- Skip to main content
- Skip to primary sidebar
- Skip to footer
- QuestionPro

- Solutions Industries Gaming Automotive Sports and events Education Government Travel & Hospitality Financial Services Healthcare Cannabis Technology Use Case AskWhy Communities Audience Contactless surveys Mobile LivePolls Member Experience GDPR Positive People Science 360 Feedback Surveys
- Resources Blog eBooks Survey Templates Case Studies Training Help center

Home Market Research Research Tools and Apps

Analytical Research: What is it, Importance + Examples
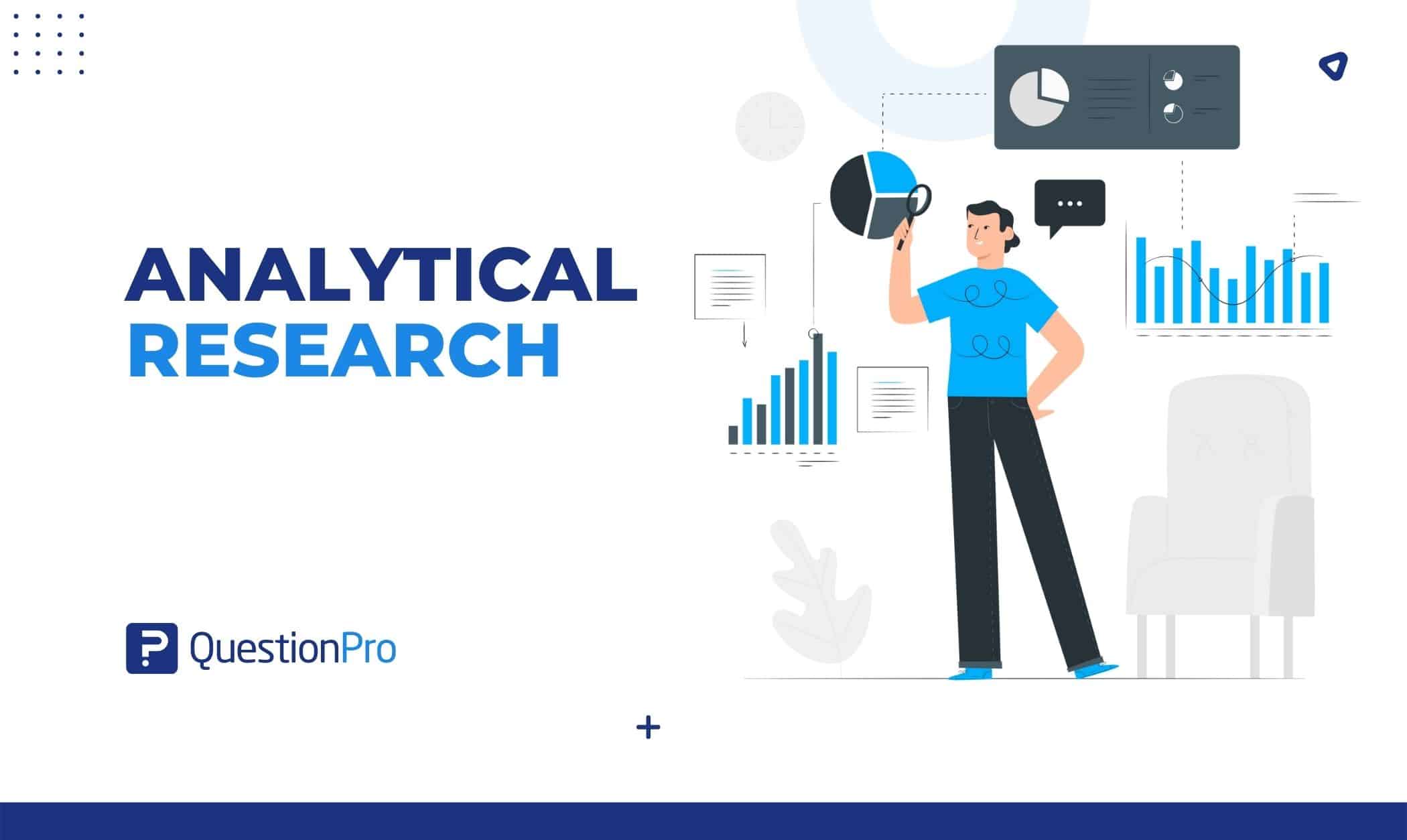
Finding knowledge is a loose translation of the word “research.” It’s a systematic and scientific way of researching a particular subject. As a result, research is a form of scientific investigation that seeks to learn more. Analytical research is one of them.
Any kind of research is a way to learn new things. In this research, data and other pertinent information about a project are assembled; after the information is gathered and assessed, the sources are used to support a notion or prove a hypothesis.
An individual can successfully draw out minor facts to make more significant conclusions about the subject matter by using critical thinking abilities (a technique of thinking that entails identifying a claim or assumption and determining whether it is accurate or untrue).
What is analytical research?
This particular kind of research calls for using critical thinking abilities and assessing data and information pertinent to the project at hand.
Determines the causal connections between two or more variables. The analytical study aims to identify the causes and mechanisms underlying the trade deficit’s movement throughout a given period.
It is used by various professionals, including psychologists, doctors, and students, to identify the most pertinent material during investigations. One learns crucial information from analytical research that helps them contribute fresh concepts to the work they are producing.
Some researchers perform it to uncover information that supports ongoing research to strengthen the validity of their findings. Other scholars engage in analytical research to generate fresh perspectives on the subject.
Various approaches to performing research include literary analysis, Gap analysis , general public surveys, clinical trials, and meta-analysis.
Importance of analytical research
The goal of analytical research is to develop new ideas that are more believable by combining numerous minute details.
The analytical investigation is what explains why a claim should be trusted. Finding out why something occurs is complex. You need to be able to evaluate information critically and think critically.
This kind of information aids in proving the validity of a theory or supporting a hypothesis. It assists in recognizing a claim and determining whether it is true.
Analytical kind of research is valuable to many people, including students, psychologists, marketers, and others. It aids in determining which advertising initiatives within a firm perform best. In the meantime, medical research and research design determine how well a particular treatment does.
Thus, analytical research can help people achieve their goals while saving lives and money.
Methods of Conducting Analytical Research
Analytical research is the process of gathering, analyzing, and interpreting information to make inferences and reach conclusions. Depending on the purpose of the research and the data you have access to, you can conduct analytical research using a variety of methods. Here are a few typical approaches:
Quantitative research
Numerical data are gathered and analyzed using this method. Statistical methods are then used to analyze the information, which is often collected using surveys, experiments, or pre-existing datasets. Results from quantitative research can be measured, compared, and generalized numerically.
Qualitative research
In contrast to quantitative research, qualitative research focuses on collecting non-numerical information. It gathers detailed information using techniques like interviews, focus groups, observations, or content research. Understanding social phenomena, exploring experiences, and revealing underlying meanings and motivations are all goals of qualitative research.
Mixed methods research
This strategy combines quantitative and qualitative methodologies to grasp a research problem thoroughly. Mixed methods research often entails gathering and evaluating both numerical and non-numerical data, integrating the results, and offering a more comprehensive viewpoint on the research issue.
Experimental research
Experimental research is frequently employed in scientific trials and investigations to establish causal links between variables. This approach entails modifying variables in a controlled environment to identify cause-and-effect connections. Researchers randomly divide volunteers into several groups, provide various interventions or treatments, and track the results.
Observational research
With this approach, behaviors or occurrences are observed and methodically recorded without any outside interference or variable data manipulation . Both controlled surroundings and naturalistic settings can be used for observational research . It offers useful insights into behaviors that occur in the actual world and enables researchers to explore events as they naturally occur.
Case study research
This approach entails thorough research of a single case or a small group of related cases. Case-control studies frequently include a variety of information sources, including observations, records, and interviews. They offer rich, in-depth insights and are particularly helpful for researching complex phenomena in practical settings.
Secondary data analysis
Examining secondary information is time and money-efficient, enabling researchers to explore new research issues or confirm prior findings. With this approach, researchers examine previously gathered information for a different reason. Information from earlier cohort studies, accessible databases, or corporate documents may be included in this.
Content analysis
Content research is frequently employed in social sciences, media observational studies, and cross-sectional studies. This approach systematically examines the content of texts, including media, speeches, and written documents. Themes, patterns, or keywords are found and categorized by researchers to make inferences about the content.
Depending on your research objectives, the resources at your disposal, and the type of data you wish to analyze, selecting the most appropriate approach or combination of methodologies is crucial to conducting analytical research.
Examples of analytical research
Analytical research takes a unique measurement. Instead, you would consider the causes and changes to the trade imbalance. Detailed statistics and statistical checks help guarantee that the results are significant.
For example, it can look into why the value of the Japanese Yen has decreased. This is so that an analytical study can consider “how” and “why” questions.
Another example is that someone might conduct analytical research to identify a study’s gap. It presents a fresh perspective on your data. Therefore, it aids in supporting or refuting notions.
Descriptive vs analytical research
Here are the key differences between descriptive research and analytical research:
Aspect | Descriptive Research | Analytical Research |
Objective | Describe and document characteristics or phenomena. | Analyze and interpret data to understand relationships or causality. |
Focus | “What” questions | “Why” and “How” questions |
Data Analysis | Summarizing information | Statistical research, hypothesis testing, qualitative research |
Goal | Provide an accurate and comprehensive description | Gain insights, make inferences, provide explanations or predictions |
Causal Relationships | Not the primary focus | Examining underlying factors, causes, or effects |
Examples | Surveys, observations, case-control study, content analysis | Experiments, statistical research, qualitative analysis |
The study of cause and effect makes extensive use of analytical research. It benefits from numerous academic disciplines, including marketing, health, and psychology, because it offers more conclusive information for addressing research issues.
QuestionPro offers solutions for every issue and industry, making it more than just survey software. For handling data, we also have systems like our InsightsHub research library.
You may make crucial decisions quickly while using QuestionPro to understand your clients and other study subjects better. Make use of the possibilities of the enterprise-grade research suite right away!
LEARN MORE FREE TRIAL
MORE LIKE THIS
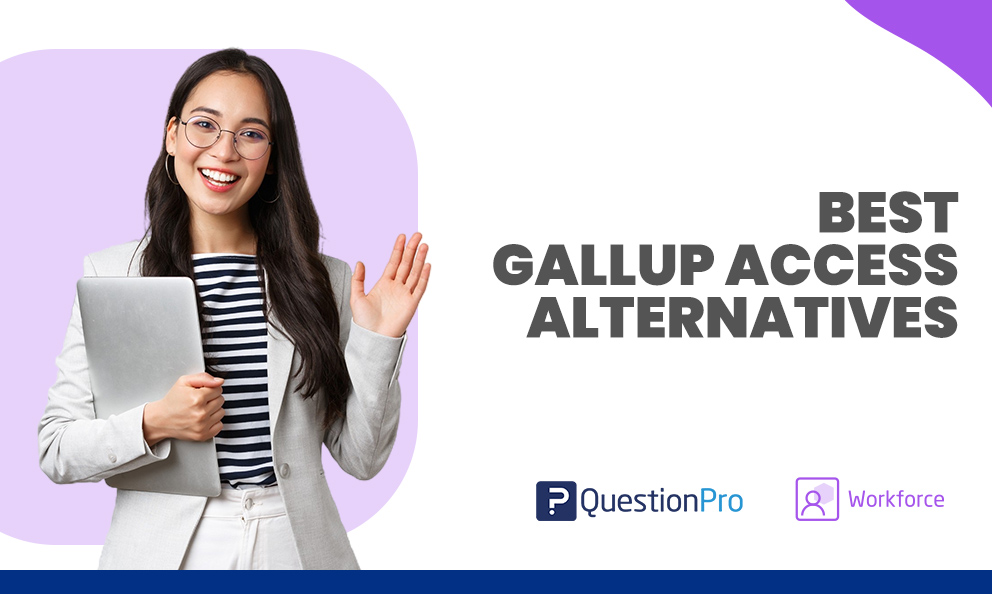
Best Gallup Access Alternatives & Competitors in 2024
Sep 6, 2024
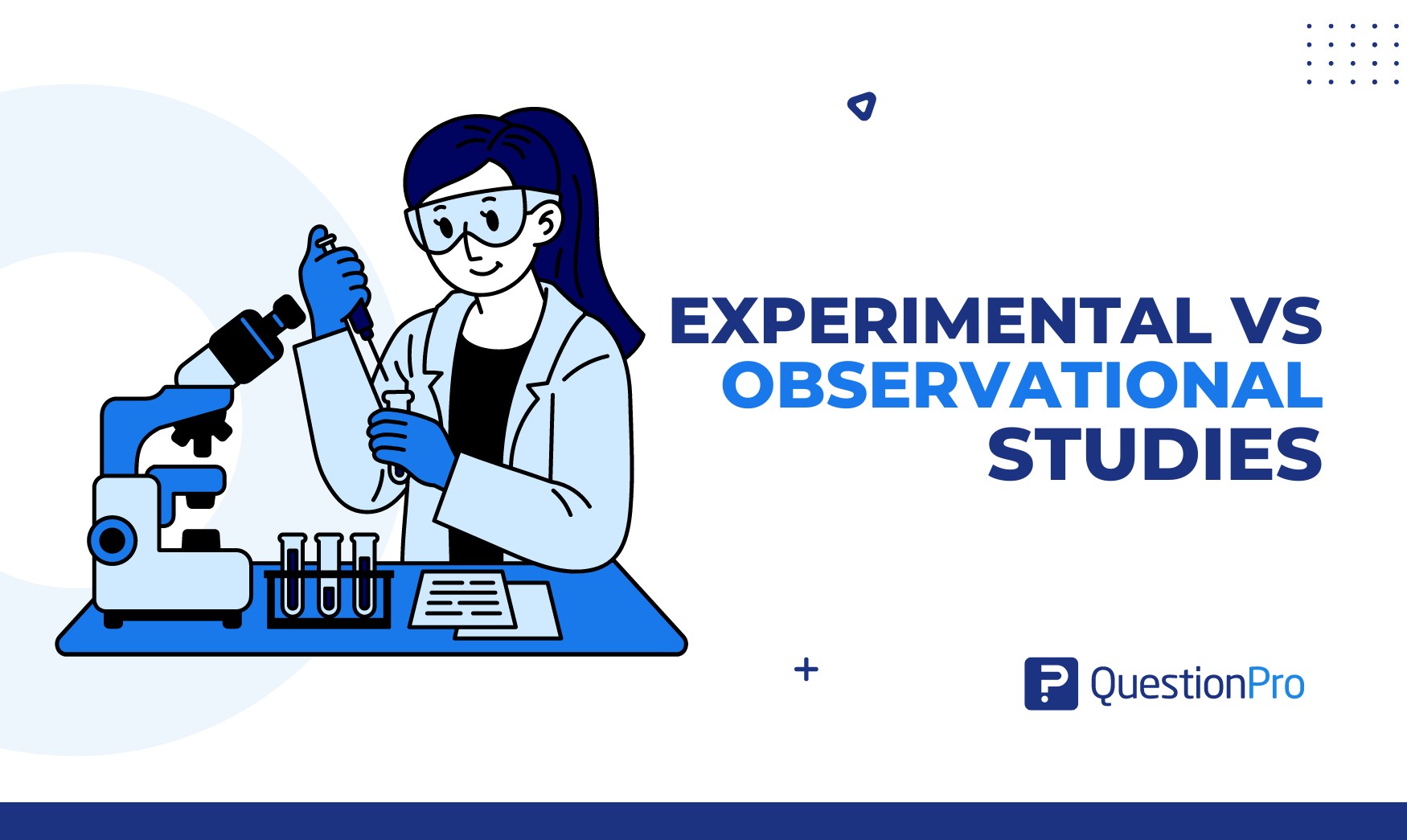
Experimental vs Observational Studies: Differences & Examples
Sep 5, 2024
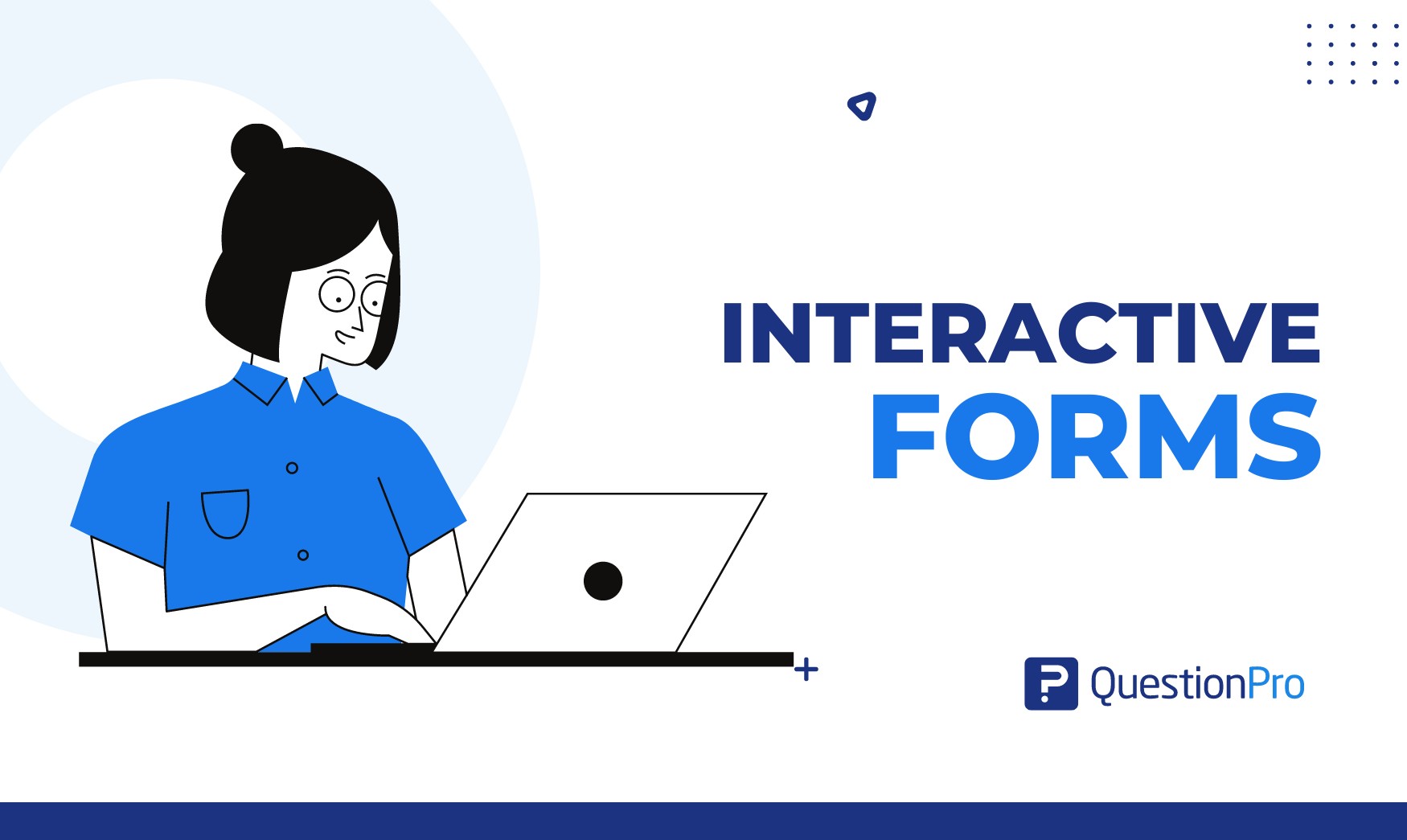
Interactive Forms: Key Features, Benefits, Uses + Design Tips
Sep 4, 2024
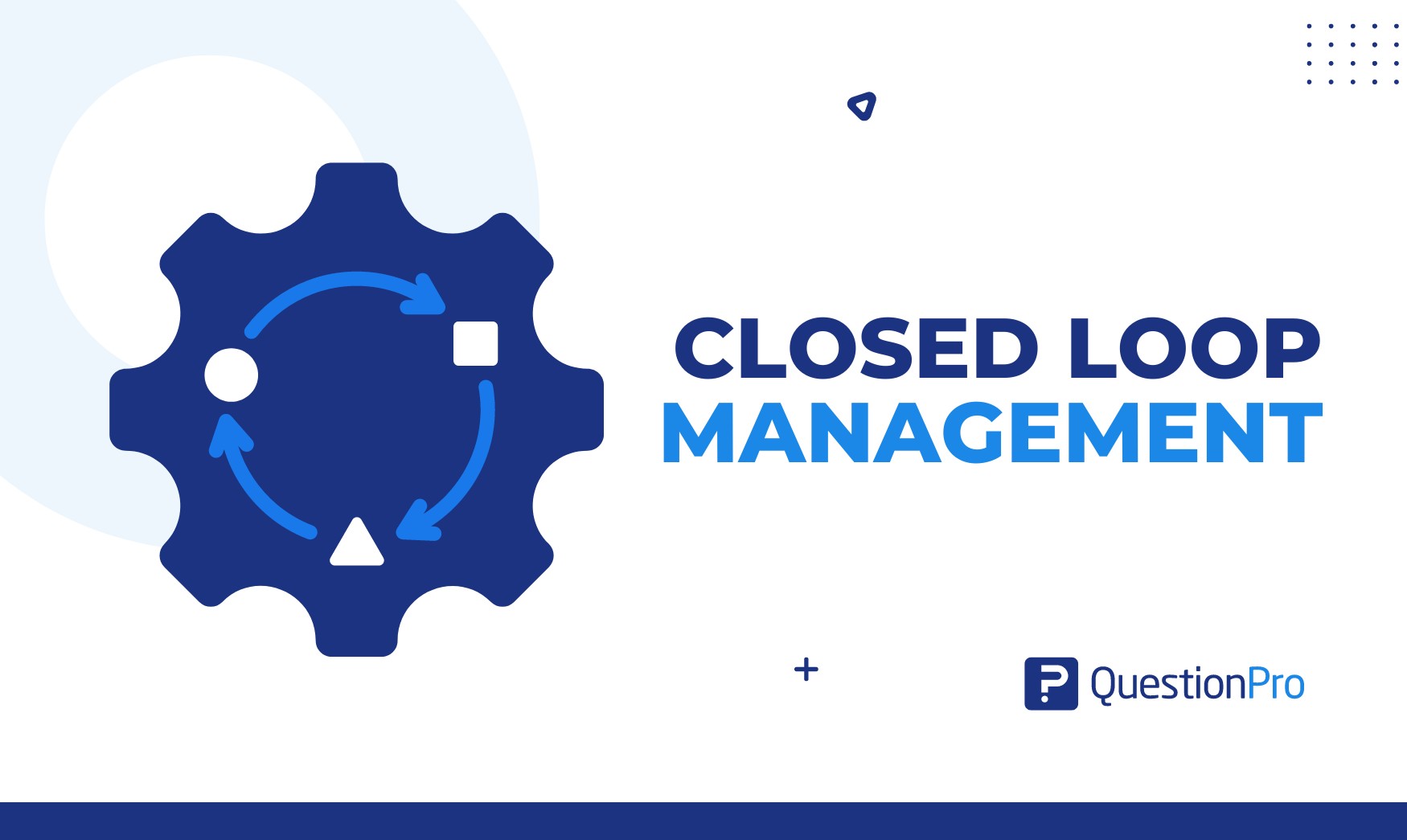
Closed-Loop Management: The Key to Customer Centricity
Sep 3, 2024
Other categories
- Academic Research
- Artificial Intelligence
- Assessments
- Brand Awareness
- Case Studies
- Communities
- Consumer Insights
- Customer effort score
- Customer Engagement
- Customer Experience
- Customer Loyalty
- Customer Research
- Customer Satisfaction
- Employee Benefits
- Employee Engagement
- Employee Retention
- Friday Five
- General Data Protection Regulation
- Insights Hub
- Life@QuestionPro
- Market Research
- Mobile diaries
- Mobile Surveys
- New Features
- Online Communities
- Question Types
- Questionnaire
- QuestionPro Products
- Release Notes
- Research Tools and Apps
- Revenue at Risk
- Survey Templates
- Training Tips
- Tuesday CX Thoughts (TCXT)
- Uncategorized
- What’s Coming Up
- Workforce Intelligence
What are Analytical Study Designs?
- Research Process
- Peer Review
Analytical study designs can be experimental or observational and each type has its own features. In this article, you'll learn the main types of designs and how to figure out which one you'll need for your study.
Updated on September 19, 2022

A study design is critical to your research study because it determines exactly how you will collect and analyze your data. If your study aims to study the relationship between two variables, then an analytical study design is the right choice.
But how do you know which type of analytical study design is best for your specific research question? It's necessary to have a clear plan before you begin data collection. Lots of researchers, sadly, speed through this or don't do it at all.
When are analytical study designs used?
A study design is a systematic plan, developed so you can carry out your research study effectively and efficiently. Having a design is important because it will determine the right methodologies for your study. Using the right study design makes your results more credible, valid, and coherent.
Descriptive vs. analytical studies
Study designs can be broadly divided into either descriptive or analytical.
Descriptive studies describe characteristics such as patterns or trends. They answer the questions of what, who, where, and when, and they generate hypotheses. They include case reports and qualitative studies.
Analytical study designs quantify a relationship between different variables. They answer the questions of why and how. They're used to test hypotheses and make predictions.
Experimental and observational
Analytical study designs can be either experimental or observational. In experimental studies, researchers manipulate something in a population of interest and examine its effects. These designs are used to establish a causal link between two variables.
In observational studies, in contrast, researchers observe the effects of a treatment or intervention without manipulating anything. Observational studies are most often used to study larger patterns over longer periods.
Experimental study designs
Experimental study designs are when a researcher introduces a change in one group and not in another. Typically, these are used when researchers are interested in the effects of this change on some outcome. It's important to try to ensure that both groups are equivalent at baseline to make sure that any differences that arise are from any introduced change.
In one study, Reiner and colleagues studied the effects of a mindfulness intervention on pain perception . The researchers randomly assigned participants into an experimental group that received a mindfulness training program for two weeks. The rest of the participants were placed in a control group that did not receive the intervention.
Experimental studies help us establish causality. This is critical in science because we want to know whether one variable leads to a change, or causes another. Establishing causality leads to higher internal validity and makes results reproducible.
Experimental designs include randomized control trials (RCTs), nonrandomized control trials (non-RCTs), and crossover designs. Read on to learn the differences.
Randomized control trials
In an RCT, one group of individuals receives an intervention or a treatment, while another does not. It's then possible to investigate what happens to the participants in each group.
Another important feature of RCTs is that participants are randomly assigned to study groups. This helps to limit certain biases and retain better control. Randomization also lets researchers pinpoint any differences in outcomes to the intervention received during the trial. RTCs are considered the gold standard in biomedical research and are considered to provide the best kind of evidence.
For example, one RCT looked at whether an exercise intervention impacts depression . Researchers randomly placed patients with depressive symptoms into intervention groups containing different types of exercise (i.e., light, moderate, or strong). Another group received usual medications or no exercise interventions.
Results showed that after the 12-week trial, patients in all exercise groups had decreased depression levels compared to the control group. This means that by using an RCT design, researchers can now safely assume that the exercise variable has a positive impact on depression.
However, RCTs are not without drawbacks. In the example above, we don't know if exercise still has a positive impact on depression in the long term. This is because it's not feasible to keep people under these controlled settings for a long time.
Advantages of RCTs
- It is possible to infer causality
- Everything is properly controlled, so very little is left to chance or bias
- Can be certain that any difference is coming from the intervention
Disadvantages of RCTs
- Expensive and can be time-consuming
- Can take years for results to be available
- Cannot be done for certain types of questions due to ethical reasons, such as asking participants to undergo harmful treatment
- Limited in how many participants researchers can adequately manage in one study or trial
- Not feasible for people to live under controlled conditions for a long time
Nonrandomized controlled trials
Nonrandomized controlled trials are a type of nonrandomized controlled studies (NRS) where the allocation of participants to intervention groups is not done randomly . Here, researchers purposely assign some participants to one group and others to another group based on certain features. Alternatively, participants can sometimes also decide which group they want to be in.
For example, in one study, clinicians were interested in the impact of stroke recovery after being in an enriched versus non-enriched hospital environment . Patients were selected for the trial if they fulfilled certain requirements common to stroke recovery. Then, the intervention group was given access to an enriched environment (i.e. internet access, reading, going outside), and another group was not. Results showed that the enriched group performed better on cognitive tasks.
NRS are useful in medical research because they help study phenomena that would be difficult to measure with an RCT. However, one of their major drawbacks is that we cannot be sure if the intervention leads to the outcome. In the above example, we can't say for certain whether those patients improved after stroke because they were in the enriched environment or whether there were other variables at play.
Advantages of NRS's
- Good option when randomized control trials are not feasible
- More flexible than RCTs
Disadvantages of NRS's
- Can't be sure if the groups have underlying differences
- Introduces risk of bias and confounds
Crossover study
In a crossover design, each participant receives a sequence of different treatments. Crossover designs can be applied to RCTs, in which each participant is randomly assigned to different study groups.
For example, one study looked at the effects of replacing butter with margarine on lipoproteins levels in individuals with cholesterol . Patients were randomly assigned to a 6-week butter diet, followed by a 6-week margarine diet. In between both diets, participants ate a normal diet for 5 weeks.
These designs are helpful because they reduce bias. In the example above, each participant completed both interventions, making them serve as their own control. However, we don't know if eating butter or margarine first leads to certain results in some subjects.
Advantages of crossover studies
- Each participant serves as their own control, reducing confounding variables
- Require fewer participants, so they have better statistical power
Disadvantages of crossover studies
- Susceptible to order effects, meaning the order in which a treatment was given may have an effect
- Carry-over effects between treatments
Observational studies
In observational studies, researchers watch (observe) the effects of a treatment or intervention without trying to change anything in the population. Observational studies help us establish broad trends and patterns in large-scale datasets or populations. They are also a great alternative when an experimental study is not an option.
Unlike experimental research, observational studies do not help us establish causality. This is because researchers do not actively control any variables. Rather, they investigate statistical relationships between them. Often this is done using a correlational approach.
For example, researchers would like to examine the effects of daily fiber intake on bone density . They conduct a large-scale survey of thousands of individuals to examine correlations of fiber intake with different health measures.
The main observational studies are case-control, cohort, and cross-sectional. Let's take a closer look at each one below.
Case-control study
A case-control is a type of observational design in which researchers identify individuals with an existing health situation (cases) and a similar group without the health issue (controls). The cases and the controls are then compared based on some measurements.
Frequently, data collection in a case-control study is retroactive (i.e., backwards in time). This is because participants have already been exposed to the event in question. Additionally, researchers must go through records and patient files to obtain the records for this study design.
For example, a group of researchers examined whether using sleeping pills puts people at risk of Alzheimer's disease . They selected 1976 individuals that received a dementia diagnosis (“cases”) with 7184 other individuals (“controls”). Cases and controls were matched on specific measures such as sex and age. Patient data was consulted to find out how much sleeping pills were consumed over the course of a certain time.
Case-control is ideal for situations where cases are easy to pick out and compare. For instance, in studying rare diseases or outbreaks.
Advantages of case-control studies
- Feasible for rare diseases
- Cheaper and easier to do than an RCT
Disadvantages of case-control studies
- Relies on patient records, which could be lost or damaged
- Potential recall and selection bias
Cohort study (longitudinal)
A cohort is a group of people who are linked in some way. For instance, a birth year cohort is all people born in a specific year. In cohort studies, researchers compare what happens to individuals in the cohort that have been exposed to some variable compared with those that haven't on different variables. They're also called longitudinal studies.
The cohort is then repeatedly assessed on variables of interest over a period of time. There is no set amount of time required for cohort studies. They can range from a few weeks to many years.
Cohort studies can be prospective. In this case, individuals are followed for some time into the future. They can also be retrospective, where data is collected on a cohort from records.
One of the longest cohort studies today is The Harvard Study of Adult Development . This cohort study has been tracking various health outcomes of 268 Harvard graduates and 456 poor individuals in Boston from 1939 to 2014. Physical screenings, blood samples, brain scans and surveys were collected on this cohort for over 70 years. This study has produced a wealth of knowledge on outcomes throughout life.
A cohort study design is a good option when you have a specific group of people you want to study over time. However, a major drawback is that they take a long time and lack control.
Advantages of cohort studies
- Ethically safe
- Allows you to study multiple outcome variables
- Establish trends and patterns
Disadvantages of cohort studies
- Time consuming and expensive
- Can take many years for results to be revealed
- Too many variables to manage
- Depending on length of study, can have many changes in research personnel
Cross-sectional study
Cross-sectional studies are also known as prevalence studies. They look at the relationship of specific variables in a population in one given time. In cross-sectional studies, the researcher does not try to manipulate any of the variables, just study them using statistical analyses. Cross-sectional studies are also called snapshots of a certain variable or time.
For example, researchers wanted to determine the prevalence of inappropriate antibiotic use to study the growing concern about antibiotic resistance. Participants completed a self-administered questionnaire assessing their knowledge and attitude toward antibiotic use. Then, researchers performed statistical analyses on their responses to determine the relationship between the variables.
Cross-sectional study designs are ideal when gathering initial data on a research question. This data can then be analyzed again later. By knowing the public's general attitudes towards antibiotics, this information can then be relayed to physicians or public health authorities. However, it's often difficult to determine how long these results stay true for.
Advantages of cross-sectional studies
- Fast and inexpensive
- Provides a great deal of information for a given time point
- Leaves room for secondary analysis
Disadvantages of cross-sectional studies
- Requires a large sample to be accurate
- Not clear how long results remain true for
- Do not provide information on causality
- Cannot be used to establish long-term trends because data is only for a given time
So, how about your next study?
Whether it's an RCT, a case-control, or even a qualitative study, AJE has services to help you at every step of the publication process. Get expert guidance and publish your work for the world to see.

The AJE Team
See our "Privacy Policy"

An official website of the United States government
Here’s how you know
Official websites use .gov A .gov website belongs to an official government organization in the United States.
Secure .gov websites use HTTPS A lock ( Lock A locked padlock ) or https:// means you’ve safely connected to the .gov website. Share sensitive information only on official, secure websites.
JavaScript appears to be disabled on this computer. Please click here to see any active alerts .
- Analytical Examples
This section presents five (5) examples illustrating the use of data analysis to support different types of evidence. Each example provides details about the analysis technique used and the type of evidence supported. These include:
- Spatial Co-occurrence with Regional Reference Sites (Example 1).
- Verified Prediction: Predicting Environmental Conditions from Biological Observations (Example 2).
- Stressor-Response Relationships from Field Observations (Example 3).
- Stressor-Response Relationships from Laboratory Studies (Example 4).
- Verified Prediction with Traits (Example 5).
Example 1. Spatial Co-occurrence with Regional Reference Sites
Introduction, analysis and results, how do i score this evidence.
We would like to determine whether stream temperatures observed at an Oregon test site are higher than those at regional reference sites. If temperatures at the test site are higher than reference expectations, then we can conclude that increased temperature spatially co-occurs with the observed impairment. Conversely, temperatures at the test site that are comparable to temperatures at regional reference sites would suggest that increased temperature does not spatially co-occur with the observed impairment.
Analytical Techniques Used
- Scatterplots
- Regression Analysis
Controlling for Natural Variability
Type of evidence supported.
- Spatial/Temporal Co-occurrence
The Oregon Department of Environment Quality (ORDEQ) deployed continuous temperature monitors in streams from 1997-2002. These temperature monitors recorded hourly temperature measurement which were then summarized as seven day average maximum temperatures in degrees C (7DAMT). Sites were also characterized by the geographic location (latitude and longitude), elevation, and catchment area. Reference sites were designated in Oregon based on land use characteristics.
Scatter plots are first used to examine the variation of stream temperature with different natural factors. The factors that are chosen (e.g., elevation, geographic location) must not be associated with local human activities. This initial data exploration suggests that stream temperature in reference sites are inversely related with both elevation and latitude (Figure 1). Next, regression analysis is used to model stream temperature as a function of elevation and latitude.

Both elevation and latitude are statistically significant (p < 0.05) predictors of stream temperature. The model explains approximately half of the overall variability in stream temperature. This model can be used to predict the reference expectations for stream temperature at other sites. That is, the reference expectation for temperature can be calculated as follows:
t = 76.6 - 0.0019E - 1.36L where t is the stream temperature, E is the elevation of the site in feet, and L is the latitude of the site in decimal degrees.
Now, suppose a biologically impaired test site of interest is located at a latitude of 43 degrees N and an elevation of 1000 ft. We monitored stream temperature at this site and found that the seven day average maximum temperature at the site was 22 °C. Temperature is listed as a candidate cause of impairment at this site, and so we would like to know whether stream temperature at the site is elevated relative to the regional reference conditions. The reference expectation for stream temperature can be predicted as follows,
t = 76.6 - 0.0019(1000) - 1.36(43) which gives a predicted reference temperature of 16.4 degrees. Most statistical software will also provide prediction intervals at a specified probability. For this case, 95% prediction intervals around the mean value are 11.4 and 21.4 degrees. Hence, the observed temperature is greater than temperatures we would expect for 95% of reference samples collected at the same elevation and latitude, suggesting that stream temperature is indeed elevated at the test site. We would conclude that at this test site, elevated stream temperature co-occurs with the biological impairment.
The CADStat Regression Prediction tool performs all of these calculations, and also determines whether conditions at test sites are within the range of experience of the set of reference sites.
Elevated temperatures co-occurs with the biological impairment so we would score this evidence as +.
Example 2. Verified Prediction: Predicting Environmental Conditions from Biological Observations
Inference model development and validation, site assessment.
We would like to determine whether observed changes in the macroinvertebrate assemblage composition at a test site in Oregon is consistent with a hypothesis that temperature has increased at the site. That is, if increased temperature is a stressor at the test site, we predict that the temperature inferred from the impaired macroinvertebrate assemblage is higher than expected. For this example, we establish our expectations for the inferred temperature using a set of regional reference sites.
- Predicting Environmental Conditions from Biological Observations
- Verified Predictions
Macroinvertebrate samples and temperature measurements were collected from small streams across the western United States by the U.S. EPA Environmental Monitoring and Assessment Program.
The Oregon Department of Environment Quality (ORDEQ) deployed continuous temperature monitors in streams from 1997-2002. These temperature monitors recorded hourly temperature measurements that were summarized as seven-day average maximum temperatures (7DAMT). Macroinvertebrate samples were also collected from these sites. Sites were characterized by the geographic location (latitude and longitude), elevation, and catchment area. Reference sites were designated in Oregon based on land use characteristics.

The accuracy with which the EMAP models predicted Oregon stream temperatures was assessed by plotting temperature inferred from the macroinvertebrate assemblage versus directly measured mean temperature (Figure 2). Agreement between inferred and directly measured temperatures was strong.
- See Spatial Co-occurrence with Regional Reference Sites
The factors that are chosen for the predictive model (e.g., elevation, geographic location) must not be associated with human activities. This initial data exploration suggested that stream temperature in reference sites varies with both elevation and latitude (Figure 3).

where t i is the stream temperature, E is the elevation of the site in feet, and L is the latitude of the site in decimal degrees.
Since the inference model seemed to provide accurate predictions of stream temperature, inferred temperature can be used to inform the verified prediction type of evidence. That is, we hypothesize that if temperature is the cause of impairment then temperatures inferred from the impaired macroinvertebrate assemblage will be higher than expected.
At the biologically impaired test site of interest we collected a macroinvertebrate sample and used the EMAP inference models to infer temperature at the test site as 21°C based on the macroinvertebrate assemblage. The biologically impaired site is located at an elevation of 1000 feet and latitude of 43° North. The expected inferred stream temperature at the site is predicted using the regression relationship developed from regional reference conditions,
t i = 50.3 - 0.0013 (1000) - 0.82( 43)
which gives a predicted reference inferred temperature of 13.7°C. 95% prediction intervals around this mean value are 10.5°C and 17.2°C, so the EMAP inferred temperature of 21°C, based on the collected macroinvertebrate assemblage, is well outside the predicted range of 95% of inferred temperatures at similar reference sites. This finding suggests that inferred stream temperature is indeed elevated at the test site. Hence, the macroinvertebrate assemblage at the test site is one that is characteristic of much warmer streams than we would expect for a stream at this elevation and latitude. At this test site, we have verified our prediction that the observed macroinvertebrate assemblage is consistent with temperatures being higher than expected.
The CADStat PECBO and Regression Prediction tools perform all the calculations described in this example.
Predictions of increased biologically-inferred temperatures have been verified (+).
- Yuan LL (2007) Maximum likelihood method for predicting environmental conditions from assemblage composition: The R package bio.infer. Journal of Statistical Software 22: Article 3.
Example 3. Stressor-Response Relationships from Field Observations
We would like to determine whether water quality variables in Long Creek, Maine (U.S. EPA 2007) are associated with three observed changes in the aquatic invertebrate community relative to the reference stream: a decrease in Ephemeroptera, Plecoptera and Trichoptera (EPT) richness; an increase in percent non-insect taxa; and a shift towards increased pollution tolerance, estimated using Hilsenhoff's Biotic Index (HBI) (Hilsenhoff 1987, 1988).
- Causal Analysis of Biological Impairment in Long Creek
- Correlation Analysis
- Stressor-Response Relationships from the Field
In this example, we present analyses relevant to two candidate causes, ionic strength (measured using specific conductivity), and zinc. If specific conductivity (or zinc) is not associated with the biological responses in the expected direction, this evidence would weaken the argument for ionic strength (or zinc) being a cause of the observed biological changes. Conversely, if specific conductivity (or zinc) is associated with the biological responses in the expected direction, this evidence would somewhat support the argument that ionic strength (or zinc) is the cause of the observed changes.
These associations can provide only weak support for a causal argument because other stressors may be correlated with increased conductivity (or zinc), and are not controlled for in this analysis. For this reason, it is important to conduct this analysis for as many of the candidate causes as possible.
Biological and water chemistry data from 8 sites along Long Creek and a similar but unimpaired reference stream, are used in this example.
Biological metrics were calculated from macroinvertebrate rockbag samples deployed throughout the study area beginning August 5-6, 1999, for a period of 32 days, following standard Maine Department of Environmental Protection (MEDEP) protocol (Davies and Tsomides 2002).
Water chemistry measurements of conductivity and zinc were made from baseflow water samples collected by MEDEP on three days in August 2000. Methods and analyses are described in MEDEP (2002). Here, the analysts assume that the differences in the collection dates for biological samples (1999) and for water chemistry samples (2000) did not affect observed relationships. Ideally, additional data would be collected as a follow-up to validate this assumption.
The data were analyzed using scatter plots (Figure 4). The project team interpreted the scatter plots by looking for linear and curvilinear trends in the data. Because only one data point from each site was available, the plots were not used to make judgments about individual sites or stream reaches. Instead, the plots were used to characterize trends across the two watersheds.
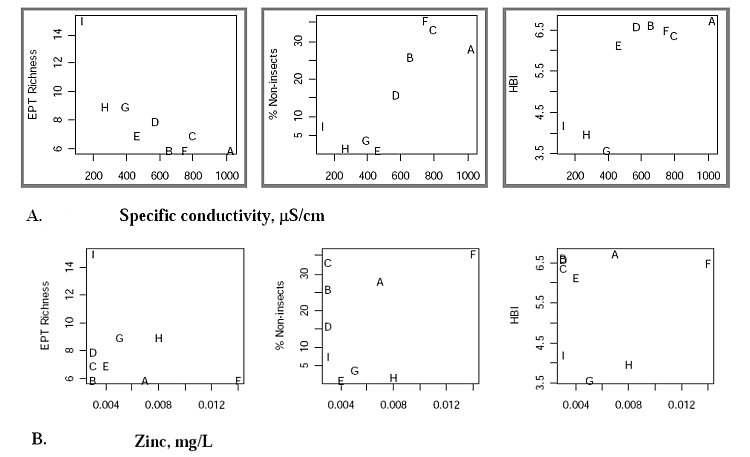
The visual interpretation of the scatterplots was supplemented with correlation coefficients (Table 1). Correlation coefficients were not evaluated for significance because of the small sample size and pseudo-replication of sites. Rather, consistent correlations of relatively large magnitude for all three biological responses were considered by the analysts to provide some support for ionic strength as a candidate cause. When evaluating this evidence, it is worth noting again that both analyses hinge on the assumption that samples of water chemistry taken in August 2000 are similar to exposures experienced by organisms in August 1999.
Specific conductivity | Zinc | |
---|---|---|
EPT Richness | -0.86 | -0.21 |
% non-insects | 0.78 | 0.026 |
HBI | 0.78 | -0.15 |
Associations between specific conductivity and all three biological responses were apparent and in the expected direction. We would score this evidence as + for each of the biological responses.
There were no clear associations between zinc and any of the three biological responses. We would score this evidence as - for each of the biological responses.
- Davies SP, Tsomides L (2002) Methods for biological sampling and analysis of Maine's rivers and streams . Maine Department of Environmental Protection, Augusta ME. DEP LW0387-B2002.
- Hilsenhoff WL (1987) An improved biotic index of organic stream pollution. Great Lakes Entomologist 20:31-39.
- Hilsenhoff WL (1988) Rapid field assessment of organic pollution with a family level biotic index. Journal of the North American Benthological Society 7(1):65-68.
- MEDEP (2002) A biological, physical, and chemical assessment of two urban streams in southern Maine: Long Creek and Red Brook. Maine Department of Environmental Protection, Augusta ME. DEP-LW0572.
- U.S. EPA (2007) Causal Analysis of Biological Impairment in Long Creek: A Sandy-Bottomed Stream in Coastal Southern Maine . U.S. Environmental Protection Agency, Office of Research and Development, National Center for Environmental Assessment, Washington DC. EPA-600-R-06-065F.
Example 4. Stress or-Response Relationships from Laboratory Studies
Laboratory toxicity data.
In this example, we ask whether organisms in Long Creek, Maine (U.S. EPA 2007) are exposed to a candidate cause (zinc) at quantities or frequencies sufficient to induce observed biological effects. We use results from laboratory studies to evaluate whether zinc in the water column under base flow conditions reached concentrations that could explain the observed decrease in Ephemeroptera, Plecoptera and Trichoptera (EPT) richness. The comparison of laboratory and field data can be performed in two ways.
- Most commonly, effective concentrations from laboratory data are compared to ambient concentrations at the affected site. If zinc concentrations associated with similar types of effects in the laboratory are similar to or lower than concentrations that have been shown to occur at the affected site, this would provide evidence that zinc concentrations are high enough to cause the effects.
- Species Sensitivity Distributions
- Stressor-Response Relationships from Laboratory Studies
Conversely, if zinc concentrations associated with similar types of effects in the laboratory are much higher than those at the affected site, then the case for zinc would be weakened. Either some other stressor is the cause of the observed decline, or zinc is acting jointly with another cause to produce the effect.
- We can also compare the magnitude of effects observed at the site with the magnitude of effects observed in the laboratory at concentrations equal to ambient concentrations. If the magnitude of effects at the site are much greater than would be predicted from the laboratory concentration-response relationship, then we would conclude that either zinc concentrations are not high enough to have caused the effects, or the laboratory organisms or endpoints are not as sensitive as the organisms or responses at the affected site. If magnitude of effects observed at the site is approximately equal to those predicted from the laboratory concentration-response relationship, then this would support the argument that zinc is the cause of the effects. Finally, if the magnitude of effects observed at the site is much less than predicted from laboratory studies, we would conclude that some physical factor (e.g., dissolved organic matter) or some biological process (e.g., replacement of sensitive insect species by tolerant species) may be reducing the effect in the field.
This example uses summaries of laboratory toxicity test results and compares these summaries with data from the site.
Two approaches were used to summarize laboratory results. First, U.S. EPA's chronic criterion value for zinc was used to represent sublethal effects and effects of extended exposures. The chronic criterion value for zinc at 100 mg/L hardness (as CaCO 3 ) is 0.12 mg/L . A chronic value for an EPT insect would be preferable, but none were available.
- ECOTOX Database
It was necessary to generate SSDs with data for total metals because greater than 90% of freshwater metals data in ECOTOX are reported as total metals. Free ion or dissolved metal concentrations would be more appropriate indicators of actual toxic exposure and be more relevant to the dissolved metal concentrations reported for Long Creek. However, this is a relatively minor problem, because nearly all metals in laboratory tests are dissolved.
SSDs were generated using LC 50 data. Since an LC 50 is a concentration that kills half of the organisms in a test population, one would expect to observe a reduction in the abundance of some species when water concentrations equal the LC 50 for that species. Data used in generating SSDs do not represent specific species present at the study area. Toxicity data are generally not available for site-specific taxa due to the diversity of species occurring in the wild and the need to perform toxicity tests with well characterized organisms.
Biological and water chemistry data from two sites along Long Creek are used in this example. EPT richness was calculated from macroinvertebrate rockbag samples deployed throughout the study area beginning August 5-6 1999, following standard Maine Department of Environmental Protection (MEDEP) protocol (Davies and Tsomides 2002).
Baseflow water samples were collected by MEDEP on three days in August 2000. Methods and analyses are described in MEDEP (2002).
The laboratory results were compared to site data by graphically comparing the proportion of decrease in EPT richness, relative to the reference site, and impaired site zinc concentrations. In addition, the SSD was used to identify 0.087 as a benchmark concentration of 10% at which 10% of species would be expected to experience lethal effects.
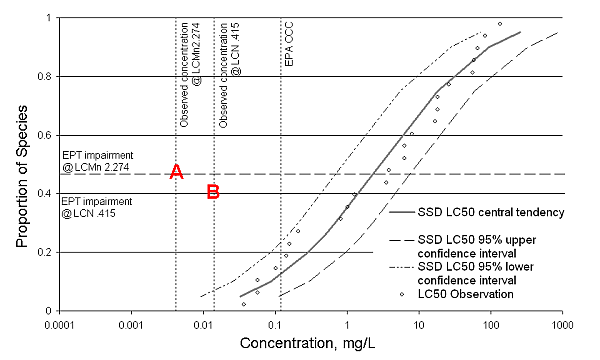
- The organisms and endpoints measured in the laboratory are relevant to EPT richness.
- The laboratory exposures are relevant to the exposures encountered by organisms in the field.
- Measured baseflow concentrations of zinc in August 2000 were similar to unmeasured concentrations in August 1999.
How do I score this evidence?
Measured concentrations are all below the EPA criterion continuous concentration. The measured concentrations at the site fall below the 10% benchmark derived from the SSD. Points corresponding to the observed impairment occur at concentrations below the lower confidence limits on the SSD curve. This weakens the case that zinc caused the observed decreases in EPT, giving a score of - (one minus).
- Davies SP, Tsomides L (2002) Methods for biological sampling and analysis of Maine's rivers and streams . Maine Department of Environmental Protection, Augusta ME. DEP LW0387-B2002.
- U.S. EPA (2007) Causal Analysis of Biological Impairment in Long Creek: A Sandy-Bottomed Stream in Coastal Southern Maine . U.S. Environmental Protection Agency, Office of Research and Development, National Center for Environmental Assessment, Washington DC. EPA-600-R-06-065F.
Example 5. Verified Prediction with Traits
In causal analysis we find that trait information is well suited to a type of evidence called verified prediction, where the knowledge of a cause's mode of action permits prediction and subsequent confirmation of previously unobserved effects. In this application, we would predict changes in the occurrence of different traits we would expect to occur if a particular stressor was present and causing biological effects. If we found that these traits do indeed occur at the impaired site, our prediction is verified and the causal hypothesis is supported by that evidence.
Analytical approaches range from basic comparisons of measurements to more formal statistical tests (see page on establishing differences from expectations). Incorporating predictions of traits into causal analysis is an area of active research, and so we present a hypothetical example below.
Existing information about the relationship between a trait and environmental gradients can be used to predict how the occurrence of a trait will differ between the test site and reference expectations. The occurrence of a trait in a community from test site is compared with a community from a reference site. If the predicted occurance of a is supported, the result would support a claim of verified prediction.
We illustrate this with an example of clinger relative richness and sediment in streams across the eastern United States. Existing literature indicates that the relative richness of clingers decreases with increased bedded sediment (Figure 5, Pollard and Yuan 2010).
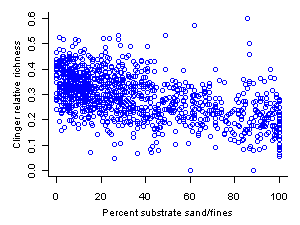
- See Interpreting Statistics to determine the confidence intervals
If the predicted pattern is observed (here, if the test site had fewer clingers than the reference site), the type of evidence "verified prediction" is scored as supported (+). If multiple predictions were verified or if the predictions were highly specific, the evidence may be convincing (+++).
- Abell R, Thieme ML, Revenga C, Bryer M, Kottelat M, Bogutskaya N, Coad B, Mandrak N, Balderas SC, Bussing W, Stiassny MLJ, Skelton P, Allen GR, Unmack P, Naseka A, Ng R, Sindorf N, Robertson J, Armijo E, Higgins JV, Heibel TJ, Wikramanayake E, Olson D, Lopez HL, Reis RE, Lundberg JG, Sabaj Perez MH, Petry P (2009) Freshwater ecoregions of the world: a new map of biogeographic units for freshwater biodiversity conservation. BioScience 58:403-414.
- Pollard AI, Yuan LL (2010) Assessing the consistency of response metrics of the invertebrate benthos: a comparison of trait- and identity-based measures. Freshwater Biology 55:1420-1429.
- CADDIS Home
- Volume 1: Stressor Identification
- Volume 2: Sources, Stressors and Responses
- Worksheet Examples
- State Examples
- Case Studies
- Volume 4: Data Analysis
- Volume 5: Causal Databases
Analytical vs. Descriptive
What's the difference.
Analytical and descriptive are two different approaches used in various fields of study. Analytical refers to the process of breaking down complex ideas or concepts into smaller components to understand their underlying principles or relationships. It involves critical thinking, logical reasoning, and the use of evidence to support arguments or conclusions. On the other hand, descriptive focuses on providing a detailed account or description of a particular phenomenon or event. It aims to present facts, observations, or characteristics without any interpretation or analysis. While analytical aims to uncover the "why" or "how" behind something, descriptive aims to provide a comprehensive picture of what is being studied. Both approaches have their own merits and are often used in combination to gain a deeper understanding of a subject matter.
Attribute | Analytical | Descriptive |
---|---|---|
Definition | Focuses on breaking down complex problems into smaller components and analyzing them individually. | Focuses on describing and summarizing data or phenomena without attempting to explain or analyze them. |
Goal | To understand the underlying causes, relationships, and patterns in data or phenomena. | To provide an accurate and objective description of data or phenomena. |
Approach | Uses logical reasoning, critical thinking, and data analysis techniques. | Relies on observation, measurement, and data collection. |
Focus | Emphasizes on the "why" and "how" questions. | Emphasizes on the "what" questions. |
Subjectivity | Objective approach, minimizing personal bias. | Subjective approach, influenced by personal interpretation. |
Examples | Statistical analysis, data mining, hypothesis testing. | Surveys, observations, case studies. |
Further Detail
Introduction.
When it comes to research and data analysis, two common approaches are analytical and descriptive methods. Both methods have their own unique attributes and serve different purposes in understanding and interpreting data. In this article, we will explore the characteristics of analytical and descriptive approaches, highlighting their strengths and limitations.
Analytical Approach
The analytical approach focuses on breaking down complex problems or datasets into smaller components to gain a deeper understanding of the underlying patterns and relationships. It involves the use of logical reasoning, critical thinking, and statistical techniques to examine data and draw conclusions. The primary goal of the analytical approach is to uncover insights, identify trends, and make predictions based on the available information.
One of the key attributes of the analytical approach is its emphasis on hypothesis testing. Researchers using this method formulate hypotheses based on existing theories or observations and then collect and analyze data to either support or refute these hypotheses. By systematically testing different variables and their relationships, the analytical approach allows researchers to make evidence-based claims and draw reliable conclusions.
Another important attribute of the analytical approach is its reliance on quantitative data. This method often involves the use of statistical tools and techniques to analyze numerical data, such as surveys, experiments, or large datasets. By quantifying variables and measuring their relationships, the analytical approach provides a rigorous and objective framework for data analysis.
Furthermore, the analytical approach is characterized by its focus on generalizability. Researchers using this method aim to draw conclusions that can be applied to a broader population or context. By using representative samples and statistical inference, the analytical approach allows researchers to make inferences about the larger population based on the analyzed data.
However, it is important to note that the analytical approach has its limitations. It may overlook important contextual factors or qualitative aspects of the data that cannot be easily quantified. Additionally, the analytical approach requires a strong understanding of statistical concepts and techniques, making it more suitable for researchers with a background in quantitative analysis.
Descriptive Approach
The descriptive approach, on the other hand, focuses on summarizing and presenting data in a meaningful and informative way. It aims to provide a clear and concise description of the observed phenomena or variables without necessarily seeking to establish causal relationships or make predictions. The primary goal of the descriptive approach is to present data in a manner that is easily understandable and interpretable.
One of the key attributes of the descriptive approach is its emphasis on data visualization. Researchers using this method often employ charts, graphs, and other visual representations to present data in a visually appealing and accessible manner. By using visual aids, the descriptive approach allows for quick and intuitive understanding of the data, making it suitable for a wide range of audiences.
Another important attribute of the descriptive approach is its flexibility in dealing with different types of data. Unlike the analytical approach, which primarily focuses on quantitative data, the descriptive approach can handle both quantitative and qualitative data. This makes it particularly useful in fields where subjective opinions, narratives, or observations play a significant role.
Furthermore, the descriptive approach is characterized by its attention to detail. Researchers using this method often provide comprehensive descriptions of the variables, including their distribution, central tendency, and variability. By presenting detailed summaries, the descriptive approach allows for a thorough understanding of the data, enabling researchers to identify patterns or trends that may not be immediately apparent.
However, it is important to acknowledge that the descriptive approach has its limitations as well. It may lack the rigor and statistical power of the analytical approach, as it does not involve hypothesis testing or inferential statistics. Additionally, the descriptive approach may be more subjective, as the interpretation of the data relies heavily on the researcher's judgment and perspective.
In conclusion, the analytical and descriptive approaches have distinct attributes that make them suitable for different research purposes. The analytical approach emphasizes hypothesis testing, quantitative data analysis, and generalizability, allowing researchers to draw evidence-based conclusions and make predictions. On the other hand, the descriptive approach focuses on data visualization, flexibility in handling different data types, and attention to detail, enabling researchers to present data in a clear and concise manner. Both approaches have their strengths and limitations, and the choice between them depends on the research objectives, available data, and the researcher's expertise. By understanding the attributes of each approach, researchers can make informed decisions and employ the most appropriate method for their specific research needs.
Comparisons may contain inaccurate information about people, places, or facts. Please report any issues.
- Privacy Policy

Home » Descriptive Analytics – Methods, Tools and Examples
Descriptive Analytics – Methods, Tools and Examples
Table of Contents

Descriptive Analytics
Definition:
Descriptive analytics focused on describing or summarizing raw data and making it interpretable. This type of analytics provides insight into what has happened in the past. It involves the analysis of historical data to identify patterns, trends, and insights. Descriptive analytics often uses visualization tools to represent the data in a way that is easy to interpret.
Descriptive Analytics in Research
Descriptive analytics plays a crucial role in research, helping investigators understand and describe the data collected in their studies. Here’s how descriptive analytics is typically used in a research setting:
- Descriptive Statistics: In research, descriptive analytics often takes the form of descriptive statistics . This includes calculating measures of central tendency (like mean, median, and mode), measures of dispersion (like range, variance, and standard deviation), and measures of frequency (like count, percent, and frequency). These calculations help researchers summarize and understand their data.
- Visualizing Data: Descriptive analytics also involves creating visual representations of data to better understand and communicate research findings . This might involve creating bar graphs, line graphs, pie charts, scatter plots, box plots, and other visualizations.
- Exploratory Data Analysis: Before conducting any formal statistical tests, researchers often conduct an exploratory data analysis, which is a form of descriptive analytics. This might involve looking at distributions of variables, checking for outliers, and exploring relationships between variables.
- Initial Findings: Descriptive analytics are often reported in the results section of a research study to provide readers with an overview of the data. For example, a researcher might report average scores, demographic breakdowns, or the percentage of participants who endorsed each response on a survey.
- Establishing Patterns and Relationships: Descriptive analytics helps in identifying patterns, trends, or relationships in the data, which can guide subsequent analysis or future research. For instance, researchers might look at the correlation between variables as a part of descriptive analytics.
Descriptive Analytics Techniques
Descriptive analytics involves a variety of techniques to summarize, interpret, and visualize historical data. Some commonly used techniques include:
Statistical Analysis
This includes basic statistical methods like mean, median, mode (central tendency), standard deviation, variance (dispersion), correlation, and regression (relationships between variables).
Data Aggregation
It is the process of compiling and summarizing data to obtain a general perspective. It can involve methods like sum, count, average, min, max, etc., often applied to a group of data.
Data Mining
This involves analyzing large volumes of data to discover patterns, trends, and insights. Techniques used in data mining can include clustering (grouping similar data), classification (assigning data into categories), association rules (finding relationships between variables), and anomaly detection (identifying outliers).
Data Visualization
This involves presenting data in a graphical or pictorial format to provide clear and easy understanding of the data patterns, trends, and insights. Common data visualization methods include bar charts, line graphs, pie charts, scatter plots, histograms, and more complex forms like heat maps and interactive dashboards.
This involves organizing data into informational summaries to monitor how different areas of a business are performing. Reports can be generated manually or automatically and can be presented in tables, graphs, or dashboards.
Cross-tabulation (or Pivot Tables)
It involves displaying the relationship between two or more variables in a tabular form. It can provide a deeper understanding of the data by allowing comparisons and revealing patterns and correlations that may not be readily apparent in raw data.
Descriptive Modeling
Some techniques use complex algorithms to interpret data. Examples include decision tree analysis, which provides a graphical representation of decision-making situations, and neural networks, which are used to identify correlations and patterns in large data sets.
Descriptive Analytics Tools
Some common Descriptive Analytics Tools are as follows:
Excel: Microsoft Excel is a widely used tool that can be used for simple descriptive analytics. It has powerful statistical and data visualization capabilities. Pivot tables are a particularly useful feature for summarizing and analyzing large data sets.
Tableau: Tableau is a data visualization tool that is used to represent data in a graphical or pictorial format. It can handle large data sets and allows for real-time data analysis.
Power BI: Power BI, another product from Microsoft, is a business analytics tool that provides interactive visualizations with self-service business intelligence capabilities.
QlikView: QlikView is a data visualization and discovery tool. It allows users to analyze data and use this data to support decision-making.
SAS: SAS is a software suite that can mine, alter, manage and retrieve data from a variety of sources and perform statistical analysis on it.
SPSS: SPSS (Statistical Package for the Social Sciences) is a software package used for statistical analysis. It’s widely used in social sciences research but also in other industries.
Google Analytics: For web data, Google Analytics is a popular tool. It allows businesses to analyze in-depth detail about the visitors on their website, providing valuable insights that can help shape the success strategy of a business.
R and Python: Both are programming languages that have robust capabilities for statistical analysis and data visualization. With packages like pandas, matplotlib, seaborn in Python and ggplot2, dplyr in R, these languages are powerful tools for descriptive analytics.
Looker: Looker is a modern data platform that can take data from any database and let you start exploring and visualizing.
When to use Descriptive Analytics
Descriptive analytics forms the base of the data analysis workflow and is typically the first step in understanding your business or organization’s data. Here are some situations when you might use descriptive analytics:
Understanding Past Behavior: Descriptive analytics is essential for understanding what has happened in the past. If you need to understand past sales trends, customer behavior, or operational performance, descriptive analytics is the tool you’d use.
Reporting Key Metrics: Descriptive analytics is used to establish and report key performance indicators (KPIs). It can help in tracking and presenting these KPIs in dashboards or regular reports.
Identifying Patterns and Trends: If you need to identify patterns or trends in your data, descriptive analytics can provide these insights. This might include identifying seasonality in sales data, understanding peak operational times, or spotting trends in customer behavior.
Informing Business Decisions: The insights provided by descriptive analytics can inform business strategy and decision-making. By understanding what has happened in the past, you can make more informed decisions about what steps to take in the future.
Benchmarking Performance: Descriptive analytics can be used to compare current performance against historical data. This can be used for benchmarking and setting performance goals.
Auditing and Regulatory Compliance: In sectors where compliance and auditing are essential, descriptive analytics can provide the necessary data and trends over specific periods.
Initial Data Exploration: When you first acquire a dataset, descriptive analytics is useful to understand the structure of the data, the relationships between variables, and any apparent anomalies or outliers.
Examples of Descriptive Analytics
Examples of Descriptive Analytics are as follows:
Retail Industry: A retail company might use descriptive analytics to analyze sales data from the past year. They could break down sales by month to identify any seasonality trends. For example, they might find that sales increase in November and December due to holiday shopping. They could also break down sales by product to identify which items are the most popular. This analysis could inform their purchasing and stocking decisions for the next year. Additionally, data on customer demographics could be analyzed to understand who their primary customers are, guiding their marketing strategies.
Healthcare Industry: In healthcare, descriptive analytics could be used to analyze patient data over time. For instance, a hospital might analyze data on patient admissions to identify trends in admission rates. They might find that admissions for certain conditions are higher at certain times of the year. This could help them allocate resources more effectively. Also, analyzing patient outcomes data can help identify the most effective treatments or highlight areas where improvement is needed.
Finance Industry: A financial firm might use descriptive analytics to analyze historical market data. They could look at trends in stock prices, trading volume, or economic indicators to inform their investment decisions. For example, analyzing the price-earnings ratios of stocks in a certain sector over time could reveal patterns that suggest whether the sector is currently overvalued or undervalued. Similarly, credit card companies can analyze transaction data to detect any unusual patterns, which could be signs of fraud.
Advantages of Descriptive Analytics
Descriptive analytics plays a vital role in the world of data analysis, providing numerous advantages:
- Understanding the Past: Descriptive analytics provides an understanding of what has happened in the past, offering valuable context for future decision-making.
- Data Summarization: Descriptive analytics is used to simplify and summarize complex datasets, which can make the information more understandable and accessible.
- Identifying Patterns and Trends: With descriptive analytics, organizations can identify patterns, trends, and correlations in their data, which can provide valuable insights.
- Inform Decision-Making: The insights generated through descriptive analytics can inform strategic decisions and help organizations to react more quickly to events or changes in behavior.
- Basis for Further Analysis: Descriptive analytics lays the groundwork for further analytical activities. It’s the first necessary step before moving on to more advanced forms of analytics like predictive analytics (forecasting future events) or prescriptive analytics (advising on possible outcomes).
- Performance Evaluation: It allows organizations to evaluate their performance by comparing current results with past results, enabling them to see where improvements have been made and where further improvements can be targeted.
- Enhanced Reporting and Dashboards: Through the use of visualization techniques, descriptive analytics can improve the quality of reports and dashboards, making the data more understandable and easier to interpret for stakeholders at all levels of the organization.
- Immediate Value: Unlike some other types of analytics, descriptive analytics can provide immediate insights, as it doesn’t require complex models or deep analytical capabilities to provide value.
Disadvantages of Descriptive Analytics
While descriptive analytics offers numerous benefits, it also has certain limitations or disadvantages. Here are a few to consider:
- Limited to Past Data: Descriptive analytics primarily deals with historical data and provides insights about past events. It does not predict future events or trends and can’t help you understand possible future outcomes on its own.
- Lack of Deep Insights: While descriptive analytics helps in identifying what happened, it does not answer why it happened. For deeper insights, you would need to use diagnostic analytics, which analyzes data to understand the root cause of a particular outcome.
- Can Be Misleading: If not properly executed, descriptive analytics can sometimes lead to incorrect conclusions. For example, correlation does not imply causation, but descriptive analytics might tempt one to make such an inference.
- Data Quality Issues: The accuracy and usefulness of descriptive analytics are heavily reliant on the quality of the underlying data. If the data is incomplete, incorrect, or biased, the results of the descriptive analytics will be too.
- Over-reliance on Descriptive Analytics: Businesses may rely too much on descriptive analytics and not enough on predictive and prescriptive analytics. While understanding past and present data is important, it’s equally vital to forecast future trends and make data-driven decisions based on those predictions.
- Doesn’t Provide Actionable Insights: Descriptive analytics is used to interpret historical data and identify patterns and trends, but it doesn’t provide recommendations or courses of action. For that, prescriptive analytics is needed.
About the author
Muhammad Hassan
Researcher, Academic Writer, Web developer
You may also like

Predictive Analytics – Techniques, Tools and...

What is Data Science? Components, Process and Tools

Big Data Analytics -Types, Tools and Methods

Blockchain Research – Methods, Types and Examples

Prescriptive Analytics – Techniques, Tools and...

What is Big Data? Types, Tools and Examples

An official website of the United States government
The .gov means it’s official. Federal government websites often end in .gov or .mil. Before sharing sensitive information, make sure you’re on a federal government site.
The site is secure. The https:// ensures that you are connecting to the official website and that any information you provide is encrypted and transmitted securely.
- Publications
- Account settings
Preview improvements coming to the PMC website in October 2024. Learn More or Try it out now .
- Advanced Search
- Journal List
- BMJ Open Access

Analytical studies: a framework for quality improvement design and analysis
Conducting studies for learning is fundamental to improvement. Deming emphasised that the reason for conducting a study is to provide a basis for action on the system of interest. He classified studies into two types depending on the intended target for action. An enumerative study is one in which action will be taken on the universe that was studied. An analytical study is one in which action will be taken on a cause system to improve the future performance of the system of interest. The aim of an enumerative study is estimation, while an analytical study focuses on prediction. Because of the temporal nature of improvement, the theory and methods for analytical studies are a critical component of the science of improvement.
Introduction: enumerative and analytical studies
Designing studies that make it possible to learn from experience and take action to improve future performance is an essential element of quality improvement. These studies use the now traditional theory established through the work of Fisher, 1 Cox, 2 Campbell and Stanley, 3 and others that is widely used in biomedicine research. These designs are used to discover new phenomena that lead to hypothesis generation, and to explore causal mechanisms, 4 as well as to evaluate efficacy and effectiveness. They include observational, retrospective, prospective, pre-experimental, quasiexperimental, blocking, factorial and time-series designs.
In addition to these classifications of studies, Deming 5 defined a distinction between analytical and enumerative studies which has proven to be fundamental to the science of improvement. Deming based his insight on the distinction between these two approaches that Walter Shewhart had made in 1939 as he helped develop measurement strategies for the then-emerging science of ‘quality control.’ 6 The difference between the two concepts lies in the extrapolation of the results that is intended, and in the target for action based on the inferences that are drawn.
A useful way to appreciate that difference is to contrast the inferences that can be made about the water sampled from two different natural sources ( figure 1 ). The enumerative approach is like the study of water from a pond. Because conditions in the bounded universe of the pond are essentially static over time, analyses of random samples taken from the pond at a given time can be used to estimate the makeup of the entire pond. Statistical methods, such as hypothesis testing and CIs, can be used to make decisions and define the precision of the estimates.

Environment in enumerative and analytical study. Internal validity diagram from Fletcher et al . 7
The analytical approach, in contrast, is like the study of water from a river. The river is constantly moving, and its physical properties are changing (eg, due to snow melt, changes in rainfall, dumping of pollutants). The properties of water in a sample from the river at any given time may not describe the river after the samples are taken and analysed. In fact, without repeated sampling over time, it is difficult to make predictions about water quality, since the river will not be the same river in the future as it was at the time of the sampling.
Deming first discussed these concepts in a 1942 paper, 8 as well as in his 1950 textbook, 9 and in a 1975 paper used the enumerative/analytical terminology to characterise specific study designs. 5 While most books on experimental design describe methods for the design and analysis of enumerative studies, Moen et al 10 describe methods for designing and learning from analytical studies. These methods are graphical and focus on prediction of future performance. The concept of analytical studies became a key element in Deming's ‘system of profound knowledge’ that serves as the intellectual foundation for improvement science. 11 The knowledge framework for the science of improvement, which combines elements of psychology, the Shewhart view of variation, the concept of systems, and the theory of knowledge, informs a number of key principles for the design and analysis of improvement studies:
- Knowledge about improvement begins and ends in experimental data but does not end in the data in which it begins.
- Observations, by themselves, do not constitute knowledge.
- Prediction requires theory regarding mechanisms of change and understanding of context.
- Random sampling from a population or universe (assumed by most statistical methods) is not possible when the population of interest is in the future.
- The conditions during studies for improvement will be different from the conditions under which the results will be used. The major source of uncertainty concerning their use is the difficulty of extrapolating study results to different contexts and under different conditions in the future.
- The wider the range of conditions included in an improvement study, the greater the degree of belief in the validity and generalisation of the conclusions.
The classification of studies into enumerative and analytical categories depends on the intended target for action as the result of the study:
- Enumerative studies assume that when actions are taken as the result of a study, they will be taken on the material in the study population or ‘frame’ that was sampled.
More specifically, the study universe in an enumerative study is the bounded group of items (eg, patients, clinics, providers, etc) possessing certain properties of interest. The universe is defined by a frame, a list of identifiable, tangible units that may be sampled and studied. Random selection methods are assumed in the statistical methods used for estimation, decision-making and drawing inferences in enumerative studies. Their aim is estimation about some aspect of the frame (such as a description, comparison or the existence of a cause–effect relationship) and the resulting actions taken on this particular frame. One feature of an enumerative study is that a 100% sample of the frame provides the complete answer to the questions posed by the study (given the methods of investigation and measurement). Statistical methods such as hypothesis tests, CIs and probability statements are appropriate to analyse and report data from enumerative studies. Estimating the infection rate in an intensive care unit for the last month is an example of a simple enumerative study.
- Analytical studies assume that the actions taken as a result of the study will be on the process or causal system that produced the frame studied, rather than the initial frame itself. The aim is to improve future performance.
In contrast to enumerative studies, an analytical study accepts as a given that when actions are taken on a system based on the results of a study, the conditions in that system will inevitably have changed. The aim of an analytical study is to enable prediction about how a change in a system will affect that system's future performance, or prediction as to which plans or strategies for future action on the system will be superior. For example, the task may be to choose among several different treatments for future patients, methods of collecting information or procedures for cleaning an operating room. Because the population of interest is open and continually shifts over time, random samples from that population cannot be obtained in analytical studies, and traditional statistical methods are therefore not useful. Rather, graphical methods of analysis and summary of the repeated samples reveal the trajectory of system behaviour over time, making it possible to predict future behaviour. Use of a Shewhart control chart to monitor and create learning to reduce infection rates in an intensive care unit is an example of a simple analytical study.
The following scenarios give examples to clarify the nature of these two types of studies.
Scenario 1: enumerative study—observation
To estimate how many days it takes new patients to see all primary care physicians contracted with a health plan, a researcher selected a random sample of 150 such physicians from the current active list and called each of their offices to schedule an appointment. The time to the next available appointment ranged from 0 to 180 days, with a mean of 38 days (95% CI 35.6 to 39.6).
This is an enumerative study, since results are intended to be used to estimate the waiting time for appointments with the plan's current population of primary care physicians.
Scenario 2: enumerative study—hypothesis generation
The researcher in scenario 1 noted that on occasion, she was offered an earlier visit with a nurse practitioner (NP) who worked with the physician being called. Additional information revealed that 20 of the 150 physicians in the study worked with one or more NPs. The next available appointment for the 130 physicians without an NP averaged 41 days (95% CI 39 to 43 days) and was 18 days (95% CI 18 to 26 days) for the 20 practices with NPs, a difference of 23 days (a 56% shorter mean waiting time).
This subgroup analysis suggested that the involvement of NPs helps to shorten waiting times, although it does not establish a cause–effect relationship, that is, it was a ‘hypothesis-generating’ study. In any event, this was clearly an enumerative study, since its results were to understand the impact of NPs on waiting times in the particular population of practices. Its results suggested that NPs might influence waiting times, but only for practices in this health plan during the time of the study. The study treated the conditions in the health plan as static, like those in a pond.
Scenario 3: enumerative study—comparison
To find out if administrative changes in a health plan had increased member satisfaction in access to care, the customer service manager replicated a phone survey he had conducted a year previously, using a random sample of 300 members. The percentage of patients who were satisfied with access had increased from 48.7% to 60.7% (Fisher exact test, p<0.004).
This enumerative comparison study was used to estimate the impact of the improvement work during the last year on the members in the plan. Attributing the increase in satisfaction to the improvement work assumes that other conditions in the study frame were static.
Scenario 4: analytical study—learning with a Shewhart chart
Each primary care clinic in a health plan reported its ‘time until the third available appointment’ twice a month, which allowed the quality manager to plot the mean waiting time for all of the clinics on Shewhart charts. Waiting times had been stable for a 12-month period through August, but the manager then noted a special cause (increase in waiting time) in September. On stratifying the data by region, she found that the special cause resulted from increases in waiting time in the Northeast region. Discussion with the regional manager revealed a shortage of primary care physicians in this region, which was predicted to become worse in the next quarter. Making some temporary assignments and increasing physician recruiting efforts resulted in stabilisation of this measure.
Documenting common and special cause variation in measures of interest through the use of Shewhart charts and run charts based on judgement samples is probably the simplest and commonest type of analytical study in healthcare. Such charts, when stable, provide a rational basis for predicting future performance.
Scenario 5: analytical study—establishing a cause–effect relationship
The researcher mentioned in scenarios 1 and 2 planned a study to test the existence of a cause–effect relationship between the inclusion of NPs in primary care offices and waiting time for new patient appointments. The variation in patient characteristics in this health plan appeared to be great enough to make the study results useful to other organisations. For the study, she recruited about 100 of the plan's practices that currently did not use NPs, and obtained funding to facilitate hiring NPs in up to 50 of those practices.
The researcher first explored the theories on mechanisms by which the incorporation of NPs into primary care clinics could reduce waiting times. Using important contextual variables relevant to these mechanisms (practice size, complexity, use of information technology and urban vs rural location), she then developed a randomised block, time-series study design. The study had the power to detect an effect of a mean waiting time of 5 days or more overall, and 10 days for the major subgroups defined by levels of the contextual variables. Since the baseline waiting time for appointments varied substantially across practices, she used the baseline as a covariate.
After completing the study, she analysed data from baseline and postintervention periods using stratified run charts and Shewhart charts, including the raw measures and measures adjusted for important covariates and effects of contextual variables. Overall waiting times decreased 12 days more in practices that included NPs than they did in control practices. Importantly, the subgroup analyses according to contextual variables revealed conditions under which the use of NPs would not be predicted to lead to reductions in waiting times. For example, practices with short baseline waiting times showed little or no improvement by employing NPs. She published the results in a leading health research journal.
This was an analytical study because the intent was to apply the learning from the study to future staffing plans in the health plan. She also published the study, so its results would be useful to primary care practices outside the health plan.
Scenario 6: analytical study—implementing improvement
The quality-improvement manager in another health plan wanted to expand the use of NPs in the plan's primary care practices, because published research had shown a reduction in waiting times for practices with NPs. Two practices in his plan already employed NPs. In one of these practices, Shewhart charts of waiting time by month showed a stable process averaging 10 days during the last 2 years. Waiting time averaged less than 7 days in the second practice, but a period when one of the physicians left the practice was associated with special causes.
The quality manager created a collaborative among the plan's primary care practices to learn how to optimise the use of NPs. Physicians in the two sites that employed NPs served as subject matter experts for the collaborative. In addition to making NPs part of their care teams, participating practices monitored appointment supply and demand, and tested other changes designed to optimise response to patient needs. Thirty sites in the plan voluntarily joined the collaborative and hired NPs. After 6 months, Shewhart charts indicated that waiting times in 25 of the 30 sites had been reduced to less than 7 days. Because waiting times in these practices had been stable over a considerable period of time, the manager predicted that future patients would continue to experience reduced times for appointments. The quality manger began to focus on a follow-up collaborative among the backlog of 70 practices that wanted to join.
This project was clearly an analytical study, since its aim was specifically to improve future waiting-time performance for participating sites and other primary care offices in the plan. Moreover, it focused on learning about the mechanisms through which contextual factors affect the impact of NPs on primary care office functions, under practice conditions that (like those in a river) will inevitably change over time.
Statistical theory in enumerative studies is used to describe the precision of estimates and the validity of hypotheses for the population studied. But since these statistical methods provide no support for extrapolation of the results outside the population that was studied, the subject experts must rely on their understanding of the mechanisms in place to extend results outside the population.
In analytical studies, the standard error of a statistic does not address the most important source of uncertainty, namely, the change in study conditions in the future. Although analytical studies need to take into account the uncertainty due to sampling, as in enumerative studies, the attributes of the study design and analysis of the data primarily deal with the uncertainty resulting from extrapolation to the future (generalisation to the conditions in future time periods). The methods used in analytical studies encourage the exploration of mechanisms through multifactor designs, contextual variables introduced through blocking and replication over time.
Prior stability of a system (as observed in graphic displays of repeated sampling over time, according to Shewhart's methods) increases belief in the results of an analytical study, but stable processes in the past do not guarantee constant system behaviour in the future. The next data point from the future is the most important on a graph of performance. Extrapolation of system behaviour to future times therefore still depends on input from subject experts who are familiar with mechanisms of the system of interest, as well as the important contextual issues. Generalisation is inherently difficult in all studies because ‘whereas the problems of internal validity are solvable within the limits of the logic of probability statistics, the problems of external validity are not logically solvable in any neat, conclusive way’ 3 (p. 17).
The diverse activities commonly referred to as healthcare improvement 12 are all designed to change the behaviour of systems over time, as reflected in the principle that ‘not all change is improvement, but all improvement is change.’ The conditions in the unbounded systems into which improvement interventions are introduced will therefore be different in the future from those in effect at the time the intervention is studied. Since the results of improvement studies are used to predict future system behaviour, such studies clearly belong to the Deming category of analytical studies. Quality improvement studies therefore need to incorporate repeated measurements over time, as well as testing under a wide range of conditions (2, 3 and 10). The ‘gold standard’ of analytical studies is satisfactory prediction over time.
Conclusions and recommendations
In light of these considerations, some important principles for drawing inferences from improvement studies include 10 :
- The analysis of data, interpretation of that analysis and actions taken as a result of the study should be closely tied to the current knowledge of experts about mechanisms of change in the relevant area. They can often use the study to discover, understand and evaluate the underlying mechanisms.
- The conditions of the study will be different from the future conditions under which the results will be used. Assessment by experts of the magnitude of this difference and its potential impact on future events should be an integral part of the interpretation of the results of the intervention.
- Show all the data before aggregation or summary.
- Plot the outcome data in the order in which the tests of change were conducted and annotate with information on the interventions.
- Use graphical displays to assess how much of the variation in the data can be explained by factors that were deliberately changed.
- Rearrange and subgroup the data to study other sources of variation (background and contextual variables).
- Summarise the results of the study with appropriate graphical displays.
Because these principles reflect the fundamental nature of improvement—taking action to change performance, over time, and under changing conditions—their application helps to bring clarity and rigour to improvement science.
Acknowledgments
The author is grateful to F Davidoff and P Batalden for their input to earlier versions of this paper.
Competing interests: None.
Provenance and peer review: Not commissioned; externally peer reviewed.

Qualitative Data Analysis Methods 101:
The “big 6” methods + examples.
By: Kerryn Warren (PhD) | Reviewed By: Eunice Rautenbach (D.Tech) | May 2020 (Updated April 2023)
Qualitative data analysis methods. Wow, that’s a mouthful.
If you’re new to the world of research, qualitative data analysis can look rather intimidating. So much bulky terminology and so many abstract, fluffy concepts. It certainly can be a minefield!
Don’t worry – in this post, we’ll unpack the most popular analysis methods , one at a time, so that you can approach your analysis with confidence and competence – whether that’s for a dissertation, thesis or really any kind of research project.
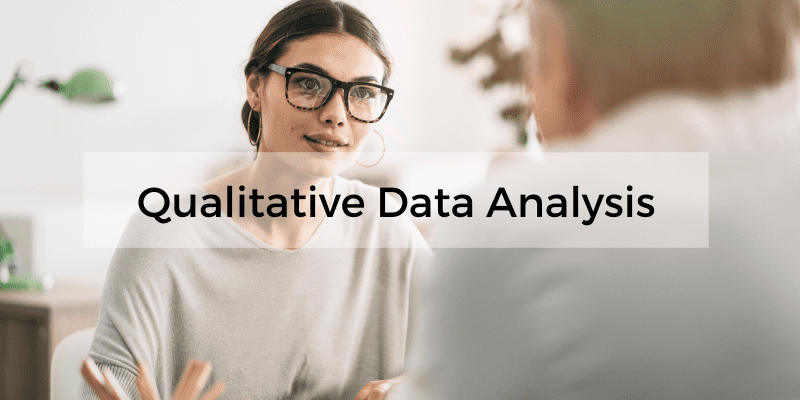
What (exactly) is qualitative data analysis?
To understand qualitative data analysis, we need to first understand qualitative data – so let’s step back and ask the question, “what exactly is qualitative data?”.
Qualitative data refers to pretty much any data that’s “not numbers” . In other words, it’s not the stuff you measure using a fixed scale or complex equipment, nor do you analyse it using complex statistics or mathematics.
So, if it’s not numbers, what is it?
Words, you guessed? Well… sometimes , yes. Qualitative data can, and often does, take the form of interview transcripts, documents and open-ended survey responses – but it can also involve the interpretation of images and videos. In other words, qualitative isn’t just limited to text-based data.
So, how’s that different from quantitative data, you ask?
Simply put, qualitative research focuses on words, descriptions, concepts or ideas – while quantitative research focuses on numbers and statistics . Qualitative research investigates the “softer side” of things to explore and describe , while quantitative research focuses on the “hard numbers”, to measure differences between variables and the relationships between them. If you’re keen to learn more about the differences between qual and quant, we’ve got a detailed post over here .
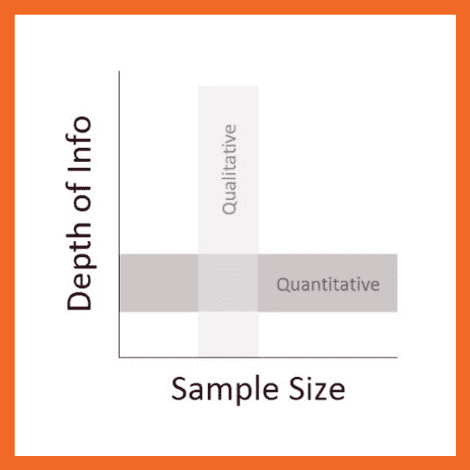
So, qualitative analysis is easier than quantitative, right?
Not quite. In many ways, qualitative data can be challenging and time-consuming to analyse and interpret. At the end of your data collection phase (which itself takes a lot of time), you’ll likely have many pages of text-based data or hours upon hours of audio to work through. You might also have subtle nuances of interactions or discussions that have danced around in your mind, or that you scribbled down in messy field notes. All of this needs to work its way into your analysis.
Making sense of all of this is no small task and you shouldn’t underestimate it. Long story short – qualitative analysis can be a lot of work! Of course, quantitative analysis is no piece of cake either, but it’s important to recognise that qualitative analysis still requires a significant investment in terms of time and effort.
Need a helping hand?
In this post, we’ll explore qualitative data analysis by looking at some of the most common analysis methods we encounter. We’re not going to cover every possible qualitative method and we’re not going to go into heavy detail – we’re just going to give you the big picture. That said, we will of course includes links to loads of extra resources so that you can learn more about whichever analysis method interests you.
Without further delay, let’s get into it.
The “Big 6” Qualitative Analysis Methods
There are many different types of qualitative data analysis, all of which serve different purposes and have unique strengths and weaknesses . We’ll start by outlining the analysis methods and then we’ll dive into the details for each.
The 6 most popular methods (or at least the ones we see at Grad Coach) are:
- Content analysis
- Narrative analysis
- Discourse analysis
- Thematic analysis
- Grounded theory (GT)
- Interpretive phenomenological analysis (IPA)
Let’s take a look at each of them…
QDA Method #1: Qualitative Content Analysis
Content analysis is possibly the most common and straightforward QDA method. At the simplest level, content analysis is used to evaluate patterns within a piece of content (for example, words, phrases or images) or across multiple pieces of content or sources of communication. For example, a collection of newspaper articles or political speeches.
With content analysis, you could, for instance, identify the frequency with which an idea is shared or spoken about – like the number of times a Kardashian is mentioned on Twitter. Or you could identify patterns of deeper underlying interpretations – for instance, by identifying phrases or words in tourist pamphlets that highlight India as an ancient country.
Because content analysis can be used in such a wide variety of ways, it’s important to go into your analysis with a very specific question and goal, or you’ll get lost in the fog. With content analysis, you’ll group large amounts of text into codes , summarise these into categories, and possibly even tabulate the data to calculate the frequency of certain concepts or variables. Because of this, content analysis provides a small splash of quantitative thinking within a qualitative method.
Naturally, while content analysis is widely useful, it’s not without its drawbacks . One of the main issues with content analysis is that it can be very time-consuming , as it requires lots of reading and re-reading of the texts. Also, because of its multidimensional focus on both qualitative and quantitative aspects, it is sometimes accused of losing important nuances in communication.
Content analysis also tends to concentrate on a very specific timeline and doesn’t take into account what happened before or after that timeline. This isn’t necessarily a bad thing though – just something to be aware of. So, keep these factors in mind if you’re considering content analysis. Every analysis method has its limitations , so don’t be put off by these – just be aware of them ! If you’re interested in learning more about content analysis, the video below provides a good starting point.
QDA Method #2: Narrative Analysis
As the name suggests, narrative analysis is all about listening to people telling stories and analysing what that means . Since stories serve a functional purpose of helping us make sense of the world, we can gain insights into the ways that people deal with and make sense of reality by analysing their stories and the ways they’re told.
You could, for example, use narrative analysis to explore whether how something is being said is important. For instance, the narrative of a prisoner trying to justify their crime could provide insight into their view of the world and the justice system. Similarly, analysing the ways entrepreneurs talk about the struggles in their careers or cancer patients telling stories of hope could provide powerful insights into their mindsets and perspectives . Simply put, narrative analysis is about paying attention to the stories that people tell – and more importantly, the way they tell them.
Of course, the narrative approach has its weaknesses , too. Sample sizes are generally quite small due to the time-consuming process of capturing narratives. Because of this, along with the multitude of social and lifestyle factors which can influence a subject, narrative analysis can be quite difficult to reproduce in subsequent research. This means that it’s difficult to test the findings of some of this research.
Similarly, researcher bias can have a strong influence on the results here, so you need to be particularly careful about the potential biases you can bring into your analysis when using this method. Nevertheless, narrative analysis is still a very useful qualitative analysis method – just keep these limitations in mind and be careful not to draw broad conclusions . If you’re keen to learn more about narrative analysis, the video below provides a great introduction to this qualitative analysis method.
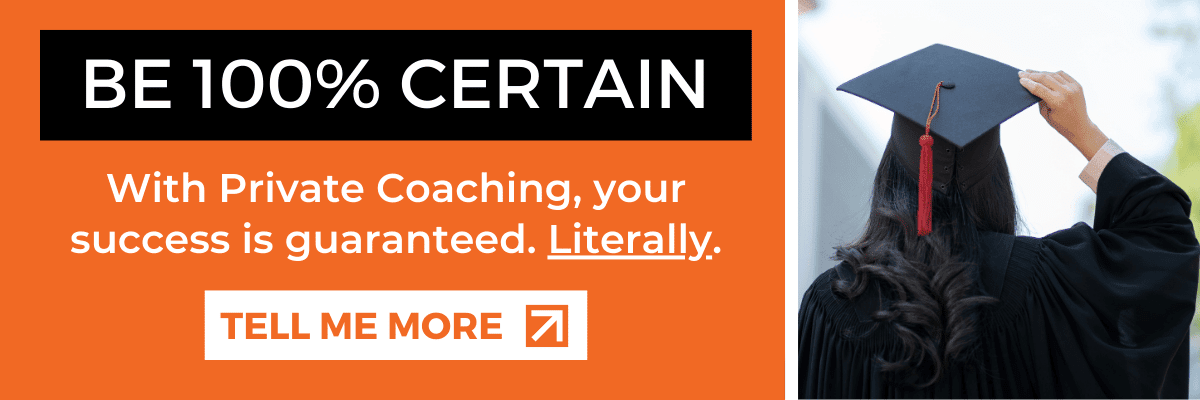
QDA Method #3: Discourse Analysis
Discourse is simply a fancy word for written or spoken language or debate . So, discourse analysis is all about analysing language within its social context. In other words, analysing language – such as a conversation, a speech, etc – within the culture and society it takes place. For example, you could analyse how a janitor speaks to a CEO, or how politicians speak about terrorism.
To truly understand these conversations or speeches, the culture and history of those involved in the communication are important factors to consider. For example, a janitor might speak more casually with a CEO in a company that emphasises equality among workers. Similarly, a politician might speak more about terrorism if there was a recent terrorist incident in the country.
So, as you can see, by using discourse analysis, you can identify how culture , history or power dynamics (to name a few) have an effect on the way concepts are spoken about. So, if your research aims and objectives involve understanding culture or power dynamics, discourse analysis can be a powerful method.
Because there are many social influences in terms of how we speak to each other, the potential use of discourse analysis is vast . Of course, this also means it’s important to have a very specific research question (or questions) in mind when analysing your data and looking for patterns and themes, or you might land up going down a winding rabbit hole.
Discourse analysis can also be very time-consuming as you need to sample the data to the point of saturation – in other words, until no new information and insights emerge. But this is, of course, part of what makes discourse analysis such a powerful technique. So, keep these factors in mind when considering this QDA method. Again, if you’re keen to learn more, the video below presents a good starting point.
QDA Method #4: Thematic Analysis
Thematic analysis looks at patterns of meaning in a data set – for example, a set of interviews or focus group transcripts. But what exactly does that… mean? Well, a thematic analysis takes bodies of data (which are often quite large) and groups them according to similarities – in other words, themes . These themes help us make sense of the content and derive meaning from it.
Let’s take a look at an example.
With thematic analysis, you could analyse 100 online reviews of a popular sushi restaurant to find out what patrons think about the place. By reviewing the data, you would then identify the themes that crop up repeatedly within the data – for example, “fresh ingredients” or “friendly wait staff”.
So, as you can see, thematic analysis can be pretty useful for finding out about people’s experiences , views, and opinions . Therefore, if your research aims and objectives involve understanding people’s experience or view of something, thematic analysis can be a great choice.
Since thematic analysis is a bit of an exploratory process, it’s not unusual for your research questions to develop , or even change as you progress through the analysis. While this is somewhat natural in exploratory research, it can also be seen as a disadvantage as it means that data needs to be re-reviewed each time a research question is adjusted. In other words, thematic analysis can be quite time-consuming – but for a good reason. So, keep this in mind if you choose to use thematic analysis for your project and budget extra time for unexpected adjustments.
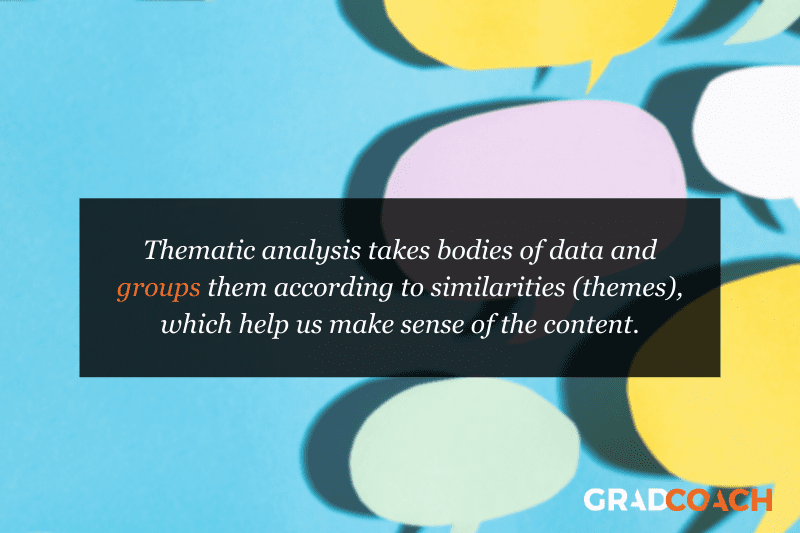
QDA Method #5: Grounded theory (GT)
Grounded theory is a powerful qualitative analysis method where the intention is to create a new theory (or theories) using the data at hand, through a series of “ tests ” and “ revisions ”. Strictly speaking, GT is more a research design type than an analysis method, but we’ve included it here as it’s often referred to as a method.
What’s most important with grounded theory is that you go into the analysis with an open mind and let the data speak for itself – rather than dragging existing hypotheses or theories into your analysis. In other words, your analysis must develop from the ground up (hence the name).
Let’s look at an example of GT in action.
Assume you’re interested in developing a theory about what factors influence students to watch a YouTube video about qualitative analysis. Using Grounded theory , you’d start with this general overarching question about the given population (i.e., graduate students). First, you’d approach a small sample – for example, five graduate students in a department at a university. Ideally, this sample would be reasonably representative of the broader population. You’d interview these students to identify what factors lead them to watch the video.
After analysing the interview data, a general pattern could emerge. For example, you might notice that graduate students are more likely to read a post about qualitative methods if they are just starting on their dissertation journey, or if they have an upcoming test about research methods.
From here, you’ll look for another small sample – for example, five more graduate students in a different department – and see whether this pattern holds true for them. If not, you’ll look for commonalities and adapt your theory accordingly. As this process continues, the theory would develop . As we mentioned earlier, what’s important with grounded theory is that the theory develops from the data – not from some preconceived idea.
So, what are the drawbacks of grounded theory? Well, some argue that there’s a tricky circularity to grounded theory. For it to work, in principle, you should know as little as possible regarding the research question and population, so that you reduce the bias in your interpretation. However, in many circumstances, it’s also thought to be unwise to approach a research question without knowledge of the current literature . In other words, it’s a bit of a “chicken or the egg” situation.
Regardless, grounded theory remains a popular (and powerful) option. Naturally, it’s a very useful method when you’re researching a topic that is completely new or has very little existing research about it, as it allows you to start from scratch and work your way from the ground up .
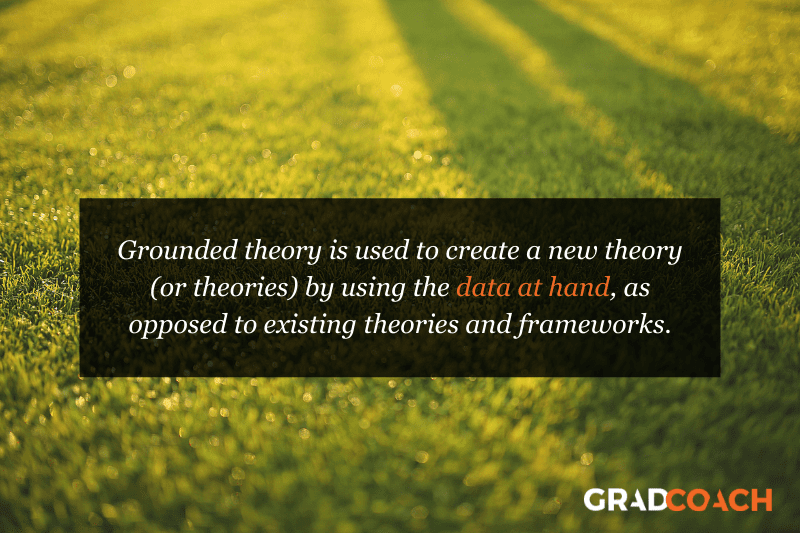
QDA Method #6: Interpretive Phenomenological Analysis (IPA)
Interpretive. Phenomenological. Analysis. IPA . Try saying that three times fast…
Let’s just stick with IPA, okay?
IPA is designed to help you understand the personal experiences of a subject (for example, a person or group of people) concerning a major life event, an experience or a situation . This event or experience is the “phenomenon” that makes up the “P” in IPA. Such phenomena may range from relatively common events – such as motherhood, or being involved in a car accident – to those which are extremely rare – for example, someone’s personal experience in a refugee camp. So, IPA is a great choice if your research involves analysing people’s personal experiences of something that happened to them.
It’s important to remember that IPA is subject – centred . In other words, it’s focused on the experiencer . This means that, while you’ll likely use a coding system to identify commonalities, it’s important not to lose the depth of experience or meaning by trying to reduce everything to codes. Also, keep in mind that since your sample size will generally be very small with IPA, you often won’t be able to draw broad conclusions about the generalisability of your findings. But that’s okay as long as it aligns with your research aims and objectives.
Another thing to be aware of with IPA is personal bias . While researcher bias can creep into all forms of research, self-awareness is critically important with IPA, as it can have a major impact on the results. For example, a researcher who was a victim of a crime himself could insert his own feelings of frustration and anger into the way he interprets the experience of someone who was kidnapped. So, if you’re going to undertake IPA, you need to be very self-aware or you could muddy the analysis.
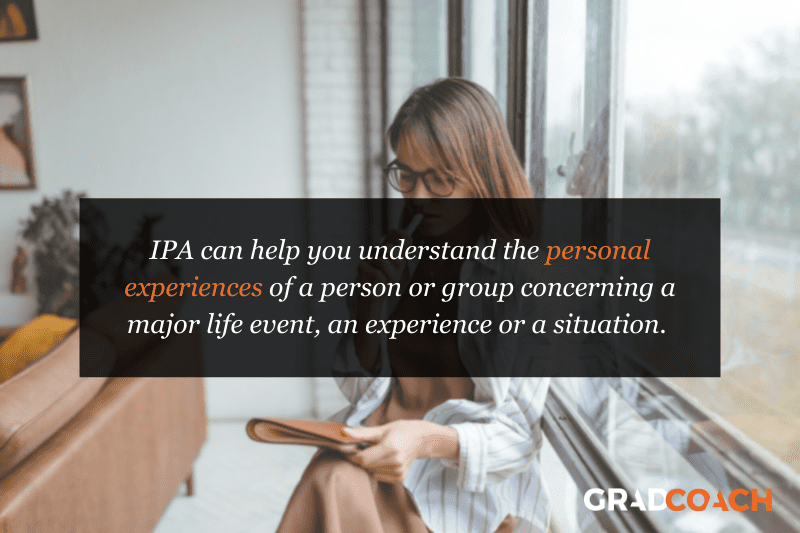
How to choose the right analysis method
In light of all of the qualitative analysis methods we’ve covered so far, you’re probably asking yourself the question, “ How do I choose the right one? ”
Much like all the other methodological decisions you’ll need to make, selecting the right qualitative analysis method largely depends on your research aims, objectives and questions . In other words, the best tool for the job depends on what you’re trying to build. For example:
- Perhaps your research aims to analyse the use of words and what they reveal about the intention of the storyteller and the cultural context of the time.
- Perhaps your research aims to develop an understanding of the unique personal experiences of people that have experienced a certain event, or
- Perhaps your research aims to develop insight regarding the influence of a certain culture on its members.
As you can probably see, each of these research aims are distinctly different , and therefore different analysis methods would be suitable for each one. For example, narrative analysis would likely be a good option for the first aim, while grounded theory wouldn’t be as relevant.
It’s also important to remember that each method has its own set of strengths, weaknesses and general limitations. No single analysis method is perfect . So, depending on the nature of your research, it may make sense to adopt more than one method (this is called triangulation ). Keep in mind though that this will of course be quite time-consuming.
As we’ve seen, all of the qualitative analysis methods we’ve discussed make use of coding and theme-generating techniques, but the intent and approach of each analysis method differ quite substantially. So, it’s very important to come into your research with a clear intention before you decide which analysis method (or methods) to use.
Start by reviewing your research aims , objectives and research questions to assess what exactly you’re trying to find out – then select a qualitative analysis method that fits. Never pick a method just because you like it or have experience using it – your analysis method (or methods) must align with your broader research aims and objectives.
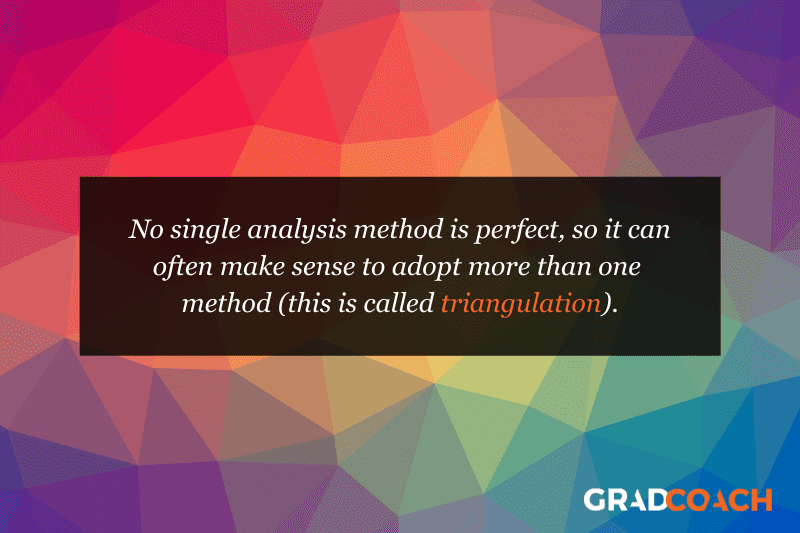
Let’s recap on QDA methods…
In this post, we looked at six popular qualitative data analysis methods:
- First, we looked at content analysis , a straightforward method that blends a little bit of quant into a primarily qualitative analysis.
- Then we looked at narrative analysis , which is about analysing how stories are told.
- Next up was discourse analysis – which is about analysing conversations and interactions.
- Then we moved on to thematic analysis – which is about identifying themes and patterns.
- From there, we went south with grounded theory – which is about starting from scratch with a specific question and using the data alone to build a theory in response to that question.
- And finally, we looked at IPA – which is about understanding people’s unique experiences of a phenomenon.
Of course, these aren’t the only options when it comes to qualitative data analysis, but they’re a great starting point if you’re dipping your toes into qualitative research for the first time.
If you’re still feeling a bit confused, consider our private coaching service , where we hold your hand through the research process to help you develop your best work.
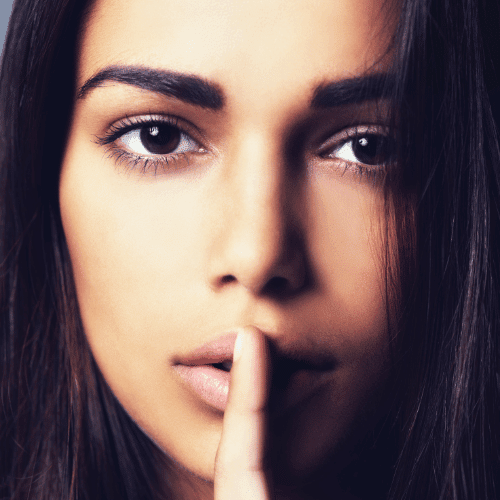
Psst... there’s more!
This post was based on one of our popular Research Bootcamps . If you're working on a research project, you'll definitely want to check this out ...
87 Comments
This has been very helpful. Thank you.
Thank you madam,
Thank you so much for this information
I wonder it so clear for understand and good for me. can I ask additional query?
Very insightful and useful
Good work done with clear explanations. Thank you.
Thanks so much for the write-up, it’s really good.
Thanks madam . It is very important .
thank you very good
Great presentation
This has been very well explained in simple language . It is useful even for a new researcher.
Great to hear that. Good luck with your qualitative data analysis, Pramod!
This is very useful information. And it was very a clear language structured presentation. Thanks a lot.
Thank you so much.
very informative sequential presentation
Precise explanation of method.
Hi, may we use 2 data analysis methods in our qualitative research?
Thanks for your comment. Most commonly, one would use one type of analysis method, but it depends on your research aims and objectives.
You explained it in very simple language, everyone can understand it. Thanks so much.
Thank you very much, this is very helpful. It has been explained in a very simple manner that even a layman understands
Thank nicely explained can I ask is Qualitative content analysis the same as thematic analysis?
Thanks for your comment. No, QCA and thematic are two different types of analysis. This article might help clarify – https://onlinelibrary.wiley.com/doi/10.1111/nhs.12048
This is my first time to come across a well explained data analysis. so helpful.
I have thoroughly enjoyed your explanation of the six qualitative analysis methods. This is very helpful. Thank you!
Thank you very much, this is well explained and useful
i need a citation of your book.
Thanks a lot , remarkable indeed, enlighting to the best
Hi Derek, What other theories/methods would you recommend when the data is a whole speech?
Keep writing useful artikel.
It is important concept about QDA and also the way to express is easily understandable, so thanks for all.
Thank you, this is well explained and very useful.
Very helpful .Thanks.
Hi there! Very well explained. Simple but very useful style of writing. Please provide the citation of the text. warm regards
The session was very helpful and insightful. Thank you
This was very helpful and insightful. Easy to read and understand
As a professional academic writer, this has been so informative and educative. Keep up the good work Grad Coach you are unmatched with quality content for sure.
Keep up the good work Grad Coach you are unmatched with quality content for sure.
Its Great and help me the most. A Million Thanks you Dr.
It is a very nice work
Very insightful. Please, which of this approach could be used for a research that one is trying to elicit students’ misconceptions in a particular concept ?
This is Amazing and well explained, thanks
great overview
What do we call a research data analysis method that one use to advise or determining the best accounting tool or techniques that should be adopted in a company.
Informative video, explained in a clear and simple way. Kudos
Waoo! I have chosen method wrong for my data analysis. But I can revise my work according to this guide. Thank you so much for this helpful lecture.
This has been very helpful. It gave me a good view of my research objectives and how to choose the best method. Thematic analysis it is.
Very helpful indeed. Thanku so much for the insight.
This was incredibly helpful.
Very helpful.
very educative
Nicely written especially for novice academic researchers like me! Thank you.
choosing a right method for a paper is always a hard job for a student, this is a useful information, but it would be more useful personally for me, if the author provide me with a little bit more information about the data analysis techniques in type of explanatory research. Can we use qualitative content analysis technique for explanatory research ? or what is the suitable data analysis method for explanatory research in social studies?
that was very helpful for me. because these details are so important to my research. thank you very much
I learnt a lot. Thank you
Relevant and Informative, thanks !
Well-planned and organized, thanks much! 🙂
I have reviewed qualitative data analysis in a simplest way possible. The content will highly be useful for developing my book on qualitative data analysis methods. Cheers!
Clear explanation on qualitative and how about Case study
This was helpful. Thank you
This was really of great assistance, it was just the right information needed. Explanation very clear and follow.
Wow, Thanks for making my life easy
This was helpful thanks .
Very helpful…. clear and written in an easily understandable manner. Thank you.
This was so helpful as it was easy to understand. I’m a new to research thank you so much.
so educative…. but Ijust want to know which method is coding of the qualitative or tallying done?
Thank you for the great content, I have learnt a lot. So helpful
precise and clear presentation with simple language and thank you for that.
very informative content, thank you.
You guys are amazing on YouTube on this platform. Your teachings are great, educative, and informative. kudos!
Brilliant Delivery. You made a complex subject seem so easy. Well done.
Beautifully explained.
Thanks a lot
Is there a video the captures the practical process of coding using automated applications?
Thanks for the comment. We don’t recommend using automated applications for coding, as they are not sufficiently accurate in our experience.
content analysis can be qualitative research?
THANK YOU VERY MUCH.
Thank you very much for such a wonderful content
do you have any material on Data collection
What a powerful explanation of the QDA methods. Thank you.
Great explanation both written and Video. i have been using of it on a day to day working of my thesis project in accounting and finance. Thank you very much for your support.
very helpful, thank you so much
The tutorial is useful. I benefited a lot.
This is an eye opener for me and very informative, I have used some of your guidance notes on my Thesis, I wonder if you can assist with your 1. name of your book, year of publication, topic etc., this is for citing in my Bibliography,
I certainly hope to hear from you
Submit a Comment Cancel reply
Your email address will not be published. Required fields are marked *
Save my name, email, and website in this browser for the next time I comment.
- Print Friendly

Descriptive vs. Analytical Research in Sociology: A Comparative Study

Table of Contents
When we delve into the world of research, particularly in fields like sociology , we encounter a myriad of methods designed to uncover the layers of human society and behavior. Two of the most fundamental research methods are descriptive and analytical research . Each plays a crucial role in understanding our world, but they do so in distinctly different ways. So, what exactly are these methods, and how do they compare when applied in the realm of social studies? Let’s embark on a comparative journey to understand these methodologies better.
Understanding Descriptive Research
Descriptive research is akin to the meticulous work of an artist attempting to capture the intricate details of a landscape. It aims to accurately describe the characteristics of a particular population or phenomenon. By painting a picture of the ‘what’ aspect, this method helps researchers to understand the prevalence of certain attributes, behaviors, or issues within a group.
Key Features of Descriptive Research
- Snapshot in time: It often involves studying a single point or period, providing a snapshot rather than a motion picture.
- Surveys and observations : Common tools include surveys , observations, and case studies .
- Quantitative data: It leans heavily on quantitative data to present findings in numerical format.
- No hypothesis testing: Unlike other research types, it doesn’t typically involve hypothesis testing.
When to Use Descriptive Research
- Establishing a baseline : When there’s a need to set a reference point for future studies or track changes over time.
- Exploratory purposes: When little is known about a topic and there’s a need to gather initial information that could inform future research.
- Policy-making: When organizations or government bodies need factual data to inform decisions and policies.
Exploring Analytical Research
On the flip side, analytical research steps beyond mere description to explore the ‘why’ and ‘how’. It’s like a detective piecing together clues to not just recount events, but to understand the relationships and causations behind them. Analytical researchers critically evaluate information to draw conclusions and generalizations that extend beyond the immediate data.
Key Characteristics of Analytical Research
- Critical evaluation: It involves a deep analysis of the available information to form judgments.
- Qualitative and quantitative data: Uses both numerical data and non-numerical data for a more comprehensive analysis.
- Hypothesis-driven: This method often starts with a hypothesis that the research is designed to test.
- Seeking patterns : Aims to identify patterns, relationships, and causations.
When to Opt for Analytical Research
- Understanding complexities: When the research question is complex and requires understanding the interplay of various factors.
- Building upon previous research: When expanding on existing knowledge or challenging prevailing theories.
- Recommendations for action: When research is aimed at providing actionable insights or solutions to problems.
Comparing Descriptive and Analytical Research in Real-World Scenarios
Imagine a sociologist aiming to tackle a pressing social issue, such as the dynamics of homelessness in urban areas. Descriptive research would enable them to establish the scale and scope of homelessness, identifying key demographics and patterns. Analytical research, however, would take these findings and probe deeper into the causes, examining the social, economic, and political factors that contribute to the situation and what can be done to alleviate it.
Advantages and Limitations
Each research type has its own set of strengths and weaknesses. Descriptive research is powerful for mapping out the landscape but may fall short in explaining the underlying reasons for observed phenomena. Analytical research, with its depth, can provide those explanations, but it may be more time-consuming and complex to conduct.
Choosing the Right Approach
Deciding between descriptive and analytical research often comes down to the specific objectives of the study. It’s not uncommon for researchers to employ both methods within the same broader research project to maximize their understanding of a topic.
In conclusion, descriptive and analytical research are two sides of the same coin, offering different lenses through which we can view and interpret the intricacies of social phenomena. By understanding their distinctions and applications, researchers can better design studies that yield rich, actionable insights into the fabric of society.
What do you think? Could a blend of both descriptive and analytical research provide a more holistic understanding of social issues? Are there situations where one method is clearly preferable over the other?
Submit a Comment Cancel reply
Your email address will not be published. Required fields are marked *
Save my name, email, and website in this browser for the next time I comment.
Submit Comment
Research Methodologies & Methods
1 Logic of Inquiry in Social Research
- A Science of Society
- Comte’s Ideas on the Nature of Sociology
- Observation in Social Sciences
- Logical Understanding of Social Reality
2 Empirical Approach
- Empirical Approach
- Rules of Data Collection
- Cultural Relativism
- Problems Encountered in Data Collection
- Difference between Common Sense and Science
- What is Ethical?
- What is Normal?
- Understanding the Data Collected
- Managing Diversities in Social Research
- Problematising the Object of Study
- Conclusion: Return to Good Old Empirical Approach
3 Diverse Logic of Theory Building
- Concern with Theory in Sociology
- Concepts: Basic Elements of Theories
- Why Do We Need Theory?
- Hypothesis Description and Experimentation
- Controlled Experiment
- Designing an Experiment
- How to Test a Hypothesis
- Sensitivity to Alternative Explanations
- Rival Hypothesis Construction
- The Use and Scope of Social Science Theory
- Theory Building and Researcher’s Values
4 Theoretical Analysis
- Premises of Evolutionary and Functional Theories
- Critique of Evolutionary and Functional Theories
- Turning away from Functionalism
- What after Functionalism
- Post-modernism
- Trends other than Post-modernism
5 Issues of Epistemology
- Some Major Concerns of Epistemology
- Rationalism
- Phenomenology: Bracketing Experience
6 Philosophy of Social Science
- Foundations of Science
- Science, Modernity, and Sociology
- Rethinking Science
- Crisis in Foundation
7 Positivism and its Critique
- Heroic Science and Origin of Positivism
- Early Positivism
- Consolidation of Positivism
- Critiques of Positivism
8 Hermeneutics
- Methodological Disputes in the Social Sciences
- Tracing the History of Hermeneutics
- Hermeneutics and Sociology
- Philosophical Hermeneutics
- The Hermeneutics of Suspicion
- Phenomenology and Hermeneutics
9 Comparative Method
- Relationship with Common Sense; Interrogating Ideological Location
- The Historical Context
- Elements of the Comparative Approach
10 Feminist Approach
- Features of the Feminist Method
- Feminist Methods adopt the Reflexive Stance
- Feminist Discourse in India
11 Participatory Method
- Delineation of Key Features
12 Types of Research
- Basic and Applied Research
- Descriptive and Analytical Research
- Empirical and Exploratory Research
- Quantitative and Qualitative Research
- Explanatory (Causal) and Longitudinal Research
- Experimental and Evaluative Research
- Participatory Action Research
13 Methods of Research
- Evolutionary Method
- Comparative Method
- Historical Method
- Personal Documents
14 Elements of Research Design
- Structuring the Research Process
15 Sampling Methods and Estimation of Sample Size
- Classification of Sampling Methods
- Sample Size
16 Measures of Central Tendency
- Relationship between Mean, Mode, and Median
- Choosing a Measure of Central Tendency
17 Measures of Dispersion and Variability
- The Variance
- The Standard Deviation
- Coefficient of Variation
18 Statistical Inference- Tests of Hypothesis
- Statistical Inference
- Tests of Significance
19 Correlation and Regression
- Correlation
- Method of Calculating Correlation of Ungrouped Data
- Method Of Calculating Correlation Of Grouped Data
20 Survey Method
- Rationale of Survey Research Method
- History of Survey Research
- Defining Survey Research
- Sampling and Survey Techniques
- Operationalising Survey Research Tools
- Advantages and Weaknesses of Survey Research
21 Survey Design
- Preliminary Considerations
- Stages / Phases in Survey Research
- Formulation of Research Question
- Survey Research Designs
- Sampling Design
22 Survey Instrumentation
- Techniques/Instruments for Data Collection
- Questionnaire Construction
- Issues in Designing a Survey Instrument
23 Survey Execution and Data Analysis
- Problems and Issues in Executing Survey Research
- Data Analysis
- Ethical Issues in Survey Research
24 Field Research – I
- History of Field Research
- Ethnography
- Theme Selection
- Gaining Entry in the Field
- Key Informants
- Participant Observation
25 Field Research – II
- Interview its Types and Process
- Feminist and Postmodernist Perspectives on Interviewing
- Narrative Analysis
- Interpretation
- Case Study and its Types
- Life Histories
- Oral History
- PRA and RRA Techniques
26 Reliability, Validity and Triangulation
- Concepts of Reliability and Validity
- Three Types of “Reliability”
- Working Towards Reliability
- Procedural Validity
- Field Research as a Validity Check
- Method Appropriate Criteria
- Triangulation
- Ethical Considerations in Qualitative Research
27 Qualitative Data Formatting and Processing
- Qualitative Data Processing and Analysis
- Description
- Classification
- Making Connections
- Theoretical Coding
- Qualitative Content Analysis
28 Writing up Qualitative Data
- Problems of Writing Up
- Grasp and Then Render
- “Writing Down” and “Writing Up”
- Write Early
- Writing Styles
- First Draft
29 Using Internet and Word Processor
- What is Internet and How Does it Work?
- Internet Services
- Searching on the Web: Search Engines
- Accessing and Using Online Information
- Online Journals and Texts
- Statistical Reference Sites
- Data Sources
- Uses of E-mail Services in Research
30 Using SPSS for Data Analysis Contents
- Introduction
- Starting and Exiting SPSS
- Creating a Data File
- Univariate Analysis
- Bivariate Analysis
31 Using SPSS in Report Writing
- Why to Use SPSS
- Working with SPSS Output
- Copying SPSS Output to MS Word Document
32 Tabulation and Graphic Presentation- Case Studies
- Structure for Presentation of Research Findings
- Data Presentation: Editing, Coding, and Transcribing
- Case Studies
- Qualitative Data Analysis and Presentation through Software
- Types of ICT used for Research
33 Guidelines to Research Project Assignment
- Overview of Research Methodologies and Methods (MSO 002)
- Research Project Objectives
- Preparation for Research Project
- Stages of the Research Project
- Supervision During the Research Project
- Submission of Research Project
- Methodology for Evaluating Research Project
Share on Mastodon
Overview of Analytic Studies
Introduction
We search for the determinants of health outcomes, first, by relying on descriptive epidemiology to generate hypotheses about associations between exposures and outcomes. Analytic studies are then undertaken to test specific hypotheses. Samples of subjects are identified and information about exposure status and outcome is collected. The essence of an analytic study is that groups of subjects are compared in order to estimate the magnitude of association between exposures and outcomes.
In their book entitled "Epidemiology Matters" Katherine Keyes and Sandro Galea discuss three fundamental options for studying samples from a population as illustrated in the video below (duration 8:30).
Learning Objectives
After successfully completing this section, the student will be able to:
- Describe the difference between descriptive and scientific/analytic epidemiologic studies in terms of information/evidence provided for medicine and public health.
- Define and explain the distinguishing features of a cohort study.
- Describe and identify the types of epidemiologic questions that can be addressed by cohort studies.
- Define and distinguish among prospective and retrospective cohort studies using the investigator as the point of reference.
- Define and explain the distinguishing features of a case-control study.
- Explain the distinguishing features of an intervention study.
- Identify the study design when reading an article or abstract.
Cohort Type Studies
A cohort is a "group." In epidemiology a cohort is a group of individuals who are followed over a period of time, primarily to assess what happens to them, i.e., their health outcomes. In cohort type studies one identifies individuals who do not have the outcome of interest initially, and groups them in subsets that differ in their exposure to some factor, e.g., smokers and non-smokers. The different exposure groups are then followed over time in order to compare the incidence of health outcomes, such as lung cancer or heart disease. As an example, the Framingham Heart Study enrolled a cohort of 5,209 residents of Framingham, MA who were between the ages of 30-62 and who did not have cardiovascular disease when they were enrolled. These subjects differed from one another in many ways: whether they smoked, how much they smoked, body mass index, eating habits, exercise habits, gender, family history of heart disease, etc. The researchers assessed these and many other characteristics or "exposures" soon after the subjects had been enrolled and before any of them had developed cardiovascular disease. The many "baseline characteristics" were assessed in a number of ways including questionnaires, physical exams, laboratory tests, and imaging studies (e.g., x-rays). They then began "following" the cohort, meaning that they kept in contact with the subjects by phone, mail, or clinic visits in order to determine if and when any of the subjects developed any of the "outcomes of interest," such as myocardial infarction (heart attack), angina, congestive heart failure, stroke, diabetes and many other cardiovascular outcomes.
Over time some subjects eventually began to develop some of the outcomes of interest. Having followed the cohort in this fashion, it was eventually possible to use the information collected to evaluate many hypotheses about what characteristics were associated with an increased risk of heart disease. For example, if one hypothesized that smoking increased the risk of heart attacks, the subjects in the cohort could be sorted based on their smoking habits, and one could compare the subset of the cohort that smoked to the subset who had never smoked. For each such comparison that one wanted to make the cohort could be grouped according to whether they had a given exposure or not, and one could measure and compare the frequency of heart attacks (i.e., the incidence) between the groups. Incidence provides an estimate of risk, so if the incidence of heart attacks is 3 times greater in smokers compared to non-smokers, it suggests an association between smoking and risk of developing a heart attack. (Various biases might also be an explanation for an apparent association. We will learn about these later in the course.) The hallmark of analytical studies, then, is that they collect information about both exposure status and outcome status, and they compare groups to identify whether there appears to be an association or a link.
The Population "At Risk"
From the discussion above, it should be obvious that one of the basic requirements of a cohort type study is that none of the subjects have the outcome of interest at the beginning of the follow-up period, and time must pass in order to determine the frequency of developing the outcome.
- For example, if one wanted to compare the risk of developing uterine cancer between postmenopausal women receiving hormone-replacement therapy and those not receiving hormones, one would consider certain eligibility criteria for the members prior to the start of the study: 1) they should be female, 2) they should be post-menopausal, and 3) they should have a uterus. Among post-menopausal women there might be a number who had had a hysterectomy already, perhaps for persistent bleeding problems or endometriosis. Since these women no longer have a uterus, one would want to exclude them from the cohort, because they are no longer at risk of developing this particular type of cancer.
- Similarly, if one wanted to compare the risk of developing diabetes among nursing home residents who exercised and those who did not, it would be important to test the subjects for diabetes at the beginning of the follow-up period in order to exclude all subjects who already had diabetes and therefore were not "at risk" of developing diabetes.
Eligible subjects have to meet certain criteria to be included as subjects in a study (inclusion criteria). One of these would be that they did not have any of the diseases or conditions that the investigators want to study, i.e., the subjects must be "at risk," of developing the outcome of interest, and the members of the cohort to be followed are sometimes referred to as "the population at risk."
However, at times decisions about who is "at risk" and eligible get complicated.
Example #1: Suppose the outcome of interest is development of measles. There may be subjects who:
- Already were known to have had clinically apparent measles and are immune to subsequent measles infection
- Had sub-clinical cases of measles that went undetected (but the subject may still be immune)
- Had a measles vaccination that conferred immunity
- Had a measles vaccination that failed to confer immunity
In this case the eligibility criteria would be shaped by the specific scientific questions being asked. One might want to compare subjects known to have had clinically apparent measles to those who had not had clinical measles and had not had a measles vaccination. Or, one could take blood sample from all potential subjects in order to measure their antibody titers (levels) to the measles virus.
Example #2: Suppose you are studying an event that can occur more that once, such as a heart attack. Again, the eligibility criteria should be shaped to fit the scientific questions that are being answered. If one were interested in the risk of a first myocardial infarction, then obviously subjects who had already had a heart attack would not be eligible for study. On the other hand, if one were interested in tertiary prevention of heart attacks, the study cohort would include people who had had heart attacks or other clinical manifestations of heart disease, and the outcome of interest would be subsequent significant cardiac events or death.
Prospective and Retrospective Cohort Studies
Cohort studies can be classified as prospective or retrospective based on when outcomes occurred in relation to the enrollment of the cohort.
Prospective Cohort Studies

In a prospective study like the Nurses Health Study baseline information is collected from all subjects in the same way using exactly the same questions and data collection methods for all subjects. The investigators design the questions and data collection procedures carefully in order to obtain accurate information about exposures before disease develops in any of the subjects. After baseline information is collected, subjects in a prospective cohort study are then followed "longitudinally," i.e. over a period of time, usually for years, to determine if and when they become diseased and whether their exposure status changes. In this way, investigators can eventually use the data to answer many questions about the associations between "risk factors" and disease outcomes. For example, one could identify smokers and non-smokers at baseline and compare their subsequent incidence of developing heart disease. Alternatively, one could group subjects based on their body mass index (BMI) and compare their risk of developing heart disease or cancer.
Key Concept: The distinguishing feature of a prospective cohort study is that at the time that the investigators begin enrolling subjects and collecting baseline exposure information, none of the subjects has developed any of the outcomes of interest.
|
Examples of Prospective Cohort Studies
- The Framingham Heart Study Home Page
- The Nurses Health Study Home Page

Pitfall: Note that in these prospective cohort studies a comparison of incidence between the groups can only take place after enough time has elapsed so that some subjects developed the outcomes of interest. Since the data analysis occurs after some outcomes have occurred, some students mistakenly would call this a retrospective study, but this is incorrect. The analysis always occurs after a certain number of events have taken place. The characteristic that distinguishes a study as prospective is that the subjects were enrolled, and baseline data was collected before any subjects developed an outcome of interest.
Retrospective Cohort Studies
In contrast, retrospective studies are conceived after some people have already developed the outcomes of interest. The investigators jump back in time to identify a cohort of individuals at a point in time before they have developed the outcomes of interest, and they try to establish their exposure status at that point in time. They then determine whether the subject subsequently developed the outcome of interest.

Suppose investigators wanted to test the hypothesis that working with the chemicals involved in tire manufacturing increases the risk of death. Since this is a fairly rare exposure, it would be advantageous to use a special exposure cohort such as employees of a large tire manufacturing factory. The employees who actually worked with chemicals used in the manufacturing process would be the exposed group, while clerical workers and management might constitute the "unexposed" group. However, rather than following these subjects for decades, it would be more efficient to use employee health and employment records over the past two or three decades as a source of data. In essence, the investigators are jumping back in time to identify the study cohort at a point in time before the outcome of interest (death) occurred. They can classify them as "exposed" or "unexposed" based on their employment records, and they can use a number of sources to determine subsequent outcome status, such as death (e.g., using health records, next of kin, National Death Index, etc.).
Key Concept: The distinguishing feature of a retrospective cohort study is that the investigators conceive the study and begin identifying and enrolling subjects . |
Retrospective cohort studies like the one described above are very efficient for studying rare or unusual exposures, but there are many potential problems here. Sometimes exposure status is not clear when it is necessary to go back in time and use whatever data is available, especially because the data being used was not designed to answer a health question. Even if it was clear who was exposed to tire manufacturing chemicals based on employee records, it would also be important to take into account (or adjust for) other differences that could have influenced mortality, i.e., confounding factors. For example, it might be important to know whether the subjects smoked, or drank, or what kind of diet they ate. However, it is unlikely that a retrospective cohort study would have accurate information on these many other risk factors.
The video below provides a brief (7:31) explanation of the distinction between retrospective and prospective cohort studies.
Link to a transcript of the video
Intervention Studies (Clinical Trials)
Intervention studies (clinical trials) are experimental research studies that compare the effectiveness of medical treatments, management strategies, prevention strategies, and other medical or public health interventions. Their design is very similar to that of a prospective cohort study. However, in cohort studies exposure status is determined by genetics, self-selection, or life circumstances, and the investigators just observe differences in outcome between those who have a given exposure and those who do not. In clinical trials exposure status (the treatment type) is assigned by the investigators . Ideally, assignment of subjects to one of the comparison groups should be done randomly in order to produce equal distributions of potentially confounding factors. Sometimes a group receiving a new treatment is compared to an untreated group, or a group receiving a placebo or a sham treatment. Sometimes, a new treatment is compared to an untreated group or to a group receiving an established treatment. For more on this topic see the module on Intervention Studies.
In summary, the characteristic that distinguishes a clinical trial from a cohort study is that the investigator assigns the exposure status in a clinical trial, while subjects' genetics, behaviors, and life circumstances determine their exposures in a cohort study.
Key Concept: Common features of both prospective and retrospective cohort studies.
|
Summarizing Data in a Cohort Study
Investigators often use contingency tables to summarize data. In essence, the table is a matrix that displays the combinations of exposure and outcome status. If one were summarizing the results of a study with two possible exposure categories and two possible outcomes, one would use a "two by two" table in which the numbers in the four cells indicate the number of subjects within each of the 4 possible categories of risk and disease status.
For example, consider data from a retrospective cohort study conducted by the Massachusetts Department of Public Health (MDPH) during an investigation of an outbreak of Giardia lamblia in Milton, MA in 2003. The descriptive epidemiology indicated that almost all of the cases belonged to a country club in Milton. The club had an adult swimming pool and a wading pool for toddlers, and the investigators suspected that the outbreak may have occurred when an infected child with a dirty diaper contaminated the water in the kiddy pool. This hypothesis was tested by conducting a retrospective cohort study. The cases of Giardia lamblia had already occurred and had been reported to MDPH via the infectious disease surveillance system (for more information on surveillance, see the Surveillance module). The investigation focused on an obvious cohort - 479 members of the country club who agreed to answer the MDPH questionnaire. The questionnaire asked, among many other things, whether the subject had been exposed to the kiddy pool. The incidence of subsequent Giardia infection was then compared between subjects who been exposed to the kiddy pool and those who had not.
The table below summarizes the findings. A total of 479 subjects completed the questionnaire, and 124 of them indicated that they had been exposed to the kiddy pool. Of these, 16 subsequently developed Giardia infection, but 108 did not. Among the 355 subjects who denied kiddy pool exposure, 14 developed Giardia infection, and the other 341 did not.
|
|
|
|
|
| 16 | 108 | 124 | 16/124 = 12.9% |
| 14 | 341 | 365 | 14/365 = 3,9% |
Organization of the data this way makes it easier to compute the cumulative incidence in each group (12.9% and 3.9% respectively). The incidence in each group provides an estimate of risk, and the groups can be compared in order to estimate the magnitude of association. (This will be addressed in much greater detail in the module on Measures of Association.) One way of quantifying the association is to calculate the relative risk, i.e., dividing the incidence in the exposed group by the incidence in the unexposed group). In this case, the risk ratio is (12.9% / 3.9%) = 3.3. This suggest that subjects who swam in the kiddy pool had 3.3 times the risk of getting Giardia infections compared to those who did not, suggesting that the kiddy pool was the source.
Unanswered Questions
If the kiddy pool was the source of contamination responsible for this outbreak, why was it that:
- Only 16 people exposed to the kiddy pool developed the infection?
- There were 14 Giardia cases among people who denied exposure to the kiddy pool?
Before you look at the answer, think about it and try to come up with a possible explanation.
Likely Explanation
Optional Links of Potential Interest
Link to the 2003 Giardia outbreak
Link to CDC page on Organizing Data

Possible Pitfall: Contingency tables can be oriented in several ways, and this can cause confusion when calculating measures of association.
There is no standard rule about how to set up contingency tables, and you will see them set up in different ways.
- With exposure status in rows and outcome status in columns
- With exposure status in columns and outcome status in rows
- With exposed group first followed by non-exposed group
- With non-exposed group first followed by exposed group
If you aren't careful, these different orientations can result in errors in calculating measures of association. One way to avoid confusion is to always set up your contingency tables in the same way. For example, in these learning modules the contingency tables almost always indicate outcome status in columns listing subjects who have the outcome of interest to the left of subjects who do not have the outcome, and exposure status of the exposed (or most exposed) group is listed in a row above those who are unexposed (or have less exposure).
The table below illustrates this arrangement.
| Those With the Outcome | Those Without the Outcome | Total |
Exposed (or most exposed) |
|
|
|
Non-exposed (or least exposed) |
|
|
|
Case-Control Studies
Cohort studies have an intuitive logic to them, but they can be very problematic when:
- The outcomes being investigated are rare;
- There is a long time period between the exposure of interest and the development of the disease; or
- It is expensive or very difficult to obtain exposure information from a cohort.
In the first case, the rarity of the disease requires enrollment of very large numbers of people. In the second case, the long period of follow-up requires efforts to keep contact with and collect outcome information from individuals. In all three situations, cost and feasibility become an important concern.
A case-control design offers an alternative that is much more efficient. The goal of a case-control study is the same as that of cohort studies, i.e. to estimate the magnitude of association between an exposure and an outcome. However, case-control studies employ a different sampling strategy that gives them greater efficiency. As with a cohort study, a case-control study attempts to identify all people who have developed the disease of interest in the defined population. This is not because they are inherently more important to estimating an association, but because they are almost always rarer than non-diseased individuals, and one of the requirements of accurate estimation of the association is that there are reasonable numbers of people in both the numerators (cases) and denominators (people or person-time) in the measures of disease frequency for both exposed and reference groups. However, because most of the denominator is made up of people who do not develop disease, the case-control design avoids the need to collect information on the entire population by selecting a sample of the underlying population.
Rothman describes the case-control strategy as follows:
"Case-control studies are best understood by considering as the starting point a , which represents a hypothetical study population in which a cohort study might have been conducted. The is the population that gives rise to the cases included in the study. If a cohort study were undertaken, we would define the exposed and unexposed cohorts (or several cohorts) and from these populations obtain denominators for the incidence rates or risks that would be calculated for each cohort. We would then identify the number of cases occurring in each cohort and calculate the risk or incidence rate for each. In a case-control study the same cases are identified and classified as to whether they belong to the exposed or unexposed cohort. Instead of obtaining the denominators for the rates or risks, however, a control group is sampled from the entire source population that gives rise to the cases. Individuals in the control group are then classified into exposed and unexposed categories. The purpose of the control group is to determine the relative size of the exposed and unexposed components of the source population." |
To illustrate this consider the following hypothetical scenario in which the source population is Plymouth County in Massachusetts, which has a total population of 6,647 (hypothetical). Thirteen people in the county have been diagnosed with an unusual disease and seven of them have a particular exposure that is suspected of being an important contributing factor. The chief problem here is that the disease is quite rare.

If I somehow had exposure and outcome information on all of the subjects in the source population and looked at the association using a cohort design, it might look like this:
| Diseased | Non-diseased | Total |
---|---|---|---|
Exposed | 7 | 1,000 | 1,007 |
Non-exposed | 6 | 5,634 | 5,640 |
Therefore, the incidence in the exposed individuals would be 7/1,007 = 0.70%, and the incidence in the non-exposed individuals would be 6/5,640 = 0.11%. Consequently, the risk ratio would be 0.70/0.11=6.52, suggesting that those who had the risk factor (exposure) had 6.5 times the risk of getting the disease compared to those without the risk factor. This is a strong association.
In this hypothetical example, I had data on all 6,647 people in the source population, and I could compute the probability of disease (i.e., the risk or incidence) in both the exposed group and the non-exposed group, because I had the denominators for both the exposed and non-exposed groups.
The problem , of course, is that I usually don't have the resources to get the data on all subjects in the population. If I took a random sample of even 5-10% of the population, I might not have any diseased people in my sample.
An alternative approach would be to use surveillance databases or administrative databases to find most or all 13 of the cases in the source population and determine their exposure status. However, instead of enrolling all of the other 5,634 residents, suppose I were to just take a sample of the non-diseased population. In fact, suppose I only took a sample of 1% of the non-diseased people and I then determined their exposure status. The data might look something like this:
| Diseased | Non-diseased | Total |
---|---|---|---|
Exposed | 7 | 10 | unknown |
Non-exposed | 6 | 56 | unknown |
With this sampling approach I can no longer compute the probability of disease in each exposure group, because I no longer have the denominators in the last column. In other words, I don't know the exposure distribution for the entire source population. However, the small control sample of non-diseased subjects gives me a way to estimate the exposure distribution in the source population. So, I can't compute the probability of disease in each exposure group, but I can compute the odds of disease in the case-control sample.
The Odds Ratio
The odds of disease among the exposed sample are 7/10, and the odds of disease in the non-exposed sample are 6/56. If I compute the odds ratio, I get (7/10) / (5/56) = 6.56, very close to the risk ratio that I computed from data for the entire population. We will consider odds ratios and case-control studies in much greater depth in a later module. However, for the time being the key things to remember are that:
- The sampling strategy for a case-control study is very different from that of cohort studies, despite the fact that both have the goal of estimating the magnitude of association between the exposure and the outcome.
- In a case-control study there is no "follow-up" period. One starts by identifying diseased subjects and determines their exposure distribution; one then takes a sample of the source population that produced those cases in order to estimate the exposure distribution in the overall source population that produced the cases. [In cohort studies none of the subjects have the outcome at the beginning of the follow-up period.]
- In a case-control study, you cannot measure incidence, because you start with diseased people and non-diseased people, so you cannot calculate relative risk.
- The case-control design is very efficient. In the example above the case-control study of only 79 subjects produced an odds ratio (6.56) that was a very close approximation to the risk ratio (6.52) that was obtained from the data in the entire population.
- Case-control studies are particularly useful when the outcome is rare is uncommon in both exposed and non-exposed people.
The Difference Between "Probability" and "Odds"?

- The odds are defined as the probability that the event will occur divided by the probability that the event will not occur.
If the probability of an event occurring is Y, then the probability of the event not occurring is 1-Y. (Example: If the probability of an event is 0.80 (80%), then the probability that the event will not occur is 1-0.80 = 0.20, or 20%.
The odds of an event represent the ratio of the (probability that the event will occur) / (probability that the event will not occur). This could be expressed as follows:
Odds of event = Y / (1-Y)
So, in this example, if the probability of the event occurring = 0.80, then the odds are 0.80 / (1-0.80) = 0.80/0.20 = 4 (i.e., 4 to 1).
- If a race horse runs 100 races and wins 25 times and loses the other 75 times, the probability of winning is 25/100 = 0.25 or 25%, but the odds of the horse winning are 25/75 = 0.333 or 1 win to 3 loses.
- If the horse runs 100 races and wins 5 and loses the other 95 times, the probability of winning is 0.05 or 5%, and the odds of the horse winning are 5/95 = 0.0526.
- If the horse runs 100 races and wins 50, the probability of winning is 50/100 = 0.50 or 50%, and the odds of winning are 50/50 = 1 (even odds).
- If the horse runs 100 races and wins 80, the probability of winning is 80/100 = 0.80 or 80%, and the odds of winning are 80/20 = 4 to 1.
NOTE that when the probability is low, the odds and the probability are very similar.
On Sept. 8, 2011 the New York Times ran an article on the economy in which the writer began by saying "If history is a guide, the odds that the American economy is falling into a double-dip recession have risen sharply in recent weeks and may even have reached 50 percent." Further down in the article the author quoted the economist who had been interviewed for the story. What the economist had actually said was, "Whether we reach the technical definition [of a double-dip recession] I think is probably close to 50-50."
Question: Was the author correct in saying that the "odds" of a double-dip recession may have reached 50 percent?
Key Concept: In a study that is designed and conducted as a case-control study, you cannot calculate incidence. Therefore, you cannot calculate risk ratio or risk difference. You can only calculate an odds ratio. However, in certain situations a case-control study is the only feasible study design. |
Which Study Design Is Best?
Decisions regarding which study design to use rest on a number of factors including::
- Uncommon Outcome: If the outcome of interest is uncommon or rare, a case-control study would usually be best.
- Uncommon Exposure: When studying an uncommon exposure, the investigators need to enroll an adequate number of subjects who have that exposure. In this situation a cohort study is best.
- Ethics of Assigning Subjects to an Exposure: If you wanted to study the association between smoking and lung cancer, It wouldn't be ethical to conduct a clinical trial in which you randomly assigned half of the subjects to smoking.
- Resources: If you have limited time, money, and personnel to gather data, it is unlikely that you will be able to conduct a prospective cohort study. A case-control study or a retrospective cohort study would be better options. The best one to choose would be dictated by whether the outcome was rare or the exposure of interest was rare.
There are some situations in which more than one study design could be used.
Smoking and Lung Cancer: For example, when investigators first sought to establish whether there was a link between smoking and lung cancer, they did a study by finding hospital subjects who had lung cancer and a comparison group of hospital patients who had diseases other than cancer. They then compared the prior exposure histories with respect to smoking and many other factors. They found that past smoking was much more common in the lung cancer cases, and they concluded that there was an association. The advantages to this approach were that they were able to collect the data they wanted relatively quickly and inexpensively, because they started with people who already had the disease of interest.
The short video below provides a nice overview of epidemiological studies.

However, there were several limitations to the study they had done. The study design did not allow them to measure the incidence of lung cancer in smokers and non-smokers, so they couldn't measure the absolute risk of smoking. They also didn't know what other diseases smoking might be associated with, and, finally, they were concerned about some of the biases that can creep into this type of study.
As a result, these investigators then initiated another study. They invited all of the male physicians in the United Kingdom to fill out questionnaires regarding their health status and their smoking status. They then focused on the healthy physicians who were willing to participate, and the investigators mailed follow-up questionnaires to them every few years. They also had a way of finding out the cause of death for any subjects who became ill and died. The study continued for about 50 years. Along the way the investigators periodically compared the incidence of death among non-smoking physicians and physicians who smoked small, moderate or heavy amounts of tobacco.
These studies were useful, because they were able to demonstrate that smokers had an increased risk of over 20 different causes of death. They were also able to measure the incidence of death in different categories, so they knew the absolute risk for each cause of death. Of course, the downside to this approach was that it took a long time, and it was very costly. So, both a case-control study and a prospective cohort study provided useful information about the association between smoking and lung cancer and other diseases, but there were distinct advantages and limitations to each approach.
Hepatitis Outbreak in Marshfield, MA
In 2004 there was an outbreak of hepatitis A on the South Shore of Massachusetts. Over a period of a few weeks there were 20 cases of hepatitis A that were reported to the MDPH, and most of the infected persons were residents of Marshfield, MA. Marshfield's health department requested help in identifying the source from MDPH. The investigators quickly performed descriptive epidemiology. The epidemic curve indicated a point source epidemic, and most of the cases lived in the Marshfield area, although some lived as far away as Boston. They conducted hypothesis-generating interviews, and taken together, the descriptive epidemiology suggested that the source was one of five or six food establishments in the Marshfield area, but it wasn't clear which one. Consequently, the investigators wanted to conduct an analytic study to determine which restaurant was the source. Which study design should have been conducted? Think about the scenario, and then open the "Quiz Me" below and choose your answer.
Link to more on the hepatitis outbreak
Case-control studies are particularly efficient for rare diseases because they begin by identifying a sufficient number of diseased people (or people have some "outcome" of interest) to enable you to do an analysis that tests associations. Case-control studies can be done in just about any circumstance, but they are particularly useful when you are dealing with rare diseases or disease for which there is a very long latent period, i.e. a long time between the causative exposure and the eventual development of disease.
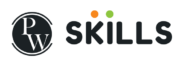
Data Analysis Techniques in Research – Methods, Tools & Examples
Varun Saharawat is a seasoned professional in the fields of SEO and content writing. With a profound knowledge of the intricate aspects of these disciplines, Varun has established himself as a valuable asset in the world of digital marketing and online content creation.
Data analysis techniques in research are essential because they allow researchers to derive meaningful insights from data sets to support their hypotheses or research objectives.
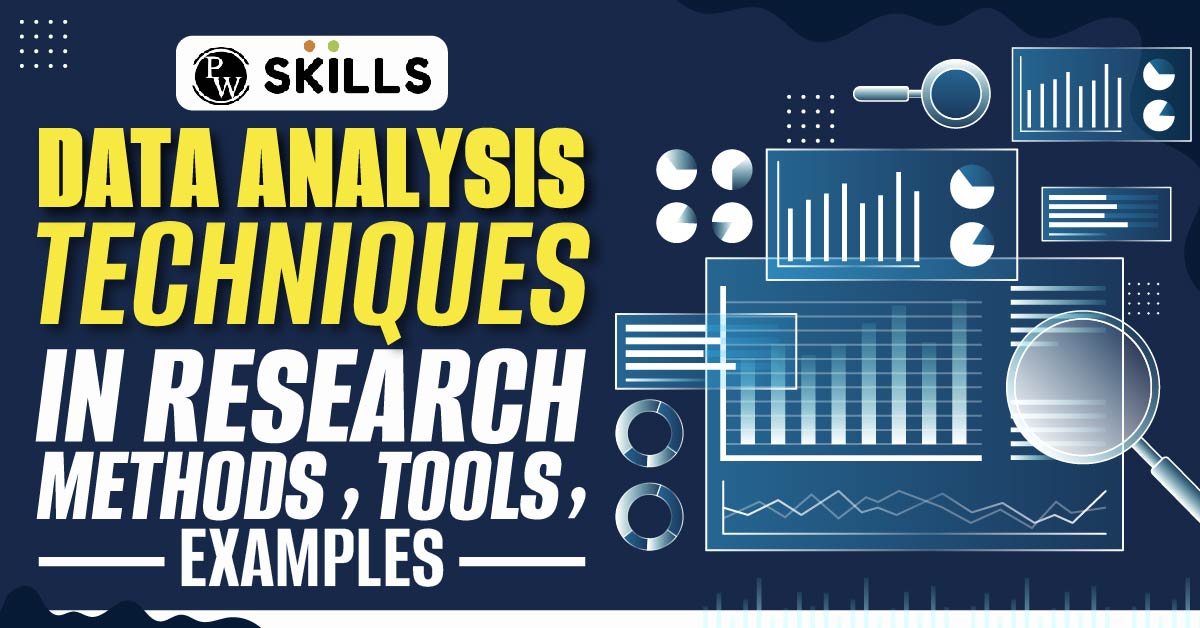
Data Analysis Techniques in Research : While various groups, institutions, and professionals may have diverse approaches to data analysis, a universal definition captures its essence. Data analysis involves refining, transforming, and interpreting raw data to derive actionable insights that guide informed decision-making for businesses.
A straightforward illustration of data analysis emerges when we make everyday decisions, basing our choices on past experiences or predictions of potential outcomes.
If you want to learn more about this topic and acquire valuable skills that will set you apart in today’s data-driven world, we highly recommend enrolling in the Data Analytics Course by Physics Wallah . And as a special offer for our readers, use the coupon code “READER” to get a discount on this course.
Table of Contents
What is Data Analysis?
Data analysis is the systematic process of inspecting, cleaning, transforming, and interpreting data with the objective of discovering valuable insights and drawing meaningful conclusions. This process involves several steps:
- Inspecting : Initial examination of data to understand its structure, quality, and completeness.
- Cleaning : Removing errors, inconsistencies, or irrelevant information to ensure accurate analysis.
- Transforming : Converting data into a format suitable for analysis, such as normalization or aggregation.
- Interpreting : Analyzing the transformed data to identify patterns, trends, and relationships.
Types of Data Analysis Techniques in Research
Data analysis techniques in research are categorized into qualitative and quantitative methods, each with its specific approaches and tools. These techniques are instrumental in extracting meaningful insights, patterns, and relationships from data to support informed decision-making, validate hypotheses, and derive actionable recommendations. Below is an in-depth exploration of the various types of data analysis techniques commonly employed in research:
1) Qualitative Analysis:
Definition: Qualitative analysis focuses on understanding non-numerical data, such as opinions, concepts, or experiences, to derive insights into human behavior, attitudes, and perceptions.
- Content Analysis: Examines textual data, such as interview transcripts, articles, or open-ended survey responses, to identify themes, patterns, or trends.
- Narrative Analysis: Analyzes personal stories or narratives to understand individuals’ experiences, emotions, or perspectives.
- Ethnographic Studies: Involves observing and analyzing cultural practices, behaviors, and norms within specific communities or settings.
2) Quantitative Analysis:
Quantitative analysis emphasizes numerical data and employs statistical methods to explore relationships, patterns, and trends. It encompasses several approaches:
Descriptive Analysis:
- Frequency Distribution: Represents the number of occurrences of distinct values within a dataset.
- Central Tendency: Measures such as mean, median, and mode provide insights into the central values of a dataset.
- Dispersion: Techniques like variance and standard deviation indicate the spread or variability of data.
Diagnostic Analysis:
- Regression Analysis: Assesses the relationship between dependent and independent variables, enabling prediction or understanding causality.
- ANOVA (Analysis of Variance): Examines differences between groups to identify significant variations or effects.
Predictive Analysis:
- Time Series Forecasting: Uses historical data points to predict future trends or outcomes.
- Machine Learning Algorithms: Techniques like decision trees, random forests, and neural networks predict outcomes based on patterns in data.
Prescriptive Analysis:
- Optimization Models: Utilizes linear programming, integer programming, or other optimization techniques to identify the best solutions or strategies.
- Simulation: Mimics real-world scenarios to evaluate various strategies or decisions and determine optimal outcomes.
Specific Techniques:
- Monte Carlo Simulation: Models probabilistic outcomes to assess risk and uncertainty.
- Factor Analysis: Reduces the dimensionality of data by identifying underlying factors or components.
- Cohort Analysis: Studies specific groups or cohorts over time to understand trends, behaviors, or patterns within these groups.
- Cluster Analysis: Classifies objects or individuals into homogeneous groups or clusters based on similarities or attributes.
- Sentiment Analysis: Uses natural language processing and machine learning techniques to determine sentiment, emotions, or opinions from textual data.
Also Read: AI and Predictive Analytics: Examples, Tools, Uses, Ai Vs Predictive Analytics

Data Analysis Techniques in Research Examples
To provide a clearer understanding of how data analysis techniques are applied in research, let’s consider a hypothetical research study focused on evaluating the impact of online learning platforms on students’ academic performance.
Research Objective:
Determine if students using online learning platforms achieve higher academic performance compared to those relying solely on traditional classroom instruction.
Data Collection:
- Quantitative Data: Academic scores (grades) of students using online platforms and those using traditional classroom methods.
- Qualitative Data: Feedback from students regarding their learning experiences, challenges faced, and preferences.
Data Analysis Techniques Applied:
1) Descriptive Analysis:
- Calculate the mean, median, and mode of academic scores for both groups.
- Create frequency distributions to represent the distribution of grades in each group.
2) Diagnostic Analysis:
- Conduct an Analysis of Variance (ANOVA) to determine if there’s a statistically significant difference in academic scores between the two groups.
- Perform Regression Analysis to assess the relationship between the time spent on online platforms and academic performance.
3) Predictive Analysis:
- Utilize Time Series Forecasting to predict future academic performance trends based on historical data.
- Implement Machine Learning algorithms to develop a predictive model that identifies factors contributing to academic success on online platforms.
4) Prescriptive Analysis:
- Apply Optimization Models to identify the optimal combination of online learning resources (e.g., video lectures, interactive quizzes) that maximize academic performance.
- Use Simulation Techniques to evaluate different scenarios, such as varying student engagement levels with online resources, to determine the most effective strategies for improving learning outcomes.
5) Specific Techniques:
- Conduct Factor Analysis on qualitative feedback to identify common themes or factors influencing students’ perceptions and experiences with online learning.
- Perform Cluster Analysis to segment students based on their engagement levels, preferences, or academic outcomes, enabling targeted interventions or personalized learning strategies.
- Apply Sentiment Analysis on textual feedback to categorize students’ sentiments as positive, negative, or neutral regarding online learning experiences.
By applying a combination of qualitative and quantitative data analysis techniques, this research example aims to provide comprehensive insights into the effectiveness of online learning platforms.
Also Read: Learning Path to Become a Data Analyst in 2024
Data Analysis Techniques in Quantitative Research
Quantitative research involves collecting numerical data to examine relationships, test hypotheses, and make predictions. Various data analysis techniques are employed to interpret and draw conclusions from quantitative data. Here are some key data analysis techniques commonly used in quantitative research:
1) Descriptive Statistics:
- Description: Descriptive statistics are used to summarize and describe the main aspects of a dataset, such as central tendency (mean, median, mode), variability (range, variance, standard deviation), and distribution (skewness, kurtosis).
- Applications: Summarizing data, identifying patterns, and providing initial insights into the dataset.
2) Inferential Statistics:
- Description: Inferential statistics involve making predictions or inferences about a population based on a sample of data. This technique includes hypothesis testing, confidence intervals, t-tests, chi-square tests, analysis of variance (ANOVA), regression analysis, and correlation analysis.
- Applications: Testing hypotheses, making predictions, and generalizing findings from a sample to a larger population.
3) Regression Analysis:
- Description: Regression analysis is a statistical technique used to model and examine the relationship between a dependent variable and one or more independent variables. Linear regression, multiple regression, logistic regression, and nonlinear regression are common types of regression analysis .
- Applications: Predicting outcomes, identifying relationships between variables, and understanding the impact of independent variables on the dependent variable.
4) Correlation Analysis:
- Description: Correlation analysis is used to measure and assess the strength and direction of the relationship between two or more variables. The Pearson correlation coefficient, Spearman rank correlation coefficient, and Kendall’s tau are commonly used measures of correlation.
- Applications: Identifying associations between variables and assessing the degree and nature of the relationship.
5) Factor Analysis:
- Description: Factor analysis is a multivariate statistical technique used to identify and analyze underlying relationships or factors among a set of observed variables. It helps in reducing the dimensionality of data and identifying latent variables or constructs.
- Applications: Identifying underlying factors or constructs, simplifying data structures, and understanding the underlying relationships among variables.
6) Time Series Analysis:
- Description: Time series analysis involves analyzing data collected or recorded over a specific period at regular intervals to identify patterns, trends, and seasonality. Techniques such as moving averages, exponential smoothing, autoregressive integrated moving average (ARIMA), and Fourier analysis are used.
- Applications: Forecasting future trends, analyzing seasonal patterns, and understanding time-dependent relationships in data.
7) ANOVA (Analysis of Variance):
- Description: Analysis of variance (ANOVA) is a statistical technique used to analyze and compare the means of two or more groups or treatments to determine if they are statistically different from each other. One-way ANOVA, two-way ANOVA, and MANOVA (Multivariate Analysis of Variance) are common types of ANOVA.
- Applications: Comparing group means, testing hypotheses, and determining the effects of categorical independent variables on a continuous dependent variable.
8) Chi-Square Tests:
- Description: Chi-square tests are non-parametric statistical tests used to assess the association between categorical variables in a contingency table. The Chi-square test of independence, goodness-of-fit test, and test of homogeneity are common chi-square tests.
- Applications: Testing relationships between categorical variables, assessing goodness-of-fit, and evaluating independence.
These quantitative data analysis techniques provide researchers with valuable tools and methods to analyze, interpret, and derive meaningful insights from numerical data. The selection of a specific technique often depends on the research objectives, the nature of the data, and the underlying assumptions of the statistical methods being used.
Also Read: Analysis vs. Analytics: How Are They Different?
Data Analysis Methods
Data analysis methods refer to the techniques and procedures used to analyze, interpret, and draw conclusions from data. These methods are essential for transforming raw data into meaningful insights, facilitating decision-making processes, and driving strategies across various fields. Here are some common data analysis methods:
- Description: Descriptive statistics summarize and organize data to provide a clear and concise overview of the dataset. Measures such as mean, median, mode, range, variance, and standard deviation are commonly used.
- Description: Inferential statistics involve making predictions or inferences about a population based on a sample of data. Techniques such as hypothesis testing, confidence intervals, and regression analysis are used.
3) Exploratory Data Analysis (EDA):
- Description: EDA techniques involve visually exploring and analyzing data to discover patterns, relationships, anomalies, and insights. Methods such as scatter plots, histograms, box plots, and correlation matrices are utilized.
- Applications: Identifying trends, patterns, outliers, and relationships within the dataset.
4) Predictive Analytics:
- Description: Predictive analytics use statistical algorithms and machine learning techniques to analyze historical data and make predictions about future events or outcomes. Techniques such as regression analysis, time series forecasting, and machine learning algorithms (e.g., decision trees, random forests, neural networks) are employed.
- Applications: Forecasting future trends, predicting outcomes, and identifying potential risks or opportunities.
5) Prescriptive Analytics:
- Description: Prescriptive analytics involve analyzing data to recommend actions or strategies that optimize specific objectives or outcomes. Optimization techniques, simulation models, and decision-making algorithms are utilized.
- Applications: Recommending optimal strategies, decision-making support, and resource allocation.
6) Qualitative Data Analysis:
- Description: Qualitative data analysis involves analyzing non-numerical data, such as text, images, videos, or audio, to identify themes, patterns, and insights. Methods such as content analysis, thematic analysis, and narrative analysis are used.
- Applications: Understanding human behavior, attitudes, perceptions, and experiences.
7) Big Data Analytics:
- Description: Big data analytics methods are designed to analyze large volumes of structured and unstructured data to extract valuable insights. Technologies such as Hadoop, Spark, and NoSQL databases are used to process and analyze big data.
- Applications: Analyzing large datasets, identifying trends, patterns, and insights from big data sources.
8) Text Analytics:
- Description: Text analytics methods involve analyzing textual data, such as customer reviews, social media posts, emails, and documents, to extract meaningful information and insights. Techniques such as sentiment analysis, text mining, and natural language processing (NLP) are used.
- Applications: Analyzing customer feedback, monitoring brand reputation, and extracting insights from textual data sources.
These data analysis methods are instrumental in transforming data into actionable insights, informing decision-making processes, and driving organizational success across various sectors, including business, healthcare, finance, marketing, and research. The selection of a specific method often depends on the nature of the data, the research objectives, and the analytical requirements of the project or organization.
Also Read: Quantitative Data Analysis: Types, Analysis & Examples
Data Analysis Tools
Data analysis tools are essential instruments that facilitate the process of examining, cleaning, transforming, and modeling data to uncover useful information, make informed decisions, and drive strategies. Here are some prominent data analysis tools widely used across various industries:
1) Microsoft Excel:
- Description: A spreadsheet software that offers basic to advanced data analysis features, including pivot tables, data visualization tools, and statistical functions.
- Applications: Data cleaning, basic statistical analysis, visualization, and reporting.
2) R Programming Language :
- Description: An open-source programming language specifically designed for statistical computing and data visualization.
- Applications: Advanced statistical analysis, data manipulation, visualization, and machine learning.
3) Python (with Libraries like Pandas, NumPy, Matplotlib, and Seaborn):
- Description: A versatile programming language with libraries that support data manipulation, analysis, and visualization.
- Applications: Data cleaning, statistical analysis, machine learning, and data visualization.
4) SPSS (Statistical Package for the Social Sciences):
- Description: A comprehensive statistical software suite used for data analysis, data mining, and predictive analytics.
- Applications: Descriptive statistics, hypothesis testing, regression analysis, and advanced analytics.
5) SAS (Statistical Analysis System):
- Description: A software suite used for advanced analytics, multivariate analysis, and predictive modeling.
- Applications: Data management, statistical analysis, predictive modeling, and business intelligence.
6) Tableau:
- Description: A data visualization tool that allows users to create interactive and shareable dashboards and reports.
- Applications: Data visualization , business intelligence , and interactive dashboard creation.
7) Power BI:
- Description: A business analytics tool developed by Microsoft that provides interactive visualizations and business intelligence capabilities.
- Applications: Data visualization, business intelligence, reporting, and dashboard creation.
8) SQL (Structured Query Language) Databases (e.g., MySQL, PostgreSQL, Microsoft SQL Server):
- Description: Database management systems that support data storage, retrieval, and manipulation using SQL queries.
- Applications: Data retrieval, data cleaning, data transformation, and database management.
9) Apache Spark:
- Description: A fast and general-purpose distributed computing system designed for big data processing and analytics.
- Applications: Big data processing, machine learning, data streaming, and real-time analytics.
10) IBM SPSS Modeler:
- Description: A data mining software application used for building predictive models and conducting advanced analytics.
- Applications: Predictive modeling, data mining, statistical analysis, and decision optimization.
These tools serve various purposes and cater to different data analysis needs, from basic statistical analysis and data visualization to advanced analytics, machine learning, and big data processing. The choice of a specific tool often depends on the nature of the data, the complexity of the analysis, and the specific requirements of the project or organization.
Also Read: How to Analyze Survey Data: Methods & Examples
Importance of Data Analysis in Research
The importance of data analysis in research cannot be overstated; it serves as the backbone of any scientific investigation or study. Here are several key reasons why data analysis is crucial in the research process:
- Data analysis helps ensure that the results obtained are valid and reliable. By systematically examining the data, researchers can identify any inconsistencies or anomalies that may affect the credibility of the findings.
- Effective data analysis provides researchers with the necessary information to make informed decisions. By interpreting the collected data, researchers can draw conclusions, make predictions, or formulate recommendations based on evidence rather than intuition or guesswork.
- Data analysis allows researchers to identify patterns, trends, and relationships within the data. This can lead to a deeper understanding of the research topic, enabling researchers to uncover insights that may not be immediately apparent.
- In empirical research, data analysis plays a critical role in testing hypotheses. Researchers collect data to either support or refute their hypotheses, and data analysis provides the tools and techniques to evaluate these hypotheses rigorously.
- Transparent and well-executed data analysis enhances the credibility of research findings. By clearly documenting the data analysis methods and procedures, researchers allow others to replicate the study, thereby contributing to the reproducibility of research findings.
- In fields such as business or healthcare, data analysis helps organizations allocate resources more efficiently. By analyzing data on consumer behavior, market trends, or patient outcomes, organizations can make strategic decisions about resource allocation, budgeting, and planning.
- In public policy and social sciences, data analysis is instrumental in developing and evaluating policies and interventions. By analyzing data on social, economic, or environmental factors, policymakers can assess the effectiveness of existing policies and inform the development of new ones.
- Data analysis allows for continuous improvement in research methods and practices. By analyzing past research projects, identifying areas for improvement, and implementing changes based on data-driven insights, researchers can refine their approaches and enhance the quality of future research endeavors.
However, it is important to remember that mastering these techniques requires practice and continuous learning. That’s why we highly recommend the Data Analytics Course by Physics Wallah . Not only does it cover all the fundamentals of data analysis, but it also provides hands-on experience with various tools such as Excel, Python, and Tableau. Plus, if you use the “ READER ” coupon code at checkout, you can get a special discount on the course.
For Latest Tech Related Information, Join Our Official Free Telegram Group : PW Skills Telegram Group
Data Analysis Techniques in Research FAQs
What are the 5 techniques for data analysis.
The five techniques for data analysis include: Descriptive Analysis Diagnostic Analysis Predictive Analysis Prescriptive Analysis Qualitative Analysis
What are techniques of data analysis in research?
Techniques of data analysis in research encompass both qualitative and quantitative methods. These techniques involve processes like summarizing raw data, investigating causes of events, forecasting future outcomes, offering recommendations based on predictions, and examining non-numerical data to understand concepts or experiences.
What are the 3 methods of data analysis?
The three primary methods of data analysis are: Qualitative Analysis Quantitative Analysis Mixed-Methods Analysis
What are the four types of data analysis techniques?
The four types of data analysis techniques are: Descriptive Analysis Diagnostic Analysis Predictive Analysis Prescriptive Analysis
- 10 Best Companies For Data Analysis Internships 2024
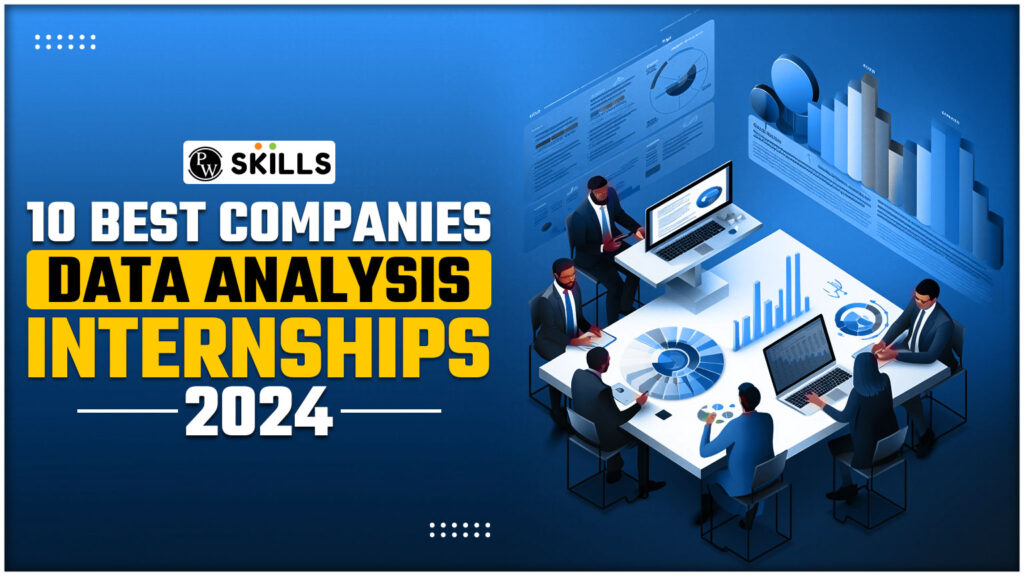
This article will help you provide the top 10 best companies for a Data Analysis Internship which will not only…
- Top Best Big Data Analytics Classes 2024
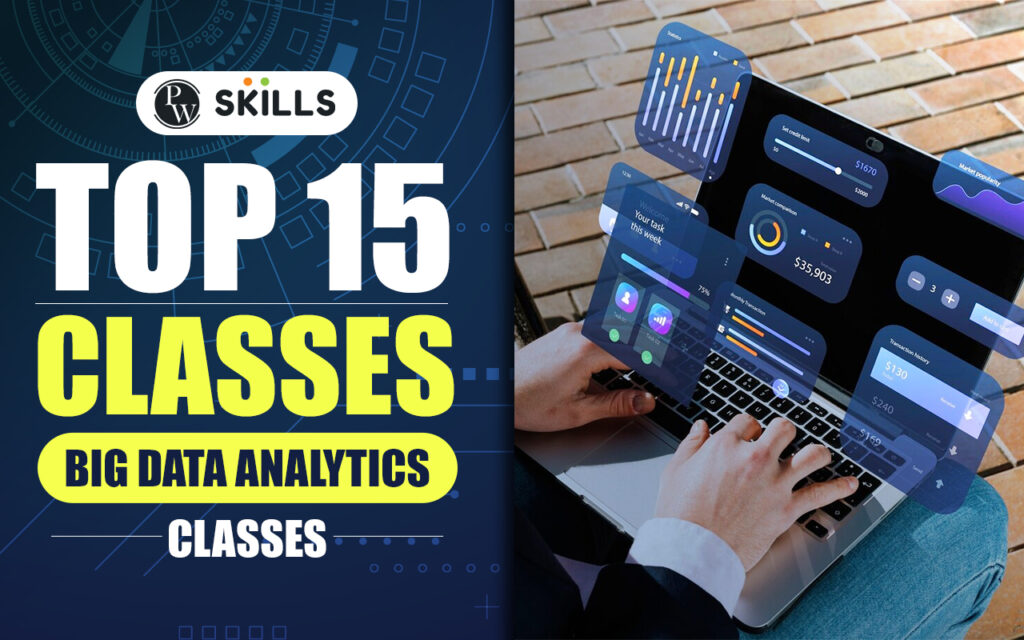
Many websites and institutions provide online remote big data analytics classes to help you learn and also earn certifications for…
- Data Analyst Roadmap 2024: Responsibilities, Skills Required, Career Path
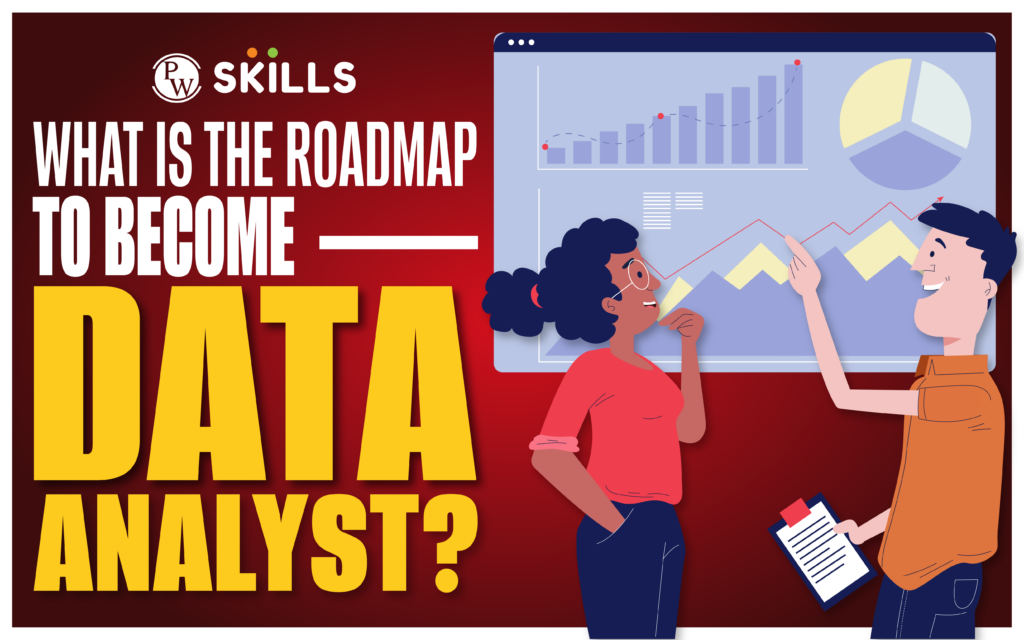
Data Analyst Roadmap: The field of data analysis is booming and is very rewarding for those with the right skills.…
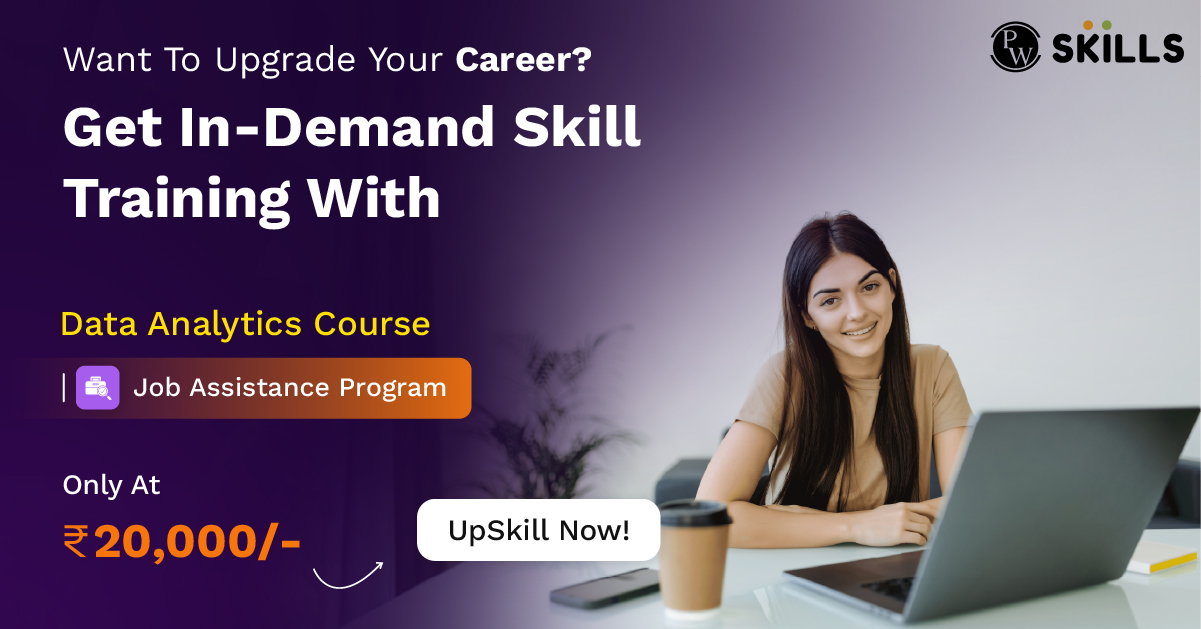
Related Articles
- The Best Data And Analytics Courses For Beginners
- Best Courses For Data Analytics: Top 10 Courses For Your Career in Trend
- BI & Analytics: What’s The Difference?
- Predictive Analysis: Predicting the Future with Data
- Graph Analytics – What Is it and Why Does It Matter?
- How to Analysis of Survey Data: Methods & Examples
- SQL For Data Analytics: A Comprehensive Guide
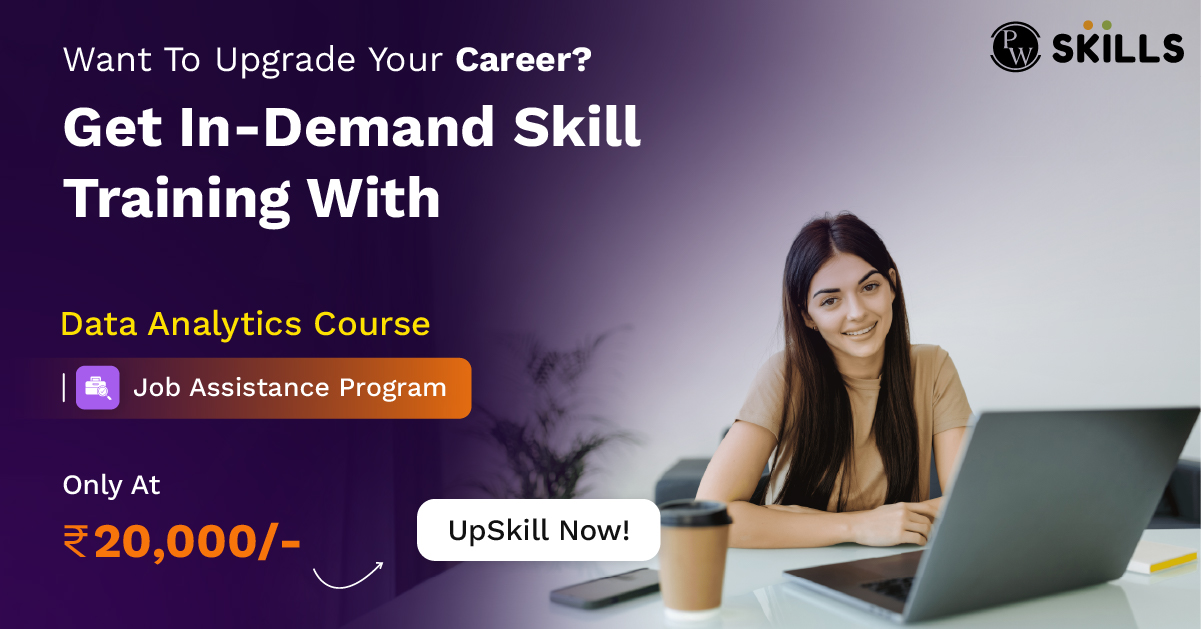
- Open access
- Published: 13 March 2022
The role of analytic direction in qualitative research
- Joanna E. M. Sale 1 , 2 , 3
BMC Medical Research Methodology volume 22 , Article number: 66 ( 2022 ) Cite this article
5588 Accesses
7 Citations
3 Altmetric
Metrics details
The literature on qualitative data analysis mostly concerns analyses pertaining to an individual research question and the organization of data within that research question. Few authors have written about the entire qualitative dataset from which multiple and separate analyses could be conducted and reported. The concept of analytic direction is a strategy that can assist qualitative researchers in deciding which findings to highlight within a dataset. The objectives of this paper were to: 1) describe the importance of analytic direction in qualitative research, and 2) provide a working example of the concept of analytic direction.
A qualitative dataset from one of the author’s research programs was selected for review. Ten potential analytic directions were identified after the initial phenomenological analysis was conducted. Three analytic directions based on the same coding template but different content areas of the data were further developed using phenomenological analysis ( n = 2) and qualitative description ( n = 1) and are the focus of this paper. Development and selection of these three analytic directions was determined partially relying on methodological criteria to promote rigour including a comprehensive examination of the data, the use of multiple analysts, direct quotations to support claims, negative case analysis, and reflexivity.
The three analytic directions addressed topics within the scope of the overall research question. Each analytic direction had its own central point or story line and each highlighted a different perspective or voice. The use of an inductive and deductive approach to analysis and how the role of theory was integrated varied in each analytic direction.
Conclusions
The concept of analytic direction enables researchers to organize their qualitative datasets in order to tell different and unique “stories”. The concept relies upon, and promotes, the conduct of rigourous qualitative research.
Peer Review reports
Reports on data analysis in qualitative research are well documented. Procedural steps have been described [ 1 , 2 , 3 , 4 , 5 , 6 , 7 ] and authors have made distinctions between the concepts of coding, analysis, and interpretation [ 1 , 2 , 8 , 9 ]. Authors have written about different researchers accessing different representations of a topic or phenomenon [ 2 , 10 ] or multiple interpretations being applied to the same transcript [ 11 ]. The literature on data analysis mostly concerns analyses pertaining to an individual research question and the organization of data within that research question. Few authors have written about the entire qualitative dataset from which multiple and separate analyses could be conducted and reported.
The data collected by qualitative researchers can be voluminous and often surpass the data pertaining to objectives outlined in grant proposals. These data may be compelling but analyses of some data are often given lower priority if they do not align directly with the stated objectives.
There comes a point during data collection and analysis where qualitative researchers must choose “which story, of the many stories available to them in a data set, to tell” (p. 376) [ 12 ]. According to Arthur Frank, “[a] fter the methods, there has to be a story” (p. 431) [ 13 ]. “Stories” should have a central point or storyline [ 12 ]. The final report can be told from the perspective of different voices [ 12 ] and organized by time such as emphasizing key turning points and milestones in the sequence of events studied [ 12 , 14 ] or by using other forms of representation such as metaphors [ 2 , 12 ]. Theory can be central or more peripheral in the account [ 15 ]. The question remains, what “story”, or “stories”, do we tell?
The concept of analytic direction
The concept of analytic direction is a strategy that can assist qualitative researchers in deciding which “stories” to highlight within a dataset. Sandelowski reports that researchers account for their data and then determine the different “paths” [ 1 ] or “analytic paths” [ 16 ] they can pursue. Others have proposed that decision-making throughout analysis implies analytic ideas at every stage of the coding process [ 8 ] and that researchers define for themselves what analytic issues are to be explored and what ideas are important [ 8 ]. Charmaz [ 17 ] reports that grounded theory researchers pursue more than one analytic direction by focusing on certain ideas first and then returning to the data to address an unfinished analysis in another area later. While the concept of analytic direction has been referenced, or alluded to, by these and other authors [ 1 , 8 , 16 , 18 , 19 ], operationalization of this concept is not well articulated. In this paper, the term analytic direction refers to a message developed by the researchers about the data that may or may not require further substantiation. An analytic direction can be presented as a single message or theme, and can stand alone or be supported by multiple sub-messages or sub-themes. Analytic directions can be developed during the coding process, in later stages of analysis, or possibly during analyses of new datasets. Relying on strategies to promote rigour can assist with the development, substantiation, and selection of analytic directions. If substantiated, each analytic direction could be the focus of an individual publication. The objectives of this paper were to: 1) describe the importance of analytic direction in qualitative research; and 2) provide a working example of the concept of analytic direction.
Why analytic direction is important
The concept of analytic direction is important because it has implications for methodological rigour. We have an obligation to conduct methodological rigourous studies [ 20 ], especially when studies require primary data collection that involves a burden to participants [ 21 ]. The author proposes that methodological rigour is embedded within, and contributes to, the concept of analytic direction. Several strategies to promote rigour that are universal to many qualitative approaches, including phenomenology, are discussed. These strategies include, but are not limited to, a comprehensive examination of the data, the use of multiple analysts, direct quotations to support claims, negative case analysis, and reflexivity. It is important to support the quality of analytic directions so that researchers can then determine which analytic directions may or may not require further substantiation. The quality of the analytic direction will also assist in determining which directions may be selected for reporting.
The relationship between analytic direction and methodological rigour
This paper focuses on the stage where data collection is considered to be complete and does not directly address how data collection, and methodological rigour related to data collection, contributes to the concept of analytic direction. The assumption is that data collection and analysis were conducted iteratively [ 22 , 23 ] and that the team decided when data collection was complete, perhaps relying upon one of the various conceptualizations of saturation discussed by Saunders and colleagues [ 24 ]. A decision about saturation would not necessarily apply to any, or all, analytic directions being developed.
The author proposes that several strategies for promoting rigour assist with the development and selection of analytic directions. One aspect of methodological rigour is that authors carry out a comprehensive examination of their data [ 5 , 25 ]. By thinking about, and engaging in, analytic direction, researchers are encouraged to attend to all of their data rather than attending only to data that interests them initially.
The use of multiple analysts promotes a comprehensive examination of the data [ 2 , 26 ] and thus, contributes to the concept of analytic direction. Different viewpoints lead to an enrichment of the analysis and can lead to a conceptual clarification of the interpretations [ 2 ]. Multiple viewpoints can be used at the level of coding but also at the level of the larger team as data collection and analysis proceeds. Discussions about the novelty, clinical significance, and relevance [ 27 ] of the analytic directions may occur at this time and continue through to the writing of the respective manuscripts. Analytic directions are relevant if they add knowledge, or increase the confidence with which existing knowledge is regarded [ 28 ]. According to Malterud [ 26 ], engaging multiple researchers in a qualitative study strengthens the design of the study, not for the purpose of consensus or identical readings of the data but to supplement and contest each others’ statements.
The use of direct quotations to support the claims made about the analytic directions (and/or themes within) is another strategy to promote rigour [ 29 ]. Not only do quotations illustrate and clarify the results but they also demonstrate whether there is substantive evidence to support the analytic directions being proposed. In contrast, data that do not support the analytic directions (and/or themes within) should be accounted for and their exclusion justified when promoting methodological rigour [ 30 ]. Authors may refer to this as attending to negative cases [ 28 ] or deviant case analysis [ 25 , 31 ]. This strategy promotes that “deviant cases” or “outliers” are not forced into categories or ignored but used instead to aid understanding or theory development [ 25 ]. For example, these cases may explain why the patterns developed from the data or the more normative behaviours are not always found in the researchers’ interpretations [ 25 , 31 ].
Reflexivity is an essential component of methodological rigour [ 26 ]. Reflexivity has been described as “an attitude of attending systematically to the context of knowledge construction, especially to the effect of the researcher, at every step of the research process” [ 26 ] (p. 484). Being reflexive means being aware of your own position in producing partial knowledge [ 32 ]. The qualitative researcher acknowledges his or her personal influence on what that partial knowledge is (for example, the data collected are dependent on the interviewer’s questions and prompts). According to Eakin and Gladstone [ 33 ], knowing one’s standpoint helps one to recognize the forces that might drive certain interpretations and stifle other conceptualizations of the data. Knowledge production is also partial because it is not possible to report all interpretations of the data and therefore, the research team has to decide what to report. Researchers engaging in the concept of analytic direction are more likely to be reflexive about what they are, and are not, reporting from their datasets.
Rationale for the chosen example
The dataset chosen for this example was from a study where the author and her team identified 10 potential analytic directions based on a compilation of the memos and team discussions pertaining to analysis and interpretation of the data. The publications developed from this dataset reflected the selection of three analytic directions that focused on different content areas [ 34 , 35 , 36 ]. The same coding template was the foundation for the three publications and the timing of the reporting was ordered based on the author’s interests. The author chose the dataset as an example primarily because it was not heavily theory-laden and therefore accessible to novice qualitative researchers. The resulting publications have practical implications for clinical and health services research and the process of developing these publications could inform graduate students who are embarking on a qualitative program of research for their thesis work.
Original research funded
The goal of the original research project was to reduce the burden of illness due to fracture through improved bone health investigation and treatment. Specifically, the aim was to examine what researchers could learn from members of a patient group. The study was approved by the Research Ethics Board at Unity Health Toronto (REB# 10–371). The study team consisted of scientists, clinicians, a policy maker, and a patient representative with expertise related to bone health. Informed by the Theory of Planned Behaviour [ 37 , 38 ], the team set out to examine members of a patient group to ask them about their intentions and actions toward bone health diagnosis and treatment and their experiences with diagnostic tests and treatment recommendations. All individuals ( n = 28) were 50+ years old and had sustained a fragility fracture. The overall project relied on a phenomenological approach conceptualized by Giorgi and Wertz [ 30 , 39 , 40 , 41 ].
We developed a master coding template of 27 broad codes that were designed to organize the data with minimal reliance on theory. The coding template was revised four times as data collection and analysis proceeded. The codes were developed from a combination of inductive and deductive codes. More specifically, inductive codes were developed from topics discussed in the interviews. Other codes were pre-specified from the overall aim of the original funded study and from the domains of the Theory of Planned Behaviour.
Development of analytic directions from the dataset
Qualitative researchers can use several strategies to develop analytic directions. The author started the organization process early in order to think about how best to maximize the data collected. Coding began after the first couple of interviews had been conducted; this is conventional advice for analysis in qualitative research [ 1 , 2 , 23 , 42 ]. As soon as the coding process began, a document specific to analysis was created. Miles and colleagues have referred to this as “analytic memoing” [ 6 ]. This document is different from other documents in which the team discusses design features, decisions, and interview logistics related to the study. Analytic ideas were added to this document after coding and discussing each transcript. The author engaged two individuals in the coding/analysis process, as multiple analysts promote a comprehensive examination of the data [ 2 , 26 ]. The author met regularly with members of the team during the process of data collection and analysis to discuss the data, interpretations of them, and different lines of inquiry. These discussions were recorded in the analysis document. Table 1 outlines the potential analytic directions considered for this paper. The 10 analytic directions were developed prior to publication of analytic direction #1. Some of these directions were posed as questions that required further analysis and substantiation. Tables were then created to help us to visualize patterns during analysis. As an example, for analytic direction #2, a table was created in which each participant was assigned a row and perceived messages from the various health care providers (for example, primary care providers and specialists) were placed in columns. Perceived messages were presented as quotations from participants. We examined the columns to compare perceived messages across provider groups for each participant and then examined the columns to compare the perceived messages within each provider group. For analytic direction #3, a table was created with each participant assigned a row and the domains of the Theory of Planned Behaviour assigned to columns. The table was populated with data in the form of quotations from each participant that we believed corresponded to each of the domains. Strategies such as matrices [ 5 , 6 ] or thematic maps [ 42 ] can also be used to visualize developing patterns when presenting or organizing data.
Selection of the three analytic directions
The number of analytic directions selected likely depends on circumstances including the quality of the data, the quality of the analysis, and available resources. The research team considered the multiple analytic directions, discussing their relevance [ 27 ], novelty, and clinical significance and also the interests of the team in order to incorporate the perspectives of the different stakeholders. It was important to the author that the content of each analytic direction was bounded in that it did not overlap with the content of the other analytic directions. For example, analytic directions #2 and #3 discuss the potential influence of others in participants’ lives. However, analytic direction #2 focused on health care providers while analytic direction #3 focused on family members, friends, and colleagues of participants and specifically excluded health care providers from the analysis based on the Theory of Planned Behaviour domain “subjective norm”. In narrowing down the list of analytic directions, the author ensured there were sufficient data (quotations) to support the claims. Cases that did not fit the general results were acknowledged in order to justify their exclusion or explain why they did not fit. For example, in analytic direction #3, we examined instances where the data did not appear to fit with the Theory of Planned Behaviour and explained what happened in these instances where the model did not appear to be predictive of intentions.
The master coding template was important as it assisted with the organization of evidence for each analytic direction. The master coding template also assisted the team with the creation of tables for each analytic direction discussed. Table 2 demonstrates the relationship between the master coding template and the three selected analytic directions.
The impetus for analytic direction #1 [ 34 ] was based on an assumption held by the author as she was working on the research proposal. Her expectation was that members of a patient group would be patient advocates who were experts in navigating for care. She was interested in what patients could learn from members of this patient group. The analytic direction for the paper came from surprise, and subsequent disappointment, that those assumptions were not supported by the data and that members of the patient group did not all appear to be advocates and experts in navigating for care. One commonality that defined the patient group was that members appeared to be in favour of taking prescribed medication.
Analytic direction #1 included elements of both inductive and deductive analysis in that codes were developed for the master coding template from the data (inductive) but the author’s expectations also influenced how those codes were combined and how the team interpreted the data (deductive). Drawing from the literature, the term “advocacy” was equated with the theoretical concept of “effective” or “activated” consumer [ 43 , 44 ]. The code “effective consumer” did not exist in the original master template, partly because we preferred to not apply theoretical labels prematurely to the data. Based on the coding template, we drew from six codes to create a table about “effective consumer” behaviours (see Table 2 ). Participants were then coded along a continuum between what was referred to as “few effective consumer behaviours” (patients who followed orders with minimal involvement in their care and demonstrating the least amount of advocacy) to “many effective consumer behaviours” (individuals demonstrating significant involvement in their care, those who demanded diagnostic testing and requested specific medications).
Analytic direction #2 [ 35 ] was developed concurrently with analytic direction #1. The role of theory was minimal in analytic direction #2 and perhaps implicit in the methodology of phenomenology which focuses on individuals’ experiences [ 23 , 39 ]. The impetus for analytic direction #2 was our proposal that messages from health care providers might determine individuals’ strategies or behaviours that were the focus of analytic direction #1. The analysis was more inductive than that of analytic direction #1 as the team had no pre-contemplated plan to examine how messages from health care providers might determine individuals’ behaviours. In conducting the analysis, the team wondered whether conflicts about what individuals did with the recommendations they received (their actions) appeared to be due to messages perceived across, and within, health care provider groups. Health care providers discussed in the interviews included clinic staff, primary care providers, specialists, nurses, physiotherapists, and chiropractors.
For analytic direction #2, we used seven of the codes in the master coding template (see Table 2 ). Five of these seven codes were also used in analytic direction #1 but for very different reasons and drawing from different data within these codes. We were interested in individuals’ understanding or interpretation of recommendations by health care providers, not how individuals interacted with health care providers or what they did with information received from health care providers. In other words, we were interested in the meaning of what health care providers reportedly said to participants and not what participants did with that information.
The publication for analytic direction #3 [ 36 ] was written 3 years after that for analytic direction #1. This was the author’s least preferred paper, despite the Theory of Planned Behaviour being the theoretical framework guiding the original funded research. Analytic direction #3 involved a primarily deductive analysis where the Theory of Planned Behaviour guided the coding and analysis. Because of the restrictions of forcing exploratory data from open-ended questions into pre-defined domains, the author selected a qualitative description approach for the research design.
Contrary to memos and reflexive notes documented by the author about the potential value of this analysis and whether the team had learned anything about the application of the Theory of Planned Behaviour in the context of our study, the pursuit of analytic direction #3 became an interesting methodological exercise for a number of reasons. We collected data on several behaviours including receiving diagnostic tests, taking supplements, exercising, attending falls prevention classes, and initiating medication. The author believed that one particular behaviour had to be selected for analysis which entailed examining the data for each of the behaviours in depth. The author chose to focus on medication initiation and/or medication use because of a longstanding interest in medication use. Also, there was sufficient data to substantiate the Theory of Planned Behaviour domains in relation to medication initiation and/or medication use. The Theory of Planned Behaviour did not appear to be particularly relevant to intentions to attend a bone mineral density test and there did not appear to be sufficient data to support any one of the non-pharmacological treatment strategies mentioned. The team also had to make decisions about what counted as “perceived behavioural control”, “subjective norms”, and “attitudes” which were the three domains of the Theory of Planned Behaviour [ 37 , 38 ]. In particular, participants’ discussions about medication side effects were problematic to conceptualize in reference to these domains. The team decided to code “ experiences with side effects” as “perceived behavioural control” but “ anticipated side effects” as an “attitude”.
For analytic direction #3, the team drew from five codes, three of which were pre-specified prior to analyzing the interviews and meant to capture the domains of the Theory of Planned Behaviour. The code “attitude to BMD testing” and “attitude to bone health treatment” were existing codes based on the Theory of Planned Behaviour. The code “subjective norm” was not part of the coding template because the team believed it was too specific. We instead examined the code “social influence” which captured a broader array of information about peers such as family members and friends. Similarly, “perceived behavioural control” was not part of the coding template because we found it too specific. Information for this domain was taken from another code labelled “bone health treatment” which captured data pertaining to participants’ medications, including past behaviour with medication and how difficult it was, or not, to take the medication. The code “intentions” was an existing code.
The three selected analytic directions varied in how the team used an inductive and deductive approach to analysis [ 15 , 45 ] and how the role of theory was integrated (“central” vs. more “peripheral”) [ 15 ]. Each publication was within the scope of the overall research goal or question. As proposed by Agee [ 46 ], this overall question offered the potential for more specific questions during analysis. Finally, each publication had its own central point [ 12 ] and highlighted a different perspective or voice [ 12 ].
The following is a summary of the three analytic directions labelled with the first few words of the titles of each publication (see Table 3 ).
Analytic direction #1 (Strategies used by a patient group; inductive and deductive-driven)
In this publication, we examined the strategies described by three groups of individuals: individuals demonstrating few effective consumer behaviours, individuals demonstrating many effective consumer behaviours, and individuals demonstrating both types of behaviours. We discussed how the continuum was contrary to our expectations of what behaviours members of a patient group would exhibit. Having acknowledged this finding, we reported that more than half of the participants described effective consumer behaviours including making requests of health care providers for referral to specialists, bone mineral density tests, and prescription medications. Our overall message was that members of a patient group described a range of effective consumer behaviours that could be incorporated as skill sets in post-fracture interventions.
Analytic direction #2 (Perceived messages about bone health; inductive-driven)
In this publication, we described the perceived messages across the different provider groups and then the perceived messages within each provider group. We reported that participants perceived that specialists were more interested in their bone health than general practitioners and that very few messages about bone health were perceived from other health care providers. We also reported that perceived messages about one’s bone health and recommendations for management across provider groups were inconsistent (for example, with regard to medication initiation). The message for analytic direction #2 was that patients perceived inconsistent messages within, and across, various healthcare providers, suggesting a need to raise awareness of bone health management guidelines to providers.
Analytic direction #3 (Theory of Planned Behaviour explains intentions to use medication; deductive-driven)
In this publication, we described the data in each domain of the Theory of Planned Behaviour and the apparent relationship between these domains and participants’ intentions with regard to medication use. Our message was that the Theory of Planned Behaviour appeared to be predictive of intentions to take prescribed medication in approximately three-quarters of participants and when it was not predictive, a positive attitude to medication was the most important domain in determining participants’ intentions.
This working example of analytic direction resulted in three publications highlighting distinct "stories”. The publications differed in a number of ways. Each publication had its own central point or story line [ 12 ]. The role of theory [ 15 ] was minimal in analytic direction #2 but was more central in analytic directions #1 and #3 with the concept of “effective” or “activated” consumer and the Theory of Planned Behaviour dominating the analyses, respectively. Acknowledging that the authentic voices of participants may always be manufactured by the authorial account [ 32 , 47 ], all papers were written from the perspective of “I” or “we”. However, we focused on participants at the forefront for analytic direction #1 and we focused on participants’ perceptions of their providers’ voices for analytic direction #2. For analytic direction #3, the voice of the research team dominated as we struggled with methodological decisions. It is proposed that the voice of the model (Theory of Planned Behaviour) also dominated in analytic direction #3.
One implication related to analytic direction is that the research team may need to modify elements of the original research design to better suit the analytic direction selected. If such a modification is made, the team should ensure theoretical consistency in how the methods and methodologies are integrated [ 48 , 49 ]. For example, Crotty [ 49 ] proposes that theoretical consistency is needed between methods, methodology, theoretical perspective, and epistemology because these four elements inform one another. Similarly, Carter and Little [ 48 ] argue that consistency between methods, methodology, and epistemology contribute to the rigour of a qualitative study. Authors should demonstrate that elements of their theoretical perspectives and research design are compatible if they are applying another methodological approach to the data. Carter and Little [ 48 ] suggest that methodologies can be combined or altered if the researcher retains a coherent epistemological position and justifies the choices made. In the funded grant, a phenomenological program of research was proposed and the data were collected through in-depth interviews conducted from a phenomenological perspective. Analytic direction #3 was not purely consistent with a phenomenological approach because of the restriction to force exploratory data into domains of a theoretical framework and so we pursued this analytic direction with a different approach (qualitative description). As pointed out by Sandelowski [ 50 ], using phenomenology and qualitative description in this way is not to be confused with misuses of methods or techniques. Unlike quantitative research, qualitative research is not produced from any “pure” use of a method, but from the use of methods that are variously textured, toned, and hued [ 50 ]. According to Sandelowski [ 50 ], qualitative description can be used in conjunction with phenomenological research in a number of ways. For example, phenomenological analyses can be applied to qualitative descriptive studies [ 50 ]. However, the pursuit of other approaches to analysis, such as grounded theory or a participatory action approach, might lead to epistemological tensions if the original study design and data collection was guided by a phenomenological approach. Future discussion about the concept of analytic direction when considering theoretical and methodological positions that differ epistemologically from the original design and conduct of the study is needed.
There are a number of other implications related to the concept of analytic direction. Practically, it is advised that researchers start to think about analytic directions early so that they are aware of the potential analytic directions being developed as soon as data collection and analysis begin. By thinking about the “larger picture” at this early stage in the research, the team is better equipped to make the most of the data collected. Having said this, one will likely never use the entire dataset. As researchers, we rarely have sufficient funds or personnel to pursue all analytic directions. Data are often set aside because researchers are eager to analyze data collected for new projects or pressured to seek future funding opportunities. Analytic directions that are not pursued can be transferred to student projects. Alternatively, it is possible to draw on a sub-set of the transcripts/observations to carry out a secondary analysis. The author has developed subsequent analytic directions that span across studies and draw from a subset of transcripts for several secondary analyses [ 51 , 52 , 53 ]. Analytic directions can also contribute to ideas for new grant proposals that enable the researcher to generate more data on analytic directions that need further substantiation and further exploration.
This paper demonstrates some guidance about how to bound each analytic direction. Bounding the analytic direction is necessary so one does not re-use the data or produce multiple, yet quite similar, papers on the same topic. Researchers are encouraged to be open and transparent and acknowledge related publications so reviewers and other audiences reading the work are able to determine for themselves that the analyses are different.
There are ethical considerations in developing an analytic direction or framing the analytic direction in a way that might be different or supplementary to the original design. It is not always feasible to obtain subsequent consent from participants for use of the data if this use differs from that of the original goal of the study. As a result, analytic directions pursued should be within the scope of the approved research ethics application. One strategy is to keep the study goal or aim broad in the research ethics submission so that it encompasses many topics that might be discussed during data collection. Another consideration is to not prematurely close a research ethics application because researchers may be able to use the data for a secondary analysis at a later date.
This paper makes novel contributions to qualitative research methodology by demonstrating how the process of analytic direction works, by operationalizing the concept and providing an example, and by describing the connection between analytic direction and rigour. This paper further contributes to the advancement of rigour by demonstrating how the development and selection of analytic directions relies on several strategies to promote rigour, such as a comprehensive examination of the data, the use of multiple analysts, providing quotations to support claims made, checking for negative cases, and reflexivity.
In conclusion, the concept of analytic direction enables researchers to organize their qualitative datasets in order to tell different and unique “stories”. The concept relies upon, and promotes, the conduct of rigourous qualitative research. As with all elements of qualitative analysis, researchers are encouraged to think about the role of analytic direction as soon as data collection commences.
Availability of data and materials
The datasets generated and/or analysed during the current study are not publicly available due to participants not consenting to having their data deposited in a public dataset but are available from the corresponding author on reasonable request.
Sandelowski M. Qualitative analysis: what it is and how to begin. ResNurs Health. 1995;18:371–5.
CAS Google Scholar
Kvale S. Interviews: an introduction to qualitative research interviewing. Thousand Oaks: Sage Publications; 1996.
Google Scholar
Saldana J. The coding manual for qualitative researchers. Los Angeles: Sage Publications; 2009.
Miller WL, Crabtree BF. The dance of interpretation. Doing qualitative research. Newbury Park: Sage Publications; 1999.
Spencer L, Ritchie J, O'Connor W. Analysis: practices, principles and processes. Qualitative research practice: a guide for social science students and researchers. Los Angeles: Sage Publications; 2003. p. 199–217.
Miles MB, Huberman AM, Saldana J. Qualitative data analysis. 3rd ed. Los Angeles: Sage Publications; 2014.
Crabtree BF, Miller WL. A template approach to text analysis: developing and using codebooks. Doing qualitative research, vol. 3. Newbury Park: Sage Publications; 1992. p. 93–109.
Coffey A, Atkinson P. Making sense of qualitative data: complementary research strategies. Thousand Oaks: Sage Publications; 1996.
Kelly M. The role of theory in qualitative health research. Fam Pract. 2010;27:285–90.
Article PubMed Google Scholar
Malterud K. Shared understanding of the qualitative research process. Guidelines for the medical researcher. Fam Pract. 1993;10(2):201–6.
Article CAS PubMed Google Scholar
Slaughter S, Dean Y, Knight H, Krieg B, Mor P, Nour V, et al. The inevitable pull of the river's current: interpretations derived from a single text using multiple research traditions. Qual Health Res. 2007;17(4):548–61.
Sandelowski M. Writing a good read: strategies for re-presenting qualitative data. Res Nurs Health. 1998;21:375–82.
Frank AW. After methods, the story: from incongruity to truth in qualitative research. Qual Health Res. 2004;14(3):430–40.
Sandelowski M. Time and qualitative research. [review] [31 refs]. Res Nurs Health. 1999;22(1):79–87.
Sandelowski M. Theory unmasked: the uses and guises of theory in qualitative research. Res Nurs Health. 1993;16:213–8.
Sandelowski M. “To be of use”: enhancing the utility of qualitative research. Nurs Outlook. 1997;45:125–32.
Charmaz K. Constructing grounded theory: a practical guide through qualitative analysis. London: Sage Publications; 2006.
Thorne S. Metasynthetic madness: what kind of monster have we created? Qual Health Res. 2017;27(1):3–12.
Sharp EA, GD DC. What does rejection have to do with it? Toward an innovative, kinesthetic analysis of qualitative data. Forum Qualitative Sozialforschung/Forum. Qual Soc Res [On-line Journal]. 2013;14(2):1–12.
Streiner DL, Norman GR. PDQ epidemiology. 2nd ed. St. Louis, Missouri: Mosby; 1996.
Ulrich CM, Wallen GR, Feister A, Grady C. Respondent burden in clinical research: when are we asking too much of subjects? IRB. 2005;27(4):17–20.
Polkinghorne DE. Language and meaning: data collection in qualitative research. J Couns Psychol. 2005;52(2):137–45.
Article Google Scholar
Schwandt TA. Dictionary of qualitative inquiry. 2nd ed. Thousand Oaks: Sage Publications, Inc.; 2001.
Saunders B, Sim J, Kingstone T, Baker S, Waterfield J, Bartlam B, et al. Saturation in qualitative research: exploring its conceptualization and operationalization. Qual Quant. 2018;52:1893–907.
Lewis J, Ritchie J. Generalising from qualitative research. In: Ritchie J, Lewis J, editors. Qualitative research practice: a guide for social science students and researchers. London: Sage Publications; 2003. p. 263–86.
Malterud K. Qualitative research: standards, challenges, and guidelines. Lancet. 2001;358(9280):483–8.
Giacomini MK, Cook DJ, for the Evidence-Based Medicine Working Group. Users’ guides to the medical literature XXIII. Qualitative research in health care B. what are the results and how do they help me care for my patients? JAMA. 2000;284(4):478–82.
Mays N, Pope C. Quality in qualitative health research. In: Pope C, Mays N, editors. Qualitative research in health care. Malden: Blackwell Publishing; 2006. p. 82–101.
Chapter Google Scholar
Dixon-Woods M, Shaw RL, Agarwal S, Smith JA. The problem of appraising qualitative research. Qual Saf Health Care. 2004;13:223–5.
Article CAS PubMed PubMed Central Google Scholar
Giorgi A. Concerning a serious misunderstanding of the essence of the phenomenological method in psychology. J Phenomenol Psychol. 2008;39:33–58.
Silverman D. Qualitative research: issues of theory, method and practice. 3rd ed. London: Sage Publications Ltd; 2011.
Finlay L. Negotiating the swamp: the opportunity and challenge of reflexivity in research practice. Qual Res. 2002;2(3):209–30.
Eakin JM, Gladstone B. “Value-adding” analysis: doing more with qualitative data. Int J Qual Methods. 2020;19:1–13.
Sale JEM, Cameron C, Hawker G, Jaglal S, Funnell L, Jain R, et al. Strategies used by an osteoporosis patient group to navigate for bone health care after a fracture. Arch Orthop Trauma Surg. 2014;134:229–35.
Sale JEM, Hawker G, Cameron C, Bogoch E, Jain R, Beaton D, et al. Perceived messages about bone health after a fracture are not consistent across healthcare providers. Rheumatol Int. 2015;35:97–103.
Sale JEM, Cameron C, Thielke S, Meadows L, Senior K. The theory of planned behaviour explains intentions to use antiresorptive medication after a fragility fracture. Rheumatol Int. 2017;37:875–82.
Ajzen I, Fishbein M. Understanding attitudes and predicting social behavior. Englewood Cliffs: Prentice-Hall; 1980.
Fishbein M, Ajzen I. Belief, attitude, intention, and behavior: an introduction to theory and research. Reading: Addison-Wesley; 1975.
Giorgi A. The theory, practice, and evaluation of the phenomenological method as a qualitative research procedure. J Phenomenol Psychol. 1997;28:235–60.
Giorgi A. The descriptive phenomenological method in psychology: a modified Husserlian approach, vol. 2009. Pittsburgh: Duquesne University Press; 2009.
Wertz FJ. Phenomenological research methods for counseling psychology. J Couns Psychol. 2005;52(2):167–77.
Braun V, Clarke V. Using thematic analysis in psychology. Qual Res Psychol. 2006;3:77–101.
Kristjansson E, Tugwell PS, Wilson AJ, Brooks PM, Driedger SM, Gallois C, et al. Development of the effective musculoskeletal consumer scale. J Rheumatol. 2007;34(6):1392–400.
PubMed Google Scholar
Hibbard JH, Stockard J, Mahoney ER, Tusler M. Development of the patient activation measure (PAM): conceptualizing and measuring activation in patients and consumers. Health Serv Res. 2004;39(4 Pt 1):1005–26.
Article PubMed PubMed Central Google Scholar
Sale JEM, Thielke S. Qualitative research is a fundamental scientific process. J Clin Epidemiol. 2018;102:129–33.
Agee J. Developing qualitative research questions: a reflective process. Int J Qual Stud Educ. 2009;22(4):431–47.
Cooper N, Burnett S. Using discursive reflexivity to enhance the qualitative research process. Qual Soc Work. 2006;5(1):111–29.
Carter SM, Little M. Justifying knowledge, justifying method, taking action: epistemologies, methodologies, and methods in qualitative research. Qual Health Res. 2007;17(10):1316–28.
Crotty M. The foundations of social research. Los Angeles: Sage Publications; 1998.
Sandelowski M. Whatever happened to qualitative description? Res Nurs Health. 2000;23:334–40.
Gheorghita A, Webster F, Thielke S, Sale JEM. Long-term experiences of pain after a fragility fracture. Osteoporos Int. 2018;29:1093–104.
Sale JEM, Ashe MC, Beaton D, Bogoch E, Frankel L. Men’s health-seeking behaviours regarding bone health after a fragility fracture: a secondary analysis of qualitative data. Osteoporos Int. 2016;27(10):3113–9.
Sale JEM, Frankel L, Paiva J, Saini J, Hui S, McKinlay J, et al. Having caregiving responsibilities affects management of fragility fractures and bone health. Osteoporos Int. 2020;31:1565–72.
Download references
Acknowledgements
Not applicable.
Funding for the work described in this paper was provided by the Canadian Institutes of Health Research (Funding Reference Number: CBO-109629). The Canadian Institutes of Health Research had no involvement in the design of the study and collection, analysis, and interpretation of the data and in the writing the manuscript.
Author information
Authors and affiliations.
Musculoskeletal Health and Outcomes Research, Li Ka Shing Knowledge Institute, St. Michael’s Hospital, Unity Health Toronto, 30 Bond Street, Toronto, Ontario, M5B 1W8, Canada
Joanna E. M. Sale
Institute of Health Policy, Management & Evaluation, University of Toronto, Health Sciences Building, 155 College Street, Suite 425, Toronto, Ontario, M5T 3M6, Canada
Department of Surgery, Faculty of Medicine, University of Toronto, 149 College Street, 5th Floor, Toronto, Ontario, M5T 1P5, Canada
You can also search for this author in PubMed Google Scholar
Contributions
Joanna Sale made substantial contributions to conception and design and analysis and interpretation of the data, drafted and revised the manuscript critically for important intellectual content, approved the final version of the manuscript submitted, and agreed to be accountable for all aspects of the work.
Author’s information
JEMS is a Scientist and Associate Professor who has been teaching qualitative research courses and lectures at the introductory and intermediate level at the University of Toronto since 2007.
Corresponding author
Correspondence to Joanna E. M. Sale .
Ethics declarations
Ethics approval and consent to participate.
The study and protocol upon which this manuscript is based was approved by the Research Ethics Board at Unity Health Toronto (REB# 10–371). All methods were carried out in accordance with the Declaration of Helsinki and the relevant guidelines and regulations set by the Research Ethics Board at Unity Health Toronto. Informed consent was obtained from all participants.
Consent for publication
Competing interests.
The author declares that she has no competing interests.
Additional information
Publisher’s note.
Springer Nature remains neutral with regard to jurisdictional claims in published maps and institutional affiliations.
Rights and permissions
Open Access This article is licensed under a Creative Commons Attribution 4.0 International License, which permits use, sharing, adaptation, distribution and reproduction in any medium or format, as long as you give appropriate credit to the original author(s) and the source, provide a link to the Creative Commons licence, and indicate if changes were made. The images or other third party material in this article are included in the article's Creative Commons licence, unless indicated otherwise in a credit line to the material. If material is not included in the article's Creative Commons licence and your intended use is not permitted by statutory regulation or exceeds the permitted use, you will need to obtain permission directly from the copyright holder. To view a copy of this licence, visit http://creativecommons.org/licenses/by/4.0/ . The Creative Commons Public Domain Dedication waiver ( http://creativecommons.org/publicdomain/zero/1.0/ ) applies to the data made available in this article, unless otherwise stated in a credit line to the data.
Reprints and permissions
About this article
Cite this article.
Sale, J.E.M. The role of analytic direction in qualitative research. BMC Med Res Methodol 22 , 66 (2022). https://doi.org/10.1186/s12874-022-01546-4
Download citation
Received : 28 September 2021
Accepted : 11 February 2022
Published : 13 March 2022
DOI : https://doi.org/10.1186/s12874-022-01546-4
Share this article
Anyone you share the following link with will be able to read this content:
Sorry, a shareable link is not currently available for this article.
Provided by the Springer Nature SharedIt content-sharing initiative
- Analytic direction
- Qualitative research
- Data analysis
- Methodological rigour
- Critical appraisal
BMC Medical Research Methodology
ISSN: 1471-2288
- General enquiries: [email protected]
How it works
Transform your enterprise with the scalable mindsets, skills, & behavior change that drive performance.
Explore how BetterUp connects to your core business systems.
We pair AI with the latest in human-centered coaching to drive powerful, lasting learning and behavior change.
Build leaders that accelerate team performance and engagement.
Unlock performance potential at scale with AI-powered curated growth journeys.
Build resilience, well-being and agility to drive performance across your entire enterprise.
Transform your business, starting with your sales leaders.
Unlock business impact from the top with executive coaching.
Foster a culture of inclusion and belonging.
Accelerate the performance and potential of your agencies and employees.
See how innovative organizations use BetterUp to build a thriving workforce.
Discover how BetterUp measurably impacts key business outcomes for organizations like yours.
Daring Leadership Institute: a groundbreaking partnership that amplifies Brené Brown's empirically based, courage-building curriculum with BetterUp’s human transformation platform.

- What is coaching?
Learn how 1:1 coaching works, who its for, and if it's right for you.
Accelerate your personal and professional growth with the expert guidance of a BetterUp Coach.
Types of Coaching
Navigate career transitions, accelerate your professional growth, and achieve your career goals with expert coaching.
Enhance your communication skills for better personal and professional relationships, with tailored coaching that focuses on your needs.
Find balance, resilience, and well-being in all areas of your life with holistic coaching designed to empower you.
Discover your perfect match : Take our 5-minute assessment and let us pair you with one of our top Coaches tailored just for you.
Find your coach
-1.png)
Research, expert insights, and resources to develop courageous leaders within your organization.
Best practices, research, and tools to fuel individual and business growth.
View on-demand BetterUp events and learn about upcoming live discussions.
The latest insights and ideas for building a high-performing workplace.
- BetterUp Briefing
The online magazine that helps you understand tomorrow's workforce trends, today.
Innovative research featured in peer-reviewed journals, press, and more.
Founded in 2022 to deepen the understanding of the intersection of well-being, purpose, and performance
We're on a mission to help everyone live with clarity, purpose, and passion.
Join us and create impactful change.
Read the buzz about BetterUp.
Meet the leadership that's passionate about empowering your workforce.

For Business
For Individuals

What are analytical skills? Examples and how to level up
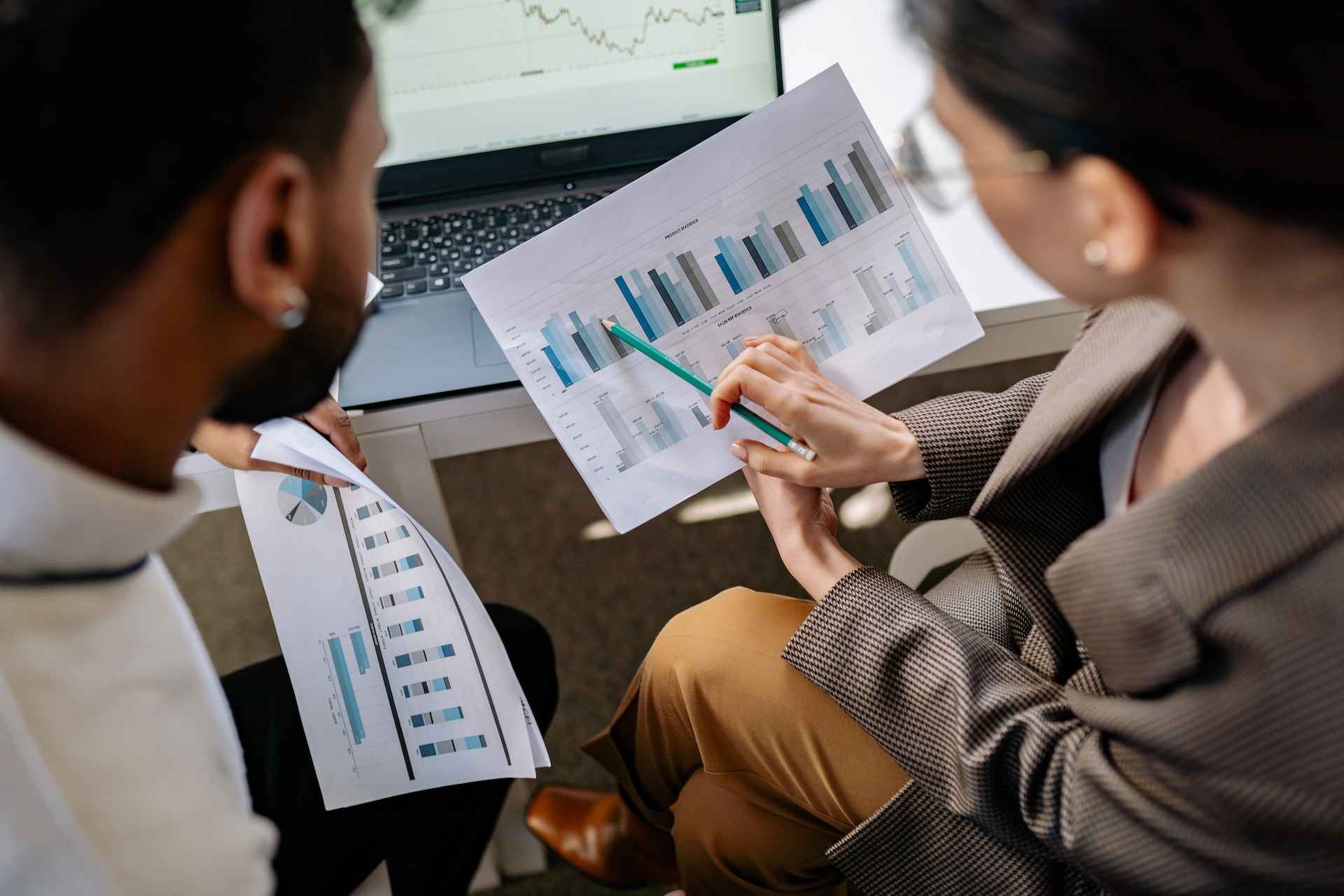
Jump to section
What are analytical skills?
Why are analytical skills important, 9 analytical skills examples, how to improve analytical skills, how to show analytical skills in a job application, the benefits of an analytical mind.
With market forecasts, performance metrics, and KPIs, work throws a lot of information at you.
If you want to stay ahead of the curve, not only do you have to make sense of the data that comes your way — you need to put it to good use. And that requires analytical skills.
You likely use analytical thinking skills every day without realizing it, like when you solve complex problems or prioritize tasks . But understanding the meaning of analysis skills in a job description, why you should include them in your professional development plan, and what makes them vital to every position can help advance your career.
Analytical skills, or analysis skills, are the ones you use to research and interpret information. Although you might associate them with data analysis, they help you think critically about an issue, make decisions , and solve problems in any context. That means anytime you’re brainstorming for a solution or reviewing a project that didn’t go smoothly, you’re analyzing information to find a conclusion. With so many applications, they’re relevant for nearly every job, making them a must-have on your resume.
Analytical skills help you think objectively about information and come to informed conclusions. Positions that consider these skills the most essential qualification grew by 92% between 1980 and 2018 , which shows just how in-demand they are. And according to Statista, global data creation will grow to more than 180 zettabytes by 2025 — a number with 21 zeros. That data informs every industry, from tech to marketing.
Even if you don’t interact with statistics and data on the job, you still need analytical skills to be successful. They’re incredibly valuable because:
- They’re transferable: You can use analysis skills in a variety of professional contexts and in different areas of your life, like making major decisions as a family or setting better long-term personal goals.
- They build agility: Whether you’re starting a new position or experiencing a workplace shift, analysis helps you understand and adapt quickly to changing conditions.
- They foster innovation: Analytical skills can help you troubleshoot processes or operational improvements that increase productivity and profitability.
- They make you an attractive candidate: Companies are always looking for future leaders who can build company value. Developing a strong analytical skill set shows potential employers that you’re an intelligent, growth-oriented candidate.
If the thought of evaluating data feels unintuitive, or if math and statistics aren’t your strong suits, don’t stress. Many examples of analytical thinking skills don’t involve numbers. You can build your logic and analysis abilities through a variety of capacities, such as:
1. Brainstorming
Using the information in front of you to generate new ideas is a valuable transferable skill that helps you innovate at work . Developing your brainstorming techniques leads to better collaboration and organizational growth, whether you’re thinking of team bonding activities or troubleshooting a project roadblock. Related skills include benchmarking, diagnosis, and judgment to adequately assess situations and find solutions.
2. Communication
Becoming proficient at analysis is one thing, but you should also know how to communicate your findings to your audience — especially if they don’t have the same context or experience as you. Strong communication skills like public speaking , active listening , and storytelling can help you strategize the best ways to get the message out and collaborate with your team . And thinking critically about how to approach difficult conversations or persuade someone to see your point relies on these skills.
3. Creativity
You might not associate analysis with your creativity skills, but if you want to find an innovative approach to an age-old problem, you’ll need to combine data with creative thinking . This can help you establish effective metrics, spot trends others miss, and see why the most obvious answer to a problem isn’t always the best. Skills that can help you to think outside the box include strategic planning, collaboration, and integration.

4. Critical thinking
Processing information and determining what’s valuable requires critical thinking skills . They help you avoid the cognitive biases that prevent innovation and growth, allowing you to see things as they really are and understand their relevance. Essential skills to turn yourself into a critical thinker are comparative analysis, business intelligence, and inference.
5. Data analytics
When it comes to large volumes of information, a skilled analytical thinker can sort the beneficial from the irrelevant. Data skills give you the tools to identify trends and patterns and visualize outcomes before they impact an organization or project’s performance. Some of the most common skills you can develop are prescriptive analysis and return on investment (ROI) analysis.
6. Forecasting
Predicting future business, market, and cultural trends better positions your organization to take advantage of new opportunities or prepare for downturns. Business forecasting requires a mix of research skills and predictive abilities, like statistical analysis and data visualization, and the ability to present your findings clearly.
7. Logical reasoning
Becoming a logical thinker means learning to observe and analyze situations to draw rational and objective conclusions. With logic, you can evaluate available facts, identify patterns or correlations, and use them to improve decision-making outcomes. If you’re looking to improve in this area, consider developing inductive and deductive reasoning skills.
8. Problem-solving
Problem-solving appears in all facets of your life — not just work. Effectively finding solutions to any issue takes analysis and logic, and you also need to take initiative with clear action plans . To improve your problem-solving skills , invest in developing visualization , collaboration, and goal-setting skills.
9. Research
Knowing how to locate information is just as valuable as understanding what to do with it. With research skills, you’ll recognize and collect data relevant to the problem you’re trying to solve or the initiative you’re trying to start. You can improve these skills by learning about data collection techniques, accuracy evaluation, and metrics.

You don’t need to earn a degree in data science to develop these skills. All it takes is time, practice, and commitment. Everything from work experience to hobbies can help you learn new things and make progress. Try a few of these ideas and stick with the ones you enjoy:
1. Document your skill set
The next time you encounter a problem and need to find solutions, take time to assess your process. Ask yourself:
- What facts are you considering?
- Do you ask for help or research on your own? What are your sources of advice?
- What does your brainstorming process look like?
- How do you make and execute a final decision?
- Do you reflect on the outcomes of your choices to identify lessons and opportunities for improvement?
- Are there any mistakes you find yourself making repeatedly?
- What problems do you constantly solve easily?
These questions can give insight into your analytical strengths and weaknesses and point you toward opportunities for growth.
2. Take courses
Many online and in-person courses can expand your logical thinking and analysis skills. They don’t necessarily have to involve information sciences. Just choose something that trains your brain and fills in your skills gaps .
Consider studying philosophy to learn how to develop your arguments or public speaking to better communicate the results of your research. You could also work on your hard skills with tools like Microsoft Excel and learn how to crunch numbers effectively. Whatever you choose, you can explore different online courses or certification programs to upskill.
3. Analyze everything
Spend time consciously and critically evaluating everything — your surroundings, work processes, and even the way you interact with others. Integrating analysis into your day-to-day helps you practice. The analytical part of your brain is like a muscle, and the more you use it, the stronger it’ll become.
After reading a book, listening to a podcast, or watching a movie, take some time to analyze what you watched. What were the messages? What did you learn? How was it delivered? Taking this approach to media will help you apply it to other scenarios in your life.
If you’re giving a presentation at work or helping your team upskill , use the opportunity to flex the analytical side of your brain. For effective teaching, you’ll need to process and analyze the topic thoroughly, which requires skills like logic and communication. You also have to analyze others’ learning styles and adjust your teachings to match them.
5. Play games
Spend your commute or weekends working on your skills in a way you enjoy. Try doing logic games like Sudoku and crossword puzzles during work breaks to foster critical thinking. And you can also integrate analytical skills into your existing hobbies. According to researcher Rakesh Ghildiyal, even team sports like soccer or hockey will stretch your capacity for analysis and strategic thinking .
6. Ask questions
According to a study in Tr ends in Cognitive Sciences, being curious improves cognitive function , helping you develop problem-solving skills, retention, and memory. Start speaking up in meetings and questioning the why and how of different decisions around you. You’ll think more critically and even help your team find breakthrough solutions they otherwise wouldn’t.
7.Seek advice
If you’re unsure what analytical skills you need to develop, try asking your manager or colleagues for feedback . Their outside perspective offers insight you might not find within, like patterns in. And if you’re looking for more consistent guidance, talking to a coach can help you spot weaknesses and set goals for the long term.
8. Pursue opportunities
Speak to your manager about participating in special projects that could help you develop and flex your skills. If you’d like to learn about SEO or market research, ask to shadow someone in the ecommerce or marketing departments. If you’re interested in business forecasting, talk to the data analysis team. Taking initiative demonstrates a desire to learn and shows leadership that you’re eager to grow.

Shining a spotlight on your analytical skills can help you at any stage of your job search. But since they take many forms, it’s best to be specific and show potential employers exactly why and how they make you a better candidate. Here are a few ways you can showcase them to the fullest:
1. In your cover letter
Your cover letter crafts a narrative around your skills and work experience. Use it to tell a story about how you put your analytical skills to use to solve a problem or improve workflow. Make sure to include concrete details to explain your thought process and solution — just keep it concise. Relate it back to the job description to show the hiring manager or recruiter you have the qualifications necessary to succeed.
2. On your resume
Depending on the type of resume you’re writing, there are many opportunities to convey your analytical skills to a potential employer. You could include them in sections like:
- Professional summary: If you decide to include a summary, describe yourself as an analytical person or a problem-solver, whichever relates best to the job posting.
- Work experience: Describe all the ways your skill for analysis has helped you perform or go above and beyond your responsibilities. Be sure to include specific details about challenges and outcomes related to the role you’re applying for to show how you use those skills.
- Skills section: If your resume has a skill-specific section, itemize the analytical abilities you’ve developed over your career. These can include hard analytical skills like predictive modeling as well as interpersonal skills like communication.
3. During a job interview
As part of your interview preparation , list your professional accomplishments and the skills that helped along the way, such as problem-solving, data literacy, or strategic thinking. Then, pull them together into confident answers to common interview questions using the STAR method to give the interviewer a holistic picture of your skill set.
Developing analytical skills isn’t only helpful in the workplace. It’s essential to life. You’ll use them daily whenever you read the news, make a major purchase, or interact with others. Learning to critically evaluate information can benefit your relationships and help you feel more confident in your decisions, whether you’re weighing your personal budget or making a big career change .
Understand Yourself Better:
Big 5 Personality Test
Elizabeth Perry, ACC
Elizabeth Perry is a Coach Community Manager at BetterUp. She uses strategic engagement strategies to cultivate a learning community across a global network of Coaches through in-person and virtual experiences, technology-enabled platforms, and strategic coaching industry partnerships. With over 3 years of coaching experience and a certification in transformative leadership and life coaching from Sofia University, Elizabeth leverages transpersonal psychology expertise to help coaches and clients gain awareness of their behavioral and thought patterns, discover their purpose and passions, and elevate their potential. She is a lifelong student of psychology, personal growth, and human potential as well as an ICF-certified ACC transpersonal life and leadership Coach.
20 examples of development opportunities that can level up your career
A roadmap for career development: how to set your course, create a networking plan in 7 easy steps, what business acumen is and 9 ways to develop it, discover how to get noticed by upper management at work, are you being passed over for a promotion here’s what to do, 8 examples for setting professional development goals at work, how to pursue jobs versus careers to achieve different goals, professional development is for everyone (we’re looking at you), how to develop critical thinking skills, why we're facing a crisis of imagination, and how to overcome it, 10 essential business skills that make an impact on your career, what are hard skills & examples for your resume, use a personal swot analysis to discover your strengths and weaknesses, 17 essential transferable skills to boost your job search, critical thinking is the one skillset you can't afford not to master, what are metacognitive skills examples in everyday life, stay connected with betterup, get our newsletter, event invites, plus product insights and research..
3100 E 5th Street, Suite 350 Austin, TX 78702
- Platform Overview
- Integrations
- Powered by AI
- BetterUp Lead™
- BetterUp Manage™
- BetterUp Care®
- Sales Performance
- Diversity & Inclusion
- Case Studies
- Why BetterUp?
- About Coaching
- Find your Coach
- Career Coaching
- Communication Coaching
- Personal Coaching
- News and Press
- Leadership Team
- Become a BetterUp Coach
- BetterUp Labs
- Center for Purpose & Performance
- Leadership Training
- Business Coaching
- Contact Support
- Contact Sales
- Privacy Policy
- Acceptable Use Policy
- Trust & Security
- Cookie Preferences
- GRE Eligibility Criteria
- GRE Registration Process
- GRE Exam Dates
- GRE Exam Pattern
- GRE Syllabus
- GRE Practice Paper
- GRE Exam Fees
- GRE Score Validity
- GRE Scholarships
- GRE Exam Fee Waiver 2024
- GRE Coaching Centres
- Best books for GRE Exam Preparation
GRE Analytical Writing Overview| Syllabus, Examples & More
The GRE Analytical Writing Assessment (AWA) is a vital part of the GRE, assessing your ability to think critically and write analytically. Aiming for a GRE Analytical Writing score above 4.5 is crucial if you’re targeting top universities. The updated format features just one task: Analyze an Issue , giving you 30 minutes to write a concise, well-structured essay.
To excel, focus on writing between 500 and 600 words across 4 to 5 paragraphs, ensuring clarity and adherence to the GRE Analytical Writing word limit . Reviewing GRE Analytical Writing examples and GRE Analytical Writing PDFs can provide essential practice and insight, helping you achieve a strong score and boost your overall GRE performance.
Table of Content
GRE Analytical Writing
Gre analytical writing pdf, gre issue essay format, gre analytical writing samples, gre analytical writing score.
The GRE Analytical Writing Assessment (AWA) now exclusively features the Analyze an Issue task. This section is designed to evaluate your critical thinking and analytical writing abilities. Unlike other sections, there is no fixed pattern for GRE AWA topics , making it essential to familiarize yourself with a wide range of issues. Staying updated on the latest GRE exam pattern is crucial to understanding the recent changes in this section.
Common GRE AWA Topics
The following are some frequently encountered themes for the GRE Analyze an Issue task:
The impact of technology on society, the role of the internet in shaping modern culture. | |
The importance of standardized testing, the value of a liberal arts education. | |
The relevance of art in contemporary society, government funding for the arts. | |
The pursuit of knowledge for its own sake, the value of curiosity-driven research. | |
The role of government in society, the balance of power between different branches of government. | |
The challenges of urbanization, the importance of sustainable city planning. | |
The role of ethics in decision-making, the relevance of ancient philosophical ideas in modern times. |
Unlock your potential for success in the GRE with our comprehensive GRE Analytical Writing PDF guide. Designed to help you excel in the Analytical Writing Assessment (AWA), this resource offers essential insights and strategies to master the GRE essay tasks. The GRE AWA section assesses your ability to think critically, develop well-structured arguments, and express your ideas clearly and effectively.
Our PDF guide includes detailed explanations of the GRE Analytical Writing format, tips for crafting compelling essays, and sample prompts with high-scoring responses. Whether you’re aiming for a top score or simply looking to improve your writing skills, this PDF provides the tools and knowledge you need to succeed in the GRE Analytical Writing section. Download now to start your journey towards GRE success!
GRE Analytical Writing PDF- Free DOWNLOAD!!!!
Important GRE Issue Essay Format are as follows:
GRE AWA Essay: Essential Tips for Success
The GRE AWA essay on an issue should be approximately 500-600 words in length, focusing on topics of general interest that can be analyzed from multiple perspectives. Remember, there are no absolute correct answers in the GRE AWA ; instead, the test evaluates your critical thinking skills and your ability to present a well-reasoned argument. The GRE Analyze an Issue task challenges you to take a stance on a given topic, providing compelling reasons and evidence to support your position.
Before you begin writing, carefully review the instructions and plan your response. Instructions typically fall into the following categories:
- Agree/Disagree with a Statement : Explain why you agree or disagree with the given statement, considering different perspectives that may support or challenge the statement.
- Position on a Recommendation : Articulate your stance on the provided recommendation, backing it up with reasons and examples.
- Extent of Agreement/Disagreement : Craft a response that discusses the extent to which you agree or disagree with a given claim.
- Balanced Argument : Write a response that discusses both sides of the argument, then explain your position.
- Consequences of a Policy : Discuss the consequences of a policy and how they influenced your decision.
Tips for Writing a Strong GRE Issue Essay
To excel in the GRE Issue Essay , consider the following tips:
- Practice Regularly : Start by practicing writing GRE Issue Essays . Writing at least three essays will help you manage your time, familiarize yourself with different prompts, and understand the factual support needed for a strong argument.
- Pick One Side : Choose one side of the argument to support. Avoid trying to argue both sides, as this can weaken your essay and make your position unclear. The examiners assess your ability to defend your chosen stance effectively.
- Use Relevant Examples : Provide relevant examples to bolster your argument. Use examples from diverse fields such as business, arts, or history, but ensure they serve to support your essay rather than dominate it.
- Follow a Structured Pattern : Organize your essay in a clear, structured manner. A well-structured essay not only provides clarity to the reader but also helps to increase your GRE AWA score .
By following these tips and practicing regularly, you’ll improve your ability to write a compelling GRE AWA essay , enhancing your chances of achieving a high score. Incorporate these strategies into your preparation to present clear, well-supported arguments that will impress GRE examiners.
Here are some examples of high-quality GRE Analytical Writing essays for the “Analyze an Issue” task. These examples illustrate how to effectively develop and present arguments, supporting a high score in the GRE AWA section:
Example 1: Technology and Society
Prompt: “Technology has made our lives easier but has also made us more isolated from each other.”
Essay: In today’s fast-paced world, technology undeniably simplifies many aspects of life, from communication to information access. However, it also contributes to a sense of isolation. For instance, while social media platforms facilitate instant communication, they often replace face-to-face interactions with impersonal digital exchanges. This shift can lead to superficial relationships and a lack of genuine human connection. Moreover, the rise of remote work, enabled by technology, has reduced daily interpersonal interactions, potentially weakening social bonds. Nonetheless, technology also fosters global connections and allows for virtual communities that can provide support and shared experiences. Balancing the benefits of technology with its potential to isolate individuals is crucial for maintaining meaningful personal connections.
Example 2: Education
Prompt: “A college education should emphasize practical skills rather than theoretical knowledge.”
Essay: The debate between practical skills and theoretical knowledge in higher education is crucial for preparing students for the workforce. Advocates for practical skills argue that such training equips students with job-ready abilities, making them more competitive in the job market. For instance, courses in coding, data analysis, and project management directly align with industry demands and provide tangible benefits. Conversely, theoretical knowledge fosters critical thinking and problem-solving skills that are also essential in any profession. For example, understanding foundational theories in economics or psychology can enhance analytical abilities and adaptability. A balanced approach, integrating both practical skills and theoretical knowledge, ensures that students are well-rounded and prepared for diverse challenges.
Example 3: Government and Power
Prompt: “Governments should prioritize economic development over environmental protection.”
Essay: The debate over whether governments should prioritize economic development or environmental protection is complex and multifaceted. Economic development fosters job creation, infrastructure improvement, and overall societal prosperity. For example, industrial growth often leads to higher employment rates and improved living standards. However, prioritizing economic growth at the expense of environmental protection can lead to long-term damage, such as climate change and loss of biodiversity. Sustainable development practices, which balance economic growth with environmental stewardship, are crucial. For instance, investing in green technologies can stimulate economic growth while preserving natural resources. Hence, a strategic approach that integrates both priorities is essential for achieving long-term prosperity and ecological balance.
GRE scores will be accessible on the official ETS website within 8-10 days following the exam date. The Analytical Writing GRE score falls between 0 and 6.0. Valid for five years, candidates must submit or send their additional score reports to their chosen institutions within this timeframe for a successful admission process. Now, let’s explore the criteria ETS considers when evaluating your AWA essays.
Here’s a brief table summarizing the GRE AWA score and its corresponding explanation:
Clear identification and deep analysis of key features; well-organized ideas with logical connections; strong language control with few to no errors. | |
Thoughtful analysis with clear identification of important features; logical idea development with minor flaws; good control of language and syntax. | |
Identifies main features with satisfactory analysis; organized ideas but may miss connections; sufficient language control with some flaws. | |
Limited analysis and poor organization; minimal support for critique; imprecise language with frequent errors. | |
No clear understanding or analysis; disorganized with irrelevant evidence; serious language, grammar, and structural issues. | |
Lacks understanding and organization; severe errors in grammar and sentence structure; incoherent response. | |
Off-topic, non-English, copied, random characters, or no response. |
|
|
|
|
|
|
|
|
|
|
|
|
|
|
|
GRE Analytical Writing- FAQs
How to write analytical writing in gre.
The Analytical Writing section of the GRE includes a 30-minute “Analyze an Issue” task. In this task, you are given a statement or opinion on a particular topic along with guidelines for your response. Your goal is to assess the issue, explore its various aspects, and construct a well-reasoned argument supported by relevant examples and explanations.
Is 3.5 a good score in analytical writing in GRE?
A score of 3.5 in GRE Analytical Writing is considered below average. Top-ranked universities generally look for higher scores, typically 4.0 or above, to meet their competitive admissions standards.
How many words should your GRE Analytical Writing essay be?
For the GRE Analytical Writing section, it’s recommended that your essay be between 500 and 600 words. Aiming for this word count ensures that you have enough space to develop your arguments fully while adhering to the GRE Analytical Writing guidelines. Keeping within this range helps demonstrate a well-structured, coherent argument and allows for a thorough analysis of the issue. Properly managing your word count is crucial for scoring well on the GRE Analytical Writing Assessment.
What is a good AWA score in GRE?
A GRE AWA score of 6 to 5 means the candidate has proper writing skills. The average AWA cutoff for US universities ranges from 4.5 and above. The average AWA score in GRE is 3.5.
Please Login to comment...
Similar reads.
- Study Abroad
- Best Twitch Extensions for 2024: Top Tools for Viewers and Streamers
- Discord Emojis List 2024: Copy and Paste
- Best Adblockers for Twitch TV: Enjoy Ad-Free Streaming in 2024
- PS4 vs. PS5: Which PlayStation Should You Buy in 2024?
- 10 Best Free VPN Services in 2024
Improve your Coding Skills with Practice
What kind of Experience do you want to share?
- Open access
- Published: 02 September 2024
Causal associations of hypothyroidism with frozen shoulder: a two-sample bidirectional Mendelian randomization study
- Bin Chen 1 ,
- Zheng-hua Zhu 1 ,
- Qing Li 2 ,
- Zhi-cheng Zuo 1 &
- Kai-long Zhou 1
BMC Musculoskeletal Disorders volume 25 , Article number: 693 ( 2024 ) Cite this article
Metrics details
Many studies have investigated the association between hypothyroidism and frozen shoulder, but their findings have been inconsistent. Furthermore, earlier research has been primarily observational, which may introduce bias and does not establish a cause-and-effect relationship. To ascertain the causal association, we performed a two-sample bidirectional Mendelian randomization (MR) analysis.
We obtained data on “Hypothyroidism” and “Frozen Shoulder” from Summary-level Genome-Wide Association Studies (GWAS) datasets that have been published. The information came from European population samples. The primary analysis utilized the inverse-variance weighted (IVW) method. Additionally, a sensitivity analysis was conducted to assess the robustness of the results.
We ultimately chose 39 SNPs as IVs for the final analysis. The results of the two MR methods we utilized in the investigation indicated that a possible causal relationship between hypothyroidism and frozen shoulder. The most significant analytical outcome demonstrated an odds ratio (OR) of 1.0577 (95% Confidence Interval (CI):1.0057–1.1123), P = 0.029, using the IVW approach. Furthermore, using the MR Egger method as a supplementary analytical outcome showed an OR of 1.1608 (95% CI:1.0318–1.3060), P = 0.017. Furthermore, the results of our sensitivity analysis indicate that there is no heterogeneity or pleiotropy in our MR analysis. In the reverse Mendelian analysis, no causal relationship was found between frozen shoulders and hypothyroidism.
Our MR analysis suggests that there may be a causal relationship between hypothyroidism and frozen shoulder.
Peer Review reports
Frozen shoulder, also known as adhesive capsulitis, is a common shoulder condition. Patients with frozen shoulder usually experience severe shoulder pain and diffuse shoulder stiffness, which is usually progressive and can lead to severe limitations in daily activities, especially with external rotation of the shoulder joint [ 1 ]. The incidence of the disease is difficult to ascertain because of its insidious onset and the fact that many patients do not choose to seek medical attention. It is estimated to affect about 2% to 5% of the population, with women affected more commonly than men (1.6:1.0) [ 2 , 3 ]. The peak occurrence of frozen shoulder is typically between the ages of 40 and 60, with a positive family history present in around 9.5% of cases [ 4 ]. However, the underlying etiology and pathophysiology of frozen shoulder remains unclear.
The prevalence of frozen shoulder has been reported to be higher in certain diseases such as dyslipidemia [ 5 ], diabetes [ 6 , 7 ], and thyroid disorders [ 4 , 8 ]. The relationship between diabetes and frozen shoulder has been established through epidemiological studies [ 9 , 10 , 11 ]. However, the relationship between thyroid disease and frozen shoulder remains unclear. Thyroid disorders include hyperthyroidism, hypothyroidism, thyroiditis, subclinical hypothyroidism, and others. Previously, some studies reported the connection between frozen shoulders and thyroid dysfunction. However, the conclusions of these studies are not consistent [ 4 , 12 , 13 , 14 , 15 , 16 ]. In addition, these studies are primarily observational and susceptible to confounding variables. Traditional observational studies can only obtain correlations, not exact causal relationships [ 17 ].
MR is a technique that utilizes genetic variants as instrumental variables (IVs) of exposure factors to determine the causal relationship between exposure factors and outcomes [ 17 , 18 ]. MR operates similarly to a randomized controlled trial as genetic variants adhere to Mendelian inheritance patterns and are randomly distributed in the population [ 19 ]. Moreover, alleles remain fixed between individuals and are not influenced by the onset or progression of disease. Consequently, causal inferences derived from MR analyses are less susceptible to confounding and reverse causality biases [ 20 , 21 ]. And with the growing number of GWAS data published by large consortia, MR studies can provide reliable results with a sufficient sample size [ 22 ]. In this study, we performed a two-sample bidirectional MR analysis to evaluate the causal relationship between hypothyroidism and frozen shoulder.
Study design description
The bidirectional MR design, which examines the relationship between hypothyroidism and frozen shoulder, is succinctly outlined in Fig. 1 . Using summary data from Genome-Wide Association Studies (GWAS) datasets, we conducted two MR analyses to explore the potential reciprocal association between hypothyroidism and frozen shoulder. In the reverse MR analyses, Frozen Shoulder was considered as the exposure and Hypothyroidism as the outcome, while the forward MR analyses focused on Hypothyroidism as the exposure. Figure 1 illustrates the key assumptions of the MR analysis.
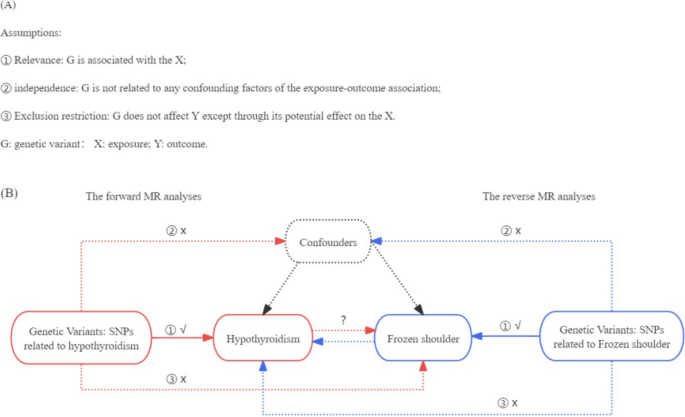
Description of the study design in this bidirectional MR study. A MR analyses depend on three core assumptions. B Research design sketches
Data source
Genetic variants associated with Hypothyroidism were extracted from published Summary-level GWAS datasets provided by the FinnGen Consortium, using the “Hypothyroidism” phenotype in this study. The GWAS included 16380353 subjects, including 22997 cases and 175475 controls. Data for Frozen Shoulder were obtained from the GWAS, which was derived from a European sample [ 23 ]. The frozen shoulder was defined based on the occurrence of one or more International Classification of Disease, 10th Revision (ICD10) codes (as shown in the supplementary material). Our MR study was conducted using publicly available studies or shared datasets and therefore did not require additional ethical statements or consent.
Selection of IV
For MR studies to yield reliable results, they must adhere to three fundamental assumptions [ 24 ], Regarding the IV selection, the following statements hold true: (1) IVs exhibit substantial correlation with exposure factors; (2) IVs do not directly impact outcomes but influence outcomes through exposure; (3) IVs are not correlated with any confounding factors that could influence exposure and outcome. Firstly, we selected single‐nucleotide polymorphisms (SNPs) from the European GWAS that met the genome-wide significance criterion ( p < 5 × 10 –8 ) and were associated with the exposure of interest as potential SNPs. Subsequently, we excluded any selected SNPs that linkage disequilibrium (LD) using the clump function (r 2 = 0.001, kb = 10000). Furthermore, palindromic SNPs and ambiguous SNPs were excluded. These excluded SNPs were not included in subsequent analyses. To evaluate weak instrumental variable effects, we utilized the F-statistic, considering genetic variants with an F-statistic < 10 as weak IVs and excluding them. Then for the second assumption, we needed to manually remove SNPs associated with outcome ( p < 5 × 10 –8 ). For the third assumption, “ IVs are not correlated with any confounding factors that could influence exposure and outcome,” implying that the IVs chosen should not have horizontal pleiotropy. The final set of SNPs meeting these criteria were utilized as IVs in the subsequent MR analysis.
MR analysis
In this study, we evaluated the relationship between hypothyroidism and frozen shoulder using two different MR methods: IVW [ 25 ] and MR-Egger regression [ 26 ]. The Wald ratio for each IV will be meta-analyzed using the IVW approach to investigate the causal relationship. In contrast to the MR-Egger technique, which remains functional even in the presence of invalid IVs, the IVW method assumes that all included genetic variants are valid instrumental variables. Furthermore, MR-Egger incorporates an intercept term to examine potential pleiotropy. If this intercept term equals 0 ( P > 0.05), the results of the MR-Egger regression model closely align with those obtained from IVW; However, if the intercept term deviates significantly from 0 ( P < 0.05), it suggests possible horizontal pleiotropy associated with these IVs. MR-Egger employed as estimation method alongside IVW. Although less efficient, these approaches can provide reliable predictions across a broader range of scenarios.
Sensitivity analysis
We performed a sensitivity analysis to investigate potential horizontal pleiotropy and heterogeneity in our study, aiming to demonstrate the robustness of our findings. Cochran’s Q test was employed to identify possible heterogeneity. Cochran’s Q statistic assessed genetic variant heterogeneity while considering significance at p < 0.05 level and I 2 > 25% as an indication of heterogeneity. on the results, we generated funnel plots. MR-Egger intercept tests were then utilized to estimate horizontal pleiotropy (with presence of an intercept and horizontal pleiotropy considered when p < 0.05). Additionally, a leave-one-out to determine if causality depended on or was influenced by any specific SNP. All statistical analyses were performed using the “TwoSampleMR” packages in R (version 3.6.3, www.r-project.org/ ) [ 27 ].
Instrumental variables
We ultimately chose 39 SNPs as IVs for the final analysis after going through the aforementioned screening process. All IVs had an F-statistic > 10, indicating a low probability of weak IV bias. Comprehensive information on each IV can be found in Appendix 1 .
Mendelian randomization results
According to the outcomes of the two MR techniques we employed for our analysis, hypothyroidism increases the risk factors for developing frozen shoulder. Specifically, as shown in the results of Table 1 , the primary analytical outcome using the IVW method revealed an OR of 1.0577 (95% CI:1.0057–1.1123), P = 0.029. Additionally, employing the MR Egger method secondary analytical outcome resulted in an OR of 1.1608 (95% CI:1.0318–1.3060), P = 0.017. Furthermore, scatter plots (Fig. 2 ) and forest plots (Fig. 3 ) were generated based on the findings of this MR study.
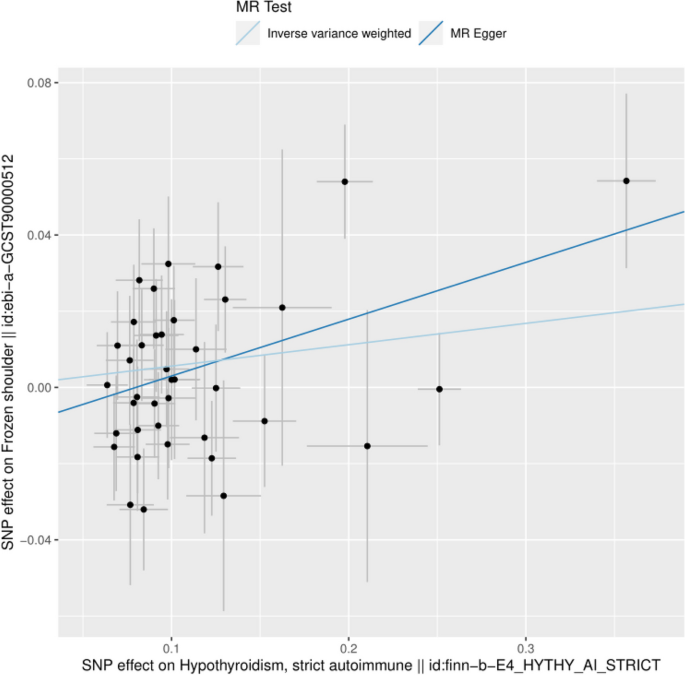
Scatterplot of MR analysis
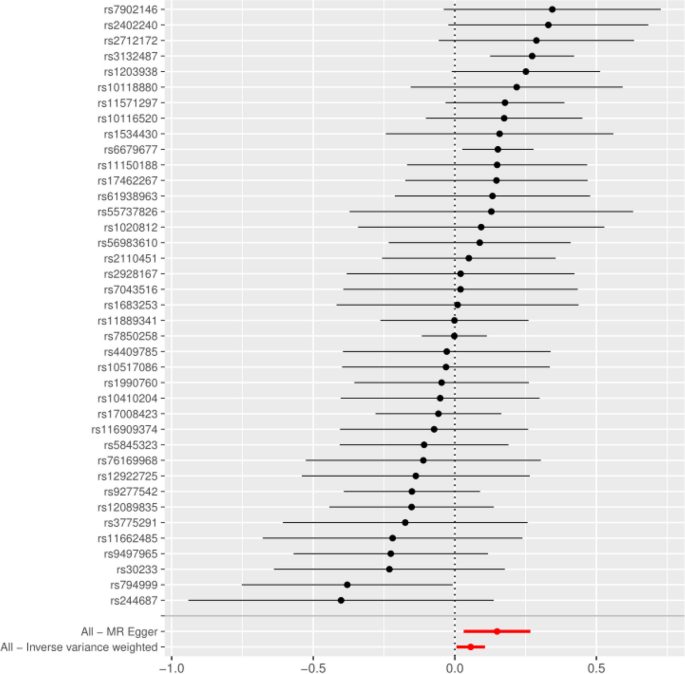
Forest plot of MR analysis
Heterogeneity and sensitivity test
The heterogeneity of causal estimates obtained for each SNP reflects their variability. A lower level of heterogeneity indicates higher reliability of MR estimates. To further validate the dependability of the results, we conducted a sensitivity analysis to examine the heterogeneity in MR. The funnel plots we created are displayed in Fig. 4 together with the results of Cochran’s Q test (Table 2 ), which revealed no heterogeneity in IVs. Additionally, the MR-Egger intercept test results (p = 0.0968) indicated no presence of pleiotropy in our data. Furthermore, the outcomes leave-one-out test demonstrated that causation remained independent and unaffected by any specific SNP (Fig. 5 ).
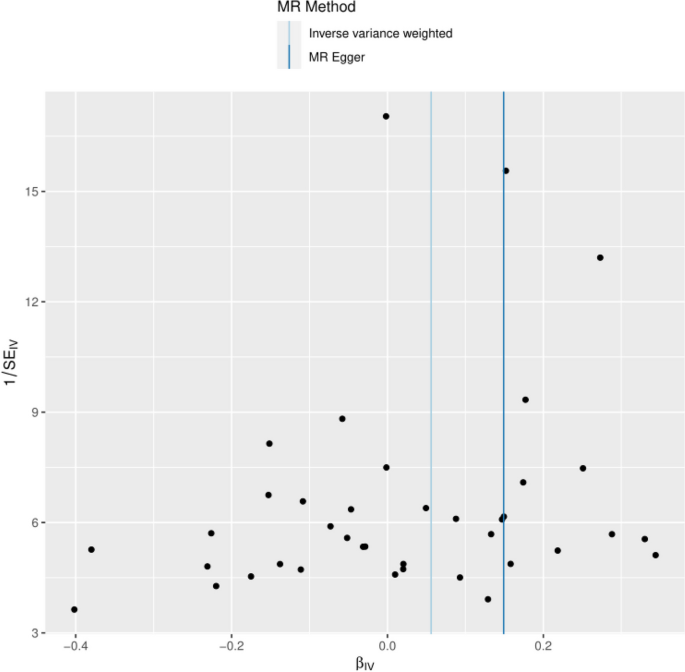
Funnel plot to assess heterogeneity
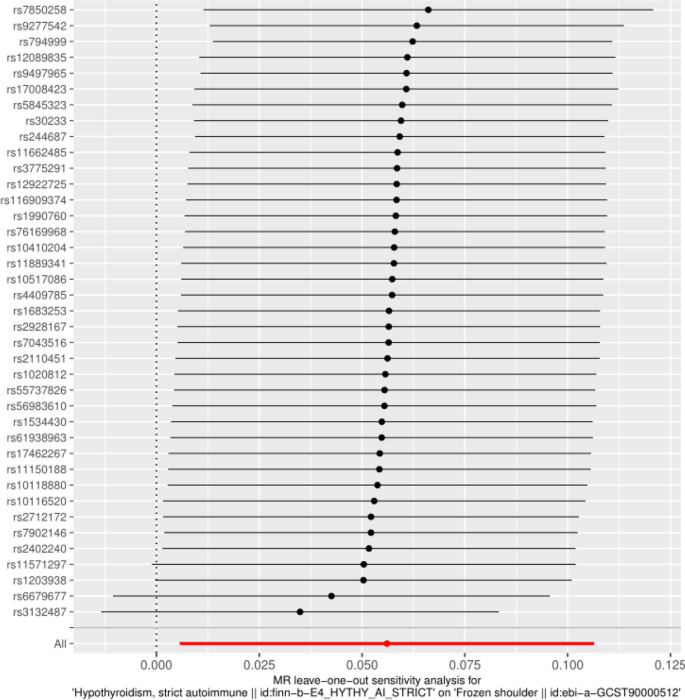
Sensitivity analysis by the leave-one-out method
Reverse Mendelian randomization analysis
In the reverse two-sample MR analysis, frozen shoulder was chosen as the exposure factor, and hypothyroidism as the outcome factor. The same threshold was set, and chain imbalance was eliminated. Finally, four SNPs were included as IVs in the reverse MR analysis. None of the four results from the MR analysis support a causal relationship between genetic susceptibility to frozen shoulder and the risk of hypothyroidism, as shown in Table 3 .
The frequent shoulder ailment known as frozen shoulder is characterized by joint pain and dysfunction. It has a significant negative impact on patient’s quality of life and increases the financial strain on families and society. Frozen shoulder can be caused by various factors, with thyroid disorders being one of them, although the exact causal relationship between them remains unclear.
There is considerable debate over whether hypothyroidism enhances the prevalence of frozen shoulder in the population. Results from Carina Cohen et al. [ 4 ] indicate that thyroid disorders, particularly hypothyroidism and the presence of benign thyroid nodules, significantly contribute to the risk of developing frozen shoulder. These factors increase the likelihood of acquiring the condition by 2.69 times [ 4 ]. A case–control study conducted in China revealed that thyroid disease is associated with an elevated risk of developing frozen shoulder [ 14 ]. Hyung Bin Park et al. also discovered a notable association between subclinical hypothyroidism and frozen shoulder [ 16 ]. Consistent with previous studies, a case–control study from Brazil reported that patients with hypothyroidism were more likely to be diagnosed with frozen shoulder than comparable patients [ 28 ]. However, there are some inconsistencies. Kiera Kingston et al. [ 13 ] discovered hypothyroidism in 8.1% of individuals with adhesive capsulitis; however, this rate was lower than the 10.3% identified in the control population [ 13 ]. Hyung et al. concluded that there was no association between them [ 15 ]. Studies by Chris et al. also questioned the relationship between heart disease, high cholesterol and thyroid disease and frozen shoulder [ 29 ]. All of these studies, we discovered, had poor scores on the evidence-based medicine scale, were vulnerable to a wide range of confounding variables, and carried a number of significant risks of bias. Additionally, conventional observational studies only provide correlations rather than precise causal links.
To overcome this shortcoming, we performed the MR analysis. The results of the two MR methods examined in this study suggest a possible causal relationship between hypothyroidism and frozen shoulder. Importantly, no substantial heterogeneity or pleiotropy was observed in these findings. Our conclusions are similar to those of Deng et al. [ 30 ]. However, our study conducted a reverse Mendelian randomization analysis and had a larger sample size. Several mechanisms may underlie this association. First, fibrosis plays a crucial role in the movement disorders associated with frozen shoulder. Hypothyroidism impairs the synthesis and breakdown of collagen, elastic fibers, and polysaccharides within soft tissues, resulting in tissue edema and fibrosis, contributing to the development of frozen shoulder [ 31 ]. Second, hypothyroidism influences various signaling pathways including growth factors, the extracellular matrix, and calcium signaling, which can impact the differentiation and functionality of osteocytes, leading to bone degeneration and subsequently progressing to frozen shoulder [ 32 ]. Third, hypothyroidism can result in reduced nerve conduction velocity, nerve fiber degeneration, and neuritis, subsequently compromising the sensory and motor functions of nerves and elevating the risk of developing frozen shoulder [ 33 ]. The outcomes of the MR analysis can be used to screen potential risk factors in advance. Accordingly, people with hypothyroidism are more likely to develop frozen shoulder. It is suggested that clinicians should pay attention to the patients with shoulder discomfort when treating hypothyroidism, and provide some ideas for early intervention, which is beneficial to the prognosis of patients.
Our research has some advantages. Firstly, by employing the MR approach, confounding factors and reverse causality were carefully controlled, at least to a large extent. Secondly, our study relied on data derived from previously published GWAS studies, which boasted a substantial sample size and encompassed numerous genetic variants. Moreover, it is worth mentioning that we also used different methods to estimate the impacts, which improves the reliability of our results. However, our MR study still has limitations. First, there may be unobserved pleiotropy beyond vertical pleiotropy. In addition, the samples for this study were all from the European population. Research results based on race may limit their generalizations to other populations. Therefore, large-scale, multi ethnic clinical and basic research may be needed to validate these issues.
With the help of two Mendelian randomization studies, we found that there may be a causal relationship between hypothyroidism and frozen shoulder, and hypothyroidism may be associated with an increased risk of frozen shoulder. However, the exact mechanism remains to be elucidated. More research is required to investigate the underlying mechanisms of this causal relationship.
Availability of data and materials
The datasets used and/or analysed during the current study available from the corresponding author on reasonable request.
Abbreviations
- Mendelian randomization
Genome-Wide Association Studies
Inverse-Variance Weighted
Confidence Interval
Instrumental Variables
Single‐Nucleotide Polymorphisms
Linkage Disequilibrium
Neviaser AS, Neviaser RJ. Adhesive capsulitis of the shoulder. J Am Acad Orthop Surg. 2011;19(9):536–42. https://doi.org/10.5435/00124635-201109000-00004 .
Article PubMed Google Scholar
Hand C, Clipsham K, Rees JL, Carr AJ. Long-term outcome of frozen shoulder. J Shoulder Elbow Surg. 2008;17(2):231–6. https://doi.org/10.1016/j.jse.2007.05.009 .
Hsu JE, Anakwenze OA, Warrender WJ, Abboud JA. Current review of adhesive capsulitis. J Shoulder Elbow Surg. 2011;20(3):502–14. https://doi.org/10.1016/j.jse.2010.08.023 .
Cohen C, Tortato S, Silva OBS, Leal MF, Ejnisman B, Faloppa F. Association between Frozen Shoulder and Thyroid Diseases: Strengthening the Evidences. Rev Bras Ortop (Sao Paulo). 2020;55(4):483–9. https://doi.org/10.1055/s-0039-3402476 .
Sung CM, Jung TS, Park HB. Are serum lipids involved in primary frozen shoulder? A case-control study. J Bone Joint Surg Am. 2014;96(21):1828–33. https://doi.org/10.2106/jbjs.m.00936 .
Huang YP, Fann CY, Chiu YH, Yen MF, Chen LS, Chen HH, et al. Association of diabetes mellitus with the risk of developing adhesive capsulitis of the shoulder: a longitudinal population-based followup study. Arthritis Care Res (Hoboken). 2013;65(7):1197–202. https://doi.org/10.1002/acr.21938 .
Arkkila PE, Kantola IM, Viikari JS, Rönnemaa T. Shoulder capsulitis in type I and II diabetic patients: association with diabetic complications and related diseases. Ann Rheum Dis. 1996;55(12):907–14. https://doi.org/10.1136/ard.55.12.907 .
Article CAS PubMed PubMed Central Google Scholar
Bowman CA, Jeffcoate WJ, Pattrick M, Doherty M. Bilateral adhesive capsulitis, oligoarthritis and proximal myopathy as presentation of hypothyroidism. Br J Rheumatol. 1988;27(1):62–4. https://doi.org/10.1093/rheumatology/27.1.62 .
Article CAS PubMed Google Scholar
Ramirez J. Adhesive capsulitis: diagnosis and management. Am Fam Physician. 2019;99(5):297–300.
PubMed Google Scholar
Wagner S, Nørgaard K, Willaing I, Olesen K, Andersen HU. Upper-extremity impairments in type 1 diabetes: results from a controlled nationwide study. Diabetes Care. 2023;46(6):1204–8. https://doi.org/10.2337/dc23-0063 .
Juel NG, Brox JI, Brunborg C, Holte KB, Berg TJ. Very High prevalence of frozen shoulder in patients with type 1 diabetes of ≥45 years’ duration: the dialong shoulder study. Arch Phys Med Rehabil. 2017;98(8):1551–9. https://doi.org/10.1016/j.apmr.2017.01.020 .
Huang SW, Lin JW, Wang WT, Wu CW, Liou TH, Lin HW. Hyperthyroidism is a risk factor for developing adhesive capsulitis of the shoulder: a nationwide longitudinal population-based study. Sci Rep. 2014;4:4183. https://doi.org/10.1038/srep04183 .
Kingston K, Curry EJ, Galvin JW, Li X. Shoulder adhesive capsulitis: epidemiology and predictors of surgery. J Shoulder Elbow Surg. 2018;27(8):1437–43. https://doi.org/10.1016/j.jse.2018.04.004 .
Li W, Lu N, Xu H, Wang H, Huang J. Case control study of risk factors for frozen shoulder in China. Int J Rheum Dis. 2015;18(5):508–13. https://doi.org/10.1111/1756-185x.12246 .
Park HB, Gwark JY, Jung J, Jeong ST. Association between high-sensitivity C-reactive protein and idiopathic adhesive capsulitis. J Bone Joint Surg Am. 2020;102(9):761–8. https://doi.org/10.2106/jbjs.19.00759 .
Park HB, Gwark JY, Jung J, Jeong ST. Involvement of inflammatory lipoproteinemia with idiopathic adhesive capsulitis accompanying subclinical hypothyroidism. J Shoulder Elbow Surg. 2022;31(10):2121–7. https://doi.org/10.1016/j.jse.2022.03.003 .
Lawlor DA, Harbord RM, Sterne JA, Timpson N, Davey Smith G. Mendelian randomization: using genes as instruments for making causal inferences in epidemiology. Stat Med. 2008;27(8):1133–63. https://doi.org/10.1002/sim.3034 .
Smith GD, Ebrahim S. ‘Mendelian randomization’: can genetic epidemiology contribute to understanding environmental determinants of disease? Int J Epidemiol. 2003;32(1):1–22. https://doi.org/10.1093/ije/dyg070 .
He Y, Zheng C, He MH, Huang JR. The causal relationship between body mass index and the risk of osteoarthritis. Int J Gen Med. 2021;14:2227–37. https://doi.org/10.2147/ijgm.s314180 .
Article PubMed PubMed Central Google Scholar
Evans DM, Davey Smith G. Mendelian randomization: new applications in the coming age of hypothesis-free causality. Annu Rev Genomics Hum Genet. 2015;16:327–50. https://doi.org/10.1146/annurev-genom-090314-050016 .
Burgess S, Butterworth A, Malarstig A, Thompson SG. Use of Mendelian randomisation to assess potential benefit of clinical intervention. BMJ. 2012;345:e7325. https://doi.org/10.1136/bmj.e7325 .
Li MJ, Liu Z, Wang P, Wong MP, Nelson MR, Kocher JP, et al. GWASdb v2: an update database for human genetic variants identified by genome-wide association studies. Nucleic Acids Res. 2016;44(D1):D869–76. https://doi.org/10.1093/nar/gkv1317 .
Green HD, Jones A, Evans JP, Wood AR, Beaumont RN, Tyrrell J, et al. A genome-wide association study identifies 5 loci associated with frozen shoulder and implicates diabetes as a causal risk factor. PLoS Genet. 2021;17(6):e1009577. https://doi.org/10.1371/journal.pgen.1009577 .
Burgess S, Davey Smith G, Davies NM, Dudbridge F, Gill D, Glymour MM, et al. Guidelines for performing Mendelian randomization investigations: update for summer 2023. Wellcome Open Res. 2019;4:186. https://doi.org/10.12688/wellcomeopenres.15555.3 .
Burgess S, Butterworth A, Thompson SG. Mendelian randomization analysis with multiple genetic variants using summarized data. Genet Epidemiol. 2013;37(7):658–65. https://doi.org/10.1002/gepi.21758 .
Bowden J, Del Greco MF, Minelli C, Davey Smith G, Sheehan NA, Thompson JR. Assessing the suitability of summary data for two-sample Mendelian randomization analyses using MR-Egger regression: the role of the I2 statistic. Int J Epidemiol. 2016;45(6):1961–1974. https://doi.org/10.1093/ije/dyw220 .
Hemani G, Zheng J, Elsworth B, Wade KH, Haberland V, Baird D, et al. The MR-Base platform supports systematic causal inference across the human phenome . Elife. 2018;7. https://doi.org/10.7554/eLife.34408 .
Schiefer M, Teixeira PFS, Fontenelle C, Carminatti T, Santos DA, Righi LD, et al. Prevalence of hypothyroidism in patients with frozen shoulder. J Shoulder Elbow Surg. 2017;26(1):49–55. https://doi.org/10.1016/j.jse.2016.04.026 .
Smith CD, White WJ, Bunker TD. The associations of frozen shoulder in patients requiring arthroscopic capsular release. Should Elb. 2012;4(2):87–9. https://doi.org/10.1111/j.1758-5740.2011.00169.x .
Article Google Scholar
Deng G, Wei Y. The causal relationship between hypothyroidism and frozen shoulder: A two-sample Mendelian randomization. Medicine (Baltimore). 2023;102(43):e35650. https://doi.org/10.1097/md.0000000000035650 .
Pandey V, Madi S. Clinical guidelines in the management of frozen shoulder: an update! Indian J Orthop. 2021;55(2):299–309. https://doi.org/10.1007/s43465-021-00351-3 .
Zhu S, Pang Y, Xu J, Chen X, Zhang C, Wu B, et al. Endocrine regulation on bone by thyroid. Front Endocrinol (Lausanne). 2022;13:873820. https://doi.org/10.3389/fendo.2022.873820 .
Baksi S, Pradhan A. Thyroid hormone: sex-dependent role in nervous system regulation and disease. Biol Sex Differ. 2021;12(1):25. https://doi.org/10.1186/s13293-021-00367-2 .
Download references
Acknowledgements
Not applicable.
This study was supported by the Project of State Key Laboratory of Radiation Medicine and Protection, Soochow University (No. GZK12023047).
Author information
Authors and affiliations.
Department of Orthopaedics, The Second Affiliated Hospital of Soochow University, Suzhou, China
Bin Chen, Zheng-hua Zhu, Zhi-cheng Zuo & Kai-long Zhou
State Key Laboratory of Radiation Medicine and Protection, Soochow University, Suzhou, 215123, China
You can also search for this author in PubMed Google Scholar
Contributions
BC: designed research, performed research, collected data, analyzed data, wrote paper. Zh Z, QL and Zc Z: collected data and verification results. Kl Z: designed research and revised article.
Corresponding author
Correspondence to Kai-long Zhou .
Ethics declarations
Ethics approval and consent to participate.
Because the study was based on a public database, did not involve animal or human studies, and was available in the form of open access and anonymous data, Institutional Review Board approval was not required.
Consent for publication
Competing interests.
The authors declare no competing interests.
Additional information
Publisher’s note.
Springer Nature remains neutral with regard to jurisdictional claims in published maps and institutional affiliations.
Supplementary Information
Supplementary material 1., supplementary material 2., rights and permissions.
Open Access This article is licensed under a Creative Commons Attribution-NonCommercial-NoDerivatives 4.0 International License, which permits any non-commercial use, sharing, distribution and reproduction in any medium or format, as long as you give appropriate credit to the original author(s) and the source, provide a link to the Creative Commons licence, and indicate if you modified the licensed material. You do not have permission under this licence to share adapted material derived from this article or parts of it. The images or other third party material in this article are included in the article’s Creative Commons licence, unless indicated otherwise in a credit line to the material. If material is not included in the article’s Creative Commons licence and your intended use is not permitted by statutory regulation or exceeds the permitted use, you will need to obtain permission directly from the copyright holder. To view a copy of this licence, visit http://creativecommons.org/licenses/by-nc-nd/4.0/ .
Reprints and permissions
About this article
Cite this article.
Chen, B., Zhu, Zh., Li, Q. et al. Causal associations of hypothyroidism with frozen shoulder: a two-sample bidirectional Mendelian randomization study. BMC Musculoskelet Disord 25 , 693 (2024). https://doi.org/10.1186/s12891-024-07826-y
Download citation
Received : 03 October 2023
Accepted : 28 August 2024
Published : 02 September 2024
DOI : https://doi.org/10.1186/s12891-024-07826-y
Share this article
Anyone you share the following link with will be able to read this content:
Sorry, a shareable link is not currently available for this article.
Provided by the Springer Nature SharedIt content-sharing initiative
- Frozen shoulder
- Hypothyroidism
BMC Musculoskeletal Disorders
ISSN: 1471-2474
- General enquiries: [email protected]
Have a language expert improve your writing
Run a free plagiarism check in 10 minutes, generate accurate citations for free.
- Knowledge Base
Methodology
- Explanatory Research | Definition, Guide, & Examples
Explanatory Research | Definition, Guide, & Examples
Published on December 3, 2021 by Tegan George and Julia Merkus. Revised on November 20, 2023.
Explanatory research is a research method that explores why something occurs when limited information is available. It can help you increase your understanding of a given topic, ascertain how or why a particular phenomenon is occurring, and predict future occurrences.
Explanatory research can also be explained as a “cause and effect” model, investigating patterns and trends in existing data that haven’t been previously investigated. For this reason, it is often considered a type of causal research .
Table of contents
When to use explanatory research, explanatory research questions, explanatory research data collection, explanatory research data analysis, step-by-step example of explanatory research, explanatory vs. exploratory research, advantages and disadvantages of explanatory research, other interesting articles, frequently asked questions about explanatory research.
Explanatory research is used to investigate how or why a phenomenon takes place. Therefore, this type of research is often one of the first stages in the research process, serving as a jumping-off point for future research. While there is often data available about your topic, it’s possible the particular causal relationship you are interested in has not been robustly studied.
Explanatory research helps you analyze these patterns, formulating hypotheses that can guide future endeavors. If you are seeking a more complete understanding of a relationship between variables, explanatory research is a great place to start. However, keep in mind that it will likely not yield conclusive results.
You analyzed their final grades and noticed that the students who take your course in the first semester always obtain higher grades than students who take the same course in the second semester.
Receive feedback on language, structure, and formatting
Professional editors proofread and edit your paper by focusing on:
- Academic style
- Vague sentences
- Style consistency
See an example
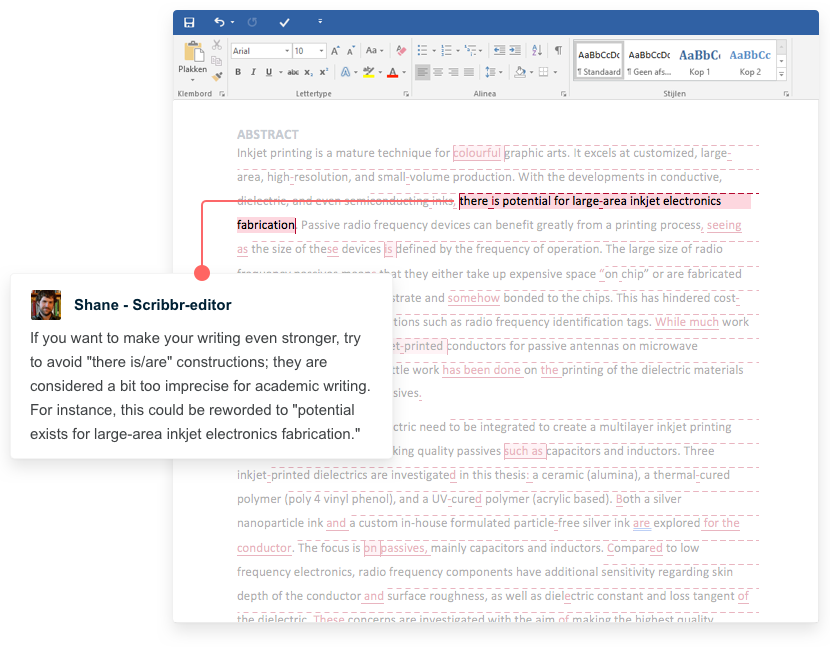
Explanatory research answers “why” and “how” questions, leading to an improved understanding of a previously unresolved problem or providing clarity for related future research initiatives.
Here are a few examples:
- Why do undergraduate students obtain higher average grades in the first semester than in the second semester?
- How does marital status affect labor market participation?
- Why do multilingual individuals show more risky behavior during business negotiations than monolingual individuals?
- How does a child’s ability to delay immediate gratification predict success later in life?
- Why are teens more likely to litter in a highly littered area than in a clean area?
After choosing your research question, there is a variety of options for research and data collection methods to choose from.
A few of the most common research methods include:
- Literature reviews
- Interviews and focus groups
- Pilot studies
- Observations
- Experiments
The method you choose depends on several factors, including your timeline, budget, and the structure of your question. If there is already a body of research on your topic, a literature review is a great place to start. If you are interested in opinions and behavior, consider an interview or focus group format. If you have more time or funding available, an experiment or pilot study may be a good fit for you.
In order to ensure you are conducting your explanatory research correctly, be sure your analysis is definitively causal in nature, and not just correlated.
Always remember the phrase “correlation doesn’t mean causation.” Correlated variables are merely associated with one another: when one variable changes, so does the other. However, this isn’t necessarily due to a direct or indirect causal link.
Causation means that changes in the independent variable bring about changes in the dependent variable. In other words, there is a direct cause-and-effect relationship between variables.
Causal evidence must meet three criteria:
- Temporal : What you define as the “cause” must precede what you define as the “effect.”
- Variation : Intervention must be systematic between your independent variable and dependent variable.
- Non-spurious : Be careful that there are no mitigating factors or hidden third variables that confound your results.
Correlation doesn’t imply causation, but causation always implies correlation. In order to get conclusive causal results, you’ll need to conduct a full experimental design .
Here's why students love Scribbr's proofreading services
Discover proofreading & editing
Your explanatory research design depends on the research method you choose to collect your data . In most cases, you’ll use an experiment to investigate potential causal relationships. We’ll walk you through the steps using an example.
Step 1: Develop the research question
The first step in conducting explanatory research is getting familiar with the topic you’re interested in, so that you can develop a research question .
Let’s say you’re interested in language retention rates in adults.
You are interested in finding out how the duration of exposure to language influences language retention ability later in life.
Step 2: Formulate a hypothesis
The next step is to address your expectations. In some cases, there is literature available on your subject or on a closely related topic that you can use as a foundation for your hypothesis . In other cases, the topic isn’t well studied, and you’ll have to develop your hypothesis based on your instincts or on existing literature on more distant topics.
You phrase your expectations in terms of a null (H 0 ) and alternative hypothesis (H 1 ):
- H 0 : The duration of exposure to a language in infancy does not influence language retention in adults who were adopted from abroad as children.
- H 1 : The duration of exposure to a language in infancy has a positive effect on language retention in adults who were adopted from abroad as children.
Step 3: Design your methodology and collect your data
Next, decide what data collection and data analysis methods you will use and write them up. After carefully designing your research, you can begin to collect your data.
You compare:
- Adults who were adopted from Colombia between 0 and 6 months of age.
- Adults who were adopted from Colombia between 6 and 12 months of age.
- Adults who were adopted from Colombia between 12 and 18 months of age.
- Monolingual adults who have not been exposed to a different language.
During the study, you test their Spanish language proficiency twice in a research design that has three stages:
- Pre-test : You conduct several language proficiency tests to establish any differences between groups pre-intervention.
- Intervention : You provide all groups with 8 hours of Spanish class.
- Post-test : You again conduct several language proficiency tests to establish any differences between groups post-intervention.
You made sure to control for any confounding variables , such as age, gender, proficiency in other languages, etc.
Step 4: Analyze your data and report results
After data collection is complete, proceed to analyze your data and report the results.
You notice that:
- The pre-exposed adults showed higher language proficiency in Spanish than those who had not been pre-exposed. The difference is even greater for the post-test.
- The adults who were adopted between 12 and 18 months of age had a higher Spanish language proficiency level than those who were adopted between 0 and 6 months or 6 and 12 months of age, but there was no difference found between the latter two groups.
To determine whether these differences are significant, you conduct a mixed ANOVA. The ANOVA shows that all differences are not significant for the pre-test, but they are significant for the post-test.
Step 5: Interpret your results and provide suggestions for future research
As you interpret the results, try to come up with explanations for the results that you did not expect. In most cases, you want to provide suggestions for future research.
However, this difference is only significant after the intervention (the Spanish class.)
You decide it’s worth it to further research the matter, and propose a few additional research ideas:
- Replicate the study with a larger sample
- Replicate the study for other maternal languages (e.g. Korean, Lingala, Arabic)
- Replicate the study for other language aspects, such as nativeness of the accent
It can be easy to confuse explanatory research with exploratory research. If you’re in doubt about the relationship between exploratory and explanatory research, just remember that exploratory research lays the groundwork for later explanatory research.
Exploratory research questions often begin with “what”. They are designed to guide future research and do not usually have conclusive results. Exploratory research is often utilized as a first step in your research process, to help you focus your research question and fine-tune your hypotheses.
Explanatory research questions often start with “why” or “how”. They help you study why and how a previously studied phenomenon takes place.
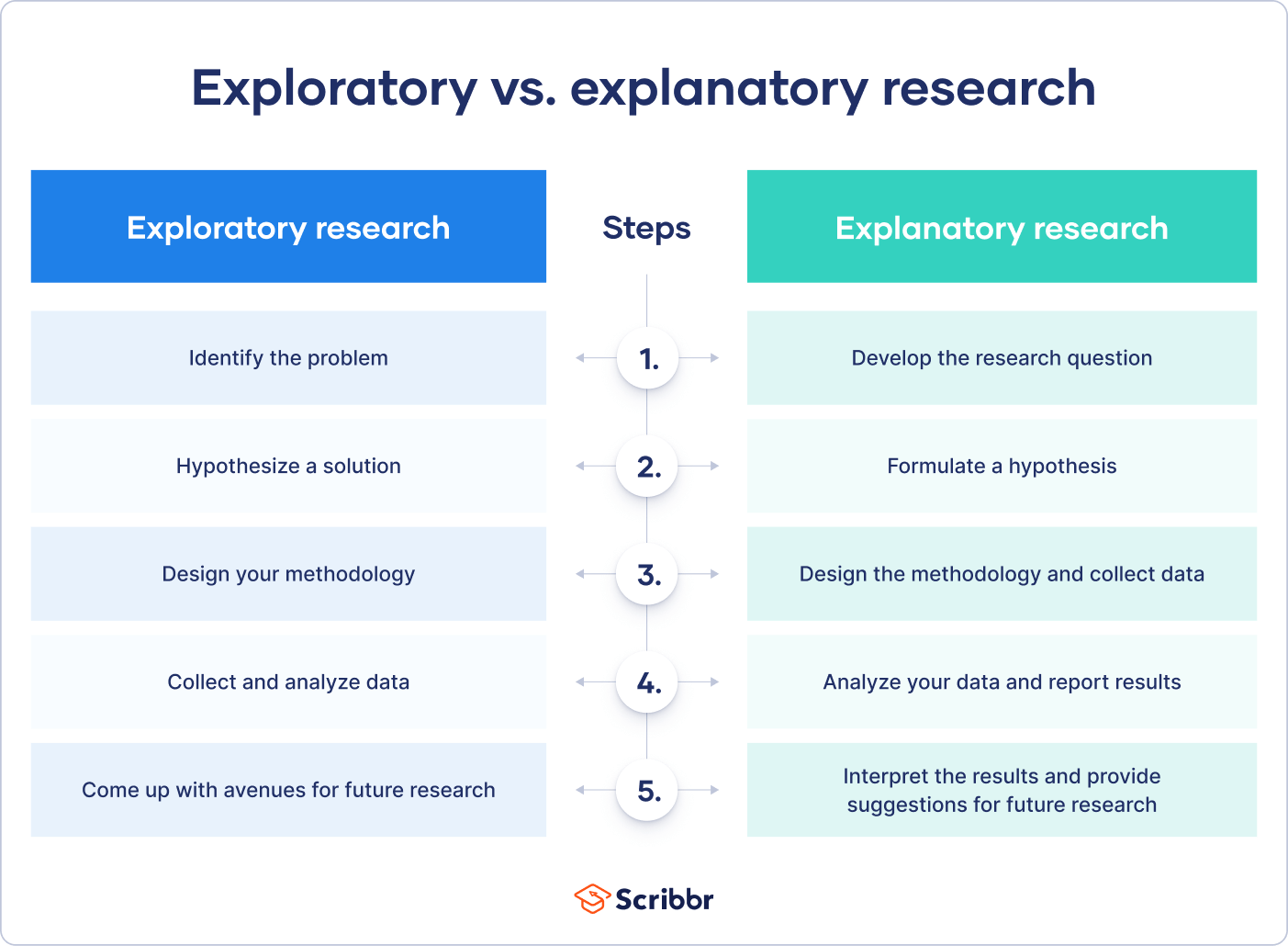
Like any other research design , explanatory research has its trade-offs: while it provides a unique set of benefits, it also has significant downsides:
- It gives more meaning to previous research. It helps fill in the gaps in existing analyses and provides information on the reasons behind phenomena.
- It is very flexible and often replicable , since the internal validity tends to be high when done correctly.
- As you can often use secondary research, explanatory research is often very cost- and time-effective, allowing you to utilize pre-existing resources to guide your research prior to committing to heavier analyses.
Disadvantages
- While explanatory research does help you solidify your theories and hypotheses, it usually lacks conclusive results.
- Results can be biased or inadmissible to a larger body of work and are not generally externally valid . You will likely have to conduct more robust (often quantitative ) research later to bolster any possible findings gleaned from explanatory research.
- Coincidences can be mistaken for causal relationships , and it can sometimes be challenging to ascertain which is the causal variable and which is the effect.
If you want to know more about statistics , methodology , or research bias , make sure to check out some of our other articles with explanations and examples.
- Normal distribution
- Degrees of freedom
- Null hypothesis
- Discourse analysis
- Control groups
- Mixed methods research
- Non-probability sampling
- Quantitative research
- Ecological validity
Research bias
- Rosenthal effect
- Implicit bias
- Cognitive bias
- Selection bias
- Negativity bias
- Status quo bias
Explanatory research is a research method used to investigate how or why something occurs when only a small amount of information is available pertaining to that topic. It can help you increase your understanding of a given topic.
Exploratory research aims to explore the main aspects of an under-researched problem, while explanatory research aims to explain the causes and consequences of a well-defined problem.
Explanatory research is used to investigate how or why a phenomenon occurs. Therefore, this type of research is often one of the first stages in the research process , serving as a jumping-off point for future research.
Quantitative research deals with numbers and statistics, while qualitative research deals with words and meanings.
Quantitative methods allow you to systematically measure variables and test hypotheses . Qualitative methods allow you to explore concepts and experiences in more detail.
Cite this Scribbr article
If you want to cite this source, you can copy and paste the citation or click the “Cite this Scribbr article” button to automatically add the citation to our free Citation Generator.
George, T. & Merkus, J. (2023, November 20). Explanatory Research | Definition, Guide, & Examples. Scribbr. Retrieved September 9, 2024, from https://www.scribbr.com/methodology/explanatory-research/
Is this article helpful?
Tegan George
Other students also liked, exploratory research | definition, guide, & examples, what is a research design | types, guide & examples, qualitative vs. quantitative research | differences, examples & methods, "i thought ai proofreading was useless but..".
I've been using Scribbr for years now and I know it's a service that won't disappoint. It does a good job spotting mistakes”

COMMENTS
Analytical Research: What is it, Importance + Examples
Descriptive and Analytical Research: What's the Difference?
The Beginner's Guide to Statistical Analysis | 5 Steps & ...
What are Analytical Study Designs?
Analytical approaches range from basic comparisons of measurements to more formal statistical tests (see page on establishing differences from expectations). Incorporating predictions of traits into causal analysis is an area of active research, and so we present a hypothetical example below.
In conclusion, the analytical and descriptive approaches have distinct attributes that make them suitable for different research purposes. The analytical approach emphasizes hypothesis testing, quantitative data analysis, and generalizability, allowing researchers to draw evidence-based conclusions and make predictions.
What Is Quantitative Research? | Definition, Uses & Methods
Abstract. In analytical observational studies, researchers try to establish an association between exposure (s) and outcome (s). Depending on the direction of enquiry, these studies can be directed forwards (cohort studies) or backwards (case-control studies). In this article, we examine the key features of these two types of studies.
Descriptive vs Analytical/Critical Writing (+ Examples)
Types of Research Designs Compared | Guide & Examples
Descriptive Analytics. Definition: Descriptive analytics focused on describing or summarizing raw data and making it interpretable. This type of analytics provides insight into what has happened in the past. It involves the analysis of historical data to identify patterns, trends, and insights. Descriptive analytics often uses visualization ...
Analytical studies: a framework for quality improvement ...
Qualitative Data Analysis Methods: Top 6 + Examples
Descriptive research would enable them to establish the scale and scope of homelessness, identifying key demographics and patterns. Analytical research, however, would take these findings and probe deeper into the causes, examining the social, economic, and political factors that contribute to the situation and what can be done to alleviate it.
Introduction. We search for the determinants of health outcomes, first, by relying on descriptive epidemiology to generate hypotheses about associations between exposures and outcomes. Analytic studies are then undertaken to test specific hypotheses. Samples of subjects are identified and information about exposure status and outcome is collected.
Data Analysis Techniques In Research - Methods, Tools & ...
Analytical Research: Examples and Advantages
The literature on qualitative data analysis mostly concerns analyses pertaining to an individual research question and the organization of data within that research question. Few authors have written about the entire qualitative dataset from which multiple and separate analyses could be conducted and reported. The concept of analytic direction is a strategy that can assist qualitative ...
In this analytical research example, the e-commerce company goes beyond merely describing the existing situation (as in descriptive research). It delves into understanding the underlying relationships between various factors and sales, aiming to draw actionable insights to improve business performance.
How to Do Thematic Analysis | Step-by-Step Guide & ...
What Are Analytical Skills? 9 Examples & Tips to Improve
What Is a Research Design | Types, Guide & ...
The GRE Analytical Writing Assessment (AWA) is a vital part of the GRE, assessing your ability to think critically and write analytically. Aiming for a GRE Analytical Writing score above 4.5 is crucial if you're targeting top universities. The updated format features just one task: Analyze an Issue, giving you 30 minutes to write a concise, well-structured essay.
Background Many studies have investigated the association between hypothyroidism and frozen shoulder, but their findings have been inconsistent. Furthermore, earlier research has been primarily observational, which may introduce bias and does not establish a cause-and-effect relationship. To ascertain the causal association, we performed a two-sample bidirectional Mendelian randomization (MR ...
Explanatory Research | Definition, Guide, & Examples