings could lead to more effective prevention and treatment strategies for multiple substance use disorders
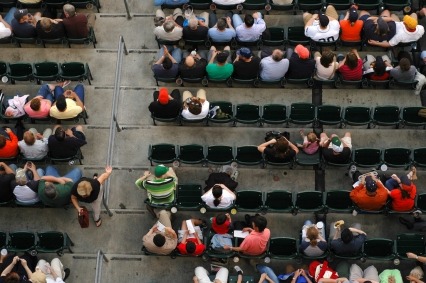
By combing through genomic data of over 1 million people, scientists have identified genes commonly inherited across addiction disorders, regardless of the substance being used. This dataset – one of the largest of its kind – may help reveal new treatment targets across multiple substance use disorders, including for people diagnosed with more than one. The findings also reinforce the role of the dopamine system in addiction, by showing that the combination of genes underlying addiction disorders was also associated with regulation of dopamine signaling.
Published today in Nature Mental Health , the study was led by researchers at the Washington University in St. Louis, along with more than 150 coauthors from around the world. It was supported by the National Institute on Drug Abuse (NIDA), the National Institute on Alcohol Abuse and Alcoholism (NIAAA), the National Institute of Mental Health (NIMH), the Eunice Kennedy Shriver National Institute of Child Health and Human Development, and the National Institute on Aging.
There has been limited knowledge of the molecular genetic underpinnings of addiction until now. Further, most clinical trials and behavioral studies have focused on individual substances, rather than addiction more broadly.
“Genetics play a key role in determining health throughout our lives, but they are not destiny. Our hope with genomic studies is to further illuminate factors that may protect or predispose a person to substance use disorders – knowledge that can be used to expand preventative services and empower individuals to make informed decisions about drug use,” said NIDA Director, Nora Volkow, M.D. “A better understanding of genetics also brings us one step closer to developing personalized interventions that are tailored to an individual’s unique biology, environment, and lived experience in order to provide the most benefits.”
In 2021, more than 46 million people in the United States aged 12 or older had at least one substance use disorder , and only 6.3% had received treatment. Moreover, people who use drugs are facing an increasingly dangerous drug supply, now often tainted with fentanyl. Approximately 107,000 people died of drug overdoses in 2021, and 37% of these deaths involved simultaneous exposure to both opioids and stimulant drugs. Drug use and addiction represent a public health crisis, characterized by high social, emotional, and financial costs to families, communities, and society.
Substance use disorders are heritable and influenced by complex interactions among multiple genes and environmental factors. In recent decades, a data-rich method, called genome-wide association, has emerged to try to identify specific genes involved in certain disorders. This method involves searching entire genomes for regions of genetic variation, called single-nucleotide polymorphisms (SNPs), that associate with the same disease, disorder, condition, or behavior among multiple people.
In this study, researchers used this method to pinpoint areas in the genome associated with general addiction risk, as well as the risk of specific substance use disorders – namely, alcohol, nicotine, cannabis, and opioid use disorders – in a sample of 1,025,550 individuals with genes indicating European ancestry and 92,630 individuals with genes indicating African ancestry.
“Using genomics, we can create a data-driven pipeline to prioritize existing medications for further study and improve chances of discovering new treatments. To do this accurately, it’s critical that the genetic evidence we gather includes globally representative populations and that we have members of communities historically underrepresented in biomedical research leading and contributing to these kinds of studies,” said Alexander Hatoum, Ph.D., a research assistant professor at Washington University in St. Louis and lead author of the study.
Dr. Hatoum and the research team discovered various molecular patterns underlying addiction, including 19 independent SNPs significantly associated with general addiction risk and 47 SNPs for specific substance disorders among the European ancestry sample. The strongest gene signals consistent across the various disorders mapped to areas in the genome known to control regulation of dopamine signaling, suggesting that genetic variation in dopamine signaling regulation, rather than in dopamine signaling itself, is central to addiction risk.
Compared to other genetic predictors, the genomic pattern identified here was also a more sensitive predictor of having two or more substance use disorders at once. The genomic pattern linked to general addiction risk also predicted higher risk of mental and physical illness, including psychiatric disorders, suicidal behavior, respiratory disease, heart disease, and chronic pain conditions. In children aged 9 or 10 years without any experience of substance use, these genes correlated with parental substance use and externalizing behavior. Compared to other genetic predictors, the genomic pattern identified here was also a more sensitive predictor of having two or more substance use disorders at once.
“Substance use disorders and mental disorders often co-occur, and we know that the most effective treatments help people address both issues at the same time. The shared genetic mechanisms between substance use and mental disorders revealed in this study underscore the importance of thinking about these disorders in tandem,” said NIMH Director Joshua A. Gordon, M.D., Ph.D.
Genomic analysis in the African ancestry sample revealed one SNP associated with general addiction risk and one substance-specific SNP for risk of alcohol use disorder. The dearth of findings here underscores ongoing disparities in data inclusion of globally representative populations that must be addressed in order to ensure data robustness and accuracy, Dr. Hatoum and co-authors note.
The inclusion of data from different ancestral groups in this study cannot and should not be used to assign or categorize variable genetic risk for substance use disorder to specific populations. As genetic information is used to better understand human health and health inequities, expansive and inclusive data collection is essential. NIDA and other Institutes at NIH supported a recently released report on responsible use and interpretation of population-level genomic data, by the National Academies of Sciences, Engineering, and Medicine. See also a corresponding statement from the NIH.
While Hatoum and colleagues have identified a genetic pattern indicating broad addiction risk, they note that substance use-specific diagnoses still have meaning. “The current study validates previous findings of alcohol-specific risk variants, and, importantly, makes this finding in a very large and more diverse study population, said NIAAA Director George F. Koob, Ph.D. “The finding of shared genetic risk variants across different substance use disorders provides insight into some of the mechanisms that underlie these disorders and the relationships with other mental health conditions. Together the findings of alcohol-specific risk variants and common addiction-related variants provide powerful support for individualized prevention and treatment.”
For more information on substance and mental health treatment programs in your area, call the free and confidential National Helpline 1-800-662-HELP (4357) or visit www.FindTreatment.gov .
This study was supported by multiple grants: NIDA ( T32DA007261 , DA054869 , R01DA054750 , K02DA032573 , U01DA055367 , K01DA051759 , DP1DA054394 , R33DA047527 ); NIAAA ( K01AA030083 , R21AA027827 , R01AA027522 , F31AA029934 , T32AA028259 ); NIMH ( K23MH121792 , T32MH014276 , R01MH120219 ); NIA ( RF1AG073593 , P30AG066614 ); NICHD ( P2CHD042849 )
- AS Hatoum, et al. Multivariate genome-wide association meta-analysis of over 1 million subjects identifies loci underlying multiple substance use disorders . Nature Mental Health. DOI: 10.1038/s44220-023-00034-y (2023).
About the National Institute on Drug Abuse (NIDA): NIDA is a component of the National Institutes of Health, U.S. Department of Health and Human Services. NIDA supports most of the world’s research on the health aspects of drug use and addiction. The Institute carries out a large variety of programs to inform policy, improve practice, and advance addiction science. For more information about NIDA and its programs, visit https://nida.nih.gov .
About the National Institute on Alcohol Abuse and Alcoholism (NIAAA): The National Institute on Alcohol Abuse and Alcoholism, part of the National Institutes of Health, is the primary U.S. agency for conducting and supporting research on the causes, consequences, prevention, and treatment of alcohol use disorder. NIAAA also disseminates research findings to general, professional, and academic audiences. Additional alcohol research information and publications are available at: https://www.niaaa.nih.gov .
About the National Institute of Mental Health (NIMH): The mission of NIMH is to transform the understanding and treatment of mental illnesses through basic and clinical research, paving the way for prevention, recovery, and cure. For more information, visit https://www.nimh.nih.gov .
About the National Institutes of Health (NIH) : NIH, the nation’s medical research agency, includes 27 Institutes and Centers and is a component of the U.S. Department of Health and Human Services. NIH is the primary federal agency conducting and supporting basic, clinical, and translational medical research, and is investigating the causes, treatments, and cures for both common and rare diseases. For more information about NIH and its programs, visit https://www.nih.gov .
About substance use disorders: Substance use disorders are chronic, treatable conditions from which people can recover. In 2021, over 46 million people in the United States had at least one substance use disorder. Substance use disorders are defined in part by continued use of substances despite negative consequences. They are also relapsing conditions, in which periods of abstinence (not using substances) can be followed by a return to use. Stigma can make individuals with substance use disorders less likely to seek treatment. Using preferred language can help accurately report on substance use and addiction. View NIDA’s online guide .
NIH…Turning Discovery Into Health ®
Related Articles
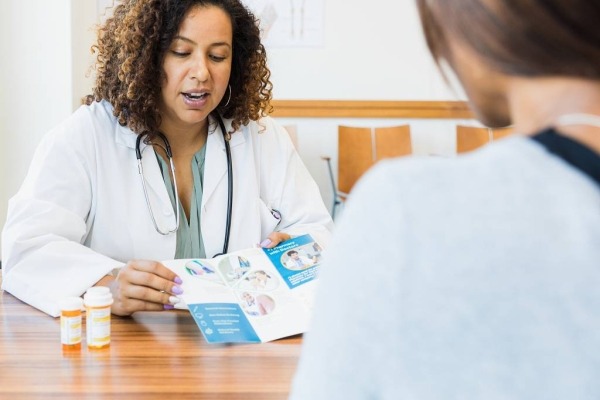
Federal study examines care following nonfatal overdose among medicare beneficiaries; identifies effective interventions and gaps in care
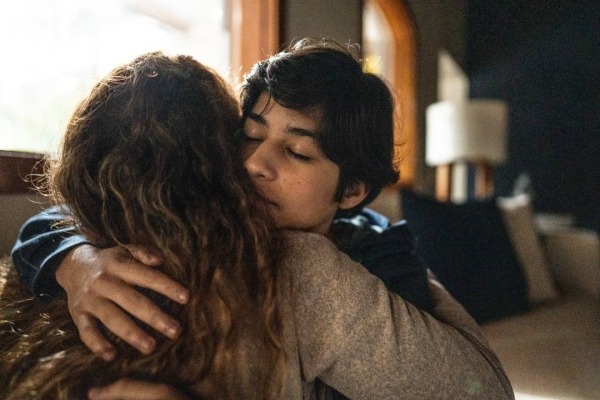
NIH-funded intervention did not impact opioid-related overdose death rates over evaluation period
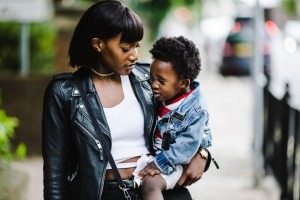
Analysis of social media language using AI models predicts depression severity for white Americans, but not Black Americans
- Seeking Patient Care?
- John P. Hussman Institute for Human Genomics (HIHG)
- Education and Training
- Division of Genomic Outreach
- Genetics Awareness Project
- What is Genetics?
What is Genetic Research?
Genetic research is the study of human DNA to find out what genes and environmental factors contribute to diseases. If we find out what causes disease, we can better detect disease, better treat disease and hopefully even prevent disease from happening in the first place!
Nearly every disease we know of has a genetic component. Depending on the disease, the genetic contribution may be very large, very small, or, most often, somewhere in between. Researchers are studying nearly every disease to find out how genetic factors may contribute. We inherit our genes from our parents. Genes tell our bodies how to develop and function. There are about 25,000 genes that make the things our bodies need to develop and work. Each gene is made up of DNA. DNA is a very long code that uses only 4 letters. We know that variations in this code can alter the way a gene works. Sometimes the variation is in only one letter. This is referred to as a SNP. Most SNPs don’t cause problems. They either contribute to the normal differences from one person to another, or have no known effect at all. However, a small number of SNPs can alter the way the gene works and contribute to disease. Sometimes the DNA code is altered by pieces that are missing or are extra. The missing or extra pieces may be as small as a one letter or as large as thousands. Depending on what is missing or extra, this may also contribute to health issues.
Research involving families
Diseases such as autism, Alzheimer disease, heart disease, and cancer are common. Approximately 1 out of every 110 children is affected by autism, 1 in 10 adults will develop Alzheimer disease and 1 in 3 adults will develop cancer in their lifetime. Since these are common diseases, we expect to see that sometimes more than one person in a family may have the condition. However, when a family health history is taken, some families have a much higher number of people with a certain complex disease than other families do. For example, imagine a family in which a great-grandmother, grandfather, and mother (three generations) were all affected by Alzheimer disease. We known that closely related members of a family share many of their genes. In fact, we share half our genes with our parents, brothers and sisters, and children! It is likely that there are genes shared by members of that family that are involved in the development of Alzheimer disease. By involving this family in genetic research, scientists can learn which genetic differences are shared by the family members who have Alzheimer disease, but not found in others in the family. These genetic differences narrow down the region where a gene might be. This technique of finding genes using a family is called a family study, or linkage analysis.
Research involving large groups of people
Another way of doing genetic research is to do DNA testing on two large groups of people. One group of people has the disease, and the other does not. Those who do not are sometimes referred to as controls. Most often, the people in this type of study are not related to one another. This technique is called association. Association studies rely on the use of thousands to millions of SNPs (described above) in our DNA. If you could imagine our DNA as huge roadmap, SNPs would be like mile markers on the highway. Scientists know the location of millions of SNPs throughout human DNA. Scientists also know that at the location of these SNPs, there is variation in the DNA code from one person to the next. For example, at one SNP, one person may have a letter T (thymine), while another person has a G (guanine). If a particular DNA SNP is seen more often in those with a disorder, such as autism or Alzheimer disease, it is possible that this SNP is associated with or is near an area of DNA that is associated with the disorder. Association studies are very large projects and require the participation of hundreds to, preferably, thousands of people with a disease and without a disease.
Research involving candidate genes
When trying to find genes that contribute to a disorder, sometimes researchers will look specifically at candidate genes. Candidate genes are those that researchers think might be involved in a disorder either because of what they do, and/or because they are located in an area of the DNA that looks interesting based on family studies or association studies described above. For example, some of the candidate genes that have been looked at for autism are known to be involved in behavior or language.
Diversity in genetic research is crucial!
It is important for all ethnic groups to be represented in genetic research. This is because people of the same ethnic group share many of the same changes and variations in their DNA with each other that they may not share with people of a different ethnic group. If only one ethnic group is involved in genetic research, we learn only about the variations in DNA that are associated with disease in that particular ethnic group.
When genetic research searching for genes involved in breast cancer was just beginning, most women who participated in genetic research studies were Caucasian. That research lead to the discovery of two very important genes (BRCA1 and BRCA2) that are now known to put women who have mutations in these genes at high risk for breast and ovarian cancer. It was found out that many mutations in the BRCA genes can lead to a high risk for cancer. However, only those mutations that were common in the Caucasian population were discovered through the initial research projects. This meant that when a non-Caucasian woman was tested for mutations in the BRCA genes by her doctor, there was a high chance that the results would not be conclusive. After many years of additional research, genetic testing for breast cancer has greatly improved for non-Caucasian women and testing of these genes is now more beneficial for them. By including all ethnic groups in genetic research, all ethnic groups can benefit from the findings of genetic research.
How to get involved in genetic research
At the John P. Hussman Institute for Human Genomics at the University of Miami’s Miller School of Medicine, our research efforts are aimed to ensure that all people, regardless of race or ethnicity, are able to benefit from the research that is done at our institute. Our researchers invite participation from the diverse South Florida community as well as larger national and international communities. Participation in a research study at the HIHG is completely voluntary. While details may vary from study to study, trained members of our team will conduct family history interviews and diagnostic evaluations. In addition, our team members will retrieve a blood or saliva sample. Some research studies ask for one-time participation only, while others request that participants are involved in several appointments. There is no cost or payment for your participation. We maintain the highest standards of confidentiality for all participants and families. Your private information is not given out to other researchers or companies. Your participation will help us to better understand the genetic and environmental causes of diseases and disorders, which may eventually lead to improved treatments and prevention methods, and hopefully a cure. Some research studies offer compensation for participation.
To learn more about the individual research studies ongoing at the HIHG, please visit the HIHG website (www.hihg.org). If you are interested in participating in one of our research projects, please visit the HIHG website or contact us:
Email : [email protected] Telephone : 1-877-686-6444 Fax : 305-243-2396
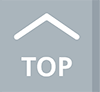
Genomic Studies
Our research.
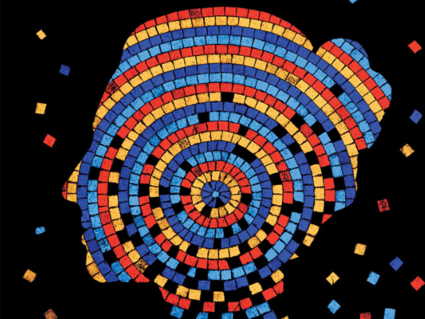
Inherited Factors and Mosaic Loss of X
NCI Media Advisory summarizes research on the influence of inherited factors on mLOX.
DCEG investigates the biological basis of inherited and acquired genetic variants associated with cancer susceptibility, utilizing genome-wide association studies, candidate gene studies, exome sequencing, and genetic mosaicism studies. DCEG scientists and their collaborators employ an array of advanced statistical methods to support these studies.
Genetic Mosaicism Studies
Genetic mosaicism results from an acquired DNA mutation that is present in only some of the body's cells. DCEG investigators are exploring the mechanisms that initiate and select for mosaic alterations and seek to elucidate how genetic mosaicism may serve as an intermediate between normal and disease states. Read more about genetic mosaicism studies.
Genome-wide Association Studies
A genome-wide association study (GWAS) is an approach that involves scanning the genomes from many different people and looking for genetic markers that can be used to predict the presence of a disease. The goal is to understand how genes contribute to the disease and to use that understanding to help develop better prevention and treatment strategies. DCEG has developed a robust research program with GWAS for a number of cancers, and more recently, exposures and survival.
Read more about genome-wide association studies .
Resources for GWAS Studies
The PLCO Atlas is an interactive tool that enables researchers to search for, visualize, and download aggregated association results from the Prostate, Lung, Colorectal, Ovarian (PLCO) Cancer Screening Study GWAS. The resource includes a comprehensive list of over 200 phenotypes collected from questionnaire data, linkage to medical records, cancer registries, and the National Death Index. Tools are available to visualize association results by interactive Manhattan plots that allow for stratification by sex as well as other diagnostic plots (e.g., Q-q plots) and resources to compare genetic correlations across phenotypes. Furthermore, descriptive characteristics of PLCO, annotation of phenotypes, and links to other pertinent genomic resources are available.
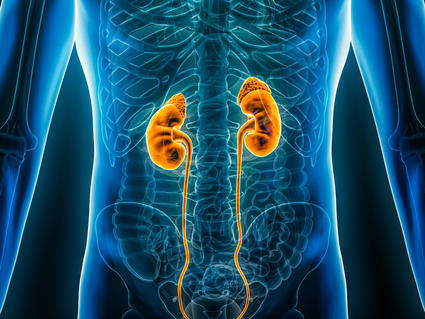
New Genomic Regions Associated with Kidney Cancer Risk
Study offers insights into the role genetics may play in the risk of developing kidney cancer.
In addition, investigators have developed a number of tools and resources that are made available to the scientific community for download and use. Explore genetic analysis software developed by DCEG investigators.
Statistical Methods
DCEG investigators collaborate with scientists at the Cancer Genomics Research (CGR) Laboratory to formulate design and analysis strategies in support of genetic association. Such strategies include determining the number of SNPs to be followed in various stages of multistage GWAS; choosing association test statistics; analyzing and adjusting for population stratification using principal component methods; conducting haplotype-based association scans; and exploring genetic pathways and interactions.
Video Tour of the Laboratory of Translational Genomics
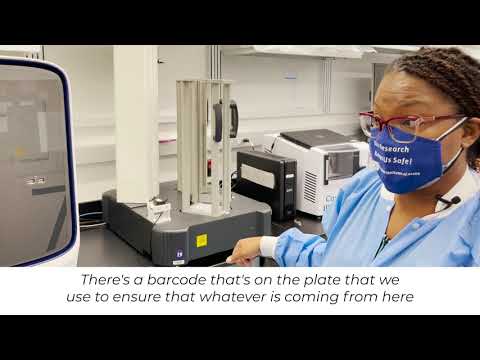
Touring an NCI Lab (Video)
What do you think? Leave a respectful comment.

Nsikan Akpan Nsikan Akpan
- Copy URL https://www.pbs.org/newshour/science/there-is-no-gay-gene-there-is-no-straight-gene-sexuality-is-just-complex-study-confirms
There is no ‘gay gene.’ There is no ‘straight gene.’ Sexuality is just complex, study confirms
There is no single gene responsible for a person being gay or a lesbian.
That’s the first thing you need to know about the largest genetic investigation of sexuality ever, which was published Thursday in Science . The study of nearly a half million people closes the door on the debate around the existence of a so-called “gay gene.”
In its stead, the report finds that human DNA cannot predict who is gay or heterosexual. Sexuality cannot be pinned down by biology, psychology or life experiences, this study and others show, because human sexual attraction is decided by all these factors.
“This is not a first study exploring the genetics of same-sex behavior, but the previous studies were small and underpowered,” Andrea Ganna, the study’s co-author and genetics research fellow at the Broad Institute and Mass General Hospital, said in a press briefing on Wednesday. “Just to give you a sense of the scale of [our] data, this is approximately 100 times bigger than any previous study on this topic.”
The study shows that genes play a small and limited role in determining sexuality. Genetic heritability — all of the information stored in our genes and passed between generations — can only explain 8 to 25 percent of why people have same-sex relations, based on the study’s results.
Moreover, the researchers found that sexuality is polygenic — meaning hundreds or even thousands of genes make tiny contributions to the trait. That pattern is similar to other heritable (but complex) characteristics like height or a proclivity toward trying new things . (Things like red/green colorblindness, freckles and dimples can be traced back to single genes ). But polygenic traits can be strongly influenced by the environment, meaning there’s no clear winner in this “nature versus nurture” debate.
It is worth keeping in mind that this study only covers some types of sexuality — gay, lesbian and cis-straight — but doesn’t offer many insights into gender identity. In other words, the team only looked at the “LGB” within the acronym LGBTQIA+.
Of course, ethical concerns arise with any attempt to use biology to explain complex human behavior like sexuality. People like Michael Bailey, a psychologist at Northwestern University who conducted much of the early research into the heritability of sexuality, warned against taking this new genetics study — or any research on sexual behavior — out of context.
For instance, Bailey added, there is no evidence that things like conversion therapy work.
“Obviously, there are environmental causes of sexual orientation. We knew that before this study.” said Bailey, citing the well-defined role that life experiences play in sexual development. “But that doesn’t mean we know how to manipulate sexual orientation mentally.”
What the scientists did
The study set out to investigate a 20-year-old genetics debate in sexuality by combing through two huge collections of DNA profiles: the UK Biobank and 23andMe.
With a reported 9 million users in its database, 23andMe is arguably the most popular, direct-to-consumer DNA testing company on the planet. The UK Biobank was established in 2007 by the medical charity The Wellcome Trust as a resource for research. It contains the DNA sequences of 500,000 middle-aged people, who were 40 to 69 years old when they were recruited between 2006 and 2010.
This study pulled the information for 477,500 people across the UK Biobank and 23andMe who had taken a survey about various life behaviors, including whether they had engaged in a sexual experience with a person of the same sex at any point in their life. About 26,800 individuals — or 5 percent of the subjects — fit this description, which is similar to the percentage reported across society more generally. All of the subjects consented to this research, including those pulled from 23andMe’s archives.
With this genetic trove available, the researchers conducted what’s known as a genome-wide association study, or GWAS. As the PBS NewsHour has reported previously, a GWAS study scans the DNA of hundreds or thousands of individuals, looking for common patterns that correspond with our health or our behaviors.
Think of all of humanity as consisting of 7 billion copies of the same book. All humans contain the same words — or individual genes — that make up how we think and how our organs function.
But the words in our respective genetic books — or their code — look slightly different. Some of my letters might be red, while some of yours are colored blue. They vary, which explains why we don’t look exactly the same nor have the same health.
This may sound counterintuitive, but those variations can also share similarities. The books that make up my family look similar to each other — in this example, they contain other shades of red.
The same applies if two people have the same height or if we’re both bald or if we’re depressed. These genetic patterns look more similar among myriad types of groupings and that’s what GWAS hunts down.
The technique can be used to suss out why certain people (and their particular genetic variations) correlate with health conditions like autism , physical traits like curly hair or colorblindness, behaviors like handedness or emotions like loneliness.
What they found
This GWAS study found that, like with many human behaviors, sexuality doesn’t have a strong genetic backing.
When the team looked for DNA patterns that had strong correlations, they found that no one gene could account for any more than 1 percent of people’s sexuality. The strongest signals came from five random genes.
Two of those genes correlated with same-sex sexuality in males, one of which is known to influence the sense of smell. One gene cropped up for females and two others showed solid patterns in both males and females. But their individual scores never passed this 1-percent mark — meaning they are all minor contributors to same-sex sexual behavior.
When the team looked more broadly across all the genomes — across the thousands of genes that they screened for the nearly 500,000 subjects — the genes similarities they found could only account for 8 to 25 percent of same-sex sexual behavior.
“It’s effectively impossible to predict an individual’s sexual behavior from their genome,” said Ben Neale, a geneticist at Massachusetts General Hospital and the Broad Institute who led the study. “Genetics is less than half of this story for sexual behavior.”
Why this study matters — and what it can’t tell us
Humans have tried to understand human sexuality for centuries — and genetics researchers joined the fray in the early 1990s after a series of studies on twins suggested homosexuality ran in families. These kinds of studies have continued through the years, going as far as pinpointing a gene on the X chromosome — Xq28 — as the culprit.
“As a teenager trying to understand myself and understand my sexuality, I looked at the internet for “the gay gene” and obviously came across Xq28,” said Fah Sathirapongsasuti, a study co-author and senior scientist at 23andMe, which he joked once led him to believe he inherited his gayness from his mother.
His comments speak to the larger narrative about using biology to define complex behaviors — like sexuality — when science is always evolving and takes time to find anything close to definitive.
Those early studies stumbled upon a concrete pattern: Sexuality can run in families and thus must have a genetic component. But back then, the scientists had no way of comprehensively exploring this issue. Genome sequencing took decades to slowly mature into what it is today, and twins alone cannot represent the genetic complexity of our species.
“We worried a lot about volunteer bias,” said Bailey, whose research includes a widely publicized study on Xq28 and gay brothers from 2018 .
Those projects — known as linkage studies — were designed to find single major genes that appeared to have a big effect on sexuality, said Dr. Alan Sanders, an associate director for psychiatric genetics at NorthShore University HealthSystem Research Institute. Sanders collaborated with Bailey on those earlier studies and said their work had always admitted that there was no single “gay gene.”
“The field has moved on more to genome wide association studies,” said Sanders, who is also a co-author of the research published Thursday in Science. “Genome wide association studies are better at mapping genes with small effects, which are at play here.”
And even this new study has a big limitation, one that has been inherent to major genomic studies for the last two decades: GWAS studies are too white .
“There’s many politically correct ways of saying this, but basically the study is mostly a Caucasian sample of European ancestry. So, it does not include peoples from Latin America, Asia and Africa,” said Dr. Eric Vilain, director of the Center for Genetic Medicine Research at Children’s National Health System. “The second limitation is that the way they lumped together what they call ‘nonheterosexuals’”
The researchers had members of the same-sex community review the study’s design and language, and they admit that their terminology and definitions for gay, lesbian and heterosexual do not reflect the full nature of the sexuality continuum.
They did attempt to examine some elements of this continuum by conducting GWAS analysis on three smaller DNA databases wherein the participants had been surveyed using the Kinsey Scale. The Kinsey Scale is a somewhat infamous test for determining the strength of a person’s feelings toward members of the same- and opposite-sexes. In other words, it tries to judge if a person leans gay, straight or bisexual.
The team found genetics cannot explain people’s scores on the Kinsey Scale.
“We discovered that the Kinsey Scale … is really an oversimplification of the diversity of sexual behavior in humans,” Neale said. Bailey disagrees, arguing that people’s feelings of sexual interest and arousal — and therefore, their readouts on the Kinsey Scale — may be too complicated to validate through genetics.
He did agree with Neale that the debate is now closed on whether any single gene is responsible for sexual orientation.
“[Our study] underscores an important role for the environment in shaping human sexual behavior and perhaps most importantly there is no single gay gene but rather the contribution of many small genetic effects scattered across the genome,” Neale said.
Nsikan Akpan is the digital science producer for PBS NewsHour and co-creator of the award-winning, NewsHour digital series ScienceScope .
Support Provided By: Learn more
Educate your inbox
Subscribe to Here’s the Deal, our politics newsletter for analysis you won’t find anywhere else.
Thank you. Please check your inbox to confirm.

A scientific approach to evaluating global anti-poverty programs
Science Aug 23
- Introduction to Genomics
- Educational Resources
- Policy Issues in Genomics
- The Human Genome Project
- Funding Opportunities
- Funded Programs & Projects
- Division and Program Directors
- Scientific Program Analysts
- Contact by Research Area
- News & Events
- Research Areas
- Research investigators
- Research Projects
- Clinical Research
- Data Tools & Resources
- Genomics & Medicine
- Family Health History
- For Patients & Families
- For Health Professionals
- Jobs at NHGRI
- Training at NHGRI
- Funding for Research Training
- Professional Development Programs
- NHGRI Culture
- Social Media
- Broadcast Media
- Image Gallery
- Press Resources
- Organization
- NHGRI Director
- Mission & Vision
- Policies & Guidance
- Institute Advisors
- Strategic Vision
- Leadership Initiatives
- Diversity, Equity, and Inclusion
- Partner with NHGRI
- Staff Search
ADHD Genetic Research Study
The Attention Deficit/Hyperactivity Disorder (ADHD) Genetic Research Study is exploring the genetic factors that contribute to ADHD, with the hope of improving treatment for this condition in the future.
ADHD often appears to run in families, and research studies have suggested that there may be a genetic component to this disorder. Individuals diagnosed with ADHD may have close blood relatives with the disorder. Scientists believe that ADHD is a complex disorder that probably involves at least two genes. Non-genetic causes such as abnormal brain development, brain injury or environmental factors are also believed to play a role in the disorder.
One of the long-range goals of this study is to facilitate the diagnosis of ADHD and the development of improved treatments, possibly including individually tailored treatments. Discovering the genes that are related to ADHD can only be done with the assistance of children that have ADHD and their families. By volunteering for this type of study, we believe that you and your family will help to bring about the benefits of research: improved diagnoses and treatment for future generations and, eventually, prevention.
The study is not a diagnostic service for ADHD, a treatment or advice service, or a "second opinion" service.
Eligibility
Because this is a genetic study, the children must be related by blood (not adopted or fostered) to the parents and to each other. Eligible families must have more than one child. At least one of the children between the ages of 7 and 18 must have a diagnosis of ADD or ADHD. Other children in the family may be either affected (have ADHD) or unaffected (not have ADHD), but must be at least 7 years of age at the time of enrollment. We would like to enroll entire families, both biological parents included, whenever possible.
No. There is no cost to you, the participant. NIH will cover screening and blood draw costs. In addition, we will provide a "thank you" gift ($10) to each participant for his or her involvement once we have received blood samples.
If you are interested in learning more about the study and/or participating in the study, please fill out the downloadable questionnaire and send it to us using the contact information below, or call the toll free number (1-888-226-6249). We will contact you as soon as possible. Participation is completely voluntary. Indicating interest in the study does not obligate you to participate. Even if at first you decide to participate but later change your mind, you can withdraw at any time.
What's Involved?
If you are interested, one of our team members will call you to take screening information and to explain details of the study and to answer your questions. If we determine that your family might qualify for the study, you will be sent informed consent forms and questionnaires in the mail for each potential enrollee in the study. The questionnaires will ask about past medical and behavioral histories. These questionnaires take approximately 10 to 20 minutes each to complete.
An additional telephone questionnaire with a staff member may also take place, which may take about 45 minutes for each child enrolled.
Once all information is obtained, it will be reviewed by the ADHD team members. If your family is eligible for the next level of our study, a blood collection kit will be sent to you to take to your health care provider to have your blood drawn and sent to us. All the instructions for obtaining the blood and mailing it back to us will be in the kit.
Personal information that is given to us by study participants is kept confidential. Blood samples are given a code that does not include your name. Information about you is kept in locked cabinets or password protected computer files. Research results from blood sample analysis will not be made available to participating families.
As with all of our research studies, participation is completely voluntary. You may decide to participate in the study but later change your mind. You may withdraw your consent to participate at any time.
Contact Information
By Phone (Toll Free): 1-800-411-1222
By Fax: Fax: (301) 480-7825 Attention: Research Coordinator/ADHD Genetic Research Study Download and complete the contact form.
By Mail: Research Coordinator/ADHD Genetic Research Study NHGRI / NIH Building 10, Room 10C103 10 Center Drive Bethesda, MD 20892
Last updated: March 17, 2014
- Introduction
- Conclusions
- Article Information
Data Sharing Statement
- Sociodemographic and Lifestyle Factors and Epigenetic Aging in US Young Adults JAMA Network Open Original Investigation July 29, 2024 This cohort study investigates the association of sociodemographic and lifestyle factors with biological age as measured by epigenetic clocks among younger adults. Kathleen Mullan Harris, PhD; Brandt Levitt, PhD; Lauren Gaydosh, PhD; Chantel Martin, PhD; Jess M. Meyer, PhD; Aura Ankita Mishra, PhD; Audrey L. Kelly, PhD; Allison E. Aiello, PhD
- Telehealth Parenting Program and Epigenetic Biomarkers in Children With Developmental Delay JAMA Network Open Original Investigation July 29, 2024 This secondary analysis of a randomized clinical trial assesses the association of a telehealth parent-child interaction training program with biomarkers associated with aging and chronic inflammation among preschool-aged children with developmental delay. Sarah M. Merrill, PhD; Christina Hogan, MS; Anne K. Bozack, PhD; Andres Cardenas, PhD; Jonathan S. Comer, PhD; Daniel M. Bagner, PhD; April Highlander, PhD; Justin Parent, PhD
- Socioeconomic Status, Lifestyle, and DNA Methylation Age JAMA Network Open Original Investigation July 29, 2024 This cohort study explores whether the rate of biological aging estimated by an epigenetic clock is associated with social determinants of health in a racially and ethnically diverse population. Alika K. Maunakea, PhD; Krit Phankitnirundorn, PhD; Rafael Peres, PhD; Christian Dye, PhD; Ruben Juarez, PhD; Catherine Walsh, PhD; Connor Slavens, BSc; S. Lani Park, PhD; Lynne R. Wilkens, DrPH; Loïc Le Marchand, MD, PhD
- Epigenetic Age Acceleration and Disparities in Posttraumatic Stress in Women JAMA Network Open Original Investigation July 29, 2024 This cohort study examines the association of epigenetic age acceleration with probable posttraumatic stress disorder and symptom severity in US women exposed to disaster. Alicia K. Smith, PhD; Seyma Katrinli, PhD; Dawayland O. Cobb, MS; Evan G. Goff, BS; Michael Simmond, BS; Grace M. Christensen, PhD, MPH; Tyler Prusisz, BS; Sierra N. Garth, MPH; Meghan Brashear, MPH; Anke Hüls, PhD, MSc; Erika J. Wolf, PhD; Edward J. Trapido, ScD; Ariane L. Rung, PhD, MPH; Nicole R. Nugent, PhD; Edward S. Peters, DMD, SM, ScD
- Childhood Maltreatment and Longitudinal Epigenetic Aging JAMA Network Open Original Investigation July 29, 2024 This cohort study examines whether childhood exposure to physical and emotional abuse and neglect is associated with the rate of epigenetic aging. Olivia D. Chang, MSW; Helen C. S. Meier, PhD; Kathryn Maguire-Jack, PhD; Pamela Davis-Kean, PhD; Colter Mitchell, PhD
- Familial Loss of a Loved One and Biological Aging JAMA Network Open Original Investigation July 29, 2024 This cohort study evaluates associations between losing a loved one and accelerated biological aging. Allison E. Aiello, PhD, MS; Aura Ankita Mishra, PhD; Chantel L. Martin, PhD; Brandt Levitt, PhD; Lauren Gaydosh, PhD; Daniel W. Belsky, PhD; Robert A. Hummer, PhD; Debra J. Umberson, PhD; Kathleen Mullan Harris, PhD
- Obesity and Early-Onset Breast Cancer in Black and White Women JAMA Network Open Original Investigation July 29, 2024 This cohort study of patients with breast cancer examines whether a race-specific association exists between obesity and early-onset breast cancer or the diagnosis of specific molecular subtypes. Sarabjeet Kour Sudan, PhD; Amod Sharma, PhD; Kunwar Somesh Vikramdeo, PhD; Wade Davis, BS; Sachin K. Deshmukh, PhD; Teja Poosarla, MD; Nicolette P. Holliday, MD; Pranitha Prodduturvar, MD; Cindy Nelson, BS; Karan P. Singh, PhD; Ajay P. Singh, PhD; Seema Singh, PhD
- Psychosocial Disadvantage During Childhood and Midlife Health JAMA Network Open Original Investigation July 29, 2024 This cohort study examines independent and additive associations of low childhood socioeconomic status and perceived stress in childhood with insulin resistance and epigenetic aging among women followed up from 10 to 40 years of age. Ryan L. Brown, PhD; Katie E. Alegria, PhD; Elissa Hamlat, PhD; A. Janet Tomiyama, PhD; Barbara Laraia, PhD; Eileen M. Crimmins, PhD; Terrie E. Moffitt, PhD; Elissa S. Epel, PhD
- Epigenetic Aging and Racialized, Economic, and Environmental Injustice JAMA Network Open Original Investigation July 29, 2024 This cross-sectional study assesses whether socially structured adversity is associated with increased epigenetic accelerated aging among US-born Black non-Hispanic, Hispanic, and White non-Hispanic adults. Nancy Krieger, PhD; Christian Testa, BS; Jarvis T. Chen, ScD; Nykesha Johnson, MPH; Sarah Holmes Watkins, PhD; Matthew Suderman, PhD; Andrew J. Simpkin, PhD; Kate Tilling, BSc, MSc, PhD; Pamela D. Waterman, MPH; Brent A. Coull, PhD; Immaculata De Vivo, PhD; George Davey Smith, MA(Oxon), MD, BChir(Cantab), MSc(Lond); Ana V. Diez Roux, MD, PhD, MPH; Caroline Relton, PhD
- Prenatal Maternal Occupation and Child Epigenetic Age Acceleration JAMA Network Open Original Investigation July 29, 2024 This cohort study of mother-infant pairs examines the association between prenatal maternal occupation and epigenetic aging among children in a Latino agricultural community in California. Saher Daredia, MPH; Anne K. Bozack, PhD; Corinne A. Riddell, PhD; Robert Gunier, PhD; Kim G. Harley, PhD; Asa Bradman, PhD; Brenda Eskenazi, PhD; Nina Holland, PhD; Julianna Deardorff, PhD; Andres Cardenas, PhD
- Advancing Health Disparities Science Through Social Epigenomics Research JAMA Network Open Special Communication July 29, 2024 This special communication introduces the studies included in this special issue as part of the National Institutes of Health National Institute on Minority Health and Health Disparities Social Epigenomics Program. Arielle S. Gillman, PhD, MPH; Eliseo J. Pérez-Stable, MD; Rina Das, PhD
See More About
Sign up for emails based on your interests, select your interests.
Customize your JAMA Network experience by selecting one or more topics from the list below.
- Academic Medicine
- Acid Base, Electrolytes, Fluids
- Allergy and Clinical Immunology
- American Indian or Alaska Natives
- Anesthesiology
- Anticoagulation
- Art and Images in Psychiatry
- Artificial Intelligence
- Assisted Reproduction
- Bleeding and Transfusion
- Caring for the Critically Ill Patient
- Challenges in Clinical Electrocardiography
- Climate and Health
- Climate Change
- Clinical Challenge
- Clinical Decision Support
- Clinical Implications of Basic Neuroscience
- Clinical Pharmacy and Pharmacology
- Complementary and Alternative Medicine
- Consensus Statements
- Coronavirus (COVID-19)
- Critical Care Medicine
- Cultural Competency
- Dental Medicine
- Dermatology
- Diabetes and Endocrinology
- Diagnostic Test Interpretation
- Drug Development
- Electronic Health Records
- Emergency Medicine
- End of Life, Hospice, Palliative Care
- Environmental Health
- Equity, Diversity, and Inclusion
- Facial Plastic Surgery
- Gastroenterology and Hepatology
- Genetics and Genomics
- Genomics and Precision Health
- Global Health
- Guide to Statistics and Methods
- Hair Disorders
- Health Care Delivery Models
- Health Care Economics, Insurance, Payment
- Health Care Quality
- Health Care Reform
- Health Care Safety
- Health Care Workforce
- Health Disparities
- Health Inequities
- Health Policy
- Health Systems Science
- History of Medicine
- Hypertension
- Images in Neurology
- Implementation Science
- Infectious Diseases
- Innovations in Health Care Delivery
- JAMA Infographic
- Law and Medicine
- Leading Change
- Less is More
- LGBTQIA Medicine
- Lifestyle Behaviors
- Medical Coding
- Medical Devices and Equipment
- Medical Education
- Medical Education and Training
- Medical Journals and Publishing
- Mobile Health and Telemedicine
- Narrative Medicine
- Neuroscience and Psychiatry
- Notable Notes
- Nutrition, Obesity, Exercise
- Obstetrics and Gynecology
- Occupational Health
- Ophthalmology
- Orthopedics
- Otolaryngology
- Pain Medicine
- Palliative Care
- Pathology and Laboratory Medicine
- Patient Care
- Patient Information
- Performance Improvement
- Performance Measures
- Perioperative Care and Consultation
- Pharmacoeconomics
- Pharmacoepidemiology
- Pharmacogenetics
- Pharmacy and Clinical Pharmacology
- Physical Medicine and Rehabilitation
- Physical Therapy
- Physician Leadership
- Population Health
- Primary Care
- Professional Well-being
- Professionalism
- Psychiatry and Behavioral Health
- Public Health
- Pulmonary Medicine
- Regulatory Agencies
- Reproductive Health
- Research, Methods, Statistics
- Resuscitation
- Rheumatology
- Risk Management
- Scientific Discovery and the Future of Medicine
- Shared Decision Making and Communication
- Sleep Medicine
- Sports Medicine
- Stem Cell Transplantation
- Substance Use and Addiction Medicine
- Surgical Innovation
- Surgical Pearls
- Teachable Moment
- Technology and Finance
- The Art of JAMA
- The Arts and Medicine
- The Rational Clinical Examination
- Tobacco and e-Cigarettes
- Translational Medicine
- Trauma and Injury
- Treatment Adherence
- Ultrasonography
- Users' Guide to the Medical Literature
- Vaccination
- Venous Thromboembolism
- Veterans Health
- Women's Health
- Workflow and Process
- Wound Care, Infection, Healing
Get the latest research based on your areas of interest.
Others also liked.
- Download PDF
- X Facebook More LinkedIn
Chiu DT , Hamlat EJ , Zhang J , Epel ES , Laraia BA. Essential Nutrients, Added Sugar Intake, and Epigenetic Age in Midlife Black and White Women : NIMHD Social Epigenomics Program . JAMA Netw Open. 2024;7(7):e2422749. doi:10.1001/jamanetworkopen.2024.22749
Manage citations:
© 2024
- Permissions
Essential Nutrients, Added Sugar Intake, and Epigenetic Age in Midlife Black and White Women : NIMHD Social Epigenomics Program
- 1 Community Health Sciences Division, School of Public Health, University of California, Berkeley
- 2 Osher Center for Integrative Health, University of California, San Francisco
- 3 Department of Psychiatry and Behavioral Sciences, University of California, San Francisco
- 4 Department of Human Genetics, University of California, Los Angeles
- Original Investigation Sociodemographic and Lifestyle Factors and Epigenetic Aging in US Young Adults Kathleen Mullan Harris, PhD; Brandt Levitt, PhD; Lauren Gaydosh, PhD; Chantel Martin, PhD; Jess M. Meyer, PhD; Aura Ankita Mishra, PhD; Audrey L. Kelly, PhD; Allison E. Aiello, PhD JAMA Network Open
- Original Investigation Telehealth Parenting Program and Epigenetic Biomarkers in Children With Developmental Delay Sarah M. Merrill, PhD; Christina Hogan, MS; Anne K. Bozack, PhD; Andres Cardenas, PhD; Jonathan S. Comer, PhD; Daniel M. Bagner, PhD; April Highlander, PhD; Justin Parent, PhD JAMA Network Open
- Original Investigation Socioeconomic Status, Lifestyle, and DNA Methylation Age Alika K. Maunakea, PhD; Krit Phankitnirundorn, PhD; Rafael Peres, PhD; Christian Dye, PhD; Ruben Juarez, PhD; Catherine Walsh, PhD; Connor Slavens, BSc; S. Lani Park, PhD; Lynne R. Wilkens, DrPH; Loïc Le Marchand, MD, PhD JAMA Network Open
- Original Investigation Epigenetic Age Acceleration and Disparities in Posttraumatic Stress in Women Alicia K. Smith, PhD; Seyma Katrinli, PhD; Dawayland O. Cobb, MS; Evan G. Goff, BS; Michael Simmond, BS; Grace M. Christensen, PhD, MPH; Tyler Prusisz, BS; Sierra N. Garth, MPH; Meghan Brashear, MPH; Anke Hüls, PhD, MSc; Erika J. Wolf, PhD; Edward J. Trapido, ScD; Ariane L. Rung, PhD, MPH; Nicole R. Nugent, PhD; Edward S. Peters, DMD, SM, ScD JAMA Network Open
- Original Investigation Childhood Maltreatment and Longitudinal Epigenetic Aging Olivia D. Chang, MSW; Helen C. S. Meier, PhD; Kathryn Maguire-Jack, PhD; Pamela Davis-Kean, PhD; Colter Mitchell, PhD JAMA Network Open
- Original Investigation Familial Loss of a Loved One and Biological Aging Allison E. Aiello, PhD, MS; Aura Ankita Mishra, PhD; Chantel L. Martin, PhD; Brandt Levitt, PhD; Lauren Gaydosh, PhD; Daniel W. Belsky, PhD; Robert A. Hummer, PhD; Debra J. Umberson, PhD; Kathleen Mullan Harris, PhD JAMA Network Open
- Original Investigation Obesity and Early-Onset Breast Cancer in Black and White Women Sarabjeet Kour Sudan, PhD; Amod Sharma, PhD; Kunwar Somesh Vikramdeo, PhD; Wade Davis, BS; Sachin K. Deshmukh, PhD; Teja Poosarla, MD; Nicolette P. Holliday, MD; Pranitha Prodduturvar, MD; Cindy Nelson, BS; Karan P. Singh, PhD; Ajay P. Singh, PhD; Seema Singh, PhD JAMA Network Open
- Original Investigation Psychosocial Disadvantage During Childhood and Midlife Health Ryan L. Brown, PhD; Katie E. Alegria, PhD; Elissa Hamlat, PhD; A. Janet Tomiyama, PhD; Barbara Laraia, PhD; Eileen M. Crimmins, PhD; Terrie E. Moffitt, PhD; Elissa S. Epel, PhD JAMA Network Open
- Original Investigation Epigenetic Aging and Racialized, Economic, and Environmental Injustice Nancy Krieger, PhD; Christian Testa, BS; Jarvis T. Chen, ScD; Nykesha Johnson, MPH; Sarah Holmes Watkins, PhD; Matthew Suderman, PhD; Andrew J. Simpkin, PhD; Kate Tilling, BSc, MSc, PhD; Pamela D. Waterman, MPH; Brent A. Coull, PhD; Immaculata De Vivo, PhD; George Davey Smith, MA(Oxon), MD, BChir(Cantab), MSc(Lond); Ana V. Diez Roux, MD, PhD, MPH; Caroline Relton, PhD JAMA Network Open
- Original Investigation Prenatal Maternal Occupation and Child Epigenetic Age Acceleration Saher Daredia, MPH; Anne K. Bozack, PhD; Corinne A. Riddell, PhD; Robert Gunier, PhD; Kim G. Harley, PhD; Asa Bradman, PhD; Brenda Eskenazi, PhD; Nina Holland, PhD; Julianna Deardorff, PhD; Andres Cardenas, PhD JAMA Network Open
- Special Communication Advancing Health Disparities Science Through Social Epigenomics Research Arielle S. Gillman, PhD, MPH; Eliseo J. Pérez-Stable, MD; Rina Das, PhD JAMA Network Open
Question Are dietary patterns, including essential nutrients and added sugar intakes, and scores of nutrient indices associated with epigenetic aging?
Findings In this cross-sectional study of 342 Black and White women at midlife, higher added sugar intake was associated with older epigenetic age, whereas higher essential, pro-epigenetic nutrient intake and higher Alternate Mediterranean Diet (aMED) and Alternate Healthy Eating Index (AHEI)–2010 scores (reflecting dietary alignment with Mediterranean diet and chronic disease prevention guidelines, respectively) were associated with younger epigenetic age.
Meaning The findings of this study suggest a tandem importance in both optimizing nutrient intake and reducing added sugar intake for epigenetic health.
Importance Nutritive compounds play critical roles in DNA replication, maintenance, and repair, and also serve as antioxidant and anti-inflammatory agents. Sufficient dietary intakes support genomic stability and preserve health.
Objective To investigate the associations of dietary patterns, including intakes of essential nutrients and added sugar, and diet quality scores of established and new nutrient indices with epigenetic age in a diverse cohort of Black and White women at midlife.
Design, Setting, and Participants This cross-sectional study included analyses (2021-2023) of past women participants of the 1987-1997 National Heart, Lung, and Blood Institute Growth and Health Study (NGHS), which examined cardiovascular health in a community cohort of Black and White females aged between 9 and 19 years. Of these participants who were recruited between 2015 and 2019 from NGHS’s California site, 342 females had valid completed diet and epigenetic assessments. The data were analyzed from October 2021 to November 2023.
Exposure Diet quality scores of established nutrient indices (Alternate Mediterranean Diet [aMED], Alternate Healthy Eating Index [AHEI]–2010); scores for a novel, a priori–developed Epigenetic Nutrient Index [ENI]; and mean added sugar intake amounts were derived from 3-day food records.
Main Outcomes and Measures GrimAge2, a second-generation epigenetic clock marker, was calculated from salivary DNA. Hypotheses were formulated after data collection. Healthier diet indicators were hypothesized to be associated with younger epigenetic age.
Results A total of 342 women composed the analytic sample (mean [SD] age, 39.2 [1.1] years; 171 [50.0%] Black and 171 [50.0%] White participants). In fully adjusted models, aMED (β, −0.41; 95% CI, −0.69 to −0.13), AHEI-2010 (β, −0.05; 95% CI, −0.08 to −0.01), and ENI (β, −0.17; 95% CI, −0.29 to −0.06) scores, and added sugar intake (β, 0.02; 95% CI, 0.01-0.04) were each significantly associated with GrimAge2 in expected directions. In combined analyses, the aforementioned results with GrimAge2 were preserved with the association estimates for aMED and added sugar intake retaining their statistical significance.
Conclusions and Relevance In this cross-sectional study, independent associations were observed for both healthy diet and added sugar intake with epigenetic age. To our knowledge, these are among the first findings to demonstrate associations between added sugar intake and epigenetic aging using second-generation epigenetic clocks and one of the first to extend analyses to a diverse population of Black and White women at midlife. Promoting diets aligned with chronic disease prevention recommendations and replete with antioxidant or anti-inflammatory and pro-epigenetic health nutrients while emphasizing low added sugar consumption may support slower cellular aging relative to chronological age, although longitudinal analyses are needed.
Epigenetic clocks powerfully predict biological age independent of chronological age. These clocks reflect altered gene and protein expression patterns, particularly those resulting from differential DNA methylation (DNAm) at CpG (5′-C-phosphate-G-3′) sites. DNAm that accumulates over time is a testament to the toll social, behavioral, and environmental forces can have on the body. 1 - 3 These alterations often result in pathogenic processes (eg, genomic instability, systemic inflammation, and oxidative stress) characteristic of aging and chronic disease. 1 , 4 , 5 As such, myriad clocks reflecting epigenetic age have been developed for a range of age- or disease-related targets. 4 , 6 The GrimAge series contains second-generation markers of epigenetic aging that account for clinical and functional biomarkers, and is most notable for its robust associations with human mortality and morbidity risk, including time to death and comorbidity counts. 6 , 7 The recently developed version 2 of the GrimAge clock (hereafter, GrimAge2) improved on the first’s predictive abilities and confirmed its applicability for people at midlife and of different racial and ethnic backgrounds. 1 , 6
Epigenetic changes are modifiable and efforts to counter epigenetic alteration in humans have centered on lifestyle factors including diet, inspiring concepts of an “epigenetic diet” and “nutriepigenetics.” 8 , 9 So far, 2 epidemiological studies have found inverse associations between higher diet quality and slower epigenetic aging using clock measures related to mortality, including the first version of GrimAge. 7 , 10 In those studies, diet measures were reflective of healthy dietary patterns (eg, the Dietary Approaches to Stop Hypertension [DASH] diet, the Alternate Mediterranean Diet [aMED] score) emphasizing consumption of fruits, vegetables, whole grains, nuts and seeds, and legumes. 8 , 11 For example, the Mediterranean-style diet is largely plant-based with emphasis on extra virgin olive oil and seafood. This makes it replete with bioactive nutrients and phytotherapeutic compounds and low in highly processed, high fat, and nutrient-poor foods, a mixture hypothesized to be protective against low-grade chronic inflammation (“inflammaging”), oxidative stress, intracellular and extracellular waste accumulation, and disrupted intracellular signaling and protein-protein interactions. Thus, such a pattern is likely effective in preventing and reversing the epigenetic changes and pathogenic processes associated with aging, disease, and decline. 4 , 8 , 12 - 14
Dietary Reference Intakes (DRIs) are an established set of nutrient-specific reference values determined by experts that guide population intakes for adequacy and toxic effects. 15 Recent thinking, however, suggests that diets may not always adequately supply nutrients and other bioactives, particularly relative to the amounts necessary to fully condition gene expression or counteract epigenetic alterations to ensure optimal physiological metabolism. 8 Macronutrients and micronutrients play crucial roles in DNA replication, damage prevention, and repair, whereas nutrient deficiencies (and excesses) can cause genomic damage to the same degree as physical or chemical exposures. 16 Given that (1) progenome effects of some micronutrients have been observed at different and higher levels than the established DRIs and (2) determination of DRIs does not solely consider genomic stability (ie, lesser susceptibility to genomic alterations), experts have called for refining the DRIs to be better aligned for genomic health maintenance. 14 , 16 - 18 Diet quality inventories, such as those for Mediterranean-style diets, have not generally incorporated DRIs, although such considerations could clarify how food-based indices compare against requirements for related nutrients (eg, those with epigenetic properties) and refine epidemiological and intervention efforts. Accordingly, for this study, a novel nutrient index theoretically associated with epigenetic health was created and its associations with epigenetic aging were tested alongside established diet quality indices.
To date, nutriepigenetic work has mostly involved older White populations and focused on healthy dietary aspects. It is therefore important to examine the associations between nutrition and epigenetic aging in more diverse samples and to better understand what specific dietary aspects could be underlying the observed associations. Nutrients with established epigenetic action should be examined, especially considering intakes relative to amounts set forth in the DRIs and nutritional recommendations. Similarly, sugar is an established pro-inflammatory and oxidative agent that has been implicated in cancer as well as cardiometabolic diseases. 19 - 21 However, in diet quality indices often studied in the epigenetic context (eg, the aMED), sugar is noticeably unaccounted for, and it has also yet to be examined alone. Given the high consumption of sugar globally and the demographic variations within, 22 - 24 elucidating this association could motivate future dietary interventions and guidelines as well as health disparities research. This study sought to examine associations of diet with GrimAge2 in a midlife cohort comprising Black and White US women. The central hypothesis was that indicators of a healthier diet may be associated with decelerated epigenetic aging, and added sugar intake with accelerated aging.
This cross-sectional study used data from the original National Heart, Lung, and Blood Institute (NHLBI) Growth and Health Study (NGHS) (1987-1999) and its follow-up (2015-2019), which studied a cohort of Black and White females aged from 9 or 10 years into midlife (age 36-43 years), examining cardiometabolic health and related determinants. The participants were recruited based on biological female sex at age 9 or 10 years. The follow-up study re-recruited women from the California site. 25 , 26 Participants (and/or their parent[s] or guardian[s]) provided demographic data and completed online or paper surveys and new assessments. Participants received remuneration and provided informed consent. The institutional review board of the University of California, Berkeley, approved all study protocols. This study followed the Strengthening the Reporting of Observational Studies in Epidemiology ( STROBE ) reporting guideline.
For inclusion in current analyses, the participants needed valid diet records and epigenetic data at midlife along with age and race and ethnicity information (participant self-reported); after excluding 5 women with epigenetic data quality issues, 342 individuals were included in the analytic sample. Complete case analyses were done. Among the 624 women who were followed up, the women composing the analytic sample were younger (39.2 years vs 39.9 years; P < .001) and had greater body mass index (BMI, calculated as weight in kilograms divided by height in meters squared) compared with women without complete diet and epigenetic data (32.5 vs 30.7; P = .02) ( Table 1 ). No differences were otherwise observed.
Participants provided saliva samples used for DNAm analyses performed by the University of California, Los Angeles Neuroscience Genomics Core (UNGC) of the Semel Institute for Neuroscience and Human Behavior using the Infinium HumanMethylation450 BeadChip platform (Illumina, Inc). DNAm profiles were generated by Horvath’s online calculator, 27 which provided (1) estimates of epigenetic age based on GrimAge2 estimation methods; and (2) assessments of data quality (again, 5 observations did not pass quality checks). GrimAge2 uses Cox proportional hazards regression models that regress time to death (due to all-cause mortality) on DNAm-based surrogates of plasma proteins, a DNAm-based estimator of smoking pack-years, age, and female sex. It was updated from GrimAge, version 1 6 by including 2 new DNAm-based estimators of plasma proteins—high-sensitivity C-reactive protein (logCRP) and hemoglobin A 1c (log A 1c )—beyond the original 7. Linear transformation of results from these models allows GrimAge2 to be taken as an epigenetic age estimate (in years). Further information can be accessed from studies on DNA treatment and isolation and advanced analysis options for generating output files 28 or GrimAge2. 1
The participants were instructed by the NGHS study staff to self-complete a 3-day food record at follow-up for 3 nonconsecutive days. 29 Data were entered into and analyzed by the Nutrition Data System for Research (NDSR) software, version 2018 (University of Minnesota Nutrition Coordinating Center).
Mean nutrient and food intakes were calculated across valid food records for each woman based on the NDSR 2018 output. These values were used to calculate the scores of 2 overall diet quality nutrient indices (aMED and the Alternate Healthy Eating Index [AHEI]–2010) and a novel index (Epigenetic Nutrient Index [ENI]) score as described below. The aMED (Mediterranean-style diet) followed published scoring methodology 30 reflecting the degree of adherence to 9 components of an anti-inflammatory, antioxidant-rich diet. The AHEI-2010 was assessed following published scoring instructions 31 and reflects the degree of adherence to 11 dietary components associated with decreased risk for chronic disease.
This study developed a novel nutrient index (ENI) after the Mediterranean-style diet, but via a nutrient-based approach rather than a food-based one. Nutrient selection was done a priori based on antioxidant and/or anti-inflammatory capacities as well as roles in DNA maintenance and repair documented in the literature. 16 , 32 , 33 Scores can range from 0 to 24, with higher scores reflecting higher DRI adherence ( Table 2 ). 34 The internal consistency of the ENI was acceptable (Cronbach α = 0.79). The ENI also demonstrated convergent validity with r = 0.51 ENI-aMED correlation as well as higher ENI scores in women from childhood households with higher annual incomes (13.9 vs 11.7, for ≥$40 000/y vs <$10 000/y, respectively) and parental educational attainment (14.7 vs 12.3, for ≥college graduate vs < high school graduate, respectively), corresponding to the literature. 36 Pearson correlations between the ENI and diet scores and added sugar intake were also calculated. The ENI score was moderately correlated with the AHEI-2010 score ( r = 0.44) but not correlated with added sugar intake. The aMED and AHEI-2010 scores were highly correlated at r = 0.73. Added sugar intake had moderate correlation with the AHEI-2010 score ( r = −0.44) and low correlation with the aMED score ( r = −0.28).
Added sugar intake was calculated as the mean across valid food records using NDSR output. The NDSR defines added sugar intake as the total sugar added to foods (eg, as syrups and sugars) during food preparation and commercial food processing. Monosaccharides and disaccharides naturally occurring in foods are not included. 35
To maximize internal validity and minimize confounding, several covariates were included. Age and sample batch were controlled for as well as naive CD8 and CD8pCD28nCD45Ran memory and effector T-cell counts, thus accounting for normal cell count variation. To control for baseline factors and their potential influence on diet and epigenetic age over time, the following parameters assessed at age 9 or 10 years (mostly parent or caregiver reported) were further adjusted for annual household income, highest parental educational attainment, number of parents in household, and number of siblings. Additionally, self-reported race (Black or White) as well as the current health and lifestyle factors of self-reported chronic conditions (yes to any of the following ever: cancer, diabetes [including gestational, prediabetes], hypertension, or hypercholesterolemia) or medication use (currently yes for any of the following conditions: diabetes, hypertension, hypercholesterolemia, or thyroid), BMI (measured), having ever smoked (yes or no), and mean daily total energy intake (as higher diet quality scores might result from higher energy intake) 37 were also included.
Descriptive analyses provided summary statistics. Linear regression models estimated unadjusted and adjusted cross-sectional associations between each of the 4 dietary exposures with GrimAge2. Per expert recommendations, unadjusted models controlled for women’s current age, sample batch, and both naive CD8 and CD8pCD28nCD45Ran memory and effector T-cell counts. Adjusted models controlled for those variables in addition to relevant sociodemographic and health behavior–related covariates already listed. To examine the association between healthy diet measures together with added sugar intake and GrimAge2, aMED, AHEI-2010, and ENI scores were each separately put into the same fully adjusted multivariable linear regression model. The threshold for statistical significance was 2-tailed (α = .05) and all statistical analyses were conducted from October 2021 to November 2023 with Stata15 SE, version 15.1 (StataCorp LLC).
The analytic sample of this study comprised 342 women (mean [SD] age at follow-up, 39.2 [1.1] years; 171 [50.0%] Black and 171 [50.0%] White participants; mean [SD] BMI, 32.5 [10.0]; 150 [43.9%] ever smokers; 164 [48.0%] ever diagnosed with a chronic condition; and 58 [17.0%] currently taking medication) ( Table 1 ). The participants were well distributed across socioeconomic status categories at baseline (9-10 years old). The participants presented with low to moderate levels of diet quality; the mean (SD) scores were 3.9 (1.9) (possible range, 0-9) on the anti-inflammatory, antioxidant Mediterranean-style pattern (aMED); 55.4 (14.7) (possible range, 0-110) on the AHEI-2010 for chronic disease risk; and 13.5 (5.0) (possible range, 0-24) on the ENI for intakes of epigenetic-relevant nutrients relative to DRIs. The participants also reported mean (SD) daily added sugar intake of 61.5 (44.6) g, although the score range was large (2.7-316.5 g).
Table 3 provides the overall unadjusted and adjusted associations between each dietary exposure of interest and GrimAge2 resulting from multivariable linear regression models. In both unadjusted and adjusted models, all dietary exposures were statistically and significantly associated with GrimAge2 in the hypothesized, anticipated direction. In adjusted models, the associations observed for each dietary exposure were slightly attenuated. Each unit increase in the scores was associated with year changes in GrimAge2, as follows: aMED (β, −0.41; 95% CI, −0.69 to −0.13), AHEI-2010 (β, −0.05; 95% CI, −0.08 to −0.01), and ENI (β, −0.17; 95% CI, −0.29 to −0.06), indicating that healthier diets were associated with decelerated epigenetic aging. Each gram increase in added sugar intake was associated with a 0.02 (95% CI, 0.01 to 0.04) increase in GrimAge2, reflecting accelerated epigenetic aging.
Table 4 illustrates the associations of healthy diet measures (aMED, AHEI-2010, and ENI scores) and added sugar intake with epigenetic aging and gives the adjusted results for each healthy diet measure and added sugar intake with GrimAge2 in the context of each other. In all instances, healthier diet measures and added sugar intake appeared to maintain their independent associations with GrimAge2 in the expected directions. Associations were statistically significant for added sugar intake in all models as well as for aMED scores; 95% CIs were more imprecise for AHEI-2010 and ENI scores.
The findings of this cross-sectional study are among the first, to our knowledge, to demonstrate the association of added sugar intake with an epigenetic clock. Further, to our knowledge, it is the first study to examine the associations of diet with GrimAge2 and extend the applicability of such results to a cohort of Black and White women at midlife. As hypothesized, measures of healthy dietary patterns (aMED, AHEI-2010 scores), and high intakes of nutrients theoretically related to epigenetics (ENI) were associated with younger epigenetic age, while a higher intake of added sugar was associated with older epigenetic age. Additionally, this study examined indicators of healthy and less healthy diets in the same model, allowing simultaneous evaluation of each in the presence of the other. Although the magnitudes of associations were diminished and some 95% CIs became wider, their statistical significance generally persisted, supporting the existence of independent epigenetic associations of both healthy and less healthy diet measures. This approach is informative, as dietary components are often examined singularly or in indices, which can lead to erroneous conclusions if key contextual dietary components are not accounted for or are obscured. From these findings, even in healthy dietary contexts, added sugar still has detrimental associations with epigenetic age. Similarly, despite higher added sugar intake, healthier dietary intakes appear to remain generally associated with younger epigenetic age.
The number of published nutriepigenetic studies, particularly on examining second-generation epigenetic clock markers, is still relatively small. However, the results of the present study are consistent with the literature. Two other studies 7 , 10 have examined GrimAge1-associated outcomes and found higher diet quality scores, including the DASH and aMED, were associated with slower epigenetic aging. However, those studies were limited to older (>50 years) and White populations, limiting their demographic generalizability. Analyses of epigenetic aging and added sugar intake are new, but findings are consistent with the larger body of epidemiological work that has drawn connections between added sugar intake and cardiometabolic disease, 19 , 20 perhaps suggesting a potential mechanism underlying such observations. Granted, point and 95% CI estimates for the added sugar–GrimAge2 associations were close to zero, suggesting a smaller role for added sugar compared with healthy dietary measures; however, more studies are needed. Nevertheless, their statistical significance was persistent.
Nutrient-based inventories can provide epidemiological contributions for genomic health studies. The idea of epigenetically critical nutrients is important for 2 reasons. First, it supports the notion that epigenetic nutrient intakes above DRI levels could boost epigenetic preservation and potentially motivate updates to nutritional guidelines, an outcome advocated for by nutriepigenetic experts. 16 - 18 In the novel ENI constructed for the present study, points were awarded based on comparisons of average daily intakes with: (1) estimated average requirements, or the requirement considered adequate for half of the healthy individuals in a population, and (2) recommended dietary allowances or adequate intakes, or where 97% to 98% or essentially all of a population’s healthy individuals’ requirements for a nutrient are met. 15 Future iterations could test varying ENI scoring parameters relative to DRIs for epigenetic benefit. Second, taking a nutrient approach suggests that any dietary pattern rich in vitamins, minerals, and other bioactives could be useful for preserving epigenetic health. This is helpful because dietary patterns are socioculturally influenced, but a nutrient focus rather than a focus on foods could help bridge cultures, class, and geography. 9 The Okinawan diet, for example, is nutritionally similar to the Mediterranean-style diet but more aligned to Asian tastes. 38 In general, the sociodemographic determinants of diet should not be discounted. Across the US population, for instance, it is known that overall diet quality is mediocre and relatively low while added sugar intake is considerably high, as also observed in the sample of the present study. However, specific nutrient intakes will vary based on the particulars of dietary patterns. 22 , 36 As dietetics and medicine progresses into the era of personalized nutrition and personalized medicine, the role of social factors including diet will be important to consider in epigenetic studies and could figure prominently in work on health disparities.
Strengths of this study are its inclusion of a diverse group of women as well as use of robust measures of diet and DNAm. It was also possible to control for several potential sociodemographic confounders.
This study also has limitations. As a cross-sectional study, it is not possible to infer causality without temporality, and therefore longitudinal studies are needed. Additionally, diet was self-reported via 3-day food records, which may lead to underestimates and overestimates of intakes depending on the nutrient. Therefore, augmenting dietary assessment with food frequency questionnaires and/or biomarkers could be helpful. 39 Also, other nutrients with pro-epigenetic properties were not included in the current ENI. Still, the Cronbach α for this first ENI version was acceptable at 0.79 and it demonstrated good convergent validity with customary socioeconomic and demographic characteristics. The tolerable upper intake levels of the DRIs were not considered in constructing the ENI. Future work should assess the prevalence of intakes beyond upper limits to assess whether toxicity could be a concern.
To our knowledge, the findings of this cross-sectional study are among the first to find associations between indicators of healthy diet as well as added sugar intake and second-generation epigenetic aging markers and one of the first to include a cohort of Black women. Higher diet quality and higher consumption of antioxidants or anti-inflammatory nutrients were associated with younger epigenetic age, whereas higher consumption of added sugar was associated with older epigenetic age. Promotion of healthy diets aligned with chronic disease prevention and decreased added sugar consumption may support slower cellular aging relative to chronological age, although longitudinal analyses are needed.
Accepted for Publication: April 29, 2024.
Published: July 29, 2024. doi:10.1001/jamanetworkopen.2024.22749
Open Access: This is an open access article distributed under the terms of the CC-BY License . © 2024 Chiu DT et al. JAMA Network Open .
Corresponding Author: Dorothy T. Chiu, PhD, Osher Center for Integrative Health, University of California, San Francisco, 1545 Divisadero St, #301D, San Francisco, CA 94115 ( [email protected] ); Barbara A. Laraia, PhD, MPH, RD, Community Health Sciences Division, School of Public Health, University of California, Berkeley, 2121 Berkeley Way, Berkeley, CA 94720 ( [email protected] ).
Author Contributions: Drs Chiu and Laraia had full access to all of the data in the study and take responsibility for the integrity of the data and the accuracy of the data analysis. Drs Epel and Laraia share co–senior authorship on this article.
Concept and design: Chiu, Hamlat, Epel, Laraia.
Acquisition, analysis, or interpretation of data: All authors.
Drafting of the manuscript: Chiu, Hamlat, Laraia.
Critical review of the manuscript for important intellectual content: Hamlat, Zhang, Epel, Laraia.
Statistical analysis: Chiu, Hamlat, Zhang.
Obtained funding: Epel, Laraia.
Administrative, technical, or material support: Chiu, Laraia.
Supervision: Epel, Laraia.
Conflict of Interest Disclosures: Dr Chiu reported receiving support from grants from the Eunice Kennedy Shriver National Institute of Child Health and Human Development (NICHD); the National Heart, Lung, and Blood Institute (NHLBI); the National Institute on Aging (NIA); and the National Center for Complementary and Integrative Health (NCCIH) during the conduct of the study. Dr Hamlat reported receiving grants from the National Institutes of Health (NIH) during the conduct of the study. Dr Laraia reported receiving grants from NIH NICHD during the conduct of the study. No other disclosures were reported.
Funding/Support: The research reported in this publication was supported by grant R01HD073568 from the Eunice Kennedy Shriver NICHD (Drs Laraia and Epel, principal investigators [PIs]); grant R56HL141878 from the NHLBI; and grants R56AG059677 and R01AG059677 from the NIA (both for Drs Epel and Laraia, PIs). The participation of Dr Chiu was supported by the University of California, San Francisco Osher Center research training fellowship program under grant T32AT003997 from NCCIH.
Role of the Funder/Sponsor: The funders had no role in the design and conduct of the study; collection, management, analysis, and interpretation of the data; preparation, review, or approval of the manuscript; and decision to submit the manuscript for publication.
Data Sharing Statement: See the Supplement .
Additional Contributions: We recognize the past and present NHLBI Growth and Health Study (NGHS) staff for their talents and dedication, without which the study and these analyses would not have been possible. We also thank the Nutrition Policy Institute for providing consultation and support with historical study data. Additionally, we express immense gratitude to Ake T. Lu, PhD, and Steve Horvath, PhD, now of Altos Labs, for their epigenetic clock expertise and consultation. Neither was financially compensated for their contributions beyond their usual salary. Of note, we thank the NGHS participants for their time and efforts over the years.
- Register for email alerts with links to free full-text articles
- Access PDFs of free articles
- Manage your interests
- Save searches and receive search alerts

Faculty, students pair up for summer Nexus Scholar research projects
8/02/2024 By | Kathy Hovis , A&S Communications
Peek into various labs on campus during the summer and you can quickly get an idea of the diversity of research pursued by Cornell faculty.
In Goldwin Smith Hall, classics and information science majors are working with faculty on preserving and digitizing valuable 19th century lexica of classical Greek authors; in Lincoln Hall, music majors are working on a website to introduce the world to Haitian classical music performers and composers; and in Weill Hall, computational biologists are running computer simulations to help researchers better understand genetic mutations.
In the College of Arts & Sciences, more students can afford to stay on campus to work in these faculty labs during the summer thanks to generous alumni who fund the Nexus Scholar Program in the College. This year, 92 students were chosen to participate.
The program, which provides hourly salaries for students, is an entry point into undergraduate research and prioritizes applicants who do not have significant research experience. Along with their lab work, students take part in professional development workshops, career exploration events and meet other students who are passionate about learning.
Uncovering genetic impacts
Nicholas Yap ’27 is working with computational biologist April Wei , assistant professor (A&S and CALS), to investigate the way that human-Neanderthal admixture could have influenced various heritable traits being passed down to present-day humans.
A computer science major, Yap is running simulations based on the population history of both humans and Neanderthals with genetic mutations through time under various scenarios. Analyzing this data could potentially help scientists understand how introgression works between other species, as well.
Wei’s previous research uses a vast dataset from the UK Biobank consisting of genetic and trait information of nearly 300,000 Brits of non-African ancestry. She and other researchers have found that 4,303 genetic variants originated from Neanderthals that are likely to have a phenotypic impact in modern humans. Genetic traits they’re studying include a person’s stature and natural immune resistance to certain diseases.
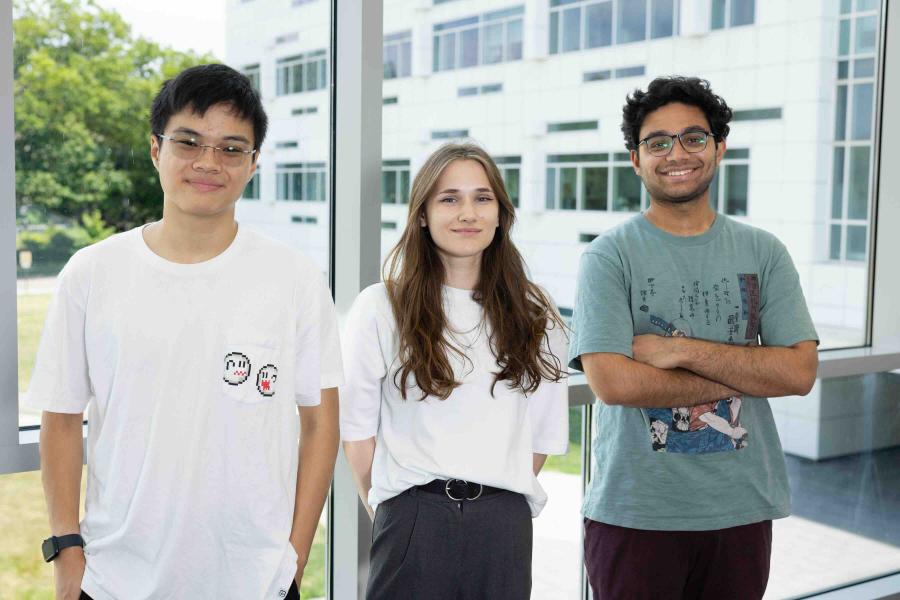
Maria Tiulchenko ’25 is also working with simulated data, but hers uses a set of simulated genomes to predict the local ancestry of a person.
“Genes are not just randomly passed to other generations, but they are passed in chunks,” said Tiulchenko, who is a physics major concentrating in computer science. “I can trace the population and trace the ancestry through different data sets and statistical analysis.”
Tiulchenko is very interested in genetics as a possible career. “I want to try to combine physics, biology and computer science,” she said.
Adita Syam ‘25 is working to build a phenotype simulator using a new data structure created in Wei’s lab that allows scientists to study millions of genomes. “This new structure makes working with genome data faster,” said Syam, who is majoring in mathematics and computer science.
“We are eager to prove we have a valuable data structure and add more features to the lab so others can adopt and use it,” Wei said. “It takes time and effort for people to realize it’s useful more broadly.”
Creating a useful Greek reference tool
Three students working with Jeffrey Rusten , classics professor emeritus (A&S), and Ethan Della Rocca, a graduate student in classics, have made great progress this summer taking physical and microfiche lexica to Aristophanes and Plato and turning them into a digital website – providing links to the text, translations into English, classification into word–families and semantic groupings and current bibliography.
Before the summer, Della Rocca created an initial digital version of the Aristophanes works using AI software, but since the text was handwritten in Greek, German and Latin, generating a new model requires more data.
Darby Bayne ‘26, Charlotte Behrend ’25 and Ryan Dwyer ’26 have been making corrections and augmenting that text all summer. Bayne is a computer science major, while Behrend and Dwyer are classics majors.
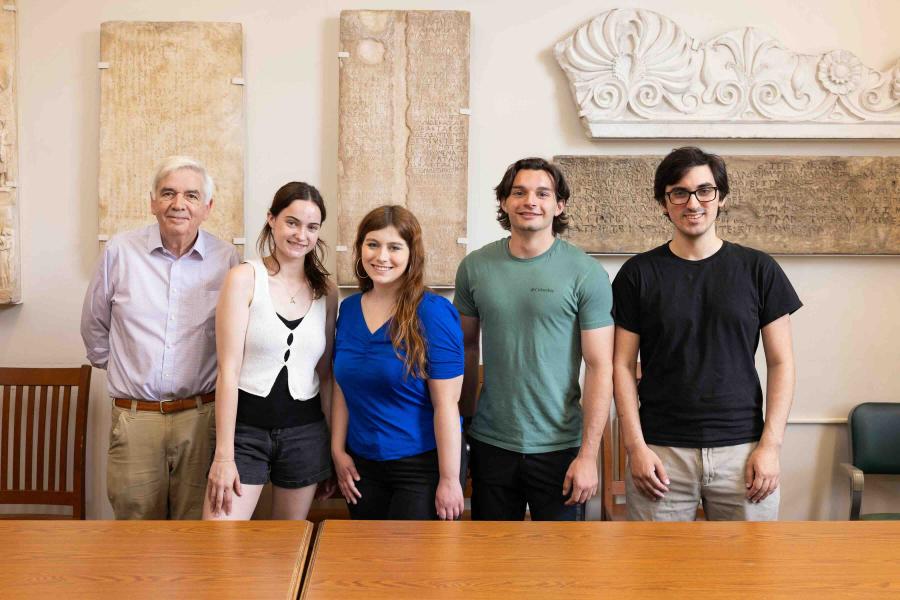
“This project started as a spreadsheet in 2017 and I have received a lot of help from undergrads and grad students over the years,” Rusten said. The lexica already developed can be found on lexeis.org but the group is also hoping to be included as a link on Logeion, a University of Chicago lexicon website.
“Research opportunities in classics for undergrads are few and far between,” Dwyer said, “and this one included a good meld of the history that is classics, along with new technology about how to interpret it.”
For Bayne, the project was “as far as you can imagine” from a typical coding summer internship, which she had last summer. “I wanted to pivot and try something different,” she said, adding that she created graphs and cluster maps with the words to identify patterns or interesting connections.
Behrend said working on the projects has “strengthened my convictions that this is what I want to study.”
Spreading the word about Haitian musical history
As a music and anthropology major, Anthony Washington ’25’s knowledge of all kinds of music and its history is vast, but he didn’t know a lot about 18th century Haitian music until he started working on research with Prof. Jean Bernard Cerin this summer.
“I didn’t realize there were Haitian Black composers doing incredible work in classical music,” Washington said, “or that (philosopher Jean-Jacques) Rousseau was a composer composing music for this Haitian song. It broadened my idea of what the world looks like.”
Cerin, assistant professor of music (A&S) and director of Cornell’s voice program, is the founder of the Lisette Project , a performance and educational effort focused on Haitian classical music. This summer, Washington and Zeke A.B. Lawrence ’25 are expanding content on the project’s website, which includes articles about composers, timelines, maps, musical scores and recordings.
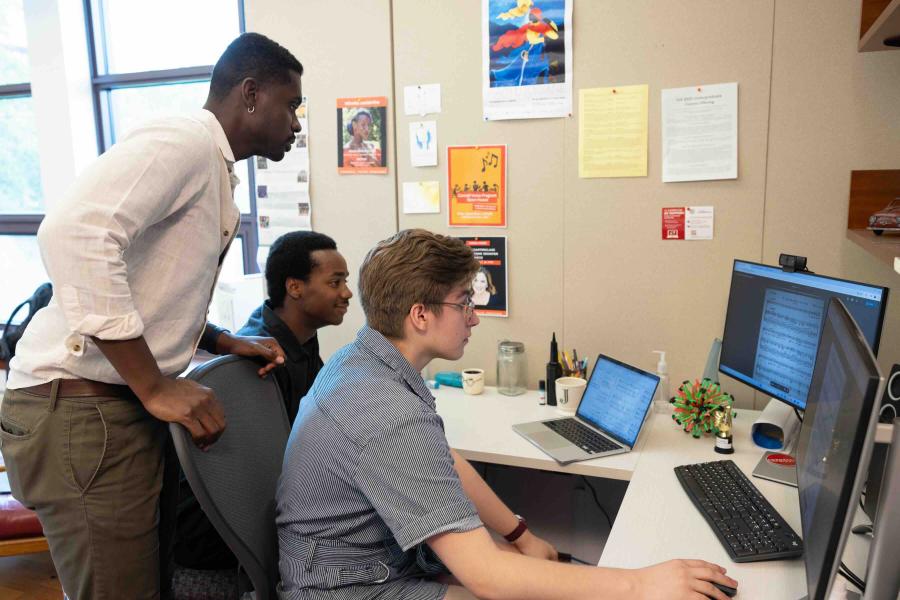
Lawrence and Washington have spent many hours transcribing, engraving and editing musical scores from hand-written manuscripts and and live performances of arrangements that Cerin and his musical collaborator Nicholas Mathew from the University of California at Berkeley have devised for their upcoming album and accompanying anthology of sheet music.
“In my work, I haven’t encountered this before, so I’m working to understand how a pianist might like to see the score,” said Lawrence, adding that the work helps them understand some of the foundations of other musical movements such as voodoo jazz in Haiti and how that may have impacted early American jazz music.
Cerin began the Lisette project in 2022 as an in-depth examination of “Lisette quitté la plaine,” the oldest surviving song text in early Haitian Creole written around 1757. The song tells the story of an enslaved man mourning the loss of his beloved who has been sold or sent away. The song has been recomposed multiple times throughout the centuries, with altered lyrics and melody.
The Lisette Project has commissioned a new version of the song, "Lisette fo'n kite La plaine" this time with lyrics written by Cerin's mother Lunise Jules and music by renowned Haitian guitarist Amos Coulanges to account for the modern day context in which Haitians are still fleeing their homes. The song will appear on the Lisette Project's upcoming album with the rest of the music the team has been editing and arranging this summer.
“I’m excited about the whole eco-system of music making — producing content for every part of the process of teaching and of performance,” Cerin said. “I am not your conventional historian, but I’m a musician who is definitely interested in history.”
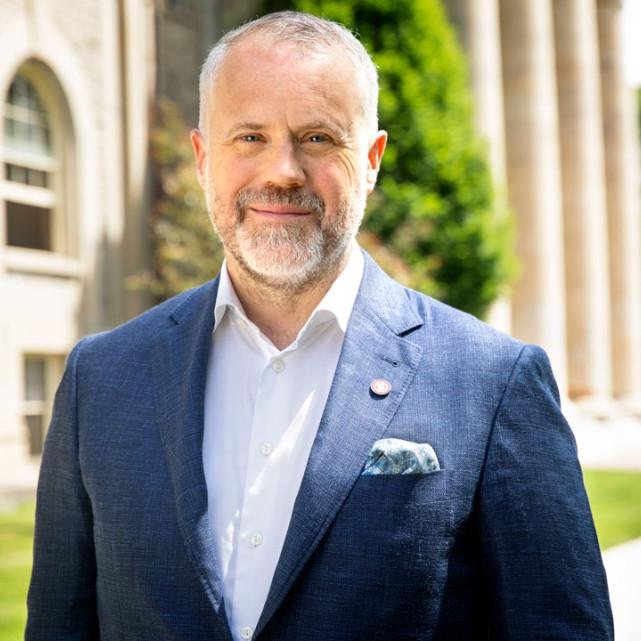
Peter Loewen named dean of Arts and Sciences
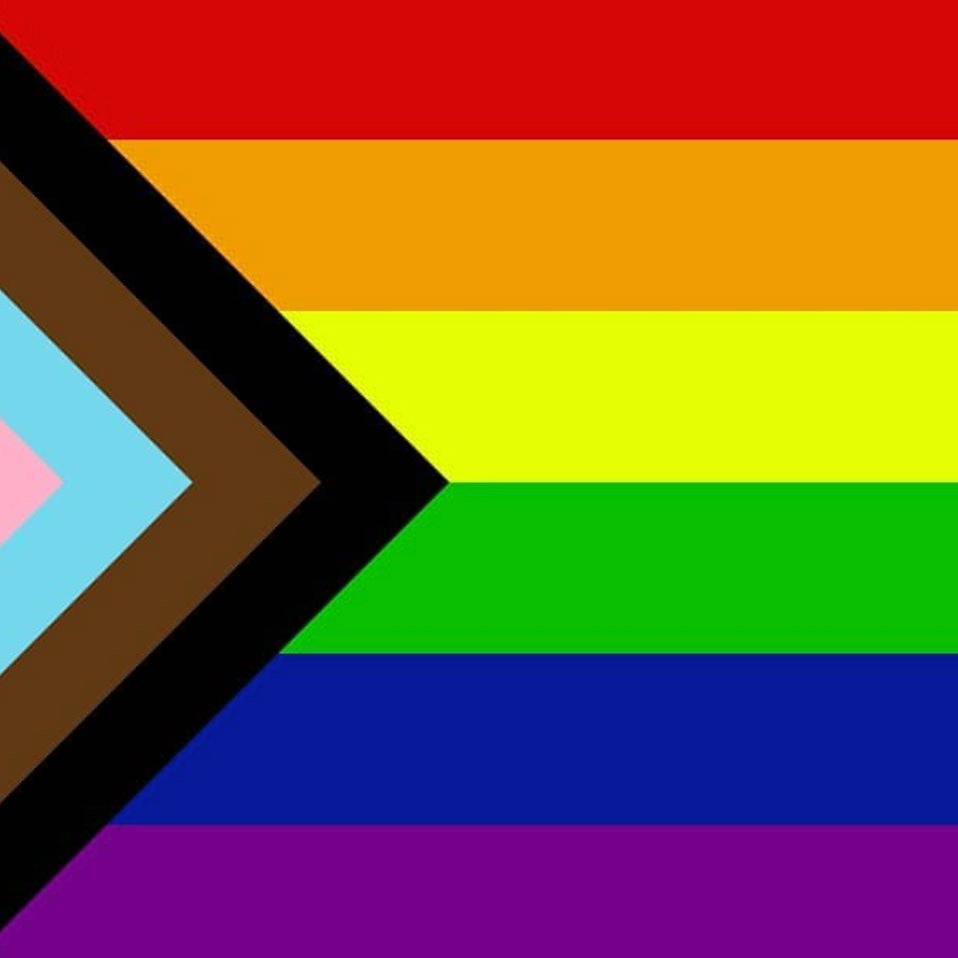
Community event will showcase trans philosophy and scholarship
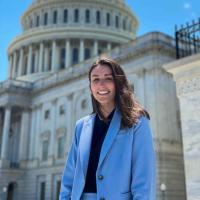
Summer Experience Grant applications now open
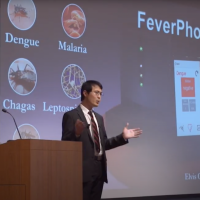
Eight students advance to 3MT finals
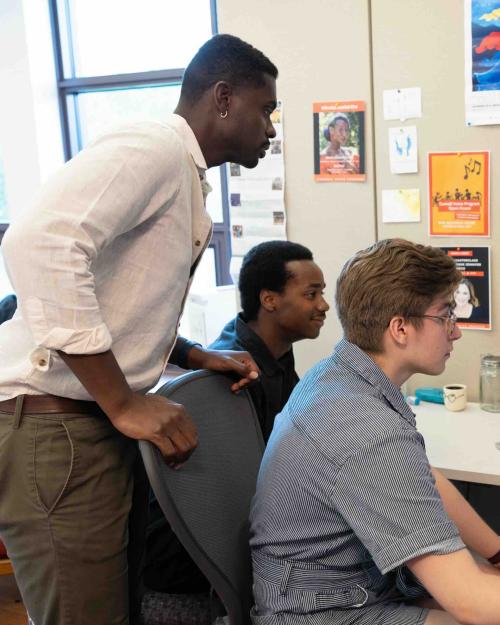

An official website of the United States government
The .gov means it’s official. Federal government websites often end in .gov or .mil. Before sharing sensitive information, make sure you’re on a federal government site.
The site is secure. The https:// ensures that you are connecting to the official website and that any information you provide is encrypted and transmitted securely.
- Publications
- Account settings
Preview improvements coming to the PMC website in October 2024. Learn More or Try it out now .
- Advanced Search
- Journal List
- HHS Author Manuscripts

Beyond Heritability: Twin Studies in Behavioral Research
Wendy johnson.
1 Department of Psychology and Centre for Cognitive Ageing and Cognitive Epidemiology, University of Edinburgh
2 Department of Psychology, University of Minnesota–Twin Cities
Eric Turkheimer
3 Department of Psychology, University of Virginia
Irving I. Gottesman
4 Department of Psychiatry, University of Minnesota–Twin Cities Medical School

Thomas J. Bouchard, Jr.
The heritability of human behavioral traits is now well established, due in large measure to classical twin studies. We see little need for further studies of the heritability of individual traits in behavioral science, but the twin study is far from having outlived its usefulness. The existence of pervasive familial influences on behavior means that selection bias is always a concern in any study of the causal effects of environmental circumstances. Twin samples continue to provide new opportunities to identify causal effects with appropriate genetic and shared environmental controls. We discuss environmental studies of discordant twin pairs and twin studies of genetic and environmental transactions in this context.
In his characteristically colorful language, twin researcher David Lykken occasionally remarked that “Behavior genetics rearranged the furniture in psychology's house” by showing again and again that virtually every trait, from social attitudes to psychopathology, shows genetic influence. It was no Saturday afternoon whim arising out of boredom when behavior genetics moved the behavioral chair over near the genetic lamp. The new arrangement was more accurate: The chair was better placed for scientific reading. By now we have a fundamental understanding that genetic influences are involved in all aspects of psychology and behavior. Turkheimer (2000) even enshrined this as the First Law of Behavioral Genetics, and the law actually underlies all of behavioral science.
Classic twin studies carried out by literally hundreds of researchers have provided an abundance of evidence for this. Throughout the world, perhaps 800,000 pairs of twins have been collected into more than 50 different study samples. Many of these samples would be of considerable epidemiological value even if they did not contain twins, because they are closely representative of the populations from which they have been drawn, having been recruited from birth and other systematic records. Like all experiments, these experiments of nature are not perfect: Differences between twins and singletons, differences between monozygotic (MZ) and dizygotic (DZ) twins, and methodological biases and limitations could be distorting estimates of the extent of genetic influence. But the sheer volume of evidence from twin studies and the corroborating evidence from adoption studies and studies of other combinations of relatives makes it unreasonable to deny the presence of genetic influences on behavior. And because the genotype pre-exists all behavior, these genetic influences have to be considered causal at some level.
BEYOND ESTIMATES OF GENETIC INFLUENCES
The word “causal” above is important. Ultimately, behavioral science is about understanding what causes behavior, psychopathology, disease, and whether particular kinds of circumstances such as substance abuse, poverty, or specific clinical interventions have causal effects on important life outcomes. But studies that can establish causal effects conclusively are rare because of ethical limitations on experimentation in humans, artificiality of laboratory conditions, and uncertainties of extrapolating from experiments with nonhuman animals to humans. Ironically, once we acknowledge the presence of genetic influences on behavior, the value of twin studies shifts from their ability to demonstrate genetic influences to their ability to illuminate causal environmental influences.
To understand why heritability estimates are no longer important, it is necessary to understand that they are completely dependent on the specifics of the samples and environmental conditions from which they are taken. When environments are homogeneous for all, all individual differences become heritable. When there are both genetic and environmental differences, most of the mechanisms through which genes exert their causal influences on behavior are not the straightforward one gene–one (bit of) trait association one learns about in high-school biology. Instead, much gene expression is contingent on the presence of other gene products, environmental circumstances, and prior levels of gene expression, sometimes even in prior generations. Causal genetic influences are thus intimately bound to causal environmental circumstances ( Johnson, 2007 ). People tend to think of heritability estimates as relatively consistent—for example, in adulthood general intelligence is 50% heritable, personality is 40%, and height is 80%—because the traits are broad, continuous, and clearly polygenic, which alone produce stability in the estimates. Moreover, we tend to average these estimates in our heads. In reality, estimates of heritability of general intelligence commonly range from 50 to 80%, personality from 20 to 50%, and even height from 70 to 95%. Such ranges can be demonstrated even within samples (e.g., Krueger, South, Johnson, & Iacono, 2008 ). We are only beginning to understand how inaccuracy of measurement, population-genetic differences, and environmental circumstances may transact to produce these differences in heritabilities. Moreover, even highly heritable traits can be strongly manipulated by the environment, so heritability has little if anything to do with controllability. For example, height is on the order of 90% heritable, yet North and South Koreans, who come from the same genetic background, presently differ in average height by a full 6 inches ( Pak, 2004 ; Schwekendiek, 2008 ).
This means that little can be gleaned from any particular heritability estimate and there is little need for further twin studies investigating the presence and magnitude of genetic influences on behavior. As in psychology 30 years ago, heritability studies do continue to have some importance in areas of the social sciences in which genetic influences have not been acknowledged. For example, Fowler, Baker, and Dawes (2008) demonstrated that more than half of the variation in voting behavior was under genetic influence. In political science, theoretical and empirical models have been able to account only for perhaps a third of the variance (e.g., Plutzer, 2002 ), so the result that more than half the variance could be attributed to genetic influences seems stunning to social scientists used to thinking of genetic influences as deterministic and behavior as the product of circumstantial influences. These scientists have the advantage, however, of realizing the role of genetics in their phenomena of interest in an era when the dependence of genetic expression on environmental circumstances, and thus the limitations of the causal inferences that can be drawn from any estimate of heritability, are becoming increasingly clear.
The presence of genetic influences does not mean that genes “cause” behavior in any preordained way. Rather, it means that genes predispose toward some (tacit or active) ongoing series of individual behavioral choices. These choices have effects on later circumstances that affect later options for genetically influenced behavioral choices, and so on. This causal chain is captured in the behavior-genetic literature through the concept of gene–environment correlation, or genetically influenced differences in environmental exposure. Gene–environment correlation may be passive, active, or evocative ( Plomin, De-Fries, McClearn, & McGuffin, 2008 ). It is passive when, for example, children receive both genes influencing antisocial behavior and abusive treatment from their parents and model the behavior they receive. It is active when people genetically inclined to be social seek social groups in which to participate and avoid spending long periods of time alone. It is evocative when children with genetically influenced difficulties with emotional control throw temper tantrums that generate angry responses from their parents. Gene–environment correlation is described in the developmental psychopathology literature as social selection, in which the association between risk and outcome reflects origin of risk in the individual rather than the effects of risk. For example, poverty may be a risk factor for schizophrenia because people at genetic risk for schizophrenia tend to drift into poverty because of inability to maintain educational and occupational performance, as well as because poverty provides the disease-triggering stress. In the epidemiological literature, gene–environment correlation is described as reverse causation or confounding, in which some to all of the association between an experience and an outcome reflects the effects of people on their environments rather than the effects of their environments on them.
USING TWIN STUDIES TO DISTINGUISH SELECTION FROM ENVIRONMENTAL CAUSATION
This is where twin studies have particular value. Whatever the label, the First Law of Behavioral Genetics implies that it is always possible that common genetic influences creating selection bias underlie any apparently causal, naturally occurring association between environmental circumstance and outcome. But it is not just common genetic influences that create confounds of this type. If poorer children attend inferior schools, live in more poorly lit homes, and do more poorly in third grade than children from wealthier families, there will be an association between home lighting and school performance. If home lighting has no direct effect on school performance, this association will be due to environmental influences shared by members of some families but not by members of other families. The twin design makes possible the control of both genetic and shared environmental background without specifying all the particular mechanisms involved, and thus makes it possible to isolate and test for the presence of the environmental effects of interest. In other words, given that genetic influences are routinely involved in behavior, the importance of twin studies lies not in their ability to estimate those genetic influences but rather because they can be used as quasi-experimental tests of environmental explanations.
To return to voting behavior, there are many reasons that people vote or do not, but some are likely to be related to personality characteristics like dutifulness, to attitudes like belief in democracy, and to environmental circumstances like access to information and ability to reach the polls. These are all transmitted in part both genetically and through culture/shared environment by parents, but exactly how is far from clear. It does not matter, however, if what we want to know is whether belief in democracy, for example, has a direct effect on voting behavior. Once we accept the presence of some lump of genetic and shared-environmental influences on both belief in democracy and voting behavior, it becomes apparent that, in MZ twins who differ in belief in democracy, any difference in their voting behavior cannot be attributed to genetic confounds nor to any aspect of the familial environment that they share, such as sociodemography, parental attitudes toward democracy, or parental voting record. Twin studies not only control for genetic selection; they also control for shared-environmental selection, without identifying either the specific genes or the specific shared-environmental influences involved. Such studies therefore provide tough tests of causal associations. The tests are not completely rigorous, of course: The within-twin pair correlations are still confounded by any other relevant variables that differ between the twins, and there could be relevant ways that life with a co-twin differs from life as a singleton. Still, in the human social sciences, where experimentation is restricted, this kind of quasiexperimental control is important in differentiating potentially causal associations from selection processes.
A SEXY EXAMPLE OF BEHAVIOR GENETICS AT WORK
Sometimes the results of applying such controls also call attention to unstated assumptions in psychological research. For example, Armour and Haynie (2007) investigated the relation between timing of first sex in adolescents and later delinquency, expressing concern that premarital sex was likely to contribute to untoward consequences for adolescents. As they had anticipated, they found a significant association, which they interpreted causally: The experience of early sexual activity contributed to later delinquent behavior. Of course, another possibility is that some other variable, either genetic or environmental, was responsible for the association. Harden, Mendle, Hill, Turkheimer, and Emery (2008) used twins from the same data set to evaluate the association after controlling for genetic and shared-environmental influences on age of first sex and contemporaneous delinquency. Even MZ twins differed considerably in age of first sex, but of course age of first sex was confounded with genetic and shared environmental influences. In contrast to Armour and Haynie's observation, however, Harden et al. found that, within twin pairs, with genetic and shared environmental confounds controlled, the twins with earlier age of first sex actually showed lower levels of delinquency than the co-twins who initiated sexual activity later. The overall association Armour and Haynie had observed was due to selection on genetic and shared environmental influences contributing to both age at first sex and delinquency rather than to age at first sex alone. These results have clear implications for understanding the role of sexual behavior in adolescent development as well as for the development of public health and sex-education programs for adolescents.
In a related vein, intelligence and education are often associated with healthier lifestyle choices ( Deary, Whalley, Batty, & Starr, 2006 ; Gottfredson, 2004 ). Controlling for genetic and shared-environmental influences, however, Johnson, Hicks, McGue, & Iacono (in press) found that both higher IQ and greater education were associated with more alcohol and nicotine use in both sexes at age 24. This suggests that the generally lower substance use among brighter and better-educated young adults results from selection on genetic and family influences contributing to an environment emphasizing both educational attainment and reduced substance use, rather than from direct application of intelligence and/or education in substance-use choices.
UNPACKING THE GENETIC AND SHARED-ENVIRONMENTAL “LUMP”
Ultimately, we want not just to identify purely environmental associations but also to understand how genetic and shared-environmental influences come together to create selection processes. Again, twin studies can help, but not through estimates of heritability. Rather, they can do so through studies of gene–environment transactions, or changes in the patterns of genetic and environmental variances with changes in environmental circumstances. In contrast to studies of discordant twin pairs, studies of gene–environment transactions rely on patterns of relative similarity in MZ and DZ twins to examine population changes in magnitudes of genetic and environmental influences of one trait with levels of another. The models used in these studies still need refinement, but they can also allow for estimation of the extent to which genetic and environmental influences on one trait are also involved in the other. When such overlap exists, changes in magnitudes of genetic and environmental influences are associated with changes in degree of overlap in regular ways. Because overlap on a trait and a putative environmental circumstance is an indication of selection bias, the models reveal patterns of both differences in genetic expression and selection effects.
For example, there is a well-known and robust negative association between income and/or socioeconomic status and physical health problems. Less affluent people tend to have poorer health and to die younger. Johnson and Krueger (2005) used a gene–environment transaction study to understand this association. There was more genetic variance in physical health problems among those with low income and/or low perceived personal control. One interpretation of this result is that more stressful living conditions associated with lower income or personal control trigger greater expression of genetic vulnerabilities to health problems. Put another way, more affluent people and those with greater perceived control are freer from “genetic destiny.”
In addition, at higher levels of income and perceived control, genetic influences on physical health problems largely overlapped those on income and perceived control. When income and perceived control were low, however, there was much less overlap. The possible interpretation? Genetically influenced personal characteristics involved in earning greater income and maintaining control of one's life are also characteristics that can be used to prevent the development of physical health problems. At the same time, the poorer health of people in lower income/perceived-control situations occurs because the environmental stress they experience causes greater expression of genetic vulnerabilities unrelated to those personal characteristics. Studies of biological responses to stress increasingly support this view. This interpretation does not depend on any specific genetic polypmorphisms: The definition of physical health problems was intentionally broad and included a variety of medical conditions.
The discovery that all behavior is partially heritable transformed psychology, but, ironically, it also transformed behavior genetics. Once we accept that basically everything—not only schizophrenia and intelligence, but also marital status and television watching—is heritable, it becomes clear that specific estimates of heritability are not very important. The omnipresence of genetic influences does not demonstrate that behavior is “less psychological” or “more biologically determined” than had originally been thought; rather it shows that behavior arises from factors intrinsic as well as extrinsic to the individual. The real implications of heritability lie not in questions of relative biological determinism but in revealing the need to understand both the mechanisms through which the individual, whether consciously or not, directs his or her own life course and his or her power to do so. In psychology, where it is not ethically possible to conduct randomized experiments on life outcomes, the natural experiment provided by the twin study can be most helpful in addressing these issues.
Recommended Reading
- Bouchard TJ., Jr. Experience Producing Drive Theory: How genes drive experience and shape personality. Acta Paediatrica, Supplement. 1997; 422 :60–64. [ PubMed ] [ Google Scholar ] An outline of a theory explaining how selection processes develop.
- Johnson W. 2007. (See References). An exploration of the quantitative measurement of interplay between genetic and environmental influences as they are manifested in population-level social forces.
- Rutter M. Proceeding from observed correlation to causal inference: The use of natural experiments. Perspectives on Psychological Science. 2007; 2 :377–395. [ PubMed ] [ Google Scholar ] A discussion of the use of twin studies and other situations with naturally occurring quasiexperimental controls to examine causality.
- Turkheimer E. Heritability and biological explanation. Psychological Review. 1998; 105 :782–791. [ PubMed ] [ Google Scholar ] An exploration of the meaning of heritability and biology to psychology.
- Visscher PM, Hill WG, Wray NR. Heritability in the genomics era: Concepts and misconceptions. Nature Reviews Genetics. 2008; 9 :255–266. [ PubMed ] [ Google Scholar ] A good exposition of the properties of and concepts involved in the heritability statistic.
- Armour S, Haynie DL. Adolescent sexual debut and later delinquency. Journal of Youth and Adolescence. 2007; 36 :141–152. [ Google Scholar ]
- Deary IJ, Whalley LJ, Batty GD, Starr JM. Physical fitness and lifetime cognitive change. Neurology. 2006; 67 :1195–1200. [ PubMed ] [ Google Scholar ]
- Fowler JH, Baker LA, Dawes CT. Genetic variation in political participation. American Political Science Review. 2008; 102 :233–248. [ Google Scholar ]
- Gottfredson LS. Intelligence: Is it the epidemiologists' elusive “fundamental cause” of social class inequalities in health? Journal of Personality and Social Psychology. 2004; 86 :174–199. [ PubMed ] [ Google Scholar ]
- Harden KP, Mendle J, Hill JE, Turkheimer E, Emery RE. Rethinking timing of first sex and delinquency. Journal of Youth and Adolescence. 2008; 37 :373–385. [ PMC free article ] [ PubMed ] [ Google Scholar ]
- Johnson W. Genetic and environmental influences on behavior: Capturing all the interplay. Psychological Review. 2007; 114 :424–440. [ PubMed ] [ Google Scholar ]
- Johnson W, Hicks BM, McGue M, Iacono WG. How intelligence and education contribute to substance use: Hints from the Minnesota Twin Family Study. Intelligence. (in press) [ PMC free article ] [ PubMed ] [ Google Scholar ]
- Johnson W, Krueger RF. Higher perceived life control decreases genetic variance in physical health: Evidence from a national twin study. Journal of Personality and Social Psychology. 2005; 88 :165–173. [ PubMed ] [ Google Scholar ]
- Krueger RF, South S, Johnson W, Iacono W. The heritability of personality is not always 50%: Gene–environment interactions and correlations between personality and parenting. Journal of Personality. 2008; 76 :1485–1521. [ PMC free article ] [ PubMed ] [ Google Scholar ]
- Pak S. The biological standard of living in the two Koreas. Economics and Human Biology. 2004; 2 :511–521. [ PubMed ] [ Google Scholar ]
- Plomin R, DeFries JC, McClearn GE, McGuffin P. Behavioral Genetics. 5th ed. Worth; New York: 2008. [ Google Scholar ]
- Plutzer E. Becoming a habitual voter: Inertia, resources, and growth in young adulthood. American Political Science Review. 2002; 96 :41–56. [ Google Scholar ]
- Schwekendiek D. Height and weight differences between North and South Korea. Journal of Biosocial Sciences. 2008; 41 :51–55. [ PubMed ] [ Google Scholar ]
- Turkheimer E. Three laws of behavior genetics and what they mean. Current Directions in Psychological Science. 2000; 9 :160–164. [ Google Scholar ]
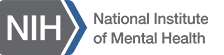
Transforming the understanding and treatment of mental illnesses.
Información en español
Celebrating 75 Years! Learn More >>
- Health Topics
- Brochures and Fact Sheets
- Help for Mental Illnesses
- Clinical Trials
Substance Use and Co-Occurring Mental Disorders
What does it mean to have substance use and co-occurring mental disorders.
Substance use disorder (SUD) is a treatable mental disorder that affects a person’s brain and behavior, leading to their inability to control their use of substances like legal or illegal drugs, alcohol, or medications. Symptoms can be moderate to severe, with addiction being the most severe form of SUD.
People with a SUD may also have other mental health disorders, and people with mental health disorders may also struggle with substance use. These other mental health disorders can include anxiety disorders , depression , attention-deficit hyperactivity disorder (ADHD) , bipolar disorder , personality disorders , and schizophrenia , among others. For more information, please see the National Institute on Drug Abuse (NIDA) Common Comorbidities with Substance Use Disorders Research Report .
Though people might have both a SUD and a mental disorder, that does not mean that one caused the other. Research suggests three possibilities that could explain why SUDs and other mental disorders may occur together:
- Common risk factors can contribute to both SUDs and other mental disorders. Both SUDs and other mental disorders can run in families, meaning certain genes may be a risk factor. Environmental factors, such as stress or trauma, can cause genetic changes that are passed down through generations and may contribute to the development of a mental disorder or a substance use disorder.
- Mental disorders can contribute to substance use and SUDs. Studies found that people with a mental disorder, such as anxiety, depression, or post-traumatic stress disorder (PTSD) , may use drugs or alcohol as a form of self-medication. However, although some drugs may temporarily help with some symptoms of mental disorders, they may make the symptoms worse over time. Additionally, brain changes in people with mental disorders may enhance the rewarding effects of substances, making it more likely they will continue to use the substance.
- Substance use and SUDs can contribute to the development of other mental disorders. Substance use may trigger changes in brain structure and function that make a person more likely to develop a mental disorder.
How are substance use disorder and co-occurring mental disorders diagnosed and treated?
When someone has a SUD and another mental health disorder, it is usually better to treat them at the same time rather than separately. People who need help for a SUD and other mental disorders should see a health care provider for each disorder. It can be challenging to make an accurate diagnosis because some symptoms are the same for both disorders, so the provider should use comprehensive assessment tools to reduce the chance of a missed diagnosis and provide the right treatment.
It also is essential that the provider tailor treatment, which may include behavioral therapies and medications, to an individual’s specific combination of disorders and symptoms. It should also take into account the person’s age, the misused substance, and the specific mental disorder(s). Talk to your health care provider to determine what treatment may be best for you and give the treatment time to work.
Behavioral therapies
Research has found several behavioral therapies that have promise for treating individuals with co-occurring substance use and mental disorders. Health care providers may recommend behavioral therapies alone or in combination with medications.
Some examples of effective behavioral therapies for adults with SUDs and different co-occurring mental disorders include:
- Cognitive behavioral therapy (CBT) is a type of talk therapy aimed at helping people learn how to cope with difficult situations by challenging irrational thoughts and changing behaviors.
- Dialectical behavior therapy (DBT) uses concepts of mindfulness and acceptance or being aware of and attentive to the current situation and emotional state. DBT also teaches skills that can help control intense emotions, reduce self-destructive behaviors (such as suicide attempts, thoughts, or urges; self-harm; and drug use), and improve relationships.
- Assertive community treatment (ACT) is a form of community-based mental health care that emphasizes outreach to the community and an individualized treatment approach.
- Therapeutic communities (TC) are a common form of long-term residential treatment that focuses on helping people develop new and healthier values, attitudes, and behaviors.
- Contingency management (CM) principles encourage healthy behaviors by offering vouchers or rewards for desired behaviors.
Behavioral therapies for children and adolescents
Some effective behavioral treatments for children and adolescents include:
- Brief strategic family therapy (BSFT) therapy targets family interactions thought to maintain or worsen adolescent SUDs and other co-occurring problem behaviors.
- Multidimensional family therapy (MDFT) works with the whole family to simultaneously address multiple and interacting adolescent problem behaviors, such as substance use, mental disorders, school problems, delinquency, and others.
- Multisystemic therapy (MST) targets key factors associated with serious antisocial behavior in children and adolescents with SUDs.
Medications
There are effective medications that treat opioid , alcohol , and nicotine addiction and lessen the symptoms of many other mental disorders. Some medications may be useful in treating multiple disorders. For more information on behavioral treatments and medications for SUDs, visit NIDA’s Drug Facts and Treatment webpages. For more information about treatment for mental disorders, visit NIMH's Health Topics webpages.
How can I find help for substance use and co-occurring mental disorders?
To find mental health treatment services in your area, call the Substance Abuse and Mental Health Services Administration (SAMHSA) National Helpline at 1-800-662-HELP (4357), visit the SAMHSA online treatment locator , or text your ZIP code to 435748.
For additional resources about finding help, visit:
NIMH's Help for Mental Illnesses page
National Cancer Institute’s Smokefree.gov website, or call their smoking quitline at 1-877-44U-QUIT (1-877-448-7848)
If you or someone you know is struggling or having thoughts of suicide, call or text the 988 Suicide & Crisis Lifeline at 988 or chat at 988lifeline.org . In life-threatening situations, call 911.
How can I find a clinical trial for substance use and co-occurring mental disorders?
Clinical trials are research studies that look at new ways to prevent, detect, or treat diseases and conditions. The goal of clinical trials is to determine if a new test or treatment works and is safe. Although individuals may benefit from being part of a clinical trial, participants should be aware that the primary purpose of a clinical trial is to gain new scientific knowledge so that others may be better helped in the future.
Researchers at NIMH and around the country conduct many studies with patients and healthy volunteers. We have new and better treatment options today because of what clinical trials uncovered years ago. Be part of tomorrow’s medical breakthroughs. Talk to your health care provider about clinical trials, their benefits and risks, and whether one is right for you.
To learn more or find a study, visit:
- NIMH’s Clinical Trials webpage : Information about participating in clinical trials related to mental disorders
- Clinicaltrials.gov: Current studies on mental illness and substance misuse : List of clinical trials funded by the National Institutes of Health (NIH) being conducted across the country
Where can I learn more about substance use and co-occurring disorders?
Brochures and other educational resources.
- National Institute on Alcohol Abuse and Alcoholism (NIAAA) Publications Order Form
- NIDA: Parents and Educators
- SAMHSA Publications and Digital Products
- Alcohol Use Disorder (also en español )
- Drug Use and Addiction (also en español )
- Mental Health and Behavior (also en español )
- Opioids and Opioid Use Disorder (also en español )
- Risks of tobacco (also en español )
- NIH Experts Discuss the Intersection of Suicide and Substance Use : Learn about common risk factors, populations at elevated risk, suicides by drug overdose, treatments, prevention, and resources for finding help.
- NIDA Common Physical and Mental Health Comorbidities with Substance Use Disorders Research Report
- NIDA Tobacco, Nicotine, and E-Cigarettes Research Report
- SAMHSA National Survey on Drug Use and Health
- Suicide Deaths Are a Major Component of the Opioid Crisis that Must Be Addressed
- NIMH and the NIH HEAL Initiative: Collaborating to address the opioid epidemic
- NIMH’s Role in the NIH HEAL Initiative
Last reviewed: March 2024
Unless otherwise specified, the information on our website and in our publications is in the public domain and may be reused or copied without permission. However, you may not reuse or copy images. Please cite the National Institute of Mental Health as the source. Read our copyright policy to learn more about our guidelines for reusing NIMH content.
Thank you for visiting nature.com. You are using a browser version with limited support for CSS. To obtain the best experience, we recommend you use a more up to date browser (or turn off compatibility mode in Internet Explorer). In the meantime, to ensure continued support, we are displaying the site without styles and JavaScript.
- View all journals
- Explore content
- About the journal
- Publish with us
- Sign up for alerts
- Open access
- Published: 29 July 2024
Genetic factors associated with reasons for clinical trial stoppage
- Olesya Razuvayevskaya 1 , 2 ,
- Irene Lopez 1 , 2 ,
- Ian Dunham 1 , 2 , 3 &
- David Ochoa ORCID: orcid.org/0000-0003-1857-278X 1 , 2
Nature Genetics ( 2024 ) Cite this article
17k Accesses
1 Citations
59 Altmetric
Metrics details
- Drug discovery
- Medical genetics
- Therapeutics
Many drug discovery projects are started but few progress fully through clinical trials to approval. Previous work has shown that human genetics support for the therapeutic hypothesis increases the chance of trial progression. Here, we applied natural language processing to classify the free-text reasons for 28,561 clinical trials that stopped before their endpoints were met. We then evaluated these classes in light of the underlying evidence for the therapeutic hypothesis and target properties. We found that trials are more likely to stop because of a lack of efficacy in the absence of strong genetic evidence from human populations or genetically modified animal models. Furthermore, certain trials are more likely to stop for safety reasons if the drug target gene is highly constrained in human populations and if the gene is broadly expressed across tissues. These results support the growing use of human genetics to evaluate targets for drug discovery programs.
Similar content being viewed by others
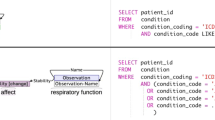
The Leaf Clinical Trials Corpus: a new resource for query generation from clinical trial eligibility criteria
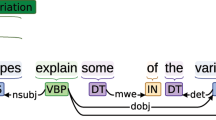
PGxCorpus, a manually annotated corpus for pharmacogenomics
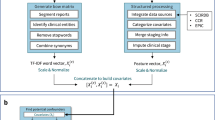
Uncovering interpretable potential confounders in electronic medical records
The drug discovery endeavor is dominated by high attrition rates, and failure remains the most likely outcome throughout the pipeline 1 . A diverse set of factors can lead to failure, with lack of efficacy or unforeseen safety issues reportedly explaining 79% of setbacks in the clinic 2 . New approaches adopted across the industry have aimed to improve success rates by systematically assessing the available evidence throughout the research and clinical pipelines 3 , 4 . Support from human genetic evidence has been repeatedly associated with successful clinical trial progression 5 , 6 , 7 , 8 , ultimately supporting two-thirds of the drugs approved by the US Food and Drug Administration (FDA) in 2021 (ref. 9 ). Further understanding of the reasons for success or failure in clinical trials could assist in reducing future attrition.
Systematically assessing the reasons for success or failure in clinical trials can be hampered by many factors. Several surveys have demonstrated a bias towards reporting positive results, with 78.3% of trials in the literature reporting successful outcomes 10 , 11 , 12 . Successful clinical trials are published significantly faster than trials reporting negative results 13 , 14 . However, access to negative results is crucial, not only for revealing efficacy tendencies and safety liabilities 15 but also for retrospective review and benchmarking of predictive methods, including machine learning.
Since 2007, the FDA has required the submission of clinical trial results to ClinicalTrials.gov, a free-to-access global databank aimed at registering clinical research studies and their results 16 , 17 . For trials halted before their scheduled endpoint, ClinicalTrials.gov provides a freeform stopping reason: termination, suspension or withdrawal 18 . A team of researchers 19 previously classified the reasons for 3,125 stopped trials and found that only 10.8% of trials stopped because of a clear negative outcome. By contrast, the majority (54.5%) fell into a set of reasons characterized as neutral in relation to the therapeutic hypothesis, such as patient recruitment or other business or administrative reasons 19 .
Here, we extended that work by training a natural language processing (NLP) model to classify stopping reasons and used this model to classify 28,561 stopped trials. We integrated our classification with evidence associating the drug target and disease from the Open Targets Platform 20 , revealing that trials stopped for lack of efficacy or safety reasons were less supported by genetic evidence. Furthermore, oncology trials involving drugs for which the target gene is constrained in human populations were more likely to stop for safety reasons, whereas drugs with targets with tissue-selective expression were less likely to pose safety risks. These observations confirm and extend previous studies recognizing the value of genetic information and selective expression in target selection.
Interpretable classification of early stoppage reasons
To catalog the reasons behind the withdrawal, termination or suspension of clinical studies, we classified every free-text reason submitted to ClinicalTrials.gov using an NLP classifier. To build a training set for our model, we revisited the manual classification reported in a previous publication of 3,124 stopped trials based on the available submissions to ClinicalTrials.gov in May 2010 (ref. 19 ). The authors of that article classified every study with a maximum of three classes following an ontological structure (Supplementary Table 1 ). Each of the classes was also assigned a higher-level category representing the outcome implications for the clinical project. For example, 33.7% of the studies were classified as stopped owing to ‘insufficient enrollment’, a neutral outcome owing to its expected independence from the therapeutic hypothesis. When inspecting submitted reasons belonging to the same curated category, we observed a strong linguistic similarity, as revealed by clustering the cosine similarity of the sentence embeddings (Extended Data Fig. 1 ). Studies stopped because of reasons linked to lack of efficacy and studies stopped because of futility have a linguistic similarity of 0.98, with both classes manually classified as ‘negative’ outcomes. Based on this clustering, we redefined the classification by merging semantically similar classes represented by low numbers of annotated sentences. Moreover, we added 447 studies that were stopped as a result of the COVID-19 pandemic (Supplementary Table 2 ), resulting in a total of 3,571 studies manually classified into at least one of 17 stop reasons and explained by six different higher-level outcome categories.
By leveraging the consistent language used by the submitters, we fine-tuned the BERT model 21 for the task of clinical trial classification into stop reasons ( Methods ). Overall, the model showed strong predictive power in the cross-validated set ( F micro = 0.91), performing strongly for the most frequent classes, such as ‘insufficient enrollment’ ( F = 0.98) or ‘COVID-19’ ( F = 1.00), but demonstrating decreased performance on linguistically complex reasons, such as trials stopped because of another study ( F = 0.71) (Supplementary Table 3 ).
To further evaluate the model, we manually curated an additional set of 1,675 stop reasons from randomly selected studies that were not included in the training set. Overall, the performance against the unseen data was lower but comparable to that of the cross-validated model ( F micro ranging from 0.70 to 0.83 depending on the choice of the annotator) (Supplementary Table 4 ), demonstrating real-world performance and reduced risk of overfitting. Interestingly, the curators demonstrated a relatively low agreement for many classes in which the machine-learning model also showed relatively weak performance, such as studies stopped because of insufficient data or met endpoint ( Methods and Extended Data Fig. 1 ).
Reasons reflect operational, clinical and biological constraints
Classification of the 28,561 stopped trials submitted to ClinicalTrials.gov before 27 November 2021 was performed using our NLP model fine-tuned on all the manually curated sentences (Supplementary Table 5 ). In total, 99% of the trials were classified with at least one of the 15 potential reasons and mapped to one of six different higher-level outcomes (Fig. 1 ). ‘Insufficient enrollment’ remained the most common reason to stop a trial (36.67%), with other reasons before the accrual of any study results also occurring in a large number of studies. A total of 977 trials (3.38%) were classified as stopped because of ‘safety or side effects’, and 2,197 studies (7.6%) were stopped because of ‘negative’ reasons, such as those questioning the efficacy or value (futility). The incidence of each stop reason reflects the purpose of each phase (Extended Data Fig. 2 ). Studies stopped because of ‘negative’ outcomes more often impacted phase II (odds ratio (OR) = 1.9, P = 2.4 × 10 − 38 ) and phase III (OR = 2.6, P = 3.64 × 10 − 55 ), whereas studies stopped as a result of ‘safety or side effects’ declined in relative incidence after phase I (OR = 2.4, P = 9.63 × 10 − 23 ) (Supplementary Table 6 ). Trials stopped because of the relocation of the study or key staff occurred more than twice as often during early phase I, highlighting the importance of good clinical practices during the foundational stages. Of the studies that provided a stop reason, 48% were indicated for oncology. This large proportion is likely to be the combined result of the specific weight of oncology indications in the aggregated portfolio—27% of drug approvals in 2022—with the reported large incidence of clinical failures in oncology (32%) compared to other indications 22 , 23 .
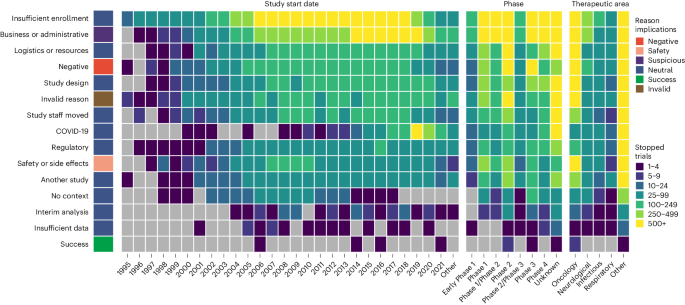
Predicted trial stop reasons are shown in rows with counts of trials per start year, clinical phase or therapeutic area shown by the color in each cell. The outcome groupings of the stopped reasons are shown using the color next to the stopped reason label. Note that trials start potentially many years before they are stopped.
Moreover, oncology studies stopped more frequently as a result of safety or side effects and were rarely stopped because of the COVID-19 pandemic (Extended Data Fig. 3 ). Alternatively, COVID-19 was the reported reason to stop respiratory studies at a higher rate than any other therapeutic area, possibly indicating increased operational difficulties.
Genetic support for stopped trials influences the outcome
To better understand the underlying reasons that might have caused the study to fail, we assessed the availability of different types of potentially causal genetic evidence for the intended pharmacological targets in the same indication (Extended Data Fig. 4 ). By using genetic evidence collated by the Open Targets Platform, we reproduced previous reports indicating that genetically supported studies are more likely to progress through the clinical pipeline (Fig. 2a ) 5 , 6 . Interestingly, we also observed that stopped trials—among all the trials at any phase—are depleted in genetic support (OR = 0.73, P = 3.4 × 10 − 69 ). A similar lack of genetic evidence was observed for the three types of stopped studies: withdrawn, terminated and suspended (Supplementary Table 7 ).
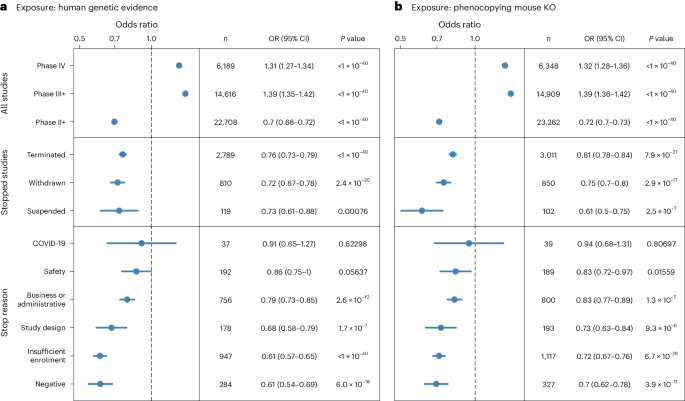
a , b , Genetic evidence support for clinical trials either from human genetics studies ( a ) or the International Mouse Phenotyping Consortium mouse knockouts (KO) that phenocopy the human disease ( b ). The panels show the odds ratio (OR) of support for the target-disease hypothesis from genetics evidence for all clinical trials split by phase (top row), stopped clinical trials (center row) and stopped clinical trials split by higher-level stopping reason (bottom row). The significance of the association between genetic evidence and trial outcome was assessed using a two-tailed Fisher’s exact test, with a P value threshold of 0.05 without multiple testing correction. The panels show the OR and 95% confidence intervals (CIs) for the association between genetic evidence and each subclass of trial. Significant ORs of >1 indicate enrichment and <1 indicate depletion.
When stratifying the stopped studies by reason, trials halted because of negative outcomes—such as lack of efficacy or futility—displayed a significant decrease of genetic support for the intended pharmacological target in the same indication (OR = 0.61, P = 6×10 −18 ) (Fig. 2a ). The depletion of genetic evidence on negative outcomes remains consistent when stratifying the indications by oncology (OR = 0.53) or non-oncology studies (OR = 0.75) (Extended Data Fig. 6 ), as well as when splitting by different sources of genetic evidence, including genome-wide association studies processed by the Open Targets Genetics Portal 24 , gene burden tests based on sequencing of large population cohorts 25 , 26 , 27 , ClinVar 28 , ClinGen Gene Validity 29 , Genomics England PanelApp 30 , gene2phenotype 31 , Orphanet 32 and Uniprot 33 (Extended Data Fig. 5 ).
Other predicted reasons for stopping the trials, such as insufficient enrollment, problems with the study design or business or administrative reasons, also present a strong to moderate depletion of genetic evidence denoting potential reduced support for the therapeutic hypothesis (Fig. 2 ). We found that studies stopped as a result of coincidental factors such as the COVID-19 pandemic have no association with the availability of genetic support for the intended target in the primary indication.
The observed associations between clinical trial outcomes and the availability of genetic support remain consistent when considering genetic information in mouse models (Fig. 2b ). Trials that were stopped because of negative factors present the weakest support among all predicted reasons (OR = 0.7, P = 4 × 10 −11 ) when genetic evidence is defined as the presence of a murine model in which the drug target homologous gene knockout causes a phenotype that mimics the indication, as reported by the International Mouse Phenotyping Consortium 34 .
Genetic factors associated with safety-associated stopped trials
Analysis of the classified stop reasons indicates that oncology trials are more likely to stop because of safety or side effects (OR = 2.14, P = 8.1 × 10 −79 ; Supplementary Table 7 ). Moreover, for all trials predicted to stop because of safety concerns, we found a significant enrichment in targets associated with driver events reported by COSMIC 35 , ClinVar 28 or IntOgen 36 (Extended Data Fig. 7 ). Examining the target properties (Fig. 3 ), we found that studies targeting genes that are highly constrained in natural populations (GnomAD pLOEUF 16th percentile) are 1.5 times more likely to stop as a result of safety concerns 37 . Furthermore, the risk of stopping because of safety declines as the genetic constraint of the target decreases. Similarly, we identified a 1.4-fold increased risk of stopping because of safety concerns when the targeted gene is classified as loss-of-function intolerant (pLI > 0.9). These findings are compatible with previous evidence indicating that constrained genes are associated with increased side effects 38 . We also identified functional genomic features that inform on increased safety risk. According to the human protein atlas, a similar 1.3-fold increased risk is observed for genes expressed with low tissue specificity 39 . Instead, studies targeting tissue-enriched genes show a lower-than-expected (OR = 0.8, P = 1.8 × 10 −4 ) likelihood of stopping because of safety. Finally, targets physically interacting with ten or more different partners according to the IntAct database (MI score > 0.42) present an increased risk of stopping as a result of safety concerns 40 . Further stratification of this analysis by indication denotes that these overall constraint signals impacting studies that are stopped because of safety are largely influenced by oncology trials.
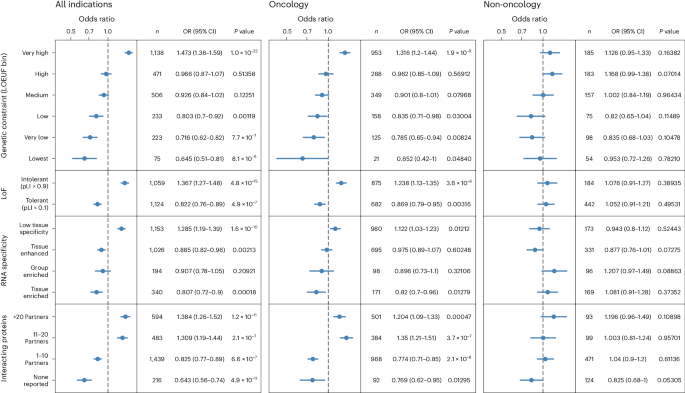
We evaluated the significance of the association between trials stopping because of safety or side effects and each variable (therapeutic area, relative genetic constraint as defined by GnomAD, tissue specificity as defined by the Human Protein Atlas and network connectivity with data from IntAct) with a two-tailed Fisher’s exact test, with a P value threshold of 0.05 without multiple testing correction. OR > 1 represents an increased risk of study stopping and OR < 1 represents protection against stopping. Error bars, 95% CI; LoF, loss of function. Detailed results are presented in Supplementary Table 7 .
Genetic evidence is increasingly leveraged by the pharmaceutical industry to add support to the therapeutic hypothesis 3 , 4 , 41 , 42 . Adding to previous observations on the role of genetic factors in overall trial success 5 , 6 , we exploited under-used data from clinical trial records to better understand the opposite outcome: why clinical trials stop. Although the availability of genetic evidence might inform future success, failure remains the most common outcome of clinical studies, and, to our knowledge, no systematic evidence exists on the relevance of genetics to de-risk negative results.
Recent reports indicate that 79% of clinical studies fail because of a lack of efficacy or safety 2 . Our analysis indicates that within the 7.9% of studies that stop early because of withdrawal, termination or suspension, the proportion of trials that failed because of efficacy or safety is only 12.7%. Stopped studies are more likely to fail because of early coincidental factors that are not necessarily linked to biological plausibility; for example, the principal investigator relocates or there is insufficient enrollment in the trial. Notwithstanding the reduced relative risk of efficacy and safety as the main causes for stopping the trial, these studies provide a significant body of unsuccessful results that are probably explained by a weak therapeutic hypothesis. Continued expansion of the recording of negative results from clinical trials, including stoppages, will be valuable. To assist in this effort, we will continue to update the classification of stopped studies through the Open Targets Platform ( https://platform.opentargets.org ) 20 . Further investigation of the study outcomes for completed studies could expand our understanding of the reasons behind unsuccessful trials, particularly after accrual of the study results.
Our analysis exploits the classified stop reasons to understand the relative importance of the causes leading to failed studies. By using a case-control approach, we conclude that genetic support is not only predictive of clinical trial progression but also protective of early trial stoppage. We illustrate different ways in which genetic causality and genetic constraint can de-risk the target selection process. However, many stopped trials, even when stopped for efficacy and safety reasons, might be explained by factors beyond the intended pharmacological target. Off-target effects, pharmacokinetics, drug delivery or toxicology are other risks not considered in this study that might also explain a set of negative outcomes. Another limitation of our study is that the reasons submitted to ClinicalTrials.gov might only represent a fraction of all the reasons contributing to the decision to halt the study. For example, we found that studies that were classified as stopped because of patient recruitment manifest weaker genetic support, an observation that we did not anticipate owing to the lack of an obvious link between enrollment and biological plausibility. Hence, we reason that a fraction of the stopped trials might present an overall lack of confidence in the therapeutic hypothesis, independently of the reported reason.
This study showcases how reflecting on past failures can inform the relative importance of the risks associated with early target identification and prioritization. Although clinical trial success is a discrete outcome, failure needs to be understood as a breakdown of many possible causes. A proper set of positive and negative outcomes such as the ones introduced in this work represent the groundwork necessary to implement quantitative or semi-automatic models to objectively de-risk any future studies.
Inclusion and ethics
This study relied solely on aggregated genetic and clinical information available in public resources. It did not make use of individual-level data, and no specific ethics approval was required. Some of the data sources, including clinical study results, clinical curation of rare variants or genome-wide associations, might present biases towards European ancestries.
NLP classification of stopped clinical trials
To quantify the semantic structure of the reasons for clinical trial stop, we analyzed the classification of the stopped clinical trials developed in a previous publication 19 . We trained a long short-term memory network to create the representations for each stop reason and averaged the embeddings across all examples of a particular class. The class embeddings were then used to calculate the cosine similarities among classes and were visualized using agglomerative hierarchical clustering (Extended Data Fig. 1 ). The hierarchical representation illustrates the clusters that are semantically close to each other, along with the number of examples per class and the parent category. Similar classes with fewer sentences were grouped together to ensure representative categories based on clinical expertise and semantic similarity. For example, the classes ‘study moved’ and ‘key staff left’ are semantically clustered together and attend to similar underlying reasons. The list of categories defined in the previous publication and their redefined groupings can be found in Supplementary Table 8 .
To validate the model on new data and expand the training set, we performed a human annotation experiment of 1,675 additional ClinicalTrials.gov studies that were not classified in the previous publication. We randomly assigned six sets of 250 unique stopped trials to each curator, including 25 overlapping trials, to estimate the inter-annotator agreement. Across 3 pairs of annotators, we estimated inter-annotator agreements of 0.8, 0.71 and 0.66 using the kappa statistic 43 .
Stop reason classification model
We fine-tuned the BERT model for the task of predicting the stop reasons on the training set of 4,500 human-annotated stopped clinical trials 21 . We used a BERT uncased pre-trained model with a one-layer feed-forward classifier consisting of a ReLU layer between the input and output layers, in which the input and output layers represent linear layers. Fine-tuning was performed by using the HuggingFace transformer library 44 . The classifier uses 50 hidden units and the ReLU activation function.
We used the last hidden state at token ‘[CLS]’ to retrieve a representation of the whole explanation and fed it into the classifier. We then applied ‘sigmoid’ over the logits to retrieve the probabilities. The best accuracy on the validation set was achieved while training the model for seven epochs with a batch size of 32, a learning rate of 5 × 10 − 5 and the Pytorch implementation of Adam’s optimizer with weights decay, in which the weight decay is set to the default value of 1 × 10 − 2 . The test set was created stratified to ensure that the relative class frequencies were considered in each fold of the test set. Given that the nature of the task does not assume that the categories are mutually exclusive and the original and new annotation tasks allowed human annotators to mark up to three categories, we treated the top three probabilities returned by the model that are above a pre-defined threshold as correct answers.
Clinical studies
We collated all clinical trials from ClinicalTrials.gov as of 27 November 2021 and classified the 28,561 stopped studies (withdrawn, suspended or terminated). Genetic traits and indications from clinical studies were harmonized using the Experimental Factor Ontology (EFO) 45 . When studies contained multiple indications, their similarity based on the EFO structure was evaluated. All indications were considered when indications were similar (for example, several oncology indications). When indications were dissimilar (for example, diabetes in malaria patients), the diseases were curated to annotate the appropriate indication for the study. Drugs reported as approved by the FDA were also considered to ensure the representation of medicines preceding the ClinicalTrials.gov resource. To map each drug or clinical candidate to its pharmacological targets, we leveraged the molecule mechanism of action from the ChEMBL database 46 . All possible annotations were used if a drug could be mapped to multiple targets. All drug targets were annotated against Ensembl gene IDs 47 when possible. To perform subsequent analyses, only drugs with a known mechanism of action were considered. The resulting dataset contains 594,375 clinical target-disease records, capturing 71,419 unique target-disease associations and 57,775 target-disease pairs in studies that stopped early 48 .
Target-disease genetic support
We integrated 13 sources available in the Open Targets Platform in April 2022 to extract a comprehensive list of genetically supported gene–disease associations. The genetic evidence was mapped to Ensembl gene identifiers and EFO identifiers as part of the Open Targets activities. To represent common disease genetics, we leveraged Open Targets Genetics 24 , a post-genome-wide association study analysis leveraging different functional genomics features. In this study, we used all gene assignments based on a locus-to-gene score above 0.05 (ref. 49 ). The other predominantly germline genetic sources included in this analysis are Gene Burden 25 , 26 , 27 , ClinVar 28 , Genomics England PanelApp 30 , Gene2Phenotype 31 , Clingen Gene–Disease Validity 29 , Uniprot 33 and Orphanet 32 . We included COSMIC Cancer Hallmarks 35 , IntOgen cancer drivers 36 and ClinVar somatic variants 50 as sources of somatic genetic evidence. As a source to capture the effects of genetic variation in animal models, we included the mouse–human phenotypic mappings reported by the International Mouse Phenotyping Consortium 34 . Genetic evidence was ontologically expanded using the EFO, resulting in 3,654,109 genetically supported gene–trait pairs. This dataset represents a redundant view of the evidence, with its only purpose being to maximize the overlap with the clinical information and minimize the issues related to the sparsity in the annotation.
Target annotations
To analyze the target factors that could influence studies stopped because of safety or side effects, we also included a set of target annotations that were independent of the study indication. Each gene was annotated with genetic constraint data from gnomAD, representing the functional impact of the presence of genetic variants, and split into six categories derived from gnomAD’s pLOEUF quantiles. We also analyzed the predicted loss-of-function intolerance, distinguishing genes as ‘LoF-intolerant’ when the pLI score is above 0.9 and as ‘LoF tolerant’ when the pLI score was below 0.1 (ref. 37 ). Moreover, each target was classified in a bin based on the number of unique interacting partners above an MI score threshold of 0.42 in the IntAct database 40 . This threshold corresponds to a physical interaction identified at least once in low-throughput studies or replicated in multiple high-throughput experiments. Additionally, target annotation for tissue specificity and distribution was retrieved from the baseline transcriptomic experiments in the Human Protein Atlas database 39 . The assessment was performed according to the categories defined by the Human Protein Atlas.
Statistics and reproducibility
No preliminary statistical analyses were conducted to determine sample sizes. The choice of clinical studies and genetic information followed an unbiased procedure. The significance of each case-control study was computed using a two-sided Fisher's exact test using all available samples. No multiple testing correction was applied to the resulting P values. All statistical tests were computed using SciPy (v.1.11.4) 50 . The code to replicate the analyses is publicly available (see Code availability).
Reporting summary
Further information on research design is available in the Nature Portfolio Reporting Summary linked to this article.
Data availability
The full training set is available for download at HuggingFace, including the curation from the previously published article 19 and the COVID-19 stopped studies 51 . The resulting model for download or interactive exploration can also be found in HuggingFace 52 . The dataset of the clinical trial stop reason predictions used in this study is available in Github 53 . The collection of clinical studies annotated with predicted stop reasons and genetic evidence can also be accessed on HuggingFace. Up-to-date predictions for newer clinical trial studies are updated quarterly in the Open Targets Platform.
Code availability
Code to reproduce the model and analysis are available on GitHub 53 .
DiMasi, J. A., Grabowski, H. G. & Hansen, R. W. Innovation in the pharmaceutical industry: new estimates of R&D costs. J. Health Econ. 47 , 20–33 (2016).
Article PubMed Google Scholar
Dowden, H. & Munro, J. Trends in clinical success rates and therapeutic focus. Nat. Rev. Drug Discov. 18 , 495–496 (2019).
Article CAS PubMed Google Scholar
Morgan, P. et al. Impact of a five-dimensional framework on R&D productivity at AstraZeneca. Nat. Rev. Drug Discov. 17 , 167–181 (2018).
Wu, S. S. et al. Reviving an R&D pipeline: a step change in the phase II success rate. Drug Discov. Today 26 , 308–314 (2021).
Nelson, M. R. et al. The support of human genetic evidence for approved drug indications. Nat. Genet. 47 , 856–860 (2015).
King, E. A., Davis, J. W. & Degner, J. F. Are drug targets with genetic support twice as likely to be approved? Revised estimates of the impact of genetic support for drug mechanisms on the probability of drug approval. PLoS Genet. 15 , e1008489 (2019).
Article PubMed PubMed Central Google Scholar
Minikel, E. V., Painter, J. L., Dong, C. C. & Nelson, M. R. Refining the impact of genetic evidence on clinical success. Nature 629 , 624–629 (2024).
Article CAS PubMed PubMed Central Google Scholar
Trajanoska, K. et al. From target discovery to clinical drug development with human genetics. Nature 620 , 737–745 (2023).
Ochoa, D. et al. Human genetics evidence supports two-thirds of the 2021 FDA-approved drugs. Nat. Rev. Drug Discov. 21 , 551 (2022).
Ioannidis, J. P. A. Why most published research findings are false. PLoS Med . 2 , e124 (2005).
Young, N. S., Ioannidis, J. P. A. & Al-Ubaydli, O. Why current publication practices may distort science. PLoS Med . 5 , e201 (2008).
Bourgeois, F. T., Murthy, S. & Mandl, K. D. Outcome reporting among drug trials registered in ClinicalTrials.gov. Ann. Intern. Med. 153 , 158–166 (2010).
Qunaj, L. et al. Delays in the publication of important clinical trial findings in oncology. JAMA Oncol. 4 , e180264 (2018).
Jones, C. W. et al. Delays in reporting and publishing trial results during pandemics: cross sectional analysis of 2009 H1N1, 2014 Ebola, and 2016 Zika clinical trials. BMC Med. Res. Methodol. 21 , 120 (2021).
Petsko, G. A. When failure should be the option. BMC Biol. 8 , 61 (2010).
Ross, J. S., Mulvey, G. K., Hines, E. M., Nissen, S. E. & Krumholz, H. M. Trial publication after registration in ClinicalTrials.gov: a cross-sectional analysis. PLoS Med . 6 , e1000144 (2009).
Califf, R. M. et al. Characteristics of clinical trials registered in ClinicalTrials.gov, 2007–2010. JAMA 307 , 1838–1847 (2012).
Al-Durra, M., Nolan, R. P., Seto, E., Cafazzo, J. A. & Eysenbach, G. Nonpublication rates and characteristics of registered randomized clinical trials in digital health: cross-sectional analysis. J. Med. Internet Res. 20 , e11924 (2018).
Pak, T. R., Rodriguez, M. & Roth, F. P. Why clinical trials are terminated. Preprint at https://doi.org/10.1101/021543 (2015).
Ochoa, D. et al. The next-generation Open Targets Platform: reimagined, redesigned, rebuilt. Nucleic Acids Res . 51 , D1353–D1359 (2023).
Devlin, J., Chang, M.-W., Lee, K. & Toutanova, K. BERT: pre-training of deep bidirectional transformers for language understanding. Preprint at https://doi.org/10.48550/arXiv.1810.04805 (2018).
Mullard, A. 2022 FDA approvals. Nat. Rev. Drug Discov. 22 , 83–88 (2023).
Harrison, R. K. Phase II and phase III failures: 2013–2015. Nat. Rev. Drug Discov. 15 , 817–818 (2016).
Ghoussaini, M. et al. Open targets genetics: systematic identification of trait-associated genes using large-scale genetics and functional genomics. Nucleic Acids Res. 49 , D1311–D1320 (2021).
Wang, Q. et al. Rare variant contribution to human disease in 281,104 UK Biobank exomes. Nature 597 , 527–532 (2021).
Backman, J. D. et al. Exome sequencing and analysis of 454,787 UK Biobank participants. Nature 599 , 628–634 (2021).
Karczewski, K. J. et al. Systematic single-variant and gene-based association testing of thousands of phenotypes in 394,841 UK Biobank exomes. Cell Genom. 2 , 100168 (2022).
Landrum, M. J. et al. ClinVar: public archive of relationships among sequence variation and human phenotype. Nucleic Acids Res. 42 , D980–D985 (2014).
McGlaughon, J. L., Goldstein, J. L., Thaxton, C., Hemphill, S. E. & Berg, J. S. The progression of the ClinGen gene clinical validity classification over time. Hum. Mutat. 39 , 1494–1504 (2018).
Martin, A. R., Williams, E. & Foulger, R. E. et al. PanelApp crowdsources expert knowledge to establish consensus diagnostic gene panels. Nat. Genet. 51 , 1560–1565 (2019).
Thormann, A. et al. Flexible and scalable diagnostic filtering of genomic variants using G2P with Ensembl VEP. Nat. Commun. 10 , 2373 (2019).
Rodwell, C. & Aymé, S. Rare disease policies to improve care for patients in Europe. Biochim. Biophys. Acta 1852 , 2329–2335 (2015).
UniProt Consortium. UniProt: a worldwide hub of protein knowledge. Nucleic Acids Res. 47 , D506–D515 (2019).
Article Google Scholar
Muñoz-Fuentes, V. et al. Correction to: the International Mouse Phenotyping Consortium (IMPC): a functional catalogue of the mammalian genome that informs conservation. Conserv. Genet. 20 , 135–136 (2019).
Bamford, S. et al. The COSMIC (Catalogue of Somatic Mutations in Cancer) database and website. Br. J. Cancer 91 , 355–358 (2004).
Gundem, G. et al. IntOGen: integration and data mining of multidimensional oncogenomic data. Nat. Methods 7 , 92–93 (2010).
Chen, S. et al. A genomic mutational constraint map using variation in 76,156 human genomes. Nature 625 , 92–100 (2024).
Duffy, Á. et al. Tissue-specific genetic features inform prediction of drug side effects in clinical trials. Sci. Adv. 6 , eabb6242 (2020).
Uhlén, M. et al. Proteomics. Tissue-based map of the human proteome. Science 347 , 1260419 (2015).
Del Toro, N. et al. The IntAct database: efficient access to fine-grained molecular interaction data. Nucleic Acids Res. 50 , D648–D653 (2022).
Barrett, J. C., Dunham, I. & Birney, E. Using human genetics to make new medicines. Nat. Rev. Genet. 16 , 561–562 (2015).
Fernando, K. et al. Achieving end-to-end success in the clinic: Pfizer’s learnings on R&D productivity. Drug Discov. Today 27 , 697–704 (2022).
Cohen, J. A coefficient of agreement for nominal scales. Educ. Psychol. Meas. 20 , 37–46 (1960).
Wolf, T. et al. HuggingFace’s transformers: state-of-the-art natural language processing. Preprint at https://doi.org/10.48550/arXiv.1910.03771 (2019).
Malone, J. et al. Modeling sample variables with an Experimental Factor Ontology. Bioinformatics 26 , 1112–1118 (2010).
Gaulton, A. et al. The ChEMBL database in 2017. Nucleic Acids Res. 45 , D945–D954 (2017).
Cunningham, F. et al. Ensembl 2022. Nucleic Acids Res. 50 , D988–D995 (2022).
Open Targets. clinical_evidence. Hugging Face https://doi.org/10.57967/HF/2611 (2024).
Mountjoy, E. et al. An open approach to systematically prioritize causal variants and genes at all published human GWAS trait-associated loci. Nat. Genet. 53 , 1527–1533 (2021).
SciPy (The SciPy Community, 2023).
Open Targets. Clinical_trial_reason_to_stop. Hugging Face https://doi.org/10.57967/HF/2600 (2024).
Open Targets. clinical_trial_stop_reasons. Hugging Face https://doi.org/10.57967/HF/2599 (2024).
López, I., Ochoa, D. & Olesya, R. OpenTargets/StopReasons: stable release. Zenodo https://doi.org/10.5281/ZENODO.11966097 (2024).
Download references
Acknowledgements
We would like to thank T. R. Pak, M. D. Rodriguez and F. P. Roth from Harvard Medical School, the Dana-Farber Cancer Institute and the Donelly Center (University of Toronto) for providing the dataset of curated stop reasons that was used for training our model. We also thank the Open Targets team for manually curating the stop reasons for the additional set of 1,675 clinical studies, including A. Hercules, A. Gonzalez, K. Tsirigos and H. Cornu. Finally, we would like to thank S. Machlitt-Northen from GlaxoSmithKline for providing detailed feedback on the redefined categories for stopped trials. I.D.'s research was funded in part by a Wellcome Trust grant (grant number 206194). For the purpose of Open Access, the authors have applied a CC-BY public copyright license to any author-accepted manuscript version arising from this submission.
Open access funding provided by European Molecular Biology Laboratory (EMBL).
Author information
Authors and affiliations.
Open Targets, Wellcome Genome Campus, Hinxton, Cambridgeshire, UK
Olesya Razuvayevskaya, Irene Lopez, Ian Dunham & David Ochoa
European Molecular Biology Laboratory, European Bioinformatics Institute (EMBL-EBI), Wellcome Genome Campus, Hinxton, Cambridgeshire, UK
Wellcome Sanger Institute, Wellcome Genome Campus, Hinxton, Cambridgeshire, UK
You can also search for this author in PubMed Google Scholar
Contributions
O.R, I.D. and D.O. designed the study. O.R. and I.L. trained the models. O.R., I.L. and D.O. conducted the analysis. O.R., I.L., I.D. and D.O. wrote the manuscript.
Corresponding author
Correspondence to David Ochoa .
Ethics declarations
Competing interests.
The authors declare no competing interests.
Peer review
Peer review information.
Nature Genetics thanks Emily King, Matthew Nelson and the other, anonymous, reviewer(s) for their contribution to the peer review of this work. Peer reviewer reports are available.
Additional information
Publisher’s note Springer Nature remains neutral with regard to jurisdictional claims in published maps and institutional affiliations.
Extended data
Extended data fig. 1 hierarchical clustering of stop reason similarity based on curation from pak et al. and 447 additional stopped trials due to covid-19..
Distances were estimated as the cosine similarity of the averaged embeddings (see Methods section).
Extended Data Fig. 2 Association between the reasons to stop along each phase of the clinical development as reported by ClinicalTrials.gov.
Underpowered reasons for stoppage were excluded. We used a two-tailed Fisher’s exact test to assess the significance of the associations, with a p-value threshold of 0.05 (n = 10,214 independent stopped trials). Significant associations are highlighted in blue. Error bars represent 95% confidence intervals for the odds ratio. All results are provided in Supplementary Table 6 .
Extended Data Fig. 3 Percentage of stopped trials predicted to be halted due to Safety or side effects or the COVID-19 pandemic as a fraction of all the trials by predominant therapeutic area.
Indications with multiple possible therapeutic areas were associated with the most severe area (for example Oncology). Overall incidence when considering all therapeutic areas was 5% for COVID-19 and 3.3% for Safety or side effects.
Extended Data Fig. 4 Representation of the data and analytical workflow defined to investigate the predictive value of genetics in all target/disease associations derived from clinical trials.
Except for the baseline expression data, all datasets were sourced from the Open Targets 22.04 release. Detailed methods can be found in the Methods section.
Extended Data Fig. 5 Association between the availability of genetic evidence and clinical trial outcomes by genetic data source.
X-axis displays the respective odds ratio and y-axis groups the studies by phase (red), stopped clinical trials (blue) and stopped clinical trials split by high-level stopping reason (green). Error bars represent 95% confidence intervals for the odds ratio.
Extended Data Fig. 6 Genetic support for stopped clinical trials in all indications, non-oncology and oncology.
Each row represents a stopping reason, with the effect size in the form of odds ratio (OR) and its 95% confidence interval represented by the dot and error bar. An odds ratio (OR) > 1 suggests that trials stopped for a given reason are more likely to have genetic support, while an OR < 1 indicates depletion. The number of trials (n) supporting each estimate is provided. Statistical significance was assessed using a two-tailed Fisher’s exact test, with a significance threshold of p < 0.05 without multiple testing correction.
Extended Data Fig. 7 Association between the availability of genetic evidence and clinical trial outcomes by somatic data source.
X-axis displays the respective odds ratio and y-axis groups the studies by phase (top row), stopped clinical trials (centre row) and stopped clinical trials split by high-level stopping reason (bottom row). Error bars represent 95% confidence intervals for the odds ratio.
Supplementary information
Reporting summary, peer review file, supplementary table.
Supplementary Tables 1–9.
Rights and permissions
Open Access This article is licensed under a Creative Commons Attribution 4.0 International License, which permits use, sharing, adaptation, distribution and reproduction in any medium or format, as long as you give appropriate credit to the original author(s) and the source, provide a link to the Creative Commons licence, and indicate if changes were made. The images or other third party material in this article are included in the article’s Creative Commons licence, unless indicated otherwise in a credit line to the material. If material is not included in the article’s Creative Commons licence and your intended use is not permitted by statutory regulation or exceeds the permitted use, you will need to obtain permission directly from the copyright holder. To view a copy of this licence, visit http://creativecommons.org/licenses/by/4.0/ .
Reprints and permissions
About this article
Cite this article.
Razuvayevskaya, O., Lopez, I., Dunham, I. et al. Genetic factors associated with reasons for clinical trial stoppage. Nat Genet (2024). https://doi.org/10.1038/s41588-024-01854-z
Download citation
Received : 15 February 2023
Accepted : 02 July 2024
Published : 29 July 2024
DOI : https://doi.org/10.1038/s41588-024-01854-z
Share this article
Anyone you share the following link with will be able to read this content:
Sorry, a shareable link is not currently available for this article.
Provided by the Springer Nature SharedIt content-sharing initiative
This article is cited by
Stopped clinical trials give evidence for the value of genetics.
Nature Genetics (2024)
Quick links
- Explore articles by subject
- Guide to authors
- Editorial policies
Sign up for the Nature Briefing: Translational Research newsletter — top stories in biotechnology, drug discovery and pharma.


IMAGES
VIDEO
COMMENTS
Participants in clinical studies help current and future generations. Through these studies, researchers develop new diagnostic tests, more effective treatments, and better ways of managing diseases with genetic components. Participants in studies are actively involved in understanding their disorder and current research.
Genomic research has evolved from seeking to understand the fundamentals of the human genetic code to examining the ways in which this code varies among people, and then applying this knowledge to ...
Genetics is the branch of science concerned with genes, heredity, and variation in living organisms. It seeks to understand the process of trait inheritance from parents to offspring, including ...
Genetics research articles from across Nature Portfolio. Genetics research is the scientific discipline concerned with the study of the role of genes in traits such as the development of disease ...
Genomics. Genomics is a broader term that refers to the study of an individual's entire genetic makeup — or genome — including the composition of each one of their genes as well as the external influences (including diet and environment) that influence the way those genes are expressed. The human genome is estimated to contain roughly 20,000 to 25,000 individual genes, according to the ...
C. Jacobsen, H. Jüppner, and D.M. MitchellN Engl J Med 2024;391:167-176. A 10-month-old boy was admitted to the hospital because of vomiting and weight loss. The calcium level was 13.2 g per ...
In celebration of the 20th anniversary of Nature Reviews Genetics, we asked 12 leading researchers to reflect on the key challenges and opportunities faced by the field of genetics and genomics.
About the National Human Genome Research Institute. At NHGRI, we are focused on advances in genomics research. Building on our leadership role in the initial sequencing of the human genome, we collaborate with the world's scientific and medical communities to enhance genomic technologies that accelerate breakthroughs and improve lives.
Based on the material mentioned so far, we will now cover some topics on how progress in population genetics, genome evolution, and phylogenic studies can be applied to medical research. Multifactorial disorders are assumed to occur through interactions between multiple genetic and environmental factors.
Genetics Research. Genetics studies the genes and DNA coding that each person inherits from their parents and how these genes can lead to disease or better health. Some inherited genes cause diseases such as Tay-Sachs or sickle cell anemia, while mutations in genes can cause genetic disorders, such as Down syndrome. However, doctors also use ...
Human genetic research involves the study of inherited human traits. Much of this research is aimed at identifying DNA mutations that can help cause specific health problems, developing methods of identifying those mutations in patients, and improving the interventions available to help patients address those problems.
To do this accurately, it's critical that the genetic evidence we gather includes globally representative populations and that we have members of communities historically underrepresented in biomedical research leading and contributing to these kinds of studies," said Alexander Hatoum, Ph.D., a research assistant professor at Washington ...
Genetic research is the study of human DNA to find out what genes and environmental factors contribute to diseases. If we find out what causes disease, we can better detect disease, better treat disease and hopefully even prevent disease from happening in the first place! Nearly every disease we know of has a genetic component.
The genetic findings may thus be considered to support efforts to reconceptualize psychiatric nosology in a more dimensional framework206, 207, such as the proposed Hierarchical Taxonomy of Psychopathology (HiTOP) 173 or Research Domain Criteria (RDoC) 174. Genetic risk for psychiatric disorders also overlaps with genetic variation in ...
Genome-wide Association Studies. A genome-wide association study (GWAS) is an approach that involves scanning the genomes from many different people and looking for genetic markers that can be used to predict the presence of a disease. The goal is to understand how genes contribute to the disease and to use that understanding to help develop ...
Family studies. Genetic factors for BD were first investigated using twin, family, and adoption studies. These studies established that family history of BD is an important clinical predictor of The onset of mood disorders in a patient and that the risk of mood disorder decreases as the genetic distance from the proband increases (Craddock & Sklar, 2013; Merikangas & Yu, 2002).
Genetic engineering articles from across Nature Portfolio. Genetic engineering is the act of modifying the genetic makeup of an organism. Modifications can be generated by methods such as gene ...
A genetics study of nearly half a million people closes the door on a long-standing debate in sexuality. ... the study's co-author and genetics research fellow at the Broad Institute and Mass ...
To that end, researchers at Duke Health and St. Jude's Research Hospital launched a study of ALS recovery patients and found certain genetic factors that appear to protect against the disease's ...
However, genetic and genomic studies are predominantly based on populations of European ancestry. As a result, the potential benefits of genomic research—including better understanding of ...
ADHD often appears to run in families, and research studies have suggested that there may be a genetic component to this disorder. Individuals diagnosed with ADHD may have close blood relatives with the disorder. Scientists believe that ADHD is a complex disorder that probably involves at least two genes. Non-genetic causes such as abnormal ...
This study followed the Strengthening the Reporting of Observational Studies in Epidemiology reporting guideline. For inclusion in current analyses, the participants needed valid diet records and epigenetic data at midlife along with age and race and ethnicity information (participant self-reported); after excluding 5 women with epigenetic data ...
The promise of genetic studies for improvements in human health has been widely espoused (for example, Hood, 1992; Fears and Poste, 1999; National Bioethics Advisory Commission, 1999), and genetic studies have achieved added significance as the end point of the Human Genome Project approaches (Pennisi, 1999). There is the strong sense that everyone at some point in the not too distant future ...
Wei's previous research uses a vast dataset from the UK Biobank consisting of genetic and trait information of nearly 300,000 Brits of non-African ancestry. She and other researchers have found that 4,303 genetic variants originated from Neanderthals that are likely to have a phenotypic impact in modern humans.
A similar study published last year had identified genetic traces of an encounter between the two groups around 250,000 years ago but the contribution of Homo sapiens DNA to Neanderthals around ...
A third law has emerged from molecular genetic research that attempts to identify specific genes responsible for widespread heritability, especially genome-wide association (GWA) studies of the ...
USING TWIN STUDIES TO DISTINGUISH SELECTION FROM ENVIRONMENTAL CAUSATION. This is where twin studies have particular value. Whatever the label, the First Law of Behavioral Genetics implies that it is always possible that common genetic influences creating selection bias underlie any apparently causal, naturally occurring association between environmental circumstance and outcome.
A recent OHSU clinical trial headed by Shinto suggests that older adults with a genetic predisposition to Alzheimer's disease may benefit from fish oil supplements. ... The study was supported by the National Institute on Aging of the National Institutes of Health grant awards R01AG043398, P30AG008017 and P30AG066518; the National Center for ...
Environmental factors, such as stress or trauma, can cause genetic changes that are passed down through generations and may contribute to the development of a mental disorder or a substance use disorder. Mental disorders can contribute to substance use and SUDs. ... Clinical trials are research studies that look at new ways to prevent, detect ...
a,b, Genetic evidence support for clinical trials either from human genetics studies (a) or the International Mouse Phenotyping Consortium mouse knockouts (KO) that phenocopy the human disease (b ...