Guide to Writing the Results and Discussion Sections of a Scientific Article
A quality research paper has both the qualities of in-depth research and good writing ( Bordage, 2001 ). In addition, a research paper must be clear, concise, and effective when presenting the information in an organized structure with a logical manner ( Sandercock, 2013 ).
In this article, we will take a closer look at the results and discussion section. Composing each of these carefully with sufficient data and well-constructed arguments can help improve your paper overall.
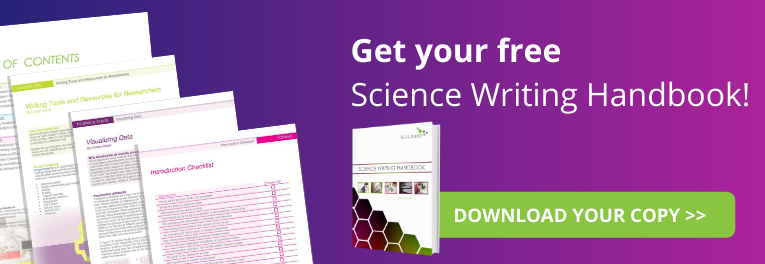
The results section of your research paper contains a description about the main findings of your research, whereas the discussion section interprets the results for readers and provides the significance of the findings. The discussion should not repeat the results.
Let’s dive in a little deeper about how to properly, and clearly organize each part.
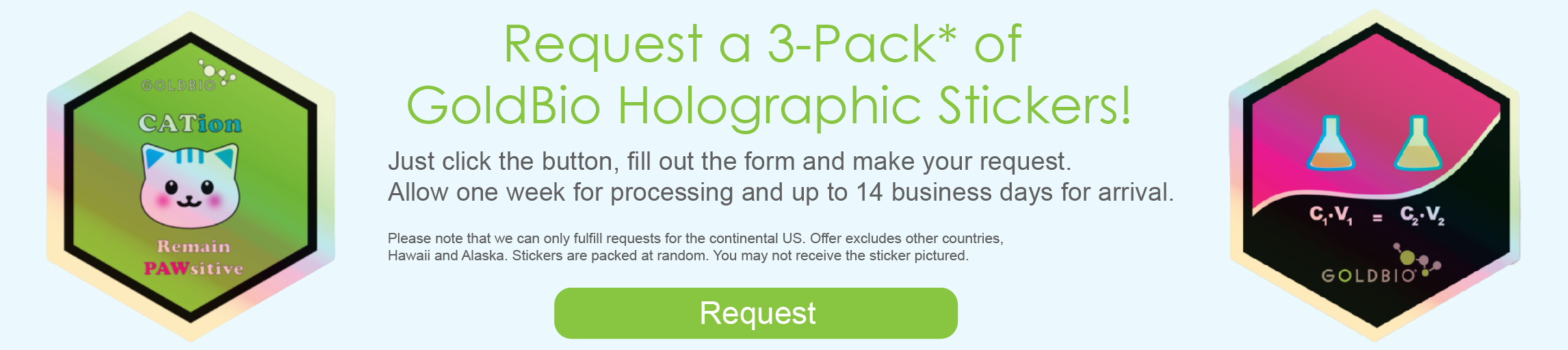

How to Organize the Results Section
Since your results follow your methods, you’ll want to provide information about what you discovered from the methods you used, such as your research data. In other words, what were the outcomes of the methods you used?
You may also include information about the measurement of your data, variables, treatments, and statistical analyses.
To start, organize your research data based on how important those are in relation to your research questions. This section should focus on showing major results that support or reject your research hypothesis. Include your least important data as supplemental materials when submitting to the journal.
The next step is to prioritize your research data based on importance – focusing heavily on the information that directly relates to your research questions using the subheadings.
The organization of the subheadings for the results section usually mirrors the methods section. It should follow a logical and chronological order.
Subheading organization
Subheadings within your results section are primarily going to detail major findings within each important experiment. And the first paragraph of your results section should be dedicated to your main findings (findings that answer your overall research question and lead to your conclusion) (Hofmann, 2013).
In the book “Writing in the Biological Sciences,” author Angelika Hofmann recommends you structure your results subsection paragraphs as follows:
- Experimental purpose
- Interpretation
Each subheading may contain a combination of ( Bahadoran, 2019 ; Hofmann, 2013, pg. 62-63):
- Text: to explain about the research data
- Figures: to display the research data and to show trends or relationships, for examples using graphs or gel pictures.
- Tables: to represent a large data and exact value
Decide on the best way to present your data — in the form of text, figures or tables (Hofmann, 2013).
Data or Results?
Sometimes we get confused about how to differentiate between data and results . Data are information (facts or numbers) that you collected from your research ( Bahadoran, 2019 ).
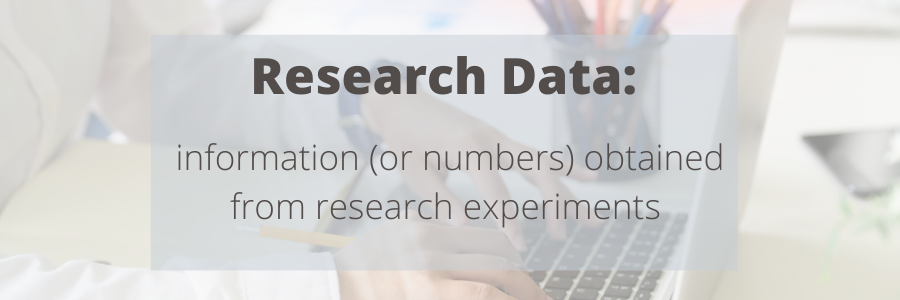
Whereas, results are the texts presenting the meaning of your research data ( Bahadoran, 2019 ).
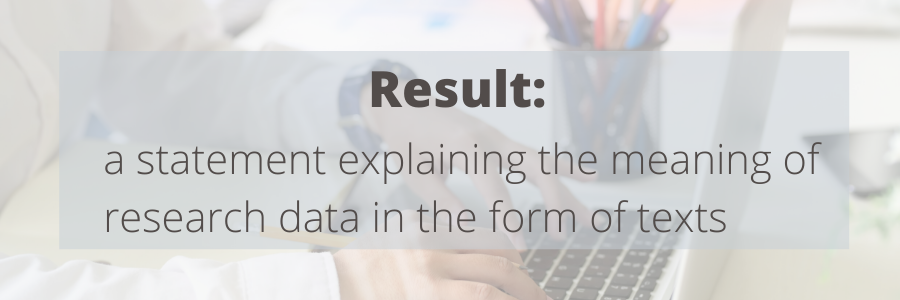
One mistake that some authors often make is to use text to direct the reader to find a specific table or figure without further explanation. This can confuse readers when they interpret data completely different from what the authors had in mind. So, you should briefly explain your data to make your information clear for the readers.
Common Elements in Figures and Tables
Figures and tables present information about your research data visually. The use of these visual elements is necessary so readers can summarize, compare, and interpret large data at a glance. You can use graphs or figures to compare groups or patterns. Whereas, tables are ideal to present large quantities of data and exact values.
Several components are needed to create your figures and tables. These elements are important to sort your data based on groups (or treatments). It will be easier for the readers to see the similarities and differences among the groups.
When presenting your research data in the form of figures and tables, organize your data based on the steps of the research leading you into a conclusion.
Common elements of the figures (Bahadoran, 2019):
- Figure number
- Figure title
- Figure legend (for example a brief title, experimental/statistical information, or definition of symbols).
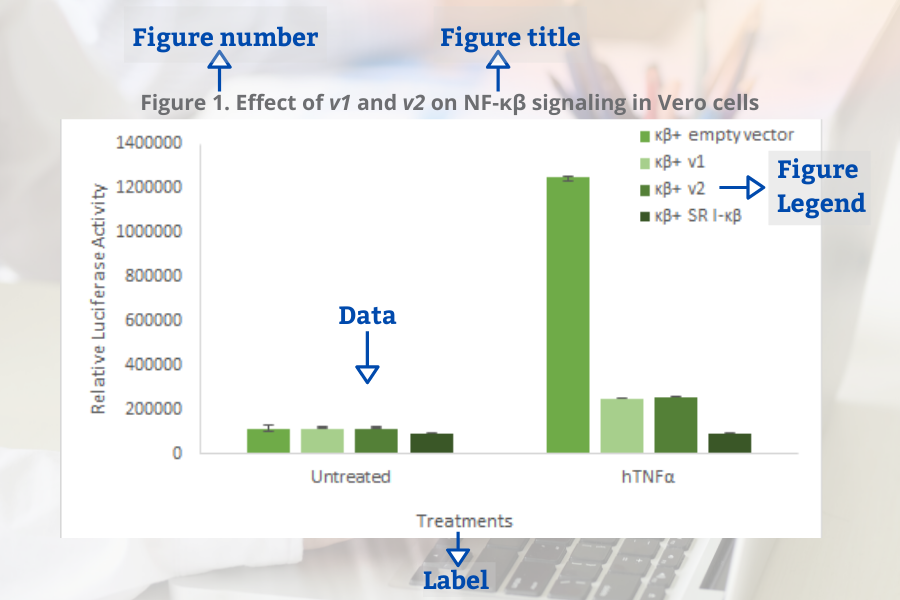
Tables in the result section may contain several elements (Bahadoran, 2019):
- Table number
- Table title
- Row headings (for example groups)
- Column headings
- Row subheadings (for example categories or groups)
- Column subheadings (for example categories or variables)
- Footnotes (for example statistical analyses)
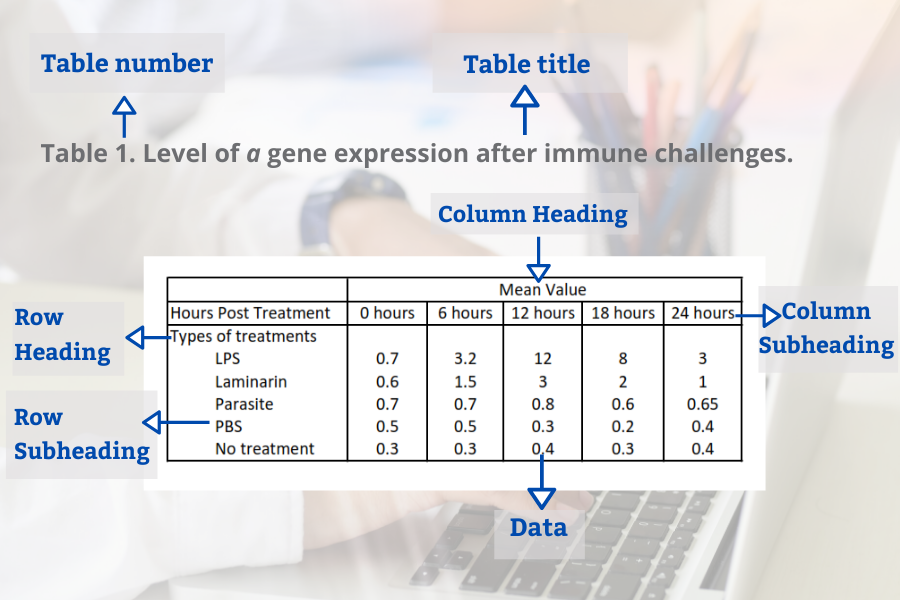
Tips to Write the Results Section
- Direct the reader to the research data and explain the meaning of the data.
- Avoid using a repetitive sentence structure to explain a new set of data.
- Write and highlight important findings in your results.
- Use the same order as the subheadings of the methods section.
- Match the results with the research questions from the introduction. Your results should answer your research questions.
- Be sure to mention the figures and tables in the body of your text.
- Make sure there is no mismatch between the table number or the figure number in text and in figure/tables.
- Only present data that support the significance of your study. You can provide additional data in tables and figures as supplementary material.
How to Organize the Discussion Section
It’s not enough to use figures and tables in your results section to convince your readers about the importance of your findings. You need to support your results section by providing more explanation in the discussion section about what you found.
In the discussion section, based on your findings, you defend the answers to your research questions and create arguments to support your conclusions.
Below is a list of questions to guide you when organizing the structure of your discussion section ( Viera et al ., 2018 ):
- What experiments did you conduct and what were the results?
- What do the results mean?
- What were the important results from your study?
- How did the results answer your research questions?
- Did your results support your hypothesis or reject your hypothesis?
- What are the variables or factors that might affect your results?
- What were the strengths and limitations of your study?
- What other published works support your findings?
- What other published works contradict your findings?
- What possible factors might cause your findings different from other findings?
- What is the significance of your research?
- What are new research questions to explore based on your findings?
Organizing the Discussion Section
The structure of the discussion section may be different from one paper to another, but it commonly has a beginning, middle-, and end- to the section.
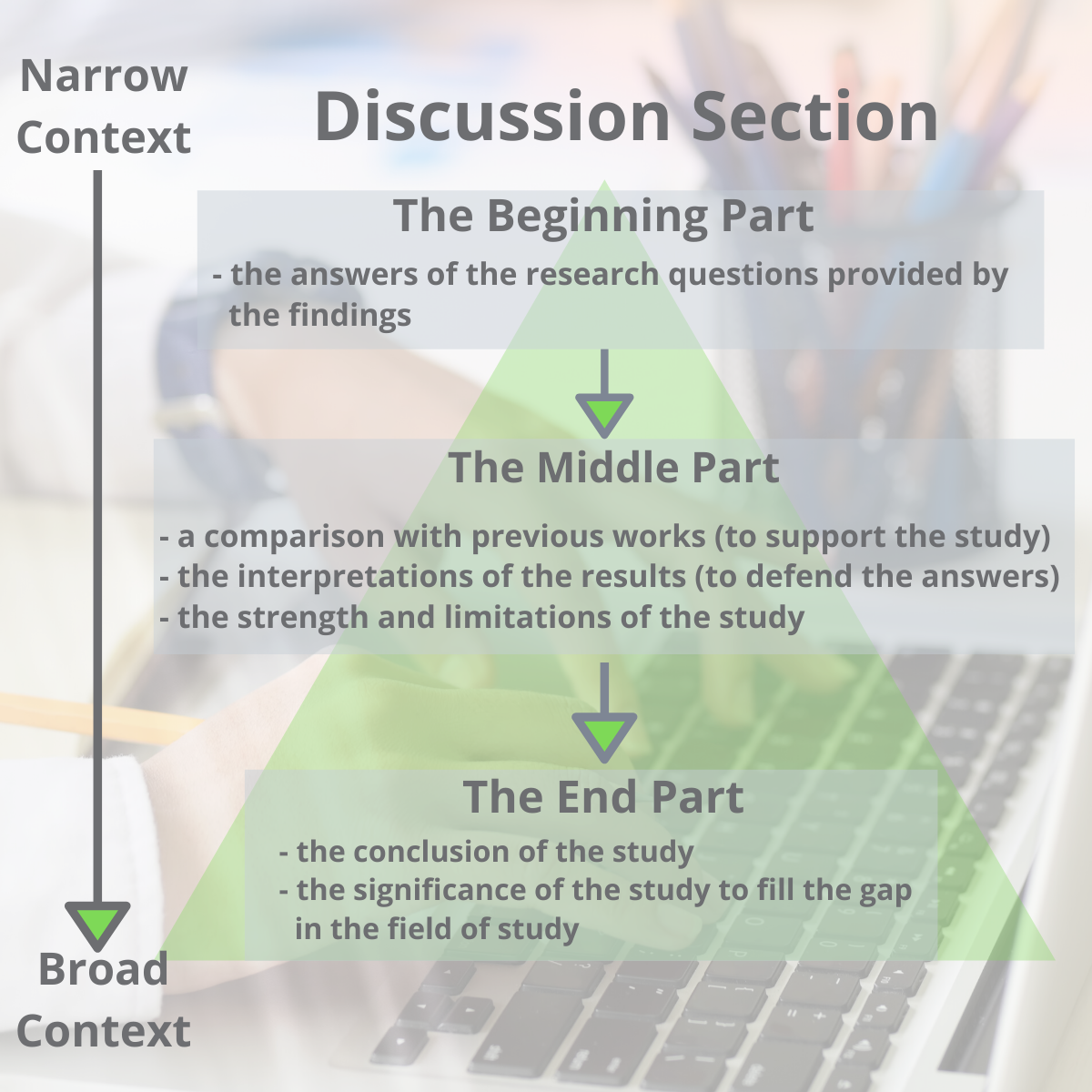
One way to organize the structure of the discussion section is by dividing it into three parts (Ghasemi, 2019):
- The beginning: The first sentence of the first paragraph should state the importance and the new findings of your research. The first paragraph may also include answers to your research questions mentioned in your introduction section.
- The middle: The middle should contain the interpretations of the results to defend your answers, the strength of the study, the limitations of the study, and an update literature review that validates your findings.
- The end: The end concludes the study and the significance of your research.
Another possible way to organize the discussion section was proposed by Michael Docherty in British Medical Journal: is by using this structure ( Docherty, 1999 ):
- Discussion of important findings
- Comparison of your results with other published works
- Include the strengths and limitations of the study
- Conclusion and possible implications of your study, including the significance of your study – address why and how is it meaningful
- Future research questions based on your findings
Finally, a last option is structuring your discussion this way (Hofmann, 2013, pg. 104):
- First Paragraph: Provide an interpretation based on your key findings. Then support your interpretation with evidence.
- Secondary results
- Limitations
- Unexpected findings
- Comparisons to previous publications
- Last Paragraph: The last paragraph should provide a summarization (conclusion) along with detailing the significance, implications and potential next steps.
Remember, at the heart of the discussion section is presenting an interpretation of your major findings.
Tips to Write the Discussion Section
- Highlight the significance of your findings
- Mention how the study will fill a gap in knowledge.
- Indicate the implication of your research.
- Avoid generalizing, misinterpreting your results, drawing a conclusion with no supportive findings from your results.
Aggarwal, R., & Sahni, P. (2018). The Results Section. In Reporting and Publishing Research in the Biomedical Sciences (pp. 21-38): Springer.
Bahadoran, Z., Mirmiran, P., Zadeh-Vakili, A., Hosseinpanah, F., & Ghasemi, A. (2019). The principles of biomedical scientific writing: Results. International journal of endocrinology and metabolism, 17(2).
Bordage, G. (2001). Reasons reviewers reject and accept manuscripts: the strengths and weaknesses in medical education reports. Academic medicine, 76(9), 889-896.
Cals, J. W., & Kotz, D. (2013). Effective writing and publishing scientific papers, part VI: discussion. Journal of clinical epidemiology, 66(10), 1064.
Docherty, M., & Smith, R. (1999). The case for structuring the discussion of scientific papers: Much the same as that for structuring abstracts. In: British Medical Journal Publishing Group.
Faber, J. (2017). Writing scientific manuscripts: most common mistakes. Dental press journal of orthodontics, 22(5), 113-117.
Fletcher, R. H., & Fletcher, S. W. (2018). The discussion section. In Reporting and Publishing Research in the Biomedical Sciences (pp. 39-48): Springer.
Ghasemi, A., Bahadoran, Z., Mirmiran, P., Hosseinpanah, F., Shiva, N., & Zadeh-Vakili, A. (2019). The Principles of Biomedical Scientific Writing: Discussion. International journal of endocrinology and metabolism, 17(3).
Hofmann, A. H. (2013). Writing in the biological sciences: a comprehensive resource for scientific communication . New York: Oxford University Press.
Kotz, D., & Cals, J. W. (2013). Effective writing and publishing scientific papers, part V: results. Journal of clinical epidemiology, 66(9), 945.
Mack, C. (2014). How to Write a Good Scientific Paper: Structure and Organization. Journal of Micro/ Nanolithography, MEMS, and MOEMS, 13. doi:10.1117/1.JMM.13.4.040101
Moore, A. (2016). What's in a Discussion section? Exploiting 2‐dimensionality in the online world…. Bioessays, 38(12), 1185-1185.
Peat, J., Elliott, E., Baur, L., & Keena, V. (2013). Scientific writing: easy when you know how: John Wiley & Sons.
Sandercock, P. M. L. (2012). How to write and publish a scientific article. Canadian Society of Forensic Science Journal, 45(1), 1-5.
Teo, E. K. (2016). Effective Medical Writing: The Write Way to Get Published. Singapore Medical Journal, 57(9), 523-523. doi:10.11622/smedj.2016156
Van Way III, C. W. (2007). Writing a scientific paper. Nutrition in Clinical Practice, 22(6), 636-640.
Vieira, R. F., Lima, R. C. d., & Mizubuti, E. S. G. (2019). How to write the discussion section of a scientific article. Acta Scientiarum. Agronomy, 41.
Related Articles
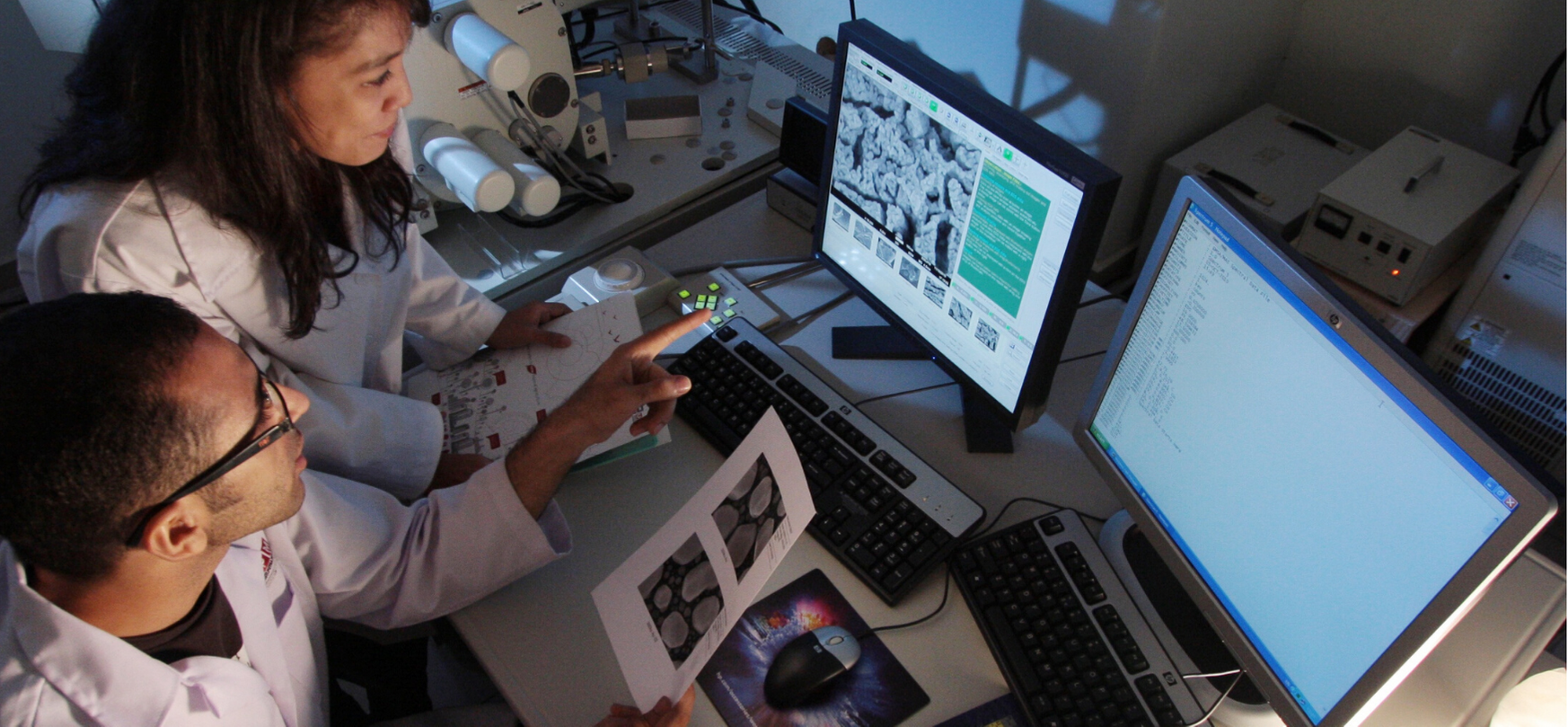
A quality research paper has both the qualities of in-depth research and good writing (Bordage, 200...
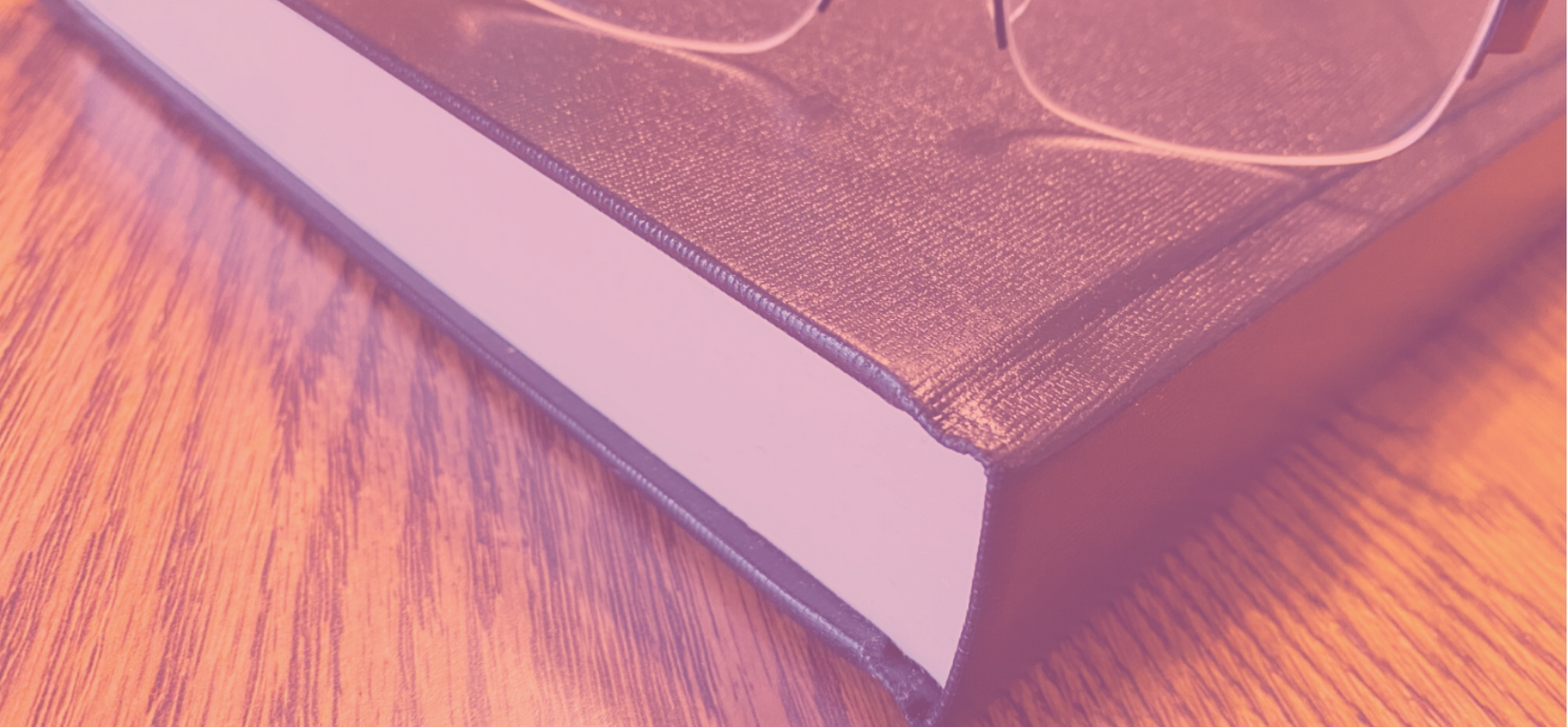
How to Survive and Complete a Thesis or a Dissertation
Writing a thesis or a dissertation can be a challenging process for many graduate students. There ar...
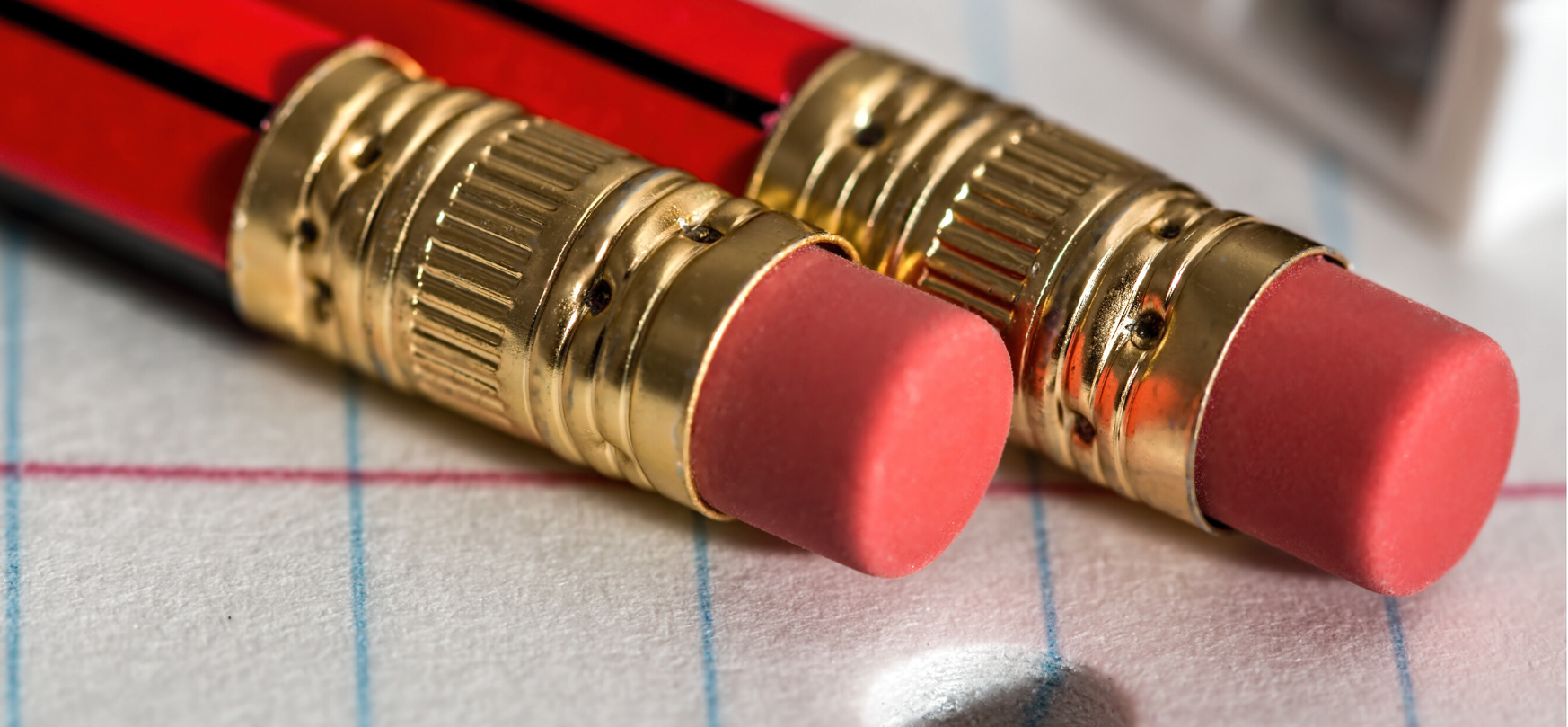
12 Ways to Dramatically Improve your Research Manuscript Title and Abstract
The first thing a person doing literary research will see is a research publication title. After tha...
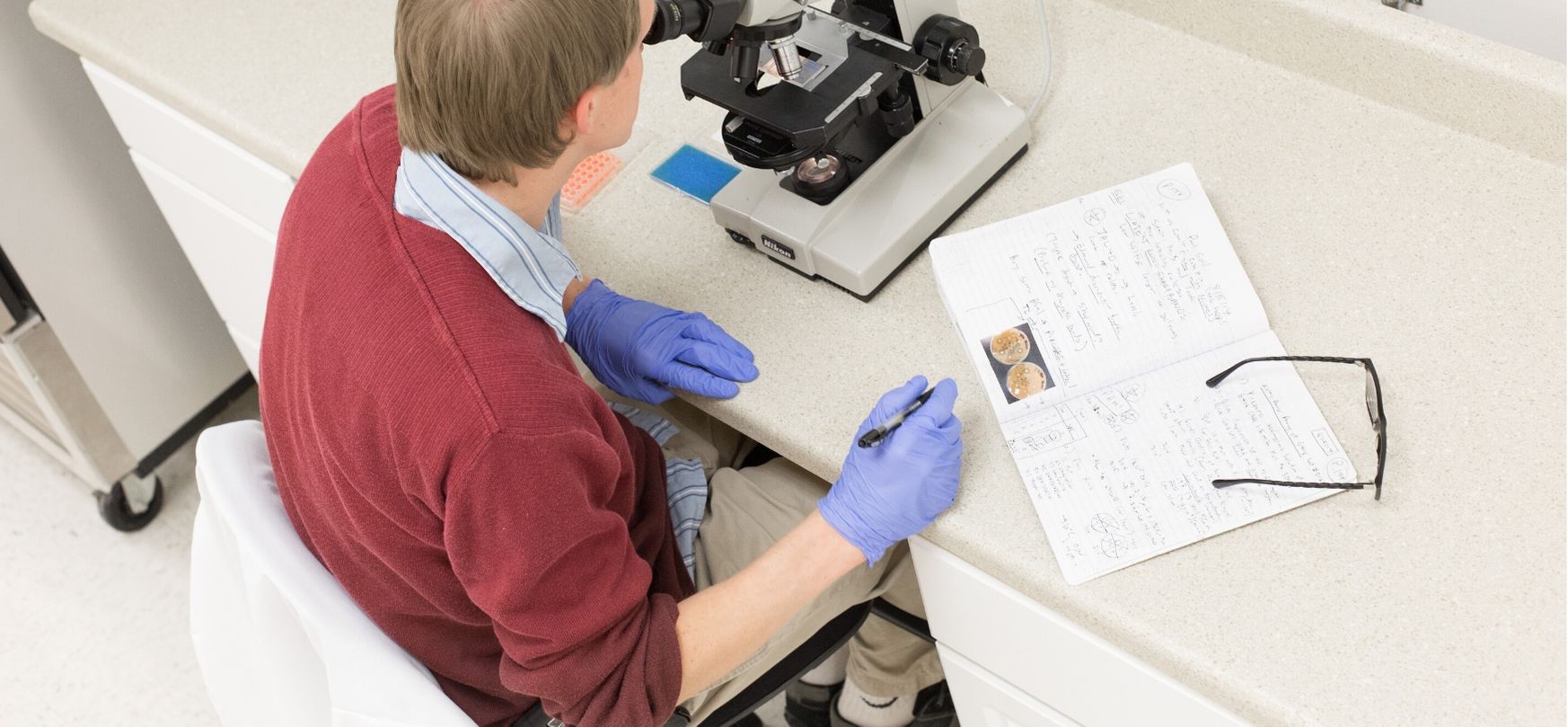
15 Laboratory Notebook Tips to Help with your Research Manuscript
Your lab notebook is a foundation to your research manuscript. It serves almost as a rudimentary dra...
Join our list to receive promos and articles.
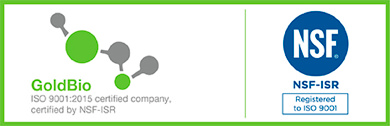
- Competent Cells
- Lab Startup
- Z')" data-type="collection" title="Products A->Z" target="_self" href="/collection/products-a-to-z">Products A->Z
- GoldBio Resources
- GoldBio Sales Team
- GoldBio Distributors
- Duchefa Direct
- Sign up for Promos
- Terms & Conditions
- ISO Certification
- Agarose Resins
- Antibiotics & Selection
- Biochemical Reagents
- Bioluminescence
- Buffers & Reagents
- Cell Culture
- Cloning & Induction
- Competent Cells and Transformation
- Detergents & Membrane Agents
- DNA Amplification
- Enzymes, Inhibitors & Substrates
- Growth Factors and Cytokines
- Lab Tools & Accessories
- Plant Research and Reagents
- Protein Research & Analysis
- Protein Expression & Purification
- Reducing Agents

Want to create or adapt books like this? Learn more about how Pressbooks supports open publishing practices.
Quantitative Data Analysis
9 Presenting the Results of Quantitative Analysis
Mikaila Mariel Lemonik Arthur
This chapter provides an overview of how to present the results of quantitative analysis, in particular how to create effective tables for displaying quantitative results and how to write quantitative research papers that effectively communicate the methods used and findings of quantitative analysis.
Writing the Quantitative Paper
Standard quantitative social science papers follow a specific format. They begin with a title page that includes a descriptive title, the author(s)’ name(s), and a 100 to 200 word abstract that summarizes the paper. Next is an introduction that makes clear the paper’s research question, details why this question is important, and previews what the paper will do. After that comes a literature review, which ends with a summary of the research question(s) and/or hypotheses. A methods section, which explains the source of data, sample, and variables and quantitative techniques used, follows. Many analysts will include a short discussion of their descriptive statistics in the methods section. A findings section details the findings of the analysis, supported by a variety of tables, and in some cases graphs, all of which are explained in the text. Some quantitative papers, especially those using more complex techniques, will include equations. Many papers follow the findings section with a discussion section, which provides an interpretation of the results in light of both the prior literature and theory presented in the literature review and the research questions/hypotheses. A conclusion ends the body of the paper. This conclusion should summarize the findings, answering the research questions and stating whether any hypotheses were supported, partially supported, or not supported. Limitations of the research are detailed. Papers typically include suggestions for future research, and where relevant, some papers include policy implications. After the body of the paper comes the works cited; some papers also have an Appendix that includes additional tables and figures that did not fit into the body of the paper or additional methodological details. While this basic format is similar for papers regardless of the type of data they utilize, there are specific concerns relating to quantitative research in terms of the methods and findings that will be discussed here.
In the methods section, researchers clearly describe the methods they used to obtain and analyze the data for their research. When relying on data collected specifically for a given paper, researchers will need to discuss the sample and data collection; in most cases, though, quantitative research relies on pre-existing datasets. In these cases, researchers need to provide information about the dataset, including the source of the data, the time it was collected, the population, and the sample size. Regardless of the source of the data, researchers need to be clear about which variables they are using in their research and any transformations or manipulations of those variables. They also need to explain the specific quantitative techniques that they are using in their analysis; if different techniques are used to test different hypotheses, this should be made clear. In some cases, publications will require that papers be submitted along with any code that was used to produce the analysis (in SPSS terms, the syntax files), which more advanced researchers will usually have on hand. In many cases, basic descriptive statistics are presented in tabular form and explained within the methods section.
The findings sections of quantitative papers are organized around explaining the results as shown in tables and figures. Not all results are depicted in tables and figures—some minor or null findings will simply be referenced—but tables and figures should be produced for all findings to be discussed at any length. If there are too many tables and figures, some can be moved to an appendix after the body of the text and referred to in the text (e.g. “See Table 12 in Appendix A”).
Discussions of the findings should not simply restate the contents of the table. Rather, they should explain and interpret it for readers, and they should do so in light of the hypothesis or hypotheses that are being tested. Conclusions—discussions of whether the hypothesis or hypotheses are supported or not supported—should wait for the conclusion of the paper.
Creating Effective Tables
When creating tables to display the results of quantitative analysis, the most important goals are to create tables that are clear and concise but that also meet standard conventions in the field. This means, first of all, paring down the volume of information produced in the statistical output to just include the information most necessary for interpreting the results, but doing so in keeping with standard table conventions. It also means making tables that are well-formatted and designed, so that readers can understand what the tables are saying without struggling to find information. For example, tables (as well as figures such as graphs) need clear captions; they are typically numbered and referred to by number in the text. Columns and rows should have clear headings. Depending on the content of the table, formatting tools may need to be used to set off header rows/columns and/or total rows/columns; cell-merging tools may be necessary; and shading may be important in tables with many rows or columns.
Here, you will find some instructions for creating tables of results from descriptive, crosstabulation, correlation, and regression analysis that are clear, concise, and meet normal standards for data display in social science. In addition, after the instructions for creating tables, you will find an example of how a paper incorporating each table might describe that table in the text.
Descriptive Statistics
When presenting the results of descriptive statistics, we create one table with columns for each type of descriptive statistic and rows for each variable. Note, of course, that depending on level of measurement only certain descriptive statistics are appropriate for a given variable, so there may be many cells in the table marked with an — to show that this statistic is not calculated for this variable. So, consider the set of descriptive statistics below, for occupational prestige, age, highest degree earned, and whether the respondent was born in this country.
To display these descriptive statistics in a paper, one might create a table like Table 2. Note that for discrete variables, we use the value label in the table, not the value.
If we were then to discuss our descriptive statistics in a quantitative paper, we might write something like this (note that we do not need to repeat every single detail from the table, as readers can peruse the table themselves): This analysis relies on four variables from the 2021 General Social Survey: occupational prestige score, age, highest degree earned, and whether the respondent was born in the United States. Descriptive statistics for all four variables are shown in Table 2. The median occupational prestige score is 47, with a range from 16 to 80. 50% of respondents had occupational prestige scores scores between 35 and 59. The median age of respondents is 53, with a range from 18 to 89. 50% of respondents are between ages 37 and 66. Both variables have little skew. Highest degree earned ranges from less than high school to a graduate degree; the median respondent has earned an associate’s degree, while the modal response (given by 39.8% of the respondents) is a high school degree. 88.8% of respondents were born in the United States. CrosstabulationWhen presenting the results of a crosstabulation, we simplify the table so that it highlights the most important information—the column percentages—and include the significance and association below the table. Consider the SPSS output below.
Table 4 shows how a table suitable for include in a paper might look if created from the SPSS output in Table 3. Note that we use asterisks to indicate the significance level of the results: * means p < 0.05; ** means p < 0.01; *** means p < 0.001; and no stars mean p > 0.05 (and thus that the result is not significant). Also note than N is the abbreviation for the number of respondents.
If we were going to discuss the results of this crosstabulation in a quantitative research paper, the discussion might look like this: A crosstabulation of respondent’s class identification and their highest degree earned, with class identification as the independent variable, is significant, with a Spearman correlation of 0.419, as shown in Table 4. Among lower class and working class respondents, more than 50% had earned a high school degree. Less than 20% of poor respondents and less than 40% of working-class respondents had earned more than a high school degree. In contrast, the majority of middle class and upper class respondents had earned at least a bachelor’s degree. In fact, 50% of upper class respondents had earned a graduate degree. CorrelationWhen presenting a correlating matrix, one of the most important things to note is that we only present half the table so as not to include duplicated results. Think of the line through the table where empty cells exist to represent the correlation between a variable and itself, and include only the triangle of data either above or below that line of cells. Consider the output in Table 5.
Table 6 shows what the contents of Table 5 might look like when a table is constructed in a fashion suitable for publication.
If we were to discuss the results of this bivariate correlation analysis in a quantitative paper, the discussion might look like this: Bivariate correlations were run among variables measuring age, occupational prestige, the highest year of school respondents completed, and family income in constant 1986 dollars, as shown in Table 6. Correlations between age and highest year of school completed and between age and family income are not significant. All other correlations are positive and significant at the p<0.001 level. The correlation between age and occupational prestige is weak; the correlations between income and occupational prestige and between income and educational attainment are moderate, and the correlation between education and occupational prestige is strong. To present the results of a regression, we create one table that includes all of the key information from the multiple tables of SPSS output. This includes the R 2 and significance of the regression, either the B or the beta values (different analysts have different preferences here) for each variable, and the standard error and significance of each variable. Consider the SPSS output in Table 7.
The regression output in shown in Table 7 contains a lot of information. We do not include all of this information when making tables suitable for publication. As can be seen in Table 8, we include the Beta (or the B), the standard error, and the significance asterisk for each variable; the R 2 and significance for the overall regression; the degrees of freedom (which tells readers the sample size or N); and the constant; along with the key to p/significance values.
If we were to discuss the results of this regression in a quantitative paper, the results might look like this: Table 8 shows the results of a regression in which age, occupational prestige, and highest year of school completed are the independent variables and family income is the dependent variable. The regression results are significant, and all of the independent variables taken together explain 15.6% of the variance in family income. Age is not a significant predictor of income, while occupational prestige and educational attainment are. Educational attainment has a larger effect on family income than does occupational prestige. For every year of additional education attained, family income goes up on average by $3,988.545; for every one-unit increase in occupational prestige score, family income goes up on average by $522.887. [1]
Social Data Analysis Copyright © 2021 by Mikaila Mariel Lemonik Arthur is licensed under a Creative Commons Attribution-NonCommercial-ShareAlike 4.0 International License , except where otherwise noted. Training videos | Faqs ![]() Academic Phrases for Writing Results & Discussion Sections of a Research PaperOverview | Abstract | Introduction | Literature Review | Materials & Methods | Results & Discussion | Conclusion & Future Work | Acknowledgements & Appendix The results and discussion sections are one of the challenging sections to write. It is important to plan this section carefully as it may contain a large amount of scientific data that needs to be presented in a clear and concise fashion. The purpose of a Results section is to present the key results of your research. Results and discussions can either be combined into one section or organized as separate sections depending on the requirements of the journal to which you are submitting your research paper. Use subsections and subheadings to improve readability and clarity. Number all tables and figures with descriptive titles. Present your results as figures and tables and point the reader to relevant items while discussing the results. This section should highlight significant or interesting findings along with P values for statistical tests. Be sure to include negative results and highlight potential limitations of the paper. You will be criticised by the reviewers if you don’t discuss the shortcomings of your research. This often makes up for a great discussion section, so do not be afraid to highlight them. The results and discussion section of your research paper should include the following:
1. FindingsFrom the short review above, key findings emerge: __ We describe the results of __, which show __ This suggests that __ We showed that __ Our findings on __ at least hint that __ This is an important finding in the understanding of the __ The present study confirmed the findings about __ Another promising finding was that __ Our results demonstrated that __ This result highlights that little is known about the __ A further novel finding is that __ Together, the present findings confirm __ The implications of these findings are discussed in __ The results demonstrate two things. First, __. Second, __ The results of the experiment found clear support for the __ This analysis found evidence for __ Planned comparisons revealed that __ Our results casts a new light on __ This section summarises the findings and contributions made. It performs well, giving good results. This gives clearly better results than __ The results confirm that this a good choice for __ From the results, it is clear that __ In this section, we will illustrate some experimental results. This delivers significantly better results due to __ The result now provides evidence to __ It leads to good results, even if the improvement is negligible. This yields increasingly good results on data. The result of this analysis is then compared with the __ The applicability of these new results are then tested on __ This is important to correctly interpret the results. The results are substantially better than __ The results lead to similar conclusion where __ Superior results are seen for __ From these results it is clear that __ Extensive results carried out show that this method improves __ We obtain good results with this simple method. However, even better results are achieved when using our algorithm. It is worth discussing these interesting facts revealed by the results of __ Overall, our method was the one that obtained the most robust results. Slightly superior results are achieved with our algorithm. The result is equal to or better than a result that is currently accepted. 2. Comparison with prior studiesThe results demonstrated in this chapter match state of the art methods. Here we compare the results of the proposed method with those of the traditional methods. These results go beyond previous reports, showing that __ In line with previous studies __ This result ties well with previous studies wherein __ Contrary to the findings of __ we did not find __ They have demonstrated that __ Others have shown that __ improves __ By comparing the results from __, we hope to determine __ However, in line with the ideas of __, it can be concluded that __ When comparing our results to those of older studies, it must be pointed out that __ We have verified that using __ produces similar results Overall these findings are in accordance with findings reported by __ Even though we did not replicate the previously reported __, our results suggest that __ A similar conclusion was reached by __ However, when comparing our results to those of older studies, it must be pointed out __ This is consistent with what has been found in previous __ A similar pattern of results was obtained in __ The findings are directly in line with previous findings These basic findings are consistent with research showing that __ Other results were broadly in line with __ 3. Limitations of your workBecause of the lack of __ we decided to not investigate __ One concern about the findings of __ was that __ Because of this potential limitation, we treat __ The limitations of the present studies naturally include __ Regarding the limitations of __, it could be argued that __ Another limitation of this __ This limitation is apparent in many __ Another limitation in __ involves the issue of __ The main limitation is the lack of __ One limitation is found in this case. One limitation of these methods however is that they __ It presents some limitations such as __ Although widely accepted, it suffers from some limitations due to __ An apparent limitation of the method is __ There are several limitations to this approach. One limitation of our implementation is that it is __ A major source of limitation is due to __ The approach utilised suffers from the limitation that __ The limitations are becoming clear __ It suffers from the same limitations associated with a __ 4. Casual argumentsA popular explanation of __ is that __ It is by now generally accepted that __ A popular explanation is that __ As it is not generally agreed that __ These are very small and difficult to observe. It is important to highlight the fact that __ It is notable that __ An important question associated with __ is __ This did not impair the __ This is important because there is __ This implies that __ is associated with __ This is indicative for lack of __ This will not be biased by __ There were also some important differences in __ It is interesting to note that, __ It is unlikely that __ This may alter or improve aspects of __ In contrast, this makes it possible to __ This is particularly important when investigating __ This has been used to successfully account for __ This introduces a possible confound in __ This was included to verify that __ 5. SpeculationsHowever, we acknowledge that there are considerable discussions among researchers as to __ We speculate that this might be due to __ There are reasons to doubt this explanation of __ It remains unclear to which degree __ are attributed to __ However, __ does seem to improve __ This does seem to depend on __ It is important to note, that the present evidence relies on __ The results show that __ does not seem to impact the __ However, the extent to which it is possible to __ is unknown Alternatively, it could simply mean that __ It is difficult to explain such results within the context of __ It is unclear whether this is a suitable for __ This appears to be a case of __ From this standpoint, __ can be considered as __ To date, __remain unknown Under certain assumptions, this can be construed as __ Because of this potential limitation, we treat __ In addition, several questions remain unanswered. At this stage of understanding, we believe__ Therefore, it remains unclear whether __ This may explain why __ 6. Deductive argumentsA difference between these __ can only be attributable to __ Nonetheless, we believe that it is well justified to __ This may raise concerns about __ which can be addressed by __ As discussed, this is due to the fact that __ Results demonstrate that this is not necessarily true. These findings support the notion that __ is not influenced by __ This may be the reason why we did not find __ In order to test whether this is equivalent across __, we __ Therefore, __ can be considered to be equivalent for __ Similar Posts![]() Academic Phrases for Writing Conclusion Section of a Research PaperIn this blog, we discuss phrases related to conclusion section such as summary of results and future work. ![]() How to Write a Research Paper? A Beginners Guide with Useful Academic PhrasesThis blog explains how to write a research paper and provides writing ideas in the form of academic phrases. ![]() Academic Phrases for Writing Literature Review Section of a Research PaperIn this blog, we discuss phrases related to literature review such as summary of previous literature, research gap and research questions. ![]() Academic Phrases for Writing Acknowledgements & Appendix Sections of a Research PaperIn this blog, we discuss phrases related to thanking colleagues, acknowledging funders and writing the appendix section. ![]() Academic Phrases for Writing Abstract Section of a Research PaperIn this blog, we discuss phrases related to the abstract section. An abstract is a self-contained and short synopsis that describes a larger work. ![]() Academic Writing Resources – Academic PhraseBank | Academic Vocabulary & Word ListsIn this blog, we review various academic writing resources such as academic phrasebank, academic wordlists, academic vocabulary training sites. 32 CommentsAwesome vocab given, I am really thankful. keep it up! Why didn’t I find this earlier? Thank you very much! Bless your soul! thank you!! very useful!!! Thank you, thank you thank you!! I’m currently writing up my PhD thesis and as a non-native English speaker, I find this site extremely useful. Thanks for making it! Very ve4y resourceful..well done Sam Plesse add me to your mailing list Email: [email protected] Hi, would like to clarify if that is “casual” or “causal”? Thanks! Hi there, it should read “causal.” Thanx for this. so helpful! Very helpful. Thanks thank you so much
thankyouuuuuu thank you very much wow thanks for the help!! Quite interesting! Thanks a lot! This is ammmaazzinggg, too bad im in my last year of university this is very handy!!! Extremely Useful. Thank-you so much. This is an excellent collection of phrases for effective writing Thank you so much, it has been helpful. I found it extremely important!!! It is a precise, brief and important guides; It is a very important which gives a guide; It is a very important guiding explanation for writing result and discussion; It is a very important guiding academic phrases for writing; thank you so much.I was in need of this.
Thank you so much!!! They are so helpful! thank its very important. This is timely, I needed it. Thanks This is very helpful. Thanks. You saved my Bachoelor thesis! Huge thanks Leave a Reply Cancel replyYour email address will not be published. Required fields are marked * Save my name, email, and website in this browser for the next time I comment.
We Trust in Human Precision20,000+ Professional Language Experts Ready to Help. Expertise in a variety of Niches. API Solutions
Value-Driven PricingUnmatched expertise at affordable rates tailored for your needs. Our services empower you to boost your productivity. ![]()
Trusted by Global LeadersGoTranscript is the chosen service for top media organizations, universities, and Fortune 50 companies. GoTranscriptOne of the Largest Online Transcription and Translation Agencies in the World. Founded in 2005. Speaker 1: In this video, we're going to explain exactly how to write up the results chapter for a quantitative study, whether that's a dissertation, thesis, or any other kind of academic research project. We'll walk you through the process step by step so that you can craft your results section with confidence. So, grab a cup of coffee, grab a cup of tea, whatever works for you, and let's jump into it. Hey, welcome to Grad Coach TV, where we demystify and simplify the oftentimes intimidating world of academic research. My name's Emma, and today we're going to explore the results chapter, which is also sometimes called the findings chapter in a dissertation or thesis. If you're new here, be sure to hit that subscribe button for more videos covering all things research related. Also, if you're looking for hands-on help with your research, check out our one-on-one coaching services, where we help you craft your research project step by step. It's basically like having a friendly professor in your pocket whenever you need it. If that sounds interesting to you, you can learn more and book a free consultation at www.gradcoach.com. All right, with that out of the way, let's get into it. Before we get into the nuts and bolts of how to write up the results chapter, it's useful to take a step back and ask the question, what exactly is a results chapter, and what purpose does it serve? If you understand both the what and the why, you'll have a much clearer direction in terms of the how. So, what's the results chapter all about then? Well, as the name suggests, the results chapter showcases the results of your quantitative analysis. In other words, it presents all the statistical data you've generated in a systematic and intuitive fashion. The results chapter is one of the most important chapters of your dissertation because it shows the reader what you found in terms of the quantitative data you've collected and analyzed. It presents the data using a clear text-based narrative, which is supported by tables, graphs, and charts. In addition to presenting these findings, it also highlights any potential issues you've come across, such as statistical outliers or unusual findings. But how's that different from the discussion chapter, you ask? Well, in the results chapter, you only present your statistical findings. Contrasted to this, in the discussion chapter, you interpret your findings and link them to prior research, in other words, your literature review, as well as your research objectives and research questions. Therefore, the key difference is that in the results chapter, you present and describe the data, while in the discussion chapter, you interpret the data and explain what it means in terms of the bigger picture. Let's take a look at an example. In your results chapter, you may have a plot that shows how respondents to a survey responded to a survey, the number of respondents per category, for instance. You may also state whether this supports one of your hypotheses by using a p-value from a statistical test. In other words, you're just presenting the facts and figures. Contrasted to this, in the discussion chapter, you will say why these statistical findings are relevant to your research question and how they compare with the existing literature. In other words, in the discussion chapter, you'll interpret your findings in relation to your research objectives. Long story short, the results chapter's job is purely to present and describe the data. So keep this in mind and make sure that you don't present anything other than the hard facts and figures. This is not the place for subjective interpretation. Now, a quick caveat. It's worth mentioning that some universities prefer you to combine the results and discussion chapters. Even so, it's still a good idea to separate the results and discussion elements within the chapter, as this ensures your findings are both described and interpreted in a consistent fashion. Typically, though, the results and discussion chapters are split up in quantitative studies. If you're unsure, chat with your research supervisor to find out what their preference is. All right, now that we've got that out of the way, we can look at how to write up the results chapter. Let's do it. There are multiple steps involved in writing up the results chapter for a quantitative study. The exact number of steps will vary from project to project and will depend on the nature of the research aims, objectives, and research questions. For example, some studies will make use of both descriptive and inferential statistics, while others will only use the former. So, in this video, we'll outline a generic process and structure that you can follow, but keep in mind that you may need to trim it down based on your specific research objectives. The first step in crafting your results chapter is to revisit your research objectives and questions. These will be, or at least should be, the driving force behind both your results and discussion chapters. During your statistical analysis, you will have generated a mountain of data, so you need to use your research objectives and questions to sift through this data and decide what's relevant. Therefore, the first step is for you to review your research objectives and research questions very closely and then ask yourself which statistical analyses and tests would specifically help you address these. For each research objective and research question, list the specific piece or pieces of analysis that address it. Keep this list handy as you'll revisit it multiple times as you craft your results chapter. At this stage, it's also useful to think about the key points that you want to raise in your discussion chapter and note these down. Every point you raise in your discussion chapter will need to be backed up in the results chapter, so you need to make sure that you lay a firm foundation there. So, jot down the main points you want to make in your discussion chapter and then list the specific piece of analysis that addresses each point. Having considered both of these areas, you should now have a short list of potential analyses and data points that you know need to be included in your results chapter in some shape or form. Next, you should draw up a rough outline of how you plan to structure your chapter. This doesn't need to be highly detailed, but you need to think about how you'll order the various analyses in your chapter so that there's a smooth logical flow. We'll discuss the standard structure of a quantitative results chapter in more detail shortly, but it's worth mentioning now that it's essential to draw up a rough outline before you start writing or you'll end up with a wishy-washy mess of information. This advice applies to any chapter, by the way. As with all chapters in your dissertation or thesis, you need to start your quantitative results chapter by providing a brief overview of what you'll do in the chapter and why. For example, you'd explain that you will start by presenting the sample demographic data to understand the composition and representativeness of the sample before moving on to X to understand Y and Z. This introduction section shouldn't be lengthy. A paragraph or two is more than enough. The aim is simply to give the reader a heads up about what you'll cover, not to provide a summary of the findings. Also, it's a good idea to weave the research questions into this section so that there's a golden thread that runs through your document. The first set of data that you'll typically present in your results chapter is an overview of the sample demographics. In other words, you'll give the reader an overview regarding the demographics of your survey respondents. For example, what age groups exist and how are they distributed? How is gender distributed? How is ethnicity distributed? What areas do the participants live in? Why is this important, you ask? Well, the purpose of this section is to assess how representative the sample is of the broader population. This is important for the sake of generalizability of the results. If your sample is not representative of the population, you won't be able to generalize your findings. This isn't necessarily a bad thing, but it's a limitation you'll need to acknowledge. Of course, to make this representativeness assessment, you'll need to already have an understanding of the demographics of the actual population you're interested in. So, make sure that you design your survey to capture the correct demographic information that you will compare your sample to. But, what if I'm not interested in generalizability, you say? Well, even if you don't intend to extrapolate your findings to the broader population, understanding your sample will allow you to interpret your findings appropriately, considering who responded. In other words, the demographic data will help you contextualize your findings accurately. For example, if 80% of your sample was aged over 65, this may be a noteworthy contextual factor to consider when interpreting the data. Similarly, if a large portion of your sample was skewed towards one gender, this would be an important contextual factor to note. Long story short, regardless of your intention to produce generalizable results, it's essential to understand and clearly communicate the demographic data of your sample. So, be sure to put in the time and effort in this section so that you can contextualize your findings accurately. Before you undertake your core statistical analysis, you need to do some checks to ensure that your data are suitable for the analysis methods and techniques you plan to use. If you try to analyze data that doesn't meet the assumptions of a specific statistical method or technique, your results will be largely meaningless. Therefore, you need to do some checks on your data before you jump into the actual analysis. Most commonly, there are two areas you need to pay attention to. The first thing you need to check is the reliability of your composite measures. When you have multiple scale-based measures that combine to capture one construct, this is called a composite measure. For example, you may have four Likert scales that all aim to measure the same thing, but are phrased in different ways. In other words, within a survey, these four scales should all receive similar ratings, assuming they are indeed measuring the same thing. This is called internal consistency. Unfortunately, internal consistency is not guaranteed, especially if you developed the scales yourself. So, you need to assess the reliability of each composite measure using a test. Cronbach's alpha is a common test used to assess internal consistency. In other words, to show that the items you're combining are more or less saying the same thing. A high alpha score means that your composite measure is internally consistent. A low alpha score means you may need to scrap one or more of the individual measures. There are tests other than Cronbach's alpha that can be used, and there's some hot debate about which one is the best, but we won't get into that here. The key takeaway is that you need to undertake some sort of testing to assess internal consistency, and you need to present those test results in this section of your chapter. Once you're comfortable that your composite measures are internally consistent, the next thing you need to look at is the shape of the data for each of your variables. What do you mean the shape of the data? Well, for each variable, you need to assess whether the data are symmetrical. In other words, normally distributed in a nice bell curve or not. This is important as it will directly impact what type of analysis methods and techniques you can use. For many common inferential tests, such as t-test and ANOVAs, we'll discuss these a bit later, don't stress, your data needs to be normally distributed. In other words, symmetrical. If it's not, you'll need to adjust your strategy and use alternative statistical tests. To assess the shape of the data, you'll usually assess a variety of fairly basic descriptive statistics, such as the mean, median, and skewness, which is exactly what we'll look at next. Now that you've laid the foundation by examining the representativeness of your sample, the reliability of your composite measures, and the shape of your data, you can get started with the actual statistical analysis. Finally. The first step is to present the descriptive statistics for your variables. As I mentioned, the descriptive statistics will help you assess the shape of your data. As I mentioned, the descriptive statistics will help you assess the shape of your data. But depending on the nature of your research, the descriptive statistics could also play an important role in directly addressing your research objectives and research questions. So, what are descriptive statistics? When we talk about descriptives, this usually includes basic statistics, such as the mean. This is simply the mathematical average of a range of numbers. The median. This is the midpoint in a range of numbers when the numbers are arranged in order. Standard deviation. This metric indicates how dispersed a range of numbers is. In other words, how close all the numbers are to the mean, the average. Skewness. This indicates how symmetrical a range of numbers is. In other words, do they tend to cluster into a smooth bell curve shape in the middle of the graph? Or do they lean to the left or the right? And lastly, kurtosis. This metric indicates whether the data are heavily or lightly tailed relative to the normal distribution. In other words, how peaked or flat the distribution is. If these statistics sound like gibberish to you, be sure to check out our video covering the basics of quantitative data analysis. I'll include the link below. When you're presenting your descriptive stats, using a large table to present all the stats for multiple variables can be a very effective way to present your data economically. This saves you a lot of space and makes it easier to compare and contrast the statistics for each variable. You can also use color coding to help make the data more easily digestible. For categorical data, for example, data that shows the percentage of people who chose or fit into each category, you can either just plain describe the percentages or numbers of people who responded to something, or you could use graphs and charts such as bar graphs and pie charts to present your data. There's no one size fits all approach here. In some cases, it will make more sense to just present the numbers in a table or a paragraph. For example, if there are only two categories. While in other cases, graphs and charts will be useful. For example, if there are multiple categories. A pro tip, when using charts and graphs, make sure that you label them simply and clearly so that your reader can easily understand them. There is nothing more frustrating than a graph that's missing axis labels. Keep in mind that although you'll be presenting tables, charts and graphs, your text content needs to present a clear narrative that can stand on its own. In other words, don't rely purely on your figures and tables to convey your point. Highlight the crucial trends and values in the body of the text. Figures and tables should complement your writing, not carry it. All right, so that covers the basics of descriptive statistics. Depending on your research aims, objectives and research questions, you may end your analysis here. However, if your study also requires inferential statistics, then it's time to get started on those. All right, on to inferentials. Unlike descriptive statistics where the focus is purely on the sample, inferential statistics are used to make predictions about the population. So this part of the results chapter is where things can get really interesting. Inferential methods, broadly speaking, can be broken up into two groups. First up, there are those analyses that compare measurements between groups, such as t-tests, which measure differences between two groups, and ANOVAs, which measure differences between multiple groups. For example, you could use ANOVA to assess the difference in average weight loss between three groups that adopted three different diets. The second type of inferential methods are those that assess relationships between variables, such as correlation analysis and regression analysis. For example, you could use correlation analysis to assess the relationship between the number of hours studied and test marks earned within a sample of students. Within each of these inferentials, some tests can be used for normally distributed data, in other words, symmetrical data. And some tests are designed specifically for use on non-normally distributed data. So it's important to make sure that you use the right analysis tool for your data shape. Remember, you would have assessed data shape in your descriptive statistics section, so make sure that you align your inferential approach with those findings. There are a seemingly endless number of analysis methods and tests that you can use to crunch your data, so it's easy to run down a rabbit hole and end up with piles of test data. Therefore, you need to be selective about which methods you use. Ultimately, the most important thing is to make sure that you adopt the analysis methods that allow you to achieve your research objectives and answer your research questions. So let those two factors guide you. As with the descriptive statistics, in this section of your results chapter, you should try to make use of figures and tables as effectively as possible. For example, if you present a correlation table, use color coding to highlight the significance of the correlation values, or present a scatter plot to visually demonstrate what the trend is. The easier you make it for your reader to digest your findings, the more effectively you'll be able to make your arguments in the next chapter. Right. With both your descriptive and inferential statistics presented, you should have now laid the foundation for your discussion chapter. So at this stage, it's a good idea to quickly revisit that list that you drew up in step one and make sure you've covered all the necessary data to support your research objectives and research questions. If you have, you're ready to take the next step. If your study requires it, the next component of your results chapter will be hypothesis testing. Not every study will need hypotheses, so don't feel like you need to shoehorn these in if you haven't got any. As with so many things in your dissertation, the need for hypotheses depends on your research aims, objectives, and questions. So what exactly is a hypothesis? Generally speaking, a hypothesis is a statement that expresses an expected difference between groups or a relationship between variables. Importantly, it's a statement that can be supported or rejected by a statistical test. In other words, it needs to be very specific and measurable and cannot leave any room for interpretation or subjectivity. For example, a statement like, there is a relationship between study hours and test marks scored could be supported or rejected by a statistical test. For example, correlation analysis. Contrasted to this, a statement like, ice cream is the meaning of life, couldn't be supported by a statistical test, as much as I wish it could be. So if your dissertation or thesis included the development of hypotheses in the literature review chapter, this section is where you'd present them once again and test them using your statistical data. If you want to learn more about hypotheses, check out our detailed post over on the Grad Coach blog. I'll include a link below this video. One last thing. If your research involved developing a theoretical framework, you can, at this stage, present that framework once again, this time populating it with the hypothesis testing data. For example, if you were developing a theoretical model of the antecedents of trust and your hypotheses related to the antecedent variables, you could present the model again, this time incorporating the test results for each variable. Right, with your quantitative analyses presented, it's time to wrap up your results chapter and transition to the discussion chapter. To conclude your results chapter, the final step is to provide a brief summary of the key findings. Brief is the key word here. Much like the chapter introduction, this shouldn't be lengthy, a paragraph or two maximum. In this section, you only need to highlight the findings most relevant to your research objectives and research questions so that you lay the foundation for the next chapter. Don't provide a lengthy recap of each and every section of results. Just remind the reader of the key takeaways and wrap it up. If you work through your results chapter step-by-step as we've discussed in this video, you should land up with a comprehensive presentation of your key data. Keep in mind that what we've discussed here is a generic structure. There's no one-size-fits-all. The exact structure and contents of your results chapter will be influenced by your specific research objectives and research questions. As I mentioned in step one, start by crafting a list that covers your research objectives and questions and mapping that to the various statistical analyses and tests you've undertaken. Revisit that list as you work through each section of your results chapter and you can rest assured that you'll be headed in a good direction. All right, so that wraps it up for today. If you enjoyed the video, hit the like button and please leave a comment if you have any questions. Also, be sure to subscribe for more research-related content. Remember, if you need a helping hand with your research, be sure to check out our private coaching service where we work with you on a one-on-one basis, chapter-by-chapter, to help you craft a winning dissertation, thesis, or research project. If that sounds interesting to you, book a free consultation with a friendly coach at www.gradcoach.com. As always, I'll include a link below. That's all for this episode of Grad Coach TV. Until next time, good luck. Grad Coach ![]() Quantitative Data Analysis: Everything You Need to Know11 min read ![]() Does the thought of quantitative data analysis bring back the horrors of math classes? We get it. But conducting quantitative data analysis doesn’t have to be hard with the right tools. Want to learn how to turn raw numbers into actionable insights on how to improve your product? In this article, we explore what quantitative data analysis is, the difference between quantitative and qualitative data analysis, and statistical methods you can apply to your data. We also walk you through the steps you can follow to analyze quantitative information, and how Userpilot can help you streamline the product analytics process. Let’s get started.
![]() Try Userpilot and Take Your Product Experience to the Next Level
![]() What is quantitative data analysis?Quantitative data analysis is about applying statistical analysis methods to define, summarize, and contextualize numerical data. In short, it’s about turning raw numbers and data into actionable insights. The analysis will vary depending on the research questions and the collected data (more on this below). Quantitative vs qualitative data analysisThe main difference between these forms of analysis lies in the collected data. Quantitative data is numerical or easily quantifiable. For example, the answers to a customer satisfaction score (CSAT) survey are quantitative since you can count the number of people who answered “very satisfied”. Qualitative feedback , on the other hand, analyzes information that requires interpretation. For instance, evaluating graphics, videos, text-based answers, or impressions. Another difference between quantitative and qualitative analysis is the questions each seeks to answer. For instance, quantitative data analysis primarily answers what happened, when it happened, and where it happened. However, qualitative data analysis answers why and how an event occurred. Quantitative data analysis also looks into identifying patterns , drivers, and metrics for different groups. However, qualitative analysis digs deeper into the sample dataset to understand underlying motivations and thinking processes. Pros of quantitative data analysisQuantitative or data-driven analysis has advantages such as:
Cons of quantitative data analysisThese are common disadvantages of data-driven analytics :
Quantitative data analysis methodsThere are two statistical methods for reviewing quantitative data and user analytics . However, before exploring these in-depth, let’s refresh these key concepts:
Here are methods for analyzing quantitative data: Descriptive statisticsDescriptive statistics, as the name implies, describe your data and help you understand your sample in more depth. It doesn’t make inferences about the entire population but only focuses on the details of your specific sample. Descriptive statistics usually include measures like the mean, median, percentage, frequency, skewness, and mode. Inferential statisticsInferential statistics aim to make predictions and test hypotheses about the real-world population based on your sample data. Here, you can use methods such as a T-test, ANOVA, regression analysis, and correlation analysis. Let’s take a look at this example. Through descriptive statistics, you identify that users under the age of 25 are more likely to skip your onboarding. You’ll need to apply inferential statistics to determine if the result is statistically significant and applicable to your entire ’25 or younger’ population. How to choose the right method for your quantitative data analysisThe type of data that you collect and the research questions that you want to answer will impact which quantitative data analysis method you choose. Here’s how to choose the right method: Determine your data typeBefore choosing the quantitative data analysis method, you need to identify which group your data belongs to:
Applying any statistical method to all data types can lead to meaningless results. Instead, identify which statistical analysis method supports your collected data types. Consider your research questionsThe specific research questions you want to answer, and your hypothesis (if you have one) impact the analysis method you choose. This is because they define the type of data you’ll collect and the relationships you’re investigating. For instance, if you want to understand sample specifics, descriptive statistics—such as tracking NPS —will work. However, if you want to determine if other variables affect the NPS, you’ll need to conduct an inferential analysis. The overarching questions vary in both of the previous examples. For calculating the NPS, your internal research question might be, “Where do we stand in customer loyalty ?” However, if you’re doing inferential analysis, you may ask, “How do various factors, such as demographics, affect NPS?” 6 steps to do quantitative data analysis and extract meaningful insightsHere’s how to conduct quantitative analysis and extract customer insights : 1. Set goals for your analysisBefore diving into data collection, you need to define clear goals for your analysis as these will guide the process. This is because your objectives determine what to look for and where to find data. These goals should also come with key performance indicators (KPIs) to determine how you’ll measure success. For example, imagine your goal is to increase user engagement. So, relevant KPIs include product engagement score , feature usage rate , user retention rate, or other relevant product engagement metrics . 2. Collect quantitative dataOnce you’ve defined your goals, you need to gather the data you’ll analyze. Quantitative data can come from multiple sources, including user surveys such as NPS, CSAT, and CES, website and application analytics , transaction records, and studies or whitepapers. Remember: This data should help you reach your goals. So, if you want to increase user engagement , you may need to gather data from a mix of sources. For instance, product analytics tools can provide insights into how users interact with your tool, click on buttons, or change text. Surveys, on the other hand, can capture user satisfaction levels . Collecting a broad range of data makes your analysis more robust and comprehensive. ![]() 3. Clean and visualize your dataRaw data is often messy and contains duplicates, outliers, or missing values that can skew your analysis. Before making any calculations, clean the data by removing these anomalies or outliers to ensure accurate results. Once cleaned, turn it into visual data by using different types of charts , graphs, or heatmaps . Visualizations and data analytics charts make it easier to spot trends, patterns, and anomalies. If you’re using Userpilot, you can choose your preferred visualizations and organize your dashboard to your liking. 4. Identify patterns and trendsWhen looking at your dashboards, identify recurring themes, unusual spikes, or consistent declines that might indicate data analytics trends or potential issues. Picture this: You notice a consistent increase in feature usage whenever you run seasonal marketing campaigns . So, you segment the data based on different promotional strategies. There, you discover that users exposed to email marketing campaigns have a 30% higher engagement rate than those reached through social media ads. In this example, the pattern suggests that email promotions are more effective in driving feature usage. If you’re a Userpilot user, you can conduct a trend analysis by tracking how your users perform certain events. ![]() 5. Share valuable insights with key stakeholdersOnce you’ve discovered meaningful insights, you have to communicate them to your organization’s key stakeholders. Do this by turning your data into a shareable analysis report , one-pager, presentation, or email with clear and actionable next steps. Your goal at this stage is for others to view and understand the data easily so they can use the insights to make data-led decisions. Following the previous example, let’s say you’ve found that email campaigns significantly boost feature usage. Your email to other stakeholders should strongly recommend increasing the frequency of these campaigns and adding the supporting data points. Take a look at how easy it is to share custom dashboards you built in Userpilot with others via email: 6. Act on the insightsData analysis is only valuable if it leads to actionable steps that improve your product or service. So, make sure to act upon insights by assigning tasks to the right persons. For example, after analyzing user onboarding data, you may find that users who completed the onboarding checklist were 3x more likely to become paying customers ( like Sked Social did! ). Now that you have actual data on the checklist’s impact on conversions, you can work on improving it, such as simplifying its steps, adding interactive features, and launching an A/B test to experiment with different versions. How can Userpilot help with analyzing quantitative dataAs you’ve seen throughout this article, using a product analytics tool can simplify your data analysis and help you get insights faster. Here are different ways in which Userpilot can help: Automatically capture quantitative dataThanks to Userpilot’s new auto-capture feature, you can automatically track every time your users click, write a text, or fill out a form in your app—no engineers or manual tagging required! Our customer analytics platform lets you use this data to build segments, trigger personalized in-app events and experiences, or launch surveys. If you don’t want to auto-capture raw data, you can turn this functionality off in your settings, as seen below: ![]() Monitor key metrics with customizable dashboards for real-time insightsUserpilot comes with template analytics dashboards , such as new user activation dashboards or customer engagement dashboards . However, you can create custom dashboards and reports to keep track of metrics that are relevant to your business in real time. For instance, you could build a customer retention analytics dashboard and include all metrics that you find relevant, such as customer stickiness , NPS, or last accessed date. Analyze experiment data with A/B and multivariate testsUserpilot lets you conduct A/B and multivariate tests , either by following a controlled or a head-to-head approach. You can track the results on a dashboard. For example, let’s say you want to test a variation of your onboarding flow to determine which leads to higher user activation . You can go to Userpilot’s Flows tab and click on Experiments. There, you’ll be able to select the type of test you want to run, for instance, a controlled A/B test , build a new flow, test it, and get the results. ![]() Use quantitative funnel analysis to increase conversion ratesWith Userpilot, you can track your customers’ journey as they complete actions and move through the funnel. Funnel analytics give you insights into your conversion rates and conversion times between two events, helping you identify areas for improvement. Imagine you want to analyze your free-to-paid conversions and the differences between devices. Just by looking at the graphic, you can draw some insights:
![]() Leverage cohort analysis to optimize retentionAnother Userpilot functionality that can help you analyze quantitative data is cohort analysis . This powerful tool lets you group users based on shared characteristics or experiences, allowing you to analyze their behavior over time and identify trends, patterns, and the long-term impact of changes on user behavior. For example, let’s say you recently released a feature and want to measure its impact on user retention. Via a cohort analysis, you can group users who started using your product after the update and compare their retention rates to previous cohorts. You can do this in Userpilot by creating segments and then tracking user segments ‘ retention rates over time. ![]() Check how many users adopted a feature with a retention tableIn Userpilot, you can use retention tables to stay on top of feature adoption . This means you can track how many users continue to use a feature over time and which features are most valuable to your users. The video below shows how to choose the features or events you want to analyze in Userpilot. As you’ve seen, to conduct quantitative analysis, you first need to identify your business and research goals. Then, collect, clean, and visualize the data to spot trends and patterns. Lastly, analyze the data, share it with stakeholders, and act upon insights to build better products and drive customer satisfaction. To stay on top of your KPIs, you need a product analytics tool. With Userpilot, you can automate data capture, analyze product analytics, and view results in shareable dashboards. Want to try it for yourself? Get a demo . Leave a comment Cancel replySave my name, email, and website in this browser for the next time I comment. ![]() Get The Insights!The fastest way to learn about Product Growth,Management & Trends. The coolest way to learn about Product Growth, Management & Trends. Delivered fresh to your inbox, weekly. ![]() The fastest way to learn about Product Growth, Management & Trends. You might also be interested in ...Heap autocapture: an in-depth review + a better alternative. Aazar Ali Shad Guide to Auto-Capture in SaaS: Benefits, Use Cases and ToolsFrequently asked questionsWhat’s the difference between results and discussion. The results chapter or section simply and objectively reports what you found, without speculating on why you found these results. The discussion interprets the meaning of the results, puts them in context, and explains why they matter. In qualitative research , results and discussion are sometimes combined. But in quantitative research , it’s considered important to separate the objective results from your interpretation of them. Frequently asked questions: DissertationDissertation word counts vary widely across different fields, institutions, and levels of education:
However, none of these are strict guidelines – your word count may be lower or higher than the numbers stated here. Always check the guidelines provided by your university to determine how long your own dissertation should be. A dissertation prospectus or proposal describes what or who you plan to research for your dissertation. It delves into why, when, where, and how you will do your research, as well as helps you choose a type of research to pursue. You should also determine whether you plan to pursue qualitative or quantitative methods and what your research design will look like. It should outline all of the decisions you have taken about your project, from your dissertation topic to your hypotheses and research objectives , ready to be approved by your supervisor or committee. Note that some departments require a defense component, where you present your prospectus to your committee orally. A thesis is typically written by students finishing up a bachelor’s or Master’s degree. Some educational institutions, particularly in the liberal arts, have mandatory theses, but they are often not mandatory to graduate from bachelor’s degrees. It is more common for a thesis to be a graduation requirement from a Master’s degree. Even if not mandatory, you may want to consider writing a thesis if you:
The conclusion of your thesis or dissertation should include the following:
The conclusion of your thesis or dissertation shouldn’t take up more than 5–7% of your overall word count. For a stronger dissertation conclusion , avoid including:
Your conclusion should leave the reader with a strong, decisive impression of your work. While it may be tempting to present new arguments or evidence in your thesis or disseration conclusion , especially if you have a particularly striking argument you’d like to finish your analysis with, you shouldn’t. Theses and dissertations follow a more formal structure than this. All your findings and arguments should be presented in the body of the text (more specifically in the discussion section and results section .) The conclusion is meant to summarize and reflect on the evidence and arguments you have already presented, not introduce new ones. A theoretical framework can sometimes be integrated into a literature review chapter , but it can also be included as its own chapter or section in your dissertation . As a rule of thumb, if your research involves dealing with a lot of complex theories, it’s a good idea to include a separate theoretical framework chapter. A literature review and a theoretical framework are not the same thing and cannot be used interchangeably. While a theoretical framework describes the theoretical underpinnings of your work, a literature review critically evaluates existing research relating to your topic. You’ll likely need both in your dissertation . While a theoretical framework describes the theoretical underpinnings of your work based on existing research, a conceptual framework allows you to draw your own conclusions, mapping out the variables you may use in your study and the interplay between them. A thesis or dissertation outline is one of the most critical first steps in your writing process. It helps you to lay out and organize your ideas and can provide you with a roadmap for deciding what kind of research you’d like to undertake. Generally, an outline contains information on the different sections included in your thesis or dissertation , such as:
When you mention different chapters within your text, it’s considered best to use Roman numerals for most citation styles. However, the most important thing here is to remain consistent whenever using numbers in your dissertation . In most styles, the title page is used purely to provide information and doesn’t include any images. Ask your supervisor if you are allowed to include an image on the title page before doing so. If you do decide to include one, make sure to check whether you need permission from the creator of the image. Include a note directly beneath the image acknowledging where it comes from, beginning with the word “ Note .” (italicized and followed by a period). Include a citation and copyright attribution . Don’t title, number, or label the image as a figure , since it doesn’t appear in your main text. Definitional terms often fall into the category of common knowledge , meaning that they don’t necessarily have to be cited. This guidance can apply to your thesis or dissertation glossary as well. However, if you’d prefer to cite your sources , you can follow guidance for citing dictionary entries in MLA or APA style for your glossary. A glossary is a collection of words pertaining to a specific topic. In your thesis or dissertation, it’s a list of all terms you used that may not immediately be obvious to your reader. In contrast, an index is a list of the contents of your work organized by page number. The title page of your thesis or dissertation goes first, before all other content or lists that you may choose to include. The title page of your thesis or dissertation should include your name, department, institution, degree program, and submission date. Glossaries are not mandatory, but if you use a lot of technical or field-specific terms, it may improve readability to add one to your thesis or dissertation. Your educational institution may also require them, so be sure to check their specific guidelines. A glossary or “glossary of terms” is a collection of words pertaining to a specific topic. In your thesis or dissertation, it’s a list of all terms you used that may not immediately be obvious to your reader. Your glossary only needs to include terms that your reader may not be familiar with, and is intended to enhance their understanding of your work. A glossary is a collection of words pertaining to a specific topic. In your thesis or dissertation, it’s a list of all terms you used that may not immediately be obvious to your reader. In contrast, dictionaries are more general collections of words. An abbreviation is a shortened version of an existing word, such as Dr. for Doctor. In contrast, an acronym uses the first letter of each word to create a wholly new word, such as UNESCO (an acronym for the United Nations Educational, Scientific and Cultural Organization). As a rule of thumb, write the explanation in full the first time you use an acronym or abbreviation. You can then proceed with the shortened version. However, if the abbreviation is very common (like PC, USA, or DNA), then you can use the abbreviated version from the get-go. Be sure to add each abbreviation in your list of abbreviations ! If you only used a few abbreviations in your thesis or dissertation , you don’t necessarily need to include a list of abbreviations . If your abbreviations are numerous, or if you think they won’t be known to your audience, it’s never a bad idea to add one. They can also improve readability, minimizing confusion about abbreviations unfamiliar to your reader. A list of abbreviations is a list of all the abbreviations that you used in your thesis or dissertation. It should appear at the beginning of your document, with items in alphabetical order, just after your table of contents . Your list of tables and figures should go directly after your table of contents in your thesis or dissertation. Lists of figures and tables are often not required, and aren’t particularly common. They specifically aren’t required for APA-Style, though you should be careful to follow their other guidelines for figures and tables . If you have many figures and tables in your thesis or dissertation, include one may help you stay organized. Your educational institution may require them, so be sure to check their guidelines. A list of figures and tables compiles all of the figures and tables that you used in your thesis or dissertation and displays them with the page number where they can be found. The table of contents in a thesis or dissertation always goes between your abstract and your introduction . You may acknowledge God in your dissertation acknowledgements , but be sure to follow academic convention by also thanking the members of academia, as well as family, colleagues, and friends who helped you. A literature review is a survey of credible sources on a topic, often used in dissertations , theses, and research papers . Literature reviews give an overview of knowledge on a subject, helping you identify relevant theories and methods, as well as gaps in existing research. Literature reviews are set up similarly to other academic texts , with an introduction , a main body, and a conclusion . An annotated bibliography is a list of source references that has a short description (called an annotation ) for each of the sources. It is often assigned as part of the research process for a paper . In a thesis or dissertation, the discussion is an in-depth exploration of the results, going into detail about the meaning of your findings and citing relevant sources to put them in context. The conclusion is more shorter and more general: it concisely answers your main research question and makes recommendations based on your overall findings. In the discussion , you explore the meaning and relevance of your research results , explaining how they fit with existing research and theory. Discuss:
Results are usually written in the past tense , because they are describing the outcome of completed actions. The results chapter of a thesis or dissertation presents your research results concisely and objectively. In quantitative research , for each question or hypothesis , state:
In qualitative research , for each question or theme, describe:
Don’t interpret or speculate in the results chapter. To automatically insert a table of contents in Microsoft Word, follow these steps:
Make sure to update your table of contents if you move text or change headings. To update, simply right click and select Update Field. All level 1 and 2 headings should be included in your table of contents . That means the titles of your chapters and the main sections within them. The contents should also include all appendices and the lists of tables and figures, if applicable, as well as your reference list . Do not include the acknowledgements or abstract in the table of contents. The abstract appears on its own page in the thesis or dissertation , after the title page and acknowledgements but before the table of contents . An abstract for a thesis or dissertation is usually around 200–300 words. There’s often a strict word limit, so make sure to check your university’s requirements. In a thesis or dissertation, the acknowledgements should usually be no longer than one page. There is no minimum length. The acknowledgements are generally included at the very beginning of your thesis , directly after the title page and before the abstract . Yes, it’s important to thank your supervisor(s) in the acknowledgements section of your thesis or dissertation . Even if you feel your supervisor did not contribute greatly to the final product, you must acknowledge them, if only for a very brief thank you. If you do not include your supervisor, it may be seen as a snub. In the acknowledgements of your thesis or dissertation, you should first thank those who helped you academically or professionally, such as your supervisor, funders, and other academics. Then you can include personal thanks to friends, family members, or anyone else who supported you during the process. Ask our teamWant to contact us directly? No problem. We are always here for you.
![]() Our team helps students graduate by offering:
Scribbr specializes in editing study-related documents . We proofread:
Scribbr’s Plagiarism Checker is powered by elements of Turnitin’s Similarity Checker , namely the plagiarism detection software and the Internet Archive and Premium Scholarly Publications content databases . The add-on AI detector is powered by Scribbr’s proprietary software. The Scribbr Citation Generator is developed using the open-source Citation Style Language (CSL) project and Frank Bennett’s citeproc-js . It’s the same technology used by dozens of other popular citation tools, including Mendeley and Zotero. You can find all the citation styles and locales used in the Scribbr Citation Generator in our publicly accessible repository on Github . ![]() Understanding Qualitative and Quantitative Data
![]() Written by: ![]() Smith Alex is a committed data enthusiast and an aspiring leader in the domain of data analytics. With a foundation in engineering and practical experience in the field of data science Summary: This article delves into qualitative and quantitative data, defining each type and highlighting their key differences. It discusses when to use each data type, the benefits of integrating both, and the challenges researchers face. Understanding these concepts is crucial for effective research design and achieving comprehensive insights. IntroductionIn the realm of research and Data Analysis , two fundamental types of data play pivotal roles: qualitative and quantitative data. Understanding the distinctions between these two categories is essential for researchers, analysts, and decision-makers alike, as each type serves different purposes and is suited to various contexts. This article will explore the definitions, characteristics, uses, and challenges associated with both qualitative and quantitative data, providing a comprehensive overview for anyone looking to enhance their understanding of data collection and analysis. Read More: Exploring 5 Statistical Data Analysis Techniques with Real-World Examples Defining Qualitative DataQualitative data is non-numerical in nature and is primarily concerned with understanding the qualities, characteristics, and attributes of a subject. This type of data is descriptive and often involves collecting information through methods such as interviews, focus groups, observations, and open-ended survey questions. The goal of qualitative data is to gain insights into the underlying motivations, opinions, and experiences of individuals or groups. Characteristics of Qualitative Data
Examples of Qualitative Data
Defining Quantitative DataQuantitative data, in contrast, is numerical and can be measured or counted. This type of data is often used to quantify variables and analyse relationships between them. Quantitative research typically employs statistical methods to test hypotheses, identify patterns, and make predictions based on numerical data. Characteristics of Quantitative Data
Examples of Quantitative Data
Key Differences Between Qualitative and Quantitative DataUnderstanding the differences between qualitative and quantitative data is crucial for selecting the appropriate research methods and analysis techniques. Here are some key distinctions: When to Use Qualitative DataQualitative data is particularly useful in situations where the research aims to explore complex phenomena, understand human behaviour, or generate new theories. Here are some scenarios where qualitative data is the preferred choice: Exploratory ResearchWhen investigating a new area of study where little is known, qualitative methods can help uncover insights and generate hypotheses. Understanding ContextQualitative data is valuable for capturing the context surrounding a particular phenomenon, providing depth to the analysis. Gaining Insights into Attitudes and BehavioursWhen the goal is to understand why individuals think or behave in a certain way, qualitative methods such as interviews can provide rich, nuanced insights. Developing TheoriesQualitative research can help in the development of theories by exploring relationships and patterns that quantitative methods may overlook. When to Use Quantitative DataQuantitative data is best suited for research that requires measurement, comparison, and statistical analysis. Here are some situations where quantitative data is the preferred choice: Testing HypothesesWhen researchers have specific hypotheses to test , quantitative methods allow for rigorous statistical analysis to confirm or reject these hypotheses. Measuring VariablesQuantitative data is ideal for measuring variables and establishing relationships between them, making it useful for experiments and surveys. Generalising FindingsWhen the goal is to generalise findings to a larger population, quantitative research provides the necessary data to support such conclusions. Identifying Patterns and TrendsQuantitative analysis can reveal patterns and trends in data that can inform decision-making and policy development. Integrating Qualitative and Quantitative DataWhile qualitative and quantitative data are distinct, they can be effectively integrated to provide a more comprehensive understanding of a research question. This mixed-methods approach combines the strengths of both types of data, allowing researchers to triangulate findings and gain deeper insights. Benefits of IntegrationIntegrating qualitative and quantitative data enhances research by combining numerical analysis with rich, descriptive insights. This mixed-methods approach allows for a comprehensive understanding of complex phenomena, validating findings and providing a more nuanced perspective on research questions.
Examples of Integration
Challenges and Considerations While qualitative and quantitative data offer distinct advantages, researchers must also be aware of the challenges and considerations associated with each type: Challenges of Qualitative DataThe challenges of qualitative data are multifaceted and can significantly impact the research process. Here are some of the primary challenges faced by researchers when working with qualitative data: Subjectivity and BiasOne of the most significant challenges in qualitative research is the inherent subjectivity involved in data collection and analysis. Researchers’ personal beliefs, assumptions, and experiences can influence their interpretation of data. Data OverloadQualitative research often generates large volumes of data, which can be overwhelming. This data overload can make it challenging to identify key themes and insights. Researchers may struggle to manage and analyse vast amounts of qualitative data, leading to potential insights being overlooked. Lack of StructureQualitative data is often unstructured, making it difficult to analyse systematically. The absence of a predefined format can lead to challenges in drawing meaningful conclusions from the data. Time-Consuming NatureQualitative analysis can be extremely time-consuming, especially when dealing with extensive data sets. The process of collecting, transcribing, and analysing qualitative data often requires significant time and resources, which can be a barrier for researchers. Challenges of Quantitative DataQuantitative data provides objective, measurable evidence, it also faces challenges in capturing the full complexity of human experiences, maintaining data accuracy, and avoiding misinterpretation of statistical results. Integrating qualitative data can help overcome some of these limitations. ![]() Limits in Capturing ComplexityQuantitative data, by its nature, can oversimplify complex phenomena and miss important nuances that qualitative data can capture. The focus on numerical measurements may not fully reflect the depth and richness of human experiences and behaviours. Chances for MisinterpretationNumbers can be twisted or misinterpreted if not analysed properly. Researchers must be cautious in interpreting statistical results, as correlation does not imply causation. Poor knowledge of statistical analysis can negatively impact the analysis and interpretation of quantitative data. Influence of Measurement ErrorsDue to the numerical nature of quantitative data, even small measurement errors can skew the entire dataset. Inaccuracies in data collection methods can lead to drawing incorrect conclusions from the analysis. Lack of ContextQuantitative experiments often do not take place in natural settings. The data may lack the context and nuance that qualitative data can provide to fully explain the phenomena being studied. Sample Size LimitationsSmall sample sizes in quantitative studies can reduce the reliability of the data. Large sample sizes are needed for more accurate statistical analysis. This also affects the ability to generalise findings to wider populations. Confirmation BiasResearchers may miss observing important phenomena due to their focus on testing pre-determined hypotheses rather than generating new theories. The confirmation bias inherent in hypothesis testing can limit the discovery of unexpected insights. In conclusion, understanding the distinctions between qualitative and quantitative data is essential for effective research and Data Analysis . Each type of data serves unique purposes and is suited to different contexts, making it crucial for researchers to select the appropriate methods based on their research objectives. By integrating both qualitative and quantitative data, researchers can gain a more comprehensive understanding of complex phenomena, leading to richer insights and more informed decision-making. As the landscape of research continues to evolve, the ability to effectively utilise and integrate both types of data will remain a valuable skill for researchers and analysts alike. Frequently Asked QuestionsWhat is the primary difference between qualitative and quantitative data. The primary difference is that qualitative data is descriptive and non-numerical, focusing on understanding qualities and experiences, while quantitative data is numerical and measurable, focusing on quantifying variables and testing hypotheses. When Should I Use Qualitative Data in My Research?Qualitative data is best used when exploring new topics, understanding complex behaviours, or generating hypotheses, particularly when context and depth are important. Can Qualitative and Quantitative Data Be Used Together?Yes, integrating qualitative and quantitative data can provide a more comprehensive understanding of a research question, allowing researchers to validate findings and gain richer insights. Reviewed by: ![]() Post written by: Smith AlexArtificial Intelligence Web Scraping vs. Web Crawling: Understanding the Differences![]() Python Metaprogramming: Unlocking the Power of Code ManipulationYou may also like. ![]()
Top Data Analytics Projects in 2024 for Beginners to Experienced
![]() Explaining Four Types of Analytics With Examples
Thank you for visiting nature.com. You are using a browser version with limited support for CSS. To obtain the best experience, we recommend you use a more up to date browser (or turn off compatibility mode in Internet Explorer). In the meantime, to ensure continued support, we are displaying the site without styles and JavaScript.
Implementation of the ARIMA model for prediction of economic variables: evidence from the health sector in Brazil
Humanities and Social Sciences Communications volume 11 , Article number: 1068 ( 2024 ) Cite this article 143 Accesses 1 Altmetric Metrics details
In recent decades, quantitative models for forecasting economic crises have garnered significant interest from researchers, policymakers, and public and private institutions. Identifying the most appropriate models for predicting economic time series behaviors during crises is a pressing challenge. Effective techniques can be instrumental in forestalling financial irregularities, thus empowering institutions to deploy remedial actions and swiftly minimizing further economic setbacks. Contemporary literature introduces various forecasting models, such as the autoregressive integrated moving average (ARIMA) model. Recognized for its statistical alignment with numerous linear models, the ARIMA model has demonstrated its efficacy across various domains. This paper delves into applying the ARIMA model to predict five critical economic time series that substantially influenced Brazil’s public and private healthcare sectors throughout the economic crisis between 2000 and 2020. These time series encompassed the variables (i) the gross domestic product—GDP, (ii) the Extended National Consumer Price Index—IPCA, (iii) the unemployment rate, (iv) the total number of health plan beneficiaries, and (v) total number of individual health plan beneficiaries. Importantly, this study provides a comprehensive outline of the ARIMA implementation process, underscoring that precise forecasting is pivotal for managers aiming to curtail financial anomalies and avert resource shortages. The findings highlight the ARIMA model’s (1, 0, 2), (2, 2, 1), (0, 1, 2), (1, 1, 2), and (2, 2, 1) viability in accurately forecasting health-related time series, exceeding 95% accuracy for economic variables analyzed. These results have significant practical implications for healthcare managers and decision-makers. By offering accurate forecasts of critical economic metrics, such as the unemployment rate and the transition of beneficiaries between public and private health systems during economic downturns, this research provides valuable insights for strategic planning within the healthcare sector. Similar content being viewed by others![]() COVID-19 forecasts via stock market indicators![]() Counterfactual time series analysis of short-term change in air pollution following the COVID-19 state of emergency in the United States![]() Global short-term forecasting of COVID-19 casesIntroduction. Research into future forecasting and evaluations of high-performing models remains pivotal in academic literature, with extensive studies underscoring their critical role (Makridakis et al., 1998 ; Armstrong, 2006 ; Magazzino et al., 2021 ; Latruwe et al., 2023 ). These quantitative models have been academically intriguing and posed practical implementation and validation challenges across various organizations, governments, and researchers (Makridakis et al., 2021 ; Witkowski et al., 2022 ). Despite these advancements, a notable gap persists in empirical studies that specifically focus on quantitative models for forecasting economic crises, especially those significantly impacting global financial and health systems (Hessel et al., 2014 ; Amarante et al., 2018 ; Morikawa, 2022 ; Ding et al., 2022 ). Understanding the nexus between economic adversity and health infrastructure is imperative, as periods of economic downturn exert significant pressure on nations’ health systems (Su et al., 2023 ; Veiga et al., 2023 ). The implications of such crises are profound, affecting public and private health systems alike, with wide-reaching effects on societal structures and individual well-being (Ferreira et al., 2017 ; Jayawardena et al., 2023 ). Economic crises manifest as a spectrum of challenges characterized by declines in GDP, liquidity shortages, inflation fluctuations, rising unemployment, and abrupt market dynamics, all of which have far-reaching societal impacts (Kaplan, 2012 ; Gomez-Gonzalez et al., 2023 ; Souza et al., 2023 ; Abebe et al., 2023 ; Ojekemi et al., 2023 ). These crises are multidimensional processes that affect resource allocation, influencing well-being and quality of life (Brotto and Dewberry, 2016 ). The emerging economies of the BRICS nations, particularly Brazil, face significant challenges in aligning with the sustainable development goals (SDGs), notably SDG 3, which aims to ensure health and well-being for all (Yang et al., 2023 ). Brazil’s experience with multiple economic crises over the past 50 years has left indelible marks on its economy and healthcare system (Ferreira and Mattos, 2014 ; Silva et al., 2022 ; EFIC, 2023 ). Each event has uniquely impacted Brazil’s healthcare landscape, from the Debt Crisis of the 1980s to the recent political and economic crisis of 2015–2016 and the subsequent health and economic upheavals (Valarini and Pohlmann, 2019 ; EFIC, 2023 ). In this backdrop, the Brazilian health system, comprising the public unified health system (SUS) and the private sector, including the supplementary health sector (SSS), has navigated these tumultuous times (Conass, 2011 ; Paim et al., 2011 ; Veiga et al., 2021 ). The economic downturns, particularly the 2019 Unemployment Crisis, led to significant shifts in beneficiary patterns between the SSS and SUS, influenced by the population’s changing purchasing power (Ferreira and Mattos, 2014 ; Silva et al., 2022 ; EFIC, 2023 ). The onset of the global health crisis in 2019 further compounded these challenges, exposing the need for resilient health systems capable of adapting to rapid economic and societal changes (Opas, 2023 ; Dekimpe and Heerde, 2023 ). In this context, predicting health system trends during economic crises becomes paramount. However, few academic studies have been guided on using forecasting models in such times (Kock and Teräsvirta, 2014 ; Edelthalhammer et al., 2016 ; Ding et al., 2022 ). Addressing this critical gap, our article employs the ARIMA model—autoregressive integrated moving average—a versatile tool across various disciplines, to predict health system behaviors during economic crises (Box and Jenkins, 1970 ; Askari and Montazerin, 2015 ). While ARIMA’s mathematical alignment with many exponential smoothing models is well recognized, its specific application in the context of economic crises, particularly in healthcare, needs to be explored (Makridakis et al., 1998 ). This paper contributes significantly by focusing on the healthcare sector and offering valuable stakeholder insights. By employing the ARIMA model in the context of Brazil’s economic crises, we provide a novel perspective on applying linear forecasting models in healthcare during periods of economic turbulence. This research not only highlights the effectiveness of ARIMA but also presents a balanced view of its utility and potential impact in practical scenarios, contributing to a deeper understanding of crisis dynamics in Brazil’s health sector. Forecasting model—ARIMALinear quantitative forecasting models, grounded in time series, operate under a central assumption: past behavioral patterns indicate future trends. This means that the demand observed in a previous period is expected to match that of a corresponding future period (Makridakis et al., 1998 ; Veiga et al., 2014 ). This category includes heuristic models such as time series decomposition, exponential smoothing, the Holt model, the Winters model, the Holt–Winters (HW) model, and integrated autoregressive moving averages (ARIMA). One of the distinct benefits of linear models is that users can understand intricate data relationships. Furthermore, these models stand out for their ease of interpretation and implementation (Chen and Tanuwijaya, 2011 ). Given these factors, this study opted for the ARIMA model, which aligns with most linear models (Makridakis et al., 1998 ). The ARIMA model, which gained traction thanks to Box and Jenkins in 1970 (Box and Jenkins, 1970 ), follows a two-step process: (i) analyzing the time series and (ii) selecting the model that most appropriately represents the data. The ARMA model is suitable for stationary data. However, when dealing with non-stationary data, it’s essential to differentiate the data series, leading to the ARIMA. There are numerous ARIMA variations, with the general non-seasonal model denoted as ARIMA ( p , d , q ): p = “order of the autoregressive part” d = “degree of first differencing involved” q = “order of the moving average part” A homogeneous non-stationary time series can often be represented using an ARIMA model of order ( p , d , q ) (Box and Jenkins, 1970 ). The challenge lies in pinpointing the optimal p , d , and q values to best characterize the series. A common approach to this challenge is to study the autocorrelation functions (ACF) and partial autocorrelation functions (PACF) of the given time series (Box and Jenkins, 1970 ; Veiga et al., 2014 ). Initially, one determines the degree of differencing ‘ d ’ needed to achieve stationarity in the series. Once ascertained, the correlation and partial autocorrelation functions help suggest possible values for ‘ p ’ and ‘ q ’ (Pindyck and Rubinfeld, 2004 ). Interestingly, in most practical forecasting scenarios, ‘ p ,’ ‘ d ’, and ‘ q ’ values beyond 0, 1, or 2 are seldom required. This implies that a limited range of values can cater to a broad spectrum of practical applications (Makridakis et al., 1998 ). If, for some reason, a time series cannot be described using a low-order ARIMA model, the task of determining ‘ p ’ and ‘ q ’ necessitates a thorough examination of the autocorrelation and partial autocorrelation functions (Pindyck and Rubinfeld, 2004 ). Re-examining the identification of ARIMA models for economic time seriesAfter estimating the ARIMA model, it’s crucial to re-evaluate the identification to ascertain if the chosen model can be optimized further. One reasonable approach for determining the most effective ARIMA model is to opt for the one with the least sum of squared errors or the highest likelihood value. However, these criteria may only sometimes be reliable. Adding more terms to the model can affect these metrics, even if they don’t improve the model’s quality. Applying a penalty to the likelihood for every added term in the model is advisable to address this issue. If incorporating an additional term doesn’t enhance the possibility beyond the penalty, it shouldn’t be included in the model (Box et al., 1994 ; Veiga et al., 2016 ). The most common probability penalty procedure is Akaike’s Information Criterion (AIC), proposed by Akaike in 1974. Assuming m = p + q + P + Q is the number of terms estimated in the model, it is possible to choose the values of p , q , P , and Q by minimizing AIC, presented in Eq. ( 1 ) (see Akaike, 1974 ): where L stands for the probability. Numerous variations of the AIC are utilized in research; notable among them are the Schwarz–Bayesian information criterion (BIC) and the final predictive error (FPE) (Brockwell and Davis, 1996 ). It is also worth noting that not all software applications generate the AIC or the likelihood value L. In situations where determining the AIC for a specific model is infeasible, an approximation of the AIC can be employed, as illustrated in Eq. ( 2 ) where \({{\sigma }}^{2}\) is the variance of the residual and n is the number of observations in series. It is important to emphasize that the AIC has little meaning by itself. It is only valid when comparing other models fitted to the same dataset. While the chosen model might appear optimal among those considered, a final validation is crucial. This is achieved by examining the model residuals to ensure no unaccounted patterns remain. For a well-fitted prediction model, the residuals post-fit should essentially be white noise. To further validate the model fit, one can employ a Portmanteau test, which uses statistics derived from the residual autocorrelation function (Makridakis et al., 1998 ). This study adopts an ex-post-facto approach, analyzing secondary data from the Institute of Applied Economic Research (IPEA) and the National Supplementary Health Agency (ANS). The subsequent sections provide detailed insights into the application process, beginning with implementing the ARIMA model, then sample selection and the experiment. ARIMA model for economic time series dataThe regression model takes the form (see Eq. ( 3 )): where cap Y sub is the predicted variable, cap X sub 1 and cap X sub p are explanatory variables, b sub 0 to b sub p are linear regression coefficients, and \({e}_{t}\) represents the error. If, however, these variables are defined as \({X}_{1}={Y}_{t-1},{X}_{2}={Y}_{t-2},{X}_{3}={Y}_{t-3},\ldots ,{X}_{p}={Y}_{t-p}\) , Eq. ( 3 ) becomes (see Eq. ( 4 )): Equation ( 4 ) still represents a regression equation. Still, it differs from Eq. ( 3 ) as it has different explanatory variables that are, in fact, previous values of the prediction variable \({Y}_{t}\) , called autoregressive (AR). Just as it is possible to regress past values of a series again, there is a time series model that uses past errors as explanatory variables (see Eq. ( 5 )): In Eq. ( 5 ), a dependence relationship is established between successive errors, and the equation is called a moving average (MA) model. Many stationary random processes cannot be modeled purely as moving averages or purely as autoregressive, as they have the qualities of both methods. In this situation, the AR model can be effectively linked to the moving average model to form a common and general class of time series models called the autoregressive moving average (ARMA) model. The ARMA model can only be used on stationary data. In practice, many time series are non-stationary, so the character of the underlying stochastic process changes over time. To extend the use of the ARMA model to non-stationary series, it is necessary to differentiate the data series. In this situation, the model is called autoregressive integrated moving average (ARIMA), popularized by Box and Jenkins in 1970 (Box and Jenkins, 1970 ). It can be said that \({Y}_{t}\) is stationary homogeneous of order d if \({w}_{t}={\Delta }^{d}{Y}_{t}\) is a stationary series. Considering that Δ denotes differentiation (see Eqs. ( 6a ) and ( 6b ): With a \({w}_{t}\) series, it is possible to return to \({Y}_{t}\) by summing \({w}_{t}\) a total of d times. This can be written as \({Y}_{t}={\Sigma }^{d}{w}_{t}\) , onde ∑ where is the summation operator (see Eqs. ( 7a ) and ( 7b )): It is worth noting that the summation operator ∑ is merely the inverse of the difference operator Δ. As \(\Delta {Y}_{t}={Y}_{t}-{Y}_{t-1}\) , it can be written that \(\Delta =1-B\) and thus \(\sum ={\Delta }^{-1}={(1-B)}^{-1}\) . When calculating this sum for a practical series, the first observation of the original series is started without differentiating ( \({Y}_{0}\) ), and then successive values of the series in difference are added. This way, if \({w}_{t}=\Delta {Y}_{t}\) , \({Y}_{t}\) can be calculated (see Eq. 8 ): If \({Y}_{t}\) were differentiated twice, such that \({w}_{t}={\Delta }^{2}Y\) , it would be possible to calculate \({Y}_{t}\) from \({w}_{t}\) by summing this term twice. After differentiating the series \({Y}_{t}\) to produce the stationary series \({w}_{t}\) , it is possible to model \({w}_{t}\) as an ARMA process. If \({\rm{w}}\_{w}_{t}={\Delta }^{d}{Y}_{t}\) is an ARMA( p,q ) process. It can be said that \({Y}_{t}\) is an integrated autoregressive and moving average process of order ( p,d,q ) or simply ARIMA( p,d, q ). If Y \({Y}_{t}=\sum {w}_{i}\) grows linearly over time, the series has a linear trend in time independent of random disturbances, which is deterministic. Model specification: ARIMATo apply the ARIMA model in forecasting, it is necessary to expand its equation to make it a conventional regression equation; that is, for the ARIMA model (0,1,1) (0,1,1)12, there is the Eq. 9 : To use Eq. ( 9 ) in forecasting a period ( Y _( t −1)), shown in Eq. ( 10 ): In Eq. ( 10 ), the term \({{e}}_{{t}+1}\) will not be known because the expected value of future random errors must be taken as zero, but from the adjusted model; it is possible to substitute the values \({{e}}_{{t}}\) , \({{e}}_{{t}-11}\) , and \({{e}}_{{t}-12}\) by their determined empirical values, which are the residuals for times t , t −11 and t −12, respectively. For forward predictions, there will be no empirical value for the ‘ e ’ term after a while, so the expected values will be considered zero. Regarding the values Y , starts a forecasting process, the values are known \({{Y}}_{{t}}\) , \({{Y}}_{{t}-11}\) and \({{Y}}_{{t}-12}\) . After a while, however, the Y value in Eq. ( 10 ) will be a predicted value, not known from past values. For large prediction intervals, it is usual to use computational packages. After identifying the ARIMA model, the maximum-likelihood estimation (MLE) method was selected, which seeks parameters that maximize the likelihood function. To apply the model in prediction, it is necessary to expand its equation to make it a conventional regression equation; for the ARIMA model (0,1,1) (0,1,1), we have Eq. ( 9 ). To use Eq. ( 10 ) in forecasting one period ( \({{Y}}_{{t}-1}\) ). As the coefficients \({\phi }_{1}\) , \({\Phi }_{1}\) , \({\theta }_{1}\) , and \({\Theta }_{1}\) are estimated from the data; Eq. ( 9 ) can be used for prediction. Notably, for clarity in Eq. ( 10 ), the constant term needs to be included. However, where μ represents the average of the Y values, the constant term should reappear on the right-hand side of Eqs. ( 9 ) and ( 10 ) (see variables 12 and 13). Equation (12), the term \({{e}}_{{t}+1}\) will not be known because the expected value of future random errors must be taken as zero, but from the fitted model, it is possible to substitute the values \({{e}}_{{t}}\) , \({{e}}_{{t}-11}\) , and \({{e}}_{{t}-12}\) by their determined empirical values, which are the residuals for the times t , t −11, and t −12, respectively. For forward predictions, there will be no empirical value for the term, and after a while, the expected values will be considered zero. Regarding the Y values, when starting a forecasting process, the \({{Y}}_{{t}}\) , \({{Y}}_{{t}-11}\) and \({{Y}}_{{t}-12}\) . Values are known. After a while, however, the Y value in Eq. ( 10 ) will be a predicted value, not known from past values. For large prediction intervals, it is usual to use computational packages. The essential elements of AR and MA models can be combined to produce various models. The ARIMA ( p , d , q ) model is obtained if a non-stationary series is added to the ARMA model. Equation ( 11 ) represents the simplest case of an ARIMA (1,1,1) model: where \(\left(1-{\phi }_{1}B\right)\) represents the AR(1) part, \(\left(1-B\right){Y}_{t}\) represents the first difference and \(+(1-{\theta }_{1}B){e}_{t}\) refers to the MA(1) portion (Pindyck and Rubinfeld, 2004 ). Sample selection—economics time seriesTo achieve the proposed objective, the following economic variables were selected (Veiga et al. 2023 ): GPD—gross domestic product—is an economic indicator that quantifies the market value of all final goods and services produced within a country’s borders each time, serving as a comprehensive measure of its overall economic activity. IPCA : Extended national consumer price index—is a primary inflation indicator in Brazil. It measures the price change of a diverse set of products and services consumed by households with a specific income range, reflecting the country’s cost of living and economic trends. Unemployment rate : The unemployment rate is a vital economic indicator representing the percentage of the jobless labor force actively seeking employment. This rate is critical for assessing an economy’s health, indicating job availability, and influencing economic policy decisions. Total number of beneficiaries of health plans : Refers to the aggregate count of individuals covered under health insurance policies. This figure encompasses those enrolled in various types of health coverage, including employer-provided plans, private health insurance, government-sponsored programs, and other health care plans, reflecting the extent of health insurance penetration in a population. Total number of individual health plan beneficiaries : This refers to the number of people with health insurance policies, distinct from group or employer-provided plans. It includes those who purchase and maintain their health insurance, typically through private health insurers or individual plans offered by government programs. The analyzed variables were chosen based on their significance within the Brazilian economic landscape and their health implications, as Veiga et al. ( 2023 ) discussed. The 20 years, from 2000 to 2020, was chosen because it encompasses the most significant crises that Brazil has faced in the last two decades. More specifically, this selection considered the unemployment crisis of 2019. During this period, a decrease in purchasing power was recorded, which led thousands of people to transition from SSS to SUS. The variables data can be accessed on the Institute of Applied Economic Research’s website, IPEA, at http://www.ipeadata.gov.br/ . As for the supplementary health sector, the data encompasses individual and collective health plans, including their variations. These datasets are available on the official website of the sector’s regulatory body, the National Supplementary Health Agency (ANS), at http://www.ans.gov.br/ . The Brazilian market is chosen due to its varied economic crises, a complex healthcare system blending public and private sectors, and a rich dataset for time series analysis, with findings applicable to similar emerging economies and addressing a gap in the literature on economic crises’ impact on healthcare. Experiment modelingTo achieve the proposed objective, the ARIMA model was applied to forecast the selected time series over 15 years (2000–2015). For greater accuracy, the model was adjusted for 10 years (2000–2010) to predict the next 5 years (2011–2015) to validate the best fit and adherence of the technique to the time series. After validation, a new forecast was made for the next 5 years (2016–2020). Predicting data behavior represents one of the leading research efforts in time series forecasting. Many theoretical and heuristic models have been developed in the last decades. Faced with different possibilities of forecasting models, it is necessary to identify, by performance indices, which methodology is most appropriate for each case. A better-performing model should have a mean error of approximately zero and a low standard residual mistake (Delurgio, 1998 ). In this sense, for validation and adherence of the ARIMA model, the mean percentage absolute error—MAPE (Eq. (7)) and the U-Theil (Eq. (8)) metrics were used, based on the Autoregressive ( p ), Integrated ( d ) values of Means Furniture ( q ) that models the ARIMA (p , d, q ). Next, the metrics to evaluate the forecast error are described. Percent mean absolute error (MAPE): the mean absolute error as a percentage of demand (Chopra and Meindl, 2003 ). In practice, a MAPE value of less than 10% can suggest potentially perfect predictions, <20% potentially good, and above 30% potentially inaccurate (Lewis, 1997 ). MAPE can be expressed as follows, as shown in Eq. ( 13 ): where \({{\rm{|E}}}_{{\rm{t}}}{\rm{|}}=\) absolute value of the error in period t ; \({\rm{|}}{{\rm{D}}}_{{\rm{t}}}{\rm{|}}=\) absolute value of real demand in period t ; \({\rm{n}}=\) all the periods. U-Theil is a relative percentage measure of the one-step-ahead discrepancies committed with the forecast (Theil, 1966 ). It is also called the coefficient of inequality. U-Theil is decisive in determining whether to use a forecasting technique. This model evaluates the fit of the predicted series to the original series. The closer to zero, the greater the adjustment of the predicted series about the original series. On the other hand, values more relative to unity indicate that the model did not make good predictions. The U-Theil is expressed in Eq. ( 14 ): where \({{y}}_{{l}}^{* }\) is the predicted value for the period t , \({{y}}_{{l}}\) is the observed value and N is the number of observations. Results and discussionAll parameters were selected to minimize the MAPE and U-Theil values throughout adjusting, applying, modeling, and training the model. The ARIMA model was implemented using the GRETL® computational platform, accessible at https://gretl.sourceforge.net . This study analyzed five sets of economic time series (15 time series) to understand the performance of the SUS and SSS health systems. It mainly focused on the economic downturns impacting Brazil from 2010 to 2020, emphasizing the 2019 unemployment crisis (see research structure incorporating the ARIMA Model in Fig. 1 ). ![]() Research structure incorporating the ARIMA Model]. Any homogeneous non-stationary time series can be represented by an ARIMA process of the order ( p , d , q ). The challenge lies in selecting the optimal values for p , d , and q to define the ARIMA model effectively. This is addressed, in part, by scrutinizing both the FAC and the FACP of the time series in question. The initial step involves determining the differentiation degree, denoted as ‘ d ’, required to render the series stationary. Potential model specifications are deduced by assessing the FAC (representing ‘ p ’) and FACP (indicating ‘ q ’). The optimal adjustment values for p , d , and q , as showcased in Tables 1 and 2 , were chosen based on the minimum values of the MAPE and U-Theil errors. The ARIMA model’s forecasting results for the variables analyzed are depicted in Figs. 2 – 6 and Table 2 . Detailed model validations are provided in Appendix 1 . As presented in Fig. 2 and Table 2 , the forecasting errors, as indicated by a MAPE of 9.06 and a U-Theil value of 0.617, validate a high level of precision when juxtaposed against the original series. ![]() Forecast made for the GDP in the period from 2016 to 2020. ![]() Forecast made for the IPCA rate in the period from 2016 to 2020. ![]() Forecast made for the unemployment rate from 2016 to 2020. ![]() Forecast made for the total plan beneficiaries’ series for the period from 2016 to 2020. ![]() Forecast made for the series of individual plan beneficiaries for the period from 2016 to 2020. Figure 2 visually captures the model’s alignment based on FAC and FACP evaluations, using a 5% significance boundary for residual analysis. The model confidently reached a minimum of 95% prediction accuracy, which is distinctly emphasized in green in Fig. 2 . The model underwent validation from 2000 to 2010 to facilitate a comparative analysis with the real series from 2010 to 2015. These results pave the way for GDP prediction utilizing the ARIMA (1, 0, 2) model. To corroborate the model’s fit, the entire series from 2000 to 2015 was considered, enabling the creation of a fresh forecast for the subsequent five years, specifically from 2016 to 2020. In Fig. 3 , the forecast errors, characterized by a MAPE value of 15.04 and a U-Theil value of 0.167 (detailed in Table 2 ), reflect a commendable degree of precision when contrasted with the original data series. This figure visualizes the model’s alignment based on the FAC and FACP evaluations, adhering to a 5% significance threshold for residual scrutiny. Impressively, the model consistently achieves a minimum of 95% prediction accuracy, a feat distinctly emphasized by the green highlight within the figure. For validation, the model was applied to the timeframe spanning from 2000 to 2010, which served as a basis for comparison with the real-time series from 2010 to 2015. The outcomes suggest employing the ARIMA (2, 2, 1) model for predicting IPCA values. To corroborate this fit, the entire series from 2000 to 2015 was utilized, setting the stage for a fresh forecast covering the subsequent half-decade, specifically 2016 to 2020. Figure 4 showcases the forecast errors, evidenced by a MAPE value of 4.14 and a U-Theil score of 0.031 (refer to Table 2 ), indicating a remarkable level of accuracy when juxtaposed against the actual unemployment rate series. This figure elucidates the model’s calibration based on the FAC and FACP, employing a 5% significance threshold for the residual assessment. Notably, the model achieves a minimum of 95% in prediction accuracy, a detail accentuated by the green shading in Fig. 4 . When applied to data from 2000 to 2010 for validation, the model’s comparison with the real-time series spanning 2010 to 2015 boasted a striking forecast accuracy of 95.86%. This suggests the feasibility of predicting the unemployment rate, especially during economic downturns. To further validate this model, the comprehensive series from 2000 to 2015 was harnessed to craft a forecast for the subsequent half-decade, explicitly focusing on the unemployment rate from 2016 to 2020. Though there wasn’t an available series for 2016–2020 to validate the model, the results still imply the potential for precise forecasting of future unemployment rate sequences using the ARIMA (0, 1, 2) model. In both Fig. 5 and Table 2 , forecast errors as gauged by MAPE at 1.27 and U-Theil at 0.0069 underscore a significant level of accuracy when juxtaposed with the actual series for ‘Total Beneficiaries.’ Figure 5 further elaborates on the calibration of the model, based on FAC and FACP, set within a 5% significance parameter for the residual analysis. Impressively, the model aligns within the anticipated accuracy threshold, reaching at least a 95% accuracy, a detail emphasized by the green shade in Fig. 5 . When we juxtapose the model’s predictions from 2000 to 2010 with the actual series from 2010 to 2015, we find a stellar forecast accuracy of 98.93%, as indicated by the MAPE. This suggests the model’s adeptness at precisely forecasting the Total Beneficiaries count under health plans. Further, to ascertain the model’s adjustment, the entire series from 2000 to 2015 was employed to devise a forecast spanning the subsequent half-decade (2016–2020). While data for the 2016–2020 timeframe wasn’t available for direct model validation, literature on forecasting metrics (Makridakis et al., 1998 ) supports the hypothesis that the ARIMA (1, 1, 2) approach can accurately predict future series for the total number of health plan beneficiaries. Figure 6 showcases forecast errors as indicated by a MAPE of 2.89 and a U-Theil of 0.577, reflecting a remarkable accuracy compared to the real series for Individual health plan beneficiaries. The adjustments made to the model, grounded in the FAC and FACP parameters, are illustrated within a 5% significance threshold for residual analysis. The model’s predictions adhere within a minimum 95% accuracy bracket, an aspect emphasized by the green highlight in Fig. 6 . Based on data from 2000 to 2010 and compared with the real series spanning 2010 to 2015, the model’s forecast achieved an accuracy of 97.11%. Such results underscore the potential of the ARIMA (2, 2, 1) method in precisely forecasting the number of individual health plan beneficiaries. To further validate the model’s adjustments, the entire series from 2000 to 2015 was used as a foundation to project the subsequent five years (2016–2020) concerning individual health plan beneficiaries. Although data were absent for the 2016–2020 timeframe for direct model validation, the existing literature and results instill confidence in the ARIMA (2, 2, 1) method’s ability to forecast the future series for individual health plan beneficiaries accurately. Table 3 displays the data variables discussed in this article, which are visually represented in Figs. 2 – 6 . For the upcoming period, from 2016 to 2020, the results derived from applying the ARIMA model are shown for the variables GDP, IPCA, Unemployment rate, Total Beneficiaries of health plans, and Individual Health Plan Beneficiaries. Alongside these predictions, percentage variations for each series have been calculated. It’s crucial to understand the methodology applied in the forecasting. Specifically, data from 2000 to 2010 was utilized to calibrate the model. The subsequent period, 2011 to 2015, was the validation phase, where the ARIMA model’s predictions were matched against real-world data. Finally, predictions from 2016 to 2020 were generated using the ARIMA model, thereby providing forecasts for the five economic indicators under study. Table 3 underscores the critical role of forecasting economic series in facilitating precise budgetary planning within the public and private healthcare sectors, especially in Brazil. An analysis of fundamental economic factors that may impact forecast values is presented. GDP : The data on GDP in Table 3 provides valuable insights into Brazil’s economic trajectory. In 2020, Brazil experienced a 3.3% contraction in its GDP, indicating economic challenges. Despite this contraction, the GDP remained substantial at R$ 7.6 trillion (Brazilian currency) (IBGE, 2022 ). A growing GDP typically signals a healthy economic environment, while a decline often signifies a recession. Economic downturns can significantly affect healthcare systems, particularly the SUS and SSS, as they may face increased demand for services due to economic hardships. The ability to forecast GDP trends, as demonstrated by the ARIMA model, is crucial for governments, organizations, and businesses in making strategic decisions during economic fluctuations. IPCA : The IPCA time series measures inflationary trends by evaluating the prices of goods and services over specific periods. Changes in IPCA directly impact household consumption patterns and can erode purchasing power. When purchasing power declines, individuals may shift from private healthcare plans (SSS) to the public healthcare system (SUS), especially during high unemployment. This transition places additional strain on the SUS infrastructure. The ARIMA model’s beneficiary count predictions align with ANS data for 2023, which indicates over 50 million exclusive SSS medical aid beneficiaries, constituting ~26% of Brazil’s population. Despite economic challenges, such as the 2019 unemployment spike and the COVID-19 pandemic, the SSS has shown resilience and growth (Veiga et al., 2023 ). The sustainability of the SSS and its continuous growth amid economic adversities reflect the complex dynamics within the Brazilian healthcare system. Sustainability of Health Plan Operators (OPSs): The Brazilian private healthcare sector, with over 700 active Health Plan Operators (OPSs) and nearly 20,000 distinct health schemes, is a significant player in healthcare preferences, ranking third among consumer priorities, trailing only home ownership and formal education (IESS, 2021 ). The OPSs have grappled with escalating healthcare expenses and sought strategies for cost containment, sometimes affecting access to specific healthcare resources. The pursuit of sustainability and the pressure on state governance by OPS decision-makers date back to the regulatory inception of the private healthcare sector in the 1990s. However, the COVID-19 pandemic has heightened the urgency for OPSs to adapt to regulatory changes and evolving beneficiary behaviors. Integrating predictive models like ARIMA into strategic planning has become indispensable in providing decision-makers with crucial insights, especially during challenging times when fiscal prudence is essential for the sector’s longevity (Machado, 2023 ). Forecasting economic factors using models like ARIMA is vital for healthcare stakeholders in Brazil, as it allows them to anticipate and respond to economic fluctuations and their impact on healthcare utilization and sustainability. The ARIMA model’s predictions offer valuable insights for informed decision-making in the public and private healthcare sectors. Table 4 presents the descriptive analysis based on the economic variables, also presented in Table 3 , which brings the results of applying the ARIMA model. The descriptive analysis shows that the GDP data over 15 years shows a mean growth of ~1.67%, with a significant standard deviation of 2.76%. This suggests variability in economic performance. The range of GDP growth extends from a low of −3.80% to a high of 6.50%, indicating some years of economic contraction and others of robust growth. The distribution is slightly skewed to the left (−0.17), suggesting a few years with meager growth rates. The average IPCA over the years is 6.83%, with a standard deviation of 2.55%, indicating fluctuating inflation rates. The maximum inflation rate observed is 12.53%, possibly due to specific economic conditions in that year. A positive skew (0.94) suggests that inflation rates are typically lower, with occasional years of high inflation. The mean unemployment rate is 8.43%, with relatively less variability (standard deviation of 1.33%). The range is between 6.70% and 10.48%, indicating a moderate fluctuation in unemployment levels. The distribution of the unemployment rate is nearly symmetric (skewness of 0.12), with a slight tendency towards higher rates. For the Total Beneficiaries of Health Plans, this figure has a significant mean of ~40 million, with a standard deviation of around 7.45 million, suggesting a substantial change over the years. The range from 30.97 million to 50.50 million indicates a significant increase in health plan beneficiaries. The distribution is close to symmetric, with a slight positive skew (0.08) and a kurtosis indicating a distribution like a normal distribution. To Individual Health Plan Beneficiaries, the mean of 67.57 million with an exceptionally high standard deviation (220.77 million) suggests a highly variable number of individual beneficiaries, possibly influenced by outliers. The skewness (3.74) and kurtosis (14.00) are significantly high, indicating a distribution with a pronounced tail and a peak different from the normal distribution, likely due to one or more outlier years. In Collective Health Plan Beneficiaries, the average of around 31.64 million beneficiaries with a standard deviation of 6.32 million shows variability but is less extreme than individual beneficiaries. The distribution has a slight positive skew (0.20) and a kurtosis-like normal distribution, suggesting a relatively regular distribution of data points around the mean. The dataset reflects significant variability in economic indicators (GDP and IPCA) and healthcare plan beneficiaries over the years. The high standard deviation and skewness in the health plan beneficiary data, especially for individual plans, hint at substantial year-to-year changes, possibly driven by policy changes, market dynamics, or other factors. The unemployment rate shows less variability compared to other economic indicators, suggesting a more stable but persistent issue in the job market. This paper investigated applying the ARIMA model to predict five critical economic time series that exerted substantial influence on Brazil’s public and private healthcare sectors throughout the economic crisis spanning from 2000 to 2020. These time series encompassed (i) GDP, (ii) IPCA, (iii) the unemployment rate, (iv) the total number of health plan beneficiaries, and (v) individual health plan beneficiaries. The ARIMA model was selected due to its proven statistical rigor, adeptly blending theoretical and empirical frameworks in the literature. Our analysis emphatically highlights the prowess of ARIMA in predicting vital economic time series for the health sector. As described in academic literature, predictions were measured against esteemed MAPE and U-Theil benchmarks. The specified orders of our ARIMA model—(1, 0, 2), (2, 2, 1), (0, 1, 2), (1, 1, 2), and (2, 2, 1)—yielded accurate forecasts, as showcased in Table 1 and further validated by Table 2 metrics. Of note was ARIMA’s aptitude in predicting the unemployment rate and total and individual beneficiaries, which are crucial metrics for the health sector. For example, the 2019 economic downturn led to a considerable population experiencing job losses and reduced purchasing power, prompting a shift from supplementary to public health systems. Forecasting such indices provides invaluable insights for strategic planning. Our results bolster the assertion that ARIMA can adeptly anticipate economic indicators influencing Brazil’s health domain, notably the SSS and SUS systems. The model’s remarkable accuracy, exceeding 95%, and simplicity and ease of implementation accentuate its value. Refining the ARIMA parameters can enhance its efficacy, especially within specific sectors like SSS and SUS. However, potential pitfalls warrant attention. Parameter estimations inherently possess uncertainty, and the model may not fully capture this. Furthermore, the assumption that historical data patterns persist might falter during crises. If ARIMA aligns impeccably with reality, it is a formidable prediction tool; deviations might lead to subpar results. Many studies in the literature highlight ARIMA’s competitive accuracy. Still, a universally superior forecasting model remains contested. As best practices suggest, optimal models are economical, user-friendly, and cost-effective. While this study illuminates this subject, its complexity demands further probing. The goal was a partial assessment of ARIMA across every economic downturn in Brazil. Evaluating non-linear AI-driven models alongside Fuzzy Logic presents a promising research trajectory for future research. Analyzing significant global phenomena, such as the COVID-19 pandemic, remains imperative. This paper offers a novel contribution by filling a literature void on forecast models tailored to empirical scenarios, offering pivotal insights for specific sectors, like health. The findings will support health sector stakeholders by championing a versatile model like ARIMA. The research stands out for its pioneering use of a linear forecasting model during a crisis, providing implications for Brazil’s health domain. This methodology has broader implications, showcasing its adaptability across various domains. ARIMA is a prevalent choice for time series forecasting across diverse fields. The literature consistently emphasizes that every model is only sometimes perfect. Like others, the ARIMA model possesses both merits and limitations. ARIMA’s primary strength is its malleability. Parameters ( p , d , q ) can be adjusted to fit diverse time series, and their transparency offers a clear statistical understanding of data interrelationships. It is also notably efficient, with many studies praising its forecasting precision for compliant series. ARIMA’s resilience to disturbances makes it apt for volatile series, and its flexibility finds applications in sectors from finance to meteorology. However, challenges persist. ARIMA requires a stationary series, and discerning optimal parameters often requires rigorous testing. Recognizing ARIMA’s potential limitations when facing abrupt data shifts is vital. While ARIMA is proficient at short-term predictions, its precision may diminish over extended periods, although specific authors in the literature acknowledge its potential for long-term forecasting. Given its linear nature, ARIMA may overlook external influences on a time series. To address this limitation, models like autoregressive integrated moving average with exogenous variables (ARIMAX) can be employed. When evaluating the suitability of ARIMA, it is crucial to balance its advantages and limitations. This topic is extensive, and while our article sheds light, further exploration remains paramount, especially within the health sector. While the study focuses on Brazil, the findings have broader implications. The insights gained can be applied to other emerging economies with similar healthcare structures and economic challenges. Thus, the study contributes to the understanding of not only Brazil’s healthcare system but also a broader global context. Policy implications(i) The accurate forecasts ARIMA provides can aid healthcare managers and policymakers in strategic planning, especially during economic downturns. This can lead to better resource allocation and preparedness for shifts in public health demand. (ii) For healthcare systems, especially in emerging economies like Brazil, ARIMA’s predictions can inform adjustments in service provision in response to economic changes, such as shifts in unemployment rates and health plan beneficiaries. Future research avenues(i) Evaluating non-linear and AI-driven models: There is a promising avenue for comparing the performance of non-linear models, including AI-driven approaches and fuzzy logic, with ARIMA in forecasting complex economic behaviors. (ii) Future research should extend to analyzing the impacts of significant global events, like the COVID-19 pandemic, employing ARIMA and other models to understand and manage healthcare systems during such crises. (iii) Expanding empirical studies in healthcare forecasting: More empirical research is needed on ARIMA and other models for specific healthcare sector challenges, particularly during economic crises. (iv) Further exploration into balancing ARIMA’s advantages, such as its adaptability and efficiency, with its limitations in handling abrupt data shifts and long-term forecasting, is necessary. This study makes a novel contribution by addressing a gap in the literature on forecast models tailored to specific empirical scenarios and offers vital insights for sectors like healthcare. With its merits and limitations, the ARIMA model is a versatile tool for forecasting time series across various domains. This research highlights the model’s effectiveness during economic crises and opens doors for further exploration within the health sector and beyond. Data availabilityThe data sets generated and analysed during the current study are available upon request. Abebe A, Temesgen A, Kebede B (2023) Modeling inflation rate factors on present consumption price index in Ethiopia: threshold autoregressive models approach. Future Bus J 9:72. https://doi.org/10.1186/s43093-023-00241-0 Article Google Scholar Akaike H (1974) Stochastic theory of minimal realization. In IEEE Transactions on Automatic Control 19:667–674. https://doi.org/10.1109/TAC.1974.1100707 Amarante JGMCdaC, Bach TM, da Silva WV, Matiollo D, Souza A, da Veiga CP (2018) Econometric analysis of cointegration and causality between markets prices toward futures contracts: evidence from the live cattle market in Brazil. Cogent Bus Manag 5(1). https://doi.org/10.1080/23311975.2018.1457861 Armstrong JC (2006) Findings from evidence-based forecasting: methods for reducing forecast error. Int J Forecast 22(3):583–598. https://doi.org/10.1016/j.ijforecast.2006.04.006 Askari S, Montazerin N (2015) A high-order multi-variable fuzzy time series forecasting algorithm based on fuzzy clustering. Expert Syst Appl 42(4):2121–2135. https://doi.org/10.1016/j.eswa.2014.09.036 Box GEP, Jenkins GM (1970) Time series analysis: forecasting and control. Holden-Day Box GEP, Jenkins GM, Reinesl GC (1994) Time series analysis: forecasting and control, 3rd edn. Prentice-Hall Brockwell PJ, Davis RA (1996) Introduction to Time Series and Forecasting. Springer. https://doi.org/10.1007/978-3-319-29854-2 Brotto VC, Dewberry E(2016) Economic crisis and social learning for the provision of public services in two Spanish municipalities J Clean Prod 112(4):3018–3027 10.1016/j.jclepro.2015.09.136 Chen S-M, Tanuwijaya K (2011) Multivariate fuzzy forecasting based on fuzzy times series and automatic clustering techniques. Expert Syst Appl 38:10594–10605. https://doi.org/10.1016/j.eswa.2011.02.098 Chopra S, Meindl P (2003) Gerenciamento da cadeia de suprimentos: estratégia, planejamento e operações. Pearson Prentice-Hall CONASS—Conselho Nacional De Secretários De Saúde (2011) Saúde Suplementar: Coleção para entender a gestão do SUS, 1st edn., vol. 12. https://www.conass.org.br/biblioteca/ccount/click.php?id=43 Dekimpe MG, Heerde HJV (2023) Retailing in times of soaring inflation: what we know, what we don’t know, and a research agenda. J Retail https://doi.org/10.1016/j.jretai.2023.07.002 Delurgio SA (1998) Forecasting: principles and applications. Irwin McGraw-Hill Ding S, Tao Z, Hu J (2022) Forecasting the economic indices of the high-tech industries in China using the grey multivariable convolution model. Appl Soft Comput. 126:109301. https://doi.org/10.1016/j.asoc.2022.109301 Edelthalhammer F, Ismail I, Volkenand J (2016) Forecasting economic crises: an empirical approach. https://www.econ.uni-muenchen.de/download/lehre-at-lmu/forecasting.pdf . Accessed 29 Aug 2023 EFIC (2023) Quais as principais crises que afetaram mercado Brasileiro. https://efic.com.br/crises-mercado-brasileiro/ . Accessed 17 July 2023 Ferreira DM, Mattos LB (2014) The contagion effect of the subprime crisis in the Brazilian Stock Market. Procedia Econ Financ 14:191–200. https://doi.org/10.1016/S2212-5671(14)00702-3 Ferreira VL, Veiga CRP, Kudlawicz-Franco C, Scalercio P, Ramires Y, Pontarolo R, Carvalho DMW, Veiga CP (2017) Generic drugs in times of economic crisis: are there changes in consumer purchase intention? J Retail Consum Serv 37:1–7. https://doi.org/10.1016/j.jretconser.2017.02.008 Gomez-Gonzalez JW, Uribe JM, Valencia OM (2023) Does economic complexity reduce the probability of a fiscal crisis? World Dev 168. https://doi.org/10.1016/j.worlddev.2023.106250 Hessel P, Vandoros S, Avendano M (2014) The differential impact of the financial crisis on health in Ireland and Greece: a quasi-experimental approach. Public Health 128:911–919. https://doi.org/10.1016/j.puhe.2014.08.004 Article CAS PubMed Google Scholar IBGE—Instituto Brasileiro de Geografia e Estatística (2016a) Agencia IBGE notícias: Em fevereiro, IPCA-15 fica em 1,42%. http://saladeimprensa.ibge.gov.br/noticias.html?view=noticia&id=1&idnoticia=3105&busca=1&t=fevereiro-ipca15-fica-1-42 . Accessed 3 Mar 2016 IBGE—Instituto Brasileiro de Geografia e Estatística (2015) Contas trimestrais: indicadores de volume e valores correntes. ftp://ftp.ibge.gov.br/Contas_Nacionais/Contas_Nacionais_Trimestrais/Fasciculo_Indicadores_IBGE/pib-vol-val_201503caderno.pdf . Accessed 19 Jan 2016 IBGE—Instituto Brasileiro de Geografia e Estatística (2016b). Agência IBGE de notícias: Em janeiro, taxa de desocupação vai a 7,6%. https://agenciadenoticias.ibge.gov.br/agencia-sala-de-imprensa/2013-agencia-de-noticias/releases/10094-em-janeiro-taxa-de-desocupacao-vai-a-7-6 . Accessed 3 Mar 2016 IBGE—Instituto Brasileiro de Geografia e Estatística (2022) Em 2020, primeiro ano da pandemia, PIB recua em 24 das 27 UFs. https://agenciadenoticias.ibge.gov.br/agencia-noticias/2012-agencia-de-noticias/noticias/35501-em-2020-primeiro-ano-da-pandemia-pib-recua-em-24-das-27-ufs#:~:text=Em%202020%2C%20o%20Produto%20Interno,3%2C3%25%20em%20volume . Accessed 28 Aug 2022 IESS—Instituto De Estudos De Saúde Suplementar (2021) Pesquisa quantitativa beneficiários e não beneficiários. https://www.iess.org.br/sites/default/files/2021-07/VoxPopuli_IESS.pdf . Accessed 25 May 2023 Jayawardena R, Kodithuwakku W, Sooriyaarachchi P (2023) The impact of the Sri Lankan economic crisis on medication adherence: an online cross-sectional survey. Dialogues Health 2:100137. https://doi.org/10.1016/j.dialog.2023.100137 Article PubMed PubMed Central Google Scholar Kaplan GA (2012) Economic crises: some thoughts on why, when and where they (might) matter for health—a tale of three countries. Soc Sci Med 74(5):643–646. https://doi.org/10.1016/j.socscimed.2011.12.013 Article PubMed Google Scholar Kock AB, Teräsvirta T (2014) Forecasting performances of three automated modelling techniques during the economic crisis 2007–2009. Int J Forecast 30(3):616–631. https://doi.org/10.1016/j.ijforecast.2013.01.003 Latruwe T, Van der Wee M, Vanleenhove P, Devriese J, Verbrugge S, Colle D (2023) A long-term forecasting and simulation model for strategic planning of hospital bed capacity. Oper Res Health Care 36:100375. https://doi.org/10.1016/j.orhc.2022.100375 Lewis CD (1997) Demand forecasting and inventory control. Wiley, New York, USA Google Scholar Machado R (2023) Planos de saúde esperam mudanças em 2023 para reverter cenário de prejuízo. Futuro da Saúde. https://futurodasaude.com.br/planos-de-saude-2023/ . Accessed 25 May 2023 Magazzino C, Mele M, Morelli G (2021) The relationship between renewable energy and economic growth in a time of Covid-19: a machine learning experiment on the Brazilian economy. Sustainability 13:1285. https://doi.org/10.3390/su13031285 Makridakis S, Fry C, Petropoulos F, Spiliotis E (2021) The future of forecasting competitions: design attributes and principles. INFORMS J Data Sci 1(1):96–113. https://doi.org/10.1287/ijds.2021.0003 Makridakis S, Wheelwright SC, Hyndman RJ (1998) Forecasting: methods and applications, 3rd edn. John Wiley & Sons, USA Morikawa M (2022) Uncertainty in long-term macroeconomic forecasts: ex post evaluation of forecasts by economics researchers. Q Rev Econ Financ 85:8–15. https://doi.org/10.1016/j.qref.2020.10.017 Ojekemi OS, Ağa M, Magazzino C (2023) Towards achieving sustainability in the BRICS economies: the role of renewable energy consumption and economic risk. Energies 16:5287. https://doi.org/10.3390/en16145287 OPAS—Organização Panamericana de Saúde (2023) Histórico da pandemia de COVID-19. https://www.paho.org/pt/covid19/historico-da-pandemia-covid-19 . Accessed 17 July 2023 Paim J, Travassos C, Almeida A, Bahia L, Macinko J (2011) The Brazilian health system: history, advances, and challenges. Lancet 377:1778–1797. https://doi.org/10.1016/S0140-6736(11)60054-8 Pindyck RS, Rubinfeld DL (2004) Econometria: modelos e previsões, 3a. tiragem. Elsevier, Rio de Janeiro Silva TG, Guillén OTC, Morcerf GAN, Modenesi AM (2022) Effects of monetary policy news on financial assets: evidence from Brazil on a bivariate VAR-GARCH model (2006–17). Emerg Mark Rev 52:100916. https://doi.org/10.1016/j.ememar.2022.100916 Souza FM, Ramser CAS, Souza AM, Da Veiga CP (2023) Spillover effects in the presence of structural breaks, persistence, and conditioned heteroscedasticity. Ann Financ Econ 18(2):2250034. https://doi.org/10.1142/S2010495222500348 Stellwagen E, Tashman L (2013) ARIMA: the models of Box and Jenkins. Foresight 30:28–33. Available on https://scholar.google.com/scholar_lookup?title=ARIMA:+The+Models+of+Box+and+Jenkins&author=Stellwagen,+E.Y.&author=Tashman,+L.&publication_year=2013&journal=Foresight+Int.+J.+Appl.+Forecast.&volume=30&pages=28–33 Su Z, Cheshmehzangi A, Mcdonnell D, Bentley BL, Ahmad J, Sangalo S, Da Veiga CP, Xiang Y-T (2023) It was the best of times, it was the worst of times: a tale of two cities—Beijing and Shanghai—why the divergent COVID-19 control outcomes? QJM: Int J Med 116(1):3–5. https://doi.org/10.1093/qjmed/hcac182 Article CAS Google Scholar Theil H (1966) Applied economic forecasting. Rand McNally Valarini E, Pohlmann M (2019) Organizational crime and corruption in Brazil a case study of the “Operation Carwash” court records. Int J Law Crime Justice 59:100340. https://doi.org/10.1016/j.ijlcj.2019.100340 Veiga CP, Veiga CRP, Catapan A, Tortato U, Silva WV (2014) Demand forecasting in food retail: a comparison between the Holt-Winters and ARIMA models. Wseas Trans Bus Econ 11:608–614 Veiga CP, Veiga CRP, Puchalski W, Coelho LS, Tortato U (2016) Demand forecasting based on natural computing approaches applied to the foodstuff retail segment. J Retail Consum Serv 31:174–181. https://doi.org/10.1016/j.jretconser.2016.03.008 Veiga CRP, da Veiga CP, Souza A et al. (2021) Cutaneous melanoma: cost of illness under Brazilian health system perspectives. BMC Health Serv Res 21:284. https://doi.org/10.1186/s12913-021-06246-1 Veiga CRPda, Veiga CPda, Su Z (2023) Supplementary health system in Brazil: business model not threatened by socioeconomic crisis. Int J Prof Bus Rev 8(1):e01225. https://doi.org/10.26668/businessreview/2023.v8i1.1225 Witkowski J, Freeman R, Vaughan JW, Pennock DM, Krause A (2022) Incentive-compatible forecasting competitions. Manag Sci 69(3):1354–1374. https://doi.org/10.1287/mnsc.2022.4410 Yang M, Magazzino C, Awosusi AA, Abdulloev N (2023) Determinants of Load capacity factor in BRICS countries: a panel data analysis. Nat Resour Forum 1–24. https://doi.org/10.1111/1477-8947.12331 Download references AcknowledgementsThe authors wish to express their gratitude to the Editor and reviewers for their kind feedback and constructive input. The author CPdV thanks the National Council for Scientific and Technological Development (CNPq), Brazil, for research grant number 312023/2022–7. Author informationAuthors and affiliations. FDC Business School, Fundação Dom Cabral, Av. Princesa Diana, 760, Nova Lima, Minas Gerais, 34018-006, Brazil Claudimar Pereira da Veiga & Diego Antonio Bittencourt Marconatto Department of Health Management, School of Nursing, Federal University of Minas Gerais (UFMG), 190 Alfredo Balena, Belo Horizonte, Minas Gerais, 30130-100, Brazil Cássia Rita Pereira da Veiga School of Management, Universidade Federal do Paraná (UFPR), Lothário Meissner, 632, Jardim Botânico, Curitiba, Paraná, 80210-170, Brazil Felipe Mendes Girotto School of Public Health, Institute for Human Rights, Southeast University, 210009, Nanjing, China You can also search for this author in PubMed Google Scholar ContributionsCPdV, CRPV, FMG, DABM, and ZS conceived the work, reviewed the literature, and drafted and edited the manuscript. All authors approved the manuscript for submission. Corresponding authorCorrespondence to Claudimar Pereira da Veiga . Ethics declarationsCompeting interests. The authors declare that they have no competing interests. CPdV was, at the time of submission, an Editorial Board Member and Guest Editor for Humanities and Social Sciences Communications . Consent for publicationThe authors have given consent for the publication of this paper. Ethics approvalNot applicable as human participants were not involved. Informed consentAdditional information. Publisher’s note Springer Nature remains neutral with regard to jurisdictional claims in published maps and institutional affiliations. Supplementary informationRights and permissions. Open Access This article is licensed under a Creative Commons Attribution 4.0 International License, which permits use, sharing, adaptation, distribution and reproduction in any medium or format, as long as you give appropriate credit to the original author(s) and the source, provide a link to the Creative Commons licence, and indicate if changes were made. The images or other third party material in this article are included in the article’s Creative Commons licence, unless indicated otherwise in a credit line to the material. If material is not included in the article’s Creative Commons licence and your intended use is not permitted by statutory regulation or exceeds the permitted use, you will need to obtain permission directly from the copyright holder. To view a copy of this licence, visit http://creativecommons.org/licenses/by/4.0/ . Reprints and permissions About this articleCite this article. Pereira da Veiga, C., Pereira da Veiga, C.R., Girotto, F.M. et al. Implementation of the ARIMA model for prediction of economic variables: evidence from the health sector in Brazil. Humanit Soc Sci Commun 11 , 1068 (2024). https://doi.org/10.1057/s41599-024-03023-3 Download citation Received : 28 November 2023 Accepted : 02 April 2024 Published : 22 August 2024 DOI : https://doi.org/10.1057/s41599-024-03023-3 Share this articleAnyone you share the following link with will be able to read this content: Sorry, a shareable link is not currently available for this article. Provided by the Springer Nature SharedIt content-sharing initiative Quick links
![]() ![]() How To Write The Results/Findings ChapterFor qualitative studies (dissertations & theses). By: Jenna Crossley (PhD). Expert Reviewed By: Dr. Eunice Rautenbach | August 2021 So, you’ve collected and analysed your qualitative data, and it’s time to write up your results chapter. But where do you start? In this post, we’ll guide you through the qualitative results chapter (also called the findings chapter), step by step. Overview: Qualitative Results Chapter
What exactly is the results chapter?The results chapter in a dissertation or thesis (or any formal academic research piece) is where you objectively and neutrally present the findings of your qualitative analysis (or analyses if you used multiple qualitative analysis methods ). This chapter can sometimes be combined with the discussion chapter (where you interpret the data and discuss its meaning), depending on your university’s preference. We’ll treat the two chapters as separate, as that’s the most common approach. In contrast to a quantitative results chapter that presents numbers and statistics, a qualitative results chapter presents data primarily in the form of words . But this doesn’t mean that a qualitative study can’t have quantitative elements – you could, for example, present the number of times a theme or topic pops up in your data, depending on the analysis method(s) you adopt. Adding a quantitative element to your study can add some rigour, which strengthens your results by providing more evidence for your claims. This is particularly common when using qualitative content analysis. Keep in mind though that qualitative research aims to achieve depth, richness and identify nuances , so don’t get tunnel vision by focusing on the numbers. They’re just cream on top in a qualitative analysis. So, to recap, the results chapter is where you objectively present the findings of your analysis, without interpreting them (you’ll save that for the discussion chapter). With that out the way, let’s take a look at what you should include in your results chapter. ![]() What should you include in the results chapter?As we’ve mentioned, your qualitative results chapter should purely present and describe your results , not interpret them in relation to the existing literature or your research questions . Any speculations or discussion about the implications of your findings should be reserved for your discussion chapter. In your results chapter, you’ll want to talk about your analysis findings and whether or not they support your hypotheses (if you have any). Naturally, the exact contents of your results chapter will depend on which qualitative analysis method (or methods) you use. For example, if you were to use thematic analysis, you’d detail the themes identified in your analysis, using extracts from the transcripts or text to support your claims. While you do need to present your analysis findings in some detail, you should avoid dumping large amounts of raw data in this chapter. Instead, focus on presenting the key findings and using a handful of select quotes or text extracts to support each finding . The reams of data and analysis can be relegated to your appendices. While it’s tempting to include every last detail you found in your qualitative analysis, it is important to make sure that you report only that which is relevant to your research aims, objectives and research questions . Always keep these three components, as well as your hypotheses (if you have any) front of mind when writing the chapter and use them as a filter to decide what’s relevant and what’s not. Need a helping hand?How do I write the results chapter?Now that we’ve covered the basics, it’s time to look at how to structure your chapter. Broadly speaking, the results chapter needs to contain three core components – the introduction, the body and the concluding summary. Let’s take a look at each of these. Section 1: IntroductionThe first step is to craft a brief introduction to the chapter. This intro is vital as it provides some context for your findings. In your introduction, you should begin by reiterating your problem statement and research questions and highlight the purpose of your research . Make sure that you spell this out for the reader so that the rest of your chapter is well contextualised. The next step is to briefly outline the structure of your results chapter. In other words, explain what’s included in the chapter and what the reader can expect. In the results chapter, you want to tell a story that is coherent, flows logically, and is easy to follow , so make sure that you plan your structure out well and convey that structure (at a high level), so that your reader is well oriented. The introduction section shouldn’t be lengthy. Two or three short paragraphs should be more than adequate. It is merely an introduction and overview, not a summary of the chapter. Pro Tip – To help you structure your chapter, it can be useful to set up an initial draft with (sub)section headings so that you’re able to easily (re)arrange parts of your chapter. This will also help your reader to follow your results and give your chapter some coherence. Be sure to use level-based heading styles (e.g. Heading 1, 2, 3 styles) to help the reader differentiate between levels visually. You can find these options in Word (example below). ![]() Section 2: BodyBefore we get started on what to include in the body of your chapter, it’s vital to remember that a results section should be completely objective and descriptive, not interpretive . So, be careful not to use words such as, “suggests” or “implies”, as these usually accompany some form of interpretation – that’s reserved for your discussion chapter. The structure of your body section is very important , so make sure that you plan it out well. When planning out your qualitative results chapter, create sections and subsections so that you can maintain the flow of the story you’re trying to tell. Be sure to systematically and consistently describe each portion of results. Try to adopt a standardised structure for each portion so that you achieve a high level of consistency throughout the chapter. For qualitative studies, results chapters tend to be structured according to themes , which makes it easier for readers to follow. However, keep in mind that not all results chapters have to be structured in this manner. For example, if you’re conducting a longitudinal study, you may want to structure your chapter chronologically. Similarly, you might structure this chapter based on your theoretical framework . The exact structure of your chapter will depend on the nature of your study , especially your research questions. As you work through the body of your chapter, make sure that you use quotes to substantiate every one of your claims . You can present these quotes in italics to differentiate them from your own words. A general rule of thumb is to use at least two pieces of evidence per claim, and these should be linked directly to your data. Also, remember that you need to include all relevant results , not just the ones that support your assumptions or initial leanings. In addition to including quotes, you can also link your claims to the data by using appendices , which you should reference throughout your text. When you reference, make sure that you include both the name/number of the appendix , as well as the line(s) from which you drew your data. As referencing styles can vary greatly, be sure to look up the appendix referencing conventions of your university’s prescribed style (e.g. APA , Harvard, etc) and keep this consistent throughout your chapter. Section 3: Concluding summaryThe concluding summary is very important because it summarises your key findings and lays the foundation for the discussion chapter . Keep in mind that some readers may skip directly to this section (from the introduction section), so make sure that it can be read and understood well in isolation. In this section, you need to remind the reader of the key findings. That is, the results that directly relate to your research questions and that you will build upon in your discussion chapter. Remember, your reader has digested a lot of information in this chapter, so you need to use this section to remind them of the most important takeaways. Importantly, the concluding summary should not present any new information and should only describe what you’ve already presented in your chapter. Keep it concise – you’re not summarising the whole chapter, just the essentials. Tips for writing an A-grade results chapterNow that you’ve got a clear picture of what the qualitative results chapter is all about, here are some quick tips and reminders to help you craft a high-quality chapter:
If you have any questions, leave a comment below and we’ll do our best to help. If you’d like 1-on-1 help with your results chapter (or any chapter of your dissertation or thesis), check out our private dissertation coaching service here or book a free initial consultation to discuss how we can help you. ![]() Psst... there’s more!This post was based on one of our popular Research Bootcamps . If you're working on a research project, you'll definitely want to check this out ... 22 CommentsThis was extremely helpful. Thanks a lot guys Hi, thanks for the great research support platform created by the gradcoach team! I wanted to ask- While “suggests” or “implies” are interpretive terms, what terms could we use for the results chapter? Could you share some examples of descriptive terms? I think that instead of saying, ‘The data suggested, or The data implied,’ you can say, ‘The Data showed or revealed, or illustrated or outlined’…If interview data, you may say Jane Doe illuminated or elaborated, or Jane Doe described… or Jane Doe expressed or stated. I found this article very useful. Thank you very much for the outstanding work you are doing. What if i have 3 different interviewees answering the same interview questions? Should i then present the results in form of the table with the division on the 3 perspectives or rather give a results in form of the text and highlight who said what? I think this tabular representation of results is a great idea. I am doing it too along with the text. Thanks That was helpful was struggling to separate the discussion from the findings this was very useful, Thank you. Very helpful, I am confident to write my results chapter now. It is so helpful! It is a good job. Thank you very much! Very useful, well explained. Many thanks. Hello, I appreciate the way you provided a supportive comments about qualitative results presenting tips I loved this! It explains everything needed, and it has helped me better organize my thoughts. What words should I not use while writing my results section, other than subjective ones. Thanks a lot, it is really helpful Thank you so much dear, i really appropriate your nice explanations about this. Thank you so much for this! I was wondering if anyone could help with how to prproperly integrate quotations (Excerpts) from interviews in the finding chapter in a qualitative research. Please GradCoach, address this issue and provide examples. what if I’m not doing any interviews myself and all the information is coming from case studies that have already done the research. Very helpful thank you. This was very helpful as I was wondering how to structure this part of my dissertation, to include the quotes… Thanks for this explanation This is very helpful, thanks! I am required to write up my results chapters with the discussion in each of them – any tips and tricks for this strategy? For qualitative studies, can the findings be structured according to the Research questions? Thank you. Do I need to include literature/references in my findings chapter? Submit a Comment Cancel replyYour email address will not be published. Required fields are marked * Save my name, email, and website in this browser for the next time I comment.
Integrations What's new? In-Product Prompts Participant Management Interview Studies Prototype Testing Card Sorting Tree Testing Live Website Testing Automated Reports Templates Gallery Choose from our library of pre-built mazes to copy, customize, and share with your own users Browse all templates Financial Services Tech & Software Product Designers Product Managers User Researchers By use case Concept & Idea Validation Wireframe & Usability Test Content & Copy Testing Feedback & Satisfaction Content Hub Educational resources for product, research and design teams Explore all resources Question Bank Maze Research Success Hub Guides & Reports Help Center Future of User Research Report The Optimal Path Podcast ![]() User Research Aug 19, 2024 • 17 minutes read Qualitative research examples: How to unlock, rich, descriptive insightsQualitative research uncovers in-depth user insights, but what does it look like? Here are seven methods and examples to help you get the data you need. ![]() Armin Tanovic Behind every what, there’s a why . Qualitative research is how you uncover that why. It enables you to connect with users and understand their thoughts, feelings, wants, needs, and pain points. There’s many methods for conducting qualitative research, and many objectives it can help you pursue—you might want to explore ways to improve NPS scores, combat reduced customer retention, or understand (and recreate) the success behind a well-received product. The common thread? All these metrics impact your business, and qualitative research can help investigate and improve that impact. In this article, we’ll take you through seven methods and examples of qualitative research, including when and how to use them. Qualitative UX research made easyConduct qualitative research with Maze, analyze data instantly, and get rich, descriptive insights that drive decision-making. ![]() 7 Qualitative research methods: An overviewThere are various qualitative UX research methods that can help you get in-depth, descriptive insights. Some are suited to specific phases of the design and development process, while others are more task-oriented. Here’s our overview of the most common qualitative research methods. Keep reading for their use cases, and detailed examples of how to conduct them.
1. User interviewsA user interview is a one-on-one conversation between a UX researcher, designer or Product Manager and a target user to understand their thoughts, perspectives, and feelings on a product or service. User interviews are a great way to get non-numerical data on individual experiences with your product, to gain a deeper understanding of user perspectives. Interviews can be structured, semi-structured, or unstructured . Structured interviews follow a strict interview script and can help you get answers to your planned questions, while semi and unstructured interviews are less rigid in their approach and typically lead to more spontaneous, user-centered insights. When to use user interviewsInterviews are ideal when you want to gain an in-depth understanding of your users’ perspectives on your product or service, and why they feel a certain way. Interviews can be used at any stage in the product design and development process, being particularly helpful during:
How to conduct user interviews: The basics
💡 A specialized user interview tool makes interviewing easier. With Maze Interview Studies , you can recruit, host, and analyze interviews all on one platform. User interviews: A qualitative research exampleLet’s say you’ve designed a recruitment platform, called Tech2Talent , that connects employers with tech talent. Before starting the design process, you want to clearly understand the pain points employers experience with existing recruitment tools'. You draft a list of ten questions for a semi-structured interview for 15 different one-on-one interviews. As it’s semi-structured, you don’t expect to ask all the questions—the script serves as more of a guide. One key question in your script is: “Have tech recruitment platforms helped you find the talent you need in the past?” Most respondents answer with a resounding and passionate ‘no’ with one of them expanding: “For our company, it’s been pretty hit or miss honestly. They let just about anyone make a profile and call themselves tech talent. It’s so hard sifting through serious candidates. I can’t see any of their achievements until I invest time setting up an interview.” You begin to notice a pattern in your responses: recruitment tools often lack easily accessible details on talent profiles. You’ve gained contextual feedback on why other recruitment platforms fail to solve user needs. 2. Focus groupsA focus group is a research method that involves gathering a small group of people—around five to ten users—to discuss a specific topic, such as their’ experience with your new product feature. Unlike user interviews, focus groups aim to capture the collective opinion of a wider market segment and encourage discussion among the group. When to use focus groupsYou should use focus groups when you need a deeper understanding of your users’ collective opinions. The dynamic discussion among participants can spark in-depth insights that might not emerge from regular interviews. Focus groups can be used before, during, and after a product launch. They’re ideal:
How to conduct focus group studies: The basics
The number of participants can make it difficult to take notes or do manual transcriptions. We recommend using a transcription or a specialized UX research tool , such as Maze, that can automatically create ready-to-share reports and highlight key user insights. Focus groups: A qualitative research exampleYou’re a UX researcher at FitMe , a fitness app that creates customized daily workouts for gym-goers. Unlike many other apps, FitMe takes into account the previous day’s workout and aims to create one that allows users to effectively rest different muscles. However, FitMe has an issue. Users are generating workouts but not completing them. They’re accessing the app, taking the necessary steps to get a workout for the day, but quitting at the last hurdle. Time to talk to users. You organize a focus group to get to the root of the drop-off issue. You invite five existing users, all of whom have dropped off at the exact point you’re investigating, and ask them questions to uncover why. A dialog develops: Participant 1: “Sometimes I’ll get a workout that I just don’t want to do. Sure, it’s a good workout—but I just don’t want to physically do it. I just do my own thing when that happens.” Participant 2: “Same here, some of them are so boring. I go to the gym because I love it. It’s an escape.” Participant 3: “Right?! I get that the app generates the best one for me on that specific day, but I wish I could get a couple of options.” Participant 4: “I’m the same, there are some exercises I just refuse to do. I’m not coming to the gym to do things I dislike.” Conducting the focus groups and reviewing the transcripts, you realize that users want options. A workout that works for one gym-goer doesn’t necessarily work for the next. A possible solution? Adding the option to generate a new workout (that still considers previous workouts)and the ability to blacklist certain exercises, like burpees. 3. Ethnographic researchEthnographic research is a research method that involves observing and interacting with users in a real-life environment. By studying users in their natural habitat, you can understand how your product fits into their daily lives. Ethnographic research can be active or passive. Active ethnographic research entails engaging with users in their natural environment and then following up with methods like interviews. Passive ethnographic research involves letting the user interact with the product while you note your observations. When to use ethnographic researchEthnographic research is best suited when you want rich insights into the context and environment in which users interact with your product. Keep in mind that you can conduct ethnographic research throughout the entire product design and development process —from problem discovery to post-launch. However, it’s mostly done early in the process:
How to conduct ethnographic research:
While ethnographic studies provide a comprehensive view of what potential users actually do, they are resource-intensive and logistically difficult. A common alternative is diary studies. Like ethnographic research, diary studies examine how users interact with your product in their day-to-day, but the data is self-reported by participants. ⚙️ Recruiting participants proving tough and time-consuming? Maze Panel makes it easy, with 400+ filters to find your ideal participants from a pool of 3 million participants. Ethnographic research: A qualitative research exampleYou're a UX researcher for a project management platform called ProFlow , and you’re conducting an ethnographic study of the project creation process with key users, including a startup’s COO. The first thing you notice is that the COO is rushing while navigating the platform. You also take note of the 46 tabs and Zoom calls opened on their monitor. Their attention is divided, and they let out an exasperated sigh as they repeatedly hit “refresh” on your website’s onboarding interface. You conclude the session with an interview and ask, “How easy or difficult did you find using ProFlow to coordinate a project?” The COO answers: “Look, the whole reason we turn to project platforms is because we need to be quick on our feet. I’m doing a million things so I need the process to be fast and simple. The actual project management is good, but creating projects and setting up tables is way too complicated.” You realize that ProFlow ’s project creation process takes way too much time for professionals working in fast-paced, dynamic environments. To solve the issue, propose a quick-create option that enables them to move ahead with the basics instead of requiring in-depth project details. 4. Qualitative observationQualitative observation is a similar method to ethnographic research, though not as deep. It involves observing your users in a natural or controlled environment and taking notes as they interact with a product. However, be sure not to interrupt them, as this compromises the integrity of the study and turns it into active ethnographic research. When to qualitative observationQualitative observation is best when you want to record how users interact with your product without anyone interfering. Much like ethnographic research, observation is best done during:
How to conduct qualitative observation:
Qualitative observation: An qualitative research exampleYou’re conducting UX research for Stackbuilder , an app that connects businesses with tools ideal for their needs and budgets. To determine if your app is easy to use for industry professionals, you decide to conduct an observation study. Sitting in with the participant, you notice they breeze past the onboarding process, quickly creating an account for their company. Yet, after specifying their company’s budget, they suddenly slow down. They open links to each tool’s individual page, confusingly switching from one tab to another. They let out a sigh as they read through each website. Conducting your observation study, you realize that users find it difficult to extract information from each tool’s website. Based on your field notes, you suggest including a bullet-point summary of each tool directly on your platform. 5. Case study researchCase studies are a UX research method that provides comprehensive and contextual insights into a real-world case over a long period of time. They typically include a range of other qualitative research methods, like interviews, observations, and ethnographic research. A case study allows you to form an in-depth analysis of how people use your product, helping you uncover nuanced differences between your users. When to use case studiesCase studies are best when your product involves complex interactions that need to be tracked over a longer period or through in-depth analysis. You can also use case studies when your product is innovative, and there’s little existing data on how users interact with it. As for specific phases in the product design and development process:
How to conduct case studies:
Case study research: A qualitative research exampleYour team has recently launched Pulse , a platform that analyzes social media posts to identify rising digital marketing trends. Pulse has been on the market for a year, and you want to better understand how it helps small businesses create successful campaigns. To conduct your case study, you begin with a series of interviews to understand user expectations, ethnographic research sessions, and focus groups. After sorting responses and observations into common themes you notice a main recurring pattern. Users have trouble interpreting the data from their dashboards, making it difficult to identify which trends to follow. With your synthesized insights, you create a report with detailed narratives of individual user experiences, common themes and issues, and recommendations for addressing user friction points. Some of your proposed solutions include creating intuitive graphs and summaries for each trend study. This makes it easier for users to understand trends and implement strategic changes in their campaigns. 6. Secondary researchSecondary research is a research method that involves collecting and analyzing documents, records, and reviews that provide you with contextual data on your topic. You’re not connecting with participants directly, but rather accessing pre-existing available data. For example, you can pull out insights from your UX research repository to reexamine how they apply to your new UX research objective. Strictly speaking, it can be both qualitative and quantitative—but today we focus on its qualitative application. When to use secondary researchRecord keeping is particularly useful when you need supplemental insights to complement, validate, or compare current research findings. It helps you analyze shifting trends amongst your users across a specific period. Some other scenarios where you need record keeping include:
Secondary research is especially valuable when your team faces budget constraints, tight deadlines, or limited resources. Through review mining and collecting older findings, you can uncover useful insights that drive decision-making throughout the product design and development process. How to conduct secondary research:
Secondary research: A qualitative research exampleSafeSurf is a cybersecurity platform that offers threat detection, security audits, and real-time reports. After conducting multiple rounds of testing, you need a quick and easy way to identify remaining usability issues. Instead of conducting another resource-intensive method, you opt for social listening and data mining for your secondary research. Browsing through your company’s X, you identify a recurring theme: many users without a background in tech find SafeSurf ’s reports too technical and difficult to read. Users struggle with understanding what to do if their networks are breached. After checking your other social media channels and review sites, the issue pops up again. With your gathered insights, your team settles on introducing a simplified version of reports, including clear summaries, takeaways, and step-by-step protocols for ensuring security. By conducting secondary research, you’ve uncovered a major usability issue—all without spending large amounts of time and resources to connect with your users. 7. Open-ended surveysOpen-ended surveys are a type of unmoderated UX research method that involves asking users to answer a list of qualitative research questions designed to uncover their attitudes, expectations, and needs regarding your service or product. Open-ended surveys allow users to give in-depth, nuanced, and contextual responses. When to use open-ended surveysUser surveys are an effective qualitative research method for reaching a large number of users. You can use them at any stage of the design and product development process, but they’re particularly useful:
How to conduct open-ended surveys:
Open-ended surveys: A qualitative research exampleYou're a UX researcher for RouteReader , a comprehensive logistics platform that allows users to conduct shipment tracking and route planning. Recently, you’ve launched a new predictive analytics feature that allows users to quickly identify and prepare for supply chain disruptions. To better understand if users find the new feature helpful, you create an open-ended, in-app survey. The questions you ask your users:
Most of the responses are positive. Users report using the predictive analytics feature to make last-minute adjustments to their route plans, and some even rely on it regularly. However, a few users find the feature hard to notice, making it difficult to adjust their routes on time. To ensure users have supply chain insights on time, you integrate the new feature into each interface so users can easily spot important information and adjust their routes accordingly. 💡 Surveys are a lot easier with a quality survey tool. Maze’s Feedback Surveys solution has all you need to ensure your surveys get the insights you need—including AI-powered follow-up and automated reports. Qualitative research vs. quantitative research: What’s the difference?Alongside qualitative research approaches, UX teams also use quantitative research methods. Despite the similar names, the two are very different. Here are some of the key differences between qualitative research and quantitative research .
Before selecting either qualitative or quantitative methods, first identify what you want to achieve with your UX research project. As a general rule of thumb, think qualitative data collection for in-depth understanding and quantitative studies for measurement and validation. Conduct qualitative research with MazeYou’ll often find that knowing the what is pointless without understanding the accompanying why . Qualitative research helps you uncover your why. So, what about how —how do you identify your 'what' and your 'why'? The answer is with a user research tool like Maze. Maze is the leading user research platform that lets you organize, conduct, and analyze both qualitative and quantitative research studies—all from one place. Its wide variety of UX research methods and advanced AI capabilities help you get the insights you need to build the right products and experiences faster. Frequently asked questions about qualitative research examplesWhat is qualitative research? Qualitative research is a research method that aims to provide contextual, descriptive, and non-numerical insights on a specific issue. Qualitative research methods like interviews, case studies, and ethnographic studies allow you to uncover the reasoning behind your user’s attitudes and opinions. Can a study be both qualitative and quantitative? Absolutely! You can use mixed methods in your research design, which combines qualitative and quantitative approaches to gain both descriptive and statistical insights. For example, user surveys can have both close-ended and open-ended questions, providing comprehensive data like percentages of user views and descriptive reasoning behind their answers. Is qualitative or quantitative research better? The choice between qualitative and quantitative research depends upon your research goals and objectives. Qualitative research methods are better suited when you want to understand the complexities of your user’s problems and uncover the underlying motives beneath their thoughts, feelings, and behaviors. Quantitative research excels in giving you numerical data, helping you gain a statistical view of your user's attitudes, identifying trends, and making predictions. What are some approaches to qualitative research? There are many approaches to qualitative studies. An approach is the underlying theory behind a method, and a method is a way of implementing the approach. Here are some approaches to qualitative research:
Information
InitiativesYou are accessing a machine-readable page. In order to be human-readable, please install an RSS reader. All articles published by MDPI are made immediately available worldwide under an open access license. No special permission is required to reuse all or part of the article published by MDPI, including figures and tables. For articles published under an open access Creative Common CC BY license, any part of the article may be reused without permission provided that the original article is clearly cited. For more information, please refer to https://www.mdpi.com/openaccess . Feature papers represent the most advanced research with significant potential for high impact in the field. A Feature Paper should be a substantial original Article that involves several techniques or approaches, provides an outlook for future research directions and describes possible research applications. Feature papers are submitted upon individual invitation or recommendation by the scientific editors and must receive positive feedback from the reviewers. Editor’s Choice articles are based on recommendations by the scientific editors of MDPI journals from around the world. Editors select a small number of articles recently published in the journal that they believe will be particularly interesting to readers, or important in the respective research area. The aim is to provide a snapshot of some of the most exciting work published in the various research areas of the journal. Original Submission Date Received: .
![]() Article Menu![]()
Find support for a specific problem in the support section of our website. Please let us know what you think of our products and services. Visit our dedicated information section to learn more about MDPI. JSmol ViewerDifficulties among teachers’ emotional regulation: analysis for the development of student well-being in chilean schools. ![]() 1. Introduction2. materials and methods, 2.1. sample, 2.2. data collection instrument, 2.3. analysis procedure, 4. discussion, 5. conclusions, author contributions, institutional review board statement, informed consent statement, data availability statement, conflicts of interest.
Share and CiteFuentes-Vilugrón, G.; Sandoval-Obando, E.; Caamaño-Navarrete, F.; Arriagada-Hernández, C.; Etchegaray-Pezo, P.; Muñoz-Troncoso, F.; Cuadrado-Gordillo, I.; del Val Martín, P.; Riquelme-Mella, E. Difficulties among Teachers’ Emotional Regulation: Analysis for the Development of Student Well-Being in Chilean Schools. Behav. Sci. 2024 , 14 , 749. https://doi.org/10.3390/bs14090749 Fuentes-Vilugrón G, Sandoval-Obando E, Caamaño-Navarrete F, Arriagada-Hernández C, Etchegaray-Pezo P, Muñoz-Troncoso F, Cuadrado-Gordillo I, del Val Martín P, Riquelme-Mella E. Difficulties among Teachers’ Emotional Regulation: Analysis for the Development of Student Well-Being in Chilean Schools. Behavioral Sciences . 2024; 14(9):749. https://doi.org/10.3390/bs14090749 Fuentes-Vilugrón, Gerardo, Eduardo Sandoval-Obando, Felipe Caamaño-Navarrete, Carlos Arriagada-Hernández, Paulo Etchegaray-Pezo, Flavio Muñoz-Troncoso, Isabel Cuadrado-Gordillo, Pablo del Val Martín, and Enrique Riquelme-Mella. 2024. "Difficulties among Teachers’ Emotional Regulation: Analysis for the Development of Student Well-Being in Chilean Schools" Behavioral Sciences 14, no. 9: 749. https://doi.org/10.3390/bs14090749 Article MetricsArticle access statistics, further information, mdpi initiatives, follow mdpi. ![]() Subscribe to receive issue release notifications and newsletters from MDPI journals ![]() |
IMAGES
VIDEO
COMMENTS
Tips to Write the Results Section. Direct the reader to the research data and explain the meaning of the data. Avoid using a repetitive sentence structure to explain a new set of data. Write and highlight important findings in your results. Use the same order as the subheadings of the methods section.
The results chapter (also referred to as the findings or analysis chapter) is one of the most important chapters of your dissertation or thesis because it shows the reader what you've found in terms of the quantitative data you've collected. It presents the data using a clear text narrative, supported by tables, graphs and charts.
After that comes a literature review, which ends with a summary of the research question(s) and/or hypotheses. A methods section, which explains the source of data, sample, and variables and quantitative techniques used, follows. Many analysts will include a short discussion of their descriptive statistics in the methods section.
Reporting quantitative research results. If you conducted quantitative research, you'll likely be working with the results of some sort of statistical analysis.. Your results section should report the results of any statistical tests you used to compare groups or assess relationships between variables.It should also state whether or not each hypothesis was supported.
Table of contents. What not to include in your discussion section. Step 1: Summarize your key findings. Step 2: Give your interpretations. Step 3: Discuss the implications. Step 4: Acknowledge the limitations. Step 5: Share your recommendations. Discussion section example. Other interesting articles.
Introduction to discussion chapter The following sections will focus on the general outcomes, results, conclusions and implications of the experiments of this thesis. Identified limitations and weaknesses of the research will also be discussed, followed by recommendations for future research. Finally, the main conclusions of this
Begin with a clear statement of the principal findings. This will reinforce the main take-away for the reader and set up the rest of the discussion. Explain why the outcomes of your study are important to the reader. Discuss the implications of your findings realistically based on previous literature, highlighting both the strengths and ...
Reporting Research Results in APA Style | Tips & Examples. Published on December 21, 2020 by Pritha Bhandari.Revised on January 17, 2024. The results section of a quantitative research paper is where you summarize your data and report the findings of any relevant statistical analyses.. The APA manual provides rigorous guidelines for what to report in quantitative research papers in the fields ...
What (exactly) is the discussion chapter? The discussion chapter is where you interpret and explain your results within your thesis or dissertation. This contrasts with the results chapter, where you merely present and describe the analysis findings (whether qualitative or quantitative).In the discussion chapter, you elaborate on and evaluate your research findings, and discuss the ...
The results section of a research paper tells the reader what you found, while the discussion section tells the reader what your findings mean. The results section should present the facts in an academic and unbiased manner, avoiding any attempt at analyzing or interpreting the data. Think of the results section as setting the stage for the ...
Return to all guides. Results and Discussion Sections in Scientific Research Reports (IMRaD) After introducing the study and describing its methodology, an IMRaD* report presents and discusses the main findings of the study. In the results section, writers systematically report their findings, and in discussion, they interpret these findings.
This handout was created to accompany the Writing in the Sciences video series. The purpose of the Results is to prepare readers for the discussion section by presenting the data in manageable chunks, in an order that corresponds with the research questions or objectives. The purpose of the Discussion section is used to contain analysis and ...
The analysis and interpretation of data is carried out in two phases. The. first part, which is based on the results of the questionnaire, deals with a quantitative. analysis of data. The second, which is based on the results of the interview and focus group. discussions, is a qualitative interpretation.
This template covers all the core components required in the results chapter of a typical dissertation, thesis or research project: The purpose of each section is explained in plain language, followed by an overview of the key elements that you need to cover. The template also includes practical examples to help you understand exactly what's ...
The results and discussion sections are one of the challenging sections to write. It is important to plan this section carefully as it may contain a large amount of scientific data that needs to be presented in a clear and concise fashion. The purpose of a Results section is to present the key results of your research.
Chapter 4-Quantitative Results and Discussion 4.1. Introduction In the previous chapter, the research design used in this study was described in detail. This included both the quantitative data collection involving the two questionnaires: BALLI and PELLEM, and the qualitative data collection which entailed a semistructured interview.
This chapter 5 presents the results of the study. First, an outline of the informants included in the study and an overview of the statistical techniques employed in the data analyses are given ...
Chapter III RESULTS AND DISCUSSION. The presentation, analysis, and interpretation of the data acquired for this study are all included in this chapter. According to the methodology, statistical tools are utilized to determine the student's perceptions towards the preservation and improvement of Central Luzon State University's landmark.
Even so, it's still a good idea to separate the results and discussion elements within the chapter, as this ensures your findings are both described and interpreted in a consistent fashion. ... number of steps will vary from project to project and will depend on the nature of the research aims, objectives, and research questions. For example ...
Chapter 3 Results and Discussion This Chapter - Free download as Word Doc (.doc / .docx), PDF File (.pdf), Text File (.txt) or read online for free. This chapter discusses the results of a study examining stereotype threat, self-efficacy, and academic performance among female engineering students. It presents five key findings: 1. Most respondents were aged 17-19, in their second year of study ...
Large sample sizes necessary. You need to use large sample sizes to achieve statistical significance and reliable results when doing quantitative analysis. Depending on the type of study you're conducting, gathering such extensive data can be resource-intensive, time-consuming, and costly. Quantitative data analysis methods
EMPIRICAL RESEARCH QUANTITATIVE. Open Access. Quality of nursing care: Predictors of patient satisfaction in a national sample ... Results. The study included 1014 patients with an average age of 51.3 (SD = 18.6), and 59.9% were females. More than two thirds of the sample reported having health insurance, and 62.7% were unemployed. The mean ...
The results chapter or section simply and objectively reports what you found, without speculating on why you found these results. The discussion interprets the meaning of the results, puts them in context, and explains why they matter. In qualitative research, results and discussion are sometimes combined. But in quantitative research, it's ...
When the goal is to generalise findings to a larger population, quantitative research provides the necessary data to support such conclusions. Identifying Patterns and Trends. Quantitative analysis can reveal patterns and trends in data that can inform decision-making and policy development. Integrating Qualitative and Quantitative Data
Table 2 MAPE and U-Theil results used to measure the deviation between out-of-sample and predictive values. Full size table The ARIMA model's forecasting results for the variables analyzed are ...
The results chapter in a dissertation or thesis (or any formal academic research piece) is where you objectively and neutrally present the findings of your qualitative analysis (or analyses if you used multiple qualitative analysis methods ). This chapter can sometimes be combined with the discussion chapter (where you interpret the data and ...
Maze is the leading user research platform that lets you organize, conduct, and analyze both qualitative and quantitative research studies—all from one place. Its wide variety of UX research methods and advanced AI capabilities help you get the insights you need to build the right products and experiences faster.
During the energy transition period and the increased supply of developed land, an analysis was conducted on the economic viability of transforming post-mining buildings. This study sought to answer the following questions: Does the circular economy create new conditions for adapting these specific industrial facilities? Should mining buildings be adapted or should they be demolished and built ...
Citation 15 The quantitative results reported herein include only the US data because this was the largest single-country ... Discussion. The results of the MOSAIC study support that patients with GA and caregivers experience a high degree of clinical, emotional, and financial burden related to GA, with considerable overlap noted between ...
Introduction. Emotional regulation, a process that involves detecting and evaluating physiological signals in response to stressful events, is a crucial aspect of preparing students for school and ensuring teachers' effectiveness, stress management, and job satisfaction. Method. This research, which adopted a quantitative approach, used a non-experimental comparative and cross-sectional ...