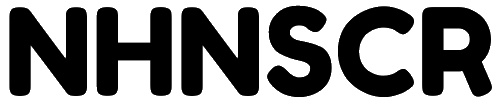

Understanding Brain Waves: A Comprehensive Guide
Have you ever wondered how your brain works? How it processes and stores information? The brain is one of the most complex organs in the human body, and studying its functionality has led to the discovery of many fascinating things, including brain waves.
In this blog, weâll explore everything you need to know about brain waves, including what they are, how they affect us, and how we can control them.
What Are Brain Waves?
Brain waves are electrical impulses that flow through the brain, creating patterns of activity. They are measured in cycles per second, or hertz (Hz). There are four main types of brain waves: alpha, beta, delta, and theta.
Each type of brain wave is associated with a different state of consciousness. Beta waves, for example, are associated with wakefulness and alertness, while delta waves are associated with deep sleep.
Hereâs a breakdown of the different types of brain waves and their associated frequencies:
- Beta Waves (12-30 Hz) â These brain waves are associated with alertness and wakefulness. They are most commonly observed in the frontal lobe of the brain.
- Alpha Waves (8-12 Hz) â These brain waves are associated with relaxation and calmness. They are most commonly observed in the occipital lobe of the brain.
- Theta Waves (4-8 Hz) â These brain waves are associated with meditation, creativity, and dreaming. They are most commonly observed in the temporal lobe of the brain.
- Delta Waves (0.5-4 Hz) â These brain waves are associated with deep sleep and unconsciousness. They are most commonly observed in the parietal and frontal lobes of the brain.
What Do Brain Waves Affect?
Brain waves have a significant impact on our overall well-being. They affect everything from our mood to our ability to concentrate.
Here are some of the things that brain waves can affect:
- Mood â Different types of brain waves are associated with different moods. For example, alpha waves are associated with relaxation and calmness, while beta waves are associated with stress and anxiety.
- Concentration â Brain waves can affect our ability to concentrate and focus. For example, theta waves, which are associated with meditation and creativity, can help us focus and be more productive.
- Sleep â Brain waves play a crucial role in sleep and can impact the quality of our sleep. Delta waves, which are associated with deep sleep, can help us get a restful nightâs sleep.
- Memory â Brain waves are also associated with memory. Theta waves, in particular, are believed to help with memory consolidation and recall.
Brain Waves During Sleep
Brain waves play a critical role in sleep, with each type of brain wave serving a different function.
During deep sleep, the brain produces delta waves, which help us rest and recharge. As we move into lighter stages of sleep, the brain produces more alpha and theta waves, which can help us transition back into wakefulness.
REM sleep is associated with beta waves, which can make our brains more active and generate vivid dreams.
Theta Brain Waves Benefits
Theta waves have been shown to have a range of benefits, including:
- Reducing Anxiety â Theta waves have a calming effect on the brain and can help reduce anxiety.
- Boosting Creativity â Theta waves are associated with creativity and can help stimulate the imagination.
- Improving Memory â Theta waves have been shown to improve memory consolidation and recall.
Gamma Brain Waves
Gamma brain waves are another type of brain wave, measuring between 25 and 100 Hz. They are associated with high levels of concentration, focus, and problem-solving.
Gamma waves have been shown to have a range of benefits, including:
- Improved Cognitive Function â Gamma waves can help improve cognitive function, including memory, perception, and attention.
- Reduced Anxiety â Gamma waves have a calming effect on the brain and can help reduce anxiety.
- Enhanced Learning â Gamma waves can help improve learning and information processing.
Brain Waves for Studying
Brain waves can be used to improve study habits and enhance learning. Here are some tips for using brain waves to study:
- Alpha Waves â Listening to alpha waves can help you relax and stay focused while studying.
- Theta Waves â Theta waves can help stimulate creativity and improve memory consolidation. Try listening to theta waves while studying.
- Gamma Waves â Gamma waves can help improve cognitive function and learning. Try listening to gamma waves while studying difficult or challenging material.
How to Measure Brain Waves at Home
Measuring brain waves used to require specialized equipment and expertise, but thanks to advances in technology, itâs now possible to measure brain waves at home.
Here are some tools that you can use to measure brain waves at home:
- Electroencephalogram (EEG) â EEG devices are used to measure brain waves and provide detailed information about brain activity.
- Brain-Sensing Headbands â There are a variety of headbands on the market that can measure brain waves, including Muse and NeuroSky.
- Mobile Apps â There are a variety of mobile apps that can measure brain waves, including Brain Waves and Brainify.
Which Frequency Is Best for the Brain?
There is no one-size-fits-all answer to which frequency is best for the brain. Different frequencies are associated with different states of consciousness and can have different effects on the brain.
Here are some general guidelines:
- Beta Waves â Use beta waves when you need to be alert and focused.
- Alpha Waves â Use alpha waves when you need to be relaxed and calm.
- Theta Waves â Use theta waves when you want to stimulate creativity and improve memory consolidation.
- Delta Waves â Use delta waves when you want to promote rest and relaxation.
How to Change Brain Waves at Will
Changing brain waves at will is challenging, but itâs not impossible. Here are some tips for changing brain waves:
- Meditation â Practicing meditation can help you learn to regulate your brain waves and enter different states of consciousness.
- Breathing Exercises â Controlled breathing exercises can help you regulate your brain waves and reduce stress and anxiety.
- Brain-Training Games â There are a variety of brain-training games and exercises that can help stimulate different types of brain waves.
Brain waves are a fascinating aspect of brain activity, offering insights into how the brain works and potentially providing opportunities for enhancing our overall wellbeing. Understanding brain waves can help us better understand ourselves and improve our mental health and cognitive function.
Now that you know more about brain waves, give some of the tips and tools weâve shared a try and see how they work for you. With practice and persistence, you can learn to control and use your brain waves to your advantage.
So, start today!
- Skip to main content
- Skip to header right navigation
- Skip to site footer
Sources of Insight
Unleash Your Greatest Potential
5 Types of Brainwave Frequencies: Gamma, Beta, Alpha, Theta, Delta

“The energy of the mind is the essence of life.” — Aristotle
Ever since I was young, I’ve been really interested in brain waves and how they work.
When I got to college, I started studying them more seriously and learned all about the different types, like Gamma, Beta, Alpha, Theta, and Delta.
It was amazing to discover how these brain waves affect the way we think, feel, and even how we do things.
I was especially fascinated by the idea that we might be able to change our brain waves to help ourselves feel better or think more clearly.
This interest in brain waves has been a big part of my studies and has kept me curious about the brain’s power.
My goal with this article is to equip you with enough insight to be able to leverage your different brainwave frequencies to unleash more of your potential.
Key Takeaways
- Your brainwaves vibrate at different frequencies affecting your mental state.
- Gamma is for intense focus, Beta for alertness, Alpha for relaxation, Theta for meditation, and Delta for deep sleep. Visualization works best in Alpha, a relaxed yet focused state that reduces stress and limiting beliefs.
- Techniques like meditation can help you shift to Alpha for more effective visualization and unlock your full potential.
- By understanding brainwaves, you can target specific mental states for tasks like learning (Gamma), concentration (Beta), or creativity (Alpha).
- Ultimately, being mindful of your brainwave state allows you to optimize your well-being for different situations.
What are Brainwave Levels?
Brainwave levels refer to the electrical activity in the brain, which can be measured by frequency in hertz (Hz).
These brainwaves are typically categorized into several states, each associated with different levels of consciousness, mental activities, and states of being.
Hereâs an overview of the main brainwave levels and their characteristics:
- Gamma Waves (30 Hz and above) : Heightened perception, learning, and cognitive functioning.
- Beta Waves (14 to 30 Hz): Active thinking, focus, problem-solving, and stress.
- Alpha Waves (8 to 14 Hz): Relaxed alertness, stress reduction, and enhanced creativity. A bridge to your subconscious.
- Theta Waves (4 to 8 Hz): Light sleep, deep meditation, creativity, and emotional processing.
- Delta Waves (0.5 to 4 Hz): Deep sleep, healing, and restoration.
Let’s walk through each in more detail…
Gamma Waves
Gamma waves are the electrical activity in your brain with the highest frequencies, ranging from 30 Hz (Hertz) and above.
They are associated with a number of important cognitive functions:
- Heightened Perception : Gamma waves are believed to play a role in binding information from different parts of the brain, creating a unified picture of the world around you. This can lead to sharper senses and a more detailed understanding of your environment.
- Learning and Memory : Gamma waves are thought to be involved in consolidating short-term memories into long-term ones. They may also be important for processing new information and integrating it with existing knowledge.
- Cognitive Functioning : Gamma waves are associated with a variety of higher-order cognitive functions, such as problem-solving, decision-making, and creativity. When your brain is generating a lot of gamma waves, you’re likely in a state of peak mental performance.
Here are some additional points to consider:
- Gamma and Focused Attention: Gamma waves are often linked to focused attention. When you’re deeply concentrated on a task, your brain may produce more gamma waves in the relevant areas.
- Not a Single Function : While gamma waves are associated with various cognitive functions, it’s important to remember they likely interact with other brainwaves to produce these effects. The brain is a complex system, and gamma waves are just one piece of the puzzle.
- Brainwave Entrainment: Some people are interested in using brainwave entrainment techniques to increase gamma wave activity. This research is ongoing, and there are mixed results about the effectiveness of these techniques.
Beta waves are the workhorses of your brain, reigning in the frequency range of 14 Hz to 30 Hz.
They’re associated with a busy, engaged mind and are crucial for various daily activities:
- Active Thinking and Focus : Beta waves are your “get things done” brainwave. They power your ability to concentrate on tasks, analyze information, and make decisions. When you’re brainstorming ideas, tackling a problem, or following instructions, your brain is likely generating a lot of beta waves.
- Problem-Solving and Critical Thinking : Beta waves are key players in dissecting problems, evaluating options, and reaching logical conclusions. They help you think critically, weigh pros and cons, and make sound judgments.
- Juggling Multiple Tasks : Beta waves are essential for multitasking. They allow you to switch between different tasks efficiently, maintain focus under pressure, and keep all the balls in the air (metaphorically speaking).
- Maintaining Alertness: Beta waves contribute to feelings of wakefulness and alertness. They keep you engaged in your surroundings and prevent your mind from wandering off into daydreams.
- Beta Waves and Stress: While beta waves are necessary for focused work, prolonged periods in the high-beta range (around 30 Hz) can be associated with stress and anxiety. Taking breaks and finding ways to relax can help regulate beta wave activity.
- Individual Differences: The optimal beta frequency for peak performance can vary from person to person. Some people may function best with slightly lower or higher beta activity.
- Learning and Memory: Although not the primary function, beta waves may play a supporting role in learning and memory consolidation by facilitating focused attention on new information.
By understanding beta waves, you can leverage them for increased productivity, sharper focus, and effective problem-solving.
However, finding a healthy balance and avoiding prolonged high-beta states is essential for maintaining mental well-being.
Alpha Waves
Alpha waves, with their calming rhythm between 8 Hz and 14 Hz, bridge the gap between wakefulness and relaxation.
They usher in a state of mind ideal for activities that benefit from both focus and inner peace.
Here’s what alpha waves bring to the table:
- Relaxed Alertness : Alpha waves aren’t about zoning out. They foster a state of calm yet focused awareness. Imagine a seasoned meditator â present and collected, yet not tense. That’s the alpha zone.
- Stress Reduction : Alpha waves act as a natural antidote to stress. By promoting relaxation, they help lower cortisol levels (the stress hormone) and induce a sense of emotional well-being.
- Enhanced Creativity: When the mind isn’t bombarded by external stimuli or internal chatter, creativity flourishes. Alpha waves create a space for ideas to flow freely, fostering innovative problem-solving and out-of-the-box thinking.
- Bridge to the Subconscious: Alpha waves are believed to act as a bridge between your conscious and subconscious mind. This allows you to tap into your intuition and access deeper levels of insight that may be hidden beneath the surface in a Beta state.
- Learning and Memory : While not the primary focus, alpha waves might play a supporting role in learning and memory. The relaxed focus of alpha can facilitate better absorption and processing of information.
- Visualization Powerhouse : The combination of relaxation and focus makes alpha waves ideal for visualization practices. In this state, you can create vivid mental pictures of your goals and intentions, which can enhance motivation and goal achievement.
- Meditation and Mindfulness: Activities like meditation and mindfulness training can help cultivate alpha wave activity. By focusing on the present moment and letting go of distractions, you can train your brain to spend more time in this beneficial state.
By understanding alpha waves, you can harness their power to reduce stress, boost creativity, and unlock your full potential.
They offer a gateway to a calmer, more focused, and ultimately more productive you.
Theta Waves
Theta waves, with their gentle hum between 4 Hz and 8 Hz, usher you into a twilight zone between wakefulness and sleep.
They unlock a unique mental state characterized by:
- Light Sleep and Daydreaming : Theta waves are prominent during light sleep stages and daydreaming. They allow your mind to wander freely, fostering imagination and spontaneous creativity.
- Deep Meditation : Theta waves play a key role in deep meditative states. They promote a sense of inner peace, detachment from external stimuli, and a heightened awareness of your internal world.
- Emotional Processing: Theta waves are believed to be involved in emotional processing and memory consolidation. They may help you connect with your emotions on a deeper level, integrate past experiences, and release emotional baggage.
- Intuition and Insight: Theta waves can facilitate access to intuition and deeper insights. By quieting the chatter of the conscious mind, they allow for a more intuitive understanding of situations and potential solutions to problems.
- Learning and Memory : Theta waves may play a supporting role in certain types of learning, particularly procedural memory (learning skills and habits). The relaxed focus of theta can enhance the absorption of new information on an unconscious level.
- Hypnosis and Hypnotherapy: Theta waves are associated with hypnotic states, where the mind becomes more receptive to suggestion. This makes theta waves a potential tool for hypnotherapy and promoting positive change.
- Creativity Spark: Theta waves can be a catalyst for creative breakthroughs. By allowing the mind to wander and make unexpected connections, they can spark new ideas and innovative solutions.
By understanding theta waves, you can leverage them for deeper relaxation, emotional processing, and accessing your creative wellspring.
They offer a gateway to a more introspective, intuitive, and imaginative state of mind.
Delta Waves
Delta waves, the slowest brainwaves with frequencies ranging from 0.5 Hz to 4 Hz, plunge you into the depths of sleep.
They’re the conductors of your restorative symphony, playing a vital role in:
- Deep Sleep and Regeneration : Delta waves are the dominant brainwave during deep sleep stages, when your body prioritizes physical and mental restoration. They promote the release of growth hormone, crucial for cell repair and tissue regeneration.
- Boosted Immunity : Deep delta wave sleep strengthens your immune system, allowing your body to better fight off illness and infection. Delta sleep is where your body repairs and recharges for the demands of the waking world.
- Memory Consolidation : While research is ongoing, delta waves are believed to play a role in memory consolidation. During deep sleep, memories are thought to be transferred from short-term to long-term storage, solidifying learning and experiences.
- Pain Management: Delta waves may contribute to pain management by promoting the release of natural painkillers in the brain. Deep sleep facilitated by delta waves allows your body to process and potentially reduce pain perception.
- Not Complete Unconsciousness : Despite being associated with deep sleep, the brain isn’t entirely inactive during delta wave dominance. Some subconscious processing and emotional regulation may still occur.
- Importance of Sufficient Delta Sleep : Getting enough deep delta wave sleep is crucial for overall health and well-being. Chronic sleep deprivation can disrupt delta wave activity and lead to a cascade of negative consequences.
- Age and Delta Waves : As we age, the production of delta waves naturally decreases. This may contribute to lighter sleep patterns experienced by some older adults.
By understanding delta waves, you can appreciate their significance for deep, restorative sleep.
Prioritizing good sleep hygiene practices can help you optimize delta wave activity and reap the benefits of a truly restful night’s sleep.
How Alpha Waves Enhance Your Visualization
Why not just practice visualization the same way Olympic athletes, people in sport, and leaders do?
This is the part that got my attention.
Typically, when people practice visualization, they are in a Beta wave state, which is alert and active thinking.
Its where limiting beliefs can get in the way, along with fear and anxiety.
Itâs where stress lives.
But when you are in an Alpha state, you can be relaxed and more creative leading to more breakthroughs and creative brilliance.
Letâs take a deeper lookâŠ
How Alpha State is Different from Beta State When You Practice Visualization
Being in an Alpha state, compared to a Beta state, can lead to feeling less fear and fewer limiting beliefs, as well as setting the stage for greater creativity.
Hereâs why:
Alpha State Characteristics:
- Relaxed Focus : The Alpha state is associated with relaxed alertness, mindfulness, and a calm, meditative state of mind.
- Reduced Anxiety : Being in Alpha reduces stress and anxiety levels, as it is linked to the brainâs resting state, which promotes feelings of calm and relaxation.
- Enhanced Creativity: Alpha waves facilitate more creative thinking and problem-solving because they allow for a freer flow of ideas, without the constraints of highly focused attention.
- Lowered Guard: In Alpha, the mental filters and barriers are lowered, allowing for less critical and more expansive thinking, reducing the impact of fear and limiting beliefs.
Beta State Characteristics:
- Active Thinking and Focus : The Beta state is associated with active, analytical thought, attention to external activities, and engagement with tasks.
- Higher Stress Levels: Prolonged periods in Beta, especially in high frequencies, can correlate with increased stress, anxiety, and overthinking.
- Limiting Beliefs: The heightened alertness and analytical thinking in Beta may reinforce limiting beliefs and fear, as the mind is more likely to focus on potential problems and risks.
Transitioning into an Alpha state can be particularly beneficial for activities requiring creativity, learning, and relaxation, as it allows for a more expansive, less judgmental mindset, paving the way for innovative thinking and reducing feelings of fear and self-doubt.
Visualization Hacks: Optimize Your Brainwaves for Success
- Visualization Works Best When You’re Relaxed : Don’t visualize in a stressed Beta state. It amplifies doubts and limitations.
- Alpha is Your Visualization Sweet Spot : This relaxed, alert state fosters creativity, reduces anxiety, and weakens limiting beliefs.
- Brainwaves Have Frequencies: These frequencies correspond to different mental states. Gamma is for intense focus, Beta for active thinking, Alpha for relaxation, Theta for daydreaming, and Delta for deep sleep.
- Visualization in Beta Can Be Counterproductive: Beta’s focus on potential problems can hijack your visualization and make it feel forced.
- Alpha = Open Mind for Success: The relaxed focus of Alpha allows expansive thinking, making it ideal for planting the seeds of your goals.
- Shift Your Brainwaves for Visualization Power: Techniques like meditation and deep breathing can help you transition into Alpha for more effective visualization.
- Visualization + Alpha = Champion’s Mindset: By optimizing your brainwaves, you can unlock the power of visualization and achieve your goals like a champion.
How To Leverage Your 5 Brain Wave Frequencies
Changing brainwave states can be quite beneficial for enhancing various mental functions, improving sleep, and fostering emotional well-being.
Here are actionable steps and examples for each type of brainwave, along with explanations for why and when you might want to alter them:
Gamma Waves (>30 Hz): Enhancing Cognitive Processing
- How to Change : Engage in tasks that require intense focus or enjoy activities that challenge your brain, like complex problem-solving or learning a new language.
- Why : Gamma waves are associated with high-level information processing, insight, and cognitive functioning.
- When : Optimize Gamma waves when you need to process information rapidly, such as studying for an exam or working on a complex project.
Beta Waves (13-30 Hz): Increasing Alertness and Concentration
- How to Change : Stimulate your mind with active engagement in work, conversations, or reading. Caffeine can also temporarily increase Beta wave activity.
- Why : Beta waves are dominant during awake, alert states where thinking, decision-making, and focus are required.
- When : Encourage Beta wave activity when you need to be fully alert and focused, like preparing for a meeting or working on a deadline.
Alpha Waves (8-13 Hz): Promoting Relaxation and Creativity
- How to Change : Practice relaxation techniques such as deep breathing, mindfulness, or gentle yoga. Close your eyes and visualize peaceful scenes, or listen to music designed to induce Alpha states.
- Why : Alpha waves are linked with states of calm alertness and bridge the conscious and subconscious mind, enhancing creativity.
- When : Foster Alpha waves when looking to reduce stress, brainstorm, or tap into your creative reserves, perhaps when seeking innovative solutions or engaging in artistic activities.
Theta Waves (4-8 Hz): Improving Meditation and Memory
- How to Change : Engage in deep meditation or use guided imagery. Techniques such as hypnosis or autopilot activities like showering can also lead to increased Theta activity.
- Why : Theta waves are present during deep relaxation, meditation, and REM sleep and are involved in memory and learning.
- When : Stimulate Theta waves for deep meditation, spiritual practices, or when seeking intuitive insights. They’re also beneficial for memory consolidation, so consider this before sleep or after learning new information.
Delta Waves (0.5-4 Hz): Enhancing Deep Sleep and Healing
- How to Change : Prioritize sleep hygiene practices such as reducing screen time before bed, ensuring a dark, cool sleep environment, and establishing a regular sleep schedule. Delta waves can also be induced through certain types of deep, transcendental meditation.
- Why : Delta waves are the slowest and are associated with deep, restorative sleep and bodily healing processes.
- When : Focus on Delta wave sleep at night for physical recovery and mental refreshment, especially after periods of high stress or illness.
By mindfully engaging in activities that promote the desired brainwave state, you can influence your mental and emotional processes to optimize your well-being for specific situations and tasks.
Use Your Brainwaves to Unlock Your Potential
We’ve looked at five different types of brainwaves: Gamma, Beta, Alpha, Theta, and Delta.
Each one helps you in its own way, like making you smarter, helping you focus, relax, get creative, or even sleep deeply.
Understanding these brainwaves is like having a secret key to control how you feel and think.
You can change your brainwaves on purpose to feel better or think clearer.
For example, you can meditate to calm down or do puzzles to sharpen your focus.
It’s pretty cool that by knowing about these brainwaves, you can make your days better.
So, use what you’ve learned to feel happier, think better, and live a more fulfilling life.
You Might Also Like
4 Ways to Improve Your Visualization Technique 5 Minute Bedtime Routine to Become Your Best How To Build Mental Toughness Like a Navy SEAL How To Practice the Silva Method How To Visualize Success More Effectively How To Visualize Your Future Self Better The Power of Mental Rehearsal to Learn New Skills
Every print subscription comes with full digital access
Science News
Brain waves may focus attention and keep information flowing.
Studies suggest the oscillations created by nerve cell activity have roles of their own

CATCH A WAVE Created by collections of busy nerve cells, brain waves may help the brain organize information, new studies suggest.
GuidoVrola/Shutterstock
Share this:
By Laura Sanders
March 13, 2018 at 1:00 pm
We canât see it, but brains hum with electrical activity. Brain waves created by the coordinated firing of huge collections of nerve cells pinball around the brain. The waves can ricochet from the front of the brain to the back, or from deep structures all the way to the scalp and then back again.
Called neuronal oscillations, these signals are known to accompany certain mental states. Quiet alpha waves ripple soothingly across the brains of meditating monks. Beta waves rise and fall during intense conversational turns. Fast gamma waves accompany sharp insights. Sluggish delta rhythms lull deep sleepers, while dreamers shift into slightly quicker theta rhythms.
Researchers have long argued over whether these waves have purpose, and what those purposes might be. Some scientists see waves as inevitable but useless by-products of the signals that really matterâ ââ messages sent by individual nerve cells. Waves are simply a consequence of collective neural behavior, and nothing more, that view holds. But a growing body of evidence suggests just the opposite: Instead of by-products of important signals, brain waves are key to how the brain operates, routing information among far-flung brain regions that need to work together.
MITâs Earl Miller is among the neuroÂscientists amassing evidence that waves are an essential part of how the brain operates. Brain oscillations deftly route information in a way that allows the brain to choose which signals in the world to pay attention to and which to ignore, his recent studies suggest.
Other research supports this view, too. Studies on people with electrodes implanted in their brains suggest brain waves, and their interactions, help enable emotion, language, vision and more.
When these waves are abnormal, brainpower suffers, studies find. Detailed looks at how the brain uses these waves raise the possibility of tweaking the signals with electrical nudgesâ ââ interventions that could lead to therapies that can correct memory problems and mental illness, for instance. Already, early attempts have led to improvements in peopleâs memory.
Types of brain waves
Scientists are studying how oscillations generated by nerve cells affect brain function. Although the boundaries between different wave types can be fuzzy, these oscillations can be grouped by frequency.
Fast gamma waves have been linked to states of high attention. 30 to 80 Hz

Beta waves may be involved in movement and complex tasks such as memory and decision making. 12 to 30 Hz

The first neuronal oscillations discovered, alpha waves appear when a relaxed person closes his eyes. 8 to 12 Hz

Theta oscillations may help the brain sort information essential for navigation. 4 to 8 Hz

Slow delta waves mark deep sleep and anesthesia. 1.5 to 4 Hz

These insights about brain waves coincide with a shift in neuroscience away from a view that reduces the brain down to the behavior of single nerve cells, or neurons. Thatâs like thinking of the brain as âa giant clock, and if you figure out each gear, youâll figure out the brain,â Miller says. But âitâs not just individual neurons in a giant clock. Itâs networks interacting in a very dynamic, fluid way.â
Central to those interactions, Miller and others think, are coordinated brain waves. âThe oscillations are the most powerful signal in the brain,â Miller says. âHow could evolution not have taken advantage of that?â
In three recent papers, Miller and colleagues argue that two different types of brain wavesâ ââ beta and gammaâ ââ work together to selectively choose the information that makes it into working memory. Gamma waves that cycle 30 to 80 times per second (30 to 80 hertz) help coordinate information streaming in from our sensesâ ââ what we feel, see and smell. In contrast, slower 12 to 30 Hz beta waves are the messages that help keep us on task by guiding the brain toward the sensory signals worth paying attention to.
These two types of brain oscillations engage in a neural seesaw : When beta waves are strong, akin to a stereo blasting, gamma waves are weak, as if the volume had been dialed down, and vice versa. Miller and colleagues saw this push-and-pull action in the brains of monkeys with implanted electrodes as the animals completed a tricky memory task, one that required the monkeys to hold several pieces of information in their minds at the same time. The results were described January 26 in Nature Communications. âAt all these complex decision points, you can see the beta and gamma doing this complex dance in a way that youâd expect if theyâre controlling working memory,â Miller says.
These two types of waves were generated in different parts of the brain, offering spatial clues about how the brain focuses itself, the researchers also found. Sensory information, organized by gamma waves, skims the superficial layers of the brain, experiments on monkeys showed. But slower, more goal-directed waves, a mix of alpha and beta waves, are deeper in the brain. And those slower, deeper waves could actually dial down the strength of the gamma waves that rippled along the outer brain. The deeper waves were selecting which sensory information to pay attention to, the researchers proposed in the Jan. 30 Proceedings of the National Academy of Sciences .
A third paper, in the Feb. 7 Neuron, shows similar interactions between gamma and beta waves while monkeys matched patterns of dots on a computer screen. Some of the patterns were clearly different but still belonged to the same category, an easy task akin to knowing that both a dog and a cat are types of animals. Other times, the patterns were harder to classify and required more sophisticated mental work, similar to knowing that trains and bicycles are both types of transportation. Gamma waves were present when the monkeys were puzzling out an easy category. But when higher-level categorization was required, beta waves started to roll.
These interactions between gamma and beta waves might be how the brain solves an information overload problem, Miller suspects. Incoming sensory input constantly bombards the brain, and much of it is meaningless. The brain needs a way to figure out if it should ignore the feeling of a scratchy shirt, but pay attention to the ringing phone. These two rhythms may offer a way for âvolitional control over what you think about,â Miller says, allowing a person to consciously choose what information to bring to mind.
Oscillations may also shape visual information as it travels through the brain, says Charles Schroeder, a neuroscientist at the Nathan S. Kline Institute for Psychiatric Research in Orangeburg, N.Y. He and colleagues are studying a different ebb and flow of oscillations from the one Miller recently described. This one probably involves a host of different kinds of waves, including theta waves, and happens in the split second when your eyes dwell on a sceneâ ââ a pause that usually lasts about 200 milliseconds.
When you look at a scene, the first half of the time it takes to stare is spent on visual information streaming into your brain. But toward the end of that fixation time, âthe information flow reverses,â Schroeder says. Different neuronal oscillations carry signals from the brainâs command center, ready to direct the eyes to the next spot. âLiterally within a tenth of a second before you move your eyes, there is this incredible flash of network activity in the front of the brain, and then the eyes move,â Schroeder says. âItâs really a dramatic thing.â Schroeder and colleagues have caught this action in monkeysâ brains, and more recently, in people implanted with electrodes as part of epilepsy treatment.
But some vision researchers still dismiss these oscillations as noise, convinced that the activity of single neuronsâ ââ and not the collective waves that result from that activityâ ââ is the key to understanding the brain, Schroeder says. âItâs still difficult to convince people that brain oscillations are functional.â
Researchers may argue over the function of brain waves for years to come, says neuroscientist and neurologist Robert Knight of the University of California, Berkeley. He believes that information, at its core, is held in the signals zipped off by neurons. But work from his lab has convinced him that oscillations help those signals reach the right spot, connecting brain areas in important ways. âYouâve got to have a way to get brain areas communicating,â he says. âWhat oscillations do is provide a routing mechanism.â
And oscillations do this quickly, he says. Human brains are incredibly fast. âWeâre handling massive amounts of information in subsecond time periods,â Knight says. âAnd you have to have some way to shape it, to control it.â Waves, he says, give the brain a way to tune out extraneous information by temporarily shutting down unnecessary communication lines.
Knight and colleagues recently spotted fast gamma waves at work as people did a wide range of tasks, including repeating words, answering questions about themselves and distinguishing male faces from female. A certain gamma wave pattern seemed to predict when people would get the right answer on these tasks, the team reported in December 2017 in Nature Human Behavior . Gamma waves, the team suspects, link up areas of the brain that are needed to turn goals into action.
If oscillations are crucial information routers in the brain, then changing them might be beneficial when information is distorted or lost. Altered oscillations have been observed in disorders such as autism, Parkinsonâs disease, depression and anxiety, and even in normal aging.
A study published February 6 in Nature Communications hints at the potential of tweaking these rhythms . Youssef Ezzyat, a neuroscientist at the University of Pennsylvania, and colleagues studied memory abilities in 25 people who had electrodes implanted in their brains as part of their epilepsy treatment.

As the researchers gave the people lists of words to remember, electrodes monitored neural oscillations. A computer algorithm then figured out which assortment of brain waves indicated when a person was likely to remember the word, an assortment that varied slightly from person to person.
When those good performance signals were missing, the researchers delivered a short burst of electricity to the brainâ ââ âa bit of a nudge to course correct,â Ezzyat says. And these nudges improved performance.
Specifically manipulating brain waves to treat brains is a long way off, Ezzyat cautions. But he and colleagues are making progress. In the meantime, his results and othersâ are powerful signs that brain waves arenât just an idle hum.
More Stories from Science News on Neuroscience

A hunger protein reverses anorexia symptoms in mice

âThen I Am Myself the Worldâ ponders what it means to be conscious

Alzheimerâs blood tests are getting better, but still have a ways to go
Psilocybin temporarily dissolves brain networks.

Bird flu has been invading the brains of mammals. Hereâs why

Breastfeeding should take a toll on bones. A brain hormone may protect them

âDo I Know You?â explores face blindness and the science of the mind

Pain may take different pathways in men and women
Subscribers, enter your e-mail address for full access to the Science News archives and digital editions.
Not a subscriber? Become one now .

Interacting Brain Waves Key to How We Process Information
Summary: Study reveals the different ways the brain parses information through interactions of waves of neural activity.
Source: Salk Institute
For years, the brain has been thought of as a biological computer that processes information through traditional circuits, whereby data zips straight from one cell to another. While that model is still accurate, a new study led by Salk Professor Thomas Albright and Staff Scientist Sergei Gepshtein shows that thereâs also a second, very different way that the brain parses information: through the interactions of waves of neural activity.
The findings, published in Science Advances  on April 22, 2022, help researchers better understand how the brain processes information.
âWe now have a new understanding of how the computational machinery of the brain is working,â says Albright, the Conrad T. Prebys Chair in Vision Research and director of Salkâs Vision Center Laboratory. âThe model helps explain how the brainâs underlying state can change, affecting peopleâs attention, focus, or ability to process information.â
Researchers have long known that waves of electrical activity exist in the brain, both during sleep and wakefulness. But the underlying theories as to how the brain processes informationâparticularly sensory information, like the sight of a light or the sound of a bellâhave revolved around information being detected by specialized brain cells and then shuttled from one neuron to the next like a relay.
This traditional model of the brain, however, couldnât explain how a single sensory cell can react so differently to the same thing under different conditions. A cell, for instance, might become activated in response to a quick flash of light when an animal is particularly alert, but will remain inactive in response to the same light if the animalâs attention is focused on something else.
Gepshtein likens the new understanding to wave-particle duality in physics and chemistryâthe idea that light and matter have properties of both particles and waves. In some situations, light behaves as if it is a particle (also known as a photon). In other situations, it behaves as if it is a wave. Particles are confined to a specific location, and waves are distributed across many locations. Both views of light are needed to explain its complex behavior.
âThe traditional view of brain function describes brain activity as an interaction of neurons. Since every neuron is confined to a specific location, this view is akin to the description of light as a particle,â says Gepshtein, director of Salkâs Collaboratory for Adaptive Sensory Technologies.
âWeâve found that in some situations, brain activity is better described as interaction of waves, which is similar to the description of light as a wave. Both views are needed for understanding the brain.â
Some sensory cell properties observed in the past were not easy to explain given the âparticleâ approach to the brain. In the new study, the team observed the activity of 139 neurons in an animal model to better understand how the cells coordinated their response to visual information. In collaboration with physicist Sergey Savelâev of Loughborough University, they created a mathematical framework to interpret the activity of neurons and to predict new phenomena.
The best way to explain how the neurons were behaving, they discovered, was through interaction of microscopic waves of activity rather than interaction of individual neurons. Rather than a flash of light activating specialized sensory cells, the researchers showed how it creates distributed patterns: waves of activity across many neighboring cells, with alternating peaks and troughs of activationâlike ocean waves.
When these waves are being simultaneously generated in different places in the brain, they inevitably crash into one another. If two peaks of activity meet, they generate an even higher activity, while if a trough of low activity meets a peak, it might cancel it out. This process is called wave interference.
âWhen youâre out in the world, there are many, many inputs and so all these different waves are generated,â says Albright. âThe net response of the brain to the world around you has to do with how all these waves interact.â
To test their mathematical model of how neural waves occur in the brain, the team designed an accompanying visual experiment. Two people were asked to detect a thin faint line (âprobeâ) located on a screen and flanked by other light patterns. How well the people performed this task, the researchers found, depended on where the probe was.
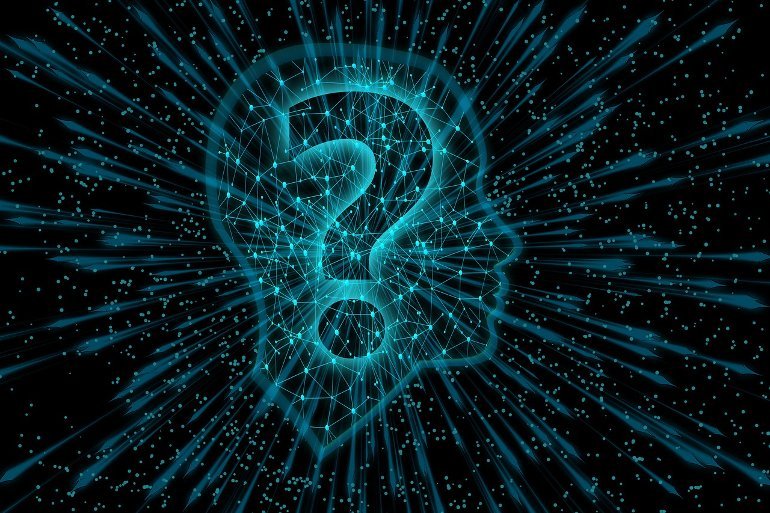
The ability to detect the probe was elevated at some locations and depressed at other locations, forming a spatial wave predicted by the model.
âYour ability to see this probe at every location will depend on how neural waves superimpose at that location,â says Gepshtein, who is also a member of Salkâs Center for the Neurobiology of Vision. âAnd weâve now proposed how the brain mediates that.â
The discovery of how neural waves interact is much more far-reaching than explaining this optical illusion. The researchers hypothesize that the same kinds of waves are being generatedâand interacting with each otherâin every part of the brainâs cortex, not just the part responsible for the analysis of visual information. That means waves generated by the brain itself, by subtle cues in the environment or internal moods, can change the waves generated by sensory inputs.
This may explain how the brainâs response to something can shift from day to day, the researchers say.
Additional co-authors of the paper include Ambarish Pawar of Salk and Sunwoo Kwon of the University of California, Berkeley.
Funding: The work was supported in part by the Salk Instituteâs Sloan-Swartz Center for Theoretical Neurobiology, the Kavli Institute for Brain and Mind, the Conrad T. Prebys Foundation, the National Institutes of Health (R01-EY018613, R01-EY029117) and the Engineering and Physical Sciences Research Council (EP/S032843/1).
About this information processing research news
Author: Salk Communications Source: Salk Institute Contact: Salk Communications – Salk Institute Image: The image is in the public domain
Original Research: Open access. “ Spatially distributed computation in cortical circuits ” by Thomas Albright et al. Science Advances
Spatially distributed computation in cortical circuits
The traditional view of neural computation in the cerebral cortex holds that sensory neurons are specialized, i.e., selective for certain dimensions of sensory stimuli. This view was challenged by evidence of contextual interactions between stimulus dimensions in which a neuronâs response to one dimension strongly depends on other dimensions.
Here, we use methods of mathematical modeling, psychophysics, and electrophysiology to address shortcomings of the traditional view.
Using a model of a generic cortical circuit, we begin with the simple demonstration that cortical responses are always distributed among neurons, forming characteristic waveforms, which we call neural waves.
When stimulated by patterned stimuli, circuit responses arise by interference of neural waves. Results of this process depend on interaction between stimulus dimensions.
Comparison of modeled responses with responses of biological vision makes it clear that the framework of neural wave interference provides a useful alternative to the standard concept of neural computation.

Two New Proteins Linked to ALS

Memory Cues May Help Reduce Alcohol Consumption

COVID’s Spike Protein Enhances Ability to Infect Brain
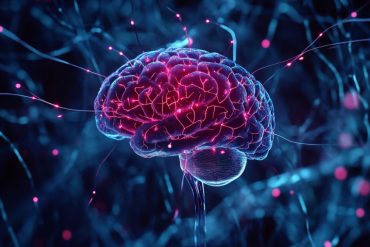
Mapping Fear: Brain Circuit That Detects and Remembers Threats Revealed
Suggestions or feedback?
MIT News | Massachusetts Institute of Technology
- Machine learning
- Sustainability
- Black holes
- Classes and programs
Departments
- Aeronautics and Astronautics
- Brain and Cognitive Sciences
- Architecture
- Political Science
- Mechanical Engineering
Centers, Labs, & Programs
- Abdul Latif Jameel Poverty Action Lab (J-PAL)
- Picower Institute for Learning and Memory
- Lincoln Laboratory
- School of Architecture + Planning
- School of Engineering
- School of Humanities, Arts, and Social Sciences
- Sloan School of Management
- School of Science
- MIT Schwarzman College of Computing
New study reveals how brain waves control working memory
Press contact :, media download.
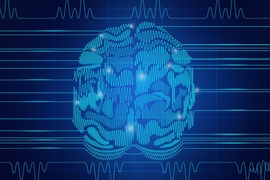
*Terms of Use:
Images for download on the MIT News office website are made available to non-commercial entities, press and the general public under a Creative Commons Attribution Non-Commercial No Derivatives license . You may not alter the images provided, other than to crop them to size. A credit line must be used when reproducing images; if one is not provided below, credit the images to "MIT."
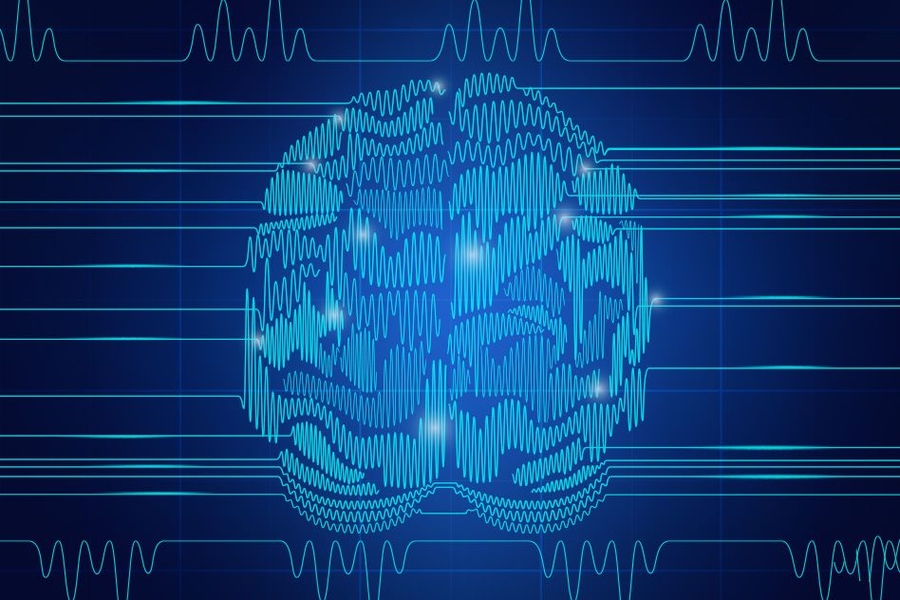
Previous image Next image
MIT neuroscientists have found evidence that the brainâs ability to control what itâs thinking about relies on low-frequency brain waves known as beta rhythms.
In a memory task requiring information to be held in working memory for short periods of time, the MIT team found that the brain uses beta waves to consciously switch between different pieces of information. The findings support the researchersâ hypothesis that beta rhythms act as a gate that determines when information held in working memory is either read out or cleared out so we can think about something else.
âThe beta rhythm acts like a brake, controlling when to express information held in working memory and allow it to influence behavior,â says Mikael Lundqvist, a postdoc at MITâs Picower Institute for Learning and Memory and the lead author of the study.
Earl Miller, the Picower Professor of Neuroscience at the Picower Institute and in the Department of Brain and Cognitive Sciences, is the senior author of the study, which appears in the Jan. 26 issue of Nature Communications .
Working in rhythm
There are millions of neurons in the brain, and each neuron produces its own electrical signals. These combined signals generate oscillations known as brain waves, which vary in frequency. In a 2016 study , Miller and Lundqvist found that gamma rhythms are associated with encoding and retrieving sensory information.
They also found that when gamma rhythms went up, beta rhythms went down, and vice versa. Previous work in their lab had shown that beta rhythms are associated with âtop-downâ information such as what the current goal is, how to achieve it, and what the rules of the task are.
All of this evidence led them to theorize that beta rhythms act as a control mechanism that determines what pieces of information are allowed to be read out from working memory â the brain function that allows control over conscious thought, Miller says.
âWorking memory is the sketchpad of consciousness, and it is under our control. We choose what to think about,â he says. âYou choose when to clear out working memory and choose when to forget about things. You can hold things in mind and wait to make a decision until you have more information.â
To test this hypothesis, the researchers recorded brain activity from the prefrontal cortex, which is the seat of working memory, in animals trained to perform a working memory task. The animals first saw one pair of objects, for example, A followed by B. Then they were shown a different pair and had to determine if it matched the first pair. A followed by B would be a match, but not B followed by A, or A followed by C. After this entire sequence, the animals released a bar if they determined that the two sequences matched.
The researchers found that brain activity varied depending on whether the two pairs matched or not. As an animal anticipated the beginning of the second sequence, it held the memory of object A, represented by gamma waves. If the next object seen was indeed A, beta waves then went up, which the researchers believe clears object A from working memory. Gamma waves then went up again, but this time the brain switched to holding information about object B, as this was now the relevant information to determine if the sequence matched.
However, if the first object shown was not a match for A, beta waves went way up, completely clearing out working memory, because the animal already knew that the sequence as a whole could not be a match.
âThe interplay between beta and gamma acts exactly as you would expect a volitional control mechanism to act,â Miller says. âBeta is acting like a signal that gates access to working memory. It clears out working memory, and can act as a switch from one thought or item to another.â
A new model
Previous models of working memory proposed that information is held in mind by steady neuronal firing. The new study, in combination with their earlier work, supports the researchersâ new hypothesis that working memory is supported by brief episodes of spiking, which are controlled by beta rhythms.
âWhen we hold things in working memory (i.e. hold something âin mindâ), we have the feeling that they are stable, like a light bulb that weâve turned on to represent some thought. For a long time, neuroscientists have thought that this must mean that the way the brain represents these thoughts is through constant activity. This study shows that this isnât the case â rather, our memories are blinking in and out of existence. Furthermore, each time a memory blinks on, it is riding on top of a wave of activity in the brain,â says Tim Buschman, an assistant professor of psychology at Princeton University who was not involved in the study.
Two other recent papers from Millerâs lab offer additional evidence for beta as a cognitive control mechanism.
In a study that recently appeared in the journal Neuron , they found similar patterns of interaction between beta and gamma rhythms in a different task involving assigning patterns of dots into categories. In cases where two patterns were easy to distinguish, gamma rhythms, carrying visual information, predominated during the identification. If the distinction task was more difficult, beta rhythms, carrying information about past experience with the categories, predominated.
In a recent paper published in the Proceedings of the National Academy of Sciences , Millerâs lab found that beta waves are produced by deep layers of the prefrontal cortex, and gamma rhythms are produced by superficial layers, which process sensory information. They also found that the beta waves were controlling the interaction of the two types of rhythms.
âWhen you find that kind of anatomical segregation and itâs in the infrastructure where you expect it to be, that adds a lot of weight to our hypothesis,â Miller says.
The researchers are now studying whether these types of rhythms control other brain functions such as attention. They also hope to study whether the interaction of beta and gamma rhythms explains why it is so difficult to hold more than a few pieces of information in mind at once.
âEventually weâd like to see how these rhythms explain the limited capacity of working memory, why we can only hold a few thoughts in mind simultaneously, and what happens when you exceed capacity,â Miller says. âYou have to have a mechanism that compensates for the fact that you overload your working memory and make decisions on which things are more important than others.â
The research was funded by the National Institute of Mental Health, the Office of Naval Research, and the Picower JFDP Fellowship.
Share this news article on:
Related links.
- Earl Miller
- Picower Institute
- Department of Brain and Cognitive Sciences
Related Topics
- Brain and cognitive sciences
- Neuroscience
Related Articles
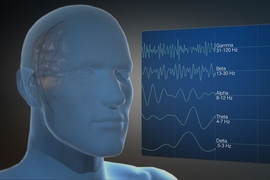
Study: Rhythmic interactions between cortical layers underlie working memory
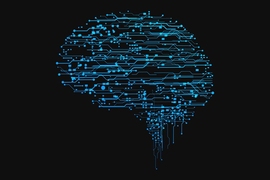
Brain waves reflect different types of learning
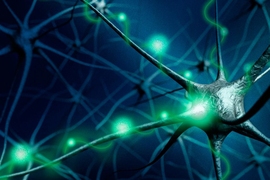
A new glimpse into working memory
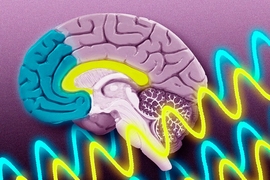
How brain waves guide memory formation
Previous item Next item
More MIT News
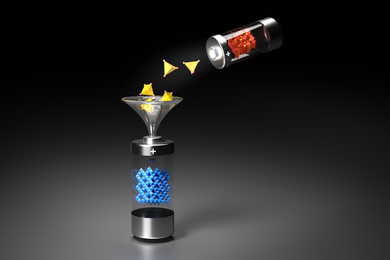
Study of disordered rock salts leads to battery breakthrough
Read full story â

Toward a code-breaking quantum computer
Uphill battles: Across the country in 75 days

3 Questions: From the bench to the battlefield
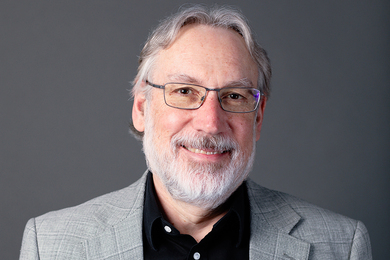
Duane Boning named vice provost for international activities

Q&A: Undergraduate admissions in the wake of the 2023 Supreme Court ruling
- More news on MIT News homepage â
Massachusetts Institute of Technology 77 Massachusetts Avenue, Cambridge, MA, USA
- Map (opens in new window)
- Events (opens in new window)
- People (opens in new window)
- Careers (opens in new window)
- Accessibility
- Social Media Hub
- MIT on Facebook
- MIT on YouTube
- MIT on Instagram
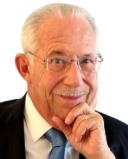
How Brain Waves Influence Your Memory
A new study discovers how the brain holds information in your working memory..
Posted June 2, 2024 | Reviewed by Lybi Ma
- Brain waves influence cognitive control and memory formation, informing how the brain manages memory.
- Working memory stores and manipulates information temporarily for tasks like learning and decision-making.
- Phase-amplitude coupling neurons synchronize with brain waves, aiding cognitive control and memory retrieval.
- New findings have implications for therapies and cognitive enhancement strategies.
Have you ever put your keys down and then completely forgotten where to find them? The brain has to work hard to protect information in your working memory from distractions. How this process works has, until recently, been unclear. A study published in Nature looked at the interactions between the front part of the brain that controls thoughts and the hippocampus, which is important for memory. They found that individual brain cells are influenced by the theta and gamma brain waves passing between these two parts of the brain and those cells have a role in cognitive control. These findings give fresh insights into how the brain manages memory, with implications for those who wish to improve their attention , decision-making , or memory retrieval.
What is working memory?
Working memory is a type of short-term memory that temporarily stores and manipulates information needed for cognitive tasks such as learning, reasoning, and comprehension. It acts as a mental workspace where information is held and processed before being encoded into long-term memory or forgotten.
Working memory is limited to holding a small amount of information (around seven items) for a brief period ranging from a few seconds to several minutes, depending on the task and the individual's cognitive abilities. Working memory coordinates the simultaneous storage and processing of information from multiple sources and plays a crucial role in higher cognitive functions like problem-solving, decision-making, and following instructions.
How does the brain make memories?
The brain makes memories through a complex process involving multiple brain regions and mechanisms. Memories are initially encoded in the hippocampus region of the brain. This process involves strengthening the connections (synapses) between neurons through repeated stimulation.
The hippocampus and the prefrontal cortex are two important brain regions in memory formation. During memory formation, the hippocampus and prefrontal cortex communicate via brain waves of different frequencies (beta and theta). Beta waves reinforce correct associations, while theta waves weaken incorrect ones, guiding what the brain learns.
The coordination of interactions between the hippocampus and prefrontal cortex is called theta-gamma phase-amplitude coupling. They work together, creating a certain rhythm. Theta waves are slower while gamma waves are faster. They are synched so that when one gets stronger, so does the other. This coordination may facilitate neural dynamics for memory and cognitive processing, integrating local sensory information processing with brain-wide cognitive control.
Studying working memory and memory retrieval
To understand the ways neurons involved in theta-gamma phase-amplitude coupling influence memory and thinking, a team of researchers at Cedars-Sinai Medical Center, Toronto Western Hospital, and Johns Hopkins Hospital, conducted a study on 36 epilepsy patients undergoing surgery for drug-resistant epilepsy using magnetic resonance imaging and electroencephalograms.
In the task, participants observed 140 rounds of different pictures. Each round started with a cross, and then one or three pictures appeared for the patient to remember. They had to hold them in memory when prompted, and later identify if a new picture matched any from that round by pressing a button.
Each session used new pictures from various categories like faces, animals, and cars, ensuring freshness. Harder rounds challenged patients with three pictures instead of one, maintaining consistent time limits for memory recall. Mixing categories prevented reliance on familiarity, encouraging active memory use over recognition.

Electrodes with at least eight wires recorded brain activity across various frequencies. The researchers examined how phase-amplitude coupling varied from trial to trial; they identified specific neurons that were sensitive to different categories of visual stimuli presented during the task that helped explain how individual neurons responded to specific types of images.
Findings and Implications
The study reveals a direct link between theta-gamma phase-amplitude coupling and the firing patterns of single neurons. Neurons showing phase-amplitude coupling synchronize with frontal theta waves, particularly when working memory load is higher, leading to faster reaction times. This suggests that phase-amplitude coupling neurons play a role in cognitive control.
To accurately retain and access memories is the main goal of cognitive control. The study demonstrated that phase-amplitude coupling neurons contribute to this process by introducing noise correlations, which improves information content at the population level. Noise correlations enhance the decodability of working memory content, particularly when involving phase-amplitude coupling neurons.
The findings of this study support a model where frontal control processes regulate working memory maintenance in brain areas like the hippocampus. The interactions between phase-amplitude coupling neurons in the broader theta-gamma phase-amplitude coupling phenomenon represent a general mechanism for top-down control in various cognitive functions beyond working memory such as attention, decision-making, speech comprehension, and long-term memory retrieval.
Understanding the memory process can lead to the development of therapies for conditions involving memory deficits or those suffering from neurological disorders. It can also lead to strategies for enhancing cognitive performance by targeting specific neural mechanisms involved in memory and cognitive control and optimizing learning and memory retention in academic settings. In summary, the study examines the connection between brain waves, cognitive control, and memory, offering promising ways to understand the neurological basis of cognition as well as opportunities to develop innovative interventions to enhance memory and cognitive function.
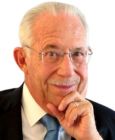
William A. Haseltine, Ph.D., is known for his pioneering work on cancer, HIV/AIDS, and genomics. He is Chair and President of the global health think tank Access Health International. His recent books include My Lifelong Fight Against Disease.
- Find a Therapist
- Find a Treatment Center
- Find a Psychiatrist
- Find a Support Group
- Find Online Therapy
- United States
- Brooklyn, NY
- Chicago, IL
- Houston, TX
- Los Angeles, CA
- New York, NY
- Portland, OR
- San Diego, CA
- San Francisco, CA
- Seattle, WA
- Washington, DC
- Asperger's
- Bipolar Disorder
- Chronic Pain
- Eating Disorders
- Passive Aggression
- Personality
- Goal Setting
- Positive Psychology
- Stopping Smoking
- Low Sexual Desire
- Relationships
- Child Development
- Self Tests NEW
- Therapy Center
- Diagnosis Dictionary
- Types of Therapy
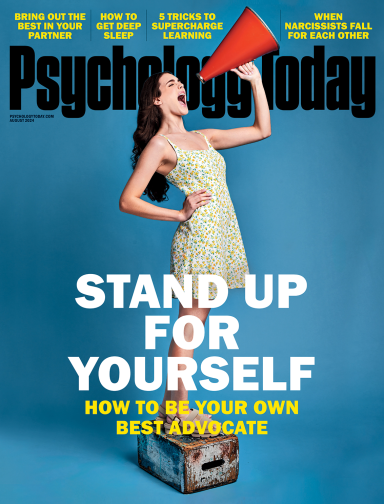
Sticking up for yourself is no easy task. But there are concrete skills you can use to hone your assertiveness and advocate for yourself.
- Emotional Intelligence
- Gaslighting
- Affective Forecasting
- Neuroscience
Thank you for visiting nature.com. You are using a browser version with limited support for CSS. To obtain the best experience, we recommend you use a more up to date browser (or turn off compatibility mode in Internet Explorer). In the meantime, to ensure continued support, we are displaying the site without styles and JavaScript.
- View all journals
- Explore content
- About the journal
- Publish with us
- Sign up for alerts
- Open access
- Published: 10 December 2021
Study of EEG characteristics while solving scientific problems with different mental effort
- Yanmei Zhu 1 , 2 ,
- Qian Wang 2 &
- Li Zhang 1 Â
Scientific Reports volume  11 , Article number: 23783 ( 2021 ) Cite this article
4758 Accesses
14 Citations
Metrics details
- Neuroscience
Studying the mental effort in problem-solving is important to the understanding of how the brain allocates cognitive resources to process information. The electroencephalogram is a promising physiological approach to assessing the online mental effort. In this study, we investigate the EEG indicators of mental effort while solving scientific problems. By manipulating the complexity of the scientific problem, the level of mental effort also changes. With the increase of mental effort, theta synchronization in the frontal region and lower alpha desynchronization in the parietal and occipital regions significantly increase. Also, upper alpha desynchronization demonstrates a widespread enhancement across the whole brain. According to the functional topography of brain activity in the theta and alpha frequency, our results suggest that the mental effort while solving scientific problems is related to working memory, visuospatial processing, semantic processing and magnitude manipulation. This study suggests the reliability of EEG to evaluate the mental effort in an educational context and provides valuable insights into improving the problem-solving abilities of students in educational practice.
Similar content being viewed by others
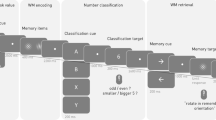
Task prioritization modulates alpha, theta and beta EEG dynamics reflecting proactive cognitive control
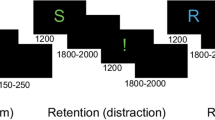
Salient distractors open the door of perception: alpha desynchronization marks sensory gating in a working memory task
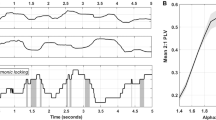
From thoughtless awareness to effortful cognition: alpha - theta cross-frequency dynamics in experienced meditators during meditation, rest and arithmetic
Introduction.
Mental effort represents the quantity of cognitive resources involved in performing a task 1 , 2 , 3 . It is seen as a combination of the perceived demand characteristics, depth of information processing, and personal expertise 1 , 4 . Perceived demand characteristics mainly depend on the inherent complexity of the task content, which is related to the degree of interaction between various information elements 4 . When element interaction is low, task information can be extracted and learned for one single element at a time since individual elements have minimal references to one another. On the other hand, highly interacting information consists of elements that heavily interrelate, which must be combined and processed simultaneously. Accordingly, the more the number of interacting information a task contains, the higher the cognitive demand and mental effort it elicits 5 . Depth of processing refers to the degree to which a person encodes information. Deeper processing implies a greater degree of semantic or cognitive analysis of input for meaning extraction and comprehension with their existing knowledge, while surface level processing is more concerned with the recognition of physical or sensory features of the stimulus 6 . Thus, deeper processing of presented information requires higher mental effort than a more surface level encoding. Furthermore, developed expertise resulting from repeated practice lowers the mental effort imposed by a familiar task 7 , 8 . Studying the mental effort in problem-solving helps to determine the online processes while individuals are working on these problems and provides a deep understanding of their learning outcomes 9 , 10 . It could also provide implications for the development of effective instructions to improve their learning performance. For example, researchers have found that lead-augmented hypertext system improves the learning performance by visualization of interacting information elements as one undivided unit 5 . This system decreases the mental effort required for the integration of the hypertext nodes with semantic space. However, many of the previous studies usually explored the mental effort imposed by well-controlled working memory tasks or other problems which required little scientific knowledge. Compared with these tasks, scientific problems require the abstract scientific conception and involve complex cognitive processes such as model representation, retrieval of scientific conception and inference calculation. Solving scientific problems will involve a set of brain resources and consequently a high level of mental effort. To our knowledge, mental effort during scientific problem solving is still seldom reported. Research on mental effort in scientific problem solving may shed light on scientific reasoning of students and suggest ways to improve their problem-solving ability in science education.
Various methods, including subjective measures, secondary task measures, and physiological measures, have been applied to the assessment of mental effort 11 , 12 , 13 . Subjective measures evaluate the mental effort by using the subjective rating scales 14 . Secondary task measures estimate the mental effort for the primary task according to accuracy and response time on the secondary task 15 . Physiological measures quantify the mental effort by measuring a variety of physiological factors such as heart rate variability, eye movement and brain activity 16 , 17 , 18 , 19 , 20 . Both subjective and secondary task measures do not allow for a continuous and noninvasive measurement of mental effort. The participant has to interrupt the main task to fill a questionnaire or to perform a secondary task during the main one. In contrast, physiological measures can continuously and objectively monitor online mental effort without interfering with task performance 21 , 22 . Also, physiological measures vary predictably in response to changes of mental state 23 . It can be used to anticipate a mental impairment such as cognitive overload and fatigue 24 , 25 . However, through subjective and secondary task measures, due to lack of the capacity to monitor covert changes in psychophysiological state, it is only possible to detect a mental impairment once it already happened. Moreover, some studies have proved that physiological measures provide higher sensitivity in discriminating different levels of mental workload compared to subjective and secondary task measures 26 , 27 . In these studies, physiological variations reliably represent implicit fluctuations in the mental states, suggesting that physiological measures have sufficient stability and sensitivity to distinguish between different degrees of mental effort.
The electroencephalogram (EEG) is a promising physiological approach to the assessment of the mental effort of students in an educational context 28 , 29 . It can directly monitor an individualâs cognitive state by recording the electrical signals produced by the brain in an authentic environment. Previous studies have examined changes in EEG signals as a function of mental effort 30 , 31 , 32 , 33 . Researchers pointed out that brain activity in the theta and alpha frequency is sensitive to effortful processing 34 , 35 , 36 , 37 . This result was found in the studies about the electrophysiological indicators of mental effort. Most studies that apply the well-controlled working memory tasks, such as N-back tasks or spatial and verbal working memory tasks, have reported both alpha and theta effects on cognitive effort. With the enhancement in memory load due to increased task difficulty, an increase in frontal theta power and decrease in parieto-occipital alpha power are usually observed 38 , 39 , 40 , 41 , 42 , 43 . Research using multitasking also revealed a strong association between mental effort and oscillations in the theta and alpha frequency. They found that a growth in the number of subtasks the participants performed simultaneously increased the amount of theta activity in the frontal region and decreased alpha activity in the parietal region 44 . In addition, a similar pattern was found in studies using arithmetic problems or some real-world simulation tasks like air traffic control 28 , 45 . These studies have shown that changes in the frontal theta and posterior alpha activity are reliable indicators of mental effort elicited by tasks of varying complexity.
Compared with the tasks in the previous work, scientific problems require abstract scientific conception and involve complex cognitive processes. Solving scientific problems activate a set of brain resources and a high level of mental effort. In this case, a refinement of EEG mental effort measure proposed by Smith and Gevins should be considered 46 . Their EEG workload model includes quantification of alpha and theta band activity recorded from the frontal brain area essential for working memory and executive control, centro-parietal area essential for visuospatial processing, occipital area essential for stimulus encoding and semantic memory processing. Changes in EEG-derived regional indices could provide information about the relative activation of different local cortical regions in response to increases in mental effort. Based on these regional indices and specialization of the underlying cortical regions, neurologically meaningful segregation of task effects on mental effort can be speculated. Using this model, Smith and Gevins identified the different levels of mental workload in a flight simulator task. The mental state of an individual, whether in a well-rested state or following a total sleep deprivation, can be determined according to regional indices reflecting both task demands and operatorâs state of alertness 46 .
In this study, we attempt to investigate the EEG indicators of mental effort during scientific problem-solving. To this end, we asked students to solve the scientific problems commonly used in the practice of science education while recording their EEG signals. By manipulating the complexity of the scientific problems, students engaged in different levels of mental effort. Their EEG signals during problem-solving were examined. We focused on analyzing the EEG activity in the theta and alpha frequency, because these two bands are particularly important indicators of effortful processing. Besides, brain activity in the lower and upper alpha frequency has been documented to be associated with differential cognitive effects. The lower alpha changes are considered to reflect general task demands, such as attentional processes, while the upper alpha changes are suggested to reflect the task-specific processes such as stimulus encoding, semantic processing and memory access 42 . Although these two alpha bands have different functional specializations, many previous studies employed the entire alpha band to explore EEG indicators of mental effort 44 , 47 , 48 . Some studies used simple working memory tasks to calculate the changes in the lower and upper alpha frequency. They found that the two alpha bands seemed to respond quite similarly in simple tasks 49 . Compared with simple tasks, solving scientific problems involves more complex cognitive processes including attention, working memory, visual processing, semantic memory and multisensory integration. We expected that task-general and task-specific processes during scientific problem solving would be reflected by the lower and upper alpha activity, respectively. For this consideration, both lower and upper alpha bands were examined in this study. We hypothesized that functional specialization of these two alpha oscillations would appear in our task requirements. They would reflect the neural responses to mental effort imposed by the particular cognitive processes while solving scientific problems. According to the functional topography for theta and alpha oscillations, we expected that mental effort imposed by general attention would be associated with posterior lower alpha activity, mental effort by working memory and executive function would be reflected by theta and upper alpha activity in the frontal area, mental effort by task-specific processes of visual processing, semantic processing and multisensory integration would be reflected by upper alpha activity in the occipital and centro-parietal areas. Moreover, with the increase of mental effort, theta oscillation would increase while both alpha bands would decrease.
Behavioral results
Mean reaction time, response accuracy and subjective effort evaluation for the low and high complexity problems are shown in Table 1 and Fig. 1 . In our study, students gave 9.6â±â0.6 and 7.4â±â1.6 correct trials for the low and high complexity problems, respectively. Wilcoxon signed-rank test reveals the main effect of problem complexity condition ( Z â=âââ4.37, p â<â0.001, for reaction time; Z â=âââ4.04, p â<â0.001, for response accuracy; Z â=âââ4.29, p â<â0.001, for subjective effort evaluation). The results reflect that high complexity problems are more demanding than the low complexity ones. Students spent more time to solve these problems, but the response accuracy declined significantly. In addition, during the interview session, students responded that these high complexity problems required more effort. All the behavioral results support that our scientific problem design and complexity categorization are reliable. The experiment successfully engaged the students in solving scientific problems with different levels of mental effort.
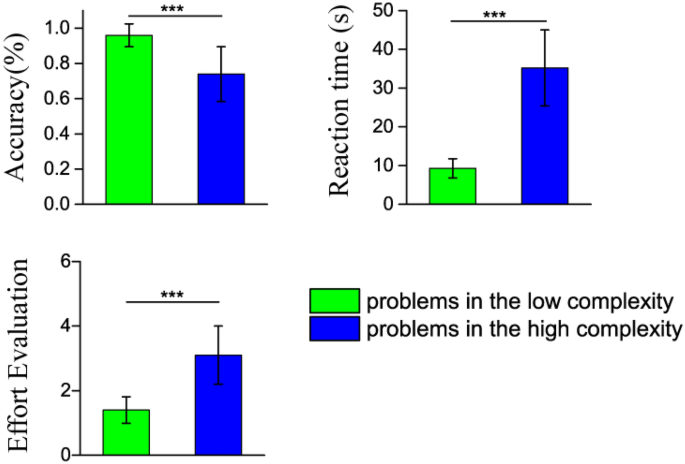
Mean reaction time, response accuracy and subjective effort evaluation for the low and high complexity problems. The behavioral results support that our scientific problem design and complexity categorization are reliable. The experiment successfully engaged the students in solving scientific problems with different levels of mental effort. *** p â<â0.001.
Electrophysiological results
Figure 2 gives the neurophysiological results. Figure 2 a illustrates the topographic maps of theta ERS and alpha ERD for the two conditions of problem complexity. Figure 2 bâd gives the statistical results of theta ERS, lower alpha band ERD, and upper alpha band ERD in the different brain regions, respectively. The results indicate that theta activity increases, and alpha activity decrease during scientific problem solving. In addition, the amplitudes of theta ERS and alpha ERD vary across brain regions between the two problem complexity conditions.
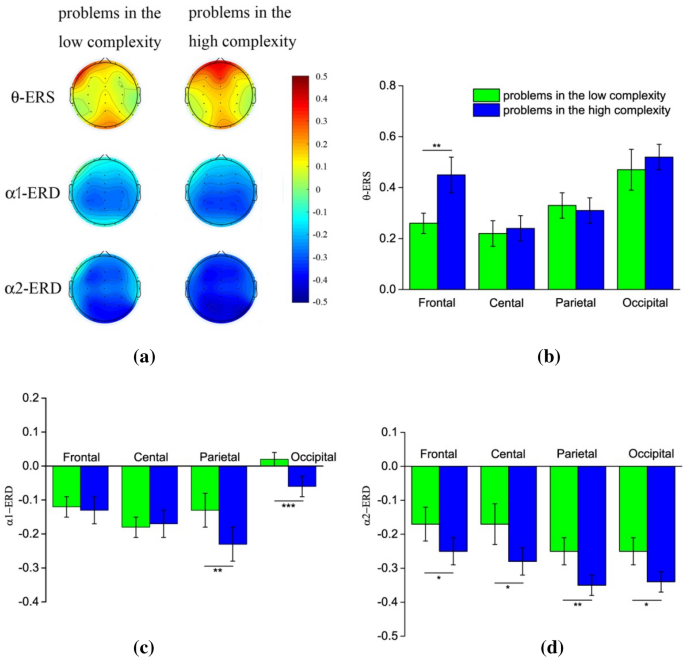
ERS/ERD in the theta and alpha bands while solving the low and high complexity scientific problems. α1 refers to lower alpha band, α2 refers to upper alpha band. With the increase of mental effort, the amplitudes of frontal theta ERS and posterior lower alpha band ERD increase, while the amplitude of upper alpha band ERD demonstrates an extensive enhancement throughout the whole brain. ( a ) Topographical maps of theta ERS and alpha ERD for the two complexity conditions. ( b ) Theta ERS for the two complexity conditions in four brain regions. ( c ) Lower alpha band ERD for the two complexity conditions in four brain regions. ( d ) Upper alpha band ERD for the two complexity conditions in four brain regions.
For the theta ERS, as shown in Fig. 2 b, a two-way repeated measures ANOVA obtains the significant main effect of brain area [ F (3,72)â=â6.98, p â<â0.01, Æ 2 â=â0.23]. The theta ERS in the frontal and occipital regions are significantly higher than those in the central and parietal regions (both p â<â0.05). The results also reveal the significant interaction of the brain area and problem complexity condition [ F (3,72)â=â24.13, p â<â0.001, Æ 2 â=â0.51]. Simple effect analysis reflects that theta ERS tends to be higher for the high complexity scientific problems than the low complex problems in the frontal region ([ F (1,24)â=â14.84, p â<â0.01]). There is no significant difference in theta ERS for two complexity conditions in other brain regions (all p â>â0.05).
For the lower alpha band ERD, as shown in Fig. 2 c, the statistical results reveal a significant main effect of brain area [ F (3,72)â=â9.08, p â<â0.001, Æ 2 â=â0.28], with a significantly stronger lower alpha band ERD at the central and parietal regions compared to the frontal and occipital regions (both p â<â0.05). The results also show a significant interaction of brain area and problem complexity condition [ F (3,72)â=â5.38, p â<â0.001, Æ 2 â=â0.18]. Simple effect analysis shows a stronger lower alpha ERD in the parietal ([ F (1,24)â=â13.03, p â<â0.01]) and occipital regions ([ F (1,24)â=â24.58, p â<â0.001]) when solving the high complexity problems, in comparison to low complexity problems. Lower alpha band ERD has no significant difference between two complexity conditions in other brain regions (all p â>â0.05).
For the upper alpha band ERD, as shown in Fig. 2 d, the results show a significant main effect of problem complexity condition [ F (1,24)â=â6.48, p â<â0.05, Æ 2 â=â0.21]. Upper alpha band ERD is much stronger for the high complexity problems. The main effect of brain area as well as the interaction of the brain area and problem complexity are both not significant (both p â>â0.05). This result suggests a significant global suppression of upper alpha activity when solving high complexity scientific problems. Paired- t test was conducted on the amplitude of upper alpha band ERD between the low and high complexity condition. The results confirm that upper alpha band ERD is significantly stronger across all brain regions ( p â<â0.05 in the frontal, central and occipital regions; p â<â0.01 in the parietal region.)
In this study, we investigate the EEG characteristics of different mental effort in solving scientific problems. By manipulating the complexity of the problem, students engaged in tasks with different levels of mental effort. Both behavioral data and interview reports show that they spent more mental effort in dealing with the high complexity scientific problems. The different amounts of mental effort are accompanied by theta ERS and alpha ERD, which exhibit the distinct topographical distribution. Particularly, an increase in mental effort significantly enhances the amplitude of frontal theta ERS, parietal and occipital lower alpha band ERD, and brain-wide upper alpha band ERD. These task-induced EEG changes reflect the mental resources required for the specific cognitive processes involved in solving scientific problems.
Brain activity in the frontal theta increases while solving scientific problems
Our findings show that frontal theta activity increases with the enhanced mental effort while solving scientific problems. Frontal theta oscillations are usually observed in EEG studies that use working memory tasks, multiple tasks, and other tasks involving executive functions. The results show that frontal theta activity is positively correlated with task demands on memory load and executive function 35 , 38 , 39 , 40 . Some studies have even found that the degree of theta synchronization is a neural signature of successful information manipulation 50 . Recently, researchers employed the real-world simulation tasks such as flight and air traffic management to investigate the EEG indicators of mental load 25 , 45 . They also found that as cognitive demands on attention, working memory load and task control increase, the enhanced mental effort leads to an increase in the frontal theta activity. These previous studies revealed that frontal theta synchronization is a reliable indicator of mental effort elicited by working memory and executive function.
Consistent with the previous studies, our results further demonstrate that the frontal theta synchronization becomes stronger as mental effort increases when solving the high complexity scientific problems. It suggests that more working memory and control capacity are allocated to accomplish these problems. This result is reasonable since more information and deeper processing are involved in high complexity scientific problems. These problems involve two or more objects. The forces acting on an object will be affected by others as well as the motion state of the system. Students need to analyze each object and the entire system at the same time. Meanwhile, they need to retrieve the physics concepts and apply them to analyze the forces acting on the objects. More interactive elements and greater control requirements expand the amount of information maintained, manipulated and controlled in working memory. Therefore, more mental effort is recruited. In contrast, for the scientific problems with the low complexity, only one object is involved, and there is no complex interaction between objects. Furthermore, in educational practice, it is common to determine the forces acting on a single object in the system. Fewer information elements and the developed expertise make the reasoning process in working memory more automated, thereby reducing online mental effort of the task. Our results suggest that frontal theta activity can reliably reflect the mental effort assigned to working memory and control processes when solving abstract scientific problems.
Brain activity in the alpha frequency decreases while solving scientific problems
In our study, both the lower and upper alpha activity decrease with the increase of mental effort while solving scientific problems. Alpha oscillations are usually found to be negatively correlated with effortful processing 27 , 42 . Alpha desynchronization is suggested to represent an intensified recruitment of relevant mental resources when task demands require more cognitive processing to perform it 51 . Studies on visual or auditory information processing, sensorimotor processing, multitasking and reasoning have proven that the quantification of alpha ERD is a particularly useful and appropriate method for measuring the level and topographical distribution of cortical activation during cognitive task performance. For example, alpha ERD is maximal in the occipital region during the visual task, maximal in the temporal region during the auditory task, and maximal in the centro-parietal region during sensorimotor task 44 , 52 , 53 , 54 , 55 . Moreover, the amplitude of alpha ERD becomes more prominent when task difficulty increases. Besides, brain activity at the lower and upper alpha frequency has been documented to reflect different cognitive demands. Desynchronization of lower alpha has been observed in responses to almost any type of task, and is believed to represent the general task requirements for basic arousal and attentional processing 42 . The ERD map consistently displays that the lower alpha desynchronization is the largest in the parietal area 42 , 56 . Moreover, it is observed that the lower alpha is highly sensitive to practice 37 . Generally, the amplitude of lower alpha activity increases significantly with the increase of practice, suggesting that performance requires lesser mental resources after the individual has completed the task proficiently. In contrast, desynchronization of upper alpha usually emerges at task-relevant sites and is considered to reflect specific task requirements, such as stimulus encoding, semantic processing and memory access 56 , 57 , 58 , 59 . Although these two alpha bands have different functional specializations, many previous studies employed the entire alpha band to explore EEG indicators of mental effort 47 , 48 . Some studies have calculated changes in the lower and upper alpha bands, but used simple tasks such as working memory tasks. They found that the two alpha bands seem to respond very similarly to mental effort in simple tasks 49 . In our study, considering that more complex cognitive processes are involved in solving scientific problems, we calculated the brain activity in the lower and higher alpha band respectively. These two alpha bands demonstrated the different effects on mental effort allocated by the abstract scientific problems.
In line with the previous studies, we found that sensitivity of online mental effort in the lower alpha band located in the parietal and occipital brain areas during solving scientific problems. According to the cognitive effects of lower alpha activity, ERD in the parietal area suggests that more general alertness and attention are imposed on stimulus representation, while ERD in the occipital region reflects that more visual attention is required for stimulus sensory processing 60 , 61 . It is reasonable because more complex visual elements are included in these problems. In addition, compared with the progressive automation of single-object problems in educational practice, complex scientific problems still require sustained and focused attention. Accordingly, the greater amplitude of lower alpha band ERD in the posterior region suggests that a higher level of mental effort is required to gain general attention when solving complex scientific problems.
Different from the previous studies which showed similar activation patterns between lower and upper alpha activity due to the application of simple tasks, we observed a distinct topographical distribution of upper alpha band ERD compared to that of lower alpha band. In our study, the upper alpha band ERD is enhanced throughout the whole brain areas with the increase of mental effort. Our results support the view of functional differences between different alpha bands, and are also in line with the statement that the functional specialization of the lower and upper alpha bands is most prominent in the frontal and central regions as the task demands increase 42 .
It is commonly observed that upper alpha band ERD is the largest at occipital site when processing various visual stimuli 56 . In these visual tasks, researchers found that desynchronization started over occipital site in the upper alpha band, followed by a parietal localized ERD in the lower alpha band. They explained that the early upper alpha band ERD at occipital site reflects visual encoding of the stimulus, including feature extraction, stimulus identification, semantic encoding and memory access, while the following parietal ERD in the lower alpha band reflects attention on encoded visual stimulus 56 . Other studies using semantic processing tasks consistently found that ERD in the occipital region is the most sensitive to the search and retrieval processes of semantic information, and is significantly correlated to the performance of semantic memory 57 . These results reflect that upper alpha in the occipital site is indicative of visual stimulus encoding and semantic memory processing. In our study, students not only need to encode the symbolic diagram and make a mental representation of current information, but also need to retrieve the physics conception from long-term memory and integrate scientific law with information presented in the problem. Mental resources for stimulus encoding and semantic memory processing are particularly higher for the complex scientific problem because it involves interactive objects in the diagram and requires deeper processing for meaning extraction and comprehension. We suggest that this heavier mental effort would be reflected by the stronger upper alpha band ERD in the occipital region.
Upper alpha band ERD in the centro-parietal regions is reported to be essential for visuospatial processing and mathematical ability 54 . It is commonly observed that centro-parietal alpha power decreases in tasks with visually presented stimuli. Several studies have demonstrated that generation of ideas in the figural domain is related to the strong decrease in the upper alpha activity at parietal site, which reflect the high visuospatial processing requirements during mental generation and manipulation of visual representation 62 , 63 . In the field of mathematics, researchers found that the parietal site supports magnitude manipulations in arithmetic tasks 64 . In addition, ERD results revealed that applying procedure strategy to solve more difficult arithmetic problems causes much stronger activation in the parietal regions than using strategy that retrieves answers directly form long-term memory for easier problems 65 . Accordingly, we suggest that the stronger upper alpha band ERD in the centro-parietal region indicates the greater mental effort for visuospatial processing of symbolic diagram in the high complexity problem. Quite similar to the difficult arithmetical problems, complex scientific problems also require a procedure strategy to analyze the forces on each object, rather than directly retrieving the answer from long-term memory. In other words, students need to first analyze the forces based on the motion state of the entire system, and then consider the forces exerted on each object according to its motion state as well as the interaction between adjacent objects. In this case, magnitude manipulations are also involved to calculate and determine the forces due to interaction between objects. The mental effort allocated by these cognitive processes would be reflected by the centro-parietal upper alpha desynchronization.
Frontal upper alpha desynchronization is usually used to examine higher order cognitive functions of executive processes, such as inhibition, and mental manipulation of information 55 , 66 . These cognitive processes are important for learning, reasoning, and comprehension. Stronger frontal upper alpha band ERD suggests that greater mental effort is allocated by executive function and working memory demands in task performance. Researchers further suggest that upper alpha activity at frontal site reflects the degree of executive control of anterior brain region over more posterior regions responsible for cognitive processes including information encoding, memory tracing, visuospatial processing and other mental operations 55 . For example, the activation of frontal brain area of a skilled chess player is lower than that of a lower-skilled player, which suggests that training or practice reduces the need for frontal executive functions since information processing in the posterior regions becomes more automated 55 . These previous investigations reveal that ERD in the frontal upper alpha band reflects mental operation of information and the executive control of cognitive processing. We speculate that the stronger frontal upper alpha ERD is indicative of mental effort exerted by higher executive function and working memory demands to reason the high complexity scientific problems. For these problems, students not only require more mental effort on mental operations of interactive information, but also need more anterior control over relative posterior brain regions responsible for specific cognitive processes.
In summary, we investigated online mental effort in solving scientific problems using the EEG. We suggest that the increase in the frontal theta activity and decrease in the anterior upper alpha activity reflect the mental effort allocated by working memory and cognitive control. The decrease in the centro-parietal upper alpha activity reflects the mental effort imposed by visuospatial processing and magnitude manipulation of information in the scientific problems. The decrease in the upper alpha activity in the occipital area reflects the stimulus encoding and semantic processing of scientific conceptions. The task-induced EEG changes suggest the mental resources required for the specific cognitive processes involved in solving scientific problems. It can provide a deep understanding of studentsâ learning outcomes. It will also provide implications for the development of effective guidance to improve their ability to solve scientific problems in educational practice.
Participants
Twenty-five healthy students majoring in engineering or science (mean ageâ=â23â±â1.8 years old, 12 males and 13 females) from Southeast University participated in this study. No physics department students were recruited. All of the participants were right-handed, had normal or correct-to-normal vision, reported no history of psychiatric or neurological disorders, and were not taking any medications. Each participant signed a written informed consent before the experiment and received monetary compensation for participation. Ethical approval for the study was obtained from the ethics committee of Southeast University. All procedures were conducted in accordance with approved guidelines and regulations.
Scientific problems
The scientific problems used in our study are items to analyze the forces acting on objects in various states of motion. Force analysis plays an important role in science learning and is a fundamental step in solving many physics problems. However, it is usually a challenging for students to conduct force analysis, especially for the objects in complex motion state. To solve these problems, students required to analyze the interaction between objects, retrieve scientific conceptions about the relationship between force and motion, as well as make inferences about presented information based on these conceptions. Cognitive resources across various brain regions were recruited and task-related mental effort were allocated. As widely used in science education practice, the problems used in our study were presented as the symbolic diagrams. These symbolic diagrams conveyed precise meanings and combined with rules of force and motion that must be used correctly 67 .
The whole task consisted of 24 scientific problems which were divided into two complex conditions. For the low complexity problems, only one object was considered. The forces acting on the object would be gravity, friction depending on the roughness of the contact surface, the supporting force of the surface, and the pulling or pushing force applied on it if present. For the high complexity problems, two or more objects were involved, so the interaction between the objects had to be carefully analyzed. Typical examples of the scientific problems in the low and high complexity conditions are illustrated in Fig. 3 . The final 24 scientific problems were selected from a bank of 100 force analysis items. The evaluation procedure was conducted by a committee composed of three experienced physics teachers and two university professors from the physics department to ensure the validity of the complexity classification. In the experiment, the students were asked to determine the total amount of force acting on an object. We assumed that the high complexity problems were more demanding and required higher mental effort. We also expected that the different amounts of online mental effort in solving scientific problems would be reflected by EEG signals. In the experiment, we also developed items as controls, in which students were only required to count the number of objects in a particular shape.
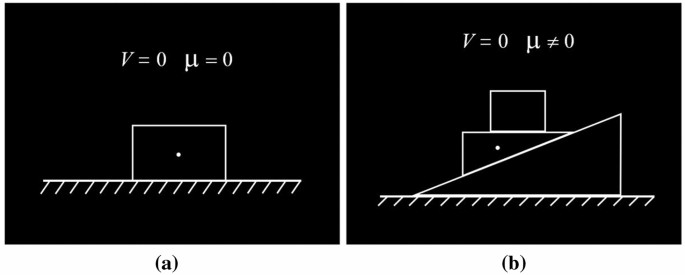
Examples of scientific problems presented in the task. Problems are illustrated as the symbolic diagrams commonly used in science education practice. Students are asked to determine the number of forces acting on the object marked by the small white dot. The velocity of the object ( V ) and the friction of contacting surfaces ( Ό ) are shown in the diagram. ( a ) Low complexity scientific problem. ( b ) High complexity scientific problem.
Experimental procedures
A total of 48 stimuli including 24 scientific problems and 24 control items were presented using E-prime 2.0. The stimuli were presented according to the event-related design, as shown in Fig. 4 . Each trial started with the presentation of a central white fixation cross on the black screen for 3000 ms. Then a scientific problem was presented and the participants were required to think silently about the forces acting on the object which was marked with a small white dot. The problem remained on the screen until the participant got the answer and requested the answer options by keystroke (Reaction 1). After that, the scientific problem disappeared from the screen and the answer options were presented. The participant selected the answer from six options by pressing the corresponding reaction button (Reaction 2). Finally, a subjective rating scale was presented to assess the level of effort put into the problem, and the participant responded by pressing the respective button (Reaction 3). After the scientific problem was presented, a control item was showed. An inter-trial interval of 3000 ms was designed as a resting period before presenting the next trial. The purpose of the application of control stimuli was to reset the mental activity of the participant to normal levels and eliminate any stressful effects of the previous scientific problem. Only EEG signals during solving scientific problems were analyzed.
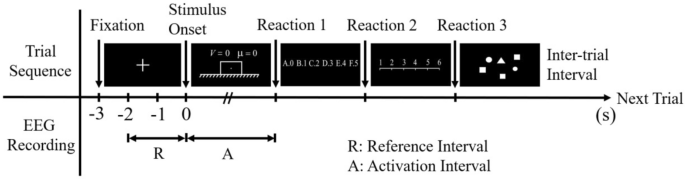
Schematic of a single trial. The time period from the onset of a scientific problem to reaction 1 serves as the activation interval, the time period from 1 to 3Â s after the onset of the fixation cross serves as the reference interval.
The participants sat comfortably about 75Â cm away from the screen to perform the task and were asked to pay attention to the task while keeping still. Two scientific problems of low and high complexity were used in the practice session for the familiarity of the experiment. The remaining problems were divided into two blocks in the formal experiment procedure. The scientific problems of varying levels of complexity were randomly presented and equally distributed between each block. The participantsâ EEG signals in the task were recorded at the same time. After the EEG recording session, participants were interviewed to explain how they solved each scientific problem and how hard they worked on it during the task.
Behavioral data analysis
The behavioral data including reaction time, response accuracy and subjective effort evaluation were recorded by E-prime software. In our study, the reaction time was identified as the time interval between onset of scientific problem and their keystroke for reaction 1. The correctness of response to each question is determined according to their keystroke of answer options for Reaction 2. Subjective effort evaluation was obtained according to their keystroke of Reaction 3. The behavioral data for each student was first averaged for problems in the low and high complexity respectively. Then, the mean and standard deviation of each behavioral data were calculated across all students for each problem condition.
We first performed the ShapiroâWilk normality test for each behavioral data. It revealed that the data did not conform to a normal distribution (all p â<â0.05). Accordingly, we conducted Wilcoxon signed-rank test to analyze the main effect of problem complexity condition on each behavioral data.
We further compared behavioral data between correct and incorrect trials for high complexity problems. For reaction time of correct and incorrect responses, wilcoxon signed-rank test revealed that reaction time of incorrect trials was comparable to that of correct trials ( Z â=âââ0.44, p â=â0.67). The same analysis was conducted to subjective evaluation of mental effort, which also showed no significant difference between two kinds of conditions ( Z â=âââ0.97, p â=â0.33). Additionally, in the interview session, the students also expressed that the amount of effort to solve these difficult problems was high no matter they successfully solved it or not. All these results suggested that the students spent a similar amount of effort to solve the high complexity problems even though they may not obtain the right answer for certain problem. For this reason, we included all trials for further EEG data analysis.
EEG recording and data analysis
EEG was recorded from 32 tin electrodes mounted on an elastic cap according to the international 10â20 system (NeuroScan Inc., Herndon, VA, USA). The electrooculography (EOG) was recorded from two electrodes on the canthi and two electrodes located above and below the right eye. All electrode impedances were maintained below 5 KΩ. EEG and EOG signals were continuously sampled at 500 Hz for off-line analysis. The EEG data were band-pass filtered between 0.5 Hz and 60 Hz. Ocular artifacts were first corrected with an eye-movement correction algorithm which employed a regression analysis in combination with artifact averaging 68 . The continuous EEG data were segmented into epochs covering reference interval and activation interval in each trial. To further remove possible artifacts, the data were submitted to Independent Component Analysis (ICA) using the runica function from the EEGLAB toolbox to clear visible artifacts, such as the components of possible ocular and muscle movements.
Considering that brain activity varies individually, event-related synchronization/ desynchronization (ERS/ERD) of EEG signals was quantified to measure cortical activation and topographical distribution in scientific problem-solving 52 . The amount of ERS/ERD at a given frequency band is defined as the percentage of power increase/decrease during the activation interval relative to the reference interval. The activation interval refers to the time period while working on a task, and the reference interval refers to a pre-stimulus time period without any task demands. In our study, the time period from the onset of a scientific problem to reaction 1 served as the activation interval, and time period from 1 to 3 s after the onset of the fixation cross served as the reference interval, as shown in Fig. 4 .
Figure 5 illustrates the steps of EEG signal processing. For each epoch, EEG time series in the reference and activation intervals were converted into the frequency domain using a fast Fourier transformation (FFT) with a sliding 500 ms window by a step of 25 ms. Therefore, each window overlapped the previous one by 475 sample points. Band power in theta (4â7 Hz), lower alpha (8â10 Hz) and upper alpha (10â12 Hz) frequencies were calculated, respectively. The percentage change in the frequency band power from the activation interval to reference interval in each trial was then quantified. Finally, the calculated change in band power was averaged across all trials for low and high complexity conditions respectively. A positive value represents ERS and a negative value suggests ERD. For statistical analyses, ERS/ERD data were aggregated over different electrode locations as shown in Fig. 6 : frontal (FP1, FP2, F3, Fz and F4), central (C3, Cz and C4), parietal (P3, Pz, and P4) and occipital (O1, Oz, and O2).
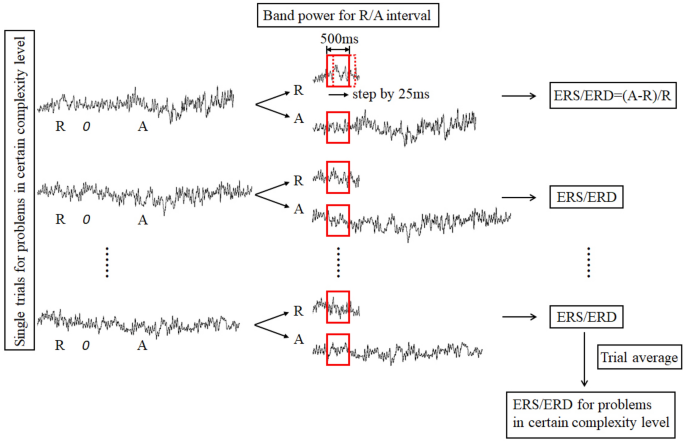
Steps of EEG signal processing to calculate ERS/ERD values.
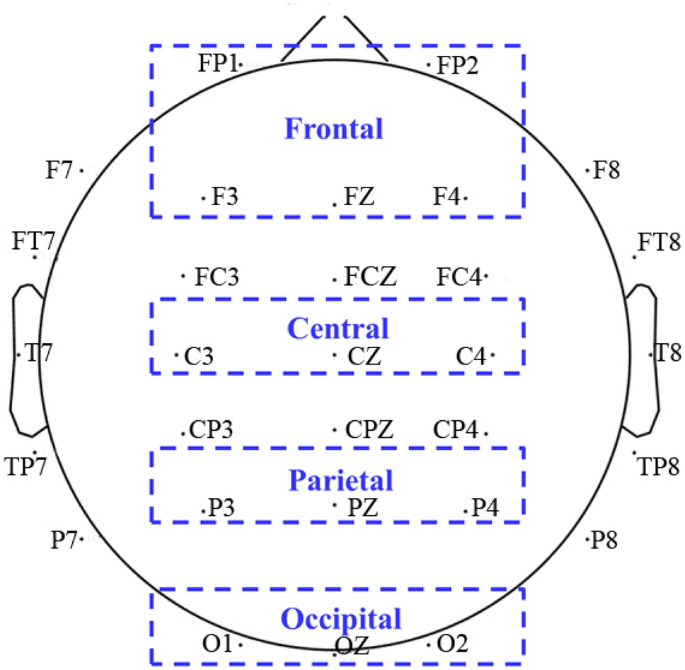
Division of the brain regions and their included electrodes for ERS/ERD calculation. Frontal (FP1, FP2, F3, Fz and F4), Central (C3, Cz and C4), Parietal (P3, Pz, and P4) and Occipital (O1, Oz, and O2).
For statically analysis, problem complexity condition (low and high complexity) and brain area (frontal, central, parietal and occipital areas) are two within-subjects variables. Therefore, we first conducted the ShapiroâWilk normality test on each set of EEG data. The statistical results revealed that each set of EEG data was normally distributed (all p â>â0.05). Then, we performed the two-way repeated measures ANOVA on ERS/ERD data to analyze the main and interaction effects of problem complexity and brain area. GreenhouseâGeisser correction was applied to correct for violations of the sphericity assumption, all post-hoc tests were bonferroni-corrected.
Kirschner, P. A. & Kirschner, F. Mental effort. In Encyclopedia of the Sciences of Learning 2182â2184 (Springer, 2012).
Chapter  Google Scholar Â
Causse, M., Chua, Z. R., Peysakhovich, V., Del Campo, N. & Matton, N. Mental workload and neural efficiency quantified in the prefrontal cortex using fnirs. Sci. Rep. 7 , 5222 (2017).
Article  ADS  PubMed  PubMed Central  Google Scholar Â
Zammouri, A., Moussa, A. A. & Mebrouk, Y. Brain-computer interface for workload estimation: Assessment of mental efforts in learning processes. Expert Syst. Appl. 112 , 138â147 (2018).
Article  Google Scholar Â
Van Merrienboer, J. J. & Sweller, J. Cognitive load theory and complex learning: Recent developments and future directions. Educ. Psychol. Rev. 17 , 147â177 (2005).
Antonenko, P. D. & Niederhauser, D. S. The influence of leads on cognitive load and learning in a hypertext environment. Comput. Human Behav. 26 , 140â150 (2010).
Craik, F. & Lockhart, R. S. Levels of processing: A framework for memory research. J. Verbal Learn. Verbal Behav. 11 , 671â684 (1972).
Rikers, R. M., Van Gerven, P. W. & Schmidt, H. G. Cognitive load theory as a tool for expertise development. Instr. Sci. 32 , 173â182 (2004).
Fairclough, S. H., Venables, L. & Tattersall, A. The influence task demand and learning on the psychophysiological response. Int. J. Psychophysiol. 56 , 171â184 (2005).
Article  PubMed  Google Scholar Â
Tasir, Z. & Pin, O. C. Trainee teachersâ mental effort in learning spreadsheet through self-instructional module based on cognitive load theory. Comput. Educ. 59 , 449â465 (2012).
Olina, Z., Reiser, R., Huang, X., Lim, J. & Park, S. Problem format and presentation sequence: Effects on learning and mental effort among us high school students. Appl. Cogn. Psychol. 20 , 299â309 (2015).
Wetzel, S., Bertel, S., Montag, M. & Zander, S. Spatial task solving on tablets: Analysing mental and physical rotation processes of 12â13 year olds. Educ. Technol. Res. Dev. 68 , 363â381 (2020).
Makransky, G., Terkidsen, T. S. & Mayer, R. E. Role of subjective and objective measures of cognitive processing during learning in explaining the spatial continuity effect. Learn. Instr. 61 , 23â24 (2019).
Matthews, G., Reinerman-Jones, L. E., Barber, D. J. & Abich, J. The psychometrics of mental workload: Multiple measures are sensitive but divergent. Hum. Factors 57 , 125â143 (2015).
Schmeck, A., Opfermann, M., Van Gog, T., Paas, F. & Leutner, D. Measuring cognitive load with subjective rating scales during problem solving: Differences between immediate and delayed ratings. Instr. Sci. 43 , 93â114 (2015).
Brunken, R., Plass, J. L. & Leutner, D. Assessment of cognitive load in multimedia learning with dual-task methodology: Auditory load and modality effects. Instr. Sci. 32 , 115â132 (2004).
Kennedy, D. O. & Scholey, A. B. Glucose administration, heart rate and cognitive performance: effects of increasing mental effort. Psychopharmacol. 149 , 63â71 (2000).
Article  CAS  Google Scholar Â
Quirins, M. et al. Conscious processing of auditory regularities induces a pupil dilation. Sci. Rep. 8 , 14819 (2018).
Toth, A. J. & Campbell, M. J. Investigating sex differences, cognitive effort, strategy, and performance on a computerised version of the mental rotations test via eye tracking. Sci. Rep. 9 , 19430 (2019).
Lelis-Torres, N., Ugrinowitsch, H., Apolinario-Souza, T., Benda, R. N. & Lage, G. M. Task engagement and mental workload involved in variation and repetition of a motor skill. Sci. Rep. 7 , 14764 (2017).
Khachouf, O. T., Chen, G., Duzzi, D., Porro, C. A. & Pagnoni, G. Voluntary modulation of mental effort investment: an fmri study. Sci. Rep. 7 , 17191 (2017).
Dirican, A. C. & Gokturk, M. Psychophysiological measures of human cognitive states applied in human computer interaction. Proc. Comput. Sci. 3 , 1361â1367 (2011).
Hogervorst, M. A., Brouwer, A. M. & Van Erp, J. B. E. Combining and comparing EEG, peripheral physiology and eye-related measures for the assessment of mental workload. Front. Neurosci. 8 , 322 (2014).
Article  PubMed  PubMed Central  Google Scholar Â
Fairclough, S. H. & Venables, L. Prediction of subjective states from psychophysiology: A multivariate approach. Biol. Psychol. 71 , 100â110 (2006).
Byrne, E. A. & Parasuraman, R. Psychophysiology and adaptive automation. Biol. Psychol. 42 , 249â268 (1996).
Article  CAS  PubMed  Google Scholar Â
Borghini, G., Astolfi, L., Vecchiato, G., Mattia, D. & Babiloni, F. Measuring neurophysiological signals in aircraft pilots and car drivers for the assessment of mental workload, fatigue and drowsiness. Neurosci. Biobehav. Rev. 44 , 58â75 (2014).
Arico, P. et al. Passive BCI in operational environments: Insights, recent advances, and future trends. IEEE Trans. Biomed. Eng. 64 , 1431â1436 (2017).
Stipacek, A., Grabner, R. H., Neuper, C., Fink, A. & Neubauer, A. C. Sensitivity of human eeg alpha band desynchronization to different working memory components and increasing levels of memory load. Neurosci. Lett. 353 , 193â196 (2003).
Walter, C., Rosenstiel, W., Bogdan, M., Gerjets, P. & Spuler, M. Online eeg-based workload adaptation of an arithmetic learning environment. Front. Hum. Neurosci. 11 , 286 (2017).
Gerjets, P., Walter, C., Rosenstiel, W., Bogdan, M. & Zander, T. O. Cognitive state monitoring and the design of adaptive instruction in digital environments: lessons learned from cognitive workload assessment using a passive brain-computer interface approach. Front. Neurosci. 8 , 385 (2014).
Kathner, I., Wriessnegger, S. C., Muller-Putz, G. R., Kubler, A. & Halder, S. Effects of mental workload and fatigue on the P300, alpha and theta band power during operation of an ERP (P300) brainâcomputer interface. Biol. Psychol. 102 , 118â129 (2014).
Antonenko, P. D., Paas, F., Grabner, R. & Van Gog, T. Using electroencephalography to measure cognitive load. Educ. Psychol. Rev. 22 , 425â438 (2010).
Brouwer, A. M. et al. Estimating workload using EEG spectral power and ERPs in the n-back task. J. Neural. Eng. 9 , 045008 (2012).
Article  ADS  PubMed  Google Scholar Â
Holm, A., Lukander, K., Korpela, J., Sallinen, M. & Muller, K. M. I. Estimating brain load from the EEG. Sci. World J. 9 , 639â651 (2009).
Spuler, M. et al. EEg-based prediction of cognitive workload induced by arithmetic: A step towards online adaptation in numerical learning. ZDM Math. Educ. 48 , 267â278 (2016).
Kawasaki, M., Kitajo, K. & Yamaguchi, Y. Dynamic links between theta executive functions and alpha storage buffers in auditory and visual working memory. Eur. J. Neurosci. 31 , 1683â1689 (2010).
PubMed  PubMed Central  Google Scholar Â
Kwon, G. et al. Individual differences in oscillatory brain activity in response to varying attentional demands during a word recall and oculomotor dual task. Front. Hum. Neurosci. 9 , 381 (2015).
Gevins, A., Smith, M. E., McEvoy, L. & Yu, D. High-resolution EEG mapping of cortical activation related to working memory: Effects of task difficulty, type of processing, and practice. Cereb. Cortex 7 , 374â385 (1997).
Sauseng, P., Griesmayr, B., Freunberger, R. & Klimesch, W. Control mechanisms in working memory: A possible function of EEG theta oscillations. Neurosci. Biobehav. Rev. 34 , 1015â1022 (2010).
Hsieh, L. T. & Ranganath, C. Frontal midline theta oscillations during working memory maintenance and episodic encoding and retrieval. Neuroimage 85 , 721â729 (2014).
Pavlov, Y. G. & Kotchoubey, B. EEG correlates of working memory performance in females. BMC Neurosci. 18 , 26 (2017).
Scharinger, C., Soutschek, A., Schubert, T. & Gerjets, P. When flanker meets the n-back: What EEG and pupil dilation data reveal about the interplay between the two central-executive working memory functions inhibition and updating. Psychophysiology 52 , 1293â1304 (2015).
Fink, A., Grabner, R. H., Neuper, C. & Neubauer, A. C. EEG alpha band dissociation with increasing task demands. Cogn. Brain Res. 24 , 252â259 (2005).
Klimesch, W. EEG alpha and theta oscillations reflect cognitive and memory performance: A review and analysis. Brain Res. Rev. 29 , 169â195 (1999).
Puma, S., Matton, N., Paubel, P. V., Raufaste, E. & El-Yagoubi, R. Using theta and alpha band power to assess cognitive workload in multitasking environments. Int. J. Psychophysiol. 123 , 111â120 (2018).
Dasari, D., Shou, G. & Ding, L. ICA-derived eeg correlates to mental fatigue, effort, and workload in a realistically simulated air traffic control task. Front. Neurosci. 11 , 297 (2017).
Smith, M. E. & Gevins, A. Neurophysiologic monitoring of mental workload and fatigue during operation of a flight simulator in Proceedings of SPIE Defense and Security Symposium, Biomonitoring for Physiological and Cognitive Performance during Military Operations (ed. Caldwell, J. A. & Wesensten, N. J.), 116â126 (Orlando, 2005).
Brouwer, A. M., Hogervorst, M. A., Holewijn, M. & Erp, J. V. Evidence for effects of task difficulty but not learning on neurophysiological variables associated with effort. Int. J. Psychophysiol. 93 , 242â252 (2014).
Dan, A. & Reiner, M. Reduced mental load in learning a motor visual task with virtual 3D method. J. Comput. Assist. Learn. 34 , 84â93 (2017).
Bastiaansen, M. C., Posthuma, D., Groot, P. F. & de Geus, E. J. Event-related alpha and theta responses in a visuo-spatial working memory task. Clin. Neurophysiol. 113 , 1882â1893 (2002).
Itthipuripat, S., Wessel, J. R. & Aron, A. R. Frontal theta is a signature of successful working memory manipulation. Exp. Brain Res. 224 , 255â262 (2013).
Gevins, A. & Smith, M. E. Neurophysiological measures of working memory and individual differences in cognitive ability and cognitive style. Cereb. Cortex 10 , 829â839 (2000).
Pfurtscheller, G. & Aranibar, A. Event-related cortical desynchronization detected by power measurements of scalp eeg. Electroencephalogr. Clin. Neurophysiol. 42 , 817â826 (1977).
Kramer, A. F. Physiological metrics of mental workload: a review of recent progress. In Multiple Task Performance (ed. Damos, D. L.) 279â328 (Taylor and Francis, 1990).
Google Scholar Â
Winsun, W. V., Sergeant, J. & Geuze, R. The functional significance of event-related desynchronization of alpha rhythm in attentional and activating tasks. Electroencephalogr. Clin. Neurophysiol. 58 , 519â524 (1984).
Grabner, R. H., Neubauer, A. C. & Stern, E. Superior performance and neural efficiency: The impact of intelligence and expertise. Brain Res. Bull. 69 , 422â439 (2006).
Pfurtscheller, G., Neuper, C. & Mohl, W. Event-related desynchronization (ERD) during visual processing. Int. J. Psychophysiol. 16 , 147â153 (1994).
Klimesch, W., Doppelmayr, M., Pachinger, T. & Ripper, B. Brain oscillations and human memory: EEG correlates in the upper alpha and theta band. Neurosci. Lett. 238 , 9â12 (1997).
Krause, C. M. et al. The effects of memory load on event-relaCated EEG desynchronization and synchronization. Clin. Neurophysiol. 111 , 2071â2078 (2000).
Klimesch, W., Vogt, F. & Doppelmayr, M. Interindividual differences in alpha and theta power reflect memory performance. Intelligence 27 , 347â362 (2000).
Malhotra, P., Coulthard, E. J. & Husain, M. Role of right posterior parietal cortex in maintaining attention to spatial locations over time. Brain 132 , 645â660 (2009).
Sauseng, P. et al. A shift of visual spatial attention is selectively associated with human EEG alpha activity. Eur. J. Neurosci. 22 , 2917â2926 (2005).
Rominger, C. et al. The creative brain in the figural domain: Distinct patterns of EEG alpha power during idea generation and idea elaboration. Neuropsychologia 118 , 13â19 (2018).
Jia, W. & Zeng, Y. EEG signals respond differently to idea generation, idea evolution and evaluation in a loosely controlled creativity experiment. Sci. Rep. 11 , 2119 (2021).
Article  ADS  CAS  PubMed  PubMed Central  Google Scholar Â
Nelson, B. D. & Shankman, S. A. Visuospatial and mathematical dysfunction in major depressive disorder and/or panic disorder: A study of parietal functioning. Cog. Emot. 30 , 417â429 (2016).
Grabner, R. H. & Smedt, B. D. Neurophysiological evidence for the validity of verbal strategy reports in mental arithmetic. Biol. Psychol. 87 , 128â136 (2011).
Klimesch, W., Doppelmayr, M., Schwaiger, J., Auinger, P. & Winkler, T. Paradoxicalâ alpha synchronization in a memory task. Cogn. Brain Res. 7 , 493â501 (1999).
Rosengrant, D., Van Heuvelen, A. & Etkina, E. Do students use and understand free-body diagrams. Phys. Rev. Phys. Educ. Res. 5 , 010108 (2009).
Semlitsch, H. V., Anderer, P., Schuster, P. & Presslich, O. A solution for reliable and valid reduction of ocular artifacts, applied to the P300 ERP. Psychophysiology 23 , 695â703 (1986).
Download references
Acknowledgements
The work is supported by the National Natural Science Foundation of China (Grant Nos. 62077013).
Author information
Authors and affiliations.
School of Early Childhood Education, Nanjing Xiaozhuang University, Nanjing, China
Yanmei Zhu & Li Zhang
School of Biological Science & Medical Engineering, Southeast University, Nanjing, China
Yanmei Zhu & Qian Wang
You can also search for this author in PubMed  Google Scholar
Contributions
Y.Z. designed the EEG study and prepared the manuscript. Q.W. carried out the EEG experiment. L.Z. wrote the code to analyze the data. All authors read and approved the final manuscript.
Corresponding author
Correspondence to Yanmei Zhu .
Ethics declarations
Competing interests.
The authors declare no competing interests.
Additional information
Publisher's note.
Springer Nature remains neutral with regard to jurisdictional claims in published maps and institutional affiliations.
Rights and permissions
Open Access This article is licensed under a Creative Commons Attribution 4.0 International License, which permits use, sharing, adaptation, distribution and reproduction in any medium or format, as long as you give appropriate credit to the original author(s) and the source, provide a link to the Creative Commons licence, and indicate if changes were made. The images or other third party material in this article are included in the article's Creative Commons licence, unless indicated otherwise in a credit line to the material. If material is not included in the article's Creative Commons licence and your intended use is not permitted by statutory regulation or exceeds the permitted use, you will need to obtain permission directly from the copyright holder. To view a copy of this licence, visit http://creativecommons.org/licenses/by/4.0/ .
Reprints and permissions
About this article
Cite this article.
Zhu, Y., Wang, Q. & Zhang, L. Study of EEG characteristics while solving scientific problems with different mental effort. Sci Rep 11 , 23783 (2021). https://doi.org/10.1038/s41598-021-03321-9
Download citation
Received : 20 April 2021
Accepted : 24 November 2021
Published : 10 December 2021
DOI : https://doi.org/10.1038/s41598-021-03321-9
Share this article
Anyone you share the following link with will be able to read this content:
Sorry, a shareable link is not currently available for this article.
Provided by the Springer Nature SharedIt content-sharing initiative
By submitting a comment you agree to abide by our Terms and Community Guidelines . If you find something abusive or that does not comply with our terms or guidelines please flag it as inappropriate.
Quick links
- Explore articles by subject
- Guide to authors
- Editorial policies
Sign up for the Nature Briefing newsletter â what matters in science, free to your inbox daily.


5 Types Of Brain Waves Frequencies: Gamma, Beta, Alpha, Theta, Delta
It is important to know that all humans display five different types of electrical patterns or “brain waves” across the cortex. The brain waves can be observed with an EEG (or an “electroencephalograph”) – a tool that allows researchers to note brain wave patterns. Each brain wave has a purpose and helps serve us in optimal mental functioning.
Our brain’s ability to become flexible and/or transition through various brain wave frequencies plays a large role in how successful we are at managing stress, focusing on tasks, and getting a good night’s sleep. If one of the five types of brain waves is either overproduced and/or under produced in our brain, it can cause problems. For this reason, it is important to understand that there is no single brain wave that is “better” or more “optimal” than the others.
Each serves a purpose to help us cope with various situations – whether it is to help us process and learn new information or help us calm down after a long stressful day. The five brain waves in order of highest frequency to lowest are as follows: gamma, beta, alpha, theta, and delta.
5 Brain Waves: Frequencies To Understand
Before I get into specifics, it is important to realize that when I refer to a certain brain wave, I am implying that a particular brain wave is “dominant.” Throughout the day in your waking state, your EEG will display all 5 types of brain waves at the same time. Â However, one particular brain wave will be dominant depending on the state of consciousness that you are in.
For example, if you are awake, but have really bad ADHD, you may have more slow wave (alpha and/or theta) activity than beta waves. During sleep usually there are combinations of the slower frequencies, but even gamma has been found to be involved in rapid-eye movement (REM). Â Below is a brief description of each brainwave state, but a more in-depth understanding can be derived from the book “ Getting Started with Neurofeedback .”
Gamma Waves
These are involved in higher processing tasks as well as cognitive functioning. Gamma waves are important for learning, memory and information processing. It is thought that the 40 Hz gamma wave is important for the binding of our senses in regards to perception and are involved in learning new material. It has been found that individuals who are mentally challenged and have learning disabilities tend to have lower gamma activity than average.
- Frequency range : 40 Hz to 100 Hz (Highest)
- Too much : Anxiety, high arousal, stress
- Too little : ADHD, depression, learning disabilities
- Optimal : Binding senses, cognition, information processing, learning, perception, REM sleep
- Increase gamma waves : Meditation
These are known as high frequency low amplitude brain waves that are commonly observed while we are awake. They are involved in conscious thought, logical thinking, and tend to have a stimulating affect. Having the right amount of beta waves allows us to focus and complete school or work-based tasks easily. Having too much beta may lead to us experiencing excessive stress and/or anxiety. The higher beta frequencies are associated with high levels of arousal. When you drink caffeine or have another stimulant, your beta activity will naturally increase. Think of these as being very fast brain waves that most people exhibit throughout the day in order to complete conscious tasks such as: critical thinking, writing, reading, and socialization.
- Frequency range : 12 Hz to 40 Hz (High)
- Too much : Adrenaline, anxiety, high arousal, inability to relax, stress
- Too little : ADHD, daydreaming, depression, poor cognition
- Optimal : Conscious focus, memory, problem solving
- Increase beta waves : Coffee, energy drinks, various stimulants
Alpha Waves
This frequency range bridges the gap between our conscious thinking and subconscious mind. In other words, alpha is the frequency range between beta and theta. It helps us calm down when necessary and promotes feelings of deep relaxation. If we become stressed, a phenomenon called “alpha blocking” may occur which involves excessive beta activity and very little alpha. Essentially the beta waves “block” out the production of alpha because we become too aroused.
- Frequency range : 8 Hz to 12 Hz (Moderate)
- Too much : Daydreaming, inability to focus, too relaxed
- Too little : Anxiety, high stress, insomnia, OCD
- Optimal : Relaxation
- Increase alpha waves : Alcohol, marijuana, relaxants, some antidepressants
Theta Waves
This particular frequency range is involved in daydreaming and sleep. Theta waves are connected to us experiencing and feeling deep and raw emotions. Too much theta activity may make people prone to bouts of depression and may make them “highly suggestible” based on the fact that they are in a deeply relaxed, semi-hypnotic state. Theta has its benefits of helping improve our intuition, creativity, and makes us feel more natural. It is also involved in restorative sleep. As long as theta isn’t produced in excess during our waking hours, it is a very helpful brain wave range.
- Frequency range : 4 Hz to 8 Hz (Slow)
- Too much : ADHD, depression, hyperactivity, impulsivity, inattentiveness
- Too little : Anxiety, poor emotional awareness, stress
- Optimal : Creativity, emotional connection, intuition, relaxation
- Increase theta waves : Depressants
Delta Waves
These are the slowest recorded brain waves in human beings. They are found most often in infants as well as young children. As we age, we tend to produce less delta even during deep sleep. They are associated with the deepest levels of relaxation and restorative, healing sleep. They have also been found to be involved in unconscious bodily functions such as regulating heart beat and digestion. Adequate production of delta waves helps us feel completely rejuvenated after we wake up from a good night’s sleep. If there is abnormal delta activity, an individual may experience learning disabilities or have difficulties maintaining conscious awareness (such as in cases of brain injuries).
- Frequency range : 0 Hz to 4 Hz (Slowest)
- Too much : Brain injuries, learning problems, inability to think, severe ADHD
- Too little : Inability to rejuvenate body, inability to revitalize the brain, poor sleep
- Optimal : Immune system, natural healing, restorative / deep sleep
- Increase delta waves : Depressants, sleep
Related Posts:
- Repetitive TMS for Alzheimer’s Disease Alters Brain Waves & Enhances Cognition (2024 Study)
- Neurofeedback For ADHD: Is It An Effective Treatment?
- Alpha Brain Waves (8-10 Hz) & Default Mode Network Linked to Self-Control (Behavioral Inhibition) (2023 Study)
- Delta Brain Waves: 0 Hz to 4 Hz
- Alpha Brain Waves: 8 Hz To 12 Hz
MHD News (100% Free)
54 thoughts on “5 types of brain waves frequencies: gamma, beta, alpha, theta, delta”.
I am not sure about marijuana increasing only the alpha waves. I would feel that the more sativa is the variety, the more the gamma waves are increased. And on the other hand: the more indica is the variety, the more theta waves are increased. (Indicas grow further from the equator or higher in altitude, whereas sativas grow closer to the equator). And the very different smells: from citrus zest to earthy chocolate.
I am a PhD student into Music Psychology with a proposed research topic into music and brain responses. This article into Alpha, Beta, Gamma, Theta and Delta has been very useful to me. I will like to know if these various frequency waves could have relationship to specific music stimuli. For instance, that a the brain may produce a particular frequency wave according brains response to a specific music stimulus. Would also be grateful if I can get other articles and reading materials towards the development of my proposal.
This site with music and brain topic might be useful: https://www.frontiersin.org/research-topics/928/the-musical-brain
Hi, could you please let me know if there are certain times in a day that certain brain waves frequency are higher or lower? Many thanks. Marie from London
Hi. Mostly that’s right. Mostly… but not exactly. What about epsilon brain waves? About ambivalence of alpha/theta waves along with highest level of gamma (or nearly even epsilon) at the most advanced stage of meditation?? It sounds unbelievable? Maybe. But this is why most meditating people have serious problems with meditation. Meditation itself as a state of mind, according to e.g. Buddhism is the one thing, and relaxation (commonly known as a “meditation”) is something completely different. :)
Can music instruments generate such waves to stimulate our brain? And if externally these waves are generated then what will be their effects to the listeners? And can we use these waves in our daily life to stimulate our brain or to increase limits of our brain?
Is there any connection (or treatment possibilities) between brain waves and hypothyroidism?
What type of brainwave is the most dominant when waking up?
Beta waves.
Hi, I’ve been exposed to theta waves for a few months. I sleep with it every night. I was starting to become suicidal. I have a long history of depression and ADHD, so nothing seemed out of the ordinary. It started to become worse. Then I switched to gamma, I kept getting sick, as in dizzy spells. I would start vomiting if I listen to gamma. Question is, is this at all normal? If not why is this happening?
Hi Cindy, I realise you posted the above a while ago, but thought I’d reply as nobody did yet. I would think that DELTA waves are the important ones during sleep, as this state is the rejuvenating, restorative one, where your body regenerates. Gamma waves are the highest alertness waves, so I would not listen to those while sleeping, it sounds like your brain / body would not get any rest.
I have had sleeping problems myself, and have realised that listening to anything while sleeping makes me feel worse. I use silicone earplugs to block out noise, and a black fabric over my eyes to block out all light, and listen to something very relaxing on Youtube BEFORE going to sleep. You could try ASMR videos, they often make people sleepy… or some sleep meditation videos.
And remember to get at least some light exercise whenever you can, it makes you more sleepy and lifts the mood (cliche but true). And maybe check with your doctor as well. All the best, take care. Liina xx
Just to clarify: not good to exercise close to bedtime, but earlier in the day :)
Yes Liina, you’re correct!!! I was listening to these waves and I wasn’t getting sound sleep. I didn’t feel good in the morning. Cindy, what I did was exercise at least 1 hour (walking, weight lifting, swim) then listen to these waves before I went to sleep until I become really tired then shut it off.
Mostly importantly, stay off caffeine and alcohol!!! I’ve stopped drinking coffee for about 5 weeks now and feel a HUGE difference. By not drinking coffee, I also found I don’t crave certain foods that go well with coffee like bagels, cream cheese, cookies, muffins, ice cream etc.
Switch to decaf teas and homemade smoothies. Force it first thing in the morning. Also, research foods and vitamins that lift and promote good moods. Stay away from people that cause you to feel down.
Cindy, Here are my thoughts on your matter: I think the brain waves you listen to are going to dramatically affect you the following day. I think much more so than light sleeping pills. The reason I believe is that the brain waves you expose yourself to at night are going to process in your subconscious and become prevalent in you following day.
I believe the sleep we receive essentially primes us for the following day. I believe the excess Theta or Theta dominant brain waves are causing your excess depression. I imagine they are causing your next day reality to be very gloomy and withdrawn. As for the Gamma brain waves, they may not have a direct, stable and well understood function such as a low beta brain wave for example.
Although there are speculations and possible explanations for gamma brain waves, I believe it is possible that they may have a unique and special purpose of their own, but not the type to be specified as a major brain wave to be trained upon yourself. I would recommend not going so intense with any brain wave training, as it may interfere with who you naturally are.
If you would like to train your brain waves, from what I’ve heard about you, I’d recommend the safer normal functioning ranges. Let me explain my summary of what that may be in my assessment:
Delta (Be careful, if that affects your day you much, you may feel like a sluggish and even like a non-functional vegetable).
Theta (Dreamlike, but not connected to reality, on it’s own it can be highly detrimental as it may make you feel depressed and out of touch, the major detriment may come from the perceptions that the self is going through feel so vivid and real, yet the confident and interconnected movements and ways of the world may be too distant. In a sense it could be likened to seeing the world from beneath the water. Everything in the world may not seem bright and happy for what it is.)
Alpha (Alpha may be like a bridge between the inner world and lower brain waves and the faster brain waves externally engaging the world and action. They may allow one to process the movements and energies of the external world by not being so in motion and busy oneself and the Alpha waves may also be in a position to contemplate deeper thoughts and other alternatives that would require teaming up Theta waves with Beta waves for example.)
SMR\low Beta waves (the entry level functioning in direct engagement to the external world and reality. It’s not so fast. It’s enough to take actions without feeling compelled or frantic. It’s probably a generally safe range for normal functioning to get tasks done carefully and be alert. Again, our brains use a lot of waves and like a manual transmission car our brains probably select what they need.)
Beta (More activity, more action, more running yourself at high speeds). With all the waves keep in mind the brain somehow manages all these different brainwaves. If you do much of any, there will probably be imposed rigidities and problems.
I recommend brain wave training only if done in moderation and done wisely. If you don’t need it then you shouldn’t do it. Some brain waves between Alpha and Beta could probably help your Theta problem. Good Luck.
Hi, which is the best brainwave should I use when I repeat positive affirmations?
I see a lot of binaural beats on Youtube. What do you make of them? Do they really send us into Alpha/theta states or just gimmicks? One more question, Does theta waves have the capacity to heal one’s mental problems? I read somewhere, it’s a magical state. Please advise. Looking forward to your reply.
Hello Jcey, Theta is used in healing practices and shamanic traditions, like with drum and rattle, which can alter the consciousness of the practitioner/healer to go into a variety of trance states. From my experience, yes, these are states that are powerful for healing, which is why humanity has used these states for thousands of years.
Also, you could say they are magical for the insight, knowledge and connection provided by going into more subconcious and more childlike imagination. Shamanic ‘journeying’ is a term used often for this, and what it interesting is that certain mystical/spiritual traditions talk about how when we sleep (which is theta/delta waves), that at least part of the soul/spirit/subtle body of the sleeper is free to journey.
Inducing theta waves via shamanic drums or binaural beats is a way of inducing this sleep like state I believe, while in waking consciousness.
Is there any way to increase or decrease these waves?
Hi, I listen to beta waves while studying and then it gets difficult to sleep at night. I go to sleep but I’m wide awake. For how many hours should I listen to these waves? And should I listen to delta waves before going to sleep?
You can benefit from alpha waves before going to sleep. Later you can benefit the most from theta (or low theta), and delta waves during the sleep. Have nice dreams.
I had an QEEG a little while ago and the researcher told me I have an extremely high level of gamma waves. He couldn’t explain what it meant, and now I’ve read the article I wonder if I have these waves all the time, since I wasn’t in a meditative state or learning new tasks? It looks like they are there all the time, is this right? What is the effect of this on my behavior and brain function?
Hi, I would like to know what kind of waves are used for opening the third eye? How can we get the best of gamma waves? Can we use gamma waves for opening the third eye?
Meditation is the activity that if practiced daily alters the brain waves to fully relax & produce feelings of well being. It is only in this state of totally being awake & yet relaxed that the 3rd Eye might open!
Be careful if the third eye opens it can be accompanied by headaches. I feel this happens because your body frequency is not high enough to handle this capability. You need more light (energy). Or you need to strengthen your pineal gland. Fluoride kills it. Change your diet.
I work in the field and in a nutshell each brain is unique. The amplitude or volume of specific frequencies is less important than relationship between the different frequencies and the values at different locations in the Brain including left right balance. So if you have the same level of high frequency activity at the Temporals as you do at the Frontal region this may be problematic.
Excess high frequency activity at the right side of the Temporals can indicate Fight or Flight activation so symptoms of stress/anxiety, sleep problems, emotional response etc. Excess high frequency activity at the left temporal region can indicate Freeze response such as emotional detachment, intellectual response etc. Efficient communication between the two sides is also important.
Medical EEGs have changed little in the past 60 years. The concept of Brainwaves such as alpha, beta etc. is somewhat antiquated. High resolution EEGs can break down the electrical activity into 42,000 individual frequencies.
Since you’re in the field, how does the electricity from brain waves coincide with exterior frequencies? Like a car radio?
Hi just found this site. I have trouble sleeping because I always wake up during the night and have trouble going back to sleep. When I do wake up I’m never refreshed and I’ve always got what they call “brain fog,” so I can’t remember anything. I would like to pass my theory test – that is my goal, but nothing sinks in. If anyone can give me any info on deep sleep which I’m not getting, I would be most grateful, thanks.
Research: Blue light toxic.
Howdy! I had two clients come in with the same complaints that you are having. I told them to go to the doctor and ask for a sleep test and both had severe sleep apnea. That “fog” you are talking about and the “nothing sinks in” will only get worse and can lead to impaired driving, “weird” accidents, illnesses and possibly even a stroke.
Normally any good delta based sleep system will help you, but if you have an underlying medical condition, like a closed airway then it won’t work as your body is fighting to breathe. Good Luck!
Can we use theta waves to make a human sleep? If yes what is better, delta or theta?
I like that it says what what can happen with too many/too little of these brain waves… But my question is, how much is too much? E.g., if I were listening to something on YouTube to stimulate Theta waves, how long would it be until I start having adverse effects, such as inattentiveness?
This may be true of naturally occurring states that haven’t been directly influenced, but the “Too much/Too little” for both Delta and Beta I can personally verify as being totally inaccurate for brainwave states induced by binaural beats and similar approaches. I’ve altered my own brainwave patterns to operate almost exclusively in Delta, and given a presentation while wearing an EEG and projecting the live feed from it onto a screen behind me to showcase what I’d accomplished. I’ve experienced no negative side effects to date several months in, and a wide variety of benefits, including the polar opposites of what you’re attributing to “too much” Delta wave activity.
HI Kyrtin – what you have described here as occurring sounds quite amazing. Do you have a dvd/video or a link of your presentation and the accompanying EEG live feed available for viewing. It would be so interesting to see. Thanks in advance.
So my question is Is my ADHD due to a lack of Gamma/Beta waves or a surplus of Theta/Delta waves? And with that being asked, what is my medication doing for me? increasing G/B or decreasing T/D?
I am writing a Sci Fi novel set in the future. I want my characters to take something to speed up sleep so they don’t spend half their lives sleeping. I was thinking about calling it a deltaREM, because it boosts the 3rd, 4th, and 5th stages of sleep. Does this sound reasonable enough for Sci Fi? Or is it too unrealistic?
Hi Eve, I realize you posted this quite a while ago but perhaps you’re still interested. First off nowadays researchers only recognize 4 stages of sleep :) (previously 5 but the 3rd and 4th Non-REM stages are now seen as the same) occuring in this order: NREM1 (light sleep), NREM2, NREM3 (deep sleep) and REM sleep. From a ‘realism perspective’, all of these seem to be important for health (except perhaps stage 1), and I cannot really imagine what ‘boosting’ any of these stages would actually mean haha.
Do you know about lucid dreaming? Why not, instead of having your characters spend less time sleeping, have them make good use of their sleep. Lucid dreaming is the ability to become aware that you are dreaming while you are dreaming, and in some cases allows you full control over the content of your dreams. People are known to use their lucid dreams for problem solving, and the practice of certain skills – basically your dream becomes a virtual reality simulator ;).
Increased activity in certain brain areas has been linked to lucid dreaming frequency, and actual stimulation of one of these areas has been shown to increase the likelihood of having a lucid dream (the dorsolateral prefrontal cortex (dlPFC) if you’re interested :P). Recently this made me think that in the future we migth actually have for example little implants in our brains that could stimulate this brain area while we are sleeping, leading to more lucid dreaming and therefore potentially useful dreaming/sleep (or just for the fun of it ofc! haha). Seems like a great little sci-fi device to me ;).
I used to be able to lucid dream, then psychiatrists put me on a lot of major cocktails & I couldn’t for a long time. Just made me a zombie. Now I’m off all that crap, using NFB or neurotherapy and beginning to be able to lucid dream again. I love it!
I think that’s perfect/awesome.
I developed epilepsy at 44 years old which is poorly controlled with meds and have 1-2 seizures per week but MRI shows all is OK. I tend to have a seizure when stressed so assume my beta is high? Is there any portable device on the market that measures brain waves so as to give me a seizure warning?
Shouldn’t an EEG machine measure your brain activity? A company called foc.us has developed an “electric shock” system and will supposedly come out with EEG electrodes in the near future.
There are portable devices out there. I have done 92 neurofeedback sessions over the last 18 months. Just this week, I completed 7 sessions of Brain Optimization. Brain State Technologies has a portable device that I have just ordered. It is backordered until April. I can’t wait to get it.
I am in a neurofeedback research project for veterans. 40 session. Have had my intake and first two sessions. Yesterday, I took some meds as prescribed between sessions. One at 3.30 and next at 5pm. Took Wellbutrin 100mg and Prazosin which I normally take at night.. Also 800mg of Ibuprofen for spinal stenosis pain which will soon be corrected.. So on this day the test tells me 5 times to take a break and relax… I say huh?
I am 3x more relaxed than the sessions before because I have no pain. So I follow orders. It is fun but still puzzling. And yes, I have been a meditator for 43 years. Then the clinician tells me I am showing an excess of the Theta waves. So here is the question. Is this a by product of the meds? Or am I just an evolved Thetan? LOL.
How would someone know if they are lacking in any brain waves? And is it beneficial to listen to a certain brain wave while studying if so which one?
By getting a quantitative EEG test (QEEG)… this would allow someone to determine whether they may be deficient in certain frequencies. Likely beta frequencies would benefit those that need to study.
Sure, induce beta frequencies, if you prefer to walk to work 20 miles away every day instead of taking the Porsche. Use gamma waves as they have been directly linked to increased IQ, enhanced cognitive function, and improved memory. Class dismissed.
What brain waves are produced when someone is in a very stressful situation, like a surgeon that have a really difficult operation?
“Stressful” situation would be a beta or high beta dominant state. However, a world-class surgeon may not be as “stressed” as a newer surgeon with a difficult operation and therefore may display more alpha activity. In both scenarios though, the surgeon would be beta-dominant as these waves are associated with being alert.
What brain waves are produced when someone, such as a soldier, is in a very dangerous situation?
Mid to high range beta waves… these are associated with heightened alertness, fear, and danger.
I had an eeg done and it showed my waves to run opposite the standard, the technician was baffled and called someone to validate what he saw. He told me I was 1 in several thousands who had brain waves that did not follow the direction that most people have. What does this mean? Please give me somewhere to find some insight. Thank you -M-
Who ordered the EEG for you? Presumably a doctor; if so, I would start with him/her. Otherwise, I might consider seeing a neurologist.
Hi, I would like to know what is the long term effect of using cannabis on Alpha waves?
Golan you may be interested in this article: http://www.ncbi.nlm.nih.gov/pmc/articles/PMC2234454/
It basically says that alpha and beta wave activity is decreased in marijuana users, in the first month (and perhaps after, the study doesn’t go that far) after they stop smoking. You may also know that chronic users experience sleep disturbances and sometimes vivid dreaming when they stop using.
It basically says that alpha and beta wave activity is decreased in marijuana users, in the first month (and perhaps after, the study doesnât go that far) after they stop smoking. You may also know that chronic users experience sleep disturbances and sometimes vivid dreaming when they stop using. Makes sense. Marijuana inhibits REM, so they are likely to have the rem rebound effect.
I was told by a clinical psychologist who practices biofeedback that my Theta brain waves were most active. I was in a deep state of mourning, but she seemed so surprised and it made me feel that I’m a bit of a freak. How normal is that for an awake state? Also, I often feel very low in energy. Is this connected?
Leave a Comment Cancel reply
This site uses Akismet to reduce spam. Learn how your comment data is processed .
Advertisement
Brain works principle followed by neural information processing: a review of novel brain theory
- Open access
- Published: 24 June 2023
- Volume 56 , pages 285â350, ( 2023 )
Cite this article
You have full access to this open access article
- Rubin Wang 1 , 2 ,
- Yihong Wang 2 ,
- Xuying Xu 2 ,
- Yuanxi Li 2 &
- Xiaochuan Pan 2 Â
3998 Accesses
4 Citations
Explore all metrics
The way the brain work and its principle of work has long been a big scientific question that scientists have dreamed of solving. However, as is known to all, the brain works at different levels, and the operation at different levels is interactional and mutually coupled. Unfortunately, until now, we still do not know how the nervous system at different levels is interacting and coupling with each other. This review provides some preliminary discussions on how to address these scientific questions, for which we propose a novel theory of the brain called neural energy. Such a theoretical and research approach can couple neural information with neural energy to address the interactions of the nervous system at various levels. Therefore, this review systematically summarizes the neural energy theories and methods proposed by our research in the field of brain science, as well as the internal relationship between mechanics and neural energy theory. Focuses on how to construct a WangâZhang (WâZ) neuron model equivalent to HodgkinâHuxley (HâH) model by using the idea of analytical dynamics. Then, based on this model, we proposed a large-scale neural model and a theoretical framework of global neural coding of the brain in the field of neuroscience. It includes information processing of multiple sensory and perceptual nervous systems such as visual perception, neural mechanism of coupling between default mode network and functional network of brain, memory switching and brain state switching, brain navigation, prediction of new working mechanism of neurons, and interpretation of experimental phenomena that are difficult to be explained by neuroscience. It is proved that the new WâZ neuron model and neural energy theory have unique functions and advantages in neural modeling, neural information processing and methodology. The idea of large-scale neuroscience research with neural energy as the core will provide a potentially powerful research method for promoting the fusion of experimental neuroscience and theoretical neuroscience in the future, and propose a widely accepted brain theory system between experimental neuroscience and theoretical neuroscience. It is of great scientific significance to abandon the shortcomings of reductive and holism research methods in the field of neuroscience, and effectively integrate their respective advantages in methodology.
Similar content being viewed by others
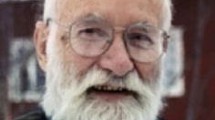
Reflections on a giant of brain science
An improved neuronal energy model that better captures of dynamic property of neuronal activity.
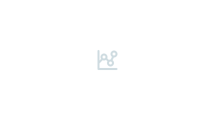
Can the activities of the large scale cortical network be expressed by neural energy? A brief review
Explore related subjects.
- Artificial Intelligence
Avoid common mistakes on your manuscript.
1 Introduction
More than 20Â years ago, a molecular neurobiologist at the University of Berkeley Professor Walter Freeman put forward the concept of neurodynamics (Freeman 2000 ). It has become a new research field to study the activities of cognition and nervous system with the theory and method of dynamics (Pouget and Latham 2002 ; Basar 1998 ; Ăelik et al. 2021 ; Brydges et al. 2020 ; Yu et al. 2020 ; Wouapi et al. 2021 ; Wang et al. 2019a ; Iribarren and Moro 2009 , 2008 ; Memmesheimer and Timme 2006 ; Navarro-LĂłpez et al. 2021 ; Buxton 2012 ; Churchland et al. 2002 ; Hipp et al. 2011a ; Ermentrout et al. 2007 ; Lakatos et al. 2008 ; Rabinovich and Huerta 2006 ; Sandrini et al. 2015 ; Hopfield 2010 ; Hu et al. 2021 ), many of scientific research achievements has been showed in this research area. Neurodynamics is more commonly known as computational neuroscience in European and American countries, while it is known as neuromechanics in Japan (Takeda 1999 ). Particularly, the Dynamic Brain Group, originally organized by Japanese scientists, developed various kinds of collaborative researches such as the organization of Dynamic Brain Forum (DBF) in the world, where many researchers in the world, who have been engaged in neuroscience from the aspect of âdynamic brainâ (Tsuda et al. 1987 ; Tsuda 1991 , 1992 ). However, in the field of experimental neuroscience, scientists prefer to use neuroinformatics to describe the basic laws of neural information processing qualitatively or quantitatively. But no matter what name we used with it, it doesn't change the fact that neuroscientists and artificial intelligence scientists have come to realized that the development of cognitive neuroscience is increasingly dependent not only on advances in experimental techniques and rigorous experimental data, but also to understand the principle of brain network signal processing and transmission and insight into the internal mechanism of neural coding distribution mode with quantitative methods from a theoretical height, so as to discover the law and nature behind the vast experimental data. In order to better understand and master the operation of the brain and deal with various brain diseases, dynamic theory is used to accurately predict the potential patients with degenerative brain diseases at an early stage (Navarro-LĂłpez et al. 2021 ; Ebrahimzadeh et al. 2021 ; Yang et al. 2021a ; Jiang et al. 2020 ; Sharma and Acharya 2021 ).
For a long time, cognitive neuroscience, which takes experiments as its basic research method, has focused on the improvement of experimental phenomena, experimental data and experimental techniques while ignoring the importance of theory. Because of this result, the brain science with hundreds of years of history has not had a systematic and complete theoretical system of its own. This abnormal phenomenon has made brain science so far developed to today is still an immature discipline. Although theoretical neuroscience was born 20Â years ago, it is not widely accepted by the academic community to have only one theory. Although theoretical neuroscientists have made a series of excellent research achievements today (Wouapi et al. 2021 ; Wang et al. 2006 , 2021a ; Clancy et al. 2017 ; Videbech 2010 ; Zhang et al. 2019 , 2020 ; Yuan et al. 2021 , 2022 ; Yao and Wang 2019 ; Maltba et al. 2022 ; Zhou et al. 2020 ; Li et al. 2020 ; Kim and Lim 2020 ; Yang et al. 2021b ), it is still difficult to widely and effectively cooperate with experimental neuroscientists to promote each other and integrate development. One of the efforts for the integration of development has been performed is to publish a textbook of comprehensive neuroscience such as âNeuroscience in the 21st Centuryâ, 3rd Edition (Pfaff and Volkow 2022 ). Among the chapters in that textbook, the following is devoted to the dynamics in neural systems: Ichiro Tsuda, Dynamics in neural systems: a dynamical systems viewpoint. The view of history, dynamic theory for neural information processing have been proposed in several aspects. Minoru Tsukada first treated the neural information processing as dynamic Markov channels from dynamics brain (Tsukada et al. 1975 ). Ichiro Tsuda proposed several dynamic aspects of the brain as a typical complex system (Kaneko and Tsuda 2001 ). Tsuda proposed Hermeneutics of the brain from the aspect that the brain interprets the external world to recognize internal images of external signals via action and sensation (Tsuda 1984 ). Tsuda first proposed a dynamic associative memory model in nonequilibrium neural networks (Tsuda et al. 1987 ), in contrast to a typical static model of memory capacity such as Hopfield model. Kazuyuki Aihara followed Tsudaâs model and confirmed the presence of dynamic associative memory, by using his chaotic neural networks (Adachi and Aihara 1997 ; Aihara et al. 1990 ). In this kind of study, Tsuda found complex dynamic transitions in his nonequilibrium neural networks, whose transition was proposed to be called âChaotic Itinerancyâ (Tsuda 1991 , 1992 , 2001 , 2013 , 2015 ; Nara and Davis 1992 ). In early 1990s, the Japanese Dynamic Brain Group was organized by Minoru Tsukada, Hiroshi Fujii, Shigetoshi Nara, Ichiro Tsuda, and Kazuyuki Aihara, and J-DBG developed various kinds of collaborative researches such as the organization of DBF, where many researchers in the world, who have been engaged in neuroscience from the aspect of âdynamic brainâ, gathered together. This activity of forum was led to the later organization of ICCN (International Conference on Cognitive Neurodynamics) and the publication of Cognitive Neurodynamics by Springer. M. Tsukada invited Masamich Sakagami in Tamagawa University to do the collaborative research on the dynamic mechanism of thoughts. An important aspect was whether or not the thoughts process can be discriminated with associative memories. M. Sakagami succeeded to make an experimental system, which can discriminate these two cognitive phases. X. Pan were enthusiastic to perform such an experiment and succeeded to find specific neurons. Finally, they published important papers (Pan et al. 2008 , 2014 ). As another important works of DBG were for Cantor coding (Tsuda and Kuroda 2001 ; Fukushima et al. 2007 ; Kuroda et al. 2009 ; Yamaguti et al. 2011 ; Ryeu et al. 2001 ), gap junction-coupled neural network models (Fujii and Tsuda 2004 ; Tsuda et al. 2004 ; Tadokoro et al. 2011 ) and complex visual hallucinations and so on (Collerton et al. 2016 ; Tsukada et al. 2015 ).
Nevertheless, there still seems to be an invisible and unbridgeable chasm between the achievements of neuroscience at all levels. These conditions prevent the mutual use, influence, and diffusion of their research findings, as well as major breakthroughs in cognitive neuroscience. As a result, the research field of cognitive neuroscience has been unable to get out of the dilemma of âblind man touching the elephantâ. Especially in consciousness, thinking, creativity generation mechanism, emotion, the nature of intelligence, prediction, visual perception generation mechanism, memory storage and call, global brain function and many other aspects of the research progress is very slow, some even no progress. Moreover, a growing number of scientists in other fields are intrigued by the multitude of unanswered scientific questions in the field of brain research and their complexity.
The basic ideas of neurodynamics have been increasingly infiltrated and embodied in many aspects, such as neuroscience, artificial intelligence, brain-like computing, bioinformation, medical diagnosis, image processing, control science, complex network and engineering applications (Bullmore and Sporns 2009 ; Ullman 2019 ; Roy et al. 2019 ; Zeng et al. 2019 ; Wang and Zhu 2016 ; Deco et al. 2015 ; Kanwisher 2010a ). Brain science is a large-scale science, which not only involves the three-dimensional (3D) intersection of many disciplines, but also poses many unprecedented challenges to many mature disciplines. For example, does the weak magnetic field inside our brains contribute to the transmission of nerve signals? If so, where is the experimental evidence? If there is no contribution, how to explain the negative power component of neurons (Wang et al. 2015a ; Wang and Wang 2018a ), and how to explain the equivalence between WangâZhang's (WâZ) neuron model and HodgkinâHuxley's (HâH) model (Wang and Wang 2018b ). A further question is whether neurons in the brain, or even without synaptic connections and nerve fiber connections between brain regions, can still transmit neural signals in the case of electromagnetic field coupling to achieve communication between neurons and various brain regions (Yang et al. 2022 ; Ma and Tang 2017 ).
As an unstable dynamic system, the brain has no controversy in the academic world. A large number of experimental data and results reveal that our brain has specific functional characteristics at any level, and its activities are highly nonlinear and complex. The highly nonlinear and complex brain dynamics as well as their various functional expressions are not only related to gene and functional genomics, biology and biochemistry, but also to solid mechanics (McIntyre et al. 2001 ), fluid mechanics (Moore and Cao 2008 ), dynamics and control (Lu et al. 2008a , b ). Our research shows that some experimental phenomena in cognitive neuroscience can be reproduced and repeated by mechanical models (Wang et al. 2015a ; Li et al. 2022a ), and some experimental data that cannot be explained by neuroscience can also be scientifically explained by our mechanical models (Peng and Wang 2021 ). We also can use mechanical models to predict new experimental phenomena and new neural mechanisms not found in neuroscience (Wang and Wang 2018b ). These results fully demonstrate the power of mechanical science in the field of brain science research.
A comprehensive review article about âNeurodynamics and Mechanicsâ profoundly elaborated the internal connection between neurodynamics and mechanics (Lu 2020 ), explained the transformation from classical mechanics to generalized mechanics, and the one-to-one correspondence between generalized mechanics and neurodynamics. The review pointed that since the twentieth century, dynamical system theory and methods have been further developed and successfully used in various mechanical systems, even nonlinear differential equations described in general system has universal theoretical significance and important application value. This indicates that modern mechanics research has broken through the traditional category of classical mechanical system and opened up a new category of âgeneralizedâ mechanical system. The research object of mechanics has expanded from âparticle or particle systemâ to the general âdynamic systemâ, the concept of âforceâ has expanded from âmechanical forceâ to the general âinteractionâ, and the concept of âmotionâ has also expanded from âconfiguration changeâ in geometric space to âstate evolutionâ in state space. These ideas are important and instructive for the modeling and calculation of mechanical science in cognitive neuroscience and the construction of the theoretical system of brain-like intelligence.
At present, there is no widely accepted theory in the field of neuroscience, thus, theoretical neuroscience and experimental neuroscience cannot effectively integrate and promote each other. This has seriously hindered the development of various fields of cognitive neuroscience, so that we cannot effectively interpret experimental data, and reveal data behind the nature and regularities form the basis of scientific predictions and explanations. In order to make a great breakthrough in neuroscience and establish a systematic and complete theoretical system of cognitive neuroscience, it is necessary to perform researches on brain theory. The core scientific question in the study of brain theory is whether human intelligent behaviors depend on the activity of a single or a few neurons or whether they are realized by the interactions at molecular to systemic levels? The answer of this core scientific question is now beyond dispute among neuroscientists. However, academic community has not yet come up with an effective solution to solve this core scientific challenge. To this end, we proposed a definition of large-scale neuroscience. The cornerstone of this definition is that a large-scale neuroscience model is on the basis of the neural energy model (Wang and Zhu 2016 ; Wang et al. 2015a , 2008 ; Wang and Wang 2018b ), and the neural energy model arises from the theory and method of analytical dynamics (Wang and Pan 2021 ). The present study aimed to quantitatively obtain the global information of neural activity by finding the relationship between neural energy and membrane potential, field potential and firing rate of network. As the global information of the neural activity can be transformed into energy for research and analysis, neural energy coding constitutes the cornerstone of large-scale neuroscience models. The main contents include the following items: (a) a new research method that can unify reductionism and holism in theory. The new method could theoretically reproduce not only the electrophysiological recordings, but also the global information of the functional neural activity using functional magnetic resonance imaging, fMRI (Yuan et al. 2021 ; Wang et al. 2015a ; Peng and Wang 2021 ; Cheng et al. 2020 ); (b) the global functional model of brain is established computationally, which can be used to construct, analyze, and describe the experimental models of neuroscience at various levels (Wang and Zhu 2016 ; Wang et al. 2020 ). Thus, the computational results at all levels are no longer mutually inapplicable, contradictory and irrelevant; (c) if a global functional model of brain cannot explain the function and energy consumption of the default mode network (DMN) and the resting state network, and how it transitions from the default model to the cognitive network and the corresponding energy transformation under task-induced condition, then, such model is not a global neural model (Yuan et al. 2021 ). The adjustable parameters of the global functional model of brain are necessarily limited and simple; (d) this type of models can be used for the analysis of experimental data hidden behind the nature and regularity. As our neural energy model meets all of the above-mentioned requirements of the global functional model of brain, and it has already accumulated and published a series of original and innovative scientific research results (Wang et al. 2008 , 2015a , 2017a , 2018a , b , 2019b , 2021a ; Yuan et al. 2021 ; Wang and Zhu 2016 ; Wang and Wang 2018a , b ; Li et al. 2022a ; Peng and Wang 2021 ; Lu 2020 ; Cheng et al. 2020 ), this has laid a firm foundation for the creation of new brain theory that can stand the testing of experimental data. The main research directions of brain theory are reflected in the following four aspects.
1.1 Brain theory: exploration of working mechanism of the brain
One of the first scientific questions that needs to be answered in the discussion of how the brain works is why neuroscience cannot explain the mechanism of about 95% of the brain's energy consumption (Fox and Raichle 2007a ; Balasubramanian 2021 ; Raichle 2010 )? Although brain only accounts for about 2% of body weight, it consumes about 20% of the body's energy. The neural energy expenditure caused by task stimuli typically accounts for only 5% of the resting brain energy consumption. Much of our understanding of the brain comes from study of the 5% of brain activity (Raichle and Mintun 2006 ). From these data, it is clear that the energy loss in the brain is almost independent of task stimuli. The following questions should be answered:
What is the nature of the persistent intrinsic activity that causes the great depletion of brain energy?
What is the relationship between the huge depletion of brain energy and cognitive function?
Is it possible that the current mainstream view in cognitive neuroscience may mislead scholars to ignore the possibility that neuroscience experiments and cognitive psychology experiments reveal only some parts of the brain activity?
The important question is that if the brain is reflex in nature, why is the energy consumption of the brain under stimulated conditions almost the same as the energy consumption of the brain under resting conditions? If brain is reflex, task-induced brain energy consumption should increase significantly, why is it less than a 5% increase? In this research area, we have published a series of research results (Wang et al. 2021a , 2018a ; Yuan et al. 2021 ). Using the large-scale neural energy theory to reveal the neural mechanism of the hemodynamic phenomenon of the brain, it was revealed that neural energy is an important marker of the activity of the nervous system, and energy features contain information about external stimuli and neural responses (Yuan et al. 2021 ; Peng and Wang 2021 ); using the neural energy theory to attain the biophysical mechanism of the mutual coupling and antagonism between the brain DMN and the working memory network, it can be demonstrated that neural energy can effectively fuse DMN s and cognitive networks to interpret and analyze the spatial information and encoded content contained in complex neural activities (Yuan et al. 2021 ). The computational simulation results are in complete agreement with the experimental data (Piccoli et al. 2015 ; Compte 2000 ; Wei et al. 2012 ; Hsieh and Ranganath 2014 ; Karlsgodt et al. 2005 ). The neural energy theory can also efficiently express the neural coding of the cognitive system in a 3D space (Hsieh and Ranganath 2014 ); the nervous system can realize the maximization of information coding under the condition of energy constraints (Wang et al. 2018a , b , 2019b ), and the neural energy coding can maximize the efficiency of intellectual exploration (Wang et al. 2017a ). Our study also demonstrated a stimulus-related increase in energy expenditure of less than 5% compared with energy expenditure under a spontaneous activity, which is consistent with brain imaging results reported by Raichle et al. (Wang et al. 2021a ), and spontaneous activity consumes most of the energy compared with task state (Fox and Raichle 2007a ; Balasubramanian 2021 ; Raichle 2010 ; Raichle and Mintun 2006 ). Therefore, the neural energy can be used to express the neural activity of the cerebral cortical network (Wang and Zhu 2016 ).
Another view is that there is experimental evidence that the neural activity of the brain exhibits quasi-critical characteristics (Williams-GarcĂa et al. 2014 ). The biological cerebral cortex generally operates near the quasi-critical point. An article published in the Physical Review Letters revealed that external input forces the neural network of the brain away from a tipping point and operates in a non-equilibrium state. Under different conditions, the brain is in a âquasi-critical stateâ that satisfies the scaling relationship (Fosque et al. 2021 ). In particular, several recent articles have scientifically concentrated on how the brain works, and have obtained experimental data for verification. They demonstrated that diverse cognitive processes set different demands on locally segregated and globally integrated brain activity. With emphasizing the multilevel, hierarchical modular structure of the functional connectivity of the brain to derive eigenmode-based measures, Wang et al. (Wang et al. 2021b ) showed that in healthy adults (range of age 22â36Â years old), the healthy brain is characterized by a balance between functional segregation and integration. Crucially, a stronger integration is associated with a better cognitive ability, and a stronger segregation fosters crystallized intelligence and processing speed, and an individualâs tendency toward balance supports a better memory. Thus, the segregationâintegration balance empowers the brain to support diverse cognitive abilities. This association between balance and cognitive abilities is not only consistent with the recently proposed Network Neuroscience Theory (NNT) of human intelligence (Barbey 2018a ), but also provides more contents to the NNT. In fact, the balance between segregation and integration requires the diversity from weak to strong functional connectivity in dynamic patterns. Using the eigenmode analysis, Wang et al. (Wang et al. xxxx) also found that the diverse functional interaction is generated by hierarchically activating and recruiting structural modes, which are inherent to the hierarchical modular organization of the structural connectome. The critical state can best explore the hierarchical modular organization, optimize the combination of intrinsic structural modes, and maximize the functional diversity.
We can conclude that whether it is the hypothesis that the operation of the brain is quasi-critical, or the hypothesis that the cognitive ability of the brain conforms to the principle of separationâintegration balance, under the condition that the experimental evidence is still lacking, we need to further analyze from the perspective of energy. Examine whether the energy in quasi-critical states, and the energy of state-space switching, is consistent with known features of complex, adaptive brain network dynamics in the presence of quasi-critical states. As for the separationâintegration balance principle of the brain, it is necessary to quantitatively describe how the DMN transitions to the cognitive neural network from the perspective of neural energy. The establishment of the global neural model of the brain must conform to two basic principles supported by experimental data as follows: (1) cost-effectivenessâthe activity of the neural network in the resting state and cognitive activity conforms to the principle of energy minimization; (2) high efficiencyâthe transmission efficiency of neural network signals in the cerebral cortex conforms to the principle of maximizing energy utilization (Laughlin and Sejnowski 2003a ; Zheng et al. 2022 ).
1.2 Modeling of cerebral neural network and dynamic analysis of cognitive function
Panayiota Poirazi and Athanasia Papoutsi presented modeling methods at three levels of abstraction, from âsingle neuronâ to âmicrocircuitâ and then to âlarge-scale network modelâ, in a recent review on the relationship between dendrites and cerebral function. This study systematically summarizes the important role of dendrites in computational modeling, and expounds the great contribution of neurodynamics theory and computational neuroscience to the important progress of neuroscience by enumerating the successful complementarity or interaction between modeling and experimental neuroscience (Poirazi and Papoutsi 2020 ). In particular, a recent review of the structure, function and control of cerebral networks published in the Nature Reviews Physics comprehensively explains the possible complex operational mechanisms of the brain from the perspective of statistical physics, and analyzes the processes of cerebral cognition, creativity and consciousness from the perspective of complex network dynamics. From the perspective of computational network biodynamics, the changes of functional networks during the processes of brain diseases and improvement were summarized (Lynn and Bassett 2019 ).
Our findings showed that large-scale neuroscientific models can not only analyze and explain the local neural activities of the brain, but also the global neural activities of the brain (Yuan et al. 2021 ; Peng and Wang 2021 ). This large-scale cerebral functional model is also a robust method to solve the conversion relationship between electroencephalography (EEG) and electrocorticography (ECoG) (Hipp et al. 2011b ). It can also be used to describe the interaction between various cerebral regions (Yuan et al. 2021 ; Peng and Wang 2021 ), which can also explain the dependence between blood oxygen signals and states of consciousness through insight and analysis of the nature and laws behind the experimental data (Raichle et al. 2018 ; Stender and Mortensen 2016 ), and the essence of intelligence (Barbey 2018b ; Kruegera et al. 2009 ), the source of creativity (Kanwisher 2010b ), the laws of encoding and decoding of the perceptual neural system (Stelnmetz et al. 2019 ; Esterman et al. 2009 ), the neurophysiological mechanism of the hemodynamic phenomenon of the brain, the description and the content of brain waves, etc. (Cohen 2017 ). Hence, neural energy models are the only option for large-scale neuroscience models until more efficient methods are found (Wang and Zhu 2016 ).
1.3 Research of dynamic coding based on classification
1.3.1 receptor coding.
The essence of cognition is the process of information processing, and the essence of information processing is expressed through neural coding. Therefore, selection of a neural coding mode plays an extremely important role in determining the cerebral functions. To date, researches on neural coding have mainly concentrated on the firing of various membrane potentials to measure and characterize various stimulus properties, including light intensity, sound intensity, temperature, pressure, and motion. As the neural activity and applicable scope revealed by different neural coding patterns are very limited, we need to explore the coding characteristics at different levels, which are of great importance to reveal the cognitive activity corresponding to various types of coding patterns. Especially, when we combine coding patterns at different levels to explore the cognitive properties of different functions, it greatly increases the complexity of neural coding research and makes it more difficult to explore the working mechanism of the brain (Wang and Zhu 2016 ).
In the field of neuroinformatics, several neural coding models have been proposed (Breakspear 2017 ). They can be mainly summarized as receptor coding, including spike count code, spike timing code, and temporal correlation code, respectively, corresponding to different assumptions of information units (Johnson and Ray 2004 ; Nirenberg and Latham 2003 ; Victor 1999 ). Receptor encoding is the premise of realizing perceptual behavior, thus, in the process of realizing vision, hearing, and smell, the dynamic encoding patterns on photoreceptors, auditory receptors, and olfactory receptors are neurophysiological responses to various stimulus information. The characteristics of these neurophysiological responses strongly depend on the mechanism of the neural dynamics of each functional circuit. It has been found that photoreceptors and auditory receptors primarily encode the firing rate and firing time of spikes on neurons (Victor 1999 ; Wang and Wang 2018c , 2020 ; Jacobs et al. 2009 ; Malnic et al. 1999 ), while olfactory receptors simultaneously encode combinations of stimulus-sensitive neurons (Miyamichi and Luo 2009 ; Xu et al. 2022a ). In the recent decade, firing rate code has become the standard for describing the properties of various types of perceptual and cortical neurons. Humans detect the surface of objects through the fine movements of their fingers and recognize surface textures. The simplest action in the process is a fingertip rubbing across the surface. During tactile detection, material properties are converted into neural signals from the somatosensory system. Aiming at the tactile sensing and coding problems involved in softness, Wang et al. simulated the touch evaluation process and compared it with the psychophysical response of the softness of fabric materials. The study found that the average firing rate of action potentials evoked by all tactile receptors in the contact area between the fingertip and the object reflects the bending stiffness of fabric-like flexible materials, and there is a linear relationship between the average firing rate and softness (Yao and Wang 2019 ; Hu and Wang 2013 ; Hu et al. 2012 ). Therefore, the encoding form in receptors is closely associated with stimulus characteristics. These findings may enable us to further study the physiological mechanisms that receptors rely on, in order to process stimulus information.
1.3.2 Coding of navigational information
In the coding of navigational information, a recent article on how odor cues are recognized as location information by recording hippocampal CA1 neuron activity is of great significance. Scholars found that using smell as a landmark in the coding of navigational information, iteratively interacting with the route integration and the smell landmark in turn, can form a long-distance cognitive space map. The location information of place cells represented by odor cues greatly improves the spatial cognition and navigation abilities of rats (Fischler-Ruiz et al. 2021 ).
An important premise in the coding of navigational information is how the nervous system orders various information in order to encode the most important information, so as to achieve advantages and avoid disadvantages and the highest efficient navigation. Researchers found that paraventricular nucleus of the thalamus (PVT) plays an important role in tracking the salience of external stimuli. The brain uses this information to learn how to measure external stimuli and to ignore or avoid certain stimuli. The results showed that the importance of PVT in selecting external stimuli is not only determined by the physical characteristics of the stimulus itself, but also is related to the internal physiological state of animals and external environment (Zhu et al. 2018a ). This provides a very important physiological basis for the coding of navigational information.
It is essential to emphasize that we biologically use the concept of neural energy to construct a computational model for the exploration of intelligence. The theory of neural energy coding is used to solve the path search problem: the model constructs a neural energy field by the power of the place cell cluster, and calculates the gradient of the neural energy field, so as to use the gradient vector to study the problem of intellectual exploration. The findings demonstrate that our proposed new model of intellectual exploration based on the neural energy not only finds optimal paths more efficiently, but also presents a biophysically meaningful learning process. This new idea verifies the importance of hippocampal place cells and synapses for spatial memory and the effectiveness of energy encoding, and provides an important theoretical basis for understanding the neural dynamics of spatial memory (Wang et al. 2017b ).
1.3.3 Coding of cortical information
The coding of cortical information is a very significant research direction in the field of neural information processing. It mainly involves time coding, first-spike latency coding, population coding, and phase coding. Compared with time coding, firing rate coding has certain advantages in signal acquisition and energy efficiency. However, the firing of a single neuron in temporal coding can contain more information (Optican and Richmond 1987 ; Thorpe et al. 2001 ). It can express the characteristics of spike activity that cannot be expressed in the firing rate coding through the rank order code of the spike and the first-spike latency coding of the neuron. Experimental data showed that in the study of visual, auditory, and somatosensory cortex, more efficient and reliable neural signals could be encoded based on the rank order code of the spike and the first-spike latency coding of the neuron (Heil 2004 ; Chase and Young 2007 ; Zhong and Wang 2021a , b , c ; Xin et al. 2019 ).
Studies revealed that both the encoding of receptors, the encoding of navigational information, and the encoding related to various cognitive information are not determined by the activity of a single or a few neurons, but also by the joint neural response of large-scale neural populations (Insel et al. 2004 ; Hipp et al. 2011c ). The population coding is produced to express such type of associative neural response. In particular, synchronized oscillation of neural populations in the cortex can be analyzed as units of information in population encoding (Hipp et al. 2011c ), thereby facilitating the study of the intrinsic relationships between coupled networks in the cerebral cortex. The research on the phenomenon of synchronous oscillation is mainly based on the theory of phase synchronization and the theory of binding. Based on the binding theory (Feldman 2012 ), we can not only study how the codes in different cerebral regions are related to produce perception, decision-making, planning, and behavior (Churchland et al. 2012 ), but also express the neural activity of the cortical network with different rhythms through the phase synchronization theory (Wang et al. 2008 , 2009 ; Wang and Zhang 2011 ; Rubin et al. 2012 ). Therefore, phase synchronization theory is widely recognized as an important mechanism to support the study of binding phenomena (Panzeri et al. 2015 ), facilitating the study of phase encoding on how synchronized oscillation depends greatly on enrichment of the population encoding. The most important disadvantage of population encoding is that it cannot handle the problem of high-dimensional nonlinear coupling (Wang and Zhu 2016 ), followed by how the macroscopic properties of population encoding can be effectively integrated with encoding at the microscale, and some important mechanisms at the microscale, such as interactions between neurons, roles of different functional neurons in the macroscopic expression of population codes, and how the sparsity of coding and combinatorial selection of local encodes cause their intrinsic connections in the encoding of large-scale neuronal populations. These problems severely limit the application of population coding in the field of complex brain dynamics research (Panzeri et al. 2015 ). To address and explore the limitations of various coding theories, we proposed a theory of neural energy coding (Wang and Zhu 2016 ).
1.3.4 Behavioral coding
How do different neuronal populations, which are widely distributed in the brain, and communicate with each other to accomplish certain complex tasks? Are there certain working principles for these widely distributed neuronal populations when performing these complex tasks? One example is that in order to complete a perceptual decision, the brain needs to process sensory information and select behaviors that lead to rewarding effects. Neurons in multiple cerebral regions mediate some aspects of these processes, and it is not clear which cerebral region processes which information and whether the processing of this information depends on similar or different neural circuits. Therefore, the study of behavioral coding has noticeably attracted neuroscientistsâ attention in recent years to solve these scientific problems.
In an article entitled âSpontaneous behaviors drive multidimensional, brainwide activityâ, Stringer et al. used two-photon calcium imaging combined with neuropixel electrode recording to monitor the activity of about 10,000 neurons in the visual cortex of awake mice. The primary visual cortex was found to encode visual and motor information related to facial movements (Stringer et al. 2019 ). In another article entitled âThirst regulates motivated behavior through modulation of brainwide neural population dynamicsâ published in the Science by Allen et al. from Stanford University, the same neuropixel electrodes were used to record nearly 24,000 neurons in 34 cerebral regions of mice in a thirsty state. It was found that the state of drinking motivation could determine the activity of neuronal population throughout the brain to convert sensory information into behavioral effects (Allen et al. 2019 ). In the third article (GrĂŒndemann, et al. 2019 ), entitled âAmygdala ensembles encode behavioral statesâ from the research group of Andreas LĂŒthi, University of Basel, Switzerland, the GRIN prism was used to study the encoding behavioral states of the basal amygdala under different behavioral paradigms, and found that in exploration during the non-exploratory and non-exploratory phases, two non-overlapping functional neuronal populations could encode opposite behavioral states. These results revealed the working state of neuronal population in different cerebral regions under complex tasks from a large-scale perspective, so as to better understand the working principle of the brain. This unprecedented large-scale recording of neuronal activity is due to the latest breakthrough in animal calcium imaging under the condition of awake activity with neuropixel electrodes, enabling us to explore scientific problems that have never been imagined more boldly and freely.
1.4 Neural energy coding
Neural energy coding is a novel coding theory based on the corresponding relationship between neural energy consumption and neural activity.
1.4.1 Studying the scientific significance of neural energy encoding
According to the fMRI findings, when a part of the body is stimulated, according to the blood oxygenation level dependent (BOLD) imaging, it was found that there was a 31% increase in blood flow in the contralateral cerebral hemisphere, which was corresponded to only 6% increase in oxygen consumption (consistent with the 5:1 result obtained by the spectroscopy). Why is the increase in blood flow not accompanied by a substantial increase in oxygen consumption? Only a proportional increase in the rate can be consistent with an increase in energy expenditure continuously provided in the bloodstream. More importantly, is the large amount of remaining neural energy loss only used for the brain's physiological metabolism or is it related to cognitive activity? If these remaining energies are relevant to cognition, in what form are they involved in cognition? At present, neuroscience cannot explain these physiological phenomena, and neural energy coding has a strong potential to scientifically explain and probe these physiological phenomena (Yuan et al. 2021 ; Peng and Wang 2021 ; Cheng et al. 2020 ).
For the large number of unsolved neuroscience problems mentioned above, it is not enough to rely solely on neuroscience experiments and new neuroscience technologies, because experiments can only observe phenomena and new neuroscience techniques can only discover previously unobserved phenomena. For instance, the experimental data cannot reveal the working mechanism and the distribution pattern of dark energy of the DMN in the brain, or they cannot reveal the coupling relationship between the DMN and the functional neural network and their quantitative relationship with the biological energy provided by the brain blood flow.
Completion of cognitive tasks is always associated with synchronized oscillation and synaptic transmission of neural activity in the network, and the neural energy consumption is generally considered to be only a small fraction (around 5%) of the overall energy expenditure. Thus, is there any other way of neural information transmission, which is one of the necessary conditions for the completion of cognitive tasks, as well as synaptic transmission? Professor Dominique Durand, an American scientist, recently published an article in the Journal of Neuroscience (Qiu et al. 2015 ), pointing out that neural information can be effectively transmitted only using the brain's electrical field to achieve cognitive tasks without the need for synaptic transmission, indicating that neural information may be involved in spontaneous neural activity. Therefore, studies based on spontaneous neural activity are likely to reveal how major energy consumption occurs in the brain and the internal relationship between the neural mechanism of energy consumption and cognitive function. Neural energy coding can unify spontaneous neural activity and task-induced neural activity in a group of neural models (Yuan et al. 2021 ; Peng and Wang 2021 ).
1.4.2 Key features of neural energy coding
As the neuronal membrane potential has a unique correspondence with neural energy (Wang et al. 2019a ), the time-varying energy flow can reflect the conduction of information flow in the network, that is to say, neural information coding can be expressed by neural energy coding. This is certain that no matter how kaleidoscopic firing patterns of neurons are, how rich and varied are the synchronized oscillation of different frequencies of networks in the nervous system, or the changes in local field potentials, the patterns of energy changes that constrain all these neural activities will always occur with the ever-changing membrane potential or the oscillation mode of the network (Wang et al. 2015b ; Wang and Wang 2014 ).
1.4.3 Advantages of neural energy coding compared with the existing coding theories
Global functional models of the brain based on energy can be used to analyze and describe experimental phenomenological neuroscience at various levels. It makes the computational results at various levels no longer mutually inapplicable, contradictory, and irrelevant. That is to say, neural information can be expressed in energy at various levels of molecules, neurons, networks, cognition, and behavior, as well as at the combination of each level, and a neural energy model can be used to unify the interactions between various levels (Yuan et al. 2021 ; Wang and Zhu 2016 ), which is impossible to do this with any traditional neural coding theory.
It is difficult to simultaneously obtain recordings from multiple cerebral regions. Although EEG and magnetoencephalography (MEG) can obtain neuronal activities from various cerebral regions, estimating cortical interactions based on these extracranial signals is a clinical challenge. The main obstacle is the lack of a theoretical tool capable of efficiently analyzing interactions among each cortical area in high-dimensional spaces. Additionally, a conversion relationship between scalp EEG and cortical potential was not found. The neural energy provides an effective solution to the above-mentioned problems.
As energy is a scalar quantity, whether it is a single neuron or a neuronal population, a network or a behavioral, linear or nonlinear neural model, their dynamic response can be used to describe the mode of neural coding by the method of neural energy superposition (Yuan et al. 2021 ; Peng and Wang 2021 ; Wang et al. 2015b ; Wang and Wang 2014 ). This provides global information about functional neural activity in the brain, which is impossible to achieve with other traditional coding theories.
The mode of network coupling oscillation can be ever-changing, and the coupling oscillation of neural network has a unique relationship with the oscillation of network energy. When the large-scale of the neural network modeling and numerical analysis becomes impossible to handle due to the extremely complexity of high-dimensional nonlinear coupling, it is suggested to use the neural energy coding to study the neural information processing. Thus, complex neural processing can be simply and easily performed without losing information (Zheng et al. 2022 ).
Our proposed model of neuronal energy could also accurately predict the presence of an unknown magnetic substance in the brain (Wang and Zhang 2006 ). After 10Â years, an important academic article was published in the Nature Materials, which experimentally proved the existence of a magnetic protein called MagR in the brain, which can be used for direction and navigation in path exploration (Qin and Xie 2016 ).
As neural energy can describe the interactions at various levels from the global perspective of cerebral activity, neural energy coding can also be used to express various existing neural coding patterns. That is to say, all types of coding patterns based on membrane potential in the past can be expressed by neural energy coding patterns, which are special cases of neural energy coding.
2 Application of analysis dynamics in neuron modeling
2.1 problem raising.
Observed from the Angle of mechanics, the growth of brain nerve system of connections between neurons function circuit formation and regeneration of nerve tissue degeneration after degradation ability, and the dynamics of the growth cone structure and their motions, and the change of the growth cone motion state and its movement trend depends on the interaction forces. Although the mechanics of neuron activity are well understood at the molecular level, all functional neural activity in the brain is based on the activity of 100 billion neurons. During the development of the nervous system, once neurons find their position in the brain, they are both the basic structural unit and the functional unit of the whole nervous system. In order to understand the complex multi-level interaction system of the brain and explore the law and essence behind the various functions of the system, according to the role and contribution of various forces of neuronal axons and dendrites in the signal transmission process. On the basis of previous studies on neurobiology and cell biology, we need to further investigate the effect of neuronal electromagnetic induction on membrane potential changes and corresponding energy metabolism. Some pioneering work has been done by Ma Jun of Lanzhou University of Technology. He pointed out that electromagnetic field effects generated by ion transport inside and outside nerve cells should be considered during electrophysiological activities to further explain the dynamic mechanism of synaptic plasticity from a physical perspective (Wu et al. 2016 ). Based on the principle of electromagnetic induction, magnetic flux was introduced into the neuron model, and induced current was used to express the electromagnetic field effect and external electromagnetic radiation effect generated by ion transport in the cell, which could explain the multi-mode oscillation of neuron electrical activity and the coupling synchronization process of neuron network internal field.
In 1952, Alan Hodgkin and Andrew Huxley gave the first quantitative description of neuron membrane potential (HâH equation), and proposed the concept of ion channel, thus revealing the veil of neuron excitability. Because this research, they won the Nobel Prize in Physiology and Medicine in 1963 and is the only two scientists who have won the Nobel Prize so far for constructing mathematical models. However, they propose that the HâH neuron model is a high-dimensional complex nonlinear equation. Due to the many parameters, although they can reflect many nonlinear properties of nerve cells, they are suitable for the study at the subcellular level. But a large number of neurons can cause considerable computational difficulty, or even impossibility. Therefore, it is not suitable for large-scale neural network and large-scale neural computing research. In order to solve these difficulties, some scholars proposed some simplified neuron models before and after the publication of HâH precise models. Typical model is HindermarshâRose nonlinear model (HR), which can be used as the basic unit of large-scale networks for the study of action potential emission characteristics of single neurons, due to its characteristic and applicable scope of much less computation and parameters than HâH model. The main disadvantage of HR model is that the rich nonlinear dynamic properties obtained under the condition of single neuron or several neurons cannot be directly extended to high-dimensional nonlinear system and neural network system, and there are many limitations in practical application. The integral-and-fire model (IF) can also be used for dynamic research of a large number of neuron clusters due to its simple calculation. However, this model is defective because it cannot record the integrity of neuron membrane potential changes. Compared with HâH model, FithzHugeâNagumo nonlinear model (FHN) has much less computation and can only be used to study the action potential properties of single neurons. The Chay model is a simplified version of HâH model, and the computational workload is relatively reduced. Its main shortcoming is that it is not suitable for large-scale neural network and large-scale neural computation. In fact, these situations not only seriously hinder the complete description of the nervous system in neurodynamics and computational neuroscience, but also seriously hinder the construction of the theoretical system of brain science. This research makes it impossible for us to understand the laws and nature behind big data in neuroscience from a theoretical height. As a result, there will continue to be a lack of a common language between experimental and theoretical neuroscience, leaving a hidden and unbridgeable gap in brain research that is supposed to understand, collaborate and develop together.
2.2 Biophysical mechanism of WâZ neuron model
In conclusion, it is necessary to find a simple and reliable neuron computing model that can perform large-scale combinatorial computation at all levels without losing the main information. The characteristics of this new neuron model are as follows: (1) It must be a neuron model equivalent to HâH model; (2) It is not only applicable to the calculation of the interaction between brain regions, but also applicable to the calculation of all levels of brain and the combination of all levels of brain; (3) At the neural nucleus, mesoscopic, complex network, macro cognitive and behavioral levels, the new neural model can ignore the secondary information but canât lose the main information; (4) The calculation is simple and reliable.
After more than 10Â years of exploration, we have preliminarily found such a new neuron model called WâZ that basically meets the above requirements (Wang et al. 2015a ; Wang and Wang 2018a , b ). When we used analytical mechanics to construct this original neuron model at the cellular level, we accidentally discovered a new working mechanism of neurons. Although HâH neuron model and WâZ neuron model are two neuron models constructed at completely different levels, they are actually equivalent (Wang and Wang 2018b ). The biggest difference between the two neuron models is that inductor elements are introduced into the WâZ model. The biological basis for the inductance element was the discovery of a long-ignored phenomenon in neuroscience experiments. These experimental phenomena provide intracellular records of isolated brain and spinal cord sections of different types of mammals, indicating that central neurons can generate various complex action potential emission patterns and spontaneously and continuously generate action potentials by internal ionic mechanisms when there is no synaptic connection. Even if there is no stimulus input, the mechanism of neuron discharge mode is strongly intrinsically related to the activity of ionic current (Byrne and Roberts 2009 ). Although Ma Jun and other authors did not see the above experimental phenomenon, they started from the perspective of physics and based on WâZ neuron model (Wang and Wang 2018b ) and the working principle of memristor (Wu et al. 2016 ; Lv et al. 2016 ). They keenly feel that the electromagnetic field effect generated by induced current in the process of ion transport in cells and the external electromagnetic radiation effect are keenly felt to contribute to the conduction of neural signals (Lv et al. 2016 ). They applied this method to study the electrical activity behavior of cardiac tissue under electromagnetic radiation and predicted the mechanism of sudden cardiac death and shock induced by electromagnetic radiation (Lv et al. 2016 ; Ma et al. 2017 ). Thus, the physical mechanism of synaptic plasticity of neurons is scientifically explained. The process of task synaptic current triggering is accompanied by the generation of electromagnetic fields. Therefore, the combination of capacitors and induction coils can be used to simulate the function of hybrid synapses (Ma et al. 2019 ).
Alan Hodgkin and Andrew Huxley show that ionic current can be described as the product of conductance and driving force (the difference between the membrane voltage and the ionic Nernst potential) in the case of constant membrane permeability. Conductance reflects the permeability of cell membrane to ions, while the driving force reflects the trend of ionic current movement of charged particles in the fluid inside and outside the cell under the dual effects of electric field gradient and concentration gradient. Lots of experimental data (Byrne and Roberts 2009 ) shows that since the resting potential of the neuron is not at the equilibrium potential level of any particular ion, various ions continue to diffuse along the concentration difference, which is evident in the generation of action potentials and synaptic potentials. Therefore, cells need to recover through active transport by the sodiumâpotassium pump, which is thought to complete transport through allosteric effects of protein phosphorylation and dephosphorylation. When the membrane potential exceeds the threshold for an action potential, it initiates a large, transient inward current (sodium current) followed by a persistent outward current (potassium current). They showed that when the membrane potential was depolarized, the sodium current (sodium conductance) was rapidly activated and then inactivated. The potassium current (potassium conductance) is activated after a delay, and a high activation level remains as long as depolarization is maintained. As the inactivation of sodium channel is slower than activation, when the sodium channel is activated but not inactivated, a large amount of sodium ions flow in, leading to the increase of sodium current, forming a positive feed. It then switches to repolarization due to sodium channel inactivation and potassium channel activation to prepare for the next action potential. All kinds of ion currents in HâH model, no matter sodium current or potassium current, are very weak in each ion channel, so it is difficult to construct their own self-induction effects at the level of ion channel. Although the magnetic field intensity in the nervous system is very weak (Liu 2002 ), the electromagnetic induction effect exists objectively. Given this objective fact, our neuronal model is built directly on the level of nerve cells, the total effect of self-induction of all types of ion channel currents can be expressed in terms of an inductance. This inductance can in fact be used to express the total effect of the magnetic field on the movement of all the ionic currents represented in the HâH model. This is the biological explanation for why inductance is introduced in the WâZ model, and the underlying reason why HâH and WâZ models are the equivalent neuron models.
2.3 Analytical dynamics reveals new working principles of neuronal activity
Based on the experimental data provided by electrophysiology and the working characteristics of neurons, we present the biophysical model of coupled neuron activity as shown in Fig. 1 . This model reflects the interaction of a single neuron with all other neurons connected to it. The mutual coupling between neurons is realized by the current formed by the input of the first neuron to the first neuron in the cerebral cortex.
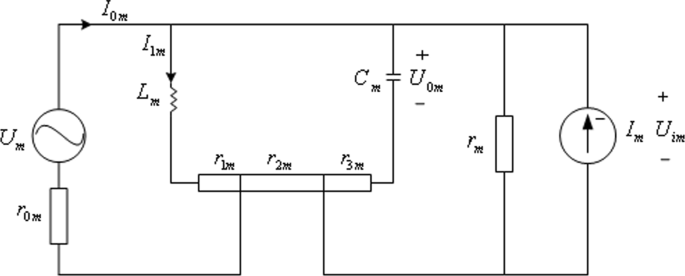
Schematic description of WâZ model (Wang et al. 2015a )
According to Fig. 1 , we get the following circuit equation:
Among them, membrane capacitance represents the accumulation of positive and negative ions inside and outside the cell membrane. It is the heat loss caused by the collision of the inner and outer membranes during ion exchange. As mentioned above, in this new neuron model, we have introduced an inductance element that is not in the HâH model, which means that each ion channel on the cell membrane produces a self-induction effect caused by a loop current flowing through multiple ions.
It contains the transport process of a large number of charged ions in nerve cells such as sodium, potassium and calcium ions in ion channels, which can trigger uniform or non-uniform electromagnetic fields inside and outside the cell, which in turn will affect the transport of charged ions. That is to say, the change of the charge distribution density in the cell and the electromagnetic field effect produced during the charge transport process will cause the induced current in the cell, which is exactly what the traditional neuron model does not consider (Ma et al. 2019 ). Represents the heat consumed by ions colliding with each other under the condition of current generation, which can be equivalent to resistance. m can be expressed as the chemical gradient inside the neuron, which will drive the flow of ions, and can be simulated by a voltage source or a current source in the electrical model. It is the loss caused by the unsatisfactory voltage source. And means that in addition to the chemical gradient, the neuron also accepts the action of other neurons, and at the same time maintains the resting potential of the neuron at rest. In order to express this function, set the input current as
Among them is to maintain the resting potential, and the rest represents the input of the surrounding N neurons to the mth neuron. m is the frequency of the action potential. It is the resistance across and the loss caused by the imperfect current source. It is stimulated by peripheral neurons when it is subliminal. When the action potential is issued, it is not affected by external influences, and is affected by the internal mechanism of neurons. The effect is that the voltage source and the current source are not produced at the same site. The voltage source mainly provides a small loop current of the ion channel, and the current source mainly accepts the stimulation of peripheral neurons, and they are almost closed to each other, but there is an internal connection between them, which can be represented by resistance. The observable physical quantities in this physical model are membrane potential and membrane current respectively.
The total power of N neurons can be expressed as
According to the circuit diagram, the power of the neuron is given by the following formula:
The parameter \(d_{im} \;(i = 1,\;2, \ldots ,6)\) can be found in literature (Wang et al. 2015a ).
Since the expression of the voltage source U m cannot be obtained from the circuit model in Fig. 1 , generally the membrane potential cannot be determined. However, the interaction between coupled neurons in the cerebral cortex is orderly and follows the law of self-organization (Gu and Liang 2007 ; Haken 1996 ), and convincing evidence is that the data provided by neuroscientists at Yale University has confirmed that the activity of neurons in the cerebral cortex requires consume energy (Raichle and Gusnard 2002 ; Maandag et al. 2007 ; Lin et al. 2010 ). Their research work shows that compared with the resting state, the energy consumption of the brain under stimulation is mainly used for the propagation of action potentials, and neurotransmitter stimulation of receptors causes the restoration of post-synaptic ion current (Lin et al. 2010 ).
The neuron cluster model established by us with m neurons under coupling conditions can describe the basic characteristics of the subthreshold and threshold electrical activity of neuron cluster through its current coupling relationship with surrounding neurons (Wang et al. 2008 , 2009 ). However, the membrane potential cannot be obtained directly from the model. If we can find the functional form of neuron energy consumption and find the constraint conditions of neuron motion equation controlled by the energy function, we can get the solution of membrane potential. Due to spontaneous electrical activity of neuron clusters obey the law of self-organization (Haken 1996 ), at the same time, according to Yale university neuroscientist on nerve signal transmission and energy dissipation of tightly coupled with the experimental results, we determine the constraint condition of the given the new neuron model is likely to be the energy function in the circuit system. In mechanical analysis, we know that for a known dynamic system, we can write down the kinetic energy and potential energy of the system, thus obtaining its Lagrange function. But in our neuron electrical model, we assume that potential energy is equal to a constant, (and power is the average energy). It can be assumed that the power consumed on the circuit model can be regarded as the energy function of the dynamic system, thus leading to the Lagrange function as the constraint condition of the circuit model, which plays a key role in describing the neuron model completely. Whether such an idea is reasonable depends on whether the results obtained by such an idea agree with the results of neuroelectrophysiological experiments. The genius of this idea is to extend the modeling of dynamical systems from classical mechanical systems to nervous systems.
According to the above ideas, suppose that the Lagrange function in the model is related to the total power of the circuit model, and its dynamics equation is given by the following equation
The solution to the above equation is
When i 0 m ( n ) is strongly stimulated and the membrane potential reaches the threshold level, we obtain the neuron membrane potential U 0m and the corresponding energy function P m , as shown in Fig. 2 .
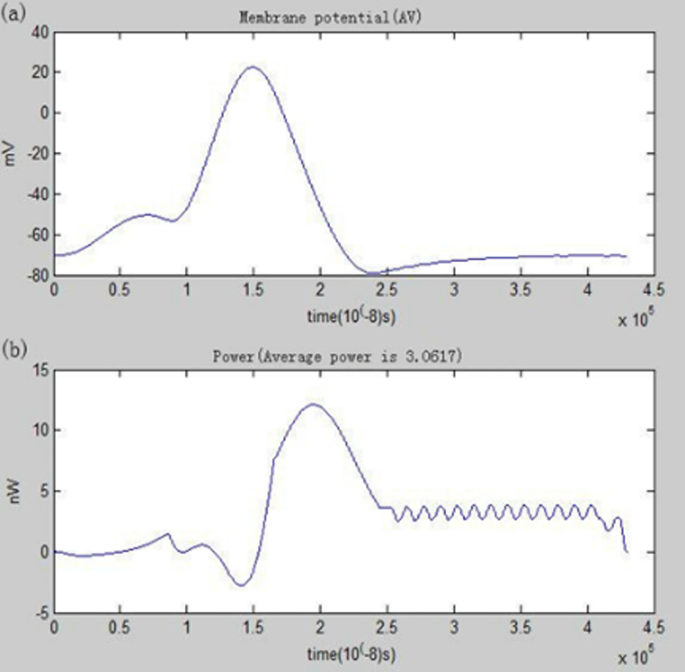
Action potential ( a ) and corresponding energy function ( b ) (Wang et al. 2015a )
According to the calculated results, the action potential waveform is completely consistent with the experimental data, thus confirming our previous judgment is correct. It is important to note that the results of the analytical mechanics calculations show for the first time a previously undiscovered phenomenon that when neurons fire an action potential, the corresponding energy expenditure does not correspond to the conventional view of neuroscience, which is that neurons only use energy. It's about absorbing energy and then expending energy before expending energy. In fact, during the production of an action potential, the energy change of the neuron is composed of two parts. One part is the negative energy of the oxygenated hemoglobin obtained from the blood stream, which is used for energy storage. On the other hand, deoxygenated hemoglobin presents positive energy for energy consumption (Wang et al. 2015a ; Wang and Wang 2018a , b ). For this novel energy calculation result, Zheng et al. (Zheng et al. 2014 , 2016 ), combined with molecular biology knowledge and existing experimental data, provided a qualitative explanation for the regulation process of ion channel opening and closing, glutamate circulation and glucose by neurons and related glial cells during action potential generation. It is pointed out that the negative energy in action potential is a process of energy storage, that is, the amount of glucose and oxygen absorbed from blood stream is greater than the amount needed to consume. In other words, stimulated neurons will lead to increased cerebral blood flow, but there is a demand for oxygen consumption during depolarization (there is no oxygen consumption at the moment), mainly in the form of energy absorption. In the repolarization stage of neurons, the energy storage has been consumed, and at this time the oxygen consumption of neurons increases significantly, which is manifested as energy consumption. In short, the neuron is not only a energy-consuming device but also an energy-storing device. From the perspective of an action potential, a neuron absorbs energy from the bloodstream and then uses it up again and again, reaching a dynamic equilibrium, suggesting that the energy storage capacity of a single neuron is limited. From the perspective of glucose and oxygen supply in blood stream, when the glucose and oxygen supply in blood stream is sufficient and the energy storage of neurons is not up to the upper limit, neurons will spontaneously reserve energy at the initial stage of action potential depolarization. This may also explain why the oxygen metabolic rate, CMRO 2 , changes more rapidly than blood flow CBF, reflecting the fact that neurons release stored oxygen in response to stimuli and then consume oxygen and glucose from the bloodstream. We have explained the neural mechanism of cerebral hemodynamic phenomena from a quantitative perspective (Peng and Wang 2021 ).
It emphasized that the existence of negative power component in the energy function corresponding to the firing of action potential by neurons is an extremely important new discovery of the working mechanism of neurons (Wang et al. 2015a ). This new mechanism reveals two previously undiscovered patterns of neuronal activity. The first is that there is a corresponding relationship between membrane potential discharge of neurons and nerve energy. The second is that neurons consume energy when they are active below the threshold value, while absorb and consume energy when they are active above the threshold value. The first law reveals a unique correspondence between functional acquisition of neuron membrane potential and energy function, which has been strongly confirmed by HâH models (Wang and Wang 2018b ). The second rule verifies an experimental phenomenon currently unexplained by neuroscience, namely, activation of brain regions increases blood flow by 31% while oxygen consumption increases by only 6% (Zheng et al. 2016 ), a relationship approximately equal to 5:1. Our calculation shows that the area of the positive and negative interval in the energy curve in Fig. 2 is approximately equal to 5:1 (Adachi and Aihara 1997 ). It should be emphasized here that the positive and negative areas in this power curve have profound neurobiological significance: the positive and negative areas well correspond to the experimental result that blood flow increases about 31% during stimulus-induced neuronal activity, while the associated oxygen consumption increases only about 6% (Zheng et al. 2016 ). The use of the negative power component can also explain the hemodynamic phenomenon of the brain, that is, the significant increase in blood flow after the activation of the designated cortical area is delayed by 7â8 s from the activation moment. It also explains why tactile perception is synchronized with the emergence of consciousness and so on. The new neuron model based on experimental data enables us to propose the concept, theory and method of energy coding in an original way (Wang et al. 2008 , 2009 , 2015a , b , 2017a , b , 2018a , b , 2019b , 2021a ; Yuan et al. 2021 ; Wang and Zhu 2016 ; Wang and Wang 2018b , 2020 , 2014 ; Li et al. 2022a ; Peng and Wang 2021 ; Zheng et al. 2014 , 2016 , 2022 ; Wang and Zhang 2006 ). This new concept and coding theory can not only explain some experimental phenomena that cannot be explained by neuroscience so far and quantitatively reveal the laws behind some experimental data, but also predict some phenomena that cannot be discovered by experimental neuroscience. When we fully understand and master the above nature of neuronal activity, we will have a new understanding of the rules of neural information processing and the principle of neural coding in the cerebral cortex. This fully reflects the influence and role of mechanics in promoting the progress of neuroscience and the influence and role of mechanics in the field of neuroscience and life science.
According to the discovery of the new working mechanism of neurons, the neuron model provided by us can also be used to quantitatively prove that the operating mode of brain works in accordance with the following criteria (Zheng et al. 2022 ; Laughlin and Sejnowski 2003b ): (1) EconomyâThe neural network activity conforms to the energy minimization principle when the brain is at rest and participating in cognitive activities (Zheng et al. 2022 ); (2) High efficiencyâthe transmission efficiency of cortical neural network signals conforms to the principle of maximum energy utilization (Zheng et al. 2022 ); (3) Self-organizing neural computationsâthe relationship between membrane potential and energy reflects the coupling relationship between neural information and cerebral blood flow (Moore and Cao 2008 ; Fox and Raichle 2007a ; Raichle and Gusnard 2002 ; Lin et al. 2010 ). In addition, using this new neuron model, not only the action potential and the corresponding energy consumption of neurons can be simulated, but also the waveforms of presynaptic excitatory potential (EPSP) and postsynaptic inhibitory potential (IPSP) and the corresponding energy of EPSP and IPSP can be simulated, and the simulation results are in complete agreement with experimental records (Wang et al. 2015a ). This original neuronal model has been supported by a large number of neuroelectrophysiological experiments. The WâZ neuron model constructed by us and the coding theory of neural energy make it possible to transform a variety of complex, coupled and highly nonlinear membrane potential firing modes into energy firing modes for coding studies (Wang et al. 2008 , 2009 , 2015a , b , 2017a , b , 2018a , b , 2019b , 2021a ,; Yuan et al. 2021 ; Wang and Zhu 2016 ; Wang and Wang 2014 , 2018b , 2020 ; Li et al. 2022a ; Peng and Wang 2021 ; Zheng et al. 2014 , 2016 , 2022 ; Wang and Zhang 2006 ). The theory holds that the encoding of neural information is closely related to the metabolism of neural energy, and the mechanism of encoding of neural information can be understood and revealed by using the energy method. What is particularly interesting is that the brain power calculated by WâZ neuron model is about 45 W, while the brain power provided by experimental data is 20 W (Wang et al. 2015a ). This is also the first time that the power consumption of our own brains has been quantified.
Thus, the discovery of this new and important working mechanism of neurons not only depends on the creative application of the theory of analytical mechanics in neuroscience, but also can perfectly bind neural information and neural energy together, laying a solid foundation for the research framework of global neural coding of the brain.
Experimental data show that the brain consumes only about 5% more energy in the task state than in the resting state (Fox and Raichle 2007a ). The maximum energy consumption in the resting state comes from the DMN, which consumes more than 95% of the brain energy consumption in the resting state (Peppiatt and Attwell 2004 ). Much of our understanding of the brain used to come from studying the activity of this 5%. Because the structure of different brain regions and their individual neural activity patterns are different, neuroscientists often use dynamic BOLD signals measured by fMRI to look at brain activity as a whole in order to obtain global information about brain activity. However, the average distribution of blood flow in the brain when the brain is activated and the nonlinear coupling relationship between blood flow and oxygen consumption make it difficult for us to obtain a quantitative and accurate understanding of the neural activity of the brain in various states, and we cannot understand the interaction between neurons in the activated brain using this technology (Clancy et al. 2017 ). At present, there is no new experimental technology in the field of neuroscience that can perfectly integrate the accuracy of neural electrophysiological recording with fMRI technology that reflects the global information of brain functional activity, including optogenetics technology which is only locally observable. If neuroscience cannot achieve such a new technique for a long time in the future, can we theoretically propose a new research method that combines the reductionism of the activity of individual neurons and the holism of the macroscopic effects of the brain, and take it as the main basis for studying the global neural activity of brain function. This new research method can not only accurately reproduce neural electrophysiology record, also can use fMRI data provided by the large repeat reproduce the global information of brain functional activity, and it also can be estimated in theory we haven't found the new phenomenon of neural activity, like found that neurons in the energy consumption negative power component so to do that involves understanding the nature of neuronal activity in the brain and to do that we need to compare the HâH neuronal model to the WâZ neuronal model, right through the analysis and research of these two types of different neuron models, we can explain what factors dominate and control the various releasing modes of complex membrane potential of neurons, so as to understand and master the nature and change rules of neural information processing and signal transduction.
2.4 Equivalence between WâZ neuron model and HâH model and its molecular biological basis
In order to verify the validity of WâZ neuron model established by analytical mechanics method, we used HâH model to calculate the energy characteristics of action potential and membrane potential (Wang and Wang 2018b ). That's because almost all previous work has been based on how neural activity causes changes in nerve energy. Under the same stimulation conditions, whether the neurons can transition from sub-threshold activity to supra-threshold activity is depended on whether the neurons can be fully energized. The two states of energy supply (both sub-threshold and supra-threshold) determine whether the ion pump can provide stable Nernst potential for sodium ions. That is, from the perspective of energy, the Nernst potential of sodium ion closely connects the sub-threshold and the supra-threshold activity. The inverse of this question is whether changes in nerve energy cause changes in neuronal activity, under insufficient energy supply, neurons will only manifest as the sub-threshold activity when stimulated, but cannot fire action potentials. In general, we are usually concerned about the imbalance distribution of neural energy caused by neural activities under stimulation. However, neural activities are also modulated and constrained by neural energy. The mechanism is that ion pump that lacks energy supply cannot provide constant Nernst potential for sodium ions, and in the early stage of the change of cell membrane permeability, it cannot provide continuous flow of sodium ions to reach the threshold potential (Hopfield 2010 ; Hu et al. 2021 ). Thus, the electrophysiological activity of neurons is strictly constrained by energy levels in the brain. When energy supply of ion pump of the sodium ion channels is insufficient, the response of neurons is sub-threshold activity to any form and intensity of stimulation. But only when the maximum power of sodium ion channels is not constrained, the neurons will be sup-threshold firing.
So it is necessary through calculating model to understand why when ion pump when there is no guarantee that a constant potential, namely when neurons system energy shortage, energy is how to regulate neuronal activity Its scientific significance is when compared to the resting state network energy consumption, the task of related part of the increase of energy consumption is small (less than or equal to 5%). So most of what we know and understand about brain function so far comes from this tiny fraction of brain activity and if we want to get a complete picture of how the brain works, we have to think about the part that consumes most of the energy which is the innate spontaneous neural activity, right. Therefore, we need to further investigate the HâH neuron model established on the level of ion channel, and explore the nature of neuron activity through the comparative study of these two types of neuron models.
The circuit model of HâH equation is shown in Fig. 3 .
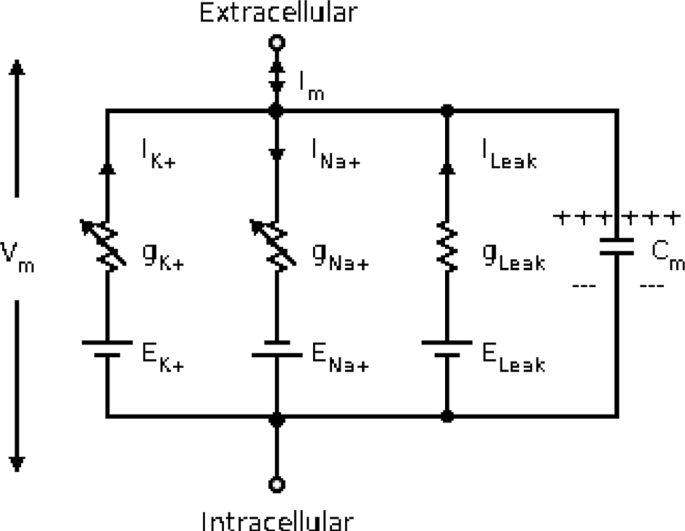
Schematic description of HâH model (Wang and Wang 2018b )
Its differential equation is described as
where C m is the membrane capacitance of neuron membrane, V m is the membrane potential, / E Na and E K are the Nernst potential of sodium ion and potassium ion respectively, El is the potential when the leakage current is zero. \(\hat{g}_{{{\text{Na}}}}\) and \(\hat{g}_{{\text{K}}}\) sodium channel and potassium channels of variable conductance, \(\hat{g}_{{{\text{Na}}}} = g_{{{\text{Na}}}} m^{3} h,\;\hat{g}_{{\text{K}}} = g_{{\text{K}}} m^{4} ,\;g_{l}\) for leakage conductance. The variable conductance of sodium and potassium channels is described by the following set of nonlinear differential equations:
Each parameter in the above equation can be found in Tsuda ( 1991 ).
In HâH equation of circuit model, the total energy can be table as follows:
Including IV m to provide energy to the outside world on the circuit system, \((i_{{{\text{Na}}}} E_{{{\text{Na}}}} + i_{{\text{K}}} E_{{\text{K}}} + i_{l} E_{l} )\) to represent the Nernst potential energy provided by the voltage source, While \(V_{m} (i_{{{\text{Na}}}} + i_{{\text{K}}} + i_{l} )\) is the energy in membrane potential difference inside and outside. In the above process of releasing action potential from neurons, if the energy consumed by the change of membrane permeability is not taken into account, the energy involved is the energy provided by oxygen and glucose carried by blood flow to neurons respectively (the energy provided by the outside world to the circuit system). The increase in glucose consumption due to brain stimulation is mainly due to activation of the sodiumâpotassium ATP pump (Zheng et al. 2014 , 2016 ; Sokoloff 2008 ; Maandag 2007 ). Described in the first two of them are under the threshold of neurons and the relationship between biological energy, and the ion concentration difference through ion channels to help spread does not consume energy From the perspective of dynamic observation, however, under the threshold of neurons into functional neurons in the process, the sum of the three types of energy and HâH model of circuit is equal to the total energy in the system. The former two types of energy (the outside world to provide energy and the energy of the voltage source) and the circuit of IV m and \(V_{m} (i_{{{\text{Na}}}} + i_{{\text{K}}} + i_{l} )\) correspond, so the energy provided by the Nernst potential \((i_{{{\text{Na}}}} E_{{{\text{Na}}}} + i_{{\text{K}}} E_{{\text{K}}} + i_{l} E_{l} )\) equals the biological energy consumption of ion pump. In fact, in this process, the sodiumâpotassium pump continuously transports ions against the ion concentration gradient, which directly consumes biological energy, that is, one ATP can pump three sodium ions and two potassium ions. This also confirms that due to the existence of the ion pump, a stable Nernst potential is provided by the continuous transport of ions, thereby providing energy for neural activity. Therefore, we can calculate the power consumed by the ion pump by using the power of the voltage source represented by the Nernst potential, that is, the nerve energy consumed by neuronal activity is:
The negative sign of the third term in Eq. ( 16 ) refers to the fact that in the circuit shown in Fig. 1 , the voltage source/and current/are in opposite directions to E Na , i Na and E K and E l (the sodium current is inward, while the potassium current and leakage flow outward). For an action potential, we can use the above equation to calculate the neural energy expend.
The calculated parameter values in Fig. 4 can be found in Wang and Wang 2018b It can be seen that although the action potential of HâH model and WâZ model has some errors in waveform (mainly because WâZ model is constructed at different levels as HâH model), the nerve energy of HâH model also has negative power component, and almost has the same dynamic characteristics as that of WâZ neuron model. This result shows that the neuron energy model proposed by us has a deep internal connection with the HâH model. From the perspective of computation, HâH neuron model needs to calculate the conductance and current of multiple ion channels, so if HâH neurons are used to construct a large number of neuron networks, it will cost a lot of computation cost. However, if WâZ neurons are used to construct the network hierarchical model with a large number of neurons, it has a greater advantage because of its greatly reduced computational complexity (see Fig. 4 ).
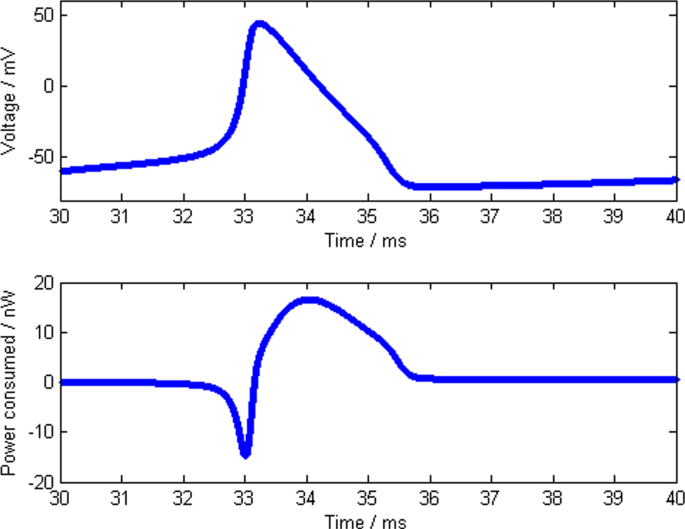
Neuron action potential and corresponding power consumption based on HâH model (Wang and Wang 2018b )
We can make biological explanation for the negative power component of neuron energy at the initial stage of firing action potential as follows.
This is mainly due to local congestion caused by nerve activity. As blood vessels dilate and blood flow increases, the amount of arterial inflow increases, leading to an increase in oxygenated hemoglobin into the blood vessels (Peppiatt and Attwell 2004 ). Neurons take oxygen mainly through oxygenated hemoglobin, but the consumption of O 2 does not increase proportionally with the increase in blood flow and O 2 (Peppiatt and Attwell 2004 ). Fox et al. observed in PET that the event-induced oxygen uptake coefficient (OEF) decreased from 40% at rest to 20%, meaning that 80% of the oxygen delivered during the event was not physiologically metabolized. This suggests that the energy requirements associated with neural activation (compared to resting state requirements) are small and that the hyperemic response of cerebral blood flow is influenced by products of non-oxidative metabolism such as lactic acid (Lin et al. 2010 ). Functional congestion plays a direct role in neuronal information processing. Local blood vessels dilate due to increased blood flow, increased blood volume and increased local vascular pressure. The anatomy shows that neurons and glial cells are located near blood vessels, so the dilation of blood vessels causes the deformation of cell membranes. Membrane deformation caused by these mechanical force signals such as blood flow, blood volume, pressure and local dilation and contraction of blood vessels can regulate ion channels sensitive to mechanical force, thus altering neural activity (Moore and Cao 2008 ; Lin et al. 2010 ; Peppiatt and Attwell 2004 ). For example, in the somatosensory cortex, sensory stimulation induced an increase in the diameter of the arterioles, a mean net increase \(10\sim 15\mu m\) , with some experimental data suggesting that the dilation was even greater \(15\mu m\) (Moore and Cao 2008 ). According to Poiseuille's theory, a 23% reduction in blood vessel diameter results in a threefold decrease in blood flow, while an increase in blood vessel diameter results in a four- or fivefold increase in blood flow. In addition, blood flow plays a dominant role in the brain's temperature. Local congestion lowers brain temperature and reduces the effect of heat due to neural activity (see Fig. 5 ) (Moore and Cao 2008 ).
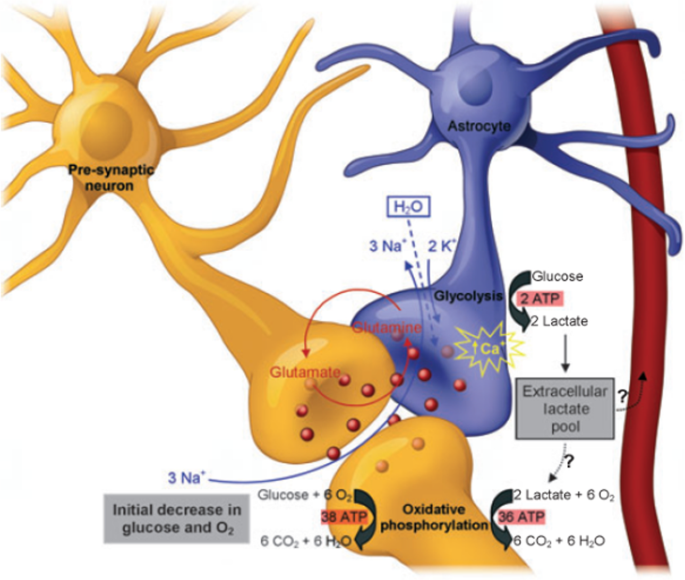
Cellular/molecular and haemodynamic changes caused by lactate products and calcium waves in astrocyte end feet (Zheng et al. 2014 )
At the molecular level, glial cells, the most abundant cell type in the brain, have long been thought to function only as support and nourishment for neurons. But glial cells have recently been shown to play a crucial role in neural activity. It not only affects the growth and development of neurons, but also may directly participate in the transmission process of nerve signals. Astrocytes are the most abundant in glial cells, and glycogen in the brain is mainly stored in astrocytes. Magistretti et al. Astrocyte lactate shuttle hypothesis, ANLSH (Pellerin and Magistretti 1994 ), indicating that astrocytes play a crucial role in nerve energy metabolism and hemodynamics. Currently, the role of brain glycogen is not completely clear, but a large number of studies (Pellerin and Magistretti 1994 ; Brown 2004a , b ; DiNuzzo et al. 2012 ) have shown that brain glycogen is a very important brain energy reserve and the material basis of brain activity.
The increased activity of Na + , K + and Ca 2+ channels increased ATP consumption and stimulates ATP production. As shown in, during glycolysis of glucose in glial cells, one molecule of glucose produces two molecules of lactic acid and two ATPs, which are just used for glutamate uptake and metabolism. A molecule of glutamate, along with three Na + , is taken into the astrocyte via the cotransporter, which activates the Na + /K + pump to restore the osmotic gradient. Glutamate enters astrocytes and is converted into substances such as glutamine, which is sent back to neighboring neurons. Moreover, Na + uptake is a passive process; activation of the Na + /K + pump and conversion of glutamate to glutamine are energy consuming processes (each consuming 1 ATP) (Figley and Stroman 2011 ). Lactate, the product of glycolysis, is transported out of the cell through the lactate shuttling protein on the cell membrane, which is then absorbed by neighboring neurons, producing 36 ATP after oxidative metabolism (Sokoloff 2008 ). It can be seen that although increased blood flow is mainly due to increased concentration of lactic acid, a product of non-oxidative metabolism. Most (98%) of the energy requirement comes from oxidative metabolic pathways (Eikenberry and Marmarelis 2015 ). So when the activity of brain tissue increases, the corresponding energy demand rises rapidly, but the brain blood flow does not change enough, resulting in a lack of blood sugar. At this point, brain glycogen is rapidly digested to meet the energy needs of brain tissue activity (Zheng et al. 2014 ).
The above are the molecular mechanisms of negative power components caused by local congestion in the brain and the mechanical mechanisms of increased blood flow caused by vascular dilation. Thus, the above on vascular smooth muscle relaxation caused by different kinds of nerve chemical reactions lead to increased blood flow to the brain, in turn, control the neural activity in the brain is a neurochemistry, neural signal transduction, cell rheology of nonlinear viscoelastic mechanics, blood, blood vessels, non-Newtonian fluid mechanics and damage mechanics of multidisciplinary crossover study, There are a lot of mechanics problems which need to be further explored and solved by mechanics workers (Zheng et al. 2016 ).
From the perspective of the circuit, the power emitted by the voltage source represented by Nernst potential is negative, indicating that the work done by other components in the circuit to the voltage source is mainly done by the surrounding glial cells and neurons through various mechanical forces. This stage of capacitor discharge releases the energy stored in the capacitor (brain glycogen in the brain). Capacitance in the HâH model corresponds to the cell membrane of the neuron, while sodium ions enter the neuron through ion channels under the action of potential gradient caused by the potential difference between inside and outside the membrane. This can be regarded as the storage of cell membrane provides energy for the inward flow of sodium ions, which exactly corresponds to the discharge of the capacitor. It can be seen from the above discussion that the movement of ions corresponds completely to the circuit model (Wang and Wang 2018b ).
Fig. 6 correspond to the enlargement of the upper and lower graphs on the right. It can be seen from Fig. 6 that when the sodium ion pump cannot provide stable Nernst potential, the activity of subthreshold membrane potential is mainly energy consumption. When the DMN is coupled with the resting state network, this explains why about 95% of the energy expenditure is devoted to the brain's intrinsic, spontaneous activity, whereas the neural energy expenditure caused by task stimulation usually accounts for only 5% of the brain energy expenditure under the resting state condition.
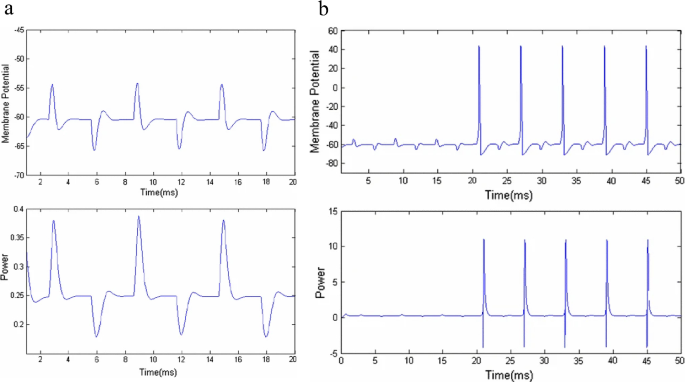
Neuron activity under different energy supply states (Wang and Wang 2018b )
In short, there are many coding theories in the field of neural coding such as frequency coding, rhythm coding, time coding, phase coding and other coding methods. However, they can only be applied to local, single or few neuron systems and isolated or closed neural networks, while the actual neural coding must be the global neural coding of a large range, various levels of coupling and the interaction of various related brain regions. Energy coding studies neural coding from the energy characteristics of neuron activity, which can reflect global, economic and high efficiency advantages (Yuan et al. 2021 ; Wang and Zhu 2016 ; Peng and Wang 2021 ; Wang et al. 2009 , 2015b , 2017a , 2018a , b , 2019b ; Wang and Wang 2014 , 2020 ; Wang and Zhang 2011 ; Zhu et al. 2018b ). Objectively, the inductance element presented in the WâZ model not only theoretically proves the contribution of electromagnetic field effect to signal conduction and information encoding, but also provides a theoretical basis for predicting the existence of an unknown magnetic substance in the brain. In the 10Â years since we first proposed the embryonic neural energy model in 2006 (Wang and Zhang 2006 ), we have seen an important academic paper published in Nature Material that experimentally demonstrated the existence of a magnetic protein called MagR in the brain. Navigation for direction and orientation in path exploration (Qin and Xie 2016 ).
In short, complex changes in action potential and subthreshold membrane potential can reveal rich dynamic properties of neuronal firing activity. Our study not only reveals the equivalence of two kinds of different neuron models, but also discovers the nature and law of neuron firing activity behind the rich dynamic properties and a large amount of experimental data. From a reductionist point of view, this is an important contribution to neuroscience.
2.5 Neural energy mechanism of cluster release and WâZ neuron model
Cluster firing is also one of the common firing patterns of neurons. However, does cluster release also have the above properties? So far, the cellular mechanism of cluster release and its biological significance remain unclear. Therefore, from the perspective of energy, we proposed a neural energy calculation method based on The Chay cluster release model, and analyzed the ion current and its energy consumption (power) per unit time under two conditions with and without stimulation. Studies have found that the power becomes negative during the depolarization process of cluster release, which is consistent with the research results of WâZ neural energy model (Zhu et al. 2019 ). Furthermore, it was found that the energy consumption of neurons in cluster firing mode was minimal, especially in the spontaneous state without stimulation. The total energy consumption of cluster firing for 30Â s was equivalent to the biological energy consumed by a single action potential. These results suggest that low-energy cluster firing is an energy-efficient way of neural information transmission that follows a brain strategy that minimizes energy. The energy efficiency of neural information transmission is considered to be an important constraint of neural information processing. It is usually measured by the energy consumed per unit of information. Most previous studies have focused on the energy efficiency of individual action potentials. However, neural information is more likely to be encoded by a spike sequence rather than a single spike. So far, it is not clear how energy efficiency depends on the discharge pattern of spike sequences. We simulate high, medium, and low frequency emission patterns based on the Chay neuron model and examine their energy efficiency. The results show that medium frequency mode is more effective than high and low frequency mode. Sparse cluster scattering (SBF) mode is the most effective, because it consumes the least energy and transmits the same amount of neural information as the high-frequency mode which consumes more energy. The SBF model minimizes energy consumption by balancing the potential energy stored in the depletion ion concentration gradient. In addition, the combination of sparse cluster release (SBF) with a single spike maximizes the neural information carried by the SBF model, thus improving the energy efficiency. In conclusion, the nervous system may prioritize limiting energy costs over maximizing information to achieve higher energy efficiency (Zhu et al. 2020 ).
3 Equivalence between HâH model and WâZ model in structural network
It has been known that the neural activity of the brain and the operation of the brain are subject to the principle of minimization of energy and maximization of signal transmission efficiency (Laughlin and Sejnowski 2003b ). This rigorous working mode of the brain has been proved by a large number of experimental data. This working principle governs the activity of the entire brain, but its role and contribution to cognition need to be further understood. In order to find the intrinsic correlation and essential connection between cognitive behavior and energy information, it is necessary to construct a series of structural and functional neural networks, and under what conditions the transformation from structural network to functional network should be solved, which involves large-scale neuroscience modeling and analysis.
3.1 Derived from the definition of large-scale neuroscience models based on analytical dynamics
Large-scale neuroscience model is based on neural energy model, and the construction of neural energy model is derived from the theory and method of analyzing dynamics. Its purpose is to quantitatively obtain the global information of brain neural activity through the correspondence between nerve energy and membrane potential, field potential and network emission rate. Since the global information of the brain can be converted into energy for study and analysis, neural energy coding forms the cornerstone of large-scale neuroscience models. Its definition is as follows:
(1) It can analyze and interpret both local and global neural activities of the brain. At the same time, it can also be used to construct, analyze and describe the experimental phenomena of neuroscience at various levels from molecular to behavioral, and can establish the global brain function model on the combination of various levels, so that the calculation results at various levels are no longer unusable, contradictory and irrelevant. (2) Global brain function model can be used to solve the scalp EEG and conversion relation between cortical potentials. It is still difficult to describe large-scale neuronal interactions throughout the brain. It is currently difficult to record damage in multiple brain regions simultaneously. Although EEG and MEG can sample neuronal activity from various regions of the brain, it is very difficult to estimate cortical interactions on the basis of these extracranial signals, the main obstacle being the lack of a theoretical tool that can effectively analyze cortical to cortical interactions in high dimensional space. In addition, there is a lack of a conversion relationship between scalp electroencephalogram and cortical potential. One promising approach to these extremely difficult problems is the neuro-energy theory. (3) Can be used for insight and analysis of experimental data behind the essence and regularity of problems (such as oxygen dependencies between signals and state of consciousness; the meaning and the content of the EEG of brain waves, etc.). (4) If a global brain activity model can under the condition of the degraded to explain the function of the DMN and static state interest, energy consumption, and under the task induced can explain the formation of the transition from the default mode to the cognitive network and the corresponding energy conversion, such a model is a global neural model. And the tunable parameters of the global model of brain function must be few and simple.
3.2 Comparison of network calculation results based on class HâH neuron model
At the level of individual neurons, we have proved the equivalence of HâH and ZâW neuron models, but we still need to prove whether HâH and ZâW neuron models are also equivalent at the level of networks. If equivalent, the WâZ neuron model can be used to study cognition and behavior. As described above, WâZ model is much simpler than HâH model in terms of calculation and has the advantage of not losing the main information. Therefore, when studying the macro behavior model related to cognition, details such as ion concentration and ion current of synaptic connection between neurons need not be considered. Their dynamic characteristics are focused on the macro expression of cognitive and behavioral coding patterns.
A simple structural neural network is constructed according to the connection mode of neurons in functional columns of cerebral cortex (Fig. 7 ).
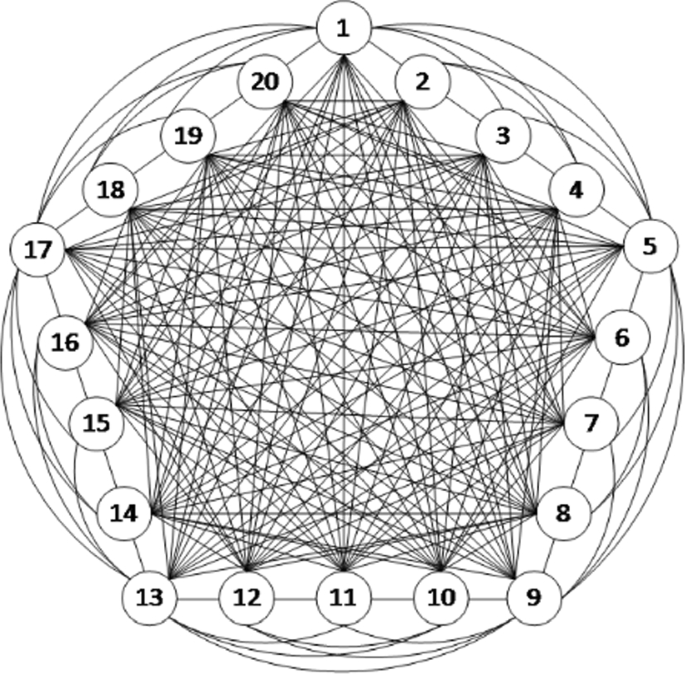
Schematic diagram of a fully connected structured network (Wang and Wang 2014 )
In the fully connected neural network structure shown in Fig. 7 , each neuron is composed of HâH model, and the coding mode of various parameter conditions and the behavioral response of the network are simulated through two different index systems (Wang et al. 2015b ). The research objective is to explore the relationship between synchronous discharge activity and network parameters of structural neural network, and to investigate the equivalence of HâH model and WâZ model under the same network structure as shown in Fig. 7 .
According to equivalent circuit Fig. 3 of HâH model, its differential equation and variable conductance of ion channel are expressed by Eqs. ( 10 ) and ( 11 ).
In the circuit model of HâH equation, the total power of the fully connected network in Fig. 7 can be obtained according to the total energy Eq. ( 12 )â( 15 ). Where IV m is the external energy provided to the circuit system, ( i Na E Na â+â i K E K â+â i l E l ) is the energy supplied by the voltage source represented by the Nernst potential, V m ( i Na â+â i K â+â i l ) is the energy in the potential difference between the inner and outer membrane. Distributed in these neurons action potential in the process, if they do not consider the change of cell membrane permeability of consumed energy, the energy involved respectively carries blood oxygen and glucose for energy provided by the neuron, inside and outside the cell membrane potential and ion pump in against the concentration of energy in the transport ion TiDuCha when consumed by biological energy (ATP), Increased glucose consumption due to brain stimulation is mainly caused by activation of the sodiumâpotassium ATP pump (Churchland et al. 2002 ; Rabinovich and Huerta 2006 ; Jiang et al. 2020 ). The first two describe the relationship between subthreshold neurons and biological energy, and the assisted diffusion of ions through ion channels along the difference in ion concentration does not consume energy. However, from a dynamic perspective, the sum of these three types of energy is equal to the total energy in the circuit system of HâH model during the transformation of subthreshold neurons into functional neurons. The first two types of energy correspond to the IV m and V m ( i Na â+â i K â+â i l ) in the circuit respectively, so the energy provided by the Nernst potential ( i Na E Na â+â i K E K â+â i l E l ) is equal to the biological energy consumed by the ion pump. In fact, in this process, the sodiumâpotassium pump is constantly transporting ions up and down the concentration gradient, which directly depletes biological energy so that one ATP can pump out three sodium ions and two potassium ions. This also confirms the existence of an ion pump, which provides a steady energy potential through the continuous transport of ions, thus providing energy for neural activity. Thus, we can calculate the power consumed by the ion pump by using the power of the voltage source represented by Nernst potential in the circuit shown in Fig. 7 , that is, the nerve energy consumed by neuron activity is shown in Eq. ( 16 ).
The third in the minus sign refers to the circuit shown in Fig. 7 , the direction of the voltage source and current E Na in contrast to the i Na , E K , E l and i K , i l potassium (sodium current towards inside cells, and electric current and leakage flow). For an action potential, we can use the above equation to calculate the amount of nerve energy expended. The calculated parameter values are determined by Zhu et al. ( 2018b ). In fully connected neural networks, the dynamic properties of each neuron are derived from the above HâH model, so the network structure is strictly defined on the basis of neurobiology. The anatomical structure of neuronal connections in the cerebral cortex indicates that the neural network inside any brain region is a fully connected structural neural network if functional connections are not considered, such as cortical functional columns (Gazzaniga et al. 2002 ). If the cortical functional column is regarded as a closed system, and a local region within the closed system is intercepted for simplicity, the network structure of this region can be expressed by a structural neural network consisting of 20 neurons as shown in Fig. 7 . To understand the energy coding mode of cortical neural network under different parameters, the connection of neural network is simplified to some extent. The connections between neurons in the figure indicate that they are coupled to each other, but the coupling strength between any two neurons is not the same, and the coupling strength between two neurons is not symmetrical. According to the principle of synaptic plasticity, statistical data from experiments show that the range of synaptic coupling strength between neurons follows a uniform distribution (Rubinov et al. 2011 ). That is, the following matrix is satisfied:
\(w_{i,j}\) represents the coupling intensity from the first neuron to the first neuron, and represents the number of neurons.
Put \(I(t)\) into Eq. ( 1 ) to obtain the membrane potential \(V_{im} (t)\) , and calculate the power consumed \(P_{i} (t)\) by the neuron through Eq. ( 3 ). Where \(I(t)\) represents the sum of current stimuli received by neurons at any time, \(I_{in} (t)\) represents the interaction between neurons, and \(I_{ext} (t)\) represents the influence of external stimuli on neurons.
Moment of Qi on behalf of each neuron's action potential distribution state, for the sake of simplicity it is simplified to the pulse of 0 or 1, when in the resting potential is 0, action potential constantly to 1, which said a neurons after issuing an action potential to another neuron stimulation time interval, which is exciting transfer delay, the range of possible values is uniformly distributed.
We use the traditional maximum correlation coefficient of synchronization index and the novel negative energy ratio to measure the synchronization activity of the network (Zhu et al. 2018b ).
The average maximum correlation coefficient is defined as follows:
where \(C_{i,j}\) is the Pearson correlation coefficient between the membrane potential of the first \(j\) neuron and the first neuron. If the Pearson correlation coefficient between any two neurons is closer to 1, it indicates that the synchronization between these two neurons is greater. Previous studies have found that two or more oscillating groups will appear in steady state if the network achieves synchronization under transient stimuli. It can be seen that when the index of maximum correlation coefficient is adopted, the closer its value is to 1, the stronger the synchronization of neurons within the oscillating group is, that is to say, the closer the network state is to the common synchronization phenomenon of multiple groups. When the value is closer to 0, it indicates that the synchronization of neurons within the oscillating group is weaker, that is, only a few neurons are synchronized.
Negative energy ratio is defined as follows: the ratio of the absolute value of negative energy consumed by the whole neural network from moment 0 to moment 0 to the sum of the absolute value of positive and negative energy.
where \(P_{i} (t)\) represents the power consumed by the neuron at the moment, and the integration \(P_{i} (t)\) in [0, \(t\) ] represents the energy consumed by the neuron during [0, \(t\) ]. \({\text{sgn}} (x) = \left\{ {\begin{array}{*{20}c} {1,} & {x > 0} \\ {0,} & {x \le 0} \\ \end{array} } \right.\) is symbolic function, \(E_{negative}\) and \(E_{positive}\) respectively represents the negative energy and positive energy consumed by the whole neural network in [0, \(t\) ].
The synchronicity of network activity is measured by the two indexes of mean maximum correlation coefficient and negative energy ratio. The larger the two indexes are, the stronger the synchronicity of network activity. Figure 8 compares the equivalence of HâH model and WâZ model through the changing relationship between the number of neurons and nerve energy:
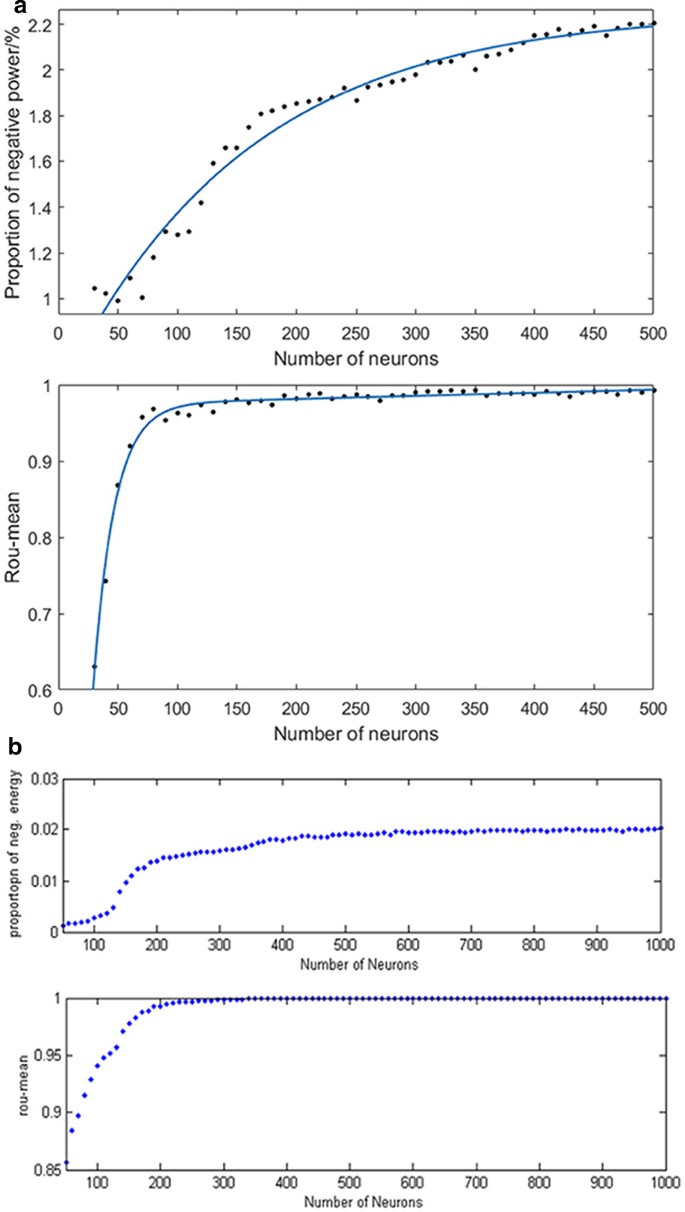
Model based on WâZ ( b ) and HâH model ( a ) (Zhu et al. 2018b ) of the negative energy ratio and all curves of maximum correlation coefficient on the number of neurons
The figure above shows that the greater the number of neurons in the network, the greater the demand for energy for synchronous oscillation of the network, that is, more energy reserves are required, and the negative energy ratio just reflects the energy stored in network activities. Like the traditional correlation coefficient method, the negative energy ratio can also reveal the synchronization state of the network, and both models show a positive correlation between the number of neurons and the network synchronization and the negative energy ratio. On the other hand, the negative energy ratio does not saturate quickly with the increase of the number of neurons, so that the number of neurons in the network can be more effectively distinguished, which is also one of the advantages of energy coding. It should be especially emphasized that, through the comparison of the left and right figures, it can be clearly seen that for an identical fully connected neuron network, the maximum correlation coefficient and negative energy ratio of HâH model and WâZ model are almost the same with the increasing number of neurons.
Figure 9 compares the equivalence of HâH model and WâZ model through the changing relationship between neuron coupling intensity and nerve energy.
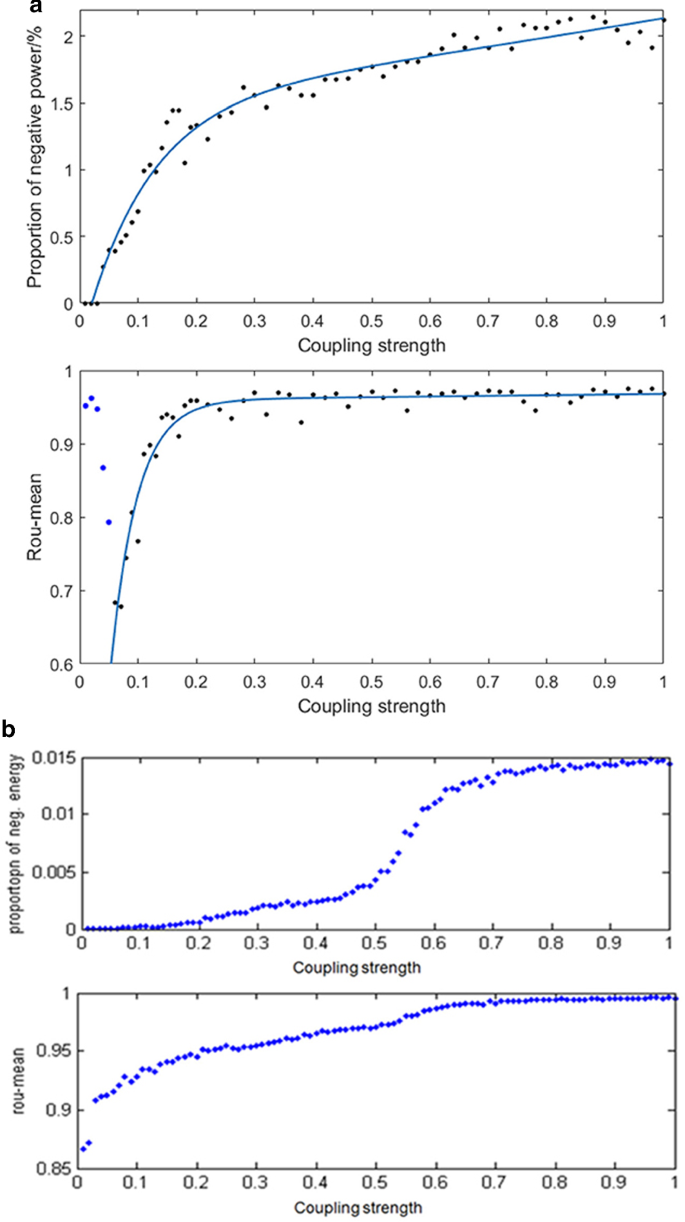
Model based on WâZ ( b ) and HâH model ( a ) (Zhu et al. 2018b ) of the negative energy ratio and both maximum correlation coefficient curves of coupling strength
Due to the coupling strength between the neurons affecting their information interaction, and the process relies on energy is required to complete, the coupling strength is larger, the synchronicity activities of the network is more intense, the stronger the information interaction between neurons, the higher the demand for energy, so you need a high energy reserve, namely reflection is higher than the negative energy. Both models show that the coupling strength is positively correlated with network synchronization and negative energy ratio. By comparing Fig. 9 left, right, and WâZ and HâH model for a same full connection neural network with increasing coupling strength between neurons, their negative energy, though there are some error, but also are increasing and the trend of growth is nearly the same, and both maximum correlation coefficient is exactly the same.
Figure 10 compares the equivalence of HâH model and WâZ model through the change relation between neuron excitation transmission delay and nerve energy.
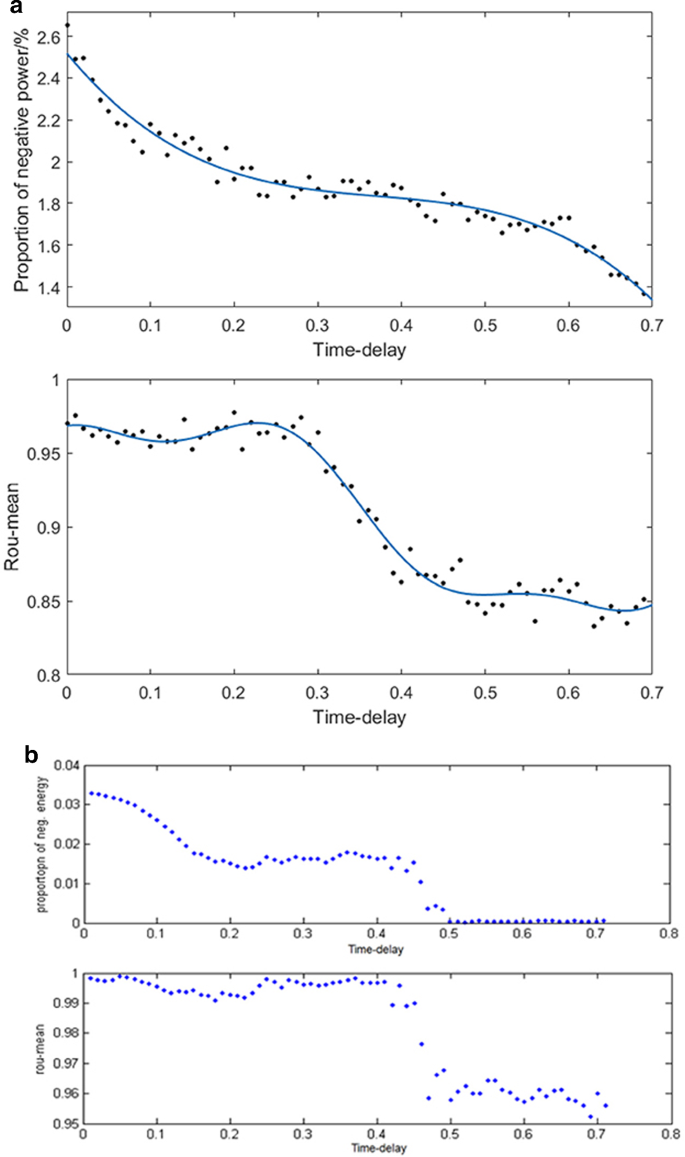
Model based on WâZ ( b ) and HâH model ( a ) (Zhu et al. 2018b ) of the negative energy ratio and both maximum correlation coefficient about excited transfer delay change curve
The longer the delay time for presynaptic neurons to release excitatory neurotransmitters to postsynaptic neurons, the weaker the correlation between the activities of presynaptic and postsynaptic neurons, the weaker the synchronous activity of the whole network, and the lower the demand for energy, thus the less energy stored in network activities, and the lower the corresponding negative energy ratio. The results based on the two different models show similar decreasing curves with the increase of excitatory transfer delay. Through the comparison of the left and right figures, it can be clearly seen that for an identical fully connected neural network, the maximum correlation coefficient and negative energy ratio of HâH model and WâZ model are almost the same with the increase of signal delay time.
In addition, in the two published papers (Cohen 2017 ; Zhu et al. 2018b ), we also carried out a large number of studies on the network neural coding in the above three cases respectively by HâH model and WâZ model, and found that the coding modes are almost the same under different parameters. Combined with the above two different synchronization indexes, the dynamic characteristics of synchronization are identical under the three conditions of increasing number of neurons, coupling strength and signal delay time. This proves that HâH model and WâZ model are equivalent at the level of structural neural network.
The results show that HâH model is suitable for the modeling, analysis and calculation of simple and local neural networks with a small number of neurons, while WâZ model is suitable for the modeling, analysis and calculation of complex networks with a large number of neurons. In particular, due to the correspondence between neural information and neural energy, and the advantages of simple calculation and no loss of main information, the neural energy theory and ZâW neuron model are very potential research methods that can be used to construct large-scale neuroscience models (Wang and Zhu 2016 ).
4 Application of WâZ model and neural energy method in functional neural networks
In fact, using neural energy method to study functional neural networks is not only very effective, but also can give scientific explanations for some experimental phenomena that neuroscience has not been able to make clear up to now from a quantitative perspective.
4.1 Neural mechanisms of cerebral hemodynamic phenomena
Neuroscientists have long been puzzled by the phenomenon of brain hemodynamics. The so-called hemodynamic phenomenon is that blood flow to the nervous system always increases significantly about 7â8Â s after the cerebral cortex is stimulated (Fox and Raichle 2007a ; Peppiatt and Attwell 2004 ). Provided according to the literature, the current neuroscience community does not give an effective theory to the phenomenon of neural mechanisms of scientific and reasonable explanation (Moore and Cao 2008 ; Maandag 2007 ), also didn't see related from neural modeling to calculate these two aspects of computer simulation and the experimental phenomenon that the hemodynamic phenomenon research reports. In order to simulate the phenomenon of blood flow delay in fMRI, we constructed a multi-level neural network based on WâZ neuron model and used the method of energy coding to give the neural energy changes that produce hemodynamic phenomena. The hemodynamic phenomenon of a large increase in cerebral blood flow in fMRI with a lag neuron activation area of 7â8Â s is reconstructed quantitatively. Since this study is based on the negative energy mechanism of neuronal activity that we have revealed (Wang et al. 2015a ), we predict that the nature of brain hemodynamic phenomena is the existence of negative energy mechanism in neural activity (Peng and Wang 2021 ). Recently, based on the anatomical structure of the visual nervous system, we constructed a large-scale neural network model consisting of various visual areas for visual information processing using neuron energy model, using which we successfully simulated the hemodynamic phenomenon of the visual system in fMRI (Peng and Wang 2021 ).
The significance of the above study lies in that it can provide a new vision for exploring the dynamic mechanism of hemodynamic phenomena in the future, and thus provide important scientific support for establishing the framework of brain theoretical research in the future.
4.2 Application of neural energy coding in brain navigation
Spatial cognition and representation are critical to animalâs survival, such as navigating to find hidden food or to avoid danger. It is believed that animal can form a cognitive map in the brain to solve spatial tasks. The concept of cognitive map was hypothesized by Tolman (Tolman 1948 ). Until 1971, O'Keefe and Dostrovsky discovered the first neural basis of cognitive map (OâKeefe and Dostrovsky 1971 ). They reported a type of neuron in the rodent hippocampus that emitted spikes whenever it ran through a specific set of spatial locations. This type of neurons is now termed as âplace cell,â and the particular subset of locations in the arena to which the place cell respond is called a âplace fieldâ. Different place cells are corresponding to different place fields, which varies in size, shape, and center. Thus, the environment is represented by the population of place cell entities in the hippocampus to support the concept of cognitive map (Wilson and McNaughton 1993 ). Animals typically recruit different place cell populations when navigating in different environments, suggesting a remapping of spatial representations in the hippocampus across environments (Alme et al. 2014 ). Other than spatial properties, the place cell also showed a striking temporal feature which is called phase precession, meaning the spike timing is progressively advancing relative to the local theta oscillation phase in the hippocampus as the rat pass a typical place field (OâKeefe and Recce 1993 ). This phenomenon extends the place cell code from a pure spatial domain to a spatialâtemporal domain. Thus, the hippocampus plays an important role not only in spatial representation, but also in spatial memory. Place cells are thought to be important for spatial tasks such as path finding, and can also act as route planners.
However, the place cell is only the first piece of the spatial computation system in the brain. It is a component of a more general circuit to represent the spatial information in a dynamic way (Moser et al. 2008 ). The place cell has particular spatial selectivity. So it is natural to ask where the spatial information received by place cell come from. The medial entorhinal cortex (MEC) is an important upstream of hippocampus and has attracted much attention during recent years. A similar type of neuron has been found in MEC called grid cell, which also responds to the location of the animal like the place cell, but multiple firing fields appear in a periodic fashion, forming a triangular grid pattern covering the entire arena (Sargolini et al. 2006 ; Hafting et al. 2005 ). It is hypothesized that projections from the grid cells to the hippocampal place cells support the generation of the place fields. From dorsal to ventral MEC, the space between the vertices of grid pattern increases in a modular manner (Sargolini et al. 2006 ; Hafting et al. 2005 ; Fyhn et al. 2004 ), while the positions of the grid vertices vary randomly, but each grid maintains a stable spatial phase (locations on the horizontal plane). These spatial features of the grid cell population are believed to form a global spatial coordinate system in the brain, which may be informatively redundant and robust, suggesting that grid cells are playing a role in path integration (Hafting et al. 2005 ; Barry et al. 2007 ), meaning tracking the locomotion of the animal. Furthermore, there is evidence that the firing fields of grid cells persist in the absence of sensory input, suggesting that the perception of self-movement is the main driver of grid cell activity, further implying that animals can continuously track and update their self-location in the environment through the grid coordinate system (Hafting et al. 2005 ; McNaughton et al. 2006 ). Grid cells together with place cells constitute a quantitative spatialâtemporal representation system for representing locations, paths, distance and associated behavioral and episodic memories.
Most of the understanding of the brainâs navigation system came from experiments or theoretical models in two-dimensional (2D) space. The studies often conduct on flat, horizontal planes, while the actual world is three dimensional (3D), and all animals, more or less, need to navigate in 3D space. However, little is known about how 3D space is encoded in the brain. Do the place field and grid fields have 3D properties? The regularly distributed firing fields of grid cells on the 2D plane constitute a metric system for navigation, and it is a difficult question how this hexagonal pattern can be generalized to volumetric space. Similarly, conclusion has not been made about the 3D counterpart of the circular or elliptical place field on the 2D plane. Evidence has suggested the grid cell firing patterns on the one-dimensional linear track can be treated as cutting the 2D grid lattices (Yoon et al. 2016 ), implying the grid cell representations may be global and the dimensionality could be higher than that of the experimental setup (Finkelstein et al. 2016 ). However, recordings from rodents do not support this hypothesis (Hayman et al. 2011 , 2015 ). In these experiments, grid cells were recorded when rats navigated in 3D space or at least a section of volumetric space, such as on a helix stairs (Hayman et al. 2011 ), titling or even vertical walls (Hayman et al. 2015 ; Casali et al. 2019 ). The results show that the grid field maintains its horizontal character but is vertically elongated on multi-layered helix and is almost indistinguishable from the grid field on the tilting plane from that on the horizontal plane. Place cell recordings from free-flying bats (Yartsev and Ulanovsky 2013 ) and grid cell recordings from crawling bats (Yartsev et al. 2011 ) have also been published, indicating the existence of volumetric place fields in 3D space and hexagonal lattice patterns in the horizontal plane in such mammals. One theoretical analysis suggests that a face-centered cubic lattice is optimal for maximizing 3D spatial resolution (Mathis et al. 2015 ). Another fact which should be taken into account is that rodents and bats have different natural movement behaviors, so their spatial encoding strategies are not necessarily identical. And conclusions about spatial representations of different species should be drawn with caution.
The complexity of the spatial computation problem forces us to consider an alternative besides electrophysiological experimentsâneurodynamical modeling, since neural systems reveal abundant dynamical properties, such as oscillation and attractor dynamics. Regarding this, two main categories of models have been developed to reveal the mechanisms that form the grid cell activity, such as periodic firing fields, phase precession, and invariance to velocity changes. One is the oscillatory interference (OI) model and the other is the attractor network model (Giocomo et al. 2011 ). The first type consists of several oscillator pairs. Inside each pair, there is a baseline oscillator and a velocity-modulated oscillator. The frequency difference between two oscillators is influenced by the speed and direction (velocity) of the animal's movement. And different pairs corresponding to different allocentric directions. Thus, each pair of oscillator continuously tracks the distance the animal travels in a fixed direction by the phase difference of the two oscillators. And multiple oscillator pairs are integrated to form a grid pattern (Burgess et al. 2007 ). The attractor network model arranges the neurons on a sheet and each neuron excites its neighbors and inhibits proximal neurons. The network can generate attractor states to represent position. Combining specific input cues and structured recurrent connections, the activity bumps of the grid cell layer move in response to the animal's motion, which guarantees the periodic pattern can be formed for each neuron (Burak and Fiete 2009 ).
OI model is often used to simulate the grid cell activity in 2D space. Classic OI model is consisted of several pairs of oscillators. Each pair has a somatic oscillator and a dendritic oscillator. Frequency of somatic oscillator is determined by the background theta rhythm and frequency of dendrite oscillator is modulated by velocity input on the basis of theta, which has an increment proportionate to the projection of velocity to the preferred direction, which is assigned to each oscillator pair. When the preferred directions corresponding to the oscillator pairs are separated by 60°, the hexagonal pattern of grid cell firing can be generated. Based on this idea, a gravity-modulated OI model has been proposed to generate grid cell activity in 3D space (Wang et al. 2021c ). The fixed preferred directions in 2D space of the classic model are changeable in this new model, which can be rotated onto the local plane. The rotation operation is presumably achieved by receiving the head direction signal with reference to gravity. The rotation axis is the intersection line of horizontal plane and body plane. By this method, the OI model can be modified to simulate the grid cell activity in 3D space for crawling animals such as rodents. Simple as it is, the results can account for the known experimental phenomena found in rats, and the model also simulate and make testable predictions of grid cell activity on novel surfaces in 3D space.
Figure 11 shows the grid pattern on the multi-layer helix stairs, which was used to recording spatial neurons in experiment (Hayman et al. 2011 ). In this figure, three âstripe patternsâ (a, Stripe pattern 1â3) is the direct results of three preferred directions and the grid pattern can be formed by the threshold product of the stripe activities (a, bottom right). Projection of the grid patterns on the ground (top view) suggests the grid cell seems not sensitive to vertical locations, and the pattern is similar on each layer of the helix track (b). The histogram of grid firing locations on every layer (from bottom to top: lowest coil to highest coil of the helix) in c further confirm this observation. The firing locations are represented by angles on each layer. Note that this phenomenon is a key finding in experiment (Hayman et al. 2011 ). The model can also generate grid patterns with different orientations and spatial periodicity (d).
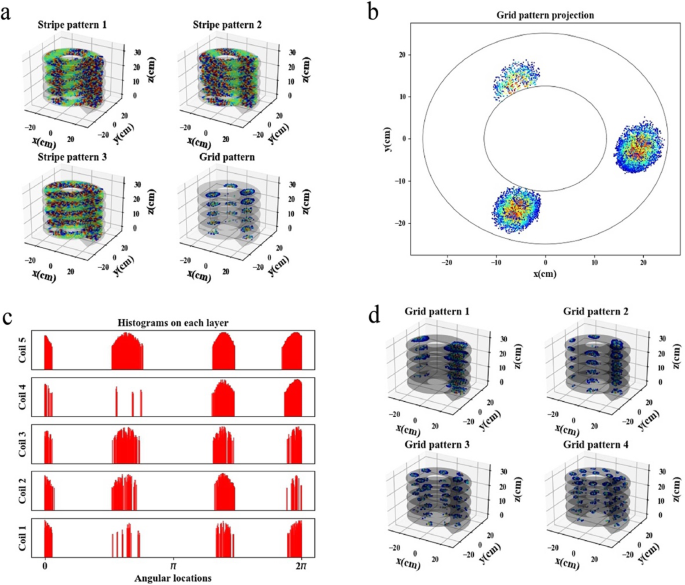
Grid firing analysis on helix track
Grid patterns on other complex surfaces in 3D space were also simulated, and it turns out that the grid cell activity can be trajectory-dependent, i.e., different trajectories can result in different grid patterns (Wang et al. 2021d ). As shown in Fig. 12 , the navigating terrains are a multimodal surface, a saddle surface and a unimodal surface. The two upper rows of Fig. 12 illustrate the stripe and grid (low right) patterns of random moving while the two lower rows of Fig. 12 are the same patterns but generated by regular trajectories, which are zigzag paths for multimodal and saddle surface and a spiraled path from top to bottom for unimodal surface. The stripes are very fuzzy and the grids are almost vanishing for random moving while the patterns generated by regular moving are quite inerratic. Mathematical analysis indicates that the condition for trajectory-independent grid pattern is quite rigorous, which demands the rotation of preferred direction vectors defined on every location on the surface forming a conservative field, and merely the horizontal and tilting plane can satisfy the condition.
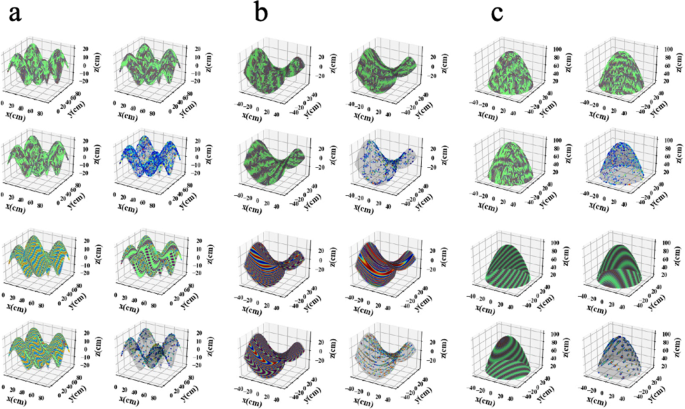
The trajectory-dependency of grid fields on smooth surfaces in 3D space
In 2D space, it is generally believed that grid cells are involved in the formation of the place field because hippocampus receive primary input from MEC. The place cells are likely integrating multiple inputs from grid cells. Studies have shown that hippocampal pyramidal neurons perform linear summation of synaptic inputs (Cash and Yuste 1999 ). An elegant mathematical model inspired by Fourier Transformation has been proposed to generate a Gaussian-type place field by linearly summing dendritic inputs from several grid cells with designed synaptic weights (Solstad et al. 2006 ). However, this model applied a sinusoidal function defined directly on the 2D plane to represent grid fields which did not consider the actual trajectory of the animal, and it did not suitable for 3D navigation either. Followed the gravity-modulated rotation scheme of the preferred directions in grid cell model, the place cell activity can be similarly modeled. Grid patterns with different orientations and wavelengths are first generated on the surfaces in 3D space, then these patterns are summed by the similar designed synaptic weights (Xu et al. 2022b ).
Examples of simulation results are showed in Fig. 13 (a: helix, b: sphere, c: multimodal surface, d: saddle surface). Trajectories were also generated by random movement. Each sub-figure contains four panels. The first column shows the place field on the manifold. The firing rates are color-coded and warmer colors indicate higher firing rates. In the upper left panel, the rate is calculated for each position along the animal's trajectory, while the lower left panel shows only the rates above the threshold (half of the maximum value) for better illustration. The upper right panel in each subplot shows the weight values of each the grid cell input, which are color-coded. The lower right panel also shows the projection of place field on the horizontal plane. The feature that place cell fires at almost the same location in each layer as the rat navigates the helix is consistent with experimental recordings (Hayman et al. 2011 ). Future experiments could be set up similar apparatus to verify the place field patterns predicted by the model.
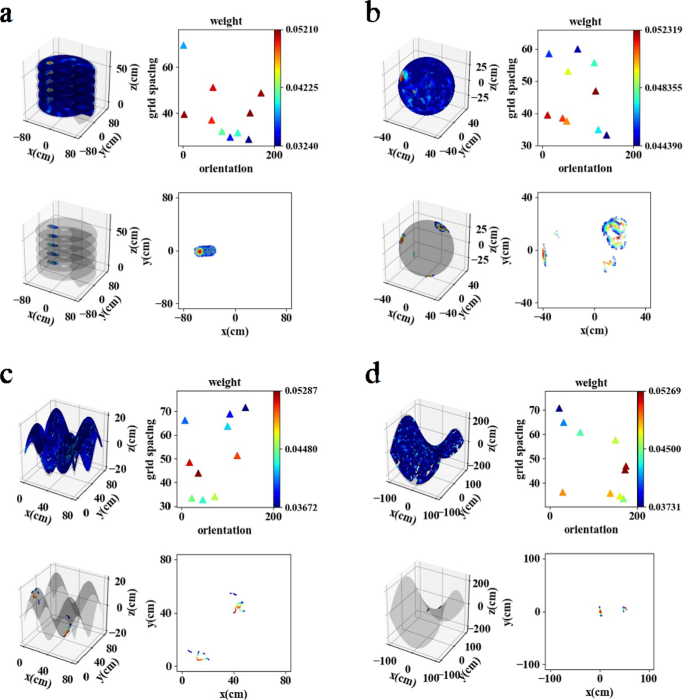
Place fields on complex 2D manifolds in 3D space
The aforementioned neurodynamical models focused on rodents, which are not a volumetric moving animal. The 3D movement of rodents is depending on the environmental apparatus. Bat is a volumetric navigating mammal which has hippocampal formation. A network model of 3D place cell based on neural energy was also proposed (Wang et al. 2018b ). This is a concrete examples of the application of neural energy theory in brain science. Neural energy was used to define place field and place field center, and the locating performance and energy consumption characteristics of the place cell system were analyzed. Figure 14 a displays the activity patterns of 16 randomly selected cells. The scatter plots in 3D space represent the different locations of the flying bat in the random search trajectory, and the firing power (in nW) of place cells at the corresponding position was coded by color. Centralized 3D place field can be generated by this energy-based model. The distribution and size of place fields as well as firing powers vary among different place cells. Maximum power is about 3000 nW among these 16 cells and larger place fields usually have higher maximal power. Then this model was used to perform locating function in 3D space. Figure 14 b shows the average locating error with respect to the size of place field. The result suggests that the locating error was not simply monotonously increasing as the place field enlarging. There always exists a minimum localization error when the place field is of the medium size. So the place field with a reasonable optimal size will most accurately preform the localization function. Notably, larger place field usually corresponding to more energy consumption, so a moderate field size (moderate energy consumption) is the optimal solution for locating by place cell network. It implies the trade-off between energy consumption and spatial coverage of the place cell. The study using energy coding method validates the principle of energy economy of the brain in encoding 3D spatial information.
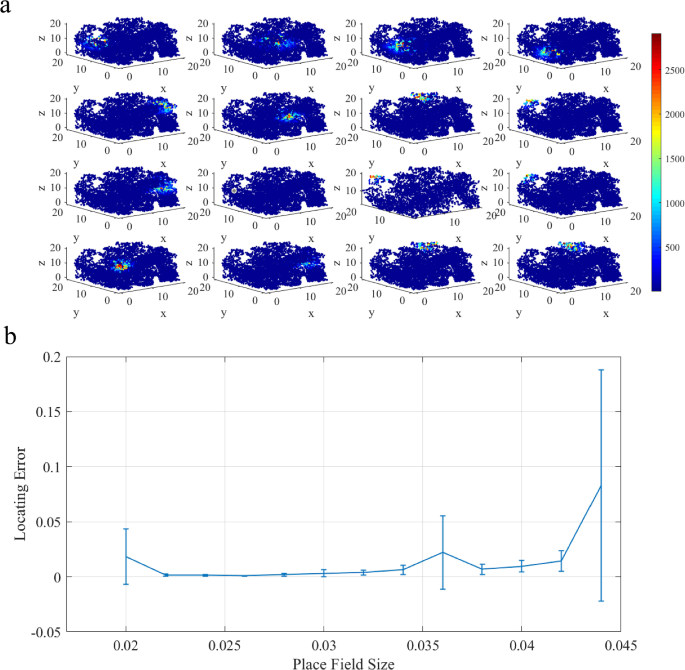
Place cell network model encoding 3D spatial information constructed by neural energy method (Wang et al. 2018b )
It has been shown that the spatial computation could vary among different species, especially in different dimensions. Is there a universal principle behind these differences? The solution of this question is another successful application of the novel neural energy method. A new perspective of understanding the place cell activity in different dimensional spaces can be provided by neural energy and information theory. Place cell fire spikes to transmit information about locations. However, neural activity such as spike is energy expensive. The neural system ought to make full use of every spike to represent the largest amount of spatial information. Inspired by this designing principle, a theoretical work tries to answer the spike allocation problem for place cell, i.e., at what location to fire a spike (and forming the place field) with finite amount of total energy can achieve the most efficient representation for spatial information (Wang et al. 2019b )? This question is actually a functional optimization problem with constrains which can be solved by a mathematical technique named calculus of variations. The place field of different species can be treated as a function defined in different dimensional spaces and the amount of information is a functional taken place field as the input function. The finite amount of neural energy is the major constraint for the place cell. The functional optimization problem with constraint can be constructed based on neural energy by these steps. The variational method gave the optimal shape of place field. When the moving trajectory is uniformly distributed in 1D, 2D or 3D space, the spike location arrangement is a Gaussian-shape place field in every dimensional space. These results are shown in Fig. 15 a (1D space), b (2D space) and c (3D space). Figure 15 d exemplifies the maximum information per spike (vertical axis) in 2D space with respect to the spatial variance and area of the 2D space. When the trajectory distribution is different, the resulting place field is also affected, implying the animalâs natural habitat and moving statistics play important roles in determining the distribution of place field. It indicates that bat flying in 3D space and rat climbing in 3D space may result in differently shaped 3D place fields, which can reconcile the inconsistency of the place field symmetry found in animal experiments. It is a potential evidence that the brain complies certain designing principles such as energy economy and information efficiency, which is a representative application of neural energy theory.
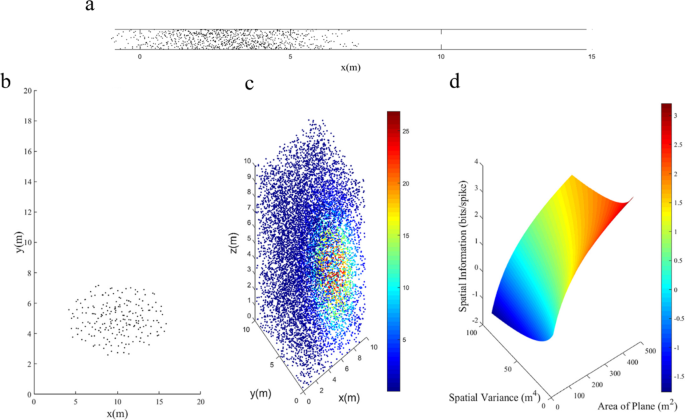
Optimal place field in different dimensional space constrained by neural energy (Wang et al. 2019b )
The neural energy has very rich behaviors no matter in single neuron level or network level. After separating and defining neural energy supply and consumption, calculating suggested that the energy properties of supra-threshold and sub-threshold, such as power synchronization of ion channels and energy utilization ratio, have significant differences. Especially the energy utilization ratio, which can rise to above 100% during sub-threshold activity, revealing an overdraft property of energy use (Wang et al. 2017b ). The neural energy method has also been used to study the transformation of different types of memory based on a neurodynamical model (Wang et al. 2019c ). A method has been developed to measure the changes in energy input of different stimuli and the corresponding energy consumption of the memory system. The results provide a comprehensive understanding in the memory transformation by an energy coding approach and also reveal the energy-efficient principle of the neural system.
The neural energy is also a promising prospective to study cognitive function such as path-finding. Based on activity pattern of hippocampal place cells, a novel model of neural energy field gradient has been proposed. A mapping among discrete spatial locations, place cell population and neural energy was constructed to define the neural energy field (Wang et al. 2017a ). The distribution pattern of firing power among the neuron cluster was utilized to encode the metric and topological information of space. Then it suggested that the energy field gradient can sever as a navigational vector. By the coupling effect of gradient and noise vectors, the model can perform an efficient and biological plausible mental exploration. It is an important example that neural energy is an effective tool to study cognitive functions of the brain.
In conclusion, energy-based large-scale neuroscience models can profoundly reveal the relationship between energy, information and spatial position in the nervous system. Its advantages are as follows:
nervous energy can efficiently express the cognitive system in 3D space of neural coding (Wang et al. 2019b );
the energy constraint conditions to maximize information coding (Wang et al. 2018a , 2018b );
the neural coding can maximize energy to improve the efficiency of intellectual discovery (Wang et al. 2017a ).
4.3 Neural energy characteristics of memory switching
Neuroscience provides a qualitative explanation of how short-term memory is transitioned to long-term memory using data from neuroanatomical experiments. However, on the basis of experimental data, from the perspective of quantitative analysis, it seems that there is no relevant research report on how short-term memory is transferred to long-term memory under different stimulus conditions. We explore the interaction between working memory and long-term memory from the perspective of energy encoding based on a bi-stable working memory model. Long-term memory was induced using the working memory model using theta cluster stimulation (TBS) and high frequency stimulation (HFS), which induced LTP in experiments (Zhu et al. 2016a , b ). Based on electrical stimulation of the physical nature of nervous system, we developed a quantitative method to determine the stimulus to the energy input of the nervous system and the corresponding energy consumption system, and at the same time, further studied the two different long-term memory inducing stimulation protocols, their minimum energy consumption, and defined the energy ratios to quantitatively describe the stimulus energy efficiency. The results show that both of these commonly used LTP-inducing stimuli can successfully stimulate long-term memory based on the bi-stable dynamic model. However, by analyzing the minimum energy consumption and energy ratio, TBS is found to be a more energy efficient stimulus mode than HFS, which is also consistent with experimental results (Wang et al. 2019c ). The reason may be that TBS can push up the system response rhythmically, gradually raising it to a high steady state. In this study, by combining neural energy and dynamics, the energy characteristics of dynamic switching in memory model were found by investigating the response characteristics of the memory system to the stimulus modes commonly used in the two experiments (Wang et al. 2019c ). This provides a strong dynamic evidence for understanding how working memory is transformed into long-term memory, which reflects the high efficiency of the nervous system energy utilization during the formation of long-term memory. This example is also a successful application of neural energy coding theory.
4.4 Dynamics and energy characteristics in spontaneous brain networks
Most current researches on the brain have concentrated on task-related brain activities, both experimentally and theoretically. However, it is of much necessary to take spontaneous activities into account when we consider how the brain works, since these spontaneous brain activities always consume most of the brain's energy. The upâdown oscillations of membrane potentials, which usually characterized by bistable and bimodal distribution, accompanied by some spontaneous spikes in up states, is considered to be one of the significant spontaneous activities. How these spontaneous phenomena occur? How much is the energy consumption of this kind of activity? Whether it implies something or not? Our work on spontaneous upâdown network have tried to answer these questions and to provide a theoretical complement to the study of spontaneous brain networks.
In this work, a network model of spontaneous upâdown oscillation has been designed, and on this basis the causes and key elements which influence the spontaneous spikes have been revealed, as well as energy characteristics of spontaneous bistable networks (Wang et al. 2019c , 2021e ). The results of our theoretical study of upâdown oscillations, as shown in Fig. 16 , specifically focus on intrinsic ion channel kinetics and synaptic transmission process. In Fig. 16 a, b, it is obvious to find that the fast sodium current is critical to the generation of spontaneous neural spikes, while the persistent sodium current plays a role in whole spontaneous fluctuation, with or without external noise. Both of them influence spontaneous firing rates and synchronous upâdown activities, which illustrated in Fig. 16 c as the combined effect. In terms of synaptic transmission, the blocking of excitatory connection reduces neural spikes and meanwhile still reveals spontaneous firing, as the experimental results recorded (Sanchez-Vives and McCormick 2000 ; Compte et al. 2003 ) (also see Fig. 16 d), which indicate that some neurons produce spikes spontaneously through intrinsic membrane mechanisms. Furthermore, energy consumption of spontaneous upâdown network and its characteristics has been concerned and the result is demonstrated in Fig. 17 . The energy consumption of neurons in spontaneous upâdown network is calculated and bistable characteristic and bimodal distribution of energy consumption is shown in Fig. 17 a, which is just in accordance with the feature of membrane potentials. At the same time, temporal and spatial characteristics of energy consumption which mostly occurs during up states and concentrates within the neuron rather than in synaptic transmission process, are reflected in Fig. 17 c. Besides, we also compare the indicator of energy with other commonly used ones, like firing rate and synchronizing rate, and expound its effectiveness and robustness as a global indicator (see Fig. 17 b). In Fig. 17 d, the results show that the energy consumption of stimulus-related energy is much smaller than that of spontaneous activity, indicating that energy consumption was driven by internal spontaneous activity rather than external stimulus, which are consistent with the evidence from brain imaging results and point of view put forward by Raichle (Raichle and Mintun 2006 ; Fox and Raichle 2007b ).
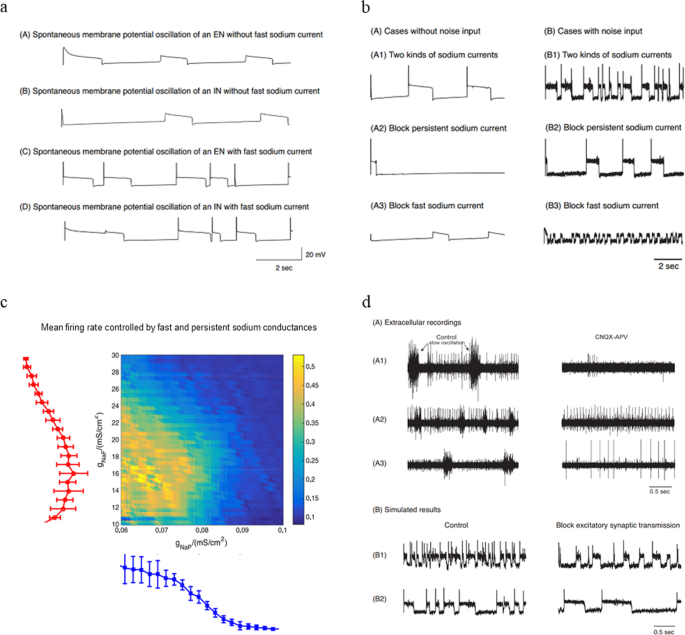
Influence of intrinsic ion channel and synaptic transmission on up and down oscillation. (Color figure online)
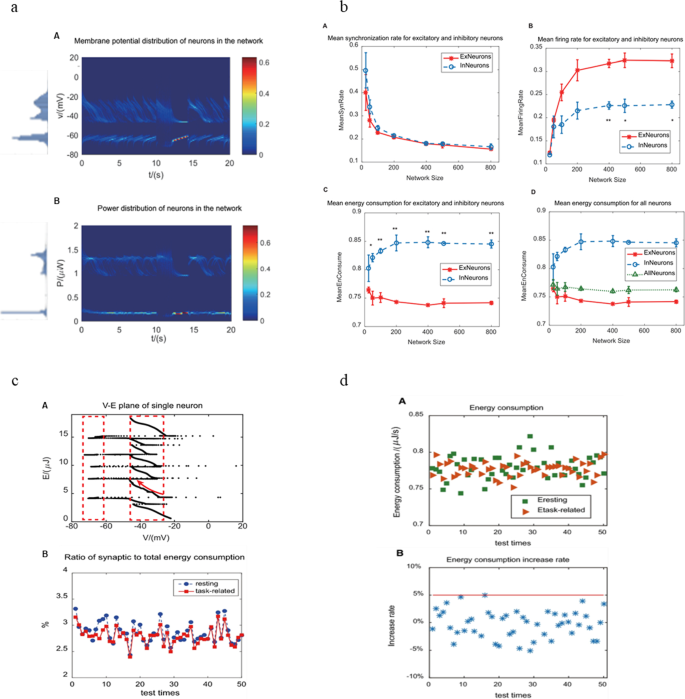
Energy characteristics in spontaneous up and down oscillation networks. (Color figure online)
Figure 16 a. Spontaneous membrane potential oscillation of two sample neuronsâan excitatory neuron (EN) and an inhibitory neuron (IN)âwith or without fast sodium current. Sample neurons with fast sodium current exhibit spontaneous spiking in up and down oscillation. Figure 16 b. Membrane potential oscillation of sample neurons under different cases with or without noise input. (A) Membrane potential oscillation of a sample neuron under cases without noise. (B) Membrane potential oscillation of a sample neuron under cases with noise input. Figure 16 c. Mean firing rate of neurons in the network controlled by persistent sodium conductance together with fast sodium conductance. (Blue) Mean firing rate of neurons in the network controlled by persistent sodium conductance. (Red) Mean firing rate of neurons in the network controlled by fast sodium conductance. Figure 16 d. Experimental and simulated results show block of excitatory synaptic transmission decreases neural firing and reveals spontaneous firing. (A) Extracellular recordings from layer V pyramidal cells: (A1)â(A3) Extracellular recordings from three example neurons (Sanchez-Vives and McCormick 2000 ; Compte et al. 2003 ). (B) Simulated results based on our network model before and after blocking excitatory synaptic transmission: (B1)â(B2) Simulated results from two example neurons.
Figure 17 a. Power and membrane potential are always stable at two states and both show bimodal distribution, whenever in spontaneous activities or during continuous external stimulus (10â12 s). (A) Membrane potential distribution of all the neurons in the network. (B) Power distribution of all the neurons in the network. Figure 17 b. The network size-dependent change of three indicators. (A) Mean synchronization rate for excitatory and inhibitory neurons. (B) Mean firing rate for excitatory and inhibitory neurons. (C) Mean energy consumption for excitatory and inhibitory neurons. (D) Mean energy consumption for all neurons (the green dotted line) in the network. Figure 17 c. Temporal and spatial characteristics of energy consumption. (A) Membrane potential versus energy consumption plane of a single neuron. (B) Mean ratio of synaptic to total energy consumption of all the neurons in the network. Figure 17 d. Spontaneous and stimulation related energy consumption of neurons in the network. (A) Mean energy consumption of neurons in the network during spontaneous and stimulated periods. (B) Stimulation related increases in energy consumption of neurons in the network.
Through the observation and analysis of the findings, we believe that these results shed light on the role of intrinsic sodium current and synaptic transmission in spontaneous firing and up and down transitions, lay the foundation for further work on spontaneous cortex activity, and would promote the progress of the energy theory in the study of spontaneous brain activity.
4.5 Biophysical mechanism of interaction between default mode network and working memory network
The question of particular interest to neuroscientists is what causes the enormous persistent expenditure of brain energy. Is it possible that the current mainstream view of cognitive neuroscience has misled researchers into ignoring or ignoring the possibility that experiments in neuroscience and cognitive psychology reveal only part of brain activity (Fox and Raichle 2007a ). The answer may lie in the brain's DMN and its resting state network. To this end, we first explore the neural mechanism of antagonism between DMN and task positive network (TPN). The results show that the synaptic connection strength has opposite effects on the positive task network (TPN) and the negative task network (TNN), thus concluding that the neural mechanism of antagonism between the DMN and the positive task network is mutual inhibition at the synaptic level (Cheng et al. 2020 ).
As shown in Figs. 18 , 19 and 20 , three different parameters (NMDA conductance parameter K5, Gaussian parameters Ï and J+âof internal preference weight of inner pyramidal cell population) strongly controlled TPN and TNN release, especially synaptic connection strength J+âhad opposite role to TPN and TNN, Thus, the antagonistic mechanism between them is caused by mutual inhibition at the synaptic level.
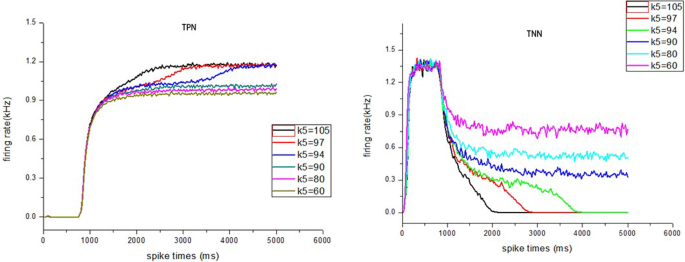
Firing rate curves of TPN (left) and TNN (right) with different synaptic conductance (Cheng et al. 2020 )
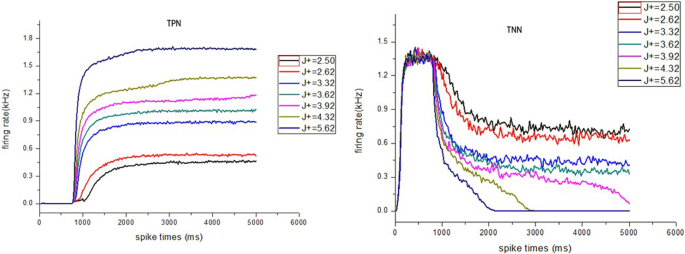
Firing rate curves of TPN (left) and TNN (right) with different \(180^{^\circ }\) (Yuan et al. 2021 )
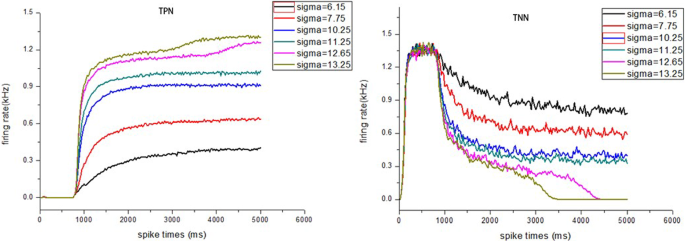
Firing rate curves of task-positive (left) and task-negative network (right) with different Ï (Yuan et al. 2021 )
We further investigate the relationship between DMN activity as the number of working memories increases. It was found that with the increase of the number of stimuli in working memory (Fig. 21 ), the neural activity of the default network decreased more rapidly (Fig. 22 ), indicating that the task was more difficult. It indicates that the default network is indispensable in the process of working memory.
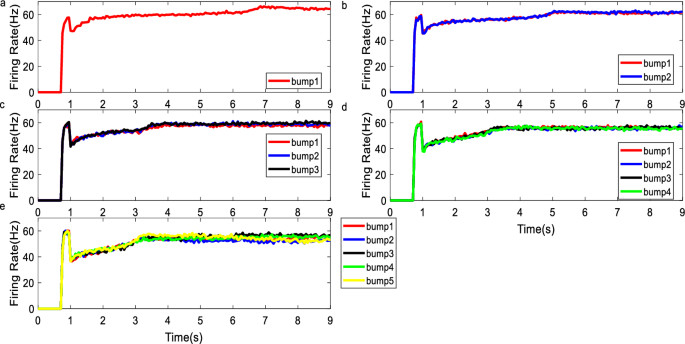
Average firing rate of excitatory neurons in the working memory network in model 1 (Yuan et al. 2021 )
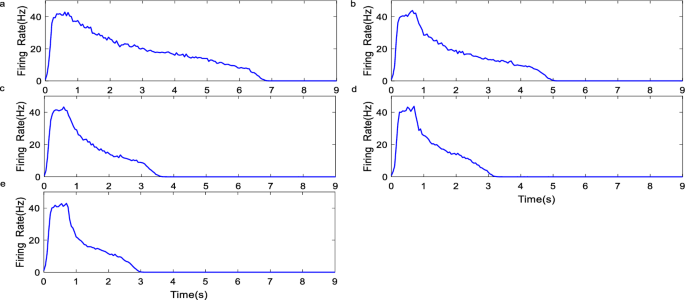
Average firing rate of excitatory neurons in the default-mode network in model 2 under various stimuli during the whole process (Cheng et al. 2020 )
The calculated results are in good agreement with the experimental data provided in Hu et al. ( 2013 ).
The energy expression of DMN and working memory network under coupling condition is consistent with the conclusion of synaptic mutual inhibition, as shown in Fig. 23 .
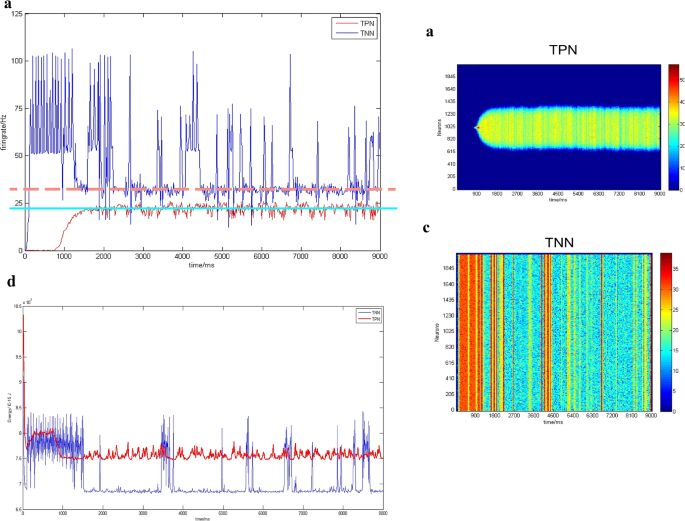
Firing results of TPNâTNN network after the introduction of AMPA with the same order of magnitude as NMDA. a Firing rate curves of excitatory population in TPN and TNN. Gaussian weight parameters: preferred direction: 180°. Red dotted line is the baseline of TNN firing rate after stimulus withdrawal. The baseline value is 32.21 Hz. Bright blue solid line is the baseline of TPN firing rate after stimulus withdrawal. The baseline value is 21.32 Hz. b Scatter plot of TPN with density color temperature. c Scatter plot of TNN with density color temperature. d Contained energy in TPN and TNN. Red curve is the contained energy of TPN, and the blue one is the contained energy of TNN (Yuan et al. 2021 ). (Color figure online)
We also studied the control and energy expression of NMDA neurotransmitter on the interaction between TPN and TNN. As shown in Fig. 24 , the energy of TPN and TNN under the action of NMDA neurotransmitter switch in TPNâTNN model was found. Furthermore, it is found that the NMDA conductance between TPN and TNN can be used as the switch of different stages of working memory and has good robustness.
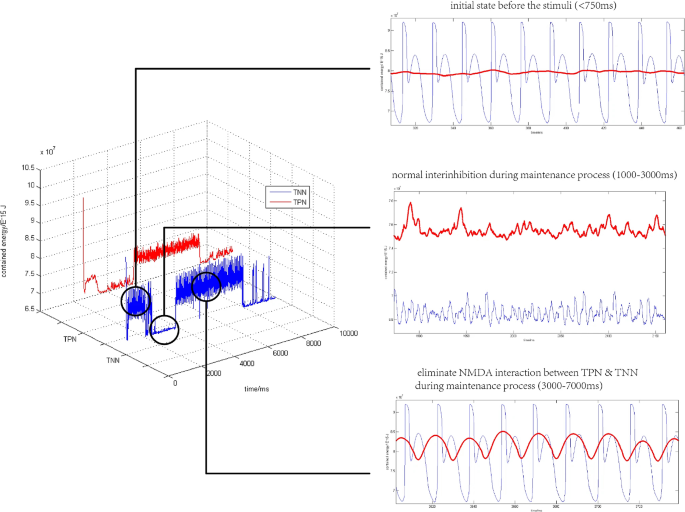
Contained energy of TPN and TNN with NMDA switch I. The whole network got stimulation in 750â1000 ms. NMDA channels between TPN and TNN was switched off in 3000â7000 ms (right below) and switched on in the rest of simulation time. Preference direction: 180°, Gaussian weight parameter: \(\sigma = 13.25,\;J^{ + } = 3.62,\;k5 = 95\) (Yuan et al. 2021 ). (Color figure online)
The particularly interesting is that we coupled TNN1, the DMN of the posterior cingulate gyrus (PCC) and TNN2, the DMN of the inferior parietal lobe (IPL), with the working memory network to study and reconstruct three stages of working memory: encoding, storage and retrieval. What needs to be emphasized is that in the information extraction stage, the information in the coding stage can be fully reflected. It was found that the antagonism between DMN and working memory network was not only negatively correlated in the traditional sense, but showed complex negative correlation and positive correlation successively in different brain regions.
Fig. 25 a is the scatter color temperature diagram of the specific emission rate of the three networks in the whole 9000 ms. Fig. 25 b is the energy curve of the three networks in the coding, maintenance and extraction phases respectively. Task negative network 2 is the last blue dotted line, task negative network 1 is the middle magenta dotted line, and task positive network is the first green solid line.
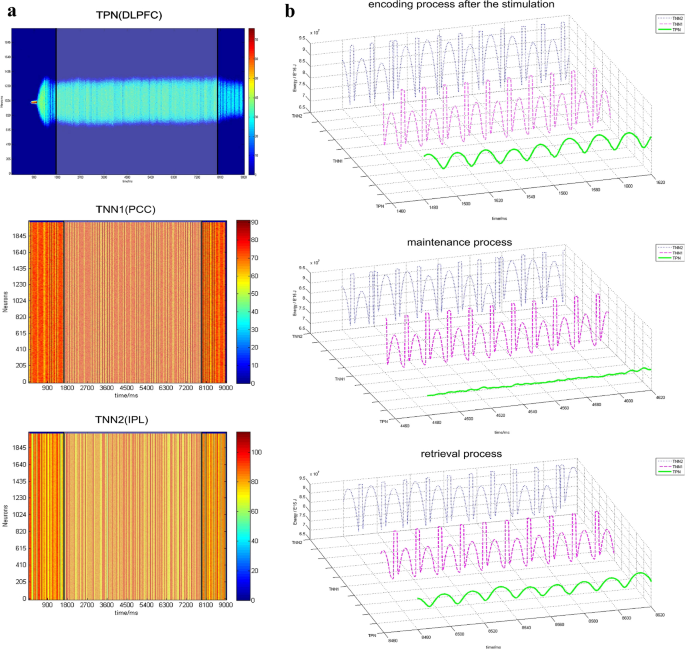
Simulation results of the whole process of working memory (Yuan et al. 2021 ). (Color figure online)
TPN is the positive network of task, TNN1 is the default network of posterior cingulate gyrus (PCC), TNN2 is the default network of IPL. Figure 25 a: Scatter color temperature diagram of TPN emission rate on the top, and emission rate of TNN1 and TNN2 in the presence of working memory in the middle and below (strong negative activation of the left and right ends and inhibition of the middle part). Figure 25 b (energy graph): Above is the code. In the middle is the maintenance phase. Note that the green line in the maintenance phase is the result of processing the coded stimulus with the past information. The picture below is the extraction (recall stage). It can be seen that the information of the coding stage is fully reflected in the recall stage.
4.6 Neural energy as a new view to explain the mechanisms of neuropsychiatric disorders
Some evidence suggests that neuropsychiatric disorders are related to energy metabolisms. At the molecular level, quantities of upstream genes associated with energy metabolisms are found to be significantly changed in animal models and human cerebrospinal fluid of major depressive disorder (Abdallah et al. 2014 ; Ă gren and Niklasson 1988 ; GĆombik et al. 2020 ; Gu et al. 2021 ; Zuccoli et al. 2017 ), schizophrenia (Zuccoli et al. 2017 ; Chase et al. 2015 ; Duarte and Xin 2019 ; Martins-de-Souza et al. 2011 ; Pruett and Meador-Woodruff 2020 ; Konradi et al. 2004 ) and bipolar disorder (Zuccoli et al. 2017 ; Konradi et al. 2004 ). At the cellular level, some studies show that abnormal glial cell activities are also the potential pathological reasons of neuropsychiatric disorders (Cui et al. 2018 ; Dietz et al. 2020 ), while glial cells are responsible for the energy supply of neurons. In addition, a large number of fMRI results have also shown that different blood flow velocities and BOLD effect exist in brains with neuropsychiatric disorder (Chen et al. 2011 ; Forbes et al. 2006 ; Gur et al. 2002 ; Jaworska et al. 2015 ; Zhou et al. 2007 ), which lead to different neural energy consumption results. However, it is unable to tell how molecular/cellular/whole-brain levels changes lead to neuropsychiatric disorders only by these experiments, because brain is highly coupled by these non-independent components. Since neural energy theory plays a significant role in encoding cognitive activities, could it also become a new explanation for neuropsychiatric disorders?
This is possible and valuable, but few researchers have been working on it. Although neuropsychiatric disorders are usually accompanied by much more complex intracellular activities (e.g., overexpression and underexpression of key proteins, interactions of different neurotransmitters and ion channels) than those in simple cognitive activities, these changes will finally act on the membrane potentials and neural population activities. With some biophysical models like HâH model (Hodgkin and Huxley 1952 ), Rallâs compartment model (Rall 1962 ) and neurotransmitter receptor binding models (Destexhe et al. 1995 ), researchers can simulate the membrane potentials with ion currents and neurotransmitters data from the available electrophysiological experiments of neuropsychiatric disorders. After that, it is easy to calculate the neuronal energy consumption of ion channels, synaptic activities and neuronal activities as well as the neuronal populationâs behaviors.
Liâs study in the field of major depressive disorder has made an exploration of the application of neural energy theory to neuropsychiatric disorder (Li et al. 2022b ). Li et al. chose medium spiny neurons (MSNs) of nucleus accumbens (NAc) as the research objective, which is a key neuronal type in dopaminergic pathway and closely related to major depressive disorder. Using HâH model, Li et al. first successfully established the membrane potential computational model of single MSN in depression and normal group by adjusting several ion channel properties. Then, using energy model (Zhu et al. 2018b ), Li et al. calculated the neuronal power and energy consumption (Fig. 26 ).
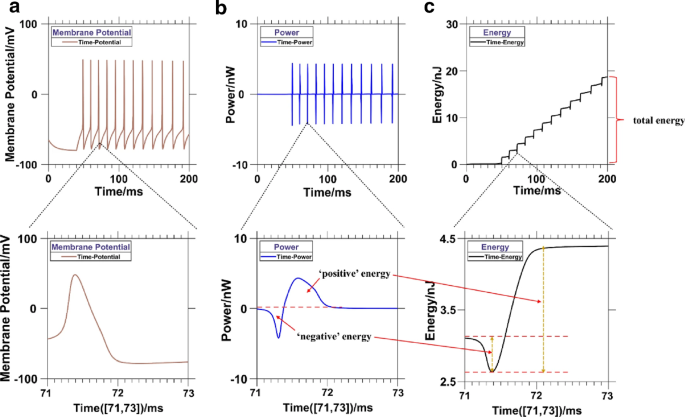
Membrane potential, neuronal power and energy in single MSN model. b and c showed the âpositiveâ and ânegativeâ components (Li et al. 2022a )
Further analyses showed differences in energy encoding patterns between the depression and normal group (Fig. 27 ): (1) the energy cost of MSN in MDD group was lower than that in control group; (2) the negative-to-total energy ratio of MSN in MDD group was higher than that in control group; and (3) the delay time of the power peak and the potential peak in MDD group were shorter than that in control group. These results are consistent with some behaviors and can be easily calculated by theoretical models, while are hardly to get only by biological experiments. In brief, it demonstrates that neural energy should be considered as an important part of decoding the mechanism of major depressive disorder, and it gives a new thought for the research of other neuropsychiatric disorders.

The abnormalities of MSN under energy model in MDD group (Li et al. 2022a ). a The total energy results. b The Negative-to-Positive energy ratio results. c The lag time (between power peak and the potential peak) results (Zhu et al. 2018b )
5 Conclusion remarks
This review article systematically summarizes how neural energy combines various levels of molecules, cells, networks, and behavior, thus systematically interpreting the so-called large-scale neuroscience theory is actually neural energy theory. Only neural energy theory makes it possible to systematically construct models of global neural activity in the brain, and to unify their respective advantages in reductionism and holism in neuroscience within a research framework. Only the neural energy theory is possible to study interactions among the microscopic, mesoscopic and macroscopic neural activities in a theoretical system from the perspective of global neural coding. Therefore, it is able to draw out the global information of how the brain works through comprehensive research and comparison of experimental data obtained at various levels (Wouapi et al. 2021 ; Navarro-LĂłpez et al. 2021 ; Churchland et al. 2002 , 2012 ; Tsuda et al. 1987 , 2004 ; Tsuda 1991 , 1992 , 1984 , 2001 , 2013 , 2015 ; Ebrahimzadeh et al. 2021 ; Yang et al. 2021a , b , 2022 ; Jiang et al. 2020 ; Sharma and Acharya 2021 ; Wang et al. 2006 , 2008 , 2009 , 2015a , b , 2017a , b , 2018a , b , 2019b , c , 2020 , 2021a , b , c , d , e ; Clancy et al. 2017 ; Videbech 2010 ; Zhang et al. 2019 , 2020 ; Yuan et al. 2022 , 2021 ; Yao and Wang 2019 ; Maltba et al. 2022 ; Zhou et al. 2020 , 2007 ; Li et al. 2020 , 2022a , b ; Kim and Lim 2020 ; Pfaff and Volkow 2022 ; Tsukada et al. 1975 , 2015 ; Kaneko and Tsuda 2001 ; Adachi and Aihara 1997 ; Aihara et al. 1990 ; Nara and Davis 1992 ; Pan et al. 2008 , 2014 ; Tsuda and Kuroda 2001 ; Fukushima et al. 2007 ; Kuroda et al. 2009 ; Yamaguti et al. 2011 ; Ryeu et al. 2001 ; Fujii and Tsuda 2004 ; Tadokoro et al. 2011 ; Collerton et al. 2016 ; Bullmore and Sporns 2009 ; Ullman 2019 ; Roy et al. 2019 ; Zeng et al. 2019 ; Wang and Zhu 2016 ; Deco et al. 2015 ; Kanwisher 2010a , b ; Wang and Wang 2018a , b , c , 2020 , 2014 ; Ma and Tang 2017 ; McIntyre et al. 2001 ; Moore and Cao 2008 ; Moore and Cao 2008 ; Lu et al. 2008a , b ; Peng and Wang 2021 ; Lu 2020 ; Wang and Pan 2021 ; Cheng et al. 2020 ; Fox and Raichle 2007a , b ; Balasubramanian 2021 ; Raichle 2010 ; Raichle and Mintun 2006 ; Piccoli et al. 2015 ; Compte 2000 ; Wei et al. 2012 ; Hsieh and Ranganath 2014 ; Karlsgodt et al. 2005 ; Williams-GarcĂa et al. 2014 ; Fosque et al. 2021 ; Barbey 2018a , b ; Wang et al. xxxx; Laughlin and Sejnowski 2003a , 2003b ; Zheng et al. 2022 , 2014 , 2016 ; Poirazi and Papoutsi 2020 ; Lynn and Bassett 2019 ; Hipp et al. 2011b ; Hipp et al. 2011c ; Raichle et al. 2018 ; Stender and Mortensen 2016 ; Kruegera et al. 2009 ; Stelnmetz et al. 2019 ; Esterman et al. 2009 ; Cohen 2017 ; Breakspear 2017 ; Johnson and Ray 2004 ; Nirenberg and Latham 2003 ; Victor 1999 ; Jacobs et al. 2009 ; Malnic et al. 1999 ; Miyamichi and Luo 2009 ; Xu et al. 2022a , b ; Hu and Wang 2013 ; Hu et al. 2012 , 2013 ; Fischler-Ruiz et al. 2021 ; Zhu et al. 2016a , b , 2018a , b , 2019 , 2020 ; Optican and Richmond 1987 ; Thorpe et al. 2001 ; Heil 2004 ; Chase and Young 2007 ; Zhong and Wang 2021a , 2021b , 2021c ; Xin et al. 2019 ; Insel et al. 2004 ; Feldman 2012 ; Wang and Zhang 2011 , 2006 ; Rubin et al. 2012 ; Panzeri et al. 2015 ; Stringer et al. 2019 ; Allen et al. 2019 ; GrĂŒndemann, et al. 2019 ; Qiu et al. 2015 ; Qin and Xie 2016 ; Wu et al. 2016 ; Byrne and Roberts 2009 ; Lv et al. 2016 ; Ma et al. 2017 , 2019 ; Liu 2002 ; Gu and Liang 2007 ; Haken 1996 ; Raichle and Gusnard 2002 ; Maandag et al. 2007 ; Lin et al. 2010 ; Peppiatt and Attwell 2004 ; Eikenberry and Marmarelis 2015 ; Sokoloff 2008 ; Maandag 2007 ; Figley and Stroman 2011 ; Pellerin and Magistretti 1994 ; Brown 2004a , 2004b ; DiNuzzo et al. 2012 ; Rong et al. 2020 ; Gazzaniga et al. 2002 ; Rubinov et al. 2011 ; Tolman 1948 ; OâKeefe and Dostrovsky 1971 ; Wilson and McNaughton 1993 ; Alme et al. 2014 ; OâKeefe and Recce 1993 ; Moser et al. 2008 ; Sargolini et al. 2006 ; Hafting et al. 2005 ; Fyhn et al. 2004 ; Barry et al. 2007 ; McNaughton et al. 2006 ; Yoon et al. 2016 ; Finkelstein et al. 2016 ; Hayman et al. 2011 , 2015 ; Casali et al. 2019 ; Yartsev and Ulanovsky 2013 ; Yartsev et al. 2011 ; Mathis et al. 2015 ; Giocomo et al. 2011 ; Burgess et al. 2007 ; Burak and Fiete 2009 ; Cash and Yuste 1999 ; Solstad et al. 2006 ; Sanchez-Vives and McCormick 2000 ; Compte et al. 2003 ; Abdallah et al. 2014 ; Ă gren and Niklasson 1988 ; GĆombik et al. 2020 ; Gu et al. 2021 ; Zuccoli et al. 2017 ; Chase et al. 2015 ; Duarte and Xin 2019 ; Martins-de-Souza et al. 2011 ; Pruett and Meador-Woodruff 2020 ; Konradi et al. 2004 ; Cui et al. 2018 ; Dietz et al. 2020 ; Chen et al. 2011 , 2021 ; Forbes et al. 2006 ; Gur et al. 2002 ; Jaworska et al. 2015 ; Hodgkin and Huxley 1952 ; Rall 1962 ; Destexhe et al. 1995 ; DĂ©li and KisvĂĄrday 2020 ). This is the only way out for us to walk out of the dilemma of blind men touching elephants in the field of neuroscience research.
Finally, we want to emphasize that the modeling and analysis methods of neural energy theory are based on neuron energy models. All the other coupling factors of neuron and neural network activity, such as the regulation of blood flow phenomenon to networks and the regulation of glial cells to neuron, have been considered as a result of the dynamic changes of neuron or coupling network.
From the above introduction, we know that the neural energy method can encode not only different stimulus information, but also the firing of individual neurons and neural oscillations at different frequencies at the neural network level. This is because (1) as a global brain function model, the neural energy model can be used to analyze and describe the experimental phenomena of neuroscience at all levels, so that the calculation results at all levels are no longer unusable, contradictory and irrelevant. That is to say, neural information can be expressed with energy at various levels of molecules, neurons, networks, cognition and behavior and the combination of all levels. Energy can be used to unify the neural model among all levels (Yuan et al. 2021 ; Wang and Zhu 2016 ; Wang et al. 2008 ); (2) Neural energy can be used together with the releasing pattern of membrane potential to interpret neural information processing (Chen et al. 2021 ); (3) Neural energy can describe the interaction of large scale neurons throughout the brain (at the combination of molecular, neuronal and network levels) (Déli and Kisvårday xxxx), which is otherwise impossible to be achieved by any traditional neural coding theory; (4) It is currently difficult to record damage in multiple brain regions simultaneously. Although EEG and MEG can sample neuronal activity from various regions of the brain, it is very difficult to estimate cortical interactions on the basis of these extracranial signals. The main obstacle is the lack of a theoretical tool that can effectively analyze cortical to cortical interactions in a high-dimensional space. In addition, there is no conversion relationship between scalp EEG and cortical potential. Nerve energy provides an effective solution to the above problems; (5) Since energy is a scalar quantity, whether it is a single neuron or a cluster, whether it is a network or a behavioral, linear or nonlinear neural model, their dynamic response can be described by the method of neural energy superposition. Thus, global information about functional neural activity in the brain can be obtained, which cannot be achieved by other traditional coding theories; (6) network coupling oscillation modes can be ever-changing, the coupling oscillation and the neural network and the network energy oscillation and there is a corresponding relationship, so when the large scale of the neural network modeling and numerical analysis for high dimensional nonlinear coupling is extremely complex and become impossible to deal with, you can use the nervous energy code to study the neural information processing, this makes complex neuroinformatics research simple and easy to process without losing information. Electrophysiological experiments in neuroscience have revealed the relationship between spontaneous brain activity and behavior, but it is difficult to give the quantitative relationship between behavior and brain energy consumption through experiments. And the importance of studying the quantitative relationship between the two lies in the future in the calculation of brain agent (Yuan et al. 2021 ; Peng and Wang 2021 ). However, there are two potential limitations of the proposed theory: (1) the technique of directly measuring brain energy supply and consumption in different temporal and spatial scales need to be further developed to advance the neural energy method; (2) the energy field model of the whole brain and its dynamic and interaction with different stimuli such as electric, magnetic or photic stimuli should be developed. These limitations are also promising future directions for research in this area. When new measuring techniques can determine the exact amount of energy consumed by a single neuron, a neural circuit and a brain area, deeper understanding about how the neural energy encodes stimulus, behavior and neural activity will be achieved. And a unified energy field model of the whole brain can provide the first-principle perspective of how the human brain interacts with the physical world. Even so, if one can master the behavior of the agent and the relationship with energy consumption, one can design the intelligent body neural chip through energy constraints, and find the agent's behavior and optimize the relationship between network parameters. So nervous energy theory and the calculation method can not only help to deeply understand macro behavior and the dependencies between the brain activity, but also can through the analysis of the brain nerve energy consumption, master the information of the whole information dynamic changes in the brain, to provide parameters of the basis for the design of agent behavior.
Data availability
We promise that the data in the paper can be publicly available and shared.
Abdallah CG, Jiang L, De Feyter HM et al (2014) Glutamate metabolism in major depressive disorder. Am J Psychiatry 171(12):1320â1327
Google Scholar Â
Adachi M, Aihara K (1997) Associative dynamics in chaotic neural networks. Neural Netw 10:83â98
MATH Â Google Scholar Â
Ă gren H, Niklasson F (1988) Creatinine and creatine in CSF: indices of brain energy metabolism in depression. J Neural Transm 74(1):55â59
Aihara K, Takabe T, Toyoda M (1990) Chaotic neural networks. Phys Lett A 144:333â340
MathSciNet  Google Scholar Â
Allen WE et al (2019) Thirst regulates motivated behavior through modulation of brainwide neural population dynamics. Science 364(6437):253
Alme CB, Miao CL, Jezek K, Treves A, Moser EI, Moser MB (2014) Place cells in the hippocampus: eleven maps for eleven rooms. Proc Natl Acad Sci USA 111(52):18428â18435
Balasubramanian V (2021) Brain power. Proc Natl Acad Sci USA 118(32):e2107022118
Barbey AK (2018a) Network neuroscience theory of human intelligence. Trends Cogn Sci 22(1):8â20
Barbey AK (2018b) Network neuroscience theory of human intelligence. Trends Cogn Sci 22:8â20
Barry C, Hayman R, Burgess N, Jeffery K (2007) Experience-dependent rescaling of entorhinal grids. Nat Neurosci 10(6):682â684
Basar E (1998) Brain function and oscillations. Springer, Berlin
Breakspear M (2017) Dynamic models of large-scale brain activity. Nat Neurosci 20:340â352
Brown AM (2004a) Brain glycogen re-awakened. J Neurochem 89:537â552
Brown AM (2004b) Energy transfer from astrocytes to axons: the role of CNS glycogen. Neurochem Int 45:529â536
Brydges CR, BarcelĂł F, Nguyen AT, Fox AM (2020) Fast fronto-parietal cortical dynamics of conflict detection and context updating in a flanker task. Cogn Neurodyn 14:795â814
Bullmore E, Sporns O (2009) Complex brain networks: graph theoretical analysis of structural and functional systems. Nat Rev Neurosci 10:186â198
Burak Y, Fiete IR (2009) Accurate path integration in continuous attractor network models of grid cells. PLoS Comput Biol 5(2):e1000291
Burgess N, Barry C, OâKeefe J (2007) An oscillatory interference model of grid cell firing. Hippocampus 17(9):801â812
Buxton RB (2012) Dynamic models of BOLD contrast. Neuroimage 62(2):953â961
Byrne JH, Roberts JL (2009) From molecules to networks. Elsevier, Amsterdam
Casali G, Bush D, Jeffery K (2019) Altered neural odometry in the vertical dimension. Proc Natl Acad Sci USA 116(10):4631â4636
Cash S, Yuste R (1999) Linear summation of excitatory inputs by CA1 pyramidal neurons. Neuron 22(2):383â394
Ăelik S, DoÄan RB, Parlatan CS, GĂŒntekin B (2021) Distinct brain oscillatory responses for the perception and identification of oneâs own body from otherâs body. Cogn Neurodyn 15:609â620
Chase SM, Young ED (2007) First-spike latency information in single neurons increases when referenced to population onset. Proc Natl Acad Sci USA 104(12):5175â5180
Chase KA, Rosen C, Gin H et al (2015) Metabolic and inflammatory genes in schizophrenia. Psychiatry Res 225(1â2):208â211
Chen CH, Suckling J, Lennox BR et al (2011) A quantitative meta-analysis of fMRI studies in bipolar disorder. Bipolar Disord 13(1):1â15
Chen H, Xie L, Wang Y, Zhang H (2021) Memory retention in pyramidal neurons: a unified model of energy-based homo and heterosynaptic plasticity with homeostasis. Cogn Neurodyn 15:675â692
Cheng X, Yuan Y, Wang R (2020) Neural antagonistic mechanism between default-mode and task-positive networks. Neurocomputing 417:74â85
Churchland MM, Cunningham JP, Kaufman MT, Foster JD, Nuyujukian P, Ryu SI, Shenoy KV (2002) Neural population dynamics during reaching. Nature 487:51â56
Churchland MM, Cunningham JP, Kaufman MT, Foster JD, Nuyujukian P, Ryu SI, Shenoy KV (2012) Neural population dynamics during reaching. Nature 487:51â56
Clancy K, Ding M, Bernat E et al (2017) Restless ârestâ: intrinsic sensory hyperactivity and disinhibition in post-traumatic stress disorder. Brain 140(7):2041â2050
Cohen MX (2017) Where does EEG come from and what does it mean? Trends Neurosci 40(4):208â218
Collerton D, Taylor J-P, Tsuda I, Fujii H, Nara S, Aihara K, Katori Y (2016) How can we see things that are not there? Current insights into complex visual hallucinations. J Conscious Stud 23(7â8):195â227
Compte A (2000) Synaptic mechanisms and network dynamics underlying spatial working memory in a cortical network model. Cereb Cortex 10(9):910â923
Compte A, Sanchez-Vives MV, Mccormick DA, Wang XJ (2003) Cellular and network mechanisms of slow oscillatory activity (< 1 Hz) and wave propagations in a cortical network model. J Neurophysiol 89(5):2707â2725
Cui Y, Yang Y, Ni Z et al (2018) Astroglial Kir4.1 in the lateral habenula drives neuronal bursts in depression. Nature 554(7692):323â327
Deco G et al (2015) Rethinking segregation and integration: contributions of whole-brain modelling. Nat Rev Neurosci 16(7):430â439
DĂ©li E, KisvĂĄrday Z (2020) The thermodynamic brain and the evolution of intellect: the role of mental energy. Cogn Neurodyn 14:743â756
Destexhe A, Mainen ZF, Sejnowski TJ (1995) Fast kinetic models for simulating AMPA, NMDA, GABA A and GABA B receptors. In: The neurobiology of computation, Springer, Berlin, pp 9â14
Dietz AG, Goldman SA, Nedergaard M (2020) Glial cells in schizophrenia: a unified hypothesis. Lancet Psychiatry 7(3):272â281
DiNuzzo M, Mangia S, Maraviglia B, Giove F (2012) The role of astrocytic glycogen in supporting the energetics of neuronal activity. Neurochem Res 37:2432â2438
Duarte J, Xin L (2019) Magnetic resonance spectroscopy in schizophrenia: evidence for glutamatergic dysfunction and impaired energy metabolism. Neurochem Res 44(1):102â116
Ebrahimzadeh E, Shams M, Jounghani AR, Fayaz F, Mirbagheri M, Hakimi N, Rajabion L, Soltanian-Zadeh H (2021) Localizing confined epileptic foci in patients with an unclear focus or presumed multifocality using a component-based EEGâfMRI method. Cogn Neurodyn 15:207â222
Eikenberry SE, Marmarelis VZ (2015) Principal dynamic mode analysis of the Hodgkin-Huxley equations. Int J Neural Syst 25(2):1550001
Ermentrout GB, GalĂĄn RF, Urban NN (2007) Relating neural dynamics to neural coding. Phys Rev Lett 99:248103
Esterman M, Chiu Y-C, Tamber-Rosenau BJ (2009) Decoding cognitive control in human parietal cortex. Proc Natl Acad Sci USA 106(42):17974â17979
Feldman J (2012) The neural binding problem(s). Cogn Neurodyn 6(5):409â419
Figley CR, Stroman PW (2011) The role(s) of astrocytes and astrocyte activity in neurometabolism, neurovascular coupling, and the production of functional neuroimaging signals. Eur J Neurosci 33:577â588
Finkelstein A, Las L, Ulanovsky N (2016) 3-D maps and compasses in the brain. Annu Rev Neurosci 39:171â196
Fischler-Ruiz W, Clark DG, Joshi N, Devi-Chou V, Kitch L, Schnitzer M, Abbott LF, Axel R (2021) Olfactory landmarks and path integration converge to form a cognitive spatial map. Neuron 109(24):4036â4049
Forbes EE, Christopher May J, Siegle GJ et al (2006) Reward-related decision-making in pediatric major depressive disorder: an fMRI study. J Child Psychol Psychiatry 47(10):1031â1040
Fosque LJ, Williams-GarcĂa RV, Beggs JM, Ortiz G (2021) Evidence for quasicritical brain dynamics. Phys Rev Lett 126:098101
Fox MD, Raichle ME (2007b) Spontaneous fluctuations in brain activity observed with functional magnetic resonance imaging. Nat Rev Neurosci 8(9):700â711
Fox MD, Raichle ME (2007a) Spontaneous fluctuations in brain activity observed with functional magnetic resonance imaging. Nature 8:710â711
Freeman WJ (2000) Neurodynamics. Springer, Berlin
Fujii H, Tsuda I (2004) Itinerant dynamics of class I neurons coupled by gap junctions. Lect Notes Comput Sci 3146:140â160
Fukushima Y, Tsukada M, Tsuda I, Yamaguti Y, Kuroda S (2007) Spatial clustering property and its self-similarity in membrane potentials of hippocampal CA1 pyramidal neurons for a spatio-temporal input sequence. Cogn Neurodyn 1:305â316
Fyhn M, Molden S, Moser EI, Moser MB (2004) Spatial representation in the entorhinal cortex. Science 305(5688):1258â1264
Gazzaniga MS, Ivry RB, Mangun GR (2002) Cognitive neuroscience. W.W. Norton & Company, London
Giocomo LM, Moser MB, Moser EI (2011) Computational models of grid cells. Neuron 71(4):589â603
GĆombik K, Detka J, Kurek A et al (2020) Impaired brain energy metabolism: involvement in depression and hypothyroidism. Front Neurosci 14:586939
GrĂŒndemann J et al (2019) Amygdala ensembles encode behavioral states. Science 364(6347):eaav8736
Gu F, Liang P (2007) Neural information processing. Beijing University of Technology Press, Beijing ( in Chinese )
Gu X, Ke S, Wang Q et al (2021) Energy metabolism in major depressive disorder: recent advances from omics technologies and imaging. Biomed Pharmacother 141:111869
Gur RE, McGrath C, Chan RM et al (2002) An fMRI study of facial emotion processing in patients with schizophrenia. Am J Psychiatry 159(12):1992â1999
Hafting T, Fyhn M, Molden S, Moser MB, Moser EI (2005) Microstructure of a spatial map in the entorhinal cortex. Nature 436(7052):801â806
Haken H (1996) Principles of brain functioning. Springer, Berlin
Hayman R, Verriotis MA, Jovalekic A, Fenton AA, Jeffery KJ (2011) Anisotropic encoding of three-dimensional space by place cells and grid cells. Nat Neurosci 14(9):1182â1188
Hayman RM, Casali G, Wilson JJ, Jeffery KJ (2015) Grid cells on steeply sloping terrain: evidence for planar rather than volumetric encoding. Front Psychol 6:925
Heil P (2004) First-spike latency of auditory neurons revisited. Curr Opin Neurobiol 14:461â467
Hipp JF, Engel AK, Siegel M (2011a) Oscillatory synchronization in large-scale cortical networks predicts perception. Neuron 69:387â396
Hipp JF, Enge AK, Siege M (2011b) Oscillatory synchronization in large-scale cortical networks predicts perception. Neuron 69:387â396
Hipp JF et al (2011c) Oscillatory synchronization in large-scale cortical networks predicts perception. Neuron 69:387â396
Hodgkin AL, Huxley AF (1952) A quantitative description of membrane current and its application to conduction and excitation in nerve. J Physiol 117(4):500
Hopfield JJ (2010) Neurodynamics of mental exploration. Proc Natl Acad Sci USA 107(4):1648â1653
Hsieh LT, Ranganath C (2014) Frontal midline theta oscillations during working memory maintenance and episodic encoding and retrieval. Neuroimage 85:721â729
Hu J, Wang R (2013) Responses of cutaneous mechanoreceptors within fingerpad to stimulus information for tactile softness sensation of materials. Cogn Neurodyn 7(5):441â447
Hu J, Yang X, Ding X, Wang R (2012) Probability of prickliness detection in a model of populations of fiber ends prickling human skin. Fibers Polym 13(1):79â86
Hu Y, Chen X, Gu H, Yang Y (2013) Resting-state glutamate and GABA concentrations predict task-induced deactivation in the default mode network. J Neurosci 33(47):18566â18573
Hu B, Xu M, Wang Z, Jiang D, Wang D, Zhang D (2021) The theoretical mechanism of Parkinsonâs oscillation frequency bands: a computational model study. Cogn Neurodyn 15:721â731
Insel TR, Landis ND, Li T-K, Sieving J, Sieving P (2004) Limits to growth: why neuroscience needs large-scale science. Nat Neurosci 7(5):426â427
Iribarren JL, Moro E (2009) Impact of human activity patterns on the dynamics of information diffusion. Phys Rev Lett 103:038702
Jacobs AL et al (2009) Ruling out and ruling in neural codes. Proc Natl Acad Sci USA 106(14):5936â5941
Jaworska N, Yang X-R, Knott V et al (2015) A review of fMRI studies during visual emotive processing in major depressive disorder. World J Biol Psychiatry 16(7):448â471
Jiang P, Yang X, Sun Z (2020) Dynamics analysis of the hippocampal neuronal model subjected to cholinergic action related with Alzheimerâs disease. Cogn Neurodyn 14:483â500
Johnson DH, Ray W (2004) Optimal stimulus coding by neural populations using rate codes. J Comput Neurosci 16:129â138
Kaneko K, Tsuda I (2001) Complex systems: chaos and beyond. Springer, Berlin
Kanwisher N (2010a) Functional specificity in the human brain: a window into the functional architecture of the mind. Proc Natl Acad Sci USA 107(25):11163â11170
Kanwisher N (2010b) Functional specificity in the human brain: a window into the functional architecture of the mind. Proc Natl Acad Sci USA 107(25):11163â11170
Karlsgodt KH et al (2005) Hippocampal activations during encoding and retrieval in a verbal working memory paradigm. Neuroimage 25(4):1224â1231
Kim S-Y, Lim W (2020) Cluster burst synchronization in a scale-free network of inhibitory bursting neurons. Cogn Neurodyn 14:69â94
Konradi C, Eaton M, MacDonald ML et al (2004) Molecular evidence for mitochondrial dysfunction in bipolar disorder. Arch Gen Psychiatry 61(3):300â308
Kruegera F, Barbey AK, McCabe K, Strenziok M, Zamboni G, Solomon J, Raymont V, Grafman J (2009) The neural bases of key competencies of emotional intelligence. Proc Natl Acad Sci USA 106(52):22486â22491
Kuroda S, Fukushima Y, Yamaguti Y, Tsukada M, Tsuda I (2009) Iterated function systems in the hippocampal CA1. Cogn Neurodyn 3(3):205â222
Lakatos P, Karmos G, Mehta AD, Ulbert I, Schroeder CE (2008) Entrainment of neuronal oscillations as a mechanism of attentional selection. Science 320:110â113
Laughlin SB, Sejnowski TJ (2003a) Communication in neuronal networks. Science 301:1870â1874
Laughlin SB, Sejnowski TJ (2003b) Communication in neural networks. Science 301:1870
Li X, Luo S, Xue F (2020) Effects of synaptic integration on the dynamics and computational performance of spiking neural network. Cogn Neurodyn 14:347â357
Li Y, Wang R, Zhang T (2022a) Two coding models of single neuron to predict the clinical symptoms in major depressive disorder. Nonlinear Dyn 107:3847â3862
Li Y, Wang R, Zhang T (2022b) Nonlinear computational models of dynamical coding patterns in depression and normal rats: from electrophysiology to energy consumption. Nonlinear Dyn 107:1â16
Lin AL, Fox PT, Hardies J, Duong TQ, Gao JH (2010) Nonlinear coupling between cerebral blood flow, oxygen consumption, and ATP production in human visual cortex. Proc Natl Acad Sci USA 107(18):8446â8451
Liu Y (2002) Electromagnetic biological effects. Beijing University of Posts and Telecommunications Press, Beijing ( in Chinese )
Lu Q (2020) Neurodynamics and mechanics. J Dyn Control 18(1):6â10 ( in Chinese )
Lu QS, Gu HG, Yang ZQ et al (2008a) Dynamics of firing patterns, synchronization and resonances in neuronal electrical activities: experiments and analysis. Acta Mech Sin 24(6):593â628
Lu Q, Liu S, Liu F et al (2008b) Research on dynamics and function of biological neural network systems. Adv Mech 38:366â393 ( in Chinese )
Lv M, Wang C, Ren G, Ma J, Song X (2016) Model of electrical activity in a neuron under magnetic flow effect. Nonlinear Dyn 85:1479â1490
Lynn CW, Bassett DS (2019) The physics of brain network structure, function and control. Nat Rev Phys 1:318â332
Ma J, Tang J (2017) A review for dynamics in neuron and neuronal network. Nonlinear Dyn 89:1569â1578
Ma J, Wu F, Hayat T et al (2017) Electromagnetic induction and radiation-induced abnormality of wave propagation in excitable media. Physica A 486:508â516
MathSciNet  MATH  Google Scholar Â
Ma J, Yang Z, Yang L et al (2019) A physical view of computational neurodynamics. J Zhejiang Univ Sci A 20(9):639â657
Maandag NJG (2007) Energetics of neuronal signaling and fMRI activity. Proc Natl Acad Sci USA 104(51):20546â20551
Maandag JG, Coman D, Sanganahalli BG et al (2007) Energetics of neuronal signaling and fMRI activity. Proc Natl Acad Sci USA 104(51):20546â20551
Malnic B, Hirono J, Sato T, Buck LB (1999) Combinatorial receptor codes for odors. Cell 96:713â723
Maltba TE, Zhao H, Tartakovsky DM (2022) Autonomous learning of nonlocal stochastic neuron dynamics. Cogn Neurodyn 16:683â705
Martins-de-Souza D, Harris LW, Guest PC et al (2011) The role of energy metabolism dysfunction and oxidative stress in schizophrenia revealed by proteomics. Antioxid Redox Signal 15(7):2067â2079
Mathis A, Stemmler MB, Herz AV (2015) Probable nature of higher-dimensional symmetries underlying mammalian grid-cell activity patterns. Elife 4:e05979
McIntyre J, Zago M, Berthoz A, Lacquaniti F (2001) Does the brain model Newtonâs laws? Nat Neurosci 4:693â694
McNaughton BL, Battaglia FP, Jensen O, Moser EI, Moser MB (2006) Path integration and the neural basis of the âcognitive map.â Nat Rev Neurosci 7(8):663â678
Memmesheimer R-M, Timme M (2006) Designing the dynamics of spiking neural networks. Phys Rev Lett 97:188101
Miyamichi K, Luo L (2009) Brain wiring by presorting axons. Science 325:44â45
Moore CI, Cao R (2008) The hemo-neural hypothesis: on the role of blood flow in information processing. J Neurophysiol 99:2035â2047
Moser EI, Kropff E, Moser MB (2008) Place cells, grid cells, and the brainâs spatial representation system. Annu Rev Neurosci 31:69â89
Nara S, Davis P (1992) Chaotic wandering and search in a cycle-memory neural network. Prog Theor Phys 88:845â855
Navarro-LĂłpez EM, Ăelikok U, Ćengör NS (2021) A dynamical model for the basal ganglia-thalamo-cortical oscillatory activity and its implications in Parkinsonâs disease. Cogn Neurodyn 15:693â720
Nirenberg S, Latham PE (2003) Decoding neuronal spike trains: how important are correlations? Proc Natl Acad Sci USA 100:7348â7353
OâKeefe J, Dostrovsky J (1971) The hippocampus as a spatial map. Preliminary evidence from unit activity in the freely-moving rat. Brain Res 34(1):171â175
OâKeefe J, Recce ML (1993) Phase relationship between hippocampal place units and the EEG theta rhythm. Hippocampus 3(3):317â330
Optican LM, Richmond BJ (1987) Temporal encoding of two-dimensional patterns by single units in primate inferior temporal cortex. III. Information theoretic analysis. J Neurophysiol 57(1):162â178
Pan X, Sawa K, Tsuda I, Tsukada M, Sakagami M (2008) Reward prediction based on stimulus categorization in primate lateral prefrontal cortex. Nat Neurosci 11:703â712
Pan X, Fan H, Sawa K, Tsuda I, Tsukada M, Sakagami M (2014) Reward inference by primate prefrontal and striatal neurons. J Neurosci 34(4):1380â1396
Panzeri S, Macke JH, Gross J, Kayser C (2015) Neural population coding: combining insights from microscopic and mass signals. Trends Cogn Sci 19(3):162â172
Pellerin L, Magistretti PJ (1994) Glutamate uptake into astrocytes stimulates aerobic glycolysis: a mechanism coupling neuronal activity to glucose utilization. Proc Natl Acad Sci USA 91:10625â10629
Peng J, Wang R (2021) Mechanism of global neural coding in fMRI hemodynamics. Nonlinear Dyn 103:883â895
Peppiatt C, Attwell D (2004) Feeding the brain. Nature 431:137â138
Pfaff DW, Volkow ND (2022) Neuroscience in the 21st century. Springer, Berlin
Piccoli T et al (2015) The default mode network and the working memory network are not anti-correlated during all phases of a working memory task. PLoS ONE 10(4):1â16
Poirazi P, Papoutsi A (2020) Illuminating dendritic function with computational models. Nat Rev Neurosci 21:303â321
Pouget A, Latham P (2002) Digitized neural networks: long-term stability from forgetful neurons. Nat Neurosci 5(8):709â710
Pruett BS, Meador-Woodruff JH (2020) Evidence for altered energy metabolism, increased lactate, and decreased pH in schizophrenia brain: a focused review and meta-analysis of human postmortem and magnetic resonance spectroscopy studies. Schizophr Res 223:29â42
Qin S, Xie C et al (2016) A magnetic protein biocompass. Nat Mater 15:217â226
Qiu C, Shivacharan RS, Zhang M, Durand DM (2015) Can neural activity propagate by endogenous electrical field? J Neurosci 35(48):15800â15811
Rabinovich MI, Huerta R (2006) Dynamics of sequential decision making. Phys Rev Lett 97:188103
Raichle ME (2010) Two views of brain function. Trends Cogn Sci 14(4):180â190
Raichle ME, Gusnard DA (2002) Appraising the brainâs energy budget. Proc Natl Acad Sci USA 99(16):10237â10239
Raichle ME, Mintun MA (2006) Brain work and brain imaging. Annu Rev Neurosci 29:449â476
Raichle ME et al (2018) Spontaneous infra-slow brain activity has unique spatiotemporal dynamics and laminar structure. Neuron 98(2):297â305
Rall W (1962) Electrophysiology of a dendritic neuron model. Biophys J 2(2Pt2):145
Rangan AV, Cai D, McLaughlin DW (2008) Quantifying neuronal network dynamics through coarse-grained event trees. Proc Natl Acad Sci USA 105(31):10990â10995
Rong W, Wang R, Zhang J, Kong W (2020) Neurodynamics analysis of cochlear hair cell activity. Theor Appl Mech Lett 10(1):8â15
Roy K, Jaiswal A, Panda P (2019) Towards spike-based machine intelligence with neuromorphic computing. Nature 575:607â617
Rubin W, Zhikang Z, Tse CK, Qu J, Cao J (2012) Neural coding in networks of multi-populations of neural oscillators. Math Comput Simul 86:52â66
Rubinov M, Sporns O, Thivierge J-P, Breakspear M (2011) Neurobiologically realistic determinants of self-organized criticality in networks of spiking neurons. PLoS Comput Biol 7(6):e1002038
Ryeu JK, Aihara K, Tsuda I (2001) Fractal encoding in a chaotic neural network. Phys Rev E 64:1â6
Sanchez-Vives MV, McCormick DA (2000) Cellular and network mechanisms of rhythmic recurrent activity in neocortex. Nat Neurosci 3(10):1027â1034
Sandrini M, Cohen LG, Censor N (2015) Modulating reconsolidation: a link to causal systems-level dynamics of human memories. Trends Cogn Sci 19(8):475â482
Sargolini F, Fyhn M, Hafting T, McNaughton BL, Witter MP, Moser MB et al (2006) Conjunctive representation of position, direction, and velocity in entorhinal cortex. Science 312(5774):758â762
Sharma M, Acharya UR (2021) Automated detection of schizophrenia using optimal wavelet-based L1 norm features extracted from single-channel EEG. Cogn Neurodyn 15:661â674
Sokoloff L (2008) The physiological and biochemical bases of functional brain imaging. Cogn Neurodyn 2:1â5
Solstad T, Moser EI, Einevoll GT (2006) From grid cells to place cells: a mathematical model. Hippocampus 16(12):1026â1031
Stelnmetz NA, Zatka-Haas P, Carandlnl M, Harrls KD (2019) Distributed coding of choice, action and engagement across the mouse brain. Nature 576:266â273
Stender J, Mortensen KN et al (2016) The minimal energetic requirement of sustained awareness after brain injury. Curr Biol 26:1494â1499
Stringer C et al (2019) Spontaneous behaviors drive multidimensional, brainwide activity. Science 364(6437):255
Tadokoro S, Yamaguti Y, Fujii H, Tsuda I (2011) Transitory behaviors in diffusively coupled nonlinear oscillators. Cogn Neurodyn 5(1):1â12
Takeda A (1999) Brain and physics. Shobo Co., Ltd., Tokyo ( in Japanese )
Thorpe S, Delorme A, Van Rullen R (2001) Spike-based strategies for rapid processing. Neural Netw 14:715â725
Tolman EC (1948) Cognitive maps in rats and men. Psychol Rev 55(4):189â208
Tsuda I (1984) A hermeneutic process of the brain. Prog Theor Phys 79:241â259
Tsuda I (1991) Chaotic itinerancy as a dynamical basis of Hermeneutics in brain and mind. World Futures 32:167â184
Tsuda I (1992) Dynamic link of memoryâchaotic memory map in nonequilibrium neural networks. Neural Netw 5:313â326
Tsuda I (2001) Toward an interpretation of dynamic neural activity in terms of chaotic dynamical systems. Behav Brain Sci 24(5):793â847
Tsuda I (2013) Chaotic itinerancy. Scholarpedia 8(1):4459
Tsuda I (2015) Chaotic itinerancy and its roles in cognitive neurodynamics. Curr Opin Neurobiol 31:67â71
Tsuda I, Kuroda S (2001) Cantor coding in the hippocampus. Jpn J Ind Appl Math 18(2):29â258
Tsuda I, Koerner E, Shimizu H (1987) Memory dynamics in asynchronous neural networks. Prog Theor Phys 78:51â71
Tsuda I, Fujii H, Tadokoro S, Yasuoka T, Yamaguti Y (2004) Chaotic itinerancy as a mechanism of irregular changes between synchronization and desynchronization in a neural network. J Integr Neurosci 3:159â182
Tsukada M, Ishii N, Sato R (1975) Temporal pattern discrimination of impulse sequences in the computer-simulated nerve cells. Biol Cybern 17:19â28
Tsukada H, Fujii H, Aihara K, Tsuda I (2015) Computational model of visual hallucination in dementia with Lewy bodies. Neural Netw 62:73â82
Ullman S (2019) Using neuroscience to develop artificial intelligence. Science 363(6428):692â693
Victor JD (1999) Temporal aspects of neural coding in the retina and lateral geniculate. Netw Comput Neural Syst 10:R1-66
Videbech P (2010) PET measurements of brain glucose metabolism and blood flow in major depressive disorder: a critical review. Acta Psychiatr Scand 101(1):11â20
Wang R, Pan X (2021) Research progress of neurodynamics in China. Cogn Neurodyn 15:1â2
Wang Z, Wang R (2014) Energy distribution property and energy coding of a structural neural network. Front Comput Neurosci. https://doi.org/10.3389/fncom.2014.00014
Article  Google Scholar Â
Wang Y, Wang R (2018a) An improved neuronal energy model that better captures of dynamic property of neuronal activity. Nonlinear Dyn 91(1):319â327
Wang R, Wang Z (2018b) The essence of neuronal activity from the consistency of two different neuron models. Nonlinear Dyn 92:973â982
Wang G, Wang R (2018c) Simulation of retinal ganglion cell response using fast independent component analysis. Cogn Neurodyn 12(6):615â624
Wang G, Wang R (2020) The relationship between sparseness and energy consumption of neural networks. Neural Plast 2020:8848901
Wang R, Zhang Z (2006) Mechanism on brain information processing: energy coding. Appl Phys Lett 89:123903
Wang R, Zhang Z (2011) Phase synchronization motion and neural coding in dynamic transmission of neural information. IEEE Trans Neural Netw 22(7):1097â1106
Wang R, Zhu Y (2016) Can the activities of the large-scale cortical network be expressed by neural energy? A brief review. Cogn Neurodyn 10:1â5
Wang Z, Kai L, Day M et al (2006) Dopaminergic control of corticostriatal long-term synaptic depression in medium spiny neurons is mediated by cholinergic interneurons. Neuron 50(3):443â452
Wang R, Zhang Z, Chen G (2008) Energy function and energy evolution on neural population. IEEE Trans Neural Netw 19(3):535â538
Wang R, Zhang Z, Chen G (2009) Energy coding and energy functions for local activities of brain. Neurocomputing 73(1â3):139â150
Wang R, Tsuda I, Zhang Z (2015a) A new work mechanism on neuronal activity. Int J Neural Syst 25(03):1450037
Wang Z, Wang R, Fang R (2015b) Energy coding in neural network with inhibitory neurons. Cogn Neurodyn 9(2):129â144
Wang Y, Wang R, Zhu Y (2017a) Optimal path-finding through mental exploration based on neural energy field gradients. Cogn Neurodyn 11(1):99â111
Wang Y, Wang R, Xu X (2017b) Neural energy supplyâconsumption properties based on Hodgkin-Huxley model. Neural Plast 2017:6207141
Wang Y, Xu X, Wang R (2018a) Intrinsic sodium currents and excitatory synaptic transmission influence spontaneous firing in up and down activities. Neural Netw 98:42â50
Wang Y, Xu X, Wang R (2018b) An energy model of place cell network in three dimensional space. Front Neurosci 12:264
Wang C, Tang J, Ma J (2019a) Minireview on signal exchange between nonlinear circuits and neurons via field coupling. Eur Phys J Spec Top 228(10):1907â1924
Wang Y, Xu X, Wang R (2019b) The place cell activity is information-efficient constrained by energy. Neural Netw 116:110â118
Wang YH, Xu XY, Zhu YT, Wang RB (2019c) Neural energy mechanism and neurodynamics of memory transformation. Nonlinear Dyn 97(1):697â714
Wang R, Lin P, Liu M et al (2019) Hierarchical connectome modes and critical state jointly maximize human brain functional diversity. Phys Rev Lett 123:038301
Wang R et al (2020) Advances in neurodynamic research. J Dyn Control 18(1):1â5 ( in Chinese )
Wang Y, Xu X, Wang R (2021a) Energy features in spontaneous up and down oscillations. Cogn Neurodyn 15:65â75
Wang R, Liu M, Cheng X et al (2021b) Segregation, integration, and balance of large-scale resting brain networks configure different cognitive abilities. Proc Natl Acad Sci USA 118(23):e2022288118
Wang YH, Xu XY, Wang RB (2021c) Modeling the grid cell activity on non-horizontal surfaces based on oscillatory interference modulated by gravity. Neural Netw 141:199â210
Wang YH, Xu XY, Pan XC, Wang RB (2021d) Grid cell activity and path integration on 2-D manifolds in 3-D space. Nonlinear Dyn 104(2):1767â1780
Wang YH, Xu XY, Wang RB (2021e) Energy features in spontaneous up and down oscillations. Cogn Neurodyn 15(1):65â75
Wei Z et al (2012) From distributed resources to limited slots in multiple-item working memory: a spiking network model with normalization. J Neurosci 32(33):11228â11240
Williams-GarcĂa RV, Moore M, Beggs JM, Ortiz G (2014) Quasicritical brain dynamics on a nonequilibrium Widom line. Phys Rev E 90:062714
Wilson MA, McNaughton BL (1993) Dynamics of the hippocampal ensemble code for space. Science 261(5124):1055â1058
Wouapi MK, Fotsin BH, Ngouonkadi EBM, Kemwoue FF, Njitacke ZT (2021) Complex bifurcation analysis and synchronization optimal control for Hindmarsh-Rose neuron model under magnetic flow effect. Cogn Neurodyn 15:315â347
Wu F, Wang C, Xu Y, Ma J (2016) Model of electrical activity in cardiac tissue under electromagnetic induction. Sci Rep 6(1):28
Xin Y, Zhong L, Zhang Y, Zhou T, Pan J, Xu N-L (2019) Sensory-to-category transformation via dynamic reorganization of ensemble structures in mouse auditory cortex. Neuron 103(5):909-921.e6
Xu X, Zhu Z, Wang Y, Wang R (2022a) Odor pattern recognition of a novel bio-inspired olfactory neural network based on kernel clustering. Commun Nonlinear Sci Numer Simul 109:106274
Xu XY, Wang YH, Wang RB (2022b) The place cell activity in three-dimensional space generated by multiple grid cell inputs. Nonlinear Dyn 108(2):1719â1731
Yamaguti Y, Kuroda S, Fukushima Y, Tsukada M, Tsuda I (2011) A mathematical model for cantor coding in the hippocampus. Neural Netw 24:43â53
Yang C, Liu Z, Wang Q, Luan G, Zhai F (2021a) Epileptic seizures in a heterogeneous excitatory network with short-term plasticity. Cogn Neurodyn 15:43â51
Yang Y, Ma J, Xu Y, Jia Y (2021b) Energy dependence on discharge mode of Izhikevich neuron driven by external stimulus under electromagnetic induction. Cogn Neurodyn 15:265â277
Yang H, Xu G, Wang H (2022) Effects of magnetic fields on stochastic resonance in Hodgkin-Huxley neuronal network driven by Gaussian noise and non-Gaussian noise. Cogn Neurodyn 16:707â717
Yao M, Wang R (2019) Neurodynamic analysis of Merkel cellâneurite complex transduction mechanism during tactile sensing. Cogn Neurodyn 13:293â302
Yartsev MM, Ulanovsky N (2013) Representation of three-dimensional space in the hippocampus of flying bats. Science 340(6130):367â372
Yartsev MM, Witter MP, Ulanovsky N (2011) Grid cells without theta oscillations in the entorhinal cortex of bats. Nature 479(7371):103â107
Yoon KJ, Lewallen S, Kinkhabwala AA, Tank DW, Fiete IR (2016) Grid cell responses in 1D environments assessed as slices through a 2D lattice. Neuron 89(5):1086â1099
Yu Y, Wang X, Wang Q, Wang Q (2020) A review of computational modeling and deep brain stimulation: applications to Parkinsonâs disease. Appl Math Mech 41:1747â1768
Yuan Z, Feng P, Fan Y, Yu Y, Wu Y (2022) Astrocytic modulation on neuronal electric mode selection induced by magnetic field effect. Cogn Neurodyn 16:183â194
Yuan Y, Pan X, Wang R (2021) Biophysical mechanism of the interaction between default mode network and working memory network. Cogn Neurodyn 15:1101â1124
Zeng G, Chen Y, Cui B, Yu S (2019) Continual learning of context-dependent processing in neural networks. Nat Mach Intell 1:364â372
Zhang T, Pan X, Xu X, Wang R (2019) A cortical model with multi-layers to study visual attentional modulation of neurons at the synaptic level. Cogn Neurodyn 13:579â599
Zhang W, Guo L, Liu D, Xu G (2020) The dynamic properties of a brain network during working memory based on the algorithm of cross-frequency coupling. Cogn Neurodyn 14:215â228
Zheng J, Wang R, Kong W, Zhang J (2022) A new patterns of self-organization activity of brain: neural energy coding. Inf Sci 608:1747â1755
Zheng H, Wang R, Qiao L, Du Y (2014) The molecular dynamics of neural metabolism during the action potential. Sci China Technol Sci 57(5):857â863
Zheng H, Wang R, Qu J (2016) Effect of different glucose supply conditions on neuronal energy metabolism. Cogn Neurodyn 10(6):563â571
Zhong H, Wang R (2021a) A new discovery on visual information dynamic changes from V1 to V2: corner encoding. Nonlinear Dyn 105:3551â3570
Zhong H, Wang R (2021b) A visual-degradation-inspired model with HSV color-encoding for contour detection. J Neurosci Methods 369:109423
Zhong H, Wang R (2021c) Neural mechanism of visual information degradation from retina to V1. Cogn Neurodyn 15:299â313
Zhou Y, Liang M, Tian L et al (2007) Functional disintegration in paranoid schizophrenia using resting-state fMRI. Schizophr Res 97(1â3):194â205
Zhou X, Xu Y, Wang G, Jia Y (2020) Ionic channel blockage in stochastic Hodgkin-Huxley neuronal model driven by multiple oscillatory signals. Cogn Neurodyn 14:569â578
Zhu Y, Wang R, Wang Y (2016a) A comparative study of the impact of theta-burst and high-frequency stimulation on memory performance. Front Hum Neurosci 10:19
Zhu Y, Wang R, Wang Y (2016b) The impact of theta-burst stimulation on memory mechanism: a modeling study. Appl Math Mech 37(3):395â402
Zhu Y, Nachtrab G, Keyes PC et al (2018a) Dynamic salience processing in paraventricular thalamus gates associative learning. Science 362(6413):423â429
Zhu Z, Wang R, Zhu F (2018b) The energy coding of a structural neural network based on the Hodgkin-Huxley model. Front Neurosci 12:122
Zhu F, Wang R, Pan X, Zhu Z (2019) Energy expenditure computation of a single bursting neuron. Cogn Neurodyn 13:75â87
Zhu F, Wang R, Aihara K, Pan X (2020) Energy-efficient firing patterns with sparse bursts in the Chay neuron model. Nonlinear Dyn 100:2657â2672
Zuccoli GS, Saia-Cereda VM, Nascimento JM et al (2017) The energy metabolism dysfunction in psychiatric disorders postmortem brains: focus on proteomic evidence. Front Neurosci 11:493
Download references
Acknowledgements
Special thanks to Professor Ichiro Tsuda for providing relevant materials on the contributions made by Japanese scientists in the field of neurodynamics. This review paper would not be complete without the valuable material they provide. Thanks also to other Japanese Professors Minoru Tsukada, Kazuyuki Aihara, Hiroshi Fujii and Shigetoshi Nara for their innovative work in the field of neurodynamics.
This study was funded by the National Natural Science Foundation of China (Nos. 11472104, 12172132, 11972195, 12272136, 11872180, 12072113).
Author information
Authors and affiliations.
School of Computer Science, Hangzhou Dianzi University, Hangzhou, China
Institute for Cognitive Neurodynamics, East China University of Science and Technology, Shanghai, China
Rubin Wang, Yihong Wang, Xuying Xu, Yuanxi Li & Xiaochuan Pan
You can also search for this author in PubMed  Google Scholar
Contributions
Rubin Wang designed the research and wrote the main manuscript. Others wrote some of the chapters and prepared figures. Yihong Wang and Xuying Xu revised the manuscript. All authors reviewed the manuscript.
Corresponding authors
Correspondence to Rubin Wang or Yihong Wang .
Ethics declarations
Conflict of interest.
All authors declare that they have no conflict of interest.
Additional information
Publisher's note.
Springer Nature remains neutral with regard to jurisdictional claims in published maps and institutional affiliations.
Rights and permissions
Open Access This article is licensed under a Creative Commons Attribution 4.0 International License, which permits use, sharing, adaptation, distribution and reproduction in any medium or format, as long as you give appropriate credit to the original author(s) and the source, provide a link to the Creative Commons licence, and indicate if changes were made. The images or other third party material in this article are included in the article's Creative Commons licence, unless indicated otherwise in a credit line to the material. If material is not included in the article's Creative Commons licence and your intended use is not permitted by statutory regulation or exceeds the permitted use, you will need to obtain permission directly from the copyright holder. To view a copy of this licence, visit http://creativecommons.org/licenses/by/4.0/ .
Reprints and permissions
About this article
Wang, R., Wang, Y., Xu, X. et al. Brain works principle followed by neural information processing: a review of novel brain theory. Artif Intell Rev 56 (Suppl 1), 285â350 (2023). https://doi.org/10.1007/s10462-023-10520-5
Download citation
Published : 24 June 2023
Issue Date : October 2023
DOI : https://doi.org/10.1007/s10462-023-10520-5
Share this article
Anyone you share the following link with will be able to read this content:
Sorry, a shareable link is not currently available for this article.
Provided by the Springer Nature SharedIt content-sharing initiative
- Brain theory
- Large-scale neuroscience
- Global neural coding
- Neural energy models
- Unification of reductionism and holism
- Find a journal
- Publish with us
- Track your research
- Brain & Nervous System
What to Know About Gamma Brain Waves

âYour brain is constantly at work, performing processes like thinking, concentrating, remembering, and more. During these activities, your brain cells constantly communicate with each other. This produces patterns or rhythms called brain waves. Gamma brain waves are one such wave pattern created by your brain.
Here is all you need to know about gamma brain waves and how they benefit you.
Understanding Brain Waves
âYour brain is made up of cells called neurons. When your brain performs neurological functions, neurons transmit information to each other via electrical signals. These signals produce rhythms or wave patterns, which are known as brain waves. â
Brain waves can be measured using electroencephalography , or EEG, and magnetoencephalography, or MEG. These techniques involve the attachment of electrodes or sensors to your head for detecting your brainâs activity.
Types of Brain Waves
âUsing EEG, brain waves are measured in cycles per second or Hertz based on their frequency or speed. This suggests that your brain waves can be fast or slow.
When you are awake and alert, your brain is more active. This results in fast brain waves. If you are sleepy or bored, they may be slower. Accordingly, you can have the following types of brain waves ranging from the slowest to the fastest: â
Delta brain waves. Your brain produces delta waves when you are completely relaxed in deep sleep . Delta waves are essential for your body to heal and restore during sleep. These are the slowest brain waves and range from 1 to 4 Hertz. â
Theta brain waves. Theta waves are produced when youâre sleeping or daydreaming when awake. These waves are associated with relaxation and low alertness. They occur when youâre lost in thought, drowsy, or sleepy. They range from 4 to 8 Hertz.Â
Alpha brain waves. Alpha waves are produced when youâre awake but your mind is in a resting state. They are associated with being calm and alert. They can help you during activities that require learning and coordinating. Â They fall right in the middle of the brain wave spectrum, ranging from 8 to 12 Hertz.
Beta brain waves. Beta waves are produced throughout your daily activities. It occurs when youâre awake, alert, busy, and focused. These waves range from 12 to 38 Hertz and can be divided into the following subtypes:
- Low beta waves. These range from 12 to 15 Hertz and occur when youâre thinking.
- Beta waves. These range from 15 to 22 Hertz and occur when youâre performing activities or focusing on something.
- High beta waves. These range from 22 to 38 Hertz. They occur when youâre excited or anxious. They also occur when you experience something new or have complex thoughts.Â
Gamma brain waves. Gamma waves are the fastest brain waves. They mainly occur when youâre highly alert and conscious. They range from 30 to 80 Hertz.
Gamma Brain Waves
âGamma brain waves have the highest frequency among all brain waves. They are associated with high levels of thought and focus. They can have different effects depending on their levels in your brain:â
- If your brain produces high levels of gamma waves, you tend to be happier and more receptive. You may also have a higher intelligence quotient or IQ and better concentration.
- If your brain produces low levels of gamma waves, you may develop learning and memory problems. You may experience lower concentration, attention span, and mental ability. Lower levels of gamma waves can even lead to learning disabilities and mental disorders.
Benefits of Gamma Brain Waves
âGamma waves are associated with higher brain functions like cognition and memory. A recent study has shown that gamma waves can improve your working memory.
Studies have also revealed that increased gamma brain wave activity can help you achieve the highest concentration levels. Gamma waves can also promote higher states of awareness and increased brain function during meditation. â
Gamma waves have the following benefits:
- They can improve your cognition and problem-solving ability
- They help you with information processing
- They can improve your memory
- They can help increase your attention span
- They can increase your awareness and mindfulness
- They may boost your brainâs immunity and function
Because of these effects, researchers have indicated the use of gamma brain waves to treat the following conditions:
- Alzheimerâs disease
- Schizophrenia
- Mild traumatic brain injury
- Attention deficit hyperactivity disorder or ADHD
- Mood disorders like depression
How to Boost Your Brain Waves
âYou can manipulate and boost your brain waves through various techniques.
Meditation. Different types of meditation and breathing exercises can increase the production of gamma waves in your brain.
Diet. Including nuts like pistachios and peanuts in your diet can help boost your gamma and delta brain waves, respectively. â
Music. A study has shown that auditory stimuli like music or binaural beats can help boost your alpha and theta brain waves.
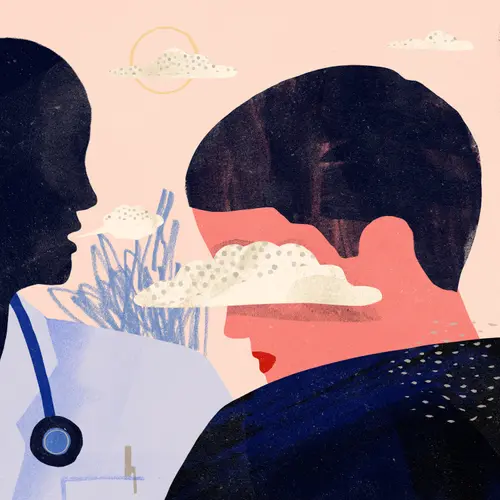
Top doctors in ,
Find more top doctors on, related links.
- Brain & Nervous System News & Features
- Brain & Nervous System Reference
- Brain & Nervous System Slideshows
- Brain & Nervous System Quizzes
- Brain & Nervous System Videos
- Find a Neurologist
- Living Better With MS
- Alzheimer's Disease
- Creutzfeldt-Jakob Disease
- Guillain-Barré Syndrome
- Lou Gehrigâs Disease (ALS)
- Multiple Sclerosis
- Parkinson's Disease
- Restless Legs Syndrome
- Spinal Muscular Atrophy
- More Related Topics
Itâs a wonderful world â and universe â out there.
Come explore with us! Â
Science News Explores
Study is first to link brainwaves to certain forms of thought.
It reveals when we are focused or instead have let our minds wander, perhaps to daydream
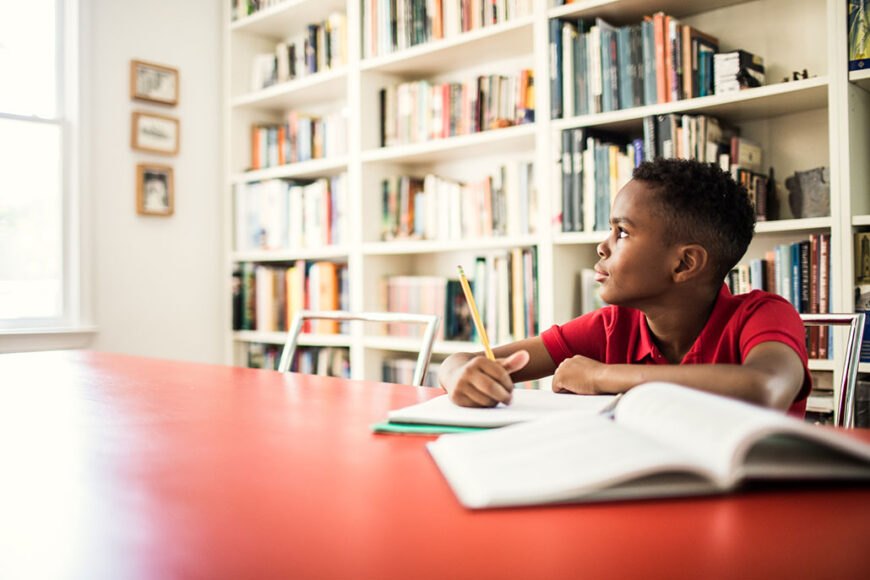
Is your mind focused? Daydreaming? Wandering freely? A new study finds that brainwaves can now answer that. And letting minds wander may signal people who are creative and better at solving tricky problems.
Juanmonino/E+/Getty Images Plus
Share this:
- Google Classroom
By Alison Pearce Stevens
March 8, 2021 at 6:30 am
Itâs a common occurrence: You sit down to study and find yourself thinking about a friend, dinner â anything but your upcoming test. Our minds wander. A lot. That so-called new train of thought has been tied to creativity and problem-solving. But some minds get stuck, fixated on the same thoughts over and over. A new study finds that patterns of electrical activity in the brain track different types of thoughts. This is the first time scientists have tied the brainâs activity to our thought patterns.
Neuroscientists are just starting to link brain activity to internal experiences, such as thoughts, says Loren Frank. Heâs a psychologist at the University of California, San Francisco who was not involved with the new work. This new study opens âup the possibility of understanding what we call thought,â he says. It has discovered âthat there are specific signatures associated with different types of internal thought processes.â This new finding, he says, âbrings us one step closer to identifying and understanding these processes.â
Julia Kam works at the University of Calgary in Alberta, Canada. As a cognitive neuroscientist, she studies the brainâs role in attention. Kam knew how much minds wander and how hard it can be to focus for a long time. She wanted to know more. Specifically, she wanted to find out whether certain patterns of activity in the brain might be linked to those different types of thoughts.
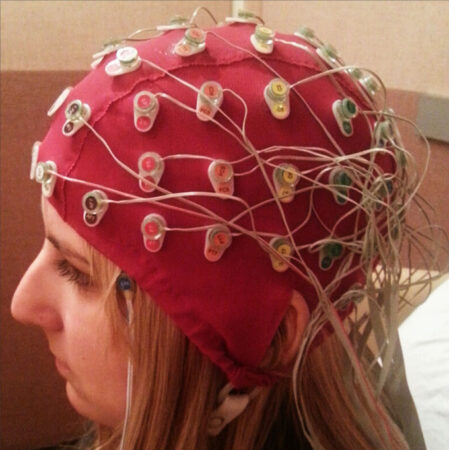
Kam previously worked at the University of California, Berkeley. While there, she teamed up with researchers there and elsewhere. They recruited 39 young adults to come into the Berkeley lab. There, the participants donned a sensor-lined cap. It recorded signals called EEGs. That stands for electroencephalograms (Ee-LEK-troh-en-SEF-uh-loh-grams). Each sensor detects brainwaves in the area just below it. EEG allows the researchers to see which parts of the brain are active at a given time.
While wearing the cap, each participant sat at a computer. An arrow appeared on the screen, pointing either right or left. When it did, they had to press the matching arrow on the keyboard. If that sounds like a simple task, it was. And that was intentional. The researchers wanted the participants to do a task that allowed their minds to wander. The arrows appeared in blocks of 18 to 30 trials. Each participant did a total of 35 blocks.
At the end of each block, researchers asked the participants what their thoughts were doing as they completed the task. Were they focused on the task? Were their thoughts wandering freely? Were they deliberately focusing on some topic? Or were their thoughts stuck on one specific thing? That last type is one that doesnât easily go away. Such thoughts might include worrying about a family member or replaying an argument with a friend.
After the participants had completed the task, the team examined the EEGs. They looked for patterns in brain activity that matched with the reported thought type. They were especially interested in patterns of what are known as alpha waves. These pulses of activity are caused by groups of brain cells firing together.
Kamâs team also looked at whether the recruitsâ thoughts had been related to the arrow task. And whether they were freely wandering, deliberately focused or stuck. Participants focused on the task during some blocks but not others, they found. Peopleâs thoughts wandered freely about as often as they were focused.
Educators and Parents, Sign Up for The Cheat Sheet
Weekly updates to help you use Science News Explores in the learning environment
Thank you for signing up!
There was a problem signing you up.
Letting thoughts flow
When the researchers matched up different thought types to specific blocks of brain activity, they found a clear connection. That was especially true in a part of the brain right behind the forehead. This frontal lobe is linked to attention, memory and problem-solving. But stronger alpha waves in this region did not indicate people had been paying attention to the task. Quite the opposite.
Alpha waves were stronger as people let their minds wander. They were weaker when people focused their thoughts â either on the task or on something else. The team had picked up a clear signal for brain activity that linked to how thoughts flow.
Kamâs team published its findings January 18 in Proceedings of the National Academy of Sciences .
âThe alpha band has been shown during creative processes, as well,â Kam says. And in this study, âthatâs when thoughts jump from one [thing] to another.â Such freely moving thoughts may be the key to creative problem-solving.
Itâs important to note that patterns of thought â whether focused or wandering â arenât inherently good or bad, Kam says. When you need to get your homework done or study for a test, you need to stay focused. But she says itâs okay to let your mind wander from time to time. âIt really depends on what your goal is at the moment.â If you want to solve a problem or do something creative, mind wandering might just be the secret to getting it done.
More Stories from Science News Explores on Brain
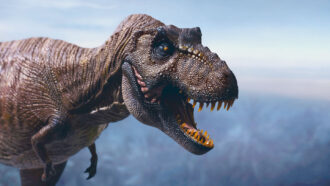
Just how brainy was a T. rex ?

Tiny treadmills reveal how fruit flies sprint
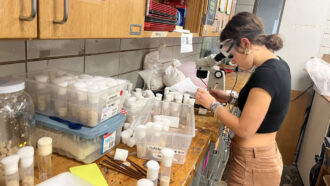
Herbal medicine could help recovery after concussion
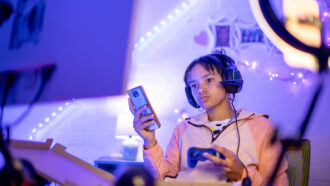
Youâre too distracted. Hereâs why that matters and what to do about it
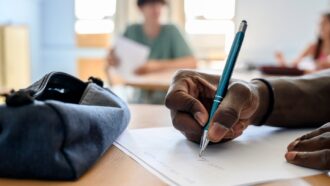
Handwriting may boost brain connections that aid memory
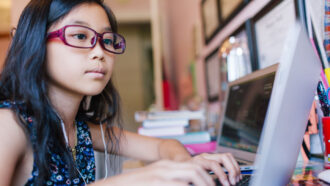
Scientists Say: Confirmation Bias
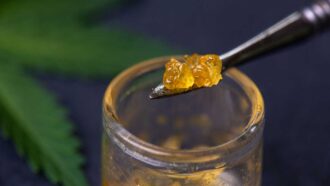
The teen brain is especially vulnerable to the harms of cannabis
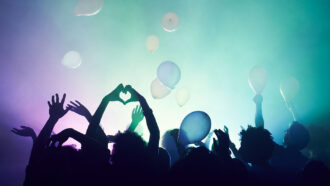
Music has the power to move us physically and emotionally. Hereâs why
- Bipolar Disorder
- Therapy Center
- When To See a Therapist
- Types of Therapy
- Best Online Therapy
- Best Couples Therapy
- Managing Stress
- Sleep and Dreaming
- Understanding Emotions
- Self-Improvement
- Healthy Relationships
- Student Resources
- Personality Types
- Sweepstakes
- Guided Meditations
- Verywell Mind Insights
- 2024 Verywell Mind 25
- Mental Health in the Classroom
- Editorial Process
- Meet Our Review Board
- Crisis Support
What Are Alpha Brain Waves?
Increasing alpha waves may reduce depression
janiecbros / Getty Images
How Are Alpha Brain Waves Measured?
What do alpha brain waves do, potential benefits of increasing alpha brain waves, tips for increasing alpha waves, what happens when alpha waves are imbalanced.
Alpha brain waves represent one pattern of electrical activity produced by the brain. The brain can produce slower or faster partners of activity, and alpha waves lie somewhere in the middle of this range.
Alpha waves usually occur when you are engaged in activities such as daydreaming, meditating, or practicing mindfulness. Research suggests that this type of brain wave may play a role in reducing symptoms of depression and improving creativity.
So what exactly is a brain wave? The brain is made up of millions of neurons that use electrical signals to transmit information. When groups of neurons fire together in a certain way to send signals to other groups of neurons, the resulting patterns are known as brain waves.
These electrical patterns are associated with different types of activity in the brain as well as different states of consciousness .
At a Glance
Alpha waves are a medium-frequency pattern of brain activity associated with restful and meditative states. These waves measure between 8 and 12 Hz, indicating the brain is active but relaxed. While researchers are still learning more, there is some evidence that alpha waves play an important role in well-being. Increasing this type of brain activity may help lower depression, combat anxiety, and increase creativity.
There are five different types of brain waves. Brain waves are measured by a tool known as an electroencephalogram (EEG). The picture that the tool creates portrays this electrical activity in a way that looks like waves.
An EEG looks at the frequency of these waves, or the cycles of activity per second. Some of these waves are faster and some are slowerâalpha waves are somewhere in the middle.
Types of Brain Waves
The five types of brain waves and their associated frequencies are:Â
- Delta waves , which are between 0.5 and 4 hertz (Hz), occur during deep states of dreamless sleep.
- Theta waves , which are between 4 and 8 Hz, occur during light sleep or deep relaxation.
- Alpha waves , which measure between 8 and 12 Hz, occur when people feel relaxed and when the brain is in an idle state without concentrating on anything.
- Beta waves , which measure between 12 and 30 Hz, are the waves that occur during most conscious, waking states. It is a fast activity that signals attentiveness and alertness.
- Gamma waves , which measure between 25 and 100 Hz, are the fastest wavelength brain waves that are linked to activities such as learning, problem-solving , and information processing.
When the brain produces alpha waves, it is because it is in a state that is relaxed and restful. This is why people experience these waves when they are doing stress-relieving activities such as meditating.
How does brain activity look on an EEG when alpha wave activity is prominent? When the brain displays this type of EEG wave, it often means that a person is focused on a specific thought and not paying attention to unwanted distractions. They tend to ignore sensory activity and are in a relaxed state.
Some evidence suggests that finding ways to boost these alpha waves may have several benefits. Some positive effects of boosting alpha waves include lowering stress, reducing anxiety , decreasing depression, and improving creative thinking.
Interest in using alpha waves grew after the development of biofeedback , a technique involving feedback from a tool that measures brain activity to learn how to consciously create alpha waves.
While more research is needed to fully understand the potential benefits, some evidence suggests that alpha waves can have several positive effects on mental well-being. Â
Reduced Depression
ï»ż A study published in the journal Translational Psychiatry found that using a technique called transcranial alternating current stimulation (tACS) to induce alpha wave activity reduced depressive symptoms in people with major depressive disorder. ï»ż ï»ż
While the studyâs sample size was small, the results show the therapeutic potential of increasing alpha waves to combat depression.
Press Play for Advice On Reducing Depression
Hosted by therapist Amy Morin, LCSW, this episode of The Verywell Mind Podcast shares how you can use behavioral activation to reduce your depression. Click below to listen now.
Follow Now : Apple Podcasts / Spotify / Google Podcasts
Greater Creativity
Increasing alpha waves may also help make you a more creative thinker. One 2015 study found that alpha wave activity was associated with spikes in creativity . This effect, they found, could be induced using non-invasive brain stimulation.
While such effects may help boost creative thought, it also offers promise for helping reduce depression. That's because boosting creativity can be a great way to break out of negative or destructive ways of thinking that play a role in depressive symptoms.
People with depressive symptoms often get stuck in repetitive patterns of thinking. Finding ways to break out of those destructive patterns may help reduce symptoms of depression.
Reducing Anxiety
Alpha waves are linked to more relaxed, restful mental states, which is why researchers also believe that they may help lower anxiety and stress.
For example, researchers have shown that increasing alpha and theta activity in the brainâs occipital lobes helped decrease anxiety and improve functioning in those with generalized anxiety disorder (GAD). Another study also found that alpha-wave neurofeedback training reduced anxiety and depression in people with GAD.
By boosting your alpha waves, you might also experience improved mood, decreased depression and anxiety, and a greater sense of calm.
Neurofeedback , also known as neurotherapy or electroencephalogram (EEG) biofeedback, is one way to learn how to consciously induce alpha waves. In this mind-body technique, people receive auditory and visual feedback about their brain activity using an EEG monitor.
While researchers continue to explore this as a therapeutic treatment for depression and anxiety, there are things that you can do to help improve your ability to experience this type of brain wave. Increase alpha wave activity by:
- Meditating : Meditation has many health benefits and has been linked to increased alpha waves.
- Deep breathing : Deep breathing can be an effective way to combat feelings of anxiety. Research also suggests that it may help boost alpha activity in critical brain areas.
- Practicing mindfulness : Studies have shown that mindfulness training can lead to increased alpha wave activity, and some research has suggested that this practice may help people who are experiencing chronic pain, anxiety, or depression.
- Yoga : The relaxed state associated with alpha waves may increase when performing yoga, and some research has suggested that regular yoga practice may enhance alpha activity in the brain.
- Aerobics : Some researchers suggest that aerobic exercise can increase alpha wave activity.
Neurofeedback is one approach that can allow you to induce these brain waves to a certain extent. Relaxation techniques such as meditation and mindfulness may also help.
While alpha waves have promise for reducing the symptoms of anxiety and depression, they can also cause problems if they are off-balance or out of sync.
For example, while alpha waves are often present right before you fall asleep, they should not occur as frequently while you are asleep. When alpha waves occur in excess during sleep, they can contribute to sleep disorders.
The brain normally produces delta waves during deep sleep. If alpha waves dominate, it disrupts sleep and leaves people feeling tired the following day.
Alexander ML, Alagapan S, Lugo CE, et al. Double-blind, randomized pilot clinical trial targeting alpha oscillations with transcranial alternating current stimulation (Tacs) for the treatment of major depressive disorder (Mdd) . Transl Psychiatry . 2019;9(1):106. doi:10.1038/s41398-019-0439-0
Lustenberger C, Boyle MR, Foulser AA, Mellin JM, Fröhlich F. Functional role of frontal alpha oscillations in creativity . Cortex . 2015;67:74-82. doi:10.1016/j.cortex.2015.03.012
Dadashi M, Birashk B, Taremian F, Asgarnejad AA, Momtazi S. Effects of increase in amplitude of occipital alpha & theta brain waves on global functioning level of patients with GAD . Basic Clin Neurosci . 2015;6(1):14-20. PMID:27504152
Hou Y, Zhang S, Li N, Huang Z, Wang L, Wang Y. Neurofeedback training improves anxiety trait and depressive symptom in GAD . Brain Behav . 2021;11(3):e02024. doi:10.1002/brb3.2024
Lagopoulos J, Xu J, Rasmussen I, Vik A, Malhi GS, Eliassen CF, Arntsen IE, Saether JG, Hollup S, Holen A, Davanger S, Ellingsen Ă. Increased theta and alpha EEG activity during nondirective meditation . J Altern Complement Med . 2009;15(11):1187-92. doi:10.1089/acm.2009.0113
MBBS (2nd Prof) student,AIIMS, Raipur, Gaurav S, Meenakshi S, et al. Effect of alterations in breathing patterns on EEG activity in normal human subjects . Int J Curr Res Med Sci . 2016;3(12):38-45. doi:10.22192/ijcrms.2016.02.12.007
Sacchet MD, LaPlante RA, Wan Q, et al. Attention drives synchronization of alpha and beta rhythms between right inferior frontal and primary sensory neocortex . Journal of Neuroscience . 2015;35(5):2074-2082. doi:10.1523/JNEUROSCI.1292-14.2015
Gutmann B, Mierau A, HĂŒlsdĂŒnker T, et al. Effects of physical exercise on individual resting state EEG alpha peak frequency . Neural Plast . 2015;2015:717312. doi:10.1155/2015/717312
By Kendra Cherry, MSEd Kendra Cherry, MS, is a psychosocial rehabilitation specialist, psychology educator, and author of the "Everything Psychology Book."
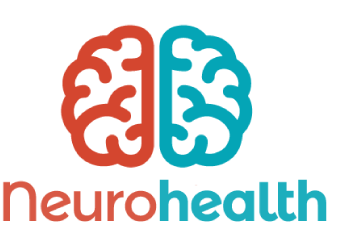
Brainwave Frequencies: What Are They?
Brain waves represent patterns of electrical activity produced by the brain. The brain is made up of billions of neurons that use electrical signals to transmit information.Â
When groups of neurons fire together in a certain way to send signals to other groups of neurons, the resulting patterns are known as brain waves. These electrical patterns are associated with different types of activity in the brain as well as different states of consciousness.
Brain Wave Types
There are 5 major types of brain waves used in research and studies:
Alpha waves , which measure between 8 and 12 Hz, occur when people feel relaxed and when the brain is in an idle state without concentrating on anything.
Beta waves , which measure between 12 and 30 Hz, are the waves that occur during most conscious, waking states. It is a fast activity that signals attentiveness and alertness.Â
Gamma waves , which measure between 25 and 100 Hz, are the fastest wavelength brain waves that are linked to activities such as learning, problem-solving, and information processing.
Delta waves , which are between 0.5 and 4 hertz (Hz), occur during deep states of dreamless sleep.
Theta waves , which are between 4 and 8 Hz, occur during light sleep or deep relaxation.
Brain Wave Functions
When the brain produces waves, it is because it is in a specific state. This is why people experience certain wave types when they engage in different types of behaviors and activities.
When the brain displays an EEG wave during testing, it often shows waves that correspond to the overall state of a personâs mind. For example; brains tend to ignore sensory activity and are in a relaxed state when alpha waves are high.
There is some evidence that suggests finding ways to boost certain types of brain waves may have a number of benefits. Some of the positive effects of boosting alpha waves include lowering stress, reducing anxiety, decreasing depression, and improving creative thinking.
Neurofeedback Therapy at NHA
Here at Neurohealth Associates, we specialize in Neurofeedback treatments. Neurofeedback may be helpful for treating your mind, especially if you are unsure about putting yourself or your child on medication. The easy, noninvasive treatments can painlessly improve your mental health condition and outlook on life. Schedule a consultation with NeuroHealth Associates today and find out how we can help you.
Original article posted by VeryWell Health.
Contact Us Today
- Full Name *
- Email This field is for validation purposes and should be left unchanged.
- February 2024
- January 2024
- December 2023
- November 2023
- October 2023
- August 2023
- February 2023
- January 2023
- December 2022
- November 2022
- October 2022
- September 2022
- August 2022
- February 2022
- January 2022
- December 2021
- November 2021
- October 2021
- September 2021
- August 2021
- February 2021
- January 2021
- December 2020
- November 2020
- October 2020
- September 2020
- August 2020
- February 2020
- January 2020
- December 2019
- November 2019
- October 2019
- September 2019
- August 2019
- February 2019
- January 2019
- December 2018
- November 2018
- October 2018
- September 2018
- August 2018
- February 2018
- January 2018
- December 2017
- November 2017
- October 2017
- September 2017
- August 2017
- February 2017
- January 2017
- December 2016
- November 2016
- October 2016
- September 2016
- August 2016
- August 2014
- October 2013
What Our Clients Are Saying

Teachers made huge comments on his math skills and behavior. I also saw this at home with understanding of what I said to him registering more with him. I saw this in his eyes: recognition. Fewer outbursts of anger.

I am extremely happy with my sonâs outcomes and feel very fortunate to have encountered Dr. Bonesteel early in my childâs life. This method has dramatically changed his ability to focus and take initiative. I feel confident that my sonâs life has been dramatically enhanced. I canât express my appreciation fully in words.

Dr. Bonesteel has masterfully, compassionately, and extremely kindly helped me navigate through a history of childhood and marital abuse, a child with twenty years of struggle with life-threatening physical and emotional illness, extended family discord, and disharmony with my child with severe depression. I am blessed to have found Neurohealth Associates.
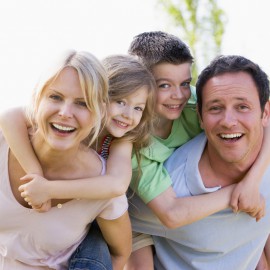
Overall, excellent experience. Very happy with Dr B and staff is wonderful. We feel like we have our family life back!
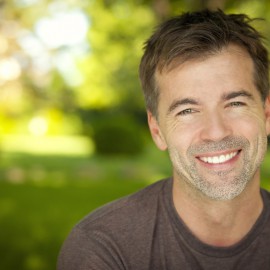
I am very thankful this technology was available for my training. I was extremely satisfied with all aspects of my training protocols.
Our Latest Posts
Managing anxiety with neurofeedback training, brain injuries & neurofeedback training, learning disorders & neurofeedback training, stay up to date.
with the latest news and information regarding neurofeedback and brain health.
- Evaluation Process
- Privacy Policy
OFFICE HOURS

IMAGES
COMMENTS
Brain waves associated with information processing or problem solving are _____ waves. beta. In short-term memory, there is increased synaptic activity by long-term potentiation. In working memory, ... The primary auditory cortex, where auditory stimuli are processed by the brain, is located in the superior part of the temporal lobe. ...
Brain waves associated with information processing or problem solving are _____ waves. theta. beta. delta. alpha. 8 of 22. Definition. there is increased synaptic activity by long-term potentiation. ... Brain waves associated with information processing or problem solving are _____ waves.
Brain waves are electrical impulses that flow through the brain, creating patterns of activity. They are measured in cycles per second, or hertz (Hz). There are four main types of brain waves: alpha, beta, delta, and theta. Each type of brain wave is associated with a different state of consciousness. Beta waves, for example, are associated ...
They may also be important for processing new information and integrating it with existing knowledge. Cognitive Functioning: Gamma waves are associated with a variety of higher-order cognitive functions, such as problem-solving, decision-making, and creativity. When your brain is generating a lot of gamma waves, you're likely in a state of ...
These interactions between gamma and beta waves might be how the brain solves an information overload problem, Miller suspects. Incoming sensory input constantly bombards the brain, and much of it ...
When these waves are being simultaneously generated in different places in the brain, they inevitably crash into one another. If two peaks of activity meet, they generate an even higher activity, while if a trough of low activity meets a peak, it might cancel it out. This process is called wave interference. "When you're out in the world ...
There are millions of neurons in the brain, and each neuron produces its own electrical signals. These combined signals generate oscillations known as brain waves, which vary in frequency. In a 2016 study, Miller and Lundqvist found that gamma rhythms are associated with encoding and retrieving sensory information.
Working memory coordinates the simultaneous storage and processing of information from multiple sources and plays a crucial role in higher cognitive functions like problem-solving, decision-making ...
According to the functional topography of brain activity in the theta and alpha frequency, our results suggest that the mental effort while solving scientific problems is related to working memory ...
It is currently known that brain waves express synchronized activity of tens of thousands of nerve cells (neurons), so a normal increase in wave intensity expresses synchronized activity of ...
Each serves a purpose to help us cope with various situations - whether it is to help us process and learn new information or help us calm down after a long stressful day. The five brain waves in order of highest frequency to lowest are as follows: gamma, beta, alpha, theta, and delta.
The way the brain work and its principle of work has long been a big scientific question that scientists have dreamed of solving. However, as is known to all, the brain works at different levels, and the operation at different levels is interactional and mutually coupled. Unfortunately, until now, we still do not know how the nervous system at different levels is interacting and coupling with ...
It is the state that most of brain is in when we have our eyes open and are listening and thinking during analytical problem solving, judgment, decision making, processing information about the world around us. ... its hypothesized that the 40Hz activity consolidates the required areas for simultaneous processing. A good memory is associated ...
These brain waves, which admittedly can be hard to measure accurately with current technology, are proof that your brain is hard at work, processing information and looking for solutions to problems.
The researchers hypothesize that the same kinds of waves are being generated -- and interacting with each other -- in every part of the brain's cortex, not just the part responsible for the ...
Gamma waves can also promote higher states of awareness and increased brain function during meditation. Gamma waves have the following benefits: They can improve your cognition and problem-solving ...
Mental Ability. Michael C. Ashton, in Individual Differences and Personality (Second Edition), 2013 10.4.5 Brain waves: averaged evoked potentials. In addition to the variables associated with the speed with which the brain can process information, other features of the brain's functioning have also been examined in relation to mental ability (see review by Jensen, 1998).
That was especially true in a part of the brain right behind the forehead. This frontal lobe is linked to attention, memory and problem-solving. But stronger alpha waves in this region did not indicate people had been paying attention to the task. Quite the opposite. Alpha waves were stronger as people let their minds wander.
At a Glance. Alpha waves are a medium-frequency pattern of brain activity associated with restful and meditative states. These waves measure between 8 and 12 Hz, indicating the brain is active but relaxed. While researchers are still learning more, there is some evidence that alpha waves play an important role in well-being.
It is a fast activity that signals attentiveness and alertness. Gamma waves, which measure between 25 and 100 Hz, are the fastest wavelength brain waves that are linked to activities such as learning, problem-solving, and information processing. Delta waves, which are between 0.5 and 4 hertz (Hz), occur during deep states of dreamless sleep.
5 benefits of increasing alpha brain waves. Improved relaxation: When your brain produces more alpha waves, you enter a state of calmness and tranquility which may help reduce feelings of stress and tension. Reduced stress and anxiety: Alpha waves can help calm the mind, lowering the production of stress hormones like cortisol.
Brain waves associated with information processing/problem solving are ____ waves. emotions/mood. motivation. sensations of pleasure/pain. The limbic system influences. BIO 1014. most inferior part of the brain. Click the card to flip đ. medulla oblongata.
Psychology questions and answers. Brain waves associated with information processing or problem solving are_________ ï»żwaves.Select one:a. ï»żkappab. ï»żdeltac. ï»żthetad. ï»żalphae. ï»żbeta.