- Privacy Policy

Home » Survey Research – Types, Methods, Examples

Survey Research – Types, Methods, Examples
Table of Contents

Survey Research
Definition:
Survey Research is a quantitative research method that involves collecting standardized data from a sample of individuals or groups through the use of structured questionnaires or interviews. The data collected is then analyzed statistically to identify patterns and relationships between variables, and to draw conclusions about the population being studied.
Survey research can be used to answer a variety of questions, including:
- What are people’s opinions about a certain topic?
- What are people’s experiences with a certain product or service?
- What are people’s beliefs about a certain issue?
Survey Research Methods
Survey Research Methods are as follows:
- Telephone surveys: A survey research method where questions are administered to respondents over the phone, often used in market research or political polling.
- Face-to-face surveys: A survey research method where questions are administered to respondents in person, often used in social or health research.
- Mail surveys: A survey research method where questionnaires are sent to respondents through mail, often used in customer satisfaction or opinion surveys.
- Online surveys: A survey research method where questions are administered to respondents through online platforms, often used in market research or customer feedback.
- Email surveys: A survey research method where questionnaires are sent to respondents through email, often used in customer satisfaction or opinion surveys.
- Mixed-mode surveys: A survey research method that combines two or more survey modes, often used to increase response rates or reach diverse populations.
- Computer-assisted surveys: A survey research method that uses computer technology to administer or collect survey data, often used in large-scale surveys or data collection.
- Interactive voice response surveys: A survey research method where respondents answer questions through a touch-tone telephone system, often used in automated customer satisfaction or opinion surveys.
- Mobile surveys: A survey research method where questions are administered to respondents through mobile devices, often used in market research or customer feedback.
- Group-administered surveys: A survey research method where questions are administered to a group of respondents simultaneously, often used in education or training evaluation.
- Web-intercept surveys: A survey research method where questions are administered to website visitors, often used in website or user experience research.
- In-app surveys: A survey research method where questions are administered to users of a mobile application, often used in mobile app or user experience research.
- Social media surveys: A survey research method where questions are administered to respondents through social media platforms, often used in social media or brand awareness research.
- SMS surveys: A survey research method where questions are administered to respondents through text messaging, often used in customer feedback or opinion surveys.
- IVR surveys: A survey research method where questions are administered to respondents through an interactive voice response system, often used in automated customer feedback or opinion surveys.
- Mixed-method surveys: A survey research method that combines both qualitative and quantitative data collection methods, often used in exploratory or mixed-method research.
- Drop-off surveys: A survey research method where respondents are provided with a survey questionnaire and asked to return it at a later time or through a designated drop-off location.
- Intercept surveys: A survey research method where respondents are approached in public places and asked to participate in a survey, often used in market research or customer feedback.
- Hybrid surveys: A survey research method that combines two or more survey modes, data sources, or research methods, often used in complex or multi-dimensional research questions.
Types of Survey Research
There are several types of survey research that can be used to collect data from a sample of individuals or groups. following are Types of Survey Research:
- Cross-sectional survey: A type of survey research that gathers data from a sample of individuals at a specific point in time, providing a snapshot of the population being studied.
- Longitudinal survey: A type of survey research that gathers data from the same sample of individuals over an extended period of time, allowing researchers to track changes or trends in the population being studied.
- Panel survey: A type of longitudinal survey research that tracks the same sample of individuals over time, typically collecting data at multiple points in time.
- Epidemiological survey: A type of survey research that studies the distribution and determinants of health and disease in a population, often used to identify risk factors and inform public health interventions.
- Observational survey: A type of survey research that collects data through direct observation of individuals or groups, often used in behavioral or social research.
- Correlational survey: A type of survey research that measures the degree of association or relationship between two or more variables, often used to identify patterns or trends in data.
- Experimental survey: A type of survey research that involves manipulating one or more variables to observe the effect on an outcome, often used to test causal hypotheses.
- Descriptive survey: A type of survey research that describes the characteristics or attributes of a population or phenomenon, often used in exploratory research or to summarize existing data.
- Diagnostic survey: A type of survey research that assesses the current state or condition of an individual or system, often used in health or organizational research.
- Explanatory survey: A type of survey research that seeks to explain or understand the causes or mechanisms behind a phenomenon, often used in social or psychological research.
- Process evaluation survey: A type of survey research that measures the implementation and outcomes of a program or intervention, often used in program evaluation or quality improvement.
- Impact evaluation survey: A type of survey research that assesses the effectiveness or impact of a program or intervention, often used to inform policy or decision-making.
- Customer satisfaction survey: A type of survey research that measures the satisfaction or dissatisfaction of customers with a product, service, or experience, often used in marketing or customer service research.
- Market research survey: A type of survey research that collects data on consumer preferences, behaviors, or attitudes, often used in market research or product development.
- Public opinion survey: A type of survey research that measures the attitudes, beliefs, or opinions of a population on a specific issue or topic, often used in political or social research.
- Behavioral survey: A type of survey research that measures actual behavior or actions of individuals, often used in health or social research.
- Attitude survey: A type of survey research that measures the attitudes, beliefs, or opinions of individuals, often used in social or psychological research.
- Opinion poll: A type of survey research that measures the opinions or preferences of a population on a specific issue or topic, often used in political or media research.
- Ad hoc survey: A type of survey research that is conducted for a specific purpose or research question, often used in exploratory research or to answer a specific research question.
Types Based on Methodology
Based on Methodology Survey are divided into two Types:
Quantitative Survey Research
Qualitative survey research.
Quantitative survey research is a method of collecting numerical data from a sample of participants through the use of standardized surveys or questionnaires. The purpose of quantitative survey research is to gather empirical evidence that can be analyzed statistically to draw conclusions about a particular population or phenomenon.
In quantitative survey research, the questions are structured and pre-determined, often utilizing closed-ended questions, where participants are given a limited set of response options to choose from. This approach allows for efficient data collection and analysis, as well as the ability to generalize the findings to a larger population.
Quantitative survey research is often used in market research, social sciences, public health, and other fields where numerical data is needed to make informed decisions and recommendations.
Qualitative survey research is a method of collecting non-numerical data from a sample of participants through the use of open-ended questions or semi-structured interviews. The purpose of qualitative survey research is to gain a deeper understanding of the experiences, perceptions, and attitudes of participants towards a particular phenomenon or topic.
In qualitative survey research, the questions are open-ended, allowing participants to share their thoughts and experiences in their own words. This approach allows for a rich and nuanced understanding of the topic being studied, and can provide insights that are difficult to capture through quantitative methods alone.
Qualitative survey research is often used in social sciences, education, psychology, and other fields where a deeper understanding of human experiences and perceptions is needed to inform policy, practice, or theory.
Data Analysis Methods
There are several Survey Research Data Analysis Methods that researchers may use, including:
- Descriptive statistics: This method is used to summarize and describe the basic features of the survey data, such as the mean, median, mode, and standard deviation. These statistics can help researchers understand the distribution of responses and identify any trends or patterns.
- Inferential statistics: This method is used to make inferences about the larger population based on the data collected in the survey. Common inferential statistical methods include hypothesis testing, regression analysis, and correlation analysis.
- Factor analysis: This method is used to identify underlying factors or dimensions in the survey data. This can help researchers simplify the data and identify patterns and relationships that may not be immediately apparent.
- Cluster analysis: This method is used to group similar respondents together based on their survey responses. This can help researchers identify subgroups within the larger population and understand how different groups may differ in their attitudes, behaviors, or preferences.
- Structural equation modeling: This method is used to test complex relationships between variables in the survey data. It can help researchers understand how different variables may be related to one another and how they may influence one another.
- Content analysis: This method is used to analyze open-ended responses in the survey data. Researchers may use software to identify themes or categories in the responses, or they may manually review and code the responses.
- Text mining: This method is used to analyze text-based survey data, such as responses to open-ended questions. Researchers may use software to identify patterns and themes in the text, or they may manually review and code the text.
Applications of Survey Research
Here are some common applications of survey research:
- Market Research: Companies use survey research to gather insights about customer needs, preferences, and behavior. These insights are used to create marketing strategies and develop new products.
- Public Opinion Research: Governments and political parties use survey research to understand public opinion on various issues. This information is used to develop policies and make decisions.
- Social Research: Survey research is used in social research to study social trends, attitudes, and behavior. Researchers use survey data to explore topics such as education, health, and social inequality.
- Academic Research: Survey research is used in academic research to study various phenomena. Researchers use survey data to test theories, explore relationships between variables, and draw conclusions.
- Customer Satisfaction Research: Companies use survey research to gather information about customer satisfaction with their products and services. This information is used to improve customer experience and retention.
- Employee Surveys: Employers use survey research to gather feedback from employees about their job satisfaction, working conditions, and organizational culture. This information is used to improve employee retention and productivity.
- Health Research: Survey research is used in health research to study topics such as disease prevalence, health behaviors, and healthcare access. Researchers use survey data to develop interventions and improve healthcare outcomes.
Examples of Survey Research
Here are some real-time examples of survey research:
- COVID-19 Pandemic Surveys: Since the outbreak of the COVID-19 pandemic, surveys have been conducted to gather information about public attitudes, behaviors, and perceptions related to the pandemic. Governments and healthcare organizations have used this data to develop public health strategies and messaging.
- Political Polls During Elections: During election seasons, surveys are used to measure public opinion on political candidates, policies, and issues in real-time. This information is used by political parties to develop campaign strategies and make decisions.
- Customer Feedback Surveys: Companies often use real-time customer feedback surveys to gather insights about customer experience and satisfaction. This information is used to improve products and services quickly.
- Event Surveys: Organizers of events such as conferences and trade shows often use surveys to gather feedback from attendees in real-time. This information can be used to improve future events and make adjustments during the current event.
- Website and App Surveys: Website and app owners use surveys to gather real-time feedback from users about the functionality, user experience, and overall satisfaction with their platforms. This feedback can be used to improve the user experience and retain customers.
- Employee Pulse Surveys: Employers use real-time pulse surveys to gather feedback from employees about their work experience and overall job satisfaction. This feedback is used to make changes in real-time to improve employee retention and productivity.
Survey Sample
Purpose of survey research.
The purpose of survey research is to gather data and insights from a representative sample of individuals. Survey research allows researchers to collect data quickly and efficiently from a large number of people, making it a valuable tool for understanding attitudes, behaviors, and preferences.
Here are some common purposes of survey research:
- Descriptive Research: Survey research is often used to describe characteristics of a population or a phenomenon. For example, a survey could be used to describe the characteristics of a particular demographic group, such as age, gender, or income.
- Exploratory Research: Survey research can be used to explore new topics or areas of research. Exploratory surveys are often used to generate hypotheses or identify potential relationships between variables.
- Explanatory Research: Survey research can be used to explain relationships between variables. For example, a survey could be used to determine whether there is a relationship between educational attainment and income.
- Evaluation Research: Survey research can be used to evaluate the effectiveness of a program or intervention. For example, a survey could be used to evaluate the impact of a health education program on behavior change.
- Monitoring Research: Survey research can be used to monitor trends or changes over time. For example, a survey could be used to monitor changes in attitudes towards climate change or political candidates over time.
When to use Survey Research
there are certain circumstances where survey research is particularly appropriate. Here are some situations where survey research may be useful:
- When the research question involves attitudes, beliefs, or opinions: Survey research is particularly useful for understanding attitudes, beliefs, and opinions on a particular topic. For example, a survey could be used to understand public opinion on a political issue.
- When the research question involves behaviors or experiences: Survey research can also be useful for understanding behaviors and experiences. For example, a survey could be used to understand the prevalence of a particular health behavior.
- When a large sample size is needed: Survey research allows researchers to collect data from a large number of people quickly and efficiently. This makes it a useful method when a large sample size is needed to ensure statistical validity.
- When the research question is time-sensitive: Survey research can be conducted quickly, which makes it a useful method when the research question is time-sensitive. For example, a survey could be used to understand public opinion on a breaking news story.
- When the research question involves a geographically dispersed population: Survey research can be conducted online, which makes it a useful method when the population of interest is geographically dispersed.
How to Conduct Survey Research
Conducting survey research involves several steps that need to be carefully planned and executed. Here is a general overview of the process:
- Define the research question: The first step in conducting survey research is to clearly define the research question. The research question should be specific, measurable, and relevant to the population of interest.
- Develop a survey instrument : The next step is to develop a survey instrument. This can be done using various methods, such as online survey tools or paper surveys. The survey instrument should be designed to elicit the information needed to answer the research question, and should be pre-tested with a small sample of individuals.
- Select a sample : The sample is the group of individuals who will be invited to participate in the survey. The sample should be representative of the population of interest, and the size of the sample should be sufficient to ensure statistical validity.
- Administer the survey: The survey can be administered in various ways, such as online, by mail, or in person. The method of administration should be chosen based on the population of interest and the research question.
- Analyze the data: Once the survey data is collected, it needs to be analyzed. This involves summarizing the data using statistical methods, such as frequency distributions or regression analysis.
- Draw conclusions: The final step is to draw conclusions based on the data analysis. This involves interpreting the results and answering the research question.
Advantages of Survey Research
There are several advantages to using survey research, including:
- Efficient data collection: Survey research allows researchers to collect data quickly and efficiently from a large number of people. This makes it a useful method for gathering information on a wide range of topics.
- Standardized data collection: Surveys are typically standardized, which means that all participants receive the same questions in the same order. This ensures that the data collected is consistent and reliable.
- Cost-effective: Surveys can be conducted online, by mail, or in person, which makes them a cost-effective method of data collection.
- Anonymity: Participants can remain anonymous when responding to a survey. This can encourage participants to be more honest and open in their responses.
- Easy comparison: Surveys allow for easy comparison of data between different groups or over time. This makes it possible to identify trends and patterns in the data.
- Versatility: Surveys can be used to collect data on a wide range of topics, including attitudes, beliefs, behaviors, and preferences.
Limitations of Survey Research
Here are some of the main limitations of survey research:
- Limited depth: Surveys are typically designed to collect quantitative data, which means that they do not provide much depth or detail about people’s experiences or opinions. This can limit the insights that can be gained from the data.
- Potential for bias: Surveys can be affected by various biases, including selection bias, response bias, and social desirability bias. These biases can distort the results and make them less accurate.
- L imited validity: Surveys are only as valid as the questions they ask. If the questions are poorly designed or ambiguous, the results may not accurately reflect the respondents’ attitudes or behaviors.
- Limited generalizability : Survey results are only generalizable to the population from which the sample was drawn. If the sample is not representative of the population, the results may not be generalizable to the larger population.
- Limited ability to capture context: Surveys typically do not capture the context in which attitudes or behaviors occur. This can make it difficult to understand the reasons behind the responses.
- Limited ability to capture complex phenomena: Surveys are not well-suited to capture complex phenomena, such as emotions or the dynamics of interpersonal relationships.
Following is an example of a Survey Sample:
Welcome to our Survey Research Page! We value your opinions and appreciate your participation in this survey. Please answer the questions below as honestly and thoroughly as possible.
1. What is your age?
- A) Under 18
- G) 65 or older
2. What is your highest level of education completed?
- A) Less than high school
- B) High school or equivalent
- C) Some college or technical school
- D) Bachelor’s degree
- E) Graduate or professional degree
3. What is your current employment status?
- A) Employed full-time
- B) Employed part-time
- C) Self-employed
- D) Unemployed
4. How often do you use the internet per day?
- A) Less than 1 hour
- B) 1-3 hours
- C) 3-5 hours
- D) 5-7 hours
- E) More than 7 hours
5. How often do you engage in social media per day?
6. Have you ever participated in a survey research study before?
7. If you have participated in a survey research study before, how was your experience?
- A) Excellent
- E) Very poor
8. What are some of the topics that you would be interested in participating in a survey research study about?
……………………………………………………………………………………………………………………………………………………………………………………………………………………………………………………………………….
9. How often would you be willing to participate in survey research studies?
- A) Once a week
- B) Once a month
- C) Once every 6 months
- D) Once a year
10. Any additional comments or suggestions?
Thank you for taking the time to complete this survey. Your feedback is important to us and will help us improve our survey research efforts.
About the author
Muhammad Hassan
Researcher, Academic Writer, Web developer
You may also like

Ethnographic Research -Types, Methods and Guide

Exploratory Research – Types, Methods and...

Focus Groups – Steps, Examples and Guide

Triangulation in Research – Types, Methods and...

Explanatory Research – Types, Methods, Guide

Phenomenology – Methods, Examples and Guide
- Skip to main content
- Skip to primary sidebar
- Skip to footer
- QuestionPro

- Solutions Industries Gaming Automotive Sports and events Education Government Travel & Hospitality Financial Services Healthcare Cannabis Technology Use Case AskWhy Communities Audience Contactless surveys Mobile LivePolls Member Experience GDPR Positive People Science 360 Feedback Surveys
- Resources Blog eBooks Survey Templates Case Studies Training Help center

Home Market Research
Survey Research: Definition, Examples and Methods
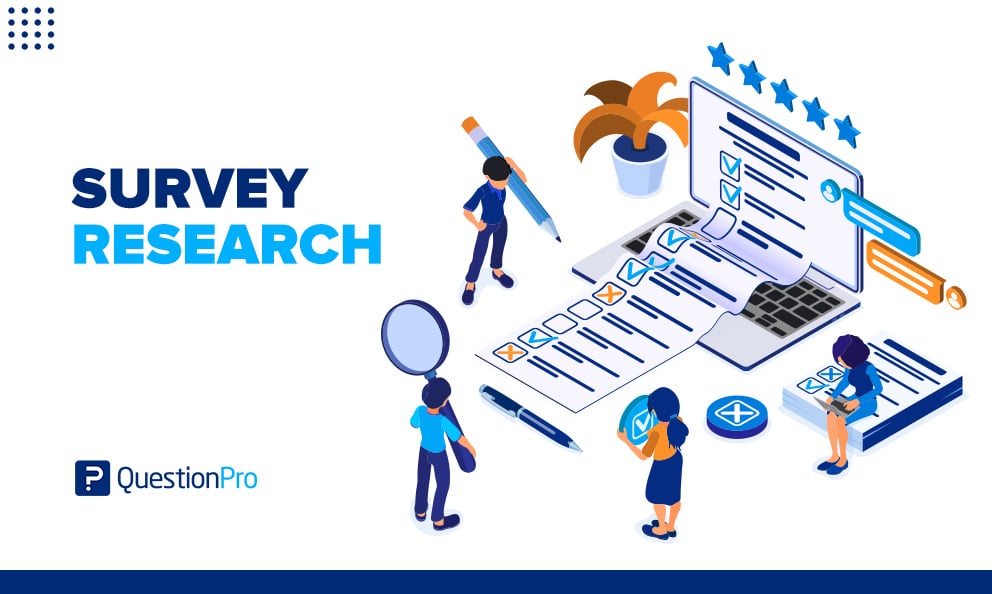
Survey Research is a quantitative research method used for collecting data from a set of respondents. It has been perhaps one of the most used methodologies in the industry for several years due to the multiple benefits and advantages that it has when collecting and analyzing data.
LEARN ABOUT: Behavioral Research
In this article, you will learn everything about survey research, such as types, methods, and examples.
Survey Research Definition
Survey Research is defined as the process of conducting research using surveys that researchers send to survey respondents. The data collected from surveys is then statistically analyzed to draw meaningful research conclusions. In the 21st century, every organization’s eager to understand what their customers think about their products or services and make better business decisions. Researchers can conduct research in multiple ways, but surveys are proven to be one of the most effective and trustworthy research methods. An online survey is a method for extracting information about a significant business matter from an individual or a group of individuals. It consists of structured survey questions that motivate the participants to respond. Creditable survey research can give these businesses access to a vast information bank. Organizations in media, other companies, and even governments rely on survey research to obtain accurate data.
The traditional definition of survey research is a quantitative method for collecting information from a pool of respondents by asking multiple survey questions. This research type includes the recruitment of individuals collection, and analysis of data. It’s useful for researchers who aim to communicate new features or trends to their respondents.
LEARN ABOUT: Level of Analysis Generally, it’s the primary step towards obtaining quick information about mainstream topics and conducting more rigorous and detailed quantitative research methods like surveys/polls or qualitative research methods like focus groups/on-call interviews can follow. There are many situations where researchers can conduct research using a blend of both qualitative and quantitative strategies.
LEARN ABOUT: Survey Sampling
Survey Research Methods
Survey research methods can be derived based on two critical factors: Survey research tool and time involved in conducting research. There are three main survey research methods, divided based on the medium of conducting survey research:
- Online/ Email: Online survey research is one of the most popular survey research methods today. The survey cost involved in online survey research is extremely minimal, and the responses gathered are highly accurate.
- Phone: Survey research conducted over the telephone ( CATI survey ) can be useful in collecting data from a more extensive section of the target population. There are chances that the money invested in phone surveys will be higher than other mediums, and the time required will be higher.
- Face-to-face: Researchers conduct face-to-face in-depth interviews in situations where there is a complicated problem to solve. The response rate for this method is the highest, but it can be costly.
Further, based on the time taken, survey research can be classified into two methods:
- Longitudinal survey research: Longitudinal survey research involves conducting survey research over a continuum of time and spread across years and decades. The data collected using this survey research method from one time period to another is qualitative or quantitative. Respondent behavior, preferences, and attitudes are continuously observed over time to analyze reasons for a change in behavior or preferences. For example, suppose a researcher intends to learn about the eating habits of teenagers. In that case, he/she will follow a sample of teenagers over a considerable period to ensure that the collected information is reliable. Often, cross-sectional survey research follows a longitudinal study .
- Cross-sectional survey research: Researchers conduct a cross-sectional survey to collect insights from a target audience at a particular time interval. This survey research method is implemented in various sectors such as retail, education, healthcare, SME businesses, etc. Cross-sectional studies can either be descriptive or analytical. It is quick and helps researchers collect information in a brief period. Researchers rely on the cross-sectional survey research method in situations where descriptive analysis of a subject is required.
Survey research also is bifurcated according to the sampling methods used to form samples for research: Probability and Non-probability sampling. Every individual in a population should be considered equally to be a part of the survey research sample. Probability sampling is a sampling method in which the researcher chooses the elements based on probability theory. The are various probability research methods, such as simple random sampling , systematic sampling, cluster sampling, stratified random sampling, etc. Non-probability sampling is a sampling method where the researcher uses his/her knowledge and experience to form samples.
LEARN ABOUT: Survey Sample Sizes
The various non-probability sampling techniques are :
- Convenience sampling
- Snowball sampling
- Consecutive sampling
- Judgemental sampling
- Quota sampling
Process of implementing survey research methods:
- Decide survey questions: Brainstorm and put together valid survey questions that are grammatically and logically appropriate. Understanding the objective and expected outcomes of the survey helps a lot. There are many surveys where details of responses are not as important as gaining insights about what customers prefer from the provided options. In such situations, a researcher can include multiple-choice questions or closed-ended questions . Whereas, if researchers need to obtain details about specific issues, they can consist of open-ended questions in the questionnaire. Ideally, the surveys should include a smart balance of open-ended and closed-ended questions. Use survey questions like Likert Scale , Semantic Scale, Net Promoter Score question, etc., to avoid fence-sitting.
LEARN ABOUT: System Usability Scale
- Finalize a target audience: Send out relevant surveys as per the target audience and filter out irrelevant questions as per the requirement. The survey research will be instrumental in case the target population decides on a sample. This way, results can be according to the desired market and be generalized to the entire population.
LEARN ABOUT: Testimonial Questions
- Send out surveys via decided mediums: Distribute the surveys to the target audience and patiently wait for the feedback and comments- this is the most crucial step of the survey research. The survey needs to be scheduled, keeping in mind the nature of the target audience and its regions. Surveys can be conducted via email, embedded in a website, shared via social media, etc., to gain maximum responses.
- Analyze survey results: Analyze the feedback in real-time and identify patterns in the responses which might lead to a much-needed breakthrough for your organization. GAP, TURF Analysis , Conjoint analysis, Cross tabulation, and many such survey feedback analysis methods can be used to spot and shed light on respondent behavior. Use a good survey analysis software . Researchers can use the results to implement corrective measures to improve customer/employee satisfaction.
Reasons to conduct survey research
The most crucial and integral reason for conducting market research using surveys is that you can collect answers regarding specific, essential questions. You can ask these questions in multiple survey formats as per the target audience and the intent of the survey. Before designing a study, every organization must figure out the objective of carrying this out so that the study can be structured, planned, and executed to perfection.
LEARN ABOUT: Research Process Steps
Questions that need to be on your mind while designing a survey are:
- What is the primary aim of conducting the survey?
- How do you plan to utilize the collected survey data?
- What type of decisions do you plan to take based on the points mentioned above?
There are three critical reasons why an organization must conduct survey research.
- Understand respondent behavior to get solutions to your queries: If you’ve carefully curated a survey, the respondents will provide insights about what they like about your organization as well as suggestions for improvement. To motivate them to respond, you must be very vocal about how secure their responses will be and how you will utilize the answers. This will push them to be 100% honest about their feedback, opinions, and comments. Online surveys or mobile surveys have proved their privacy, and due to this, more and more respondents feel free to put forth their feedback through these mediums.
- Present a medium for discussion: A survey can be the perfect platform for respondents to provide criticism or applause for an organization. Important topics like product quality or quality of customer service etc., can be put on the table for discussion. A way you can do it is by including open-ended questions where the respondents can write their thoughts. This will make it easy for you to correlate your survey to what you intend to do with your product or service.
- Strategy for never-ending improvements: An organization can establish the target audience’s attributes from the pilot phase of survey research . Researchers can use the criticism and feedback received from this survey to improve the product/services. Once the company successfully makes the improvements, it can send out another survey to measure the change in feedback keeping the pilot phase the benchmark. By doing this activity, the organization can track what was effectively improved and what still needs improvement.
Survey Research Scales
There are four main scales for the measurement of variables:
- Nominal Scale: A nominal scale associates numbers with variables for mere naming or labeling, and the numbers usually have no other relevance. It is the most basic of the four levels of measurement.
- Ordinal Scale: The ordinal scale has an innate order within the variables along with labels. It establishes the rank between the variables of a scale but not the difference value between the variables.
- Interval Scale: The interval scale is a step ahead in comparison to the other two scales. Along with establishing a rank and name of variables, the scale also makes known the difference between the two variables. The only drawback is that there is no fixed start point of the scale, i.e., the actual zero value is absent.
- Ratio Scale: The ratio scale is the most advanced measurement scale, which has variables that are labeled in order and have a calculated difference between variables. In addition to what interval scale orders, this scale has a fixed starting point, i.e., the actual zero value is present.
Benefits of survey research
In case survey research is used for all the right purposes and is implemented properly, marketers can benefit by gaining useful, trustworthy data that they can use to better the ROI of the organization.
Other benefits of survey research are:
- Minimum investment: Mobile surveys and online surveys have minimal finance invested per respondent. Even with the gifts and other incentives provided to the people who participate in the study, online surveys are extremely economical compared to paper-based surveys.
- Versatile sources for response collection: You can conduct surveys via various mediums like online and mobile surveys. You can further classify them into qualitative mediums like focus groups , and interviews and quantitative mediums like customer-centric surveys. Due to the offline survey response collection option, researchers can conduct surveys in remote areas with limited internet connectivity. This can make data collection and analysis more convenient and extensive.
- Reliable for respondents: Surveys are extremely secure as the respondent details and responses are kept safeguarded. This anonymity makes respondents answer the survey questions candidly and with absolute honesty. An organization seeking to receive explicit responses for its survey research must mention that it will be confidential.
Survey research design
Researchers implement a survey research design in cases where there is a limited cost involved and there is a need to access details easily. This method is often used by small and large organizations to understand and analyze new trends, market demands, and opinions. Collecting information through tactfully designed survey research can be much more effective and productive than a casually conducted survey.
There are five stages of survey research design:
- Decide an aim of the research: There can be multiple reasons for a researcher to conduct a survey, but they need to decide a purpose for the research. This is the primary stage of survey research as it can mold the entire path of a survey, impacting its results.
- Filter the sample from target population: Who to target? is an essential question that a researcher should answer and keep in mind while conducting research. The precision of the results is driven by who the members of a sample are and how useful their opinions are. The quality of respondents in a sample is essential for the results received for research and not the quantity. If a researcher seeks to understand whether a product feature will work well with their target market, he/she can conduct survey research with a group of market experts for that product or technology.
- Zero-in on a survey method: Many qualitative and quantitative research methods can be discussed and decided. Focus groups, online interviews, surveys, polls, questionnaires, etc. can be carried out with a pre-decided sample of individuals.
- Design the questionnaire: What will the content of the survey be? A researcher is required to answer this question to be able to design it effectively. What will the content of the cover letter be? Or what are the survey questions of this questionnaire? Understand the target market thoroughly to create a questionnaire that targets a sample to gain insights about a survey research topic.
- Send out surveys and analyze results: Once the researcher decides on which questions to include in a study, they can send it across to the selected sample . Answers obtained from this survey can be analyzed to make product-related or marketing-related decisions.
Survey examples: 10 tips to design the perfect research survey
Picking the right survey design can be the key to gaining the information you need to make crucial decisions for all your research. It is essential to choose the right topic, choose the right question types, and pick a corresponding design. If this is your first time creating a survey, it can seem like an intimidating task. But with QuestionPro, each step of the process is made simple and easy.
Below are 10 Tips To Design The Perfect Research Survey:
- Set your SMART goals: Before conducting any market research or creating a particular plan, set your SMART Goals . What is that you want to achieve with the survey? How will you measure it promptly, and what are the results you are expecting?
- Choose the right questions: Designing a survey can be a tricky task. Asking the right questions may help you get the answers you are looking for and ease the task of analyzing. So, always choose those specific questions – relevant to your research.
- Begin your survey with a generalized question: Preferably, start your survey with a general question to understand whether the respondent uses the product or not. That also provides an excellent base and intro for your survey.
- Enhance your survey: Choose the best, most relevant, 15-20 questions. Frame each question as a different question type based on the kind of answer you would like to gather from each. Create a survey using different types of questions such as multiple-choice, rating scale, open-ended, etc. Look at more survey examples and four measurement scales every researcher should remember.
- Prepare yes/no questions: You may also want to use yes/no questions to separate people or branch them into groups of those who “have purchased” and those who “have not yet purchased” your products or services. Once you separate them, you can ask them different questions.
- Test all electronic devices: It becomes effortless to distribute your surveys if respondents can answer them on different electronic devices like mobiles, tablets, etc. Once you have created your survey, it’s time to TEST. You can also make any corrections if needed at this stage.
- Distribute your survey: Once your survey is ready, it is time to share and distribute it to the right audience. You can share handouts and share them via email, social media, and other industry-related offline/online communities.
- Collect and analyze responses: After distributing your survey, it is time to gather all responses. Make sure you store your results in a particular document or an Excel sheet with all the necessary categories mentioned so that you don’t lose your data. Remember, this is the most crucial stage. Segregate your responses based on demographics, psychographics, and behavior. This is because, as a researcher, you must know where your responses are coming from. It will help you to analyze, predict decisions, and help write the summary report.
- Prepare your summary report: Now is the time to share your analysis. At this stage, you should mention all the responses gathered from a survey in a fixed format. Also, the reader/customer must get clarity about your goal, which you were trying to gain from the study. Questions such as – whether the product or service has been used/preferred or not. Do respondents prefer some other product to another? Any recommendations?
Having a tool that helps you carry out all the necessary steps to carry out this type of study is a vital part of any project. At QuestionPro, we have helped more than 10,000 clients around the world to carry out data collection in a simple and effective way, in addition to offering a wide range of solutions to take advantage of this data in the best possible way.
From dashboards, advanced analysis tools, automation, and dedicated functions, in QuestionPro, you will find everything you need to execute your research projects effectively. Uncover insights that matter the most!
MORE LIKE THIS
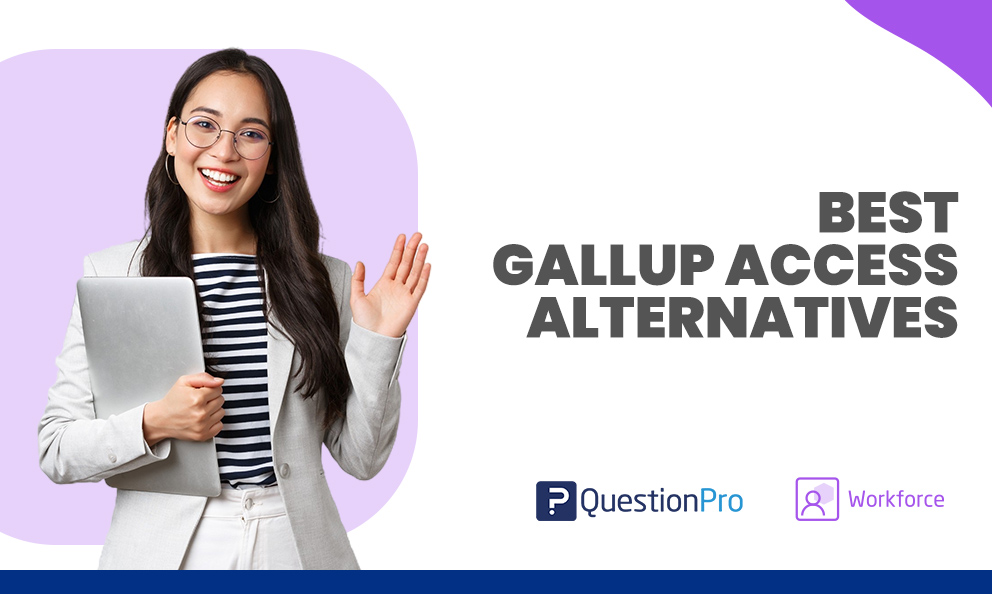
Best Gallup Access Alternatives & Competitors in 2024
Sep 6, 2024
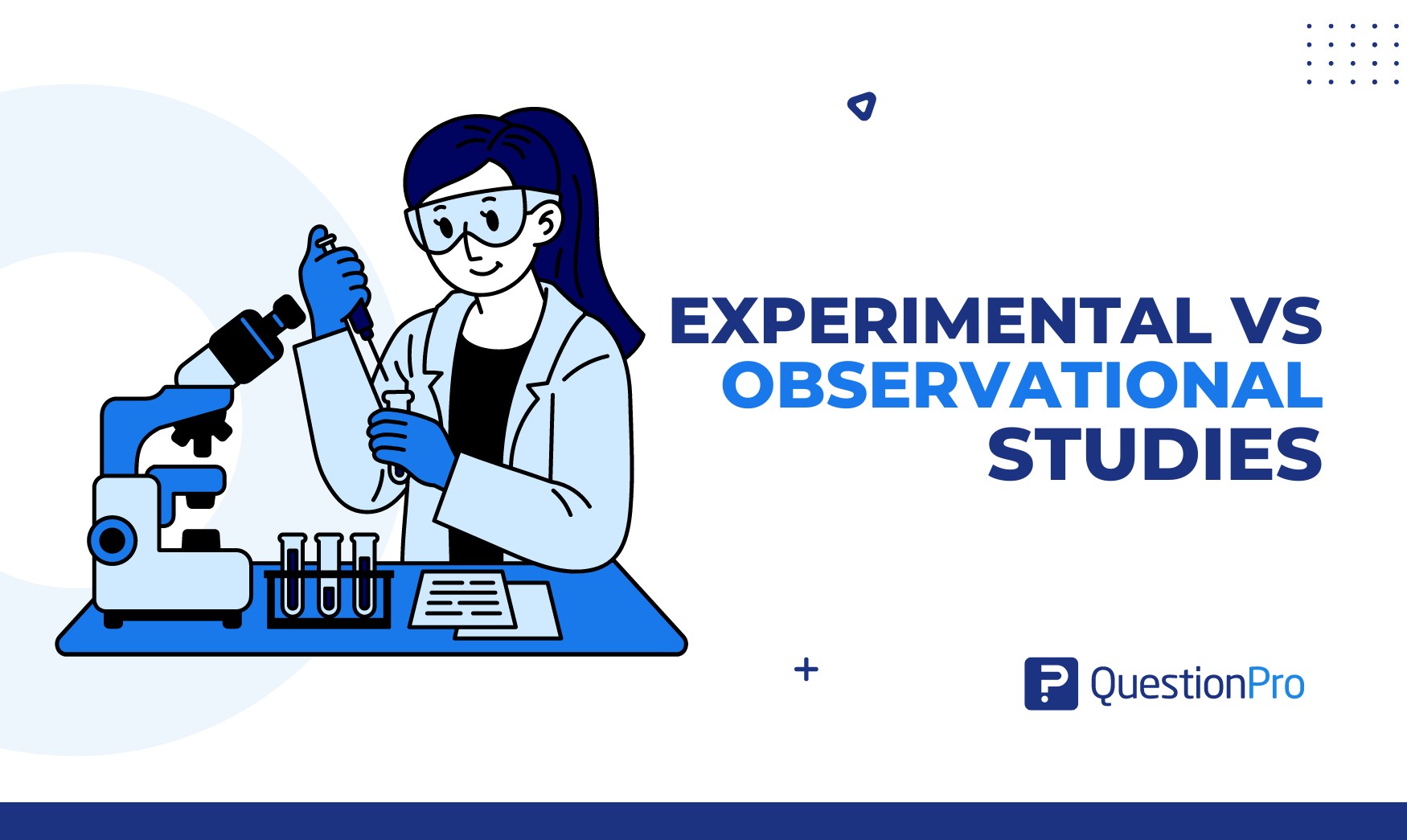
Experimental vs Observational Studies: Differences & Examples
Sep 5, 2024
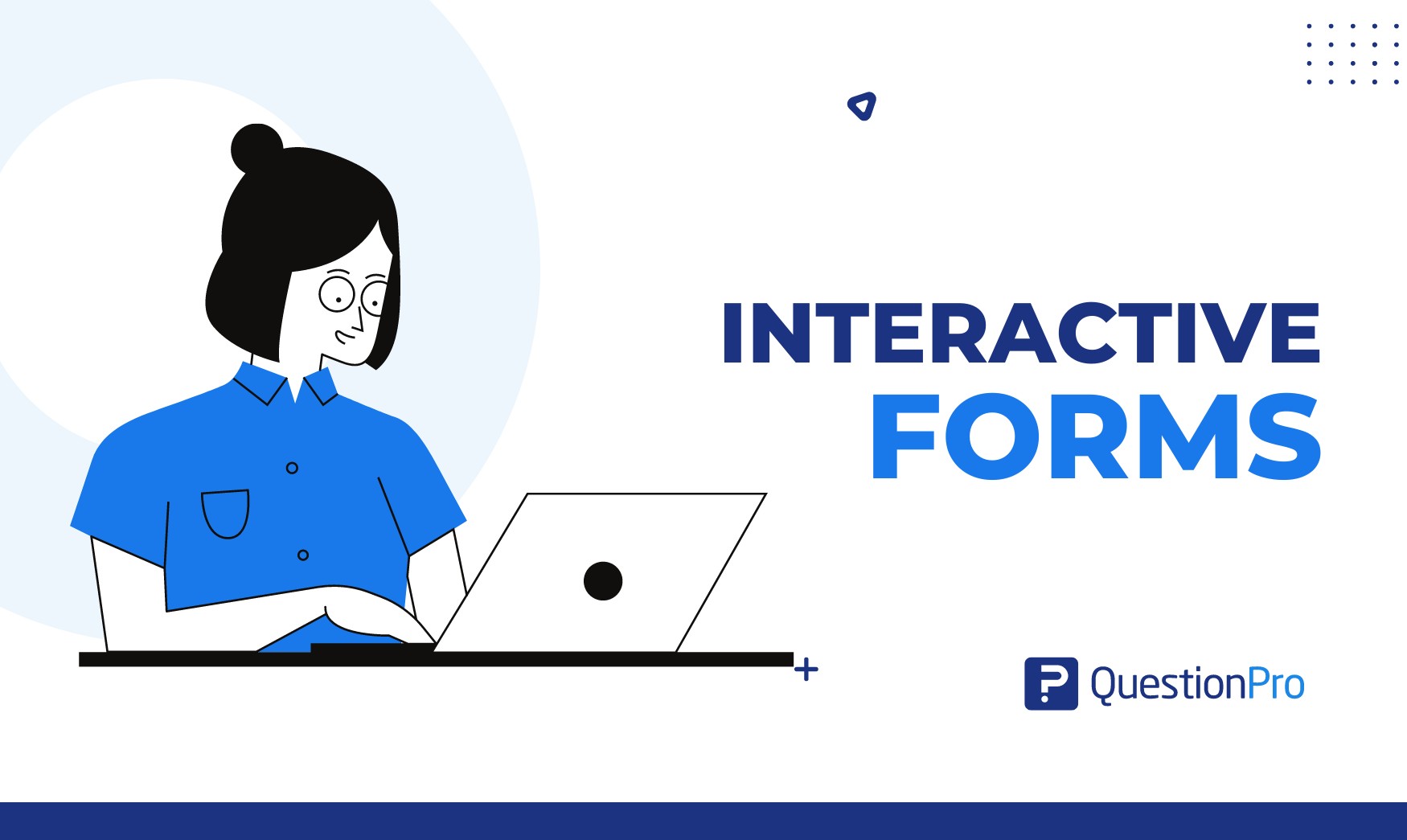
Interactive Forms: Key Features, Benefits, Uses + Design Tips
Sep 4, 2024
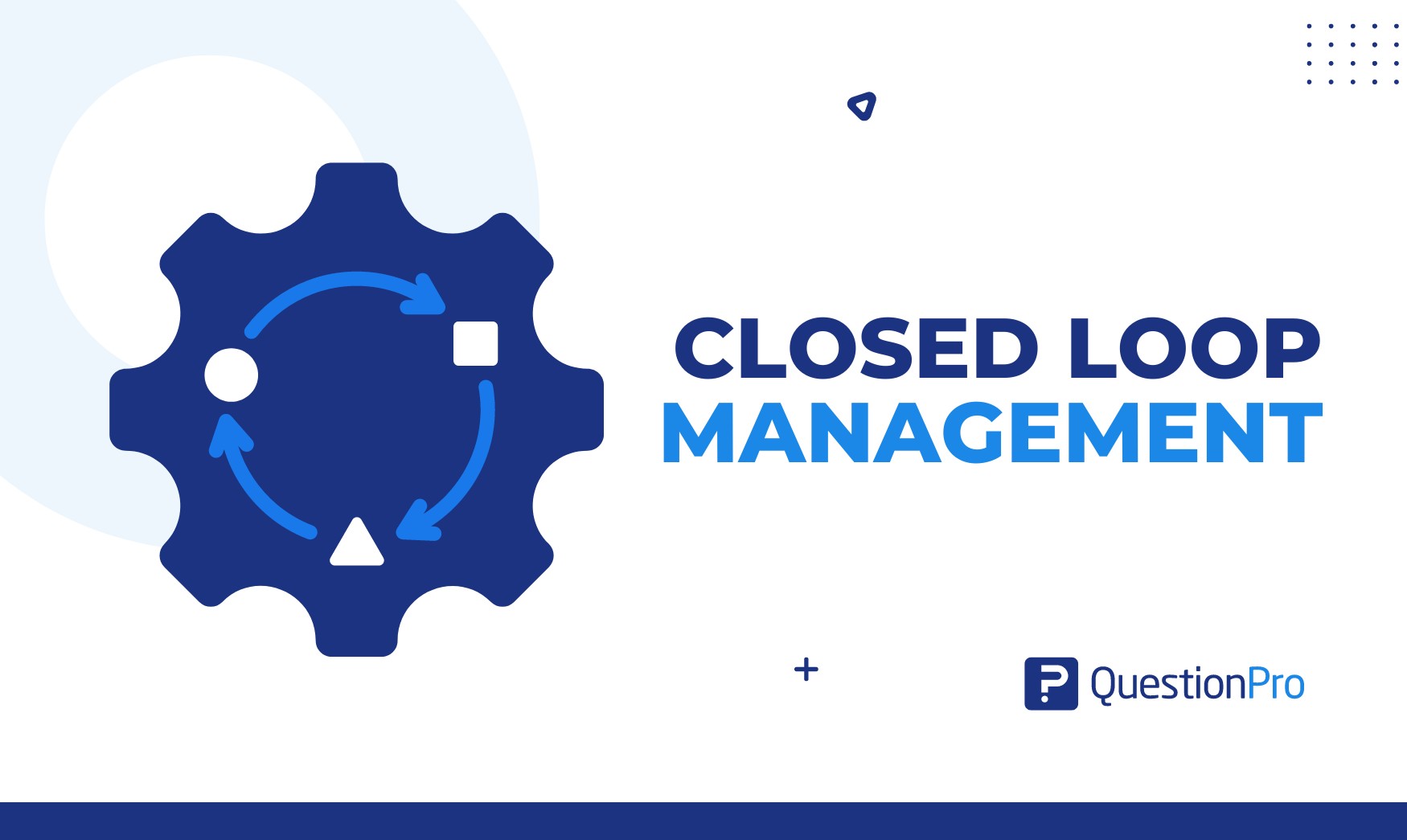
Closed-Loop Management: The Key to Customer Centricity
Sep 3, 2024
Other categories
- Academic Research
- Artificial Intelligence
- Assessments
- Brand Awareness
- Case Studies
- Communities
- Consumer Insights
- Customer effort score
- Customer Engagement
- Customer Experience
- Customer Loyalty
- Customer Research
- Customer Satisfaction
- Employee Benefits
- Employee Engagement
- Employee Retention
- Friday Five
- General Data Protection Regulation
- Insights Hub
- Life@QuestionPro
- Market Research
- Mobile diaries
- Mobile Surveys
- New Features
- Online Communities
- Question Types
- Questionnaire
- QuestionPro Products
- Release Notes
- Research Tools and Apps
- Revenue at Risk
- Survey Templates
- Training Tips
- Tuesday CX Thoughts (TCXT)
- Uncategorized
- What’s Coming Up
- Workforce Intelligence
Have a language expert improve your writing
Run a free plagiarism check in 10 minutes, generate accurate citations for free.
- Knowledge Base
Methodology
- What Is a Research Design | Types, Guide & Examples
What Is a Research Design | Types, Guide & Examples
Published on June 7, 2021 by Shona McCombes . Revised on September 5, 2024 by Pritha Bhandari.
A research design is a strategy for answering your research question using empirical data. Creating a research design means making decisions about:
- Your overall research objectives and approach
- Whether you’ll rely on primary research or secondary research
- Your sampling methods or criteria for selecting subjects
- Your data collection methods
- The procedures you’ll follow to collect data
- Your data analysis methods
A well-planned research design helps ensure that your methods match your research objectives and that you use the right kind of analysis for your data.
You might have to write up a research design as a standalone assignment, or it might be part of a larger research proposal or other project. In either case, you should carefully consider which methods are most appropriate and feasible for answering your question.
Table of contents
Step 1: consider your aims and approach, step 2: choose a type of research design, step 3: identify your population and sampling method, step 4: choose your data collection methods, step 5: plan your data collection procedures, step 6: decide on your data analysis strategies, other interesting articles, frequently asked questions about research design.
- Introduction
Before you can start designing your research, you should already have a clear idea of the research question you want to investigate.
There are many different ways you could go about answering this question. Your research design choices should be driven by your aims and priorities—start by thinking carefully about what you want to achieve.
The first choice you need to make is whether you’ll take a qualitative or quantitative approach.
Qualitative approach | Quantitative approach |
---|---|
and describe frequencies, averages, and correlations about relationships between variables |
Qualitative research designs tend to be more flexible and inductive , allowing you to adjust your approach based on what you find throughout the research process.
Quantitative research designs tend to be more fixed and deductive , with variables and hypotheses clearly defined in advance of data collection.
It’s also possible to use a mixed-methods design that integrates aspects of both approaches. By combining qualitative and quantitative insights, you can gain a more complete picture of the problem you’re studying and strengthen the credibility of your conclusions.
Practical and ethical considerations when designing research
As well as scientific considerations, you need to think practically when designing your research. If your research involves people or animals, you also need to consider research ethics .
- How much time do you have to collect data and write up the research?
- Will you be able to gain access to the data you need (e.g., by travelling to a specific location or contacting specific people)?
- Do you have the necessary research skills (e.g., statistical analysis or interview techniques)?
- Will you need ethical approval ?
At each stage of the research design process, make sure that your choices are practically feasible.
Here's why students love Scribbr's proofreading services
Discover proofreading & editing
Within both qualitative and quantitative approaches, there are several types of research design to choose from. Each type provides a framework for the overall shape of your research.
Types of quantitative research designs
Quantitative designs can be split into four main types.
- Experimental and quasi-experimental designs allow you to test cause-and-effect relationships
- Descriptive and correlational designs allow you to measure variables and describe relationships between them.
Type of design | Purpose and characteristics |
---|---|
Experimental | relationships effect on a |
Quasi-experimental | ) |
Correlational | |
Descriptive |
With descriptive and correlational designs, you can get a clear picture of characteristics, trends and relationships as they exist in the real world. However, you can’t draw conclusions about cause and effect (because correlation doesn’t imply causation ).
Experiments are the strongest way to test cause-and-effect relationships without the risk of other variables influencing the results. However, their controlled conditions may not always reflect how things work in the real world. They’re often also more difficult and expensive to implement.
Types of qualitative research designs
Qualitative designs are less strictly defined. This approach is about gaining a rich, detailed understanding of a specific context or phenomenon, and you can often be more creative and flexible in designing your research.
The table below shows some common types of qualitative design. They often have similar approaches in terms of data collection, but focus on different aspects when analyzing the data.
Type of design | Purpose and characteristics |
---|---|
Grounded theory | |
Phenomenology |
Your research design should clearly define who or what your research will focus on, and how you’ll go about choosing your participants or subjects.
In research, a population is the entire group that you want to draw conclusions about, while a sample is the smaller group of individuals you’ll actually collect data from.
Defining the population
A population can be made up of anything you want to study—plants, animals, organizations, texts, countries, etc. In the social sciences, it most often refers to a group of people.
For example, will you focus on people from a specific demographic, region or background? Are you interested in people with a certain job or medical condition, or users of a particular product?
The more precisely you define your population, the easier it will be to gather a representative sample.
- Sampling methods
Even with a narrowly defined population, it’s rarely possible to collect data from every individual. Instead, you’ll collect data from a sample.
To select a sample, there are two main approaches: probability sampling and non-probability sampling . The sampling method you use affects how confidently you can generalize your results to the population as a whole.
Probability sampling | Non-probability sampling |
---|---|
Probability sampling is the most statistically valid option, but it’s often difficult to achieve unless you’re dealing with a very small and accessible population.
For practical reasons, many studies use non-probability sampling, but it’s important to be aware of the limitations and carefully consider potential biases. You should always make an effort to gather a sample that’s as representative as possible of the population.
Case selection in qualitative research
In some types of qualitative designs, sampling may not be relevant.
For example, in an ethnography or a case study , your aim is to deeply understand a specific context, not to generalize to a population. Instead of sampling, you may simply aim to collect as much data as possible about the context you are studying.
In these types of design, you still have to carefully consider your choice of case or community. You should have a clear rationale for why this particular case is suitable for answering your research question .
For example, you might choose a case study that reveals an unusual or neglected aspect of your research problem, or you might choose several very similar or very different cases in order to compare them.
Data collection methods are ways of directly measuring variables and gathering information. They allow you to gain first-hand knowledge and original insights into your research problem.
You can choose just one data collection method, or use several methods in the same study.
Survey methods
Surveys allow you to collect data about opinions, behaviors, experiences, and characteristics by asking people directly. There are two main survey methods to choose from: questionnaires and interviews .
Questionnaires | Interviews |
---|---|
) |
Observation methods
Observational studies allow you to collect data unobtrusively, observing characteristics, behaviors or social interactions without relying on self-reporting.
Observations may be conducted in real time, taking notes as you observe, or you might make audiovisual recordings for later analysis. They can be qualitative or quantitative.
Quantitative observation | |
---|---|
Other methods of data collection
There are many other ways you might collect data depending on your field and topic.
Field | Examples of data collection methods |
---|---|
Media & communication | Collecting a sample of texts (e.g., speeches, articles, or social media posts) for data on cultural norms and narratives |
Psychology | Using technologies like neuroimaging, eye-tracking, or computer-based tasks to collect data on things like attention, emotional response, or reaction time |
Education | Using tests or assignments to collect data on knowledge and skills |
Physical sciences | Using scientific instruments to collect data on things like weight, blood pressure, or chemical composition |
If you’re not sure which methods will work best for your research design, try reading some papers in your field to see what kinds of data collection methods they used.
Secondary data
If you don’t have the time or resources to collect data from the population you’re interested in, you can also choose to use secondary data that other researchers already collected—for example, datasets from government surveys or previous studies on your topic.
With this raw data, you can do your own analysis to answer new research questions that weren’t addressed by the original study.
Using secondary data can expand the scope of your research, as you may be able to access much larger and more varied samples than you could collect yourself.
However, it also means you don’t have any control over which variables to measure or how to measure them, so the conclusions you can draw may be limited.
As well as deciding on your methods, you need to plan exactly how you’ll use these methods to collect data that’s consistent, accurate, and unbiased.
Planning systematic procedures is especially important in quantitative research, where you need to precisely define your variables and ensure your measurements are high in reliability and validity.
Operationalization
Some variables, like height or age, are easily measured. But often you’ll be dealing with more abstract concepts, like satisfaction, anxiety, or competence. Operationalization means turning these fuzzy ideas into measurable indicators.
If you’re using observations , which events or actions will you count?
If you’re using surveys , which questions will you ask and what range of responses will be offered?
You may also choose to use or adapt existing materials designed to measure the concept you’re interested in—for example, questionnaires or inventories whose reliability and validity has already been established.
Reliability and validity
Reliability means your results can be consistently reproduced, while validity means that you’re actually measuring the concept you’re interested in.
Reliability | Validity |
---|---|
) ) |
For valid and reliable results, your measurement materials should be thoroughly researched and carefully designed. Plan your procedures to make sure you carry out the same steps in the same way for each participant.
If you’re developing a new questionnaire or other instrument to measure a specific concept, running a pilot study allows you to check its validity and reliability in advance.
Sampling procedures
As well as choosing an appropriate sampling method , you need a concrete plan for how you’ll actually contact and recruit your selected sample.
That means making decisions about things like:
- How many participants do you need for an adequate sample size?
- What inclusion and exclusion criteria will you use to identify eligible participants?
- How will you contact your sample—by mail, online, by phone, or in person?
If you’re using a probability sampling method , it’s important that everyone who is randomly selected actually participates in the study. How will you ensure a high response rate?
If you’re using a non-probability method , how will you avoid research bias and ensure a representative sample?
Data management
It’s also important to create a data management plan for organizing and storing your data.
Will you need to transcribe interviews or perform data entry for observations? You should anonymize and safeguard any sensitive data, and make sure it’s backed up regularly.
Keeping your data well-organized will save time when it comes to analyzing it. It can also help other researchers validate and add to your findings (high replicability ).
On its own, raw data can’t answer your research question. The last step of designing your research is planning how you’ll analyze the data.
Quantitative data analysis
In quantitative research, you’ll most likely use some form of statistical analysis . With statistics, you can summarize your sample data, make estimates, and test hypotheses.
Using descriptive statistics , you can summarize your sample data in terms of:
- The distribution of the data (e.g., the frequency of each score on a test)
- The central tendency of the data (e.g., the mean to describe the average score)
- The variability of the data (e.g., the standard deviation to describe how spread out the scores are)
The specific calculations you can do depend on the level of measurement of your variables.
Using inferential statistics , you can:
- Make estimates about the population based on your sample data.
- Test hypotheses about a relationship between variables.
Regression and correlation tests look for associations between two or more variables, while comparison tests (such as t tests and ANOVAs ) look for differences in the outcomes of different groups.
Your choice of statistical test depends on various aspects of your research design, including the types of variables you’re dealing with and the distribution of your data.
Qualitative data analysis
In qualitative research, your data will usually be very dense with information and ideas. Instead of summing it up in numbers, you’ll need to comb through the data in detail, interpret its meanings, identify patterns, and extract the parts that are most relevant to your research question.
Two of the most common approaches to doing this are thematic analysis and discourse analysis .
Approach | Characteristics |
---|---|
Thematic analysis | |
Discourse analysis |
There are many other ways of analyzing qualitative data depending on the aims of your research. To get a sense of potential approaches, try reading some qualitative research papers in your field.
If you want to know more about the research process , methodology , research bias , or statistics , make sure to check out some of our other articles with explanations and examples.
- Simple random sampling
- Stratified sampling
- Cluster sampling
- Likert scales
- Reproducibility
Statistics
- Null hypothesis
- Statistical power
- Probability distribution
- Effect size
- Poisson distribution
Research bias
- Optimism bias
- Cognitive bias
- Implicit bias
- Hawthorne effect
- Anchoring bias
- Explicit bias
A research design is a strategy for answering your research question . It defines your overall approach and determines how you will collect and analyze data.
A well-planned research design helps ensure that your methods match your research aims, that you collect high-quality data, and that you use the right kind of analysis to answer your questions, utilizing credible sources . This allows you to draw valid , trustworthy conclusions.
Quantitative research designs can be divided into two main categories:
- Correlational and descriptive designs are used to investigate characteristics, averages, trends, and associations between variables.
- Experimental and quasi-experimental designs are used to test causal relationships .
Qualitative research designs tend to be more flexible. Common types of qualitative design include case study , ethnography , and grounded theory designs.
The priorities of a research design can vary depending on the field, but you usually have to specify:
- Your research questions and/or hypotheses
- Your overall approach (e.g., qualitative or quantitative )
- The type of design you’re using (e.g., a survey , experiment , or case study )
- Your data collection methods (e.g., questionnaires , observations)
- Your data collection procedures (e.g., operationalization , timing and data management)
- Your data analysis methods (e.g., statistical tests or thematic analysis )
A sample is a subset of individuals from a larger population . Sampling means selecting the group that you will actually collect data from in your research. For example, if you are researching the opinions of students in your university, you could survey a sample of 100 students.
In statistics, sampling allows you to test a hypothesis about the characteristics of a population.
Operationalization means turning abstract conceptual ideas into measurable observations.
For example, the concept of social anxiety isn’t directly observable, but it can be operationally defined in terms of self-rating scores, behavioral avoidance of crowded places, or physical anxiety symptoms in social situations.
Before collecting data , it’s important to consider how you will operationalize the variables that you want to measure.
A research project is an academic, scientific, or professional undertaking to answer a research question . Research projects can take many forms, such as qualitative or quantitative , descriptive , longitudinal , experimental , or correlational . What kind of research approach you choose will depend on your topic.
Cite this Scribbr article
If you want to cite this source, you can copy and paste the citation or click the “Cite this Scribbr article” button to automatically add the citation to our free Citation Generator.
McCombes, S. (2024, September 05). What Is a Research Design | Types, Guide & Examples. Scribbr. Retrieved September 9, 2024, from https://www.scribbr.com/methodology/research-design/
Is this article helpful?
Shona McCombes
Other students also liked, guide to experimental design | overview, steps, & examples, how to write a research proposal | examples & templates, ethical considerations in research | types & examples, get unlimited documents corrected.
✔ Free APA citation check included ✔ Unlimited document corrections ✔ Specialized in correcting academic texts
Have a language expert improve your writing
Run a free plagiarism check in 10 minutes, automatically generate references for free.
- Knowledge Base
- Methodology
- Doing Survey Research | A Step-by-Step Guide & Examples
Doing Survey Research | A Step-by-Step Guide & Examples
Published on 6 May 2022 by Shona McCombes . Revised on 10 October 2022.
Survey research means collecting information about a group of people by asking them questions and analysing the results. To conduct an effective survey, follow these six steps:
- Determine who will participate in the survey
- Decide the type of survey (mail, online, or in-person)
- Design the survey questions and layout
- Distribute the survey
- Analyse the responses
- Write up the results
Surveys are a flexible method of data collection that can be used in many different types of research .
Table of contents
What are surveys used for, step 1: define the population and sample, step 2: decide on the type of survey, step 3: design the survey questions, step 4: distribute the survey and collect responses, step 5: analyse the survey results, step 6: write up the survey results, frequently asked questions about surveys.
Surveys are used as a method of gathering data in many different fields. They are a good choice when you want to find out about the characteristics, preferences, opinions, or beliefs of a group of people.
Common uses of survey research include:
- Social research: Investigating the experiences and characteristics of different social groups
- Market research: Finding out what customers think about products, services, and companies
- Health research: Collecting data from patients about symptoms and treatments
- Politics: Measuring public opinion about parties and policies
- Psychology: Researching personality traits, preferences, and behaviours
Surveys can be used in both cross-sectional studies , where you collect data just once, and longitudinal studies , where you survey the same sample several times over an extended period.
Prevent plagiarism, run a free check.
Before you start conducting survey research, you should already have a clear research question that defines what you want to find out. Based on this question, you need to determine exactly who you will target to participate in the survey.
Populations
The target population is the specific group of people that you want to find out about. This group can be very broad or relatively narrow. For example:
- The population of Brazil
- University students in the UK
- Second-generation immigrants in the Netherlands
- Customers of a specific company aged 18 to 24
- British transgender women over the age of 50
Your survey should aim to produce results that can be generalised to the whole population. That means you need to carefully define exactly who you want to draw conclusions about.
It’s rarely possible to survey the entire population of your research – it would be very difficult to get a response from every person in Brazil or every university student in the UK. Instead, you will usually survey a sample from the population.
The sample size depends on how big the population is. You can use an online sample calculator to work out how many responses you need.
There are many sampling methods that allow you to generalise to broad populations. In general, though, the sample should aim to be representative of the population as a whole. The larger and more representative your sample, the more valid your conclusions.
There are two main types of survey:
- A questionnaire , where a list of questions is distributed by post, online, or in person, and respondents fill it out themselves
- An interview , where the researcher asks a set of questions by phone or in person and records the responses
Which type you choose depends on the sample size and location, as well as the focus of the research.
Questionnaires
Sending out a paper survey by post is a common method of gathering demographic information (for example, in a government census of the population).
- You can easily access a large sample.
- You have some control over who is included in the sample (e.g., residents of a specific region).
- The response rate is often low.
Online surveys are a popular choice for students doing dissertation research , due to the low cost and flexibility of this method. There are many online tools available for constructing surveys, such as SurveyMonkey and Google Forms .
- You can quickly access a large sample without constraints on time or location.
- The data is easy to process and analyse.
- The anonymity and accessibility of online surveys mean you have less control over who responds.
If your research focuses on a specific location, you can distribute a written questionnaire to be completed by respondents on the spot. For example, you could approach the customers of a shopping centre or ask all students to complete a questionnaire at the end of a class.
- You can screen respondents to make sure only people in the target population are included in the sample.
- You can collect time- and location-specific data (e.g., the opinions of a shop’s weekday customers).
- The sample size will be smaller, so this method is less suitable for collecting data on broad populations.
Oral interviews are a useful method for smaller sample sizes. They allow you to gather more in-depth information on people’s opinions and preferences. You can conduct interviews by phone or in person.
- You have personal contact with respondents, so you know exactly who will be included in the sample in advance.
- You can clarify questions and ask for follow-up information when necessary.
- The lack of anonymity may cause respondents to answer less honestly, and there is more risk of researcher bias.
Like questionnaires, interviews can be used to collect quantitative data : the researcher records each response as a category or rating and statistically analyses the results. But they are more commonly used to collect qualitative data : the interviewees’ full responses are transcribed and analysed individually to gain a richer understanding of their opinions and feelings.
Next, you need to decide which questions you will ask and how you will ask them. It’s important to consider:
- The type of questions
- The content of the questions
- The phrasing of the questions
- The ordering and layout of the survey
Open-ended vs closed-ended questions
There are two main forms of survey questions: open-ended and closed-ended. Many surveys use a combination of both.
Closed-ended questions give the respondent a predetermined set of answers to choose from. A closed-ended question can include:
- A binary answer (e.g., yes/no or agree/disagree )
- A scale (e.g., a Likert scale with five points ranging from strongly agree to strongly disagree )
- A list of options with a single answer possible (e.g., age categories)
- A list of options with multiple answers possible (e.g., leisure interests)
Closed-ended questions are best for quantitative research . They provide you with numerical data that can be statistically analysed to find patterns, trends, and correlations .
Open-ended questions are best for qualitative research. This type of question has no predetermined answers to choose from. Instead, the respondent answers in their own words.
Open questions are most common in interviews, but you can also use them in questionnaires. They are often useful as follow-up questions to ask for more detailed explanations of responses to the closed questions.
The content of the survey questions
To ensure the validity and reliability of your results, you need to carefully consider each question in the survey. All questions should be narrowly focused with enough context for the respondent to answer accurately. Avoid questions that are not directly relevant to the survey’s purpose.
When constructing closed-ended questions, ensure that the options cover all possibilities. If you include a list of options that isn’t exhaustive, you can add an ‘other’ field.
Phrasing the survey questions
In terms of language, the survey questions should be as clear and precise as possible. Tailor the questions to your target population, keeping in mind their level of knowledge of the topic.
Use language that respondents will easily understand, and avoid words with vague or ambiguous meanings. Make sure your questions are phrased neutrally, with no bias towards one answer or another.
Ordering the survey questions
The questions should be arranged in a logical order. Start with easy, non-sensitive, closed-ended questions that will encourage the respondent to continue.
If the survey covers several different topics or themes, group together related questions. You can divide a questionnaire into sections to help respondents understand what is being asked in each part.
If a question refers back to or depends on the answer to a previous question, they should be placed directly next to one another.
Before you start, create a clear plan for where, when, how, and with whom you will conduct the survey. Determine in advance how many responses you require and how you will gain access to the sample.
When you are satisfied that you have created a strong research design suitable for answering your research questions, you can conduct the survey through your method of choice – by post, online, or in person.
There are many methods of analysing the results of your survey. First you have to process the data, usually with the help of a computer program to sort all the responses. You should also cleanse the data by removing incomplete or incorrectly completed responses.
If you asked open-ended questions, you will have to code the responses by assigning labels to each response and organising them into categories or themes. You can also use more qualitative methods, such as thematic analysis , which is especially suitable for analysing interviews.
Statistical analysis is usually conducted using programs like SPSS or Stata. The same set of survey data can be subject to many analyses.
Finally, when you have collected and analysed all the necessary data, you will write it up as part of your thesis, dissertation , or research paper .
In the methodology section, you describe exactly how you conducted the survey. You should explain the types of questions you used, the sampling method, when and where the survey took place, and the response rate. You can include the full questionnaire as an appendix and refer to it in the text if relevant.
Then introduce the analysis by describing how you prepared the data and the statistical methods you used to analyse it. In the results section, you summarise the key results from your analysis.
A Likert scale is a rating scale that quantitatively assesses opinions, attitudes, or behaviours. It is made up of four or more questions that measure a single attitude or trait when response scores are combined.
To use a Likert scale in a survey , you present participants with Likert-type questions or statements, and a continuum of items, usually with five or seven possible responses, to capture their degree of agreement.
Individual Likert-type questions are generally considered ordinal data , because the items have clear rank order, but don’t have an even distribution.
Overall Likert scale scores are sometimes treated as interval data. These scores are considered to have directionality and even spacing between them.
The type of data determines what statistical tests you should use to analyse your data.
A questionnaire is a data collection tool or instrument, while a survey is an overarching research method that involves collecting and analysing data from people using questionnaires.
Cite this Scribbr article
If you want to cite this source, you can copy and paste the citation or click the ‘Cite this Scribbr article’ button to automatically add the citation to our free Reference Generator.
McCombes, S. (2022, October 10). Doing Survey Research | A Step-by-Step Guide & Examples. Scribbr. Retrieved 9 September 2024, from https://www.scribbr.co.uk/research-methods/surveys/
Is this article helpful?
Shona McCombes
Other students also liked, qualitative vs quantitative research | examples & methods, construct validity | definition, types, & examples, what is a likert scale | guide & examples.
Doing Quantitative Research with a Survey
- First Online: 24 December 2020
Cite this chapter
- Alistair McBeath 2
3122 Accesses
5 Citations
The use of surveys within counselling and psychotherapy is becoming an increasingly important tool used both by professional bodies canvassing their members’ opinions and by researchers studying treatment efficacy and client outcomes. Surveys are key in driving organisational change within the therapy world and also in giving clients a voice about their experience of treatment and therapy. This chapter offers an introduction to how surveys work and how to construct them, and allows practitioners to be able to assess the relevance and validity of survey-based information, as well as how to design surveys for their own research activity.
This is a preview of subscription content, log in via an institution to check access.
Access this chapter
Subscribe and save.
- Get 10 units per month
- Download Article/Chapter or eBook
- 1 Unit = 1 Article or 1 Chapter
- Cancel anytime
- Available as PDF
- Read on any device
- Instant download
- Own it forever
- Available as EPUB and PDF
- Compact, lightweight edition
- Dispatched in 3 to 5 business days
- Free shipping worldwide - see info
Tax calculation will be finalised at checkout
Purchases are for personal use only
Institutional subscriptions
Similar content being viewed by others
Using Online Surveys Creatively in Counselling and Psychotherapy Research
Survey Methodology
Survey Research Major Methodological Flaws: Caveat Lector
Estimate based on BACP-accredited membership of approximately 11,000 plus UKCP membership of approximately 9000 (Source BACP and UKCP).
BACP. (2017). Press release. Older people are not getting the mental health support they deserve. Retrieved from https://www.bacp.co.uk/news/news-from-bacp/2017/1-october-2017-older-people-are-not-getting-the/7
British Psychoanalytic Council. (2012). Quality psychotherapy services in the NHS. Summary findings from the UK Council for Psychotherapy and British Psychoanalytic Council members’ survey. Retrieved from https://www.bpc.org.uk/sites/psychoanalytic-council.org/files/Summary%20findings%20from%20NHS%20survey.pd
Bräuninger, I. (2014). Dance movement therapy with the elderly: An international Internet-based survey under-taken with practitioners. An International Journal for Theory, Research and Practice, 9 , 138–153. https://doi.org/10.1080/17432979.2014.914977 .
Article Google Scholar
Cook, J. M., Tatyana, B., Elhai, J., Schnurr, P. P., & Coyne, J. C. (2010). What do psychotherapists really do in practice? An Internet study of over 2,000 practitioners. Psychotherapy (Chicago, Ill.), 47 (2), 260–267. https://doi.org/10.1037/a0019788 .
Ivey, G., & Phillips, L. (2016). Psychotherapy clients’ attitudes to personal psychotherapy for psychotherapists. Asia Pacific Journal of Counselling and Psychotherapy, 7 (1-2), 101–117. https://doi.org/10.1080/21507686.2016.1157087 .
Love, M., & Farber, B. A. (2018). Honesty in psychotherapy: Results of an online survey comparing high vs. low self-concealers. Psychotherapy Research, 25 (9), 1–14. https://doi.org/10.1080/10503307.2017.1417652 .
McBeath, A. G. (2019). The motivations of psychotherapists: An in-depth survey. Counselling and Psychotherapy Research, 19 (4), 377–387. https://doi.org/10.1002/capr.12225 .
McBeath, A. G., Bager-Charleson, S., & Abarbanel, A. (2019). Therapists and Academic Writing: ‘Once upon a time psychotherapy practitioners and researchers were the same people. European Journal for Qualitative Research in Psychotherapy, 19 , 103–116.
Google Scholar
Mind. (2010). The protection we deserve: Findings from a service user survey on the regulation of counsellors and psychotherapists. Retrieved from http://www.cpcab.co.uk/Content/Publicdocs/The%20Protection%20We%20Deserve%20-%20survey%20report.pdf
Richardson, J., Sheean, L., & Bambling, M. (2009). Becoming a psychotherapist or counsellor: A survey of psychotherapy and counselling trainers. Psychotherapy in Australia, 16 (1), 70–80.
Smith, J. A., & Osborn, S. (2008). Interpretative phenomenological analysis. In J. A. Smith (Ed.), Qualitative psychology (pp. 53–80). London: Sage.
Download references
Author information
Authors and affiliations.
Metanoia Institute, London, UK
Alistair McBeath
You can also search for this author in PubMed Google Scholar
Corresponding author
Correspondence to Alistair McBeath .
Editor information
Editors and affiliations.
Sofie Bager-Charleson & Alistair McBeath &
Rights and permissions
Reprints and permissions
Copyright information
© 2020 The Author(s)
About this chapter
McBeath, A. (2020). Doing Quantitative Research with a Survey. In: Bager-Charleson, S., McBeath, A. (eds) Enjoying Research in Counselling and Psychotherapy. Palgrave Macmillan, Cham. https://doi.org/10.1007/978-3-030-55127-8_10
Download citation
DOI : https://doi.org/10.1007/978-3-030-55127-8_10
Published : 24 December 2020
Publisher Name : Palgrave Macmillan, Cham
Print ISBN : 978-3-030-55126-1
Online ISBN : 978-3-030-55127-8
eBook Packages : Behavioral Science and Psychology Behavioral Science and Psychology (R0)
Share this chapter
Anyone you share the following link with will be able to read this content:
Sorry, a shareable link is not currently available for this article.
Provided by the Springer Nature SharedIt content-sharing initiative
- Publish with us
Policies and ethics
- Find a journal
- Track your research
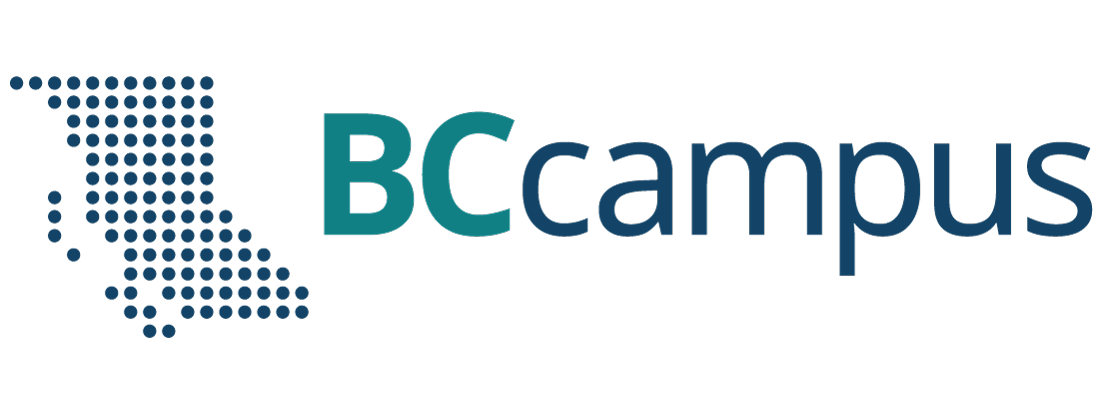
Want to create or adapt books like this? Learn more about how Pressbooks supports open publishing practices.
Chapter 9: Survey Research
Overview of Survey Research
Learning Objectives
- Define what survey research is, including its two important characteristics.
- Describe several different ways that survey research can be used and give some examples.
What Is Survey Research?
Survey research is a quantitative and qualitative method with two important characteristics. First, the variables of interest are measured using self-reports. In essence, survey researchers ask their participants (who are often called respondents in survey research) to report directly on their own thoughts, feelings, and behaviours. Second, considerable attention is paid to the issue of sampling. In particular, survey researchers have a strong preference for large random samples because they provide the most accurate estimates of what is true in the population. In fact, survey research may be the only approach in psychology in which random sampling is routinely used. Beyond these two characteristics, almost anything goes in survey research. Surveys can be long or short. They can be conducted in person, by telephone, through the mail, or over the Internet. They can be about voting intentions, consumer preferences, social attitudes, health, or anything else that it is possible to ask people about and receive meaningful answers. Although survey data are often analyzed using statistics, there are many questions that lend themselves to more qualitative analysis.
Most survey research is nonexperimental. It is used to describe single variables (e.g., the percentage of voters who prefer one presidential candidate or another, the prevalence of schizophrenia in the general population) and also to assess statistical relationships between variables (e.g., the relationship between income and health). But surveys can also be experimental. The study by Lerner and her colleagues is a good example. Their use of self-report measures and a large national sample identifies their work as survey research. But their manipulation of an independent variable (anger vs. fear) to assess its effect on a dependent variable (risk judgments) also identifies their work as experimental.
History and Uses of Survey Research
Survey research may have its roots in English and American “social surveys” conducted around the turn of the 20th century by researchers and reformers who wanted to document the extent of social problems such as poverty (Converse, 1987) [1] . By the 1930s, the US government was conducting surveys to document economic and social conditions in the country. The need to draw conclusions about the entire population helped spur advances in sampling procedures. At about the same time, several researchers who had already made a name for themselves in market research, studying consumer preferences for American businesses, turned their attention to election polling. A watershed event was the presidential election of 1936 between Alf Landon and Franklin Roosevelt. A magazine called Literary Digest conducted a survey by sending ballots (which were also subscription requests) to millions of Americans. Based on this “straw poll,” the editors predicted that Landon would win in a landslide. At the same time, the new pollsters were using scientific methods with much smaller samples to predict just the opposite—that Roosevelt would win in a landslide. In fact, one of them, George Gallup, publicly criticized the methods of Literary Digest before the election and all but guaranteed that his prediction would be correct. And of course it was. (We will consider the reasons that Gallup was right later in this chapter.) Interest in surveying around election times has led to several long-term projects, notably the Canadian Election Studies which has measured opinions of Canadian voters around federal elections since 1965. Anyone can access the data and read about the results of the experiments in these studies.
From market research and election polling, survey research made its way into several academic fields, including political science, sociology, and public health—where it continues to be one of the primary approaches to collecting new data. Beginning in the 1930s, psychologists made important advances in questionnaire design, including techniques that are still used today, such as the Likert scale. (See “What Is a Likert Scale?” in Section 9.2 “Constructing Survey Questionnaires” .) Survey research has a strong historical association with the social psychological study of attitudes, stereotypes, and prejudice. Early attitude researchers were also among the first psychologists to seek larger and more diverse samples than the convenience samples of university students that were routinely used in psychology (and still are).
Survey research continues to be important in psychology today. For example, survey data have been instrumental in estimating the prevalence of various mental disorders and identifying statistical relationships among those disorders and with various other factors. The National Comorbidity Survey is a large-scale mental health survey conducted in the United States . In just one part of this survey, nearly 10,000 adults were given a structured mental health interview in their homes in 2002 and 2003. Table 9.1 presents results on the lifetime prevalence of some anxiety, mood, and substance use disorders. (Lifetime prevalence is the percentage of the population that develops the problem sometime in their lifetime.) Obviously, this kind of information can be of great use both to basic researchers seeking to understand the causes and correlates of mental disorders as well as to clinicians and policymakers who need to understand exactly how common these disorders are.
Disorder | Average | Female | Male |
---|---|---|---|
Generalized anxiety disorder | 5.7 | 7.1 | 4.2 |
Obsessive-compulsive disorder | 2.3 | 3.1 | 1.6 |
Major depressive disorder | 16.9 | 20.2 | 13.2 |
Bipolar disorder | 4.4 | 4.5 | 4.3 |
Alcohol abuse | 13.2 | 7.5 | 19.6 |
Drug abuse | 8.0 | 4.8 | 11.6 |
And as the opening example makes clear, survey research can even be used to conduct experiments to test specific hypotheses about causal relationships between variables. Such studies, when conducted on large and diverse samples, can be a useful supplement to laboratory studies conducted on university students. Although this approach is not a typical use of survey research, it certainly illustrates the flexibility of this method.
Key Takeaways
- Survey research is a quantitative approach that features the use of self-report measures on carefully selected samples. It is a flexible approach that can be used to study a wide variety of basic and applied research questions.
- Survey research has its roots in applied social research, market research, and election polling. It has since become an important approach in many academic disciplines, including political science, sociology, public health, and, of course, psychology.
Discussion: Think of a question that each of the following professionals might try to answer using survey research.
- a social psychologist
- an educational researcher
- a market researcher who works for a supermarket chain
- the mayor of a large city
- the head of a university police force
- Converse, J. M. (1987). Survey research in the United States: Roots and emergence, 1890–1960 . Berkeley, CA: University of California Press. ↵
- The lifetime prevalence of a disorder is the percentage of people in the population that develop that disorder at any time in their lives. ↵
A quantitative approach in which variables are measured using self-reports from a sample of the population.
Participants of a survey.
Research Methods in Psychology - 2nd Canadian Edition Copyright © 2015 by Paul C. Price, Rajiv Jhangiani, & I-Chant A. Chiang is licensed under a Creative Commons Attribution-NonCommercial-ShareAlike 4.0 International License , except where otherwise noted.
Share This Book

What Are Quantitative Survey Questions? Types and Examples
Learn all about quantitative research surveys, including types of quantitative survey questions, question formats, and quantitative question examples.
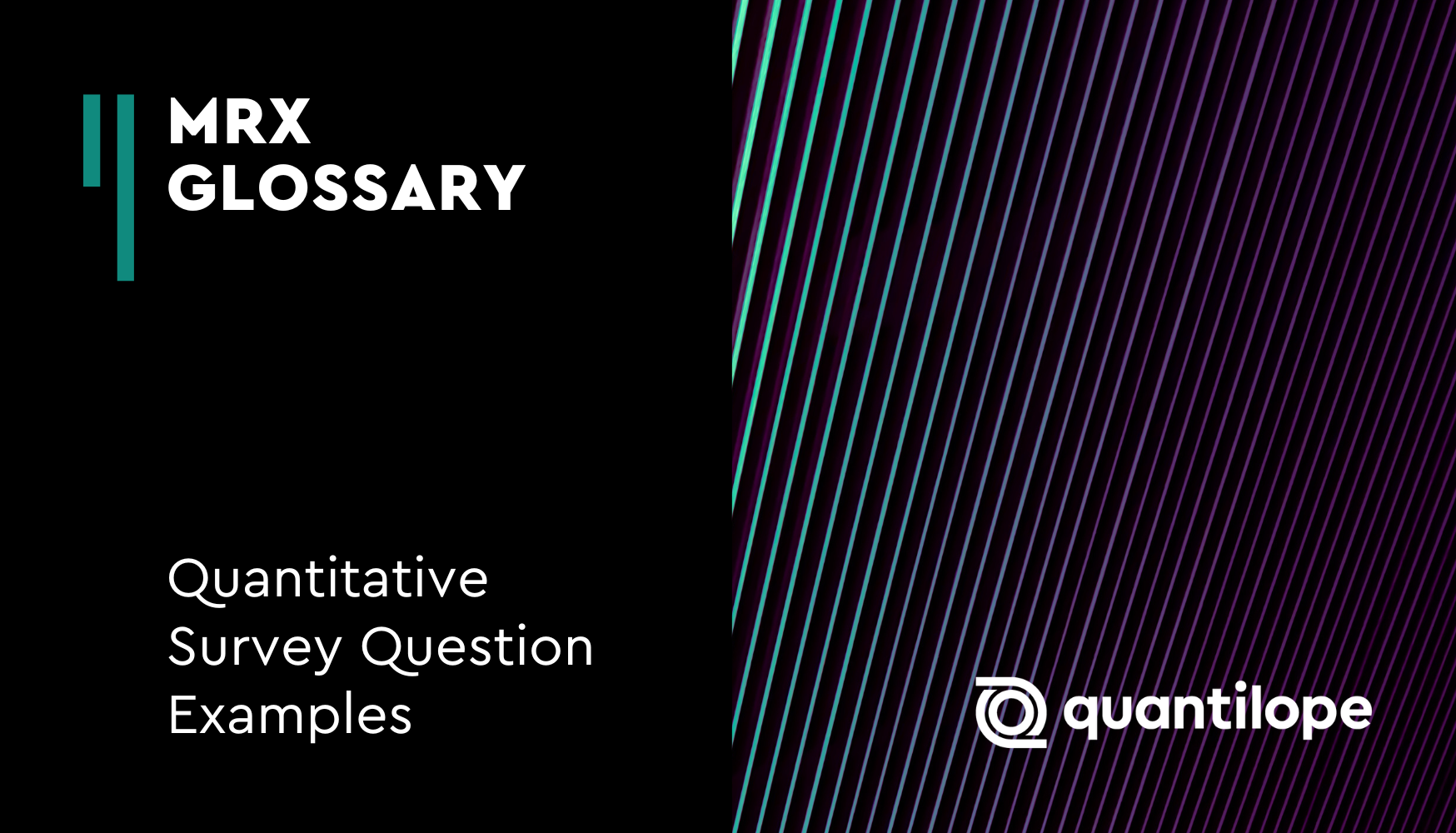
Jan 29, 2024
quantilope is the Consumer Intelligence Platform for all end-to-end research needs

Table of contents:
- Types of quantitative survey questions - with examples
- Quantitative question formats
- How to write quantitative survey questions
- Examples of quantitative survey questions
Leveraging quantilope for your quantitative survey
In a quantitative research study brands will gather numeric data for most of their questions through formats like numerical scale questions or ranking questions. However, brands can also include some non-quantitative questions throughout their quantitative study - like open-ended questions, where respondents will type in their own feedback to a question prompt. Even so, open-ended answers can be numerically coded to sift through feedback easily (e.g. anyone who writes in 'Pepsi' in a soda study would be assigned the number '1', to look at Pepsi feedback as a whole). One of the biggest benefits of using a quantitative research approach is that insights around a research topic can undergo statistical analysis; the same can’t be said for qualitative data like focus group feedback or interviews. Another major difference between quantitative and qualitative research methods is that quantitative surveys require respondents to choose from a limited number of choices in a close-ended question - generating clear, actionable takeaways. However, these distinct quantitative takeaways often pair well with freeform qualitative responses - making quant and qual a great team to use together. The rest of this article focuses on quantitative research, taking a closer look at quantitative survey question types and question formats/layouts.
Back to table of contents
Types of dropdown#toggle" data-dropdown-placement-param="top" data-term-id="281139745">quantitative survey questions - with examples
Quantitative questions come in many forms, each with different benefits depending on dropdown#toggle" data-dropdown-placement-param="top" data-term-id="281139784">your dropdown#toggle" data-dropdown-placement-param="top" data-term-id="281139740">market research objectives. Below we’ll explore some of these dropdown#toggle" data-dropdown-placement-param="top" data-term-id="281139745">quantitative dropdown#toggle" data-dropdown-placement-param="top" data-term-id="281139785">survey question dropdown#toggle" data-dropdown-menu-id-param="menu_term_281139785" data-dropdown-placement-param="top" data-term-id="281139785"> types, which are commonly used together in a single survey to keep things interesting for dropdown#toggle" data-dropdown-placement-param="top" data-term-id="281139737">respondents . The style of questioning used during dropdown#toggle" data-dropdown-placement-param="top" data-term-id="281139739">quantitative dropdown#toggle" data-dropdown-placement-param="top" data-term-id="281139750">data dropdown#toggle" data-dropdown-menu-id-param="menu_term_281139750" data-dropdown-placement-param="top" data-term-id="281139750"> collection is important, as a good mix of the right types of questions will deliver rich data, limit dropdown#toggle" data-dropdown-placement-param="top" data-term-id="281139737">respondent fatigue, and optimize the dropdown#toggle" data-dropdown-placement-param="top" data-term-id="281139757">response rate . dropdown#toggle" data-dropdown-placement-param="top" data-term-id="281139742">Questionnaires should be enjoyable - and varying the dropdown#toggle" data-dropdown-placement-param="top" data-term-id="281139755">types of dropdown#toggle" data-dropdown-menu-id-param="menu_term_281139755" data-dropdown-placement-param="top" data-term-id="281139755">quantitative research dropdown#toggle" data-dropdown-placement-param="top" data-term-id="281139755"> questions used throughout your survey will help achieve that.
Descriptive survey questions
dropdown#toggle" data-dropdown-placement-param="top" data-term-id="281139763">Descriptive research questions (also known as usage and attitude, or, U&A questions) seek a general indication or prediction about how a dropdown#toggle" data-dropdown-placement-param="top" data-term-id="281139773">group of people behaves or will behave, how that group is characterized, or how a group thinks.
For example, a business might want to know what portion of adult men shave, and how often they do so. To find this out, they will survey men (the dropdown#toggle" data-dropdown-placement-param="top" data-term-id="281139743">target audience ) and ask descriptive questions about their frequency of shaving (e.g. daily, a few times a week, once per week, and so on.) Each of these frequencies get assigned a numerical ‘code’ so that it’s simple to chart and analyze the data later on; daily might be assigned ‘5’, a few times a week might be assigned ‘4’, and so on. That way, brands can create charts using the ‘top two’ and ‘bottom two’ values in a descriptive question to view these metrics side by side.
Another business might want to know how important local transit issues are to residents, so dropdown#toggle" data-dropdown-placement-param="top" data-term-id="281139745">quantitative survey questions will allow dropdown#toggle" data-dropdown-placement-param="top" data-term-id="281139737">respondents to indicate the degrees of opinion attached to various transit issues. Perhaps the transit business running this survey would use a sliding numeric scale to see how important a particular issue is.
Comparative survey questions
dropdown#toggle" data-dropdown-placement-param="top" data-term-id="281139782">Comparative research questions are concerned with comparing individuals or groups of people based on one or more variables. These questions might be posed when a business wants to find out which segment of its dropdown#toggle" data-dropdown-placement-param="top" data-term-id="281139743">target audience might be more profitable, or which types of products might appeal to different sets of consumers.
For example, a business might want to know how the popularity of its chocolate bars is spread out across its entire customer base (i.e. do women prefer a certain flavor? Are children drawn to candy bars by certain packaging attributes? etc.). Questions in this case will be designed to profile and ‘compare’ segments of the market.
Other businesses might be looking to compare coffee consumption among older and younger consumers (i.e. dropdown#toggle" data-dropdown-placement-param="top" data-term-id="281139741">demographic segments), the difference in smartphone usage between younger men and women, or how women from different regions differ in their approach to skincare.
Relationship-based survey questions
As the name suggests, relationship-based survey questions are concerned with the relationship between two or more variables within one or more dropdown#toggle" data-dropdown-placement-param="top" data-term-id="281139741">demographic groups. This might be a dropdown#toggle" data-dropdown-placement-param="top" data-term-id="281139759">causal link between one thing and the other - for example, the consumption of caffeine and dropdown#toggle" data-dropdown-placement-param="top" data-term-id="281139737">respondents ’ reported energy levels throughout the day. In this case, a coffee or energy drink brand might be interested in how energy levels differ between those who drink their caffeinated line of beverages and those who drink decaf/non-caffeinated beverages.
Alternatively, it might be a case of two or more factors co-existing, without there necessarily being a dropdown#toggle" data-dropdown-placement-param="top" data-term-id="281139759">causal link - for example, a particular type of air freshener being more popular amongst a certain dropdown#toggle" data-dropdown-placement-param="top" data-term-id="281139741">demographic (maybe one that is controlled wirelessly via Bluetooth is more popular among younger homeowners than one that’s plugged into the wall with no controls). Knowing that millennials favor air fresheners which have options for swapping out scents and setting up schedules would be valuable information for new product development.
Advanced method survey questions
Aside from descriptive, comparative, and relationship-based survey questions, brands can opt to include advanced methodologies in their quantitative dropdown#toggle" data-dropdown-placement-param="top" data-term-id="281139742">questionnaire for richer depth. Though advanced methods are more complex in terms of the insights output, quantilope’s Consumer Intelligence Platform automates the setup and analysis of these methods so that researchers of any background or skillset can leverage them with ease.
With quantilope’s pre-programmed suite of 12 advanced methodologies , including MaxDiff , TURF , Implicit , and more, users can drag and drop any of these into a dropdown#toggle" data-dropdown-placement-param="top" data-term-id="281139742">questionnaire and customize for their own dropdown#toggle" data-dropdown-placement-param="top" data-term-id="281139740">market research objectives.
For example, consider a beverage company that’s looking to expand its flavor profiles. This brand would benefit from a MaxDiff which forces dropdown#toggle" data-dropdown-placement-param="top" data-term-id="281139737">respondents to make tradeoff decisions between a set of flavors. A dropdown#toggle" data-dropdown-placement-param="top" data-term-id="281139737">respondent might say that coconut is their most-preferred flavor, and lime their least (when in a consideration set with strawberry), yet later on in the MaxDiff that same dropdown#toggle" data-dropdown-placement-param="top" data-term-id="281139737">respondent may say Strawberry is their most-preferred flavor (over black cherry and kiwi). While this is just one example of an advanced method, instantly you can see how much richer and more actionable these quantitative metrics become compared to a standard usage and attitude question .
Advanced methods can be used alongside descriptive, comparison, or relationship questions to add a new layer of context wherever a business sees fit. Back to table of contents
Quantitative question formats
So we’ve covered the kinds of dropdown#toggle" data-dropdown-placement-param="top" data-term-id="281139736">quantitative research questions you might want to answer using dropdown#toggle" data-dropdown-placement-param="top" data-term-id="281139740">market research , but how do these translate into the actual format of questions that you might include on your dropdown#toggle" data-dropdown-placement-param="top" data-term-id="281139742">questionnaire ?
Thinking ahead to your reporting process during your dropdown#toggle" data-dropdown-placement-param="top" data-term-id="281139742">questionnaire setup is actually quite important, as the available chart types differ among the types of questions asked; some question data is compatible with bar chart displays, others pie charts, others in trended line graphs, etc. Also consider how well the questions you’re asking will translate onto different devices that your dropdown#toggle" data-dropdown-placement-param="top" data-term-id="281139737">respondents might be using to complete the survey (mobile, PC, or tablet).
Single Select questions
Single select questions are the simplest form of quantitative questioning, as dropdown#toggle" data-dropdown-placement-param="top" data-term-id="281139737">respondents are asked to choose just one answer from a list of items, which tend to be ‘either/or’, ‘yes/no’, or ‘true/false’ questions. These questions are useful when you need to get a clear answer without any qualifying nuances.
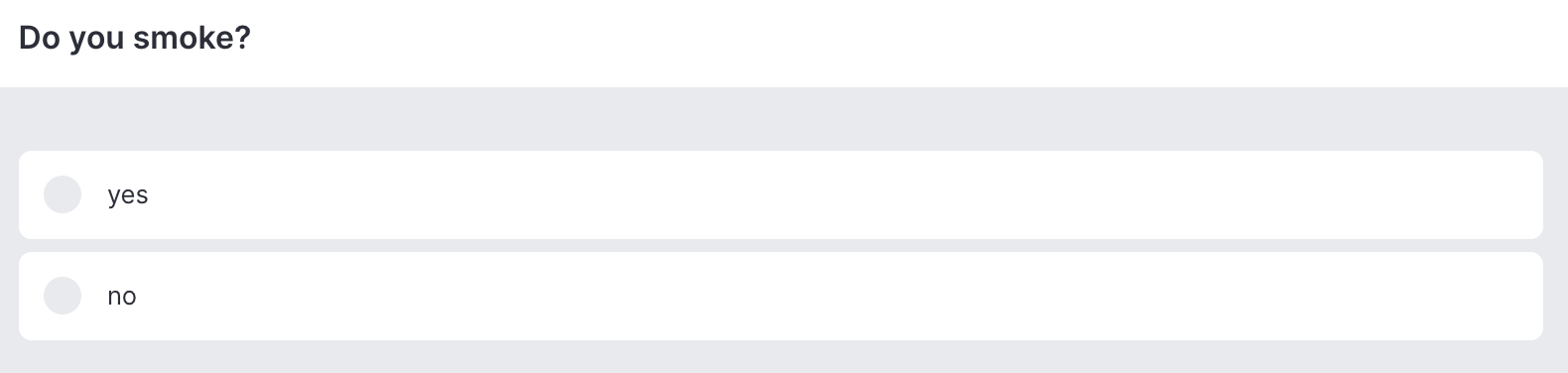
Multi-select questions
Multi-select questions (aka, dropdown#toggle" data-dropdown-placement-param="top" data-term-id="281139767">multiple choice ) offer more flexibility for responses, allowing for a number of responses on a single question. dropdown#toggle" data-dropdown-placement-param="top" data-term-id="281139737">Respondents can be asked to ‘check all that apply’ or a cap can be applied (e.g. ‘select up to 3 choices’).
For example:
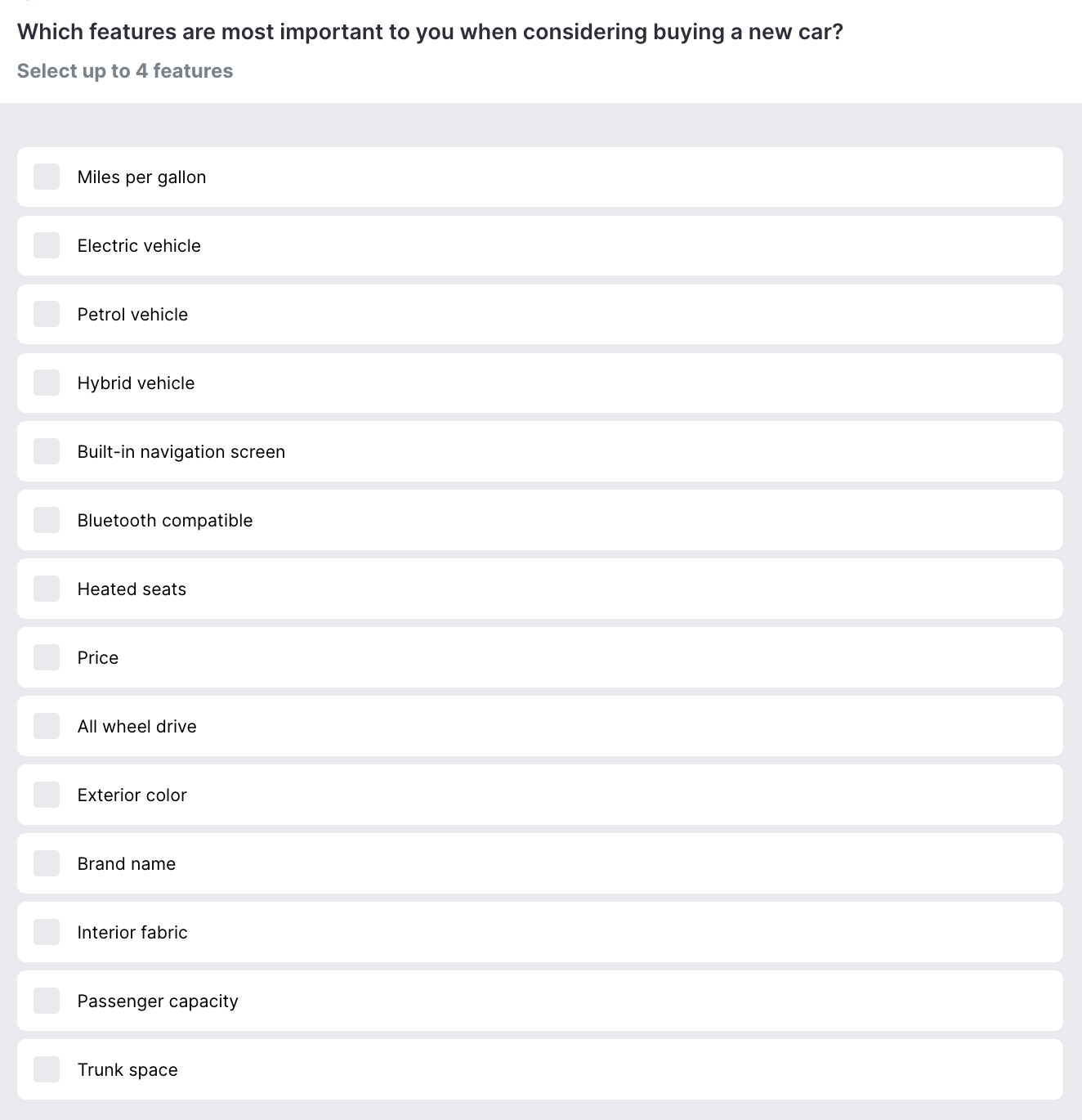
Aside from asking text-based questions like the above examples, a brand could also use a single or multi-select question to ask respondents to select the image they prefer more (like different iterations of a logo design, packaging options, branding colors, etc.).
dropdown#toggle" data-dropdown-placement-param="top" data-term-id="281139749">Likert dropdown#toggle" data-dropdown-placement-param="top" data-term-id="281139766">scale dropdown#toggle" data-dropdown-menu-id-param="menu_term_281139766" data-dropdown-placement-param="top" data-term-id="281139766"> questions
A dropdown#toggle" data-dropdown-placement-param="top" data-term-id="281139749">Likert scale is widely used as a convenient and easy-to-interpret rating method. dropdown#toggle" data-dropdown-placement-param="top" data-term-id="281139737">Respondents find it easy to indicate their degree of feelings by selecting the response they most identify with.
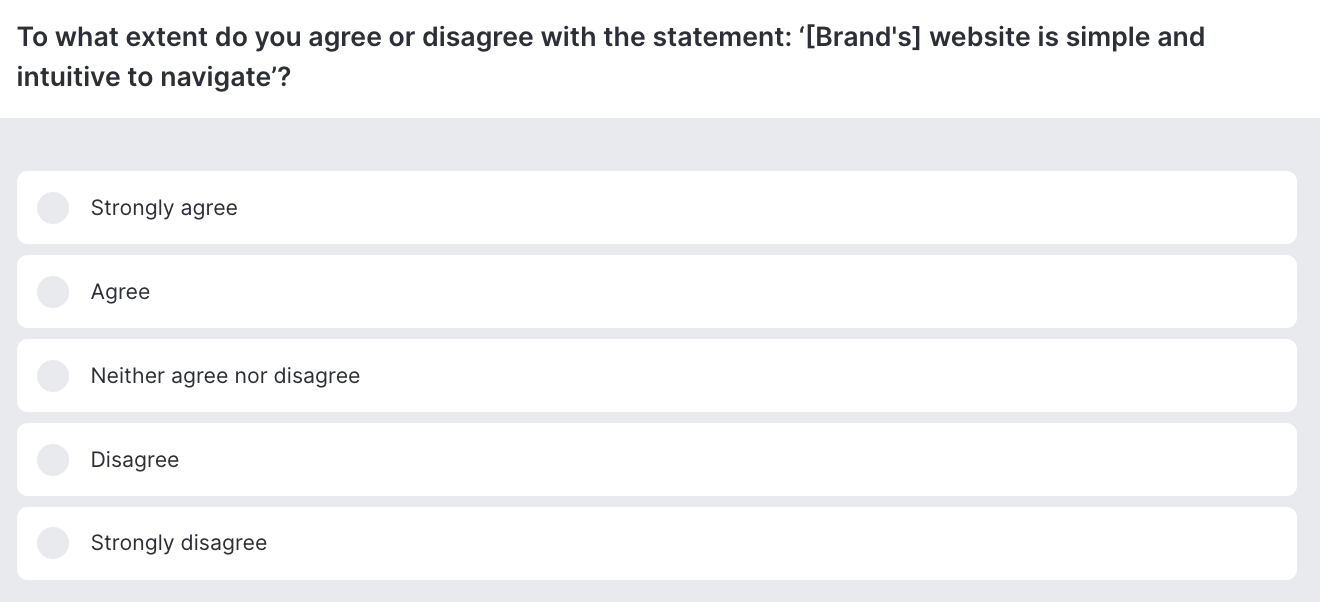
Slider scales
Slider scales are another good interactive way of formatting questions. They allow dropdown#toggle" data-dropdown-placement-param="top" data-term-id="281139737">respondents to customize their level of feeling about a question, with a bit more variance and nuance allowed than a numeric scale:
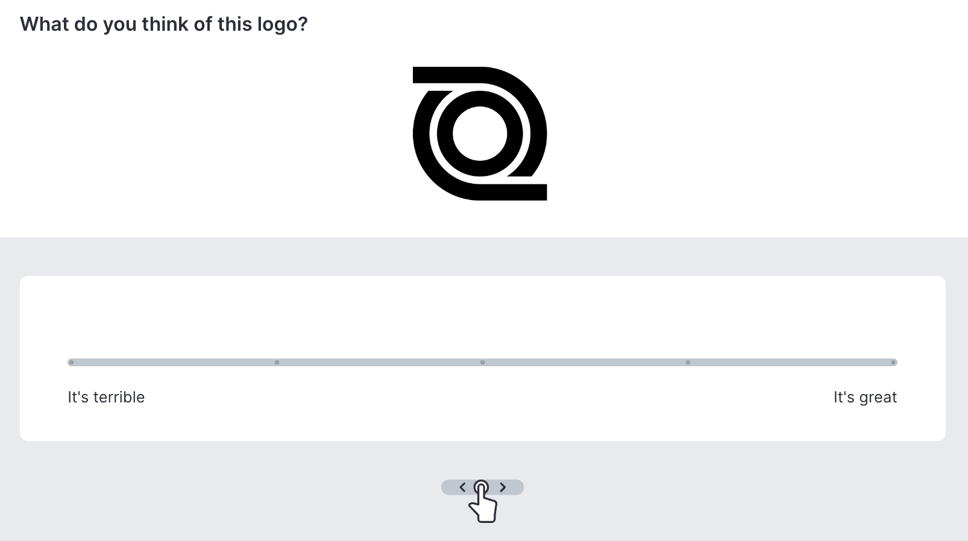
One particularly common use of a slider scale in a dropdown#toggle" data-dropdown-placement-param="top" data-term-id="281139740">market dropdown#toggle" data-dropdown-placement-param="top" data-term-id="281139770">research dropdown#toggle" data-dropdown-menu-id-param="menu_term_281139770" data-dropdown-placement-param="top" data-term-id="281139770"> study is known as a NPS (Net Promoter Score) - a way to measure dropdown#toggle" data-dropdown-placement-param="top" data-term-id="281139775">customer experience and loyalty . A 0-10 scale is used to ask customers how likely they are to recommend a brand’s product or services to others. The NPS score is calculated by subtracting the percentage of ‘detractors’ (those who respond with a 0-6) from the percentage of promoters (those who respond with a 9-10). dropdown#toggle" data-dropdown-placement-param="top" data-term-id="281139737">Respondents who select 7-8 are known as ‘passives’.
For example:

Drag and drop questions
Drag-and-drop question formats are a more ‘gamified’ approach to survey capture as they ask dropdown#toggle" data-dropdown-placement-param="top" data-term-id="281139737">respondents to do more than simply check boxes or slide a scale. Drag-and-drop question formats are great for ranking exercises - asking dropdown#toggle" data-dropdown-placement-param="top" data-term-id="281139737">respondents to place answer options in a certain order by dragging with their mouse. For example, you could ask survey takers to put pizza toppings in order of preference by dragging options from a list of possible answers to a box displaying their personal preferences:
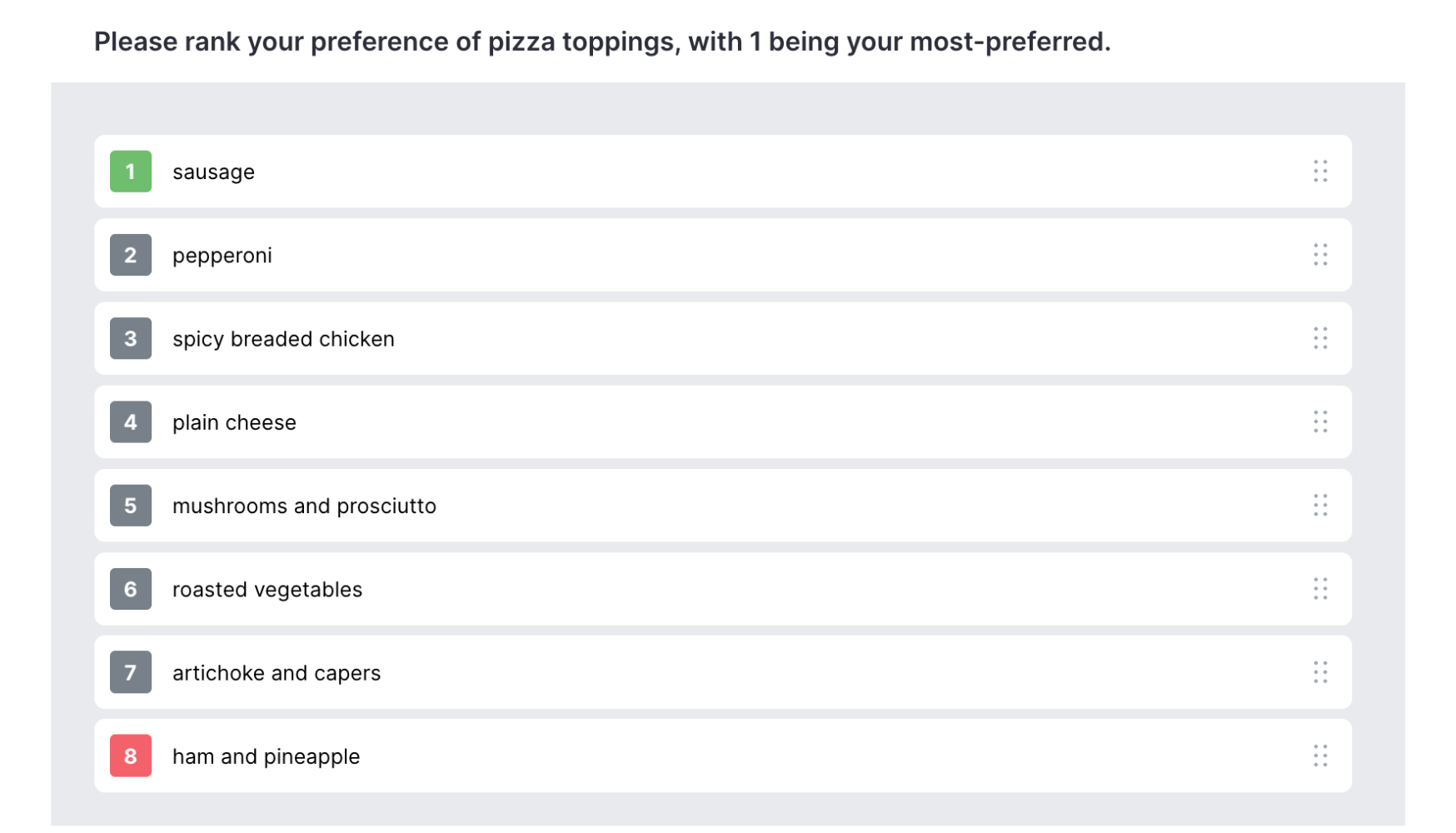
Matrix questions
Matrix questions are a great way to consolidate a number of questions that ask for the same type of response (e.g. single select yes/no, true/false, or multi-select lists). They are mutually beneficial - making a survey look less daunting for the dropdown#toggle" data-dropdown-placement-param="top" data-term-id="281139737">respondent , and easier for a brand to set up than asking multiple separate questions.
Items in a matrix question are presented one by one, as respondents cycle through the pages selecting one answer for each coffee flavor shown.
-1.png?width=1500&height=800&name=Untitled%20design%20(5)-1.png)
While the above example shows a single-matrix question - meaning a respondent can only select one answer per element (in this case, coffee flavors), a matrix setup can also be used for multiple-choice questions - allowing respondents to choose multiple answers per element shown, or for rating questions - allowing respondents to assign a rating (e.g. 1-5) for a list of elements at once. Back to table of contents
How to write dropdown#toggle" data-dropdown-placement-param="top" data-term-id="281139745">quantitative survey questions
We’ve reviewed the types of questions you might ask in a quantitative survey, and how you might format those questions, but now for the actual crafting of the content.
When considering which questions to include in your survey, you’ll first want to establish what your research goals are and how these relate to your business goals. For example, thinking about the three types of dropdown#toggle" data-dropdown-placement-param="top" data-term-id="281139745">quantitative survey questions explained above - descriptive, comparative, and relationship-based - which type (or which combination) will best meet your research needs? The questions you ask dropdown#toggle" data-dropdown-placement-param="top" data-term-id="281139737">respondents may be phrased in similar ways no matter what kind of layout you leverage, but you should have a good idea of how you’ll want to analyze the results as that will make it much easier to correctly set up your survey.
Quantitative questions tend to start with words like ‘how much,’ ‘how often,’ ‘to what degree,’ ‘what do you think of,’ ‘which of the following’ - anything that establishes what consumers do or think and that can be assigned a numerical code or value. Be sure to also include ‘other’ or ‘none of the above’ options in your quant questions, accommodating those who don’t feel the pre-set answers reflect their true opinion. As mentioned earlier, you can always include a small number of dropdown#toggle" data-dropdown-placement-param="top" data-term-id="281139748">open-ended questions in your quant survey to account for any ideas or expanded feedback that the pre-coded questions don’t (or can’t) cover. Back to table of contents
Examples of dropdown#toggle" data-dropdown-placement-param="top" data-term-id="281139745">quantitative survey questions
dropdown#toggle" data-dropdown-placement-param="top" data-term-id="281139745">Quantitative survey questions impose limits on the answers that dropdown#toggle" data-dropdown-placement-param="top" data-term-id="281139737">respondents can choose from, and this is a good thing when it comes to measuring consumer opinions on a large scale and comparing across dropdown#toggle" data-dropdown-placement-param="top" data-term-id="281139737">respondents . A large volume of freeform, open-ended answers is interesting when looking for themes from qualitative studies, but impractical to wade through when dealing with a large dropdown#toggle" data-dropdown-placement-param="top" data-term-id="281139756">sample size , and impossible to subject to dropdown#toggle" data-dropdown-placement-param="top" data-term-id="281139774">statistical analysis .
For example, a quantitative survey might aim to establish consumers' smartphone habits. This could include their frequency of buying a new smartphone, the considerations that drive purchase, which features they use their phone for, and how much they like their smartphone.
Some examples of quantitative survey questions relating to these habits would be:
Q. How often do you buy a new smartphone?
[single select question]
More than once per year
Every 1-2 years
Every 3-5 years
Every 6+ years
Q. Thinking about when you buy a smartphone, please rank the following factors in order of importance:
[drag and drop ranking question]
screen size
storage capacity
Q. How often do you use the following features on your smartphone?
[matrix question]
|
|
|
| ||
Q. How do you feel about your current smartphone?
[sliding scale]
I love it <-------> I hate it
Answers from these above questions, and others within the survey, would be analyzed to paint a picture of smartphone usage and attitude trends across a population and its sub-groups. dropdown#toggle" data-dropdown-placement-param="top" data-term-id="281139738">Qualitative research might then be carried out to explore those findings further - for example, people’s detailed attitudes towards their smartphones, how they feel about the amount of time they spend on it, and how features could be improved. Back to table of contents
quantilope’s Consumer Intelligence Platform specializes in automated, advanced survey insights so that researchers of any skill level can benefit from quick, high-quality consumer insights. With 12 advanced methods to choose from and a wide variety of quantitative question formats, quantilope is your one-stop-shop for all things dropdown#toggle" data-dropdown-placement-param="top" data-term-id="281139740">market research (including its dropdown#toggle" data-dropdown-placement-param="top" data-term-id="281139776">in-depth dropdown#toggle" data-dropdown-placement-param="top" data-term-id="281139738">qualitative research solution - inColor ).
When it comes to building your survey, you decide how you want to go about it. You can start with a blank slate and drop questions into your survey from a pre-programmed list, or you can get a head start with a survey dropdown#toggle" data-dropdown-placement-param="top" data-term-id="281139765">template for a particular business use case (like concept testing ) and customize from there. Once your survey is ready to launch, simply specify your dropdown#toggle" data-dropdown-placement-param="top" data-term-id="281139743">target audience , connect any panel (quantilope is panel agnostic), and watch as dropdown#toggle" data-dropdown-placement-param="top" data-term-id="281139737">respondents dropdown#toggle" data-dropdown-placement-param="top" data-term-id="281139783">answer questions in your survey in real-time by monitoring the fieldwork section of your project. AI-driven dropdown#toggle" data-dropdown-placement-param="top" data-term-id="281139764">data analysis takes the raw data and converts it into actionable findings so you never have to worry about manual calculations or statistical testing.
Whether you want to run your quantitative study entirely on your own or with the help of a classically trained research team member, the choice is yours on quantilope’s platform. For more information on how quantilope can help with your next dropdown#toggle" data-dropdown-placement-param="top" data-term-id="281139736">quantitative dropdown#toggle" data-dropdown-placement-param="top" data-term-id="281139768">research dropdown#toggle" data-dropdown-menu-id-param="menu_term_281139768" data-dropdown-placement-param="top" data-term-id="281139768"> project , get in touch below!
Get in touch to learn more about quantitative research with quantilope!
Latest articles.
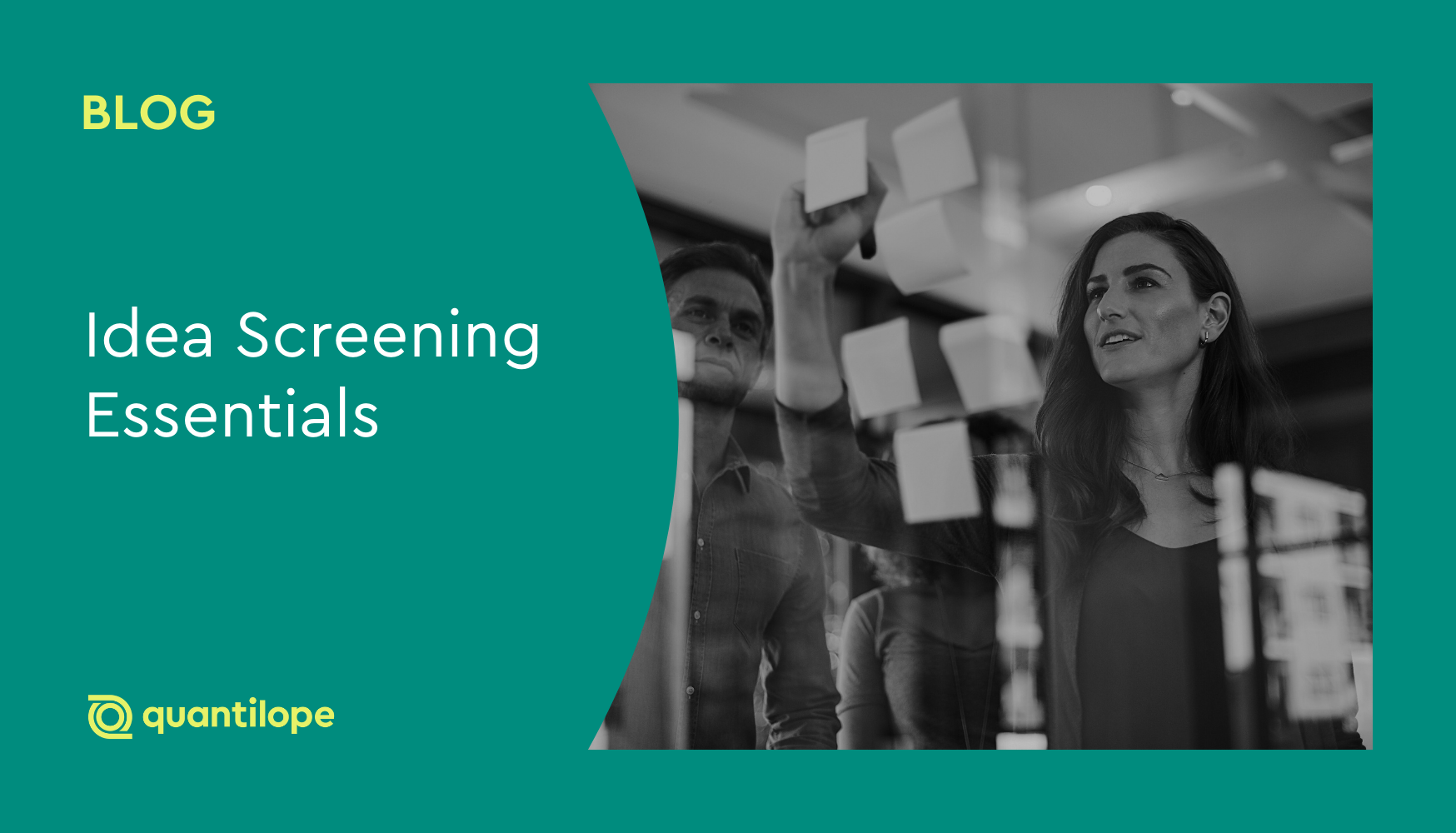
The Essential Guide to Idea Screening: Turning Concepts Into Reality
In this guide, we'll break down the essentials of idea screening, starting by defining its purpose and exploring the top techniques for suc...
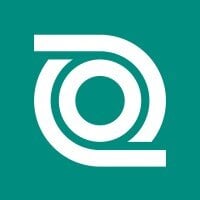
September 04, 2024
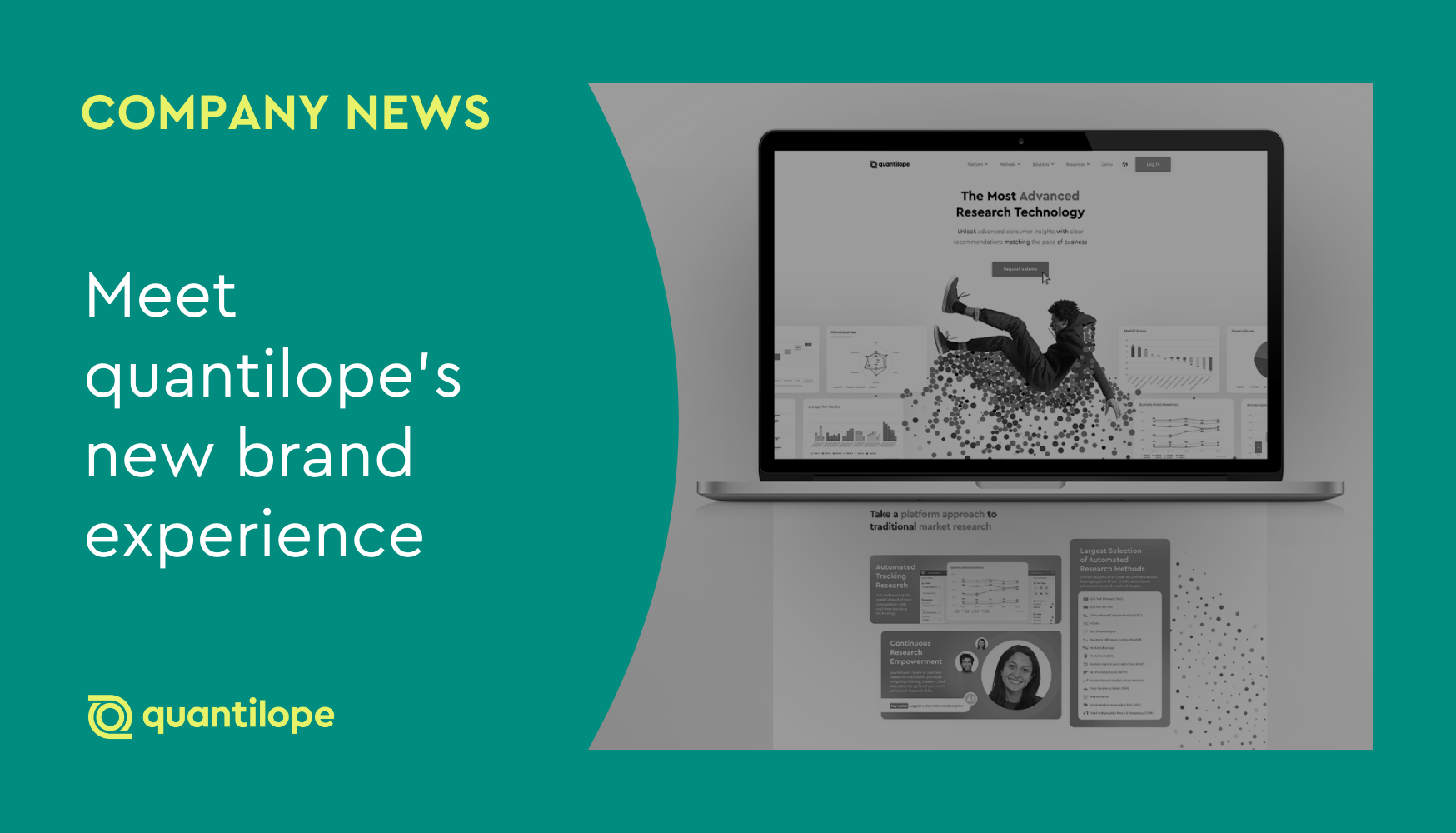
The New quantilope Brand Experience
Introducing quantilope's new brand experience featuring a brighter, fresher look and feel.
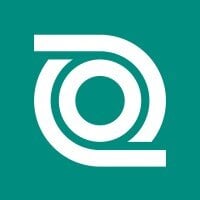
Unleash Brand Growth With Our Better Brand Health Tracking Certification
This Certification Course from the quantilope Academy empowers researchers to learn how to effectively grow their brand through Better Bran...
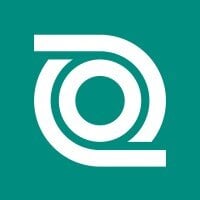
August 15, 2024
When are surveys qualitative or quantitative research: Learn the difference!
- December 9, 2019
Are surveys qualitative or quantitative methods of data collection?
What is a qualitative survey, what types of survey questions are analyzed qualitatively, benefits of using a qualitative survey, types of a survey questionnaire in qualitative research, what is a quantitative survey, benefits of using a quantitative survey, an example of a survey with quantitative data, qualitative versus quantitative survey question examples, when to use qualitative versus quantitative research.
If you are new to the world of creating surveys, you might have a few questions about what a survey is and the different types. At SurveyPlanet, we give you the tools you need to create any survey. We also want to help you understand how to create better surveys that serve your distinct purposes.
Interested in finding out when a survey is a part of qualitative versus quantitative research?
What is a survey and how to know if it is qualitative or quantitative?
A survey is a method of gathering information from a select sample of people. Responses can be used to gain insights and data that enable drawing conclusions about a subject. The sample size of a survey represents a larger population and there are two different types of research: qualitative and quantitative. The type of research determines which kind of questions to ask.
A survey can be qualitative or quantitative. If you create a questionnaire with answer options using a scale then it is quantitative. If you have questions that require detailed responses then it is qualitative. Mixed-method surveys involve both.
A qualitative survey collects data in order to describe a topic. In other words, the survey is more interested in learning about opinions, views, and impressions than numerical data. Qualitative surveys are less structured and offer insights into the way people think, their motivations, and attitudes toward a topic. Such surveys are more difficult to analyze but can supply much-needed depth to research. Qualitative surveys give answers to “why” and “how.”
Many of the most powerful surveys are qualitative. They collect data that enables an understanding of people’s attitudes, motivations, and experiences. Qualitative surveys provide a deeper level of insight into consumer behavior and preferences than quantitative surveys.
Qualitative research survey questions examples
Qualitative questions are a useful research method when the goal is describing certain phenomena rather than getting an exact answer. Therefore, instead of sitting down one-on-one with participants, survey questions have a short-answer box that respondents can use to express themselves. Qualitative research questions are open-ended and are useful for market research and other data collection purposes.
Read our “ How to Analyze Survey Data: Learn What to Do With Survey Responses? “ to optimize data collection and analysis.
For the most part, qualitative surveys are completely exploratory. Their main purpose is to understand the way a targeted group thinks—its opinions and attitudes about a particular topic. During the analysis phase, every word written by respondents can be analyzed to form a hypothesis.
Although this type of survey is great for learning more about personal opinions, it’s best suited for a small sample size. Conclusions aren’t necessarily representative of the targeted group, and instead only a small portion of it.
Despite small sample sizes, qualitative surveys are essential for identifying weak points in business operations. Once identified, create related questions to include in a quantitative survey, which often are not carried out without doing qualitative research beforehand.
Examples of qualitative research surveys
There are many different ways to use qualitative research, with qualitative questions often used in interviews that collect data from one person about one topic. If the plan is to send a qualitative research survey to employees about job satisfaction or company culture, interviewing a few employees first is a good start. This way, you have an idea of what topics to bring up as well as possible follow-up questions. Think of qualitative surveys as a way to gain insight that will help in the creation of a comprehensive quantitative survey down the line.
Another example of qualitative research is a case study, which is like interviews in that they collect data from one source and are primarily focused on opinions. If you want to use a case study as a marketing tool to attract more customers, conduct a one-on-one interview and ask participants a series of questions about your business that can be showcased on your website.
Expert opinions are another example of qualitative research in which an expert weighs in on a topic. Again, this is a way to gather insights from a single source about a specific topic.
Yet another example of qualitative research is focus groups, where A small sample size is asked for opinions on a certain subject. Focus groups allow the reactions of individuals to be gauged in a free-flowing setting. This is a great way to test a new product or marketing strategy.
You can also collect the same type of information by conducting a qualitative survey.
A quantitative survey collects facts and numbers from respondents. It’s most commonly used to prove or disprove a hypothesis after completing qualitative research. The analysis phase looks at the statistical data to draw conclusions, such as proving or disproving a specific hypothesis. Choosing the right type of survey to distribute depends on the ultimate goal of the research.
There are several benefits to using quantitative surveys to collect data. For one, they allow the testing and substantiation of conclusions previously developed. The analysis phase is usually straightforward since it involves looking at numbers. Short, written answers do not need to be analyzed. There are no opinions or detailed answers involved.
On the flip side, quantitative surveys aren’t always ideal because a large sample size is required to come to a credible conclusion. For example, a survey sent to 100 people to sample the operations of a business with millions of customers is not credible. It’s safe to say that the answers of 100 participants won’t represent the entire customer base. Read about analyzing survey data correctly here .
Quantitative research can be conducted by carrying out one of two types of surveys. The first is a cross-sectional survey, which gives multiple variables to analyze during a particular time period. It’s most common in the health care, retail, and small to medium-sized enterprise (SME) industries.
The other type is a longitudinal survey. This type of survey is commonly conducted over a certain amount of time, anywhere from days to years. The purpose is to observe changes in behavior or thought processes over time. For example, the buying habits of a teenager can be tracked through their adult years. This type of survey is ideal for long-term feedback on services or products, or when a certain sequence of events is important.
To sum up and better illustrate the theme, we prepared a table with quantitative and qualitative survey question examples that will aid in the writing of an excellent survey.
Qualitative questions example | Quantitative questions examples |
What is your most important consideration when choosing a certain service? | How often do you use our services? |
What are your impressions of our services? | How likely are you to use our services again? |
Describe the last time you used our services. | On a scale of 1–5, how satisfied were you with your customer service experience? |
How would you describe your experience? | How strongly do you agree with the following statement: |
How would you improve our services? | Would you recommend our services to a friend? |
So, when do you use a qualitative survey as opposed to a quantitative survey? Use qualitative research when the main objective is to understand respondents’ motivations and opinions or gather insights with which to create a hypothesis. Use quantitative research to measure findings from qualitative research. The data gathered from quantitative research will usually allow for a conclusion to be drawn, while qualitative research only allows for the development of a hypothesis.
If you’re ready to test out either qualitative or quantitative research, it’s time to create a survey and get started. SurveyPlanet has many different themes and pre-made surveys to help. Sign up for a free account today! If you want to gather more insights and further expand your research, our Pro plan gives access to even more features (such as question branching).
Photo by John Schnobrich on Unsplash
Quickonomics
Survey Data
Published Sep 8, 2024
Definition of Survey Data
Survey data is information collected from a predefined group of respondents using a structured questionnaire or interview process. The purpose of gathering survey data is to gain insights into the views, behaviors, or characteristics of a particular population. Surveys can be conducted via various methods, including online questionnaires, telephone interviews, face-to-face interactions, and mailed paper surveys. The data collected through surveys can be either quantitative (numerical) or qualitative (descriptive), depending on the nature of the questions asked.
Imagine a company launching a new product line and wanting to understand potential customer reactions. To do this, they conduct a survey among a random sample of their target market. The survey includes questions about customer preferences, buying habits, and demographics such as age, income, and location. By analyzing the responses, the company can gauge interest in their product, identify target demographics, and refine their marketing strategies accordingly.
For example, if a significant number of respondents indicate a preference for eco-friendly packaging, the company might emphasize this feature in their product development and advertising. Similarly, survey data showing a higher interest among younger consumers could lead to targeted social media campaigns to reach this demographic more effectively.
Why Survey Data Matters
Survey data plays a crucial role in decision-making for businesses, governments, and researchers. Here are some key reasons why survey data is important:
- Market Research: Businesses use surveys to understand customer needs, preferences, and satisfaction levels, which helps them develop products and services that better meet market demands.
- Policy Making: Governments and public organizations collect survey data to inform policy decisions, such as public health initiatives, educational programs, and social services.
- Academic Research: Researchers utilize surveys to gather empirical data for studies in fields like sociology, psychology, and economics, contributing to academic knowledge and theory development.
- Benchmarking and Performance Measurement: Organizations use survey data to benchmark performance, assess employee satisfaction, and identify areas for improvement.
Frequently Asked Questions (FAQ)
What are the common methods of collecting survey data.
There are several methods for collecting survey data:
- Online Surveys: Conducted via websites or email, online surveys are popular due to their cost-effectiveness and wide reach. Tools like SurveyMonkey and Google Forms are commonly used.
- Telephone Surveys: Researchers call respondents to collect data. This method allows for more in-depth responses but can be more expensive and time-consuming.
- Face-to-Face Surveys: Conducted in person, these surveys allow for detailed questioning and observation of respondent behavior but can be resource-intensive.
- Mail Surveys: Questionnaires are sent by postal mail and returned by respondents. While this method can reach a broad audience, response rates tend to be lower compared to other methods.
How do researchers ensure the reliability and validity of survey data?
Researchers take several steps to ensure the reliability and validity of survey data:
- Questionnaire Design: Questions are carefully crafted to be clear, unbiased, and relevant to the survey objectives.
- Sampling Techniques: Random sampling, stratified sampling, and other methods are used to ensure the survey sample adequately represents the population.
- Pilot Testing: Surveys are pre-tested with a small group to identify any issues and make necessary adjustments.
- Data Collection Monitoring: Researchers track survey administration to ensure consistent data collection across different respondents and minimize errors.
What are some challenges in collecting and analyzing survey data?
Collecting and analyzing survey data can present various challenges:
- Response Bias: Respondents may provide socially desirable answers or may not accurately recall information, leading to biased data.
- Low Response Rates: Particularly with mail and online surveys, achieving a high response rate can be difficult, which may affect the representativeness of the data.
- Data Quality: Incomplete or incorrectly filled-out surveys can compromise data quality and require extensive cleaning and validation.
- Analysis Complexity: Analyzing survey data, especially when dealing with large datasets or complex statistical techniques, can be resource-intensive and require specialized skills.
Survey data remains a valuable tool for gaining insights and making informed decisions, but addressing these challenges is key to obtaining reliable and actionable results.
To provide the best experiences, we and our partners use technologies like cookies to store and/or access device information. Consenting to these technologies will allow us and our partners to process personal data such as browsing behavior or unique IDs on this site and show (non-) personalized ads. Not consenting or withdrawing consent, may adversely affect certain features and functions.
Click below to consent to the above or make granular choices. Your choices will be applied to this site only. You can change your settings at any time, including withdrawing your consent, by using the toggles on the Cookie Policy, or by clicking on the manage consent button at the bottom of the screen.

An official website of the United States government
The .gov means it’s official. Federal government websites often end in .gov or .mil. Before sharing sensitive information, make sure you’re on a federal government site.
The site is secure. The https:// ensures that you are connecting to the official website and that any information you provide is encrypted and transmitted securely.
- Publications
- Account settings
Preview improvements coming to the PMC website in October 2024. Learn More or Try it out now .
- Advanced Search
- Journal List
- J Korean Med Sci
- v.37(16); 2022 Apr 25

A Practical Guide to Writing Quantitative and Qualitative Research Questions and Hypotheses in Scholarly Articles
Edward barroga.
1 Department of General Education, Graduate School of Nursing Science, St. Luke’s International University, Tokyo, Japan.
Glafera Janet Matanguihan
2 Department of Biological Sciences, Messiah University, Mechanicsburg, PA, USA.
The development of research questions and the subsequent hypotheses are prerequisites to defining the main research purpose and specific objectives of a study. Consequently, these objectives determine the study design and research outcome. The development of research questions is a process based on knowledge of current trends, cutting-edge studies, and technological advances in the research field. Excellent research questions are focused and require a comprehensive literature search and in-depth understanding of the problem being investigated. Initially, research questions may be written as descriptive questions which could be developed into inferential questions. These questions must be specific and concise to provide a clear foundation for developing hypotheses. Hypotheses are more formal predictions about the research outcomes. These specify the possible results that may or may not be expected regarding the relationship between groups. Thus, research questions and hypotheses clarify the main purpose and specific objectives of the study, which in turn dictate the design of the study, its direction, and outcome. Studies developed from good research questions and hypotheses will have trustworthy outcomes with wide-ranging social and health implications.
INTRODUCTION
Scientific research is usually initiated by posing evidenced-based research questions which are then explicitly restated as hypotheses. 1 , 2 The hypotheses provide directions to guide the study, solutions, explanations, and expected results. 3 , 4 Both research questions and hypotheses are essentially formulated based on conventional theories and real-world processes, which allow the inception of novel studies and the ethical testing of ideas. 5 , 6
It is crucial to have knowledge of both quantitative and qualitative research 2 as both types of research involve writing research questions and hypotheses. 7 However, these crucial elements of research are sometimes overlooked; if not overlooked, then framed without the forethought and meticulous attention it needs. Planning and careful consideration are needed when developing quantitative or qualitative research, particularly when conceptualizing research questions and hypotheses. 4
There is a continuing need to support researchers in the creation of innovative research questions and hypotheses, as well as for journal articles that carefully review these elements. 1 When research questions and hypotheses are not carefully thought of, unethical studies and poor outcomes usually ensue. Carefully formulated research questions and hypotheses define well-founded objectives, which in turn determine the appropriate design, course, and outcome of the study. This article then aims to discuss in detail the various aspects of crafting research questions and hypotheses, with the goal of guiding researchers as they develop their own. Examples from the authors and peer-reviewed scientific articles in the healthcare field are provided to illustrate key points.
DEFINITIONS AND RELATIONSHIP OF RESEARCH QUESTIONS AND HYPOTHESES
A research question is what a study aims to answer after data analysis and interpretation. The answer is written in length in the discussion section of the paper. Thus, the research question gives a preview of the different parts and variables of the study meant to address the problem posed in the research question. 1 An excellent research question clarifies the research writing while facilitating understanding of the research topic, objective, scope, and limitations of the study. 5
On the other hand, a research hypothesis is an educated statement of an expected outcome. This statement is based on background research and current knowledge. 8 , 9 The research hypothesis makes a specific prediction about a new phenomenon 10 or a formal statement on the expected relationship between an independent variable and a dependent variable. 3 , 11 It provides a tentative answer to the research question to be tested or explored. 4
Hypotheses employ reasoning to predict a theory-based outcome. 10 These can also be developed from theories by focusing on components of theories that have not yet been observed. 10 The validity of hypotheses is often based on the testability of the prediction made in a reproducible experiment. 8
Conversely, hypotheses can also be rephrased as research questions. Several hypotheses based on existing theories and knowledge may be needed to answer a research question. Developing ethical research questions and hypotheses creates a research design that has logical relationships among variables. These relationships serve as a solid foundation for the conduct of the study. 4 , 11 Haphazardly constructed research questions can result in poorly formulated hypotheses and improper study designs, leading to unreliable results. Thus, the formulations of relevant research questions and verifiable hypotheses are crucial when beginning research. 12
CHARACTERISTICS OF GOOD RESEARCH QUESTIONS AND HYPOTHESES
Excellent research questions are specific and focused. These integrate collective data and observations to confirm or refute the subsequent hypotheses. Well-constructed hypotheses are based on previous reports and verify the research context. These are realistic, in-depth, sufficiently complex, and reproducible. More importantly, these hypotheses can be addressed and tested. 13
There are several characteristics of well-developed hypotheses. Good hypotheses are 1) empirically testable 7 , 10 , 11 , 13 ; 2) backed by preliminary evidence 9 ; 3) testable by ethical research 7 , 9 ; 4) based on original ideas 9 ; 5) have evidenced-based logical reasoning 10 ; and 6) can be predicted. 11 Good hypotheses can infer ethical and positive implications, indicating the presence of a relationship or effect relevant to the research theme. 7 , 11 These are initially developed from a general theory and branch into specific hypotheses by deductive reasoning. In the absence of a theory to base the hypotheses, inductive reasoning based on specific observations or findings form more general hypotheses. 10
TYPES OF RESEARCH QUESTIONS AND HYPOTHESES
Research questions and hypotheses are developed according to the type of research, which can be broadly classified into quantitative and qualitative research. We provide a summary of the types of research questions and hypotheses under quantitative and qualitative research categories in Table 1 .
Quantitative research questions | Quantitative research hypotheses |
---|---|
Descriptive research questions | Simple hypothesis |
Comparative research questions | Complex hypothesis |
Relationship research questions | Directional hypothesis |
Non-directional hypothesis | |
Associative hypothesis | |
Causal hypothesis | |
Null hypothesis | |
Alternative hypothesis | |
Working hypothesis | |
Statistical hypothesis | |
Logical hypothesis | |
Hypothesis-testing | |
Qualitative research questions | Qualitative research hypotheses |
Contextual research questions | Hypothesis-generating |
Descriptive research questions | |
Evaluation research questions | |
Explanatory research questions | |
Exploratory research questions | |
Generative research questions | |
Ideological research questions | |
Ethnographic research questions | |
Phenomenological research questions | |
Grounded theory questions | |
Qualitative case study questions |
Research questions in quantitative research
In quantitative research, research questions inquire about the relationships among variables being investigated and are usually framed at the start of the study. These are precise and typically linked to the subject population, dependent and independent variables, and research design. 1 Research questions may also attempt to describe the behavior of a population in relation to one or more variables, or describe the characteristics of variables to be measured ( descriptive research questions ). 1 , 5 , 14 These questions may also aim to discover differences between groups within the context of an outcome variable ( comparative research questions ), 1 , 5 , 14 or elucidate trends and interactions among variables ( relationship research questions ). 1 , 5 We provide examples of descriptive, comparative, and relationship research questions in quantitative research in Table 2 .
Quantitative research questions | |
---|---|
Descriptive research question | |
- Measures responses of subjects to variables | |
- Presents variables to measure, analyze, or assess | |
What is the proportion of resident doctors in the hospital who have mastered ultrasonography (response of subjects to a variable) as a diagnostic technique in their clinical training? | |
Comparative research question | |
- Clarifies difference between one group with outcome variable and another group without outcome variable | |
Is there a difference in the reduction of lung metastasis in osteosarcoma patients who received the vitamin D adjunctive therapy (group with outcome variable) compared with osteosarcoma patients who did not receive the vitamin D adjunctive therapy (group without outcome variable)? | |
- Compares the effects of variables | |
How does the vitamin D analogue 22-Oxacalcitriol (variable 1) mimic the antiproliferative activity of 1,25-Dihydroxyvitamin D (variable 2) in osteosarcoma cells? | |
Relationship research question | |
- Defines trends, association, relationships, or interactions between dependent variable and independent variable | |
Is there a relationship between the number of medical student suicide (dependent variable) and the level of medical student stress (independent variable) in Japan during the first wave of the COVID-19 pandemic? |
Hypotheses in quantitative research
In quantitative research, hypotheses predict the expected relationships among variables. 15 Relationships among variables that can be predicted include 1) between a single dependent variable and a single independent variable ( simple hypothesis ) or 2) between two or more independent and dependent variables ( complex hypothesis ). 4 , 11 Hypotheses may also specify the expected direction to be followed and imply an intellectual commitment to a particular outcome ( directional hypothesis ) 4 . On the other hand, hypotheses may not predict the exact direction and are used in the absence of a theory, or when findings contradict previous studies ( non-directional hypothesis ). 4 In addition, hypotheses can 1) define interdependency between variables ( associative hypothesis ), 4 2) propose an effect on the dependent variable from manipulation of the independent variable ( causal hypothesis ), 4 3) state a negative relationship between two variables ( null hypothesis ), 4 , 11 , 15 4) replace the working hypothesis if rejected ( alternative hypothesis ), 15 explain the relationship of phenomena to possibly generate a theory ( working hypothesis ), 11 5) involve quantifiable variables that can be tested statistically ( statistical hypothesis ), 11 6) or express a relationship whose interlinks can be verified logically ( logical hypothesis ). 11 We provide examples of simple, complex, directional, non-directional, associative, causal, null, alternative, working, statistical, and logical hypotheses in quantitative research, as well as the definition of quantitative hypothesis-testing research in Table 3 .
Quantitative research hypotheses | |
---|---|
Simple hypothesis | |
- Predicts relationship between single dependent variable and single independent variable | |
If the dose of the new medication (single independent variable) is high, blood pressure (single dependent variable) is lowered. | |
Complex hypothesis | |
- Foretells relationship between two or more independent and dependent variables | |
The higher the use of anticancer drugs, radiation therapy, and adjunctive agents (3 independent variables), the higher would be the survival rate (1 dependent variable). | |
Directional hypothesis | |
- Identifies study direction based on theory towards particular outcome to clarify relationship between variables | |
Privately funded research projects will have a larger international scope (study direction) than publicly funded research projects. | |
Non-directional hypothesis | |
- Nature of relationship between two variables or exact study direction is not identified | |
- Does not involve a theory | |
Women and men are different in terms of helpfulness. (Exact study direction is not identified) | |
Associative hypothesis | |
- Describes variable interdependency | |
- Change in one variable causes change in another variable | |
A larger number of people vaccinated against COVID-19 in the region (change in independent variable) will reduce the region’s incidence of COVID-19 infection (change in dependent variable). | |
Causal hypothesis | |
- An effect on dependent variable is predicted from manipulation of independent variable | |
A change into a high-fiber diet (independent variable) will reduce the blood sugar level (dependent variable) of the patient. | |
Null hypothesis | |
- A negative statement indicating no relationship or difference between 2 variables | |
There is no significant difference in the severity of pulmonary metastases between the new drug (variable 1) and the current drug (variable 2). | |
Alternative hypothesis | |
- Following a null hypothesis, an alternative hypothesis predicts a relationship between 2 study variables | |
The new drug (variable 1) is better on average in reducing the level of pain from pulmonary metastasis than the current drug (variable 2). | |
Working hypothesis | |
- A hypothesis that is initially accepted for further research to produce a feasible theory | |
Dairy cows fed with concentrates of different formulations will produce different amounts of milk. | |
Statistical hypothesis | |
- Assumption about the value of population parameter or relationship among several population characteristics | |
- Validity tested by a statistical experiment or analysis | |
The mean recovery rate from COVID-19 infection (value of population parameter) is not significantly different between population 1 and population 2. | |
There is a positive correlation between the level of stress at the workplace and the number of suicides (population characteristics) among working people in Japan. | |
Logical hypothesis | |
- Offers or proposes an explanation with limited or no extensive evidence | |
If healthcare workers provide more educational programs about contraception methods, the number of adolescent pregnancies will be less. | |
Hypothesis-testing (Quantitative hypothesis-testing research) | |
- Quantitative research uses deductive reasoning. | |
- This involves the formation of a hypothesis, collection of data in the investigation of the problem, analysis and use of the data from the investigation, and drawing of conclusions to validate or nullify the hypotheses. |
Research questions in qualitative research
Unlike research questions in quantitative research, research questions in qualitative research are usually continuously reviewed and reformulated. The central question and associated subquestions are stated more than the hypotheses. 15 The central question broadly explores a complex set of factors surrounding the central phenomenon, aiming to present the varied perspectives of participants. 15
There are varied goals for which qualitative research questions are developed. These questions can function in several ways, such as to 1) identify and describe existing conditions ( contextual research question s); 2) describe a phenomenon ( descriptive research questions ); 3) assess the effectiveness of existing methods, protocols, theories, or procedures ( evaluation research questions ); 4) examine a phenomenon or analyze the reasons or relationships between subjects or phenomena ( explanatory research questions ); or 5) focus on unknown aspects of a particular topic ( exploratory research questions ). 5 In addition, some qualitative research questions provide new ideas for the development of theories and actions ( generative research questions ) or advance specific ideologies of a position ( ideological research questions ). 1 Other qualitative research questions may build on a body of existing literature and become working guidelines ( ethnographic research questions ). Research questions may also be broadly stated without specific reference to the existing literature or a typology of questions ( phenomenological research questions ), may be directed towards generating a theory of some process ( grounded theory questions ), or may address a description of the case and the emerging themes ( qualitative case study questions ). 15 We provide examples of contextual, descriptive, evaluation, explanatory, exploratory, generative, ideological, ethnographic, phenomenological, grounded theory, and qualitative case study research questions in qualitative research in Table 4 , and the definition of qualitative hypothesis-generating research in Table 5 .
Qualitative research questions | |
---|---|
Contextual research question | |
- Ask the nature of what already exists | |
- Individuals or groups function to further clarify and understand the natural context of real-world problems | |
What are the experiences of nurses working night shifts in healthcare during the COVID-19 pandemic? (natural context of real-world problems) | |
Descriptive research question | |
- Aims to describe a phenomenon | |
What are the different forms of disrespect and abuse (phenomenon) experienced by Tanzanian women when giving birth in healthcare facilities? | |
Evaluation research question | |
- Examines the effectiveness of existing practice or accepted frameworks | |
How effective are decision aids (effectiveness of existing practice) in helping decide whether to give birth at home or in a healthcare facility? | |
Explanatory research question | |
- Clarifies a previously studied phenomenon and explains why it occurs | |
Why is there an increase in teenage pregnancy (phenomenon) in Tanzania? | |
Exploratory research question | |
- Explores areas that have not been fully investigated to have a deeper understanding of the research problem | |
What factors affect the mental health of medical students (areas that have not yet been fully investigated) during the COVID-19 pandemic? | |
Generative research question | |
- Develops an in-depth understanding of people’s behavior by asking ‘how would’ or ‘what if’ to identify problems and find solutions | |
How would the extensive research experience of the behavior of new staff impact the success of the novel drug initiative? | |
Ideological research question | |
- Aims to advance specific ideas or ideologies of a position | |
Are Japanese nurses who volunteer in remote African hospitals able to promote humanized care of patients (specific ideas or ideologies) in the areas of safe patient environment, respect of patient privacy, and provision of accurate information related to health and care? | |
Ethnographic research question | |
- Clarifies peoples’ nature, activities, their interactions, and the outcomes of their actions in specific settings | |
What are the demographic characteristics, rehabilitative treatments, community interactions, and disease outcomes (nature, activities, their interactions, and the outcomes) of people in China who are suffering from pneumoconiosis? | |
Phenomenological research question | |
- Knows more about the phenomena that have impacted an individual | |
What are the lived experiences of parents who have been living with and caring for children with a diagnosis of autism? (phenomena that have impacted an individual) | |
Grounded theory question | |
- Focuses on social processes asking about what happens and how people interact, or uncovering social relationships and behaviors of groups | |
What are the problems that pregnant adolescents face in terms of social and cultural norms (social processes), and how can these be addressed? | |
Qualitative case study question | |
- Assesses a phenomenon using different sources of data to answer “why” and “how” questions | |
- Considers how the phenomenon is influenced by its contextual situation. | |
How does quitting work and assuming the role of a full-time mother (phenomenon assessed) change the lives of women in Japan? |
Qualitative research hypotheses | |
---|---|
Hypothesis-generating (Qualitative hypothesis-generating research) | |
- Qualitative research uses inductive reasoning. | |
- This involves data collection from study participants or the literature regarding a phenomenon of interest, using the collected data to develop a formal hypothesis, and using the formal hypothesis as a framework for testing the hypothesis. | |
- Qualitative exploratory studies explore areas deeper, clarifying subjective experience and allowing formulation of a formal hypothesis potentially testable in a future quantitative approach. |
Qualitative studies usually pose at least one central research question and several subquestions starting with How or What . These research questions use exploratory verbs such as explore or describe . These also focus on one central phenomenon of interest, and may mention the participants and research site. 15
Hypotheses in qualitative research
Hypotheses in qualitative research are stated in the form of a clear statement concerning the problem to be investigated. Unlike in quantitative research where hypotheses are usually developed to be tested, qualitative research can lead to both hypothesis-testing and hypothesis-generating outcomes. 2 When studies require both quantitative and qualitative research questions, this suggests an integrative process between both research methods wherein a single mixed-methods research question can be developed. 1
FRAMEWORKS FOR DEVELOPING RESEARCH QUESTIONS AND HYPOTHESES
Research questions followed by hypotheses should be developed before the start of the study. 1 , 12 , 14 It is crucial to develop feasible research questions on a topic that is interesting to both the researcher and the scientific community. This can be achieved by a meticulous review of previous and current studies to establish a novel topic. Specific areas are subsequently focused on to generate ethical research questions. The relevance of the research questions is evaluated in terms of clarity of the resulting data, specificity of the methodology, objectivity of the outcome, depth of the research, and impact of the study. 1 , 5 These aspects constitute the FINER criteria (i.e., Feasible, Interesting, Novel, Ethical, and Relevant). 1 Clarity and effectiveness are achieved if research questions meet the FINER criteria. In addition to the FINER criteria, Ratan et al. described focus, complexity, novelty, feasibility, and measurability for evaluating the effectiveness of research questions. 14
The PICOT and PEO frameworks are also used when developing research questions. 1 The following elements are addressed in these frameworks, PICOT: P-population/patients/problem, I-intervention or indicator being studied, C-comparison group, O-outcome of interest, and T-timeframe of the study; PEO: P-population being studied, E-exposure to preexisting conditions, and O-outcome of interest. 1 Research questions are also considered good if these meet the “FINERMAPS” framework: Feasible, Interesting, Novel, Ethical, Relevant, Manageable, Appropriate, Potential value/publishable, and Systematic. 14
As we indicated earlier, research questions and hypotheses that are not carefully formulated result in unethical studies or poor outcomes. To illustrate this, we provide some examples of ambiguous research question and hypotheses that result in unclear and weak research objectives in quantitative research ( Table 6 ) 16 and qualitative research ( Table 7 ) 17 , and how to transform these ambiguous research question(s) and hypothesis(es) into clear and good statements.
Variables | Unclear and weak statement (Statement 1) | Clear and good statement (Statement 2) | Points to avoid |
---|---|---|---|
Research question | Which is more effective between smoke moxibustion and smokeless moxibustion? | “Moreover, regarding smoke moxibustion versus smokeless moxibustion, it remains unclear which is more effective, safe, and acceptable to pregnant women, and whether there is any difference in the amount of heat generated.” | 1) Vague and unfocused questions |
2) Closed questions simply answerable by yes or no | |||
3) Questions requiring a simple choice | |||
Hypothesis | The smoke moxibustion group will have higher cephalic presentation. | “Hypothesis 1. The smoke moxibustion stick group (SM group) and smokeless moxibustion stick group (-SLM group) will have higher rates of cephalic presentation after treatment than the control group. | 1) Unverifiable hypotheses |
Hypothesis 2. The SM group and SLM group will have higher rates of cephalic presentation at birth than the control group. | 2) Incompletely stated groups of comparison | ||
Hypothesis 3. There will be no significant differences in the well-being of the mother and child among the three groups in terms of the following outcomes: premature birth, premature rupture of membranes (PROM) at < 37 weeks, Apgar score < 7 at 5 min, umbilical cord blood pH < 7.1, admission to neonatal intensive care unit (NICU), and intrauterine fetal death.” | 3) Insufficiently described variables or outcomes | ||
Research objective | To determine which is more effective between smoke moxibustion and smokeless moxibustion. | “The specific aims of this pilot study were (a) to compare the effects of smoke moxibustion and smokeless moxibustion treatments with the control group as a possible supplement to ECV for converting breech presentation to cephalic presentation and increasing adherence to the newly obtained cephalic position, and (b) to assess the effects of these treatments on the well-being of the mother and child.” | 1) Poor understanding of the research question and hypotheses |
2) Insufficient description of population, variables, or study outcomes |
a These statements were composed for comparison and illustrative purposes only.
b These statements are direct quotes from Higashihara and Horiuchi. 16
Variables | Unclear and weak statement (Statement 1) | Clear and good statement (Statement 2) | Points to avoid |
---|---|---|---|
Research question | Does disrespect and abuse (D&A) occur in childbirth in Tanzania? | How does disrespect and abuse (D&A) occur and what are the types of physical and psychological abuses observed in midwives’ actual care during facility-based childbirth in urban Tanzania? | 1) Ambiguous or oversimplistic questions |
2) Questions unverifiable by data collection and analysis | |||
Hypothesis | Disrespect and abuse (D&A) occur in childbirth in Tanzania. | Hypothesis 1: Several types of physical and psychological abuse by midwives in actual care occur during facility-based childbirth in urban Tanzania. | 1) Statements simply expressing facts |
Hypothesis 2: Weak nursing and midwifery management contribute to the D&A of women during facility-based childbirth in urban Tanzania. | 2) Insufficiently described concepts or variables | ||
Research objective | To describe disrespect and abuse (D&A) in childbirth in Tanzania. | “This study aimed to describe from actual observations the respectful and disrespectful care received by women from midwives during their labor period in two hospitals in urban Tanzania.” | 1) Statements unrelated to the research question and hypotheses |
2) Unattainable or unexplorable objectives |
a This statement is a direct quote from Shimoda et al. 17
The other statements were composed for comparison and illustrative purposes only.
CONSTRUCTING RESEARCH QUESTIONS AND HYPOTHESES
To construct effective research questions and hypotheses, it is very important to 1) clarify the background and 2) identify the research problem at the outset of the research, within a specific timeframe. 9 Then, 3) review or conduct preliminary research to collect all available knowledge about the possible research questions by studying theories and previous studies. 18 Afterwards, 4) construct research questions to investigate the research problem. Identify variables to be accessed from the research questions 4 and make operational definitions of constructs from the research problem and questions. Thereafter, 5) construct specific deductive or inductive predictions in the form of hypotheses. 4 Finally, 6) state the study aims . This general flow for constructing effective research questions and hypotheses prior to conducting research is shown in Fig. 1 .

Research questions are used more frequently in qualitative research than objectives or hypotheses. 3 These questions seek to discover, understand, explore or describe experiences by asking “What” or “How.” The questions are open-ended to elicit a description rather than to relate variables or compare groups. The questions are continually reviewed, reformulated, and changed during the qualitative study. 3 Research questions are also used more frequently in survey projects than hypotheses in experiments in quantitative research to compare variables and their relationships.
Hypotheses are constructed based on the variables identified and as an if-then statement, following the template, ‘If a specific action is taken, then a certain outcome is expected.’ At this stage, some ideas regarding expectations from the research to be conducted must be drawn. 18 Then, the variables to be manipulated (independent) and influenced (dependent) are defined. 4 Thereafter, the hypothesis is stated and refined, and reproducible data tailored to the hypothesis are identified, collected, and analyzed. 4 The hypotheses must be testable and specific, 18 and should describe the variables and their relationships, the specific group being studied, and the predicted research outcome. 18 Hypotheses construction involves a testable proposition to be deduced from theory, and independent and dependent variables to be separated and measured separately. 3 Therefore, good hypotheses must be based on good research questions constructed at the start of a study or trial. 12
In summary, research questions are constructed after establishing the background of the study. Hypotheses are then developed based on the research questions. Thus, it is crucial to have excellent research questions to generate superior hypotheses. In turn, these would determine the research objectives and the design of the study, and ultimately, the outcome of the research. 12 Algorithms for building research questions and hypotheses are shown in Fig. 2 for quantitative research and in Fig. 3 for qualitative research.

EXAMPLES OF RESEARCH QUESTIONS FROM PUBLISHED ARTICLES
- EXAMPLE 1. Descriptive research question (quantitative research)
- - Presents research variables to be assessed (distinct phenotypes and subphenotypes)
- “BACKGROUND: Since COVID-19 was identified, its clinical and biological heterogeneity has been recognized. Identifying COVID-19 phenotypes might help guide basic, clinical, and translational research efforts.
- RESEARCH QUESTION: Does the clinical spectrum of patients with COVID-19 contain distinct phenotypes and subphenotypes? ” 19
- EXAMPLE 2. Relationship research question (quantitative research)
- - Shows interactions between dependent variable (static postural control) and independent variable (peripheral visual field loss)
- “Background: Integration of visual, vestibular, and proprioceptive sensations contributes to postural control. People with peripheral visual field loss have serious postural instability. However, the directional specificity of postural stability and sensory reweighting caused by gradual peripheral visual field loss remain unclear.
- Research question: What are the effects of peripheral visual field loss on static postural control ?” 20
- EXAMPLE 3. Comparative research question (quantitative research)
- - Clarifies the difference among groups with an outcome variable (patients enrolled in COMPERA with moderate PH or severe PH in COPD) and another group without the outcome variable (patients with idiopathic pulmonary arterial hypertension (IPAH))
- “BACKGROUND: Pulmonary hypertension (PH) in COPD is a poorly investigated clinical condition.
- RESEARCH QUESTION: Which factors determine the outcome of PH in COPD?
- STUDY DESIGN AND METHODS: We analyzed the characteristics and outcome of patients enrolled in the Comparative, Prospective Registry of Newly Initiated Therapies for Pulmonary Hypertension (COMPERA) with moderate or severe PH in COPD as defined during the 6th PH World Symposium who received medical therapy for PH and compared them with patients with idiopathic pulmonary arterial hypertension (IPAH) .” 21
- EXAMPLE 4. Exploratory research question (qualitative research)
- - Explores areas that have not been fully investigated (perspectives of families and children who receive care in clinic-based child obesity treatment) to have a deeper understanding of the research problem
- “Problem: Interventions for children with obesity lead to only modest improvements in BMI and long-term outcomes, and data are limited on the perspectives of families of children with obesity in clinic-based treatment. This scoping review seeks to answer the question: What is known about the perspectives of families and children who receive care in clinic-based child obesity treatment? This review aims to explore the scope of perspectives reported by families of children with obesity who have received individualized outpatient clinic-based obesity treatment.” 22
- EXAMPLE 5. Relationship research question (quantitative research)
- - Defines interactions between dependent variable (use of ankle strategies) and independent variable (changes in muscle tone)
- “Background: To maintain an upright standing posture against external disturbances, the human body mainly employs two types of postural control strategies: “ankle strategy” and “hip strategy.” While it has been reported that the magnitude of the disturbance alters the use of postural control strategies, it has not been elucidated how the level of muscle tone, one of the crucial parameters of bodily function, determines the use of each strategy. We have previously confirmed using forward dynamics simulations of human musculoskeletal models that an increased muscle tone promotes the use of ankle strategies. The objective of the present study was to experimentally evaluate a hypothesis: an increased muscle tone promotes the use of ankle strategies. Research question: Do changes in the muscle tone affect the use of ankle strategies ?” 23
EXAMPLES OF HYPOTHESES IN PUBLISHED ARTICLES
- EXAMPLE 1. Working hypothesis (quantitative research)
- - A hypothesis that is initially accepted for further research to produce a feasible theory
- “As fever may have benefit in shortening the duration of viral illness, it is plausible to hypothesize that the antipyretic efficacy of ibuprofen may be hindering the benefits of a fever response when taken during the early stages of COVID-19 illness .” 24
- “In conclusion, it is plausible to hypothesize that the antipyretic efficacy of ibuprofen may be hindering the benefits of a fever response . The difference in perceived safety of these agents in COVID-19 illness could be related to the more potent efficacy to reduce fever with ibuprofen compared to acetaminophen. Compelling data on the benefit of fever warrant further research and review to determine when to treat or withhold ibuprofen for early stage fever for COVID-19 and other related viral illnesses .” 24
- EXAMPLE 2. Exploratory hypothesis (qualitative research)
- - Explores particular areas deeper to clarify subjective experience and develop a formal hypothesis potentially testable in a future quantitative approach
- “We hypothesized that when thinking about a past experience of help-seeking, a self distancing prompt would cause increased help-seeking intentions and more favorable help-seeking outcome expectations .” 25
- “Conclusion
- Although a priori hypotheses were not supported, further research is warranted as results indicate the potential for using self-distancing approaches to increasing help-seeking among some people with depressive symptomatology.” 25
- EXAMPLE 3. Hypothesis-generating research to establish a framework for hypothesis testing (qualitative research)
- “We hypothesize that compassionate care is beneficial for patients (better outcomes), healthcare systems and payers (lower costs), and healthcare providers (lower burnout). ” 26
- Compassionomics is the branch of knowledge and scientific study of the effects of compassionate healthcare. Our main hypotheses are that compassionate healthcare is beneficial for (1) patients, by improving clinical outcomes, (2) healthcare systems and payers, by supporting financial sustainability, and (3) HCPs, by lowering burnout and promoting resilience and well-being. The purpose of this paper is to establish a scientific framework for testing the hypotheses above . If these hypotheses are confirmed through rigorous research, compassionomics will belong in the science of evidence-based medicine, with major implications for all healthcare domains.” 26
- EXAMPLE 4. Statistical hypothesis (quantitative research)
- - An assumption is made about the relationship among several population characteristics ( gender differences in sociodemographic and clinical characteristics of adults with ADHD ). Validity is tested by statistical experiment or analysis ( chi-square test, Students t-test, and logistic regression analysis)
- “Our research investigated gender differences in sociodemographic and clinical characteristics of adults with ADHD in a Japanese clinical sample. Due to unique Japanese cultural ideals and expectations of women's behavior that are in opposition to ADHD symptoms, we hypothesized that women with ADHD experience more difficulties and present more dysfunctions than men . We tested the following hypotheses: first, women with ADHD have more comorbidities than men with ADHD; second, women with ADHD experience more social hardships than men, such as having less full-time employment and being more likely to be divorced.” 27
- “Statistical Analysis
- ( text omitted ) Between-gender comparisons were made using the chi-squared test for categorical variables and Students t-test for continuous variables…( text omitted ). A logistic regression analysis was performed for employment status, marital status, and comorbidity to evaluate the independent effects of gender on these dependent variables.” 27
EXAMPLES OF HYPOTHESIS AS WRITTEN IN PUBLISHED ARTICLES IN RELATION TO OTHER PARTS
- EXAMPLE 1. Background, hypotheses, and aims are provided
- “Pregnant women need skilled care during pregnancy and childbirth, but that skilled care is often delayed in some countries …( text omitted ). The focused antenatal care (FANC) model of WHO recommends that nurses provide information or counseling to all pregnant women …( text omitted ). Job aids are visual support materials that provide the right kind of information using graphics and words in a simple and yet effective manner. When nurses are not highly trained or have many work details to attend to, these job aids can serve as a content reminder for the nurses and can be used for educating their patients (Jennings, Yebadokpo, Affo, & Agbogbe, 2010) ( text omitted ). Importantly, additional evidence is needed to confirm how job aids can further improve the quality of ANC counseling by health workers in maternal care …( text omitted )” 28
- “ This has led us to hypothesize that the quality of ANC counseling would be better if supported by job aids. Consequently, a better quality of ANC counseling is expected to produce higher levels of awareness concerning the danger signs of pregnancy and a more favorable impression of the caring behavior of nurses .” 28
- “This study aimed to examine the differences in the responses of pregnant women to a job aid-supported intervention during ANC visit in terms of 1) their understanding of the danger signs of pregnancy and 2) their impression of the caring behaviors of nurses to pregnant women in rural Tanzania.” 28
- EXAMPLE 2. Background, hypotheses, and aims are provided
- “We conducted a two-arm randomized controlled trial (RCT) to evaluate and compare changes in salivary cortisol and oxytocin levels of first-time pregnant women between experimental and control groups. The women in the experimental group touched and held an infant for 30 min (experimental intervention protocol), whereas those in the control group watched a DVD movie of an infant (control intervention protocol). The primary outcome was salivary cortisol level and the secondary outcome was salivary oxytocin level.” 29
- “ We hypothesize that at 30 min after touching and holding an infant, the salivary cortisol level will significantly decrease and the salivary oxytocin level will increase in the experimental group compared with the control group .” 29
- EXAMPLE 3. Background, aim, and hypothesis are provided
- “In countries where the maternal mortality ratio remains high, antenatal education to increase Birth Preparedness and Complication Readiness (BPCR) is considered one of the top priorities [1]. BPCR includes birth plans during the antenatal period, such as the birthplace, birth attendant, transportation, health facility for complications, expenses, and birth materials, as well as family coordination to achieve such birth plans. In Tanzania, although increasing, only about half of all pregnant women attend an antenatal clinic more than four times [4]. Moreover, the information provided during antenatal care (ANC) is insufficient. In the resource-poor settings, antenatal group education is a potential approach because of the limited time for individual counseling at antenatal clinics.” 30
- “This study aimed to evaluate an antenatal group education program among pregnant women and their families with respect to birth-preparedness and maternal and infant outcomes in rural villages of Tanzania.” 30
- “ The study hypothesis was if Tanzanian pregnant women and their families received a family-oriented antenatal group education, they would (1) have a higher level of BPCR, (2) attend antenatal clinic four or more times, (3) give birth in a health facility, (4) have less complications of women at birth, and (5) have less complications and deaths of infants than those who did not receive the education .” 30
Research questions and hypotheses are crucial components to any type of research, whether quantitative or qualitative. These questions should be developed at the very beginning of the study. Excellent research questions lead to superior hypotheses, which, like a compass, set the direction of research, and can often determine the successful conduct of the study. Many research studies have floundered because the development of research questions and subsequent hypotheses was not given the thought and meticulous attention needed. The development of research questions and hypotheses is an iterative process based on extensive knowledge of the literature and insightful grasp of the knowledge gap. Focused, concise, and specific research questions provide a strong foundation for constructing hypotheses which serve as formal predictions about the research outcomes. Research questions and hypotheses are crucial elements of research that should not be overlooked. They should be carefully thought of and constructed when planning research. This avoids unethical studies and poor outcomes by defining well-founded objectives that determine the design, course, and outcome of the study.
Disclosure: The authors have no potential conflicts of interest to disclose.
Author Contributions:
- Conceptualization: Barroga E, Matanguihan GJ.
- Methodology: Barroga E, Matanguihan GJ.
- Writing - original draft: Barroga E, Matanguihan GJ.
- Writing - review & editing: Barroga E, Matanguihan GJ.
We Trust in Human Precision
20,000+ Professional Language Experts Ready to Help. Expertise in a variety of Niches.
API Solutions
- API Pricing
- Cost estimate
- Customer loyalty program
- Educational Discount
- Non-Profit Discount
- Green Initiative Discount1
Value-Driven Pricing
Unmatched expertise at affordable rates tailored for your needs. Our services empower you to boost your productivity.

- Special Discounts
- Enterprise transcription solutions
- Enterprise translation solutions
- Transcription/Caption API
- AI Transcription Proofreading API
Trusted by Global Leaders
GoTranscript is the chosen service for top media organizations, universities, and Fortune 50 companies.
GoTranscript
One of the Largest Online Transcription and Translation Agencies in the World. Founded in 2005.
Speaker 1: Welcome to this overview of quantitative research methods. This tutorial will give you the big picture of quantitative research and introduce key concepts that will help you determine if quantitative methods are appropriate for your project study. First, what is educational research? Educational research is a process of scholarly inquiry designed to investigate the process of instruction and learning, the behaviors, perceptions, and attributes of students and teachers, the impact of institutional processes and policies, and all other areas of the educational process. The research design may be quantitative, qualitative, or a mixed methods design. The focus of this overview is quantitative methods. The general purpose of quantitative research is to explain, predict, investigate relationships, describe current conditions, or to examine possible impacts or influences on designated outcomes. Quantitative research differs from qualitative research in several ways. It works to achieve different goals and uses different methods and design. This table illustrates some of the key differences. Qualitative research generally uses a small sample to explore and describe experiences through the use of thick, rich descriptions of detailed data in an attempt to understand and interpret human perspectives. It is less interested in generalizing to the population as a whole. For example, when studying bullying, a qualitative researcher might learn about the experience of the victims and the experience of the bully by interviewing both bullies and victims and observing them on the playground. Quantitative studies generally use large samples to test numerical data by comparing or finding correlations among sample attributes so that the findings can be generalized to the population. If quantitative researchers were studying bullying, they might measure the effects of a bully on the victim by comparing students who are victims and students who are not victims of bullying using an attitudinal survey. In conducting quantitative research, the researcher first identifies the problem. For Ed.D. research, this problem represents a gap in practice. For Ph.D. research, this problem represents a gap in the literature. In either case, the problem needs to be of importance in the professional field. Next, the researcher establishes the purpose of the study. Why do you want to do the study, and what do you intend to accomplish? This is followed by research questions which help to focus the study. Once the study is focused, the researcher needs to review both seminal works and current peer-reviewed primary sources. Based on the research question and on a review of prior research, a hypothesis is created that predicts the relationship between the study's variables. Next, the researcher chooses a study design and methods to test the hypothesis. These choices should be informed by a review of methodological approaches used to address similar questions in prior research. Finally, appropriate analytical methods are used to analyze the data, allowing the researcher to draw conclusions and inferences about the data, and answer the research question that was originally posed. In quantitative research, research questions are typically descriptive, relational, or causal. Descriptive questions constrain the researcher to describing what currently exists. With a descriptive research question, one can examine perceptions or attitudes as well as more concrete variables such as achievement. For example, one might describe a population of learners by gathering data on their age, gender, socioeconomic status, and attributes towards their learning experiences. Relational questions examine the relationship between two or more variables. The X variable has some linear relationship to the Y variable. Causal inferences cannot be made from this type of research. For example, one could study the relationship between students' study habits and achievements. One might find that students using certain kinds of study strategies demonstrate greater learning, but one could not state conclusively that using certain study strategies will lead to or cause higher achievement. Causal questions, on the other hand, are designed to allow the researcher to draw a causal inference. A causal question seeks to determine if a treatment variable in a program had an effect on one or more outcome variables. In other words, the X variable influences the Y variable. For example, one could design a study that answered the question of whether a particular instructional approach caused students to learn more. The research question serves as a basis for posing a hypothesis, a predicted answer to the research question that incorporates operational definitions of the study's variables and is rooted in the literature. An operational definition matches a concept with a method of measurement, identifying how the concept will be quantified. For example, in a study of instructional strategies, the hypothesis might be that students of teachers who use Strategy X will exhibit greater learning than students of teachers who do not. In this study, one would need to operationalize learning by identifying a test or instrument that would measure learning. This approach allows the researcher to create a testable hypothesis. Relational and causal research relies on the creation of a null hypothesis, a version of the research hypothesis that predicts no relationship between variables or no effect of one variable on another. When writing the hypothesis for a quantitative question, the null hypothesis and the research or alternative hypothesis use parallel sentence structure. In this example, the null hypothesis states that there will be no statistical difference between groups, while the research or alternative hypothesis states that there will be a statistical difference between groups. Note also that both hypothesis statements operationalize the critical thinking skills variable by identifying the measurement instrument to be used. Once the research questions and hypotheses are solidified, the researcher must select a design that will create a situation in which the hypotheses can be tested and the research questions answered. Ideally, the research design will isolate the study's variables and control for intervening variables so that one can be certain of the relationships being tested. In educational research, however, it is extremely difficult to establish sufficient controls in the complex social settings being studied. In our example of investigating the impact of a certain instructional strategy in the classroom on student achievement, each day the teacher uses a specific instructional strategy. After school, some of the students in her class receive tutoring. Other students have parents that are very involved in their child's academic progress and provide learning experiences in the home. These students may do better because they received extra help, not because the teacher's instructional strategy is more effective. Unless the researcher can control for the intervening variable of extra help, it will be impossible to effectively test the study's hypothesis. Quantitative research designs can fall into two broad categories, experimental and quasi-experimental. Classic experimental designs are those that randomly assign subjects to either a control or treatment comparison group. The researcher can then compare the treatment group to the control group to test for an intervention's effect, known as a between-subject design. It is important to note that the control group may receive a standard treatment or may receive a treatment of any kind. Quasi-experimental designs do not randomly assign subjects to groups, but rather take advantage of existing groups. A researcher can still have a control and comparison group, but assignment to the groups is not random. The use of a control group is not required. However, the researcher may choose a design in which a single group is pre- and post-tested, known as a within-subjects design. Or a single group may receive only a post-test. Since quasi-experimental designs lack random assignment, the researcher should be aware of the threats to validity. Educational research often attempts to measure abstract variables such as attitudes, beliefs, and feelings. Surveys can capture data about these hard-to-measure variables, as well as other self-reported information such as demographic factors. A survey is an instrument used to collect verifiable information from a sample population. In quantitative research, surveys typically include questions that ask respondents to choose a rating from a scale, select one or more items from a list, or other responses that result in numerical data. Studies that use surveys or tests need to include strategies that establish the validity of the instrument used. There are many types of validity that need to be addressed. Face validity. Does the test appear at face value to measure what it is supposed to measure? Content validity. Content validity includes both item validity and sampling validity. Item validity ensures that the individual test items deal only with the subject being addressed. Sampling validity ensures that the range of item topics is appropriate to the subject being studied. For example, item validity might be high, but if all the items only deal with one aspect of the subjects, then sampling validity is low. Content validity can be established by having experts in the field review the test. Concurrent validity. Does a new test correlate with an older, established test that measures the same thing? Predictive validity. Does the test correlate with another related measure? For example, GRE tests are used at many colleges because these schools believe that a good grade on this test increases the probability that the student will do well at the college. Linear regression can establish the predictive validity of a test. Construct validity. Does the test measure the construct it is intended to measure? Establishing construct validity can be a difficult task when the constructs being measured are abstract. But it can be established by conducting a number of studies in which you test hypotheses regarding the construct, or by completing a factor analysis to ensure that you have the number of constructs that you say you have. In addition to ensuring the validity of instruments, the quantitative researcher needs to establish their reliability as well. Strategies for establishing reliability include Test retest. Correlates scores from two different administrations of the same test. Alternate forms. Correlates scores from administrations of two different forms of the same test. Split half reliability. Treats each half of one test or survey as a separate administration and correlates the results from each. Internal consistency. Uses Cronbach's coefficient alpha to calculate the average of all possible split halves. Quantitative research almost always relies on a sample that is intended to be representative of a larger population. There are two basic sampling strategies, random and non-random, and a number of specific strategies within each of these approaches. This table provides examples of each of the major strategies. The next section of this tutorial provides an overview of the procedures in conducting quantitative data analysis. There are specific procedures for conducting the data collection, preparing for and analyzing data, presenting the findings, and connecting to the body of existing research. This process ensures that the research is conducted as a systematic investigation that leads to credible results. Data comes in various sizes and shapes, and it is important to know about these so that the proper analysis can be used on the data. In 1946, S.S. Stevens first described the properties of measurement systems that allowed decisions about the type of measurement and about the attributes of objects that are preserved in numbers. These four types of data are referred to as nominal, ordinal, interval, and ratio. First, let's examine nominal data. With nominal data, there is no number value that indicates quantity. Instead, a number has been assigned to represent a certain attribute, like the number 1 to represent male and the number 2 to represent female. In other words, the number is just a label. You could also assign numbers to represent race, religion, or any other categorical information. Nominal data only denotes group membership. With ordinal data, there is again no indication of quantity. Rather, a number is assigned for ranking order. For example, satisfaction surveys often ask respondents to rank order their level of satisfaction with services or programs. The next level of measurement is interval data. With interval data, there are equal distances between two values, but there is no natural zero. A common example is the Fahrenheit temperature scale. Differences between the temperature measurements make sense, but ratios do not. For instance, 20 degrees Fahrenheit is not twice as hot as 10 degrees Fahrenheit. You can add and subtract interval level data, but they cannot be divided or multiplied. Finally, we have ratio data. Ratio is the same as interval, however ratios, means, averages, and other numerical formulas are all possible and make sense. Zero has a logical meaning, which shows the absence of, or having none of. Examples of ratio data are height, weight, speed, or any quantities based on a scale with a natural zero. In summary, nominal data can only be counted. Ordinal data can be counted and ranked. Interval data can also be added and subtracted, and ratio data can also be used in ratios and other calculations. Determining what type of data you have is one of the most important aspects of quantitative analysis. Depending on the research question, hypotheses, and research design, the researcher may choose to use descriptive and or inferential statistics to begin to analyze the data. Descriptive statistics are best illustrated when viewed through the lens of America's pastimes. Sports, weather, economy, stock market, and even our retirement portfolio are presented in a descriptive analysis. Basic terminology for descriptive statistics are terms that we are most familiar in this discipline. Frequency, mean, median, mode, range, variance, and standard deviation. Simply put, you are describing the data. Some of the most common graphic representations of data are bar graphs, pie graphs, histograms, and box and whisker graphs. Attempting to reach conclusions and make causal inferences beyond graphic representations or descriptive analyses is referred to as inferential statistics. In other words, examining the college enrollment of the past decade in a certain geographical region would assist in estimating what the enrollment for the next year might be. Frequently in education, the means of two or more groups are compared. When comparing means to assist in answering a research question, one can use a within-group, between-groups, or mixed-subject design. In a within-group design, the researcher compares measures of the same subjects across time, therefore within-group, or under different treatment conditions. This can also be referred to as a dependent-group design. The most basic example of this type of quasi-experimental design would be if a researcher conducted a pretest of a group of students, subjected them to a treatment, and then conducted a post-test. The group has been measured at different points in time. In a between-group design, subjects are assigned to one of the two or more groups. For example, Control, Treatment 1, Treatment 2. Ideally, the sampling and assignment to groups would be random, which would make this an experimental design. The researcher can then compare the means of the treatment group to the control group. When comparing two groups, the researcher can gain insight into the effects of the treatment. In a mixed-subjects design, the researcher is testing for significant differences between two or more independent groups while subjecting them to repeated measures. Choosing a statistical test to compare groups depends on the number of groups, whether the data are nominal, ordinal, or interval, and whether the data meet the assumptions for parametric tests. Nonparametric tests are typically used with nominal and ordinal data, while parametric tests use interval and ratio-level data. In addition to this, some further assumptions are made for parametric tests that the data are normally distributed in the population, that participant selection is independent, and the selection of one person does not determine the selection of another, and that the variances of the groups being compared are equal. The assumption of independent participant selection cannot be violated, but the others are more flexible. The t-test assesses whether the means of two groups are statistically different from each other. This analysis is appropriate whenever you want to compare the means of two groups, and especially appropriate as the method of analysis for a quasi-experimental design. When choosing a t-test, the assumptions are that the data are parametric. The analysis of variance, or ANOVA, assesses whether the means of more than two groups are statistically different from each other. When choosing an ANOVA, the assumptions are that the data are parametric. The chi-square test can be used when you have non-parametric data and want to compare differences between groups. The Kruskal-Wallis test can be used when there are more than two groups and the data are non-parametric. Correlation analysis is a set of statistical tests to determine whether there are linear relationships between two or more sets of variables from the same list of items or individuals, for example, achievement and performance of students. The tests provide a statistical yes or no as to whether a significant relationship or correlation exists between the variables. A correlation test consists of calculating a correlation coefficient between two variables. Again, there are parametric and non-parametric choices based on the assumptions of the data. Pearson R correlation is widely used in statistics to measure the strength of the relationship between linearly related variables. Spearman-Rank correlation is a non-parametric test that is used to measure the degree of association between two variables. Spearman-Rank correlation test does not assume any assumptions about the distribution. Spearman-Rank correlation test is used when the Pearson test gives misleading results. Often a Kendall-Taw is also included in this list of non-parametric correlation tests to examine the strength of the relationship if there are less than 20 rankings. Linear regression and correlation are similar and often confused. Sometimes your methodologist will encourage you to examine both the calculations. Calculate linear correlation if you measured both variables, x and y. Make sure to use the Pearson parametric correlation coefficient if you are certain you are not violating the test assumptions. Otherwise, choose the Spearman non-parametric correlation coefficient. If either variable has been manipulated using an intervention, do not calculate a correlation. While linear regression does indicate the nature of the relationship between two variables, like correlation, it can also be used to make predictions because one variable is considered explanatory while the other is considered a dependent variable. Establishing validity is a critical part of quantitative research. As with the nature of quantitative research, there is a defined approach or process for establishing validity. This also allows for the findings transferability. For a study to be valid, the evidence must support the interpretations of the data, the data must be accurate, and their use in drawing conclusions must be logical and appropriate. Construct validity concerns whether what you did for the program was what you wanted to do, or whether what you observed was what you wanted to observe. Construct validity concerns whether the operationalization of your variables are related to the theoretical concepts you are trying to measure. Are you actually measuring what you want to measure? Internal validity means that you have evidence that what you did in the study, i.e., the program, caused what you observed, i.e., the outcome, to happen. Conclusion validity is the degree to which conclusions drawn about relationships in the data are reasonable. External validity concerns the process of generalizing, or the degree to which the conclusions in your study would hold for other persons in other places and at other times. Establishing reliability and validity to your study is one of the most critical elements of the research process. Once you have decided to embark upon the process of conducting a quantitative study, use the following steps to get started. First, review research studies that have been conducted on your topic to determine what methods were used. Consider the strengths and weaknesses of the various data collection and analysis methods. Next, review the literature on quantitative research methods. Every aspect of your research has a body of literature associated with it. Just as you would not confine yourself to your course textbooks for your review of research on your topic, you should not limit yourself to your course texts for your review of methodological literature. Read broadly and deeply from the scholarly literature to gain expertise in quantitative research. Additional self-paced tutorials have been developed on different methodologies and techniques associated with quantitative research. Make sure that you complete all of the self-paced tutorials and review them as often as needed. You will then be prepared to complete a literature review of the specific methodologies and techniques that you will use in your study. Thank you for watching.


An official website of the United States government
Here's how you know
Official websites use .gov A .gov website belongs to an official government organization in the United States.
Secure .gov websites use HTTPS A lock ( ) or https:// means you’ve safely connected to the .gov website. Share sensitive information only on official, secure websites.
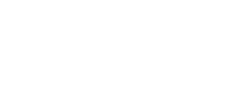
- Digg
Latest Earthquakes | Chat Share Social Media
A robust quantitative method to distinguish runoff-generated debris flows from floods
Debris flows and floods generated by rainfall runoff occur in rocky mountainous landscapes and burned steeplands. Flow type is commonly identified post-event through interpretation of depositional structures, but these may be poorly preserved or misinterpreted. Prior research indicates that discharge magnitude is commonly amplified in debris flows relative to floods due to volumetric bulking and increased frictional resistance. Here, we use this flow amplification to develop a metric (Q*) to separate debris flows from floods based on the ratio of observed peak discharge to the theoretical maximum water discharge from rainfall runoff. We compile 642 observations of floods and debris flows and demonstrate that Q* distinguishes flow type to ∼92% accuracy. Q* allows for accurate identification of debris flows through simple channel cross-section surveys rather than through qualitative interpretation of deposits, and therefore should increase the performance of models and engineered structures that require accurate flow-type observations.
Citation Information
Publication Year | 2024 |
---|---|
Title | A robust quantitative method to distinguish runoff-generated debris flows from floods |
DOI | |
Authors | David B. Cavagnaro, Scott W. McCoy, Jason W. Kean, Matthew A. Thomas, Donald N. Lindsay, Brian W. McArdell, Jacob Hirschberg |
Publication Type | Article |
Publication Subtype | Journal Article |
Series Title | Geophysical Research Letters |
Index ID | |
Record Source | |
USGS Organization | Geologic Hazards Science Center - Landslides / Earthquake Geology |
Related Content
Research hydrologist, matthew a thomas.
- Methodology
- Open access
- Published: 07 September 2024
Highly accurate and sensitive absolute quantification of bacterial strains in human fecal samples
- Fuyong Li 1 , 2 ,
- Junhong Liu 2 ,
- María X. Maldonado-Gómez 3 ,
- Steven A. Frese 4 ,
- Michael G. Gänzle 2 &
- Jens Walter 2 , 5 , 6
Microbiome volume 12 , Article number: 168 ( 2024 ) Cite this article
2 Altmetric
Metrics details
Next-generation sequencing (NGS) approaches have revolutionized gut microbiome research and can provide strain-level resolution, but these techniques have limitations in that they are only semi-quantitative, suffer from high detection limits, and generate data that is compositional. The present study aimed to systematically compare quantitative PCR (qPCR) and droplet digital PCR (ddPCR) for the absolute quantification of Limosilactobacillus reuteri strains in human fecal samples and to develop an optimized protocol for the absolute quantification of bacterial strains in fecal samples.
Using strain-specific PCR primers for L. reuteri 17938, ddPCR showed slightly better reproducibility, but qPCR was almost as reproducible and showed comparable sensitivity (limit of detection [LOD] around 10 4 cells/g feces) and linearity ( R 2 > 0.98) when kit-based DNA isolation methods were used. qPCR further had a wider dynamic range and is cheaper and faster. Based on these findings, we conclude that qPCR has advantages over ddPCR for the absolute quantification of bacterial strains in fecal samples. We provide an optimized and easy-to-follow step-by-step protocol for the design of strain-specific qPCR assays, starting from primer design from genome sequences to the calibration of the PCR system. Validation of this protocol to design PCR assays for two L. reuteri strains, PB-W1 and DSM 20016 T , resulted in a highly accurate qPCR with a detection limit in spiked fecal samples of around 10 3 cells/g feces. Applying our strain-specific qPCR assays to fecal samples collected from human subjects who received live L. reuteri PB-W1 or DSM 20016 T during a human trial demonstrated a highly accurate quantification and sensitive detection of these two strains, with a much lower LOD and a broader dynamic range compared to NGS approaches (16S rRNA gene sequencing and whole metagenome sequencing).
Conclusions
Based on our analyses, we consider qPCR with kit-based DNA extraction approaches the best approach to accurately quantify gut bacteria at the strain level in fecal samples. The provided step-by-step protocol will allow scientists to design highly sensitive strain-specific PCR systems for the accurate quantification of bacterial strains of not only L. reuteri but also other bacterial taxa in a broad range of applications and sample types.
Video Abstract
Introduction
The human gut microbiota is a complex microbial community dominated by bacteria [ 1 , 2 ] which plays an important role in host physiology and health, including the development of the immune system [ 3 ], colonization resistance against pathogens [ 4 ], nutrition utilization [ 5 ], and neural development [ 6 ]. Altered microbiota configurations (often termed dysbiosis) have been associated with not only intestinal diseases (e.g., colorectal cancer, inflammatory bowel disease) [ 7 , 8 ] but also a complex range of chronic diseases (e.g., obesity, cardiovascular disease, diabetes, allergies) [ 9 , 10 ]. Therefore, characterizing the gut microbial composition is a crucial step to explore its role in host physiology and to develop strategies aiming at microbiome modulation to improve health. As many microbial functional capacities are strain specific [ 11 ] due to strain-level genomic variations [ 12 , 13 ], determining and quantifying individual strains are essential to establish connections between a certain group of gut microorganisms and host physiological status.
The vast majority of microbiome studies rely on next-generation sequencing (NGS; e.g., 16S rRNA gene sequencing and whole metagenome sequencing [WMS]) to characterize microbial communities, which has revolutionized the field over the last two decades. WMS not only allows a community-wide analysis but also can achieve strain-level resolution [ 14 ]. However, NGS data has limitations in that it is compositional (and thus only semi-quantitative) and suffers from a limited dynamic range and low sensitivity. Many studies have implicated alterations in the absolute abundance of specific species or strains, sometimes at very low levels, in effects of host physiology [ 15 , 16 , 17 , 18 ], demonstrating the importance of quantitative and sensitive detection methods. The inclusion of quantitative methods such as quantitative PCR (qPCR) [ 19 ] and flow cytometry [ 20 ] can be used to make NGS data more quantitative, but the data remains compositional, and detection limits are high. Therefore, there is a clear need for quantitative techniques that allow the accurate and sensitive detection and absolute quantification of specific microbial species or strains, such as studies that use probiotics or live biotherapeutics, which track target microbes with lower abundance (e.g., after vertical transmission, fecal microbiota transplantation, or translocation to host tissues), as well as confirming the presence of bacterial species or strains in low-biomass samples.
qPCR has been widely used to quantify members of the gastrointestinal microbiota (e.g., Bifidobacterium longum , Bifidobacterium adolescentis , Bifidobacterium animalis subsp. lactis , Limosilactobacillus reuteri , Lacticaseibacillus casei , and Limosilactobacillus fermentum ) at the strain level [ 21 , 22 , 23 , 24 , 25 ]. However, qPCR has several limitations: (i) it is potentially affected by PCR efficiency and relies on external standards [ 26 ], and (ii) it is susceptible to inhibitors existing in the environmental or fecal samples [ 27 , 28 , 29 ]. Compared to qPCR, droplet digital PCR (ddPCR) is regarded as a more accurate and sensitive approach that does not require a calibration curve. ddPCR is based on individual amplification of targets in thousands of nanoliter-scale PCR reactions [ 30 ]. It has been applied to detect trace nucleic acid targets from clinical samples (e.g., blood and tissue) [ 30 , 31 , 32 ] and to quantify microorganisms from environmental and animal samples [ 33 , 34 , 35 ]. However, the performance of qPCR and ddPCR in terms of detection and quantification for target microorganisms from human fecal samples has not been systematically evaluated and compared. In addition, detailed and standardized protocols for strain-specific PCR primer design and accompanying validation workflows that are easily applicable have not been published to date.
The overall objective of this study was to design an optimized PCR-based approach for the quantitative detection of bacterial strains in human fecal samples in terms of sensitivity (limit of detection [LOD]), accuracy, reproducibility, time, and cost. To achieve this, we systematically compared qPCR and ddPCR in combination with three well-established DNA extraction methods for the strain-specific quantification of an L. reuteri strain in human fecal samples. Based on these comparisons and information from previous studies [ 21 , 22 , 23 ], we developed an easy-to-follow, step-by-step protocol for strain-specific qPCR assays that includes the identification of strain-specific marker genes and designing and validating the primers. We applied this protocol in designing strain-specific qPCR assays for two L. reuteri strains, PB-W1 and DSM 20016 T , and validated the PCR assays using spiked fecal samples as well as samples collected from human subjects who received live L. reuteri PB-W1 or DSM 20016 T as a part of a human trial, allowing direct comparisons between qPCR and NGS approaches.
Materials and methods
Growth conditions of bacteria.
L. reuteri strains were grown on MRS agar plates (BD Difco Microbiology, Houston, TX, USA) for 48 h in an anaerobic chamber at 37 °C. Single colonies were picked and transferred to MRS broth (BD Difco Microbiology) and subcultured twice (24 h for the first subculture and 8 h for the second subculture to ensure bacterial cells are in the late exponential phase or early stationary phase and therefore highly active and alive) [ 36 , 37 ]. Bacteria were harvested and used to spike human fecal samples (see the section below). Cell numbers in 8-h cultures were determined by quantitative plating on MRS agar plates.
Fecal samples spiked with L. reuteri DSM 17938
Human fecal samples were collected from nine healthy individuals at the University of Alberta Human Nutrition Research Unit (Edmonton, Canada). All procedures were approved by the Health Research Ethics Board — Biomedical Panel of the University of Alberta (protocol no. Pro00077565). The absence of L. reuteri DSM 17938 was confirmed using qPCR as described previously [ 38 ], and all samples were L. reuteri DSM 17938 negative (Table S 1 ). To evaluate and compare the performance of qPCR and ddPCR, three L. reuteri -negative fecal samples were selected, and aliquots of each sample were spiked with known quantities of L. reuteri DSM 17938: serial dilutions of the 8-h subcultured L. reuteri DSM 17938 with cell numbers were prepared with ice-cold phosphate buffered saline (PBS: NaCl 8 g, KCl 0.2 g, Na 2 HPO 4 1.44 g, KH 2 PO 4 0.24 g, Milli-Q Water 1 L, pH 7.0), resulting in fecal aliquots with L. reuteri DSM 17938 of 9.3 × 10 7 , 9.3 × 10 6 , 9.3 × 10 5 , 9.3 × 10 4 , 4.7 × 10 4 , 2.3 × 10 4 , 1.2 × 10 4 , and 5.9 × 10 3 cells/g (Fig. S 1 ). These spiked aliquots were stored at − 80 °C until DNA isolation.
DNA extraction from fecal samples
Three well-established protocols for isolating total DNA from human fecal samples were tested and compared in this study: a phenol–chloroform-based method [ 39 ], a modified method based on the QIAamp Fast DNA Stool Mini Kit (Qiagen, Valencia, CA, USA) [ 22 , 40 ], and an optimized kit-based method based on the protocol Q described previously [ 41 ]. The purity of DNA was determined spectrophotometrically. Detailed procedures of these DNA isolation methods, including recipes for solutions and equipment used, are provided in Supplementary File 2.
Phenol–chloroform-based method (PC)
One gram of stool sample was weighted and diluted tenfold in ice-cold PBS buffer. Samples were vortexed vigorously, and 1 ml of the solution (i.e., 0.1 g of raw sample) was centrifuged (8000 × g for 5 min at 4 °C) and washed for three times with ice-cold PBS buffer. After centrifugation, cell pellets were resuspended in 750-µl lysis buffer and incubated at 37 °C for 20 min. After 85 µl of 10% SDS solution and 30-µl proteinase K (20 mg/ml) were added, the mixtures were incubated at 60 °C for another 30 min. Then 500 µl of phenol–chloroform-isoamyl alcohol (25:24:1) was added, and the DNA isolation was conducted following the procedures as described before [ 39 ].
QIAamp fast DNA stool mini kit-based method (QK)
This method was adapted from our previous publications [ 22 , 40 ] with minor modifications. Fecal samples were washed with ice-cold PBS as described above for the method PC. Cell pellets were resuspended in 100 µl of lysis buffer and incubated at 37 °C for 30 min. Then 1 ml of buffer InhibitEX was added, and samples were homogenized thoroughly by vortexing and bead beating. After that, DNA was extracted with the use of QIAamp Fast DNA Stool Mini Kit (Qiagen).
Protocol Q-based method (PQ)
We followed the procedures of protocol Q developed previously [ 41 ] but further optimized it through adding two pre-treatment steps. Specifically, prior to conducting the original protocol Q, fecal samples were washed in ice-cold PBS as described for the method PC and incubated with 100 µl of lysis buffer at 37 °C for 30 min.
Quantification of L. reuteri DSM 17938 in fecal samples using ddPCR
Cell numbers of L. reuteri DSM 17938 were determined for the spiked fecal aliquots using the strain-specific primers developed previously [ 38 ]. This primer pair (1694f: 5′-TTAAGGATGCAAACCCGAAC-3′ and 1694r: 5′-CCTTGTCACCTGGAACCACT-3′) targets a chromosome-located surface protein gene that has a single copy on the genome, and the length of the target region is 177 bp [ 38 ]. ddPCR was performed using EvaGreen intercalating DNA dye to detect positive droplets. Each ddPCR reaction contained 1 μl of DNA (given the high original concentration, DNA extracted using PC and PQ was treated with a tenfold and threefold dilution, respectively), 12.5 μl of 2 × EvaGreen Supermix (Bio-Rad Laboratories Inc., Hercules, CA, USA), 200-nM primer each, and ddH 2 O to bring the per-reaction volume to 25 μl in each well of a 96-well plate. The plate was put into a QX200 Auto Droplet Generator (Bio-Rad Laboratories Inc.), and EvaGreen droplet generation oil (Bio-Rad Laboratories Inc.) was added according to the manufacturer’s manual. PCR reactions were conducted in a Bio-Rad C1000 Touch Thermal Cycler (Bio-Rad Laboratories Inc.) as follows: (Step 1) 95 °C for 5 min, (Step 2) 95 °C for 30 s, (Step 3) 62 °C for 1 min, (Step 4) repeat steps 2 and 3 for 39 cycles, (Step 5) 4 °C for 5 min, (Step 6) 90 °C for 5 min, and (Step 7) hold at 4 °C. After the reaction, the plate was placed in the block of a Bio-Rad QX200 Droplet Reader (Bio-Rad Laboratories Inc.). Droplets were read one at a time, and data were analyzed using QuantaSoft Analysis Pro 1.0 (Bio-Rad Laboratories Inc.).
Quantification of L. reuteri DSM 17938 in fecal samples using qPCR
L. reuteri DSM 17938 was quantified by qPCR in the same samples and with the same primers as used for ddPCR [ 38 ]. The standard curve was constructed based on serial dilutions of L. reuteri DSM 17938 genomic DNA. The original copy number of the standard material was calculated based on the DNA concentration determined spectrophotometrically using a NanoDrop (Thermo Fisher Scientific, Waltham, MA, USA). This generated a standard curve ranging from 4 × 10 5 to 4 gene copies per microliter (µl), which covered a dynamic range of 4 × 10 8 to 4 × 10 3 copy numbers of L. reuteri per gram of fecal samples and showed excellent linearity ( R 2 > 0.99) and acceptable efficiency ( E = 89%; Fig. S 2 ). The 20 µl of PCR mixture consisted of 1 µl of DNA, 10 µl of SensiFAST SYBR Hi-ROX Mix (FroggaBio, Vaughan, ON, Canada), 0.6 µl of each primer (10 µM), and 8.4 µl of nuclease-free water. The PCR program was composed of an initial denaturation step for 3 min at 95 °C, followed by 40 cycles of denaturation for 5 s at 95 °C, annealing and extension for 30 s at 62 °C, and fluorescent signal acquisition. PCR was performed using a HT 7900 machine (Thermo Fisher Scientific). After 40 cycles of PCR amplification, melting curves were evaluated to verify the correct product. The reaction program for melting curve analysis included a denaturation for 15 s at 95 °C, lowered to 60 °C for 1 min, and increased to 95 °C for 15 s with continuous fluorescence readings. The melting curve analysis showed one specific peak at around 87.5 °C (Fig. S 3 ), confirming the good quality of the amplification, and there is nonspecific amplification or primer dimers.
Comparison between ddPCR and qPCR
For both approaches, data for gene copies per reaction were transformed to absolute copy numbers per gram feces following the formula ( C × V T )/( V U × M ), where C is gene copies measured per reaction, V T is the elution volume of extracted DNA, V U is the volume of DNA used, and M is the amount of fecal sample used in DNA extraction. To systematically compare the two assays, parameters representing reproducibility, linearity, sensitivity, and accuracy, as well as the required time and costs, were evaluated. Specifically, the coefficient of variation (CV; the ratio between the standard deviation and the mean of three samples at each spiking concentration) was calculated to determine the reproducibility. Linearity measures how well the copy numbers evaluated by PCR assays related to the actual spiked cell series, which was assessed by calculating the R 2 of the linear regression model with spiked cell numbers as the dependent variable and copy numbers of PCR as the independent variable. To evaluate the sensitivity, the limit of detection (LOD; i.e., the lowest cell number at which target bacteria can differentially detected over the background noise) was determined. More specifically, the LOD was defined as the lowest amount of spiked cells that could be detected in more than 95% of replicates ( n = 9 for each spiked concentration, including both technical and experimental replicates in this study) [ 42 ]. For qPCR, as we observed the noise amplification from background (blank fecal samples without spiked L. reuteri ) after 35 cycles, a threshold Cq value > 35 was considered undetected. For ddPCR, single well thresholding was used to distinguish positive and negative reactions through grouping droplets based on software’s default setting. The accuracy of the two PCR assays was evaluated by determining the recovery rate, calculated as the ratio between the copy numbers per gram of feces measured from PCR and the cell numbers spiked [ 43 ]. Costs for both assays were compared on the basis of being performed on 96-well plates. Each 96-well plate accommodates 25 samples (in triplicate, excluding 6 serial dilutions as standards and 1 non-template-negative control) for qPCR and 30 samples (in triplicate, excluding 1 positive control and 1 negative control) for ddPCR. Cost for labor was not considered in this estimation.
Design and in silico evaluation of strain-specific primers
Strain-specific primers were designed based on gene sequences identified to be unique to particular strains using the Single Genes tool in IMG/MER phylogenetic profiler function from Joint Genome Institute (JGI; https://jgi.doe.gov/ ). The general workflow for developing and evaluating the strain-specific primers in silico is summarized in Fig. 1 , and the in-detail protocol is summarized in Supplementary File 3. Validation of this protocol was performed using two L. reuteri strains, PB-W1 and DSM 20016 T . L. reuteri strain PB-W1 was isolated from a fecal sample of a rural Papua New Guinean [ 44 ]. DSM 20016 T is the type strain of L. reuteri that clusters in the phylogenetic lineage II of the species [ 13 , 45 , 46 ], which was recently described as subspecies L. reuteri subsp. reuteri [ 47 ]. Due to the high genetic homogeneity of lineage II [ 46 ], strain-specific genes could not be identified for the strains within this lineage, and thus, lineage-specific primers were designed. As the prevalence of L. reuteri in the human gastrointestinal tract in industrialized societies is low [ 46 , 48 , 49 ], lineage-specific primers were considered specific enough for the detection of DSM 20016 T in human fecal samples. Primer sets were designed by the Primer3Plus program [ 50 ]. The quality of primers was evaluated in silico using OligoAnalyzer (Integrated DNA Technologies, Coralville, IA, USA). The specificities of designed primers were verified in silico using Primer BLAST. Sequences and amplicon size of the primers that resulted from this process are listed in Table 1 .
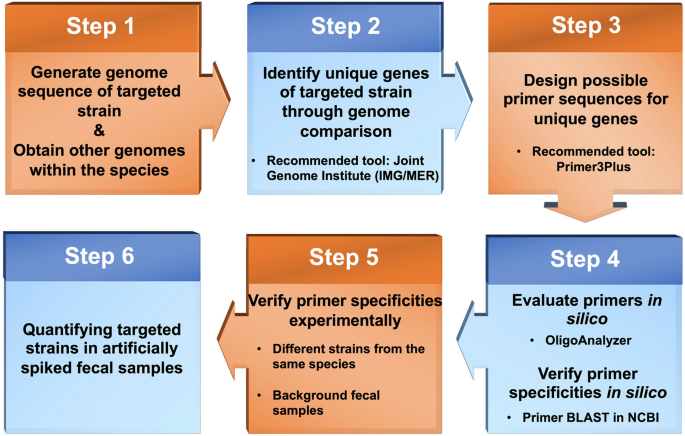
A flow chart of main steps for designing and validating strain-specific primers
Experimental validation of strain-specific qPCR systems
The specificity of primer pairs was established by qPCR with genomic DNA from each of 19 human-origin L. reuteri strains (Table 2 ) and then further assessed against the background DNA of the nine fecal samples (Table S 1 ). The absence of L. reuteri in these fecal samples was confirmed by plating on L. reuteri selective isolation medium (LRIM), as described previously [ 12 ]. To evaluate the performance of primers in quantifying L. reuteri from human stools, three fecal samples without detectable L. reuteri were selected and spiked with PB-W1 and DSM 20016 T , respectively, following the same procedure as described for DSM 17938 (Fig. S 1 ) with specific bacterial concentrations (2.2 × 10 8 , 2.2 × 10 7 , 2.2 × 10 6 , 2.2 × 10 5 , 8.9 × 10 4 , 3.5 × 10 4 , 1.4 × 10 4 , 5.6 × 10 3 , 2.2 × 10 3 , 9.1 × 10 2 cells/g feces for PB-W1 and 1.0 × 10 8 , 1.0 × 10 7 , 1.0 × 10 6 , 1.0 × 10 5 , 1.0 × 10 4 , 4.1 × 10 3 , 1.6 × 10 3 , 6.5 × 10 2 cells/g feces for DSM 20016 T ). After extracting DNA using the method PQ, cell numbers of L. reuteri PB-W1 and DSM 20016 T were determined using qPCR with the respective primer pairs. The 20 µl of PCR mixture consisted of 1 µl of DNA, 10 µl of SensiFAST SYBR Hi-ROX Mix (FroggaBio), 0.8 µl of each primer (at a concentration of 0.8 μM), and 8.2 µl of nuclease-free water. The PCR cycling was composed of an initial denaturation for 3 min at 95 °C, followed by 40 cycles of denaturation at 95 °C for 5 s, annealing at 63 °C for 10 s, and extension at 72 °C for 15 s. Standard curves for L. reuteri PB-W1 and DSM 20016 T were generated with serial dilutions of genomic DNA of PB-W1 and DSM 20016 T from standardized cultures from which cell numbers were determined by quantitative plating.
Validation of strain-specific qPCR assays in human subjects who received live L. reuteri
To further validate the specificity and accuracy of our strain-specific qPCR assays, we conducted an analysis using a subset of fecal samples obtained from a recently completed human clinical trial (ClinicalTrials.gov Identifier: NCT03501082). These samples were collected at seven different time points over a period of 21 days during the control phase with no dietary intervention, including baseline and days 4, 6, 8, 12, 16, and 21. On day 4 of the trial, a single dose of approximately 2.25 × 10 10 viable cells of either L. reuteri strain PB-W1 or DSM 20016 T was administrated to 8 and 11 participants, respectively. The respective strain-specific qPCR assay was used in the two groups as described above; selective culture and NGS approaches (i.e., 16S rRNA gene sequencing and whole metagenome sequencing [WMS]) were also applied.
Microbial genomic DNA of each fecal sample was extracted using method QK, and the absolute abundance of PB-W1 and DSM 20016 T was estimated as described above. To determine the viable cells of L. reuteri in the fecal samples, we performed quantitative culture on the LRIM under the anaerobic condition at 45 °C for 48 h, which has been validated to be sufficiently specific for the quantification of L. reuteri [ 12 ]. 16S rRNA gene sequencing was performed for all the fecal samples, targeting the V5–V6 region using the primer pair 784F (5′-RGGATTAGATACCC-3′) and 1064R (5′-CGACRRCCATGCANCACCT-3′). This protocol has been shown to efficiently detect L. reuteri in human fecal samples, with sufficient taxonomic resolution in the V5–V6 region [ 48 ]. Amplicons were sequenced on the Illumina MiSeq PE300 platform (2 × 300 bp paired-end sequencing) at the University of Minnesota Genomics Center (Minneapolis, MN, USA). Baseline and day 8 (4 days after probiotic administration) samples were subjected to WMS using the Illumina NovaSeq6000 S4 PE150 platform (2 × 150 bp paired-end sequencing) at the Génome Québec Innovation Centre (Montréal, QC, Canada). The relative abundance of L. reuteri at the species level in the 16S rRNA gene sequencing and WMS datasets was determined using QIIME2 (based on the SILVA database, version 138) [ 51 ] and MetaPhlAn 4.0 [ 52 ], respectively. After denoising, merging paired-end reads, and eliminating chimeras, an average of 31,060 ± 1316 (mean ± SEM) 16S rRNA gene reads per sample were retained for subsequent analysis. For the WMS dataset, an average of 30.2 ± 2.0 (mean ± SEM) million read pairs per sample remained for our analysis after quality control, removing sequences identified as bacteriophage phiX174, and filtering out human DNA reads.
Statistical analysis
All the statistical analyses (e.g., descriptive statistics, R 2 , and linear regression) of this study were conducted using R 3.4.2 [ 53 ].
Comparison of three DNA extraction methods
The DNA concentrations varied substantially among three protocols. PC generated the highest DNA concentration (1525 ± 290 ng/μl; mean ± SD), followed by PQ (694 ± 76 ng/μl) and QK (259 ± 41 ng/μl) (Table S 2 ). All DNA solutions showed adequate A 260/280 nm values (range, 1.95–2.12), with PQ slightly higher, indicating a more efficient removal of protein from DNA (Table S 2 ). Meanwhile, A 260/230 nm value, indicating contaminations of phenol, carbohydrate, or humic acid, showed excellent values for the kit-based DNA isolation methods (2.10 ± 0.15 for PQ and 1.99 ± 0.15 for QK), while PC did not perform well (1.74 ± 0.16) (Table S 2 ). Overall, although all three DNA isolation protocols produced nucleic acids with acceptable quantity for downstream PCR-based methods, DNA isolated with the kit-based protocols (QK and PQ) showed higher quality.
Comparison between qPCR and ddPCR
Performance of qPCR and ddPCR in detecting and quantifying L. reuteri strain DSM 17938 in fecal samples, in aspects of reproducibility, linearity, sensitivity, and accuracy, was evaluated and compared based on DNA isolated from human stools of three subjects spiked with established cell numbers of DSM 17938.
Reproducibility
The coefficient of variation (CV) among three aliquots at each spiking concentration was calculated as a measure of the reproducibility, and a CV ≤ 25% has been suggested previously as the threshold of acceptable reproducibility [ 54 ]. ddPCR had a higher reproducibility as its CV values were consistently lower than qPCR for DNA isolation methods QK and PC (QK: 0.30–5.91% of ddPCR vs. 1.77–5.61% of qPCR; PC: 0.59–3.01% of ddPCR vs. 1.58–6.85% of qPCR; Table 3 ), which is consistent with the literature [ 55 , 56 , 57 ]. However, when the DNA isolation method PQ was applied, CVs were comparable between ddPCR and qPCR (range of CV 1.07–5.41% for ddPCR vs. range of CV 0.60–3.67% for qPCR) (Table 3 ). With lower cell numbers of L. reuteri spiked into the stool aliquots, the CV values of ddPCR and qPCR increased, meaning their precision decreased. However, CV values among three fecal aliquots at each spiking cell density of L. reuteri were all lower than 25%, demonstrating a high reproducibility across the tested dynamic range for both ddPCR and qPCR.
Linearity is a measure of how well the copy numbers of the target strain estimated by PCR relate to the actual spiked cell serial dilution. Both qPCR ( R 2 = 0.9801 and 0.9927 for QK and PQ, respectively) and ddPCR ( R 2 = 0.9926 and 0.9906 for QK and PQ, respectively) exhibited high linearities based on kit-based DNA isolation approaches (QK and PQ) (Fig. 2 ), which are all above the acceptable R 2 threshold of 0.98 [ 58 ]. This indicates that both qPCR and ddPCR accurately quantify L. reuteri from fecal samples if kit-based DNA isolation protocols are chosen. We also examined the consistency between qPCR and ddPCR based on the DNA isolated using PQ and confirmed that these two PCR techniques had a high level of agreement with each other ( y = 1.0607x–0.4746, R 2 = 0.9913; Fig. S4). The linearities of qPCR and ddPCR were both declined when PC was used ( R 2 = 0.95 and 0.97 for qPCR and ddPCR, respectively), which are lower than the acceptable R 2 threshold of 0.98.
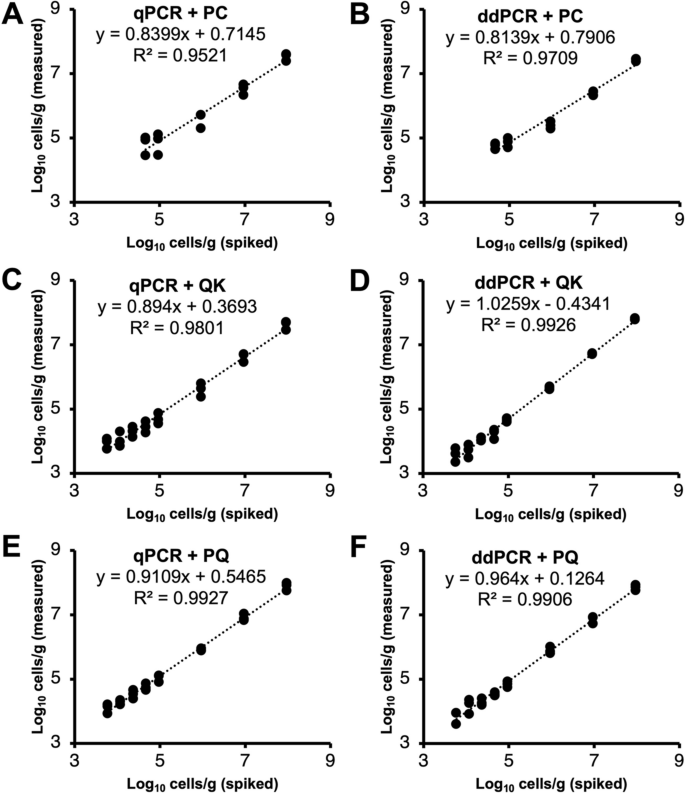
Relationships between actual spiked cells/g of L. reuteri DSM 17938 and measured cells/g from qPCR and ddPCR assays, based on three different DNA extraction methods. Phenol–chloroform-based method (PC) combined with qPCR ( A ) and ddPCR ( B ). QIAamp fast stool DNA kit-based method (QK) combined with qPCR ( C ) and ddPCR ( D ). Protocol Q-based method combined with qPCR ( E ) and ddPCR ( F ). Spiked cells/g of L. reuteri DSM 17938 was estimated from quantitative culture on the MRS plate. Each bacterial concentration was conducted in biological triplicates
Sensitivity
The limit of detection (LOD) was determined to reflect the sensitivity of qPCR and ddPCR. By using the DNA extraction methods QK and PQ, L. reuteri DSM 17938 could still be detected at the input of 3.77 Log 10 cells/g feces in both qPCR and ddPCR analyses, with no differences between the two methods (Table 3 ). LOD of both PCR approaches was 4.67 Log 10 cells/g feces when the method PC was applied (Table 3 ). Therefore, the LOD were equal for both qPCR and ddPCR (Table 3 & Fig. 2 ), while the sensitivity mainly depended on the DNA isolation approach. Better performance of detection and quantification was observed when methods QK and PQ were used comparing with PC. Given that DNA isolated with PC performed consistently poorer in terms of linearity and sensitivity in both ddPCR and qPCR, this method was excluded from further analyses.
The accuracy of qPCR and ddPCR was evaluated by determining the recovery rate, which is defined as the percentage of cells detected out of the theoretical spiking concentration [ 43 ]. PQ resulted in higher accuracy for both qPCR and ddPCR compared to QK (Fig. 3 ), indicating a higher efficiency of PQ in the harvest of DNA from L. reuteri . When the sample contained a high density of L. reuteri (4.97–7.97 Log 10 cells/g), ddPCR and qPCR showed comparable accuracy, regardless of PQ or QK was used (Fig. 3 ). However, when L. reuteri was spiked at a low cell density (from 3.77 to 4.37 Log 10 cells/g), the choice of DNA isolation approach and PCR assay affected the accuracy of quantification. Specifically, within this L. reuteri spiking range, qPCR had a higher accuracy than ddPCR when QK was applied to isolate DNA, while both qPCR and ddPCR overestimated the cell numbers of L. reuteri when PQ was used (Fig. 3 ). Nevertheless, the discrepancy caused by this overestimation was < 0.34 Log 10 cells/g, and therefore, this bias may be negligible when quantifying gut bacteria in fecal samples.
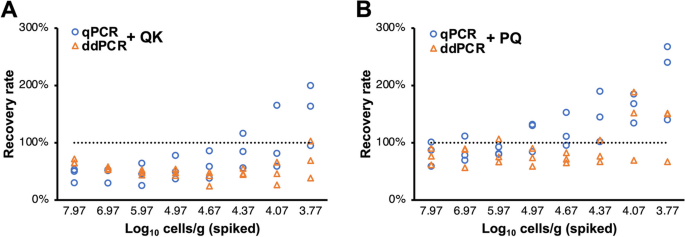
The accuracy (represented by the recovery rate) of qPCR and ddPCR. DNA extracted from fecal samples (biological triplicates) spiked with L. reuteri DSM 17938 using QK ( A ) and PQ ( B ) were analyzed by qPCR and ddPCR. The recovery rate is defined as the ratio between the cell numbers per gram feces measured and the actual cell numbers spiked, as suggested previously [ 43 ]. Results were compared with 100% recovery which is indicated by the dotted line. QK, QIAamp Fast Stool DNA Kit-based method and PQ, protocol Q-based method
Assuming that all samples, standard materials, and controls are run in triplicate, as shown in Table 4 , the cost per sample for ddPCR was almost four times as high as that for qPCR (CAD $9.6 vs. CAD $2.5). The time spent conducting ddPCR (6.5 h) was also higher when compared to qPCR (2.5 h). Therefore, when additionally considering labor cost, the costs of ddPCR become much higher than qPCR.
Our systematic comparison of qPCR and ddPCR showed that both approaches, combined with kit-based DNA extraction methods (PQ and QK), allowed a highly reproducible, accurate, and sensitive quantification of a bacterial strain ( L. reuteri DSM 17938) in human fecal samples. ddPCR is slightly better in terms of reproducibility, but the performance of qPCR is comparable when appropriate DNA isolation methods were used and is much cheaper and faster. Therefore, after the outcomes mentioned above are all considered, we recommend the combination of the DNA extraction method PQ and qPCR as the optimal strategy to quantify L. reuteri in human stool, although method QK is almost as good and less time-consuming when compared to PQ.
A step-by-step protocol for highly accurate and sensitive quantification of bacterial strains in human fecal samples
Based on the findings obtained above and our previous studies that used qPCR [ 21 , 22 , 23 ], we developed a step-by-step protocol for the design of qPCR assays for the accurate quantification of bacterial strains in human fecal samples. The protocol starts from the design of strain-specific primers, followed by the in silico and experimental validation of the primer specificity. The protocol is available as Supplementary File 3.
Validation of the protocol of strain-specific qPCR assays
The protocol was validated using PCR assays developed for two L. reuteri strains (PB-W1 and DSM 20016 T ):
Design of strain-specific primers
Genome sequences of 31 L. reuteri strains available in public databases, representing six reported phylogenetic lineages, were obtained from JGI genome portal (Table S 3 ). Genome comparisons using the IMG/MER phylogenetic profiler function from JGI identified 96 genes that were unique in PB-W1, and 86 genes were specific to lineage II that contains DSM 20016 T . The competence factor transport accessory protein ( ComB ) gene (IMG gene ID 2880791457) was selected for PB-W1, and a gene encoding a transposase-like protein (IMG gene ID 2760858907) was selected for DSM 20016 T , as the basis for primer design, respectively. Primers were designed for each of these two genes using Primer3Plus [ 50 ], and primer pairs PLD-F + PLD-R (for PB-W1) and DHP-F + DHP-R (for DSM 20016 T ) were chosen (Table 1 ).
In silico evaluation of strain-specific primers
The technical characteristics of these two primer pairs were evaluated using OligoAnalyzer. The melting temperatures of hairpin structures were determined at 48.8 °C, 45.3 °C, 25.2 °C, and 37.2 °C, respectively, which were much lower than the annealing temperature (63 °C), making hairpins unlikely. The self-dimer and hetero-dimer analyses revealed that delta G values of these two primer pairs (> − 6.34 kcal/mole and − 6.53 kcal/mole, respectively) were higher than the OligoAnalyzer recommended threshold of − 9 kcal/mole for forming dimers, indicating that the designed primers are not likely to induce primer dimers. BLAST analysis against the entire NCBI NR database resulted in no hits from microbial genomes for primers PLD-F and PLD-R (primers for PB-W1), while hits for DHP-F and DHP-R (primers for DSM 20016 T ) were only from strains belonging to lineage II of L. reuteri . Thus, in silico analysis indicated sufficient specificity for both primer pairs.
Experimental validation of primer specificity in vitro
The specificity of designed primer sets was tested using qPCR against genomic DNA of the 2 target strains and 19 L. reuteri strains of human origin (Table 2 ). Most strains other than those for which the primers were designed showed no amplification (Table 2 ). The primer set for PB-W1 was highly specific for PB-W1 (Cq of qPCR = 10), while the Cq for all other strains were over 35. The primer set for DSM 20016 T amplified the genomic DNA of this strain with a Cq of 9.5; other strains of lineage II were not tested as they are identical at this target site, while strains not belonging to lineage II all showed Cq over 33. The difference of Cq between target and non-target strains was therefore at least 25 and 23.5 cycles for PB-W1 and DSM 20016 T , respectively (Table 2 ), which relates to a cell number difference of more than 10 7 cells/g feces. Therefore, with the same amount of DNA, non-target strains would only have a negligible impact on the quantification of target strains.
The primer specificity was further validated using qPCR against complex microbial communities of nine human fecal samples (Table S 1 ). No positive amplification was observed with the primer set for PB-W1, while three fecal samples showed positive readings when the primer set for DSM 20016 T was tested. For these three DSM 20016 T positive samples, two of them were merely present at low densities (3.90 and 4.15 Log 10 cells/g feces). The only stool sample showing high numbers (5.53 Log 10 cells/g feces) (Table S 1 ) contained culturable L. reuteri which could explain the positive PCR reading. Therefore, we concluded that our designed primer sets for PB-W1 and DSM 20016 T showed high specificity to detect and quantify target L. reuteri strains in fecal samples.
Evaluation of strain-specific qPCR assays using spiked fecal samples
The qPCR assays were evaluated with three human fecal samples negative for L. reuteri that were then spiked with known cell numbers of either PB-W1 (2.96 to 8.34 Log 10 cells/g feces) or DSM 20016 T (2.81 to 8.01 Log 10 cells/g feces), and DNA isolation was based on method PQ. The qPCR amplification efficiency was 94.2% for the PB-W1 assay and 93.7% for the DSM 20016 T assay, both laying in the recommended efficiency range of 90–110% [ 58 ]. The LOD was 2.96 and 2.81 Log 10 cells/g feces for the PB-W1 and DSM 20016 T assays, respectively (Table 5 & Fig. 4 ), thus outperforming the qPCR assay for L. reuteri DSM 17938 described above. Both qPCR assays showed remarkably high reproducibility (CV of PB-W1 assay ranged from 0.69 to 3.97%, and CV of DSM 20016 T assay ranged from 0.54 to 7.17%) and high linearities between the numbers of cells spiked and the copy numbers measured by qPCR ( R 2 > 99%; Table 5 & Fig. 4 ). To evaluate the accuracy of the qPCR assays, we compared the discrepancy between the copy numbers estimated by qPCR and the actual cell numbers spiked. The differences ranged from − 0.30 to − 0.15 Log 10 cells/g (equal to 50.1–70.8% of recovery rate) for PB-W1 and from − 0.51 to 0.15 Log 10 cells/g (equal to 30.9–141.3% of recovery rate) (Table 5 ), which is negligible when quantifying gut bacteria in human stools.
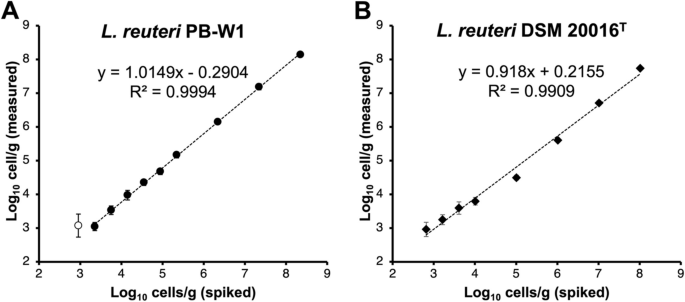
Linearity between the number of L. reuteri cells spiked to stool samples and the cell number measured using our designed qPCR assays for PB-W1 ( A ) and DSM 20016 T ( B ). The regression line was created between the spiking of 3.35 and 8.34 Log 10 cells/g feces for PB-W1 and between 2.81 and 8.01 Log 10 cells/g feces for DSM 20016 T , respectively, within which detection was reliable. As amplification products were still detectable but lacked reproducibility and linearity with an input of PB-W1 (2.96 Log 10 cells/g feces), it was shown in open round to highlight the difference. Each error bar displays the standard deviation (SD) from three replicates
Evaluation of strain-specific qPCR assays in fecal samples collected during a human trial
To validate our qPCR assays in a real-world setting and compare findings with those obtained with NGS approaches (i.e., 16S rRNA gene sequencing and whole metagenome sequencing [WMS]), we capitalized on fecal samples collected during the control phase (no dietary intervention) of a human trial that involved the administration of a single dose of L. reuteri PB-W1 or DSM 20016 T to different groups of individuals. Fecal samples before (baseline) and after the administration of the strains were included.
We first compared findings of qPCR, culture, 16S rRNA gene sequencing, and WMS at baseline and 4 days after administration of the live L. reuteri cells (Fig. 5 A), as we had data of all four approaches available at these time points. All baseline samples except one were negative in qPCR assays, with the one positive sample for PB-W1 being just slightly about the detection limit (3.46 Log 10 cells/g). Four days after administration of the strains, L. reuteri becomes detectable in all subjects by qPCR and culture, with a broad dynamic range ranging from 3.35 to 7.74 Log 10 cells/g feces for qPCR and 3.00 to 6.56 Log 10 cells/g feces for culture. In contrast, L. reuteri was only detectable, at very low relative abundance, by 16S rRNA gene sequencing and WMG in one sample per group (Fig. 5 A).
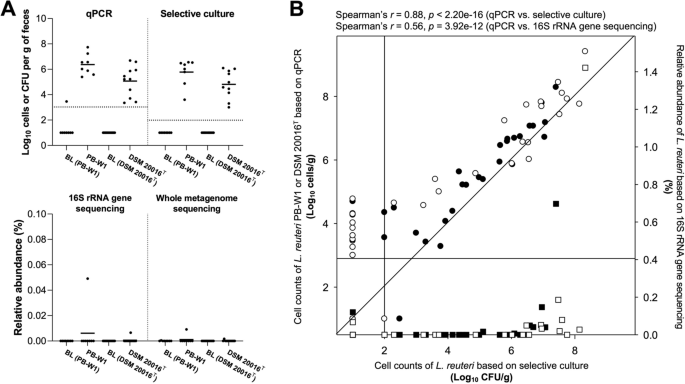
A Quantification of L. reuteri in human fecal samples collected at baseline (BL; prior to L. reuteri administration) and after treatment (4 days after receiving a single dose of L. reuteri PB-W1 or DSM 20016 T ). Quantification was performed using strain-specific qPCR, selective culture, 16S rRNA gene sequencing, and whole metagenome sequencing (WMS). The horizontal dotted lines denote the detection limits of qPCR and selective culture, at around three and two Log 10 cells/g feces, respectively. Values below the detection limit were plotted with a numerical value of one. B Parity plot comparing viable cell counts of L. reuteri matching the respective inocula in individuals receiving PB-W1 (open symbols) or DSM 20016 T (black symbols) to the absolute abundance of the two strains as determined by qPCR (circles) or to relative abundance of L. reuteri determined by 16S rRNA gene sequencing (squares). The diagonal line denotes unity, while the horizontal line denotes the detection limit of qPCR at around three Log 10 cells/g and the vertical line denotes the detection limit of selective culture at two Log 10 cells/g feces. The detection limit of 16S rRNA gene sequencing is not shown, as it differs from sample to sample depending on the total cell count and the number of reads per sample. The symbols represent samples obtained from 19 individuals who received either L. reuteri PB-W1 ( n = 8) or DSM 20016 T ( n = 11)
We then characterized findings from qPCR, culture, and 16S rRNA gene sequencing for all samples from baseline to the end of the monitoring period (day 21). Cell numbers of L. reuteri ranged from 9.41 Log 10 cells/g to below the detection limit of 3 Log 10 cells/g for qPCR and from 8.33 Log 10 cells/g to below the detection limit of 2 Log 10 cells/g based on selective culture. Correlation between qPCR and selective culture data revealed a strong correlation (Spearman’s r = 0.88, p < 2.20e-16; Fig. 5 B), although qPCR showed consistently higher cell numbers (Fig. 5 B), likely due to qPCR detecting DNA from inactive and/or dead cells that are not cultivable. The relative abundance of L. reuteri , as determined by 16S rRNA gene sequencing, ranged from 0 to 1.42%, with most of the samples, especially those with cell numbers of < 10 6 as per qPCR, showing no detectable L. reuteri .
In this study, we systematically compared the performance of qPCR and ddPCR in combination with three DNA extraction methods (two kit-based methods [QK and PQ] and a phenol–chloroform-based method [PC]). Based on these findings, we provided an easy-to-follow, step-by-step protocol for strain-specific qPCR assay development and validated this protocol by designing two PCR assays for two L. reuteri strains. The qPCR assays not only enabled absolute quantification with high accuracy but also exhibited a much lower LOD and a broader dynamic range for detecting L. reuteri in human fecal samples, especially when compared to NGS approaches (i.e., 16S rRNA gene sequencing and WMS). These findings demonstrate the feasibility of our approach to achieve the accurate and sensitive absolute quantification of bacterial strains in human stools, and the protocol will provide a resource for scientists to design strain-specific qPCR for their own applications and target bacterial strains.
Among the three DNA extraction methods tested in this study, PQ is the most robust protocol for human stool samples with the highest yield and purity of DNA, and it is therefore compatible with not only sequencing-based approaches, for which it was developed [ 41 ], but also PCR-based quantification. However, PQ requires more than twice as much time as QK. Although slightly less robust, QK produced DNA with sufficient quality and purity that performed well with both PCR approaches, which makes QK a good compromise between the time required and DNA quality. PC harvested a higher amount of DNA than PQ and QK but with much lower quality and purity. This might be due to a large amount of DNA extracted from the background and the insufficient removal of co-extracted PCR inhibitors using phenol–chloroform extractions, while protocols using silica columns (QK and PQ) substantially enhanced the separation of DNA and potential PCR inhibitors [ 59 ].
Our findings demonstrate that ddPCR had higher reproducibility than qPCR, and it therefore could improve the inter-study comparability among different laboratories. However, we did not find higher sensitivity of ddPCR than qPCR. In addition, qPCR is more practical than ddPCR for most applications for several reasons: first, we found that qPCR exhibited equal sensitivity (LOD) as ddPCR for quantification of L. reuteri . Second, given that ddPCR cannot detect more than 5 Log 10 gene copies per reaction due to the limited number of droplets generated [ 30 ], qPCR has a broader dynamic range than ddPCR. This gives qPCR more flexibility when implemented in studies where samples of large microbial loads are detected. Specifically, with the method PQ, the Cq value of qPCR at the concentration of 7.97 Log 10 cells/g feces was around 18 (data not shown), and therefore, the upper limit of qPCR reaches 10 Log 10 cells/g feces. But ddPCR was nearly 80% saturated at the same amount of input and is thus theoretically unable to detect over eight Log 10 cells/g feces, leading to a two Log 10 difference between qPCR and ddPCR. Third, with respect to economic aspects, qPCR is less expensive and less time- and labor-intensive compared with ddPCR. Accordingly, we consider qPCR a superior approach to ddPCR for quantifying bacteria in human fecal samples.
Constructing an unbiased external standard curve is critical for achieving an accurate quantification using qPCR. We attempted two different strategies for the standard curve construction. To quantify L. reuteri DSM 17938, we made the standard curve by determining the amounts of nucleic acids in reference materials (genomic DNA of this strain) spectrophotometrically. We observed a higher number of gene copies determined via qPCR than ddPCR, which is consistent with other studies [ 29 , 60 ]. The reason is that spectrophotometers indistinguishably quantify compounds with absorbance at 260 nm, resulting in an overestimation of DNA in reference materials. Apart from this strategy, cell numbers quantified by plating, flow cytometry, or microscopy could also be used as the calibrator for qPCR [ 23 , 29 , 61 ]. When we developed the strain- and lineage-specific qPCR assays for PB-W1 and DSM 20016 T , we constructed the standard curves using serial dilutions of genomic DNA of standardized cultures with known cell counts of L. reuteri as determined by plating on MRS media. For this approach, only viable cells in reference materials are taken into consideration. Cells of L. reuteri used for calibration of the PCR assays were harvested at 8 h of growth, i.e., before the dead cells account for a relevant proportion of bacterial cells [ 36 ]. Standard curves constructed using both strategies showed high linearity ( R 2 > 0.99) and acceptable efficiency (89–115%), and no apparent difference was observed. Therefore, both strategies could be applied for qPCR quantification.
Our primary motivation for the development of an easy-to-follow, step-by-step protocol for strain-specific qPCR (Supplementary File 3) was to provide a resource that scientists can follow even if they are not experts in comparative genomics. The protocol starts from the design of strain-specific primers using software that are easy to apply, followed by an in-detail description of both in silico and experimental validation procedures to ensure the primer specificity. When combined with appropriate DNA extraction methods, this approach results in highly accurate qPCR systems with high sensitivity. Our validation of qPCR assays designed with this approach using human fecal samples demonstrated a much lower LOD and a wider dynamic range for detecting and quantifying target bacteria strains, especially when compared to NGS approaches. The protocol we described is based on our previous successful work that used qPCR to quantify various target bacterial strains from the microbial communities, including Bifidobacterium longum , Bifidobacterium adolescentis , Bifidobacterium animalis subsp. lactis , L. reuteri , Lacticaseibacillus case , and Limosilactobacillus fermentum [ 21 , 22 , 23 , 24 , 25 ]. This confirms the wide applicability of the protocol presented in this study: it can easily be adapted to other bacterial taxa and sample types, but it is important that appropriate modifications are made to the DNA extraction method, which has to be compatible with both the specific sample type and the bacterial isolate.
We describe a strain-specific qPCR assay with high reproducibility, linearity, and accuracy, which further outperformed ddPCR in terms of dynamic range, cost, and time. Considering all these factors, we suggest the combination of qPCR with kit-based DNA extraction methods as the best option to quantify gut microbial members in human fecal samples at the strain level. To our knowledge, our study is the first to successfully push the LOD of a target strain to around three Log 10 cells/g feces. The step-by-step protocol for strain-specific primer design and in silico and experimental validation described here will have a broad range of applications for scientists to detect and quantify various bacterial strains from a variety of circumstances (not only fecal samples but also other gut samples, such as biopsy and swab samples), including but not limited to evaluating the persistence of probiotics and live biotherapeutics, detecting pathogens or other disease-associated microbes, tracking bacterial strains during vertical transmission and fecal microbiota transplantation, and confirming and establishing the bacterial load in low-biomass samples.
Availability of data and materials
The original sequencing data of 16S rRNA gene sequencing and whole metagenome sequencing have been uploaded to the NCBI Sequence Read Archive (SRA), and the accession number is PRJNA1000186. All the other data and materials have been all described in the manuscript.
Forster SC, Kumar N, Anonye BO, Almeida A, Viciani E, Stares MD, et al. A human gut bacterial genome and culture collection for improved metagenomic analyses. Nat Biotechnol. 2019;37:186–92.
Article PubMed PubMed Central CAS Google Scholar
Yatsunenko T, Rey FE, Manary MJ, Trehan I, Dominguez-Bello MG, Contreras M, et al. Human gut microbiome viewed across age and geography. Nature. 2012;486:222–7.
Geva-Zatorsky N, Sefik E, Kua L, Pasman L, Tan TG, Ortiz-Lopez A, et al. Mining the human gut microbiota for immunomodulatory organisms. Cell. 2017;168:928–943.e911.
Buffie CG, Bucci V, Stein RR, McKenney PT, Ling L, Gobourne A, et al. Precision microbiome reconstitution restores bile acid mediated resistance to Clostridium difficile. Nature. 2015;517:205–8.
Article PubMed CAS Google Scholar
Conlon MA, Bird AR. The impact of diet and lifestyle on gut microbiota and human health. Nutrients. 2014;7:17–44.
Article PubMed PubMed Central Google Scholar
Rogers GB, Keating DJ, Young RL, Wong ML, Licinio J, Wesselingh S. From gut dysbiosis to altered brain function and mental illness: mechanisms and pathways. Mol Psychiatry. 2016;21:738–48.
Kostic AD, Chun E, Robertson L, Glickman JN, Gallini CA, Michaud M, et al. Fusobacterium nucleatum potentiates intestinal tumorigenesis and modulates the tumor-immune microenvironment. Cell Host Microbe. 2013;14:207–15.
Nishida A, Inoue R, Inatomi O, Bamba S, Naito Y, Andoh A. Gut microbiota in the pathogenesis of inflammatory bowel disease. Clin J Gastroenterol. 2018;11:1–10.
Article PubMed Google Scholar
Karlsson FH, Tremaroli V, Nookaew I, Bergström G, Behre CJ, Fagerberg B, et al. Gut metagenome in European women with normal, impaired and diabetic glucose control. Nature. 2013;498:99–103.
Wang Z, Klipfell E, Bennett BJ, Koeth R, Levison BS, Dugar B, et al. Gut flora metabolism of phosphatidylcholine promotes cardiovascular disease. Nature. 2011;472:57–63.
Segata N. On the road to strain-resolved comparative metagenomics. mSystems. 2018;3(2):e00190–1.
Duar RM, Frese SA, Lin XB, Fernando SC, Burkey TE, Tasseva G, et al. Experimental evaluation of host adaptation of Lactobacillus reuteri to different vertebrate species. Appl Environ Microbiol. 2017;83(12):e00132–17.
Frese SA, Benson AK, Tannock GW, Loach DM, Kim J, Zhang M, et al. The evolution of host specialization in the vertebrate gut symbiont Lactobacillus reuteri. PLoS Genet. 2011;7: e1001314.
Beghini F, McIver LJ, Blanco-Míguez A, Dubois L, Asnicar F, Maharjan S, et al. Integrating taxonomic, functional, and strain-level profiling of diverse microbial communities with bioBakery 3. Elife. 2021;10.
Barlow JT, Leite G, Romano AE, Sedighi R, Chang C, Celly S, et al. Quantitative sequencing clarifies the role of disruptor taxa, oral microbiota, and strict anaerobes in the human small-intestine microbiome. Microbiome. 2021;9:214.
Wang X, Howe S, Deng F, Zhao J. Current applications of absolute bacterial quantification in microbiome studies and decision-making regarding different biological questions. Microorganisms. 2021;9(9):1797.
Depommier C, Everard A, Druart C, Plovier H, Van Hul M, Vieira-Silva S, et al. Supplementation with Akkermansia muciniphila in overweight and obese human volunteers: a proof-of-concept exploratory study. Nat Med. 2019;25:1096–103.
Zmora N, Zilberman-Schapira G, Suez J, Mor U, Dori-Bachash M, Bashiardes S, et al. Personalized gut mucosal colonization resistance to empiric probiotics is associated with unique host and microbiome features. Cell. 2018;174:1388–1405.e1321.
Jian C, Luukkonen P, Yki-Järvinen H, Salonen A, Korpela K. Quantitative PCR provides a simple and accessible method for quantitative microbiota profiling. PLoS ONE. 2020;15: e0227285.
Vandeputte D, Kathagen G, D’Hoe K, Vieira-Silva S, Valles-Colomer M, Sabino J, et al. Quantitative microbiome profiling links gut community variation to microbial load. Nature. 2017;551:507–11.
Krumbeck JA, Rasmussen HE, Hutkins RW, Clarke J, Shawron K, Keshavarzian A, et al. Probiotic Bifidobacterium strains and galactooligosaccharides improve intestinal barrier function in obese adults but show no synergism when used together as synbiotics. Microbiome. 2018;6:121.
Maldonado-Gómez MX, Martínez I, Bottacini F, O’Callaghan A, Ventura M, van Sinderen D, et al. Stable engraftment of Bifidobacterium longum AH1206 in the human gut depends on individualized features of the resident microbiome. Cell Host Microbe. 2016;20:515–26.
Martínez I, Maldonado-Gomez MX, Gomes-Neto JC, Kittana H, Ding H, Schmaltz R, et al. Experimental evaluation of the importance of colonization history in early-life gut microbiota assembly. Elife. 2018;7:e36521.
Zhao X, Wang W, Blaine A, Kane ST, Zijlstra RT, Gänzle MG. Impact of probiotic Lactobacillus sp. on autochthonous lactobacilli in weaned piglets. J Appl Microbiol. 2019;126:242–54.
Yang Y, Galle S, Le MH, Zijlstra RT, Gänzle MG. Feed fermentation with reuteran- and levan-producing Lactobacillus reuteri reduces colonization of weanling pigs by enterotoxigenic Escherichia coli. Appl Environ Microbiol. 2015;81:5743–52.
Pinheiro LB, Coleman VA, Hindson CM, Herrmann J, Hindson BJ, Bhat S, et al. Evaluation of a droplet digital polymerase chain reaction format for DNA copy number quantification. Anal Chem. 2012;84:1003–11.
Doi H, Takahara T, Minamoto T, Matsuhashi S, Uchii K, Yamanaka H. Droplet digital polymerase chain reaction (PCR) outperforms real-time PCR in the detection of environmental DNA from an invasive fish species. Environ Sci Technol. 2015;49:5601–8.
Nechvatal JM, Ram JL, Basson MD, Namprachan P, Niec SR, Badsha KZ, et al. Fecal collection, ambient preservation, and DNA extraction for PCR amplification of bacterial and human markers from human feces. J Microbiol Methods. 2008;72:124–32.
Yang R, Paparini A, Monis P, Ryan U. Comparison of next-generation droplet digital PCR (ddPCR) with quantitative PCR (qPCR) for enumeration of Cryptosporidium oocysts in faecal samples. Int J Parasitol. 2014;44:1105–13.
Hindson BJ, Ness KD, Masquelier DA, Belgrader P, Heredia NJ, Makarewicz AJ, et al. High-throughput droplet digital PCR system for absolute quantitation of DNA copy number. Anal Chem. 2011;83:8604–10.
Miotke L, Lau BT, Rumma RT, Ji HP. High sensitivity detection and quantitation of DNA copy number and single nucleotide variants with single color droplet digital PCR. Anal Chem. 2014;86:2618–24.
Strain MC, Lada SM, Luong T, Rought SE, Gianella S, Terry VH, et al. Highly precise measurement of HIV DNA by droplet digital PCR. PLoS ONE. 2013;8: e55943.
Cavé L, Brothier E, Abrouk D, Bouda PS, Hien E, Nazaret S. Efficiency and sensitivity of the digital droplet PCR for the quantification of antibiotic resistance genes in soils and organic residues. Appl Microbiol Biotechnol. 2016;100:10597–608.
Gobert G, Cotillard A, Fourmestraux C, Pruvost L, Miguet J, Boyer M. Droplet digital PCR improves absolute quantification of viable lactic acid bacteria in faecal samples. J Microbiol Methods. 2018;148:64–73.
Palumbo JD, O’Keeffe TL, Fidelibus MW. Characterization of Aspergillus section Nigri species populations in vineyard soil using droplet digital PCR. Lett Appl Microbiol. 2016;63:458–65.
Walter J, Loach DM, Alqumber M, Rockel C, Hermann C, Pfitzenmaier M, et al. D-alanyl ester depletion of teichoic acids in Lactobacillus reuteri 100–23 results in impaired colonization of the mouse gastrointestinal tract. Environ Microbiol. 2007;9:1750–60.
Zhang Z, Wang K, Oh JH, Zhang S, van Pijkeren JP, Cheng CC, et al. A phylogenetic view on the role of glycerol for growth enhancement and reuterin formation in Limosilactobacillus reuteri. Front Microbiol. 2020;11: 601422.
Egervärn M, Lindmark H, Olsson J, Roos S. Transferability of a tetracycline resistance gene from probiotic Lactobacillus reuteri to bacteria in the gastrointestinal tract of humans. Antonie Van Leeuwenhoek. 2010;97:189–200.
Martínez I, Wallace G, Zhang C, Legge R, Benson AK, Carr TP, et al. Diet-induced metabolic improvements in a hamster model of hypercholesterolemia are strongly linked to alterations of the gut microbiota. Appl Environ Microbiol. 2009;75:4175–84.
Martínez I, Kim J, Duffy PR, Schlegel VL, Walter J. Resistant starches types 2 and 4 have differential effects on the composition of the fecal microbiota in human subjects. PLoS ONE. 2010;5: e15046.
Costea PI, Zeller G, Sunagawa S, Pelletier E, Alberti A, Levenez F, et al. Towards standards for human fecal sample processing in metagenomic studies. Nat Biotechnol. 2017;35:1069–76.
Kralik P, Ricchi M. A basic guide to real time PCR in microbial diagnostics: definitions, parameters, and everything. Front Microbiol. 2017;8:108.
Wang Y, Cooper R, Bergelson S, Feschenko M. Quantification of residual BHK DNA by a novel droplet digital PCR technology. J Pharm Biomed Anal. 2018;159:477–82.
Li F, Li X, Cheng CC, Bujdoš D, Tollenaar S, Simpson DJ, et al. A phylogenomic analysis of Limosilactobacillus reuteri reveals ancient and stable evolutionary relationships with rodents and birds and zoonotic transmission to humans. BMC Biol. 2023;21:53.
Oh PL, Benson AK, Peterson DA, Patil PB, Moriyama EN, Roos S, et al. Diversification of the gut symbiont Lactobacillus reuteri as a result of host-driven evolution. Isme j. 2010;4:377–87.
Walter J, Britton RA, Roos S. Host-microbial symbiosis in the vertebrate gastrointestinal tract and the Lactobacillus reuteri paradigm. Proc Natl Acad Sci U S A. 2011;108(Suppl 1):4645–52.
Li F, Cheng CC, Zheng J, Liu J, Quevedo RM, Li J, et al. Limosilactobacillus balticus sp. nov., Limosilactobacillus agrestis sp. nov., Limosilactobacillus albertensis sp. nov., Limosilactobacillus rudii sp. nov. and Limosilactobacillus fastidiosus sp. nov., five novel Limosilactobacillus species isolated from the vertebrate gastrointestinal tract, and proposal of six subspecies of Limosilactobacillus reuteri adapted to the gastrointestinal tract of specific vertebrate hosts. Int J Syst Evol Microbiol. 2021;71(2):004644.
Martínez I, Stegen JC, Maldonado-Gómez MX, Eren AM, Siba PM, Greenhill AR, et al. The gut microbiota of rural Papua New Guineans: composition, diversity patterns, and ecological processes. Cell Rep. 2015;11:527–38.
Walter J, Hertel C, Tannock GW, Lis CM, Munro K, Hammes WP. Detection of Lactobacillus, Pediococcus, Leuconostoc, and Weissella species in human feces by using group-specific PCR primers and denaturing gradient gel electrophoresis. Appl Environ Microbiol. 2001;67:2578–85.
Untergasser A, Nijveen H, Rao X, Bisseling T, Geurts R, Leunissen JA. Primer3Plus, an enhanced web interface to Primer3. Nucleic Acids Res. 2007;35:W71–74.
Bolyen E, Rideout JR, Dillon MR, Bokulich NA, Abnet CC, Al-Ghalith GA, et al. Reproducible, interactive, scalable and extensible microbiome data science using QIIME 2. Nat Biotechnol. 2019;37:852–7.
Blanco-Míguez A, Beghini F, Cumbo F, McIver LJ, Thompson KN, Zolfo M, et al. Extending and improving metagenomic taxonomic profiling with uncharacterized species using MetaPhlAn 4. Nat Biotechnol. 2023;41(11):1633–44.
R Core Team: R: a language and environment for statistical computing. In . Vienna, Austria: R Foundation for Statistical Computing; 2014.
Villa C, Costa J, Gondar C, Oliveira M, Mafra I. Effect of food matrix and thermal processing on the performance of a normalised quantitative real-time PCR approach for lupine (Lupinus albus) detection as a potential allergenic food. Food Chem. 2018;262:251–9.
Hindson CM, Chevillet JR, Briggs HA, Gallichotte EN, Ruf IK, Hindson BJ, et al. Absolute quantification by droplet digital PCR versus analog real-time PCR. Nat Methods. 2013;10:1003–5.
Stauber J, Shaikh N, Ordiz MI, Tarr PI, Manary MJ. Droplet digital PCR quantifies host inflammatory transcripts in feces reliably and reproducibly. Cell Immunol. 2016;303:43–9.
Yuan D, Cui M, Yu S, Wang H, Jing R. Droplet digital PCR for quantification of PML-RARα in acute promyelocytic leukemia: a comprehensive comparison with real-time PCR. Anal Bioanal Chem. 2019;411:895–903.
Broeders S, Huber I, Grohmann L, Berben G, Taverniers I, Mazzara M, et al. Guidelines for validation of qualitative real-time PCR methods. Trends Food Sci Technol. 2014;37:115–26.
Article CAS Google Scholar
Yang DY, Eng B, Waye JS, Dudar JC, Saunders SR. Technical note: improved DNA extraction from ancient bones using silica-based spin columns. Am J Phys Anthropol. 1998;105:539–43.
Cao Y, Raith MR, Griffith JF. Droplet digital PCR for simultaneous quantification of general and human-associated fecal indicators for water quality assessment. Water Res. 2015;70:337–49.
Cao Y, Griffith JF, Dorevitch S, Weisberg SB. Effectiveness of qPCR permutations, internal controls and dilution as means for minimizing the impact of inhibition while measuring Enterococcus in environmental waters. J Appl Microbiol. 2012;113:66–75.
Download references
Acknowledgements
The authors would like to thank Junjie Li and Rodrigo Margain Quevedo for technical assistance and Dr. Xiaoxi Lin, Dr. Zhihong Zhang, Dr. Urmila Basu, and Naomi Hotte for advice and guidance.
This work was financially supported by the Campus Alberta Innovates Program (CAIP; Canada), the Weston Family Microbiome Initiative (the W. Garfield Weston Foundation ; Canada), the Irish Government’s National Development Plan from Science Foundation Ireland (Ireland), and the “Hundred Talents Program” Research Start-up Fund of Zhejiang University (Zhejiang University; China). FL was also supported by the Alberta Innovates Postgraduate Fellowship (Alberta Innovates; Canada).
Author information
Authors and affiliations.
Department of Animal Science and Technology, College of Animal Sciences, Zhejiang University, Hangzhou, 310058, China
Department of Agricultural, Food and Nutritional Science, University of Alberta, Edmonton, AB, T6G 2E1, Canada
Fuyong Li, Junhong Liu, Michael G. Gänzle & Jens Walter
One Bio Inc., Sacramento, CA, 95833, USA
María X. Maldonado-Gómez
Department of Nutrition, University of Nevada, Reno, NV, 89557, USA
Steven A. Frese
Department of Biological Sciences, University of Alberta, Edmonton, AB, T6G 2E1, Canada
Jens Walter
School of Microbiology, Department of Medicine, and APC Microbiome Ireland, University College Cork, Cork, T12 YT20, Ireland
You can also search for this author in PubMed Google Scholar
Contributions
FL and JW designed this study; FL and JL performed bacteria growth, artificial spiking of stool samples, DNA isolation, ddPCR, qPCR, identification of strain- and lineage-specific genes, primer design and validation, and statistical analyses; FL and JL collected fecal samples from the human clinical trial, and conducted qPCR, selective culture, 16S rRNA gene sequencing, and whole metagenome sequencing; FL, JL, MXM, SF, MGG, and JW were responsible for protocol development and optimization, data interpretation, and manuscript writing.
Corresponding authors
Correspondence to Fuyong Li or Jens Walter .
Ethics declarations
Ethics approval and consent to participate.
The present study received research ethics approval from the Health Research Ethics Board — Biomedical Panel of the University of Alberta (no. Pro00077565), and fecal samples were collected from health human subjects with written informed consent at the University of Alberta Human Nutrition Research Unit (Edmonton, Canada).
Consent for publication
Not applicable.
Competing interests
The authors declare no competing interests.
Additional information
Publisher's note.
Springer Nature remains neutral with regard to jurisdictional claims in published maps and institutional affiliations.
Supplementary Information
40168_2024_1881_moesm1_esm.docx.
Supplementary Material 1: Supplementary figures. Figure S1. Preparation of fecal samples spiked with known numbers of bacteria. L. reuteri DSM 17938 was grown on MRS agar for 48 hours in an anaerobic chamber at 37 °C. After that, single colonies were picked and transferred to MRS broth, and sub-cultured twice (24 hours for the first sub-culture, and 8 hours for the second sub-culture to ensure bacterial cells stay at the late exponential phase or early stationary phase). Cell numbers in cultures were determined by plating a ten-fold serial dilution on MRS agar. Then serial dilutions of bacteria with exact cell numbers were prepared by dilution, and mixed with 0.1 g stool aliquots. Figure S2. qPCR standards were established by a 10-fold serial dilution of genomic DNA of L. reuteri DSM 17938, and each dot represent the average Cq of six replicates from three independent runs. Figure S3. Melt curves for stool samples spiked with L. reuteri DSM 17938 in qPCR. (A-C), results obtained from spiked samples of three individuals and there was one specific peak at around 87.5℃, which indicated the amplification of target sequence for all reactions. Figure S4. Correlation between qPCR and ddPCR for fecal samples spiked with L. reuteri DSM 17938 when the method PQ was used. Solid line, regression line; dash line, y = x. All dots are results obtained in qPCR and ddPCR. Supplementary tables. Table S1. Screen the existence of L. reuteri DSM 17938 among nine human stools and test the specificities of the designed primers for L. reuteri PB-W1 and DSM 20016 T based on the fecal background DNA. Table S2. Quantities and qualities of DNA extracted using different DNA isolation methods. Table S3. L. reuteri genomes available in Joint Genome Institute (JGI) genome portal selected for the present study.
40168_2024_1881_MOESM2_ESM.docx
Supplementary Material 2. Procedures of the three DNA extraction methods tested in the present study. Phenol chloroform-based method (PC). QIAamp fast stool DNA kit-based method (QK). Protocol Q-based method (PQ).
40168_2024_1881_MOESM3_ESM.docx
Supplementary Material 3. A step-by-step protocol for the design of strain-specific qPCR assays for the strain-level detection and quantification of bacteria in fecal samples.
Rights and permissions
Open Access This article is licensed under a Creative Commons Attribution-NonCommercial-NoDerivatives 4.0 International License, which permits any non-commercial use, sharing, distribution and reproduction in any medium or format, as long as you give appropriate credit to the original author(s) and the source, provide a link to the Creative Commons licence, and indicate if you modified the licensed material. You do not have permission under this licence to share adapted material derived from this article or parts of it. The images or other third party material in this article are included in the article’s Creative Commons licence, unless indicated otherwise in a credit line to the material. If material is not included in the article’s Creative Commons licence and your intended use is not permitted by statutory regulation or exceeds the permitted use, you will need to obtain permission directly from the copyright holder. To view a copy of this licence, visit http://creativecommons.org/licenses/by-nc-nd/4.0/ .
Reprints and permissions
About this article
Cite this article.
Li, F., Liu, J., Maldonado-Gómez, M.X. et al. Highly accurate and sensitive absolute quantification of bacterial strains in human fecal samples. Microbiome 12 , 168 (2024). https://doi.org/10.1186/s40168-024-01881-2
Download citation
Received : 26 January 2023
Accepted : 26 July 2024
Published : 07 September 2024
DOI : https://doi.org/10.1186/s40168-024-01881-2
Share this article
Anyone you share the following link with will be able to read this content:
Sorry, a shareable link is not currently available for this article.
Provided by the Springer Nature SharedIt content-sharing initiative
- DNA extraction
- Strain-specific primers
- Lactobacillus
- Limosilactobacillus reuteri
ISSN: 2049-2618
- General enquiries: [email protected]

IMAGES
VIDEO
COMMENTS
Survey Research | Definition, Examples & Methods
Survey Research. Definition: Survey Research is a quantitative research method that involves collecting standardized data from a sample of individuals or groups through the use of structured questionnaires or interviews. The data collected is then analyzed statistically to identify patterns and relationships between variables, and to draw conclusions about the population being studied.
Understanding and Evaluating Survey Research - PMC
Survey Research: Definition, Examples and Methods
What is Quantitative Research Design? Definition, Types, ...
What Is Quantitative Research? | Definition, Uses & Methods
What Is a Research Design | Types, Guide & ...
The survey is a common technique in quantitative observational research (Creswell 2014); it provides a numeric description of sample characteristics or the whole population under study (Balnaves and Caputi 2001).It is widely used in a variety of fields to generate information.
Doing Survey Research | A Step-by-Step Guide & Examples
Historically, surveys have been seen as an exclusively quantitative research method but this is really an outmoded view. A carefully designed survey can generate significant amounts of qualitative data that can enhance and illuminate the quantitative product of surveys (e.g. McBeath 2019; McBeath et al. 2019).
Survey research is a quantitative and qualitative method with two important characteristics. First, the variables of interest are measured using self-reports. In essence, survey researchers ask their participants (who are often called respondents in survey research) to report directly on their own thoughts, feelings, and behaviours.
A quick guide to survey research - PMC
Survey Research - an overview
Designing, Conducting, and Reporting Survey Studies
What Are Quantitative Survey Questions? Types and ...
Types of quantitative research
(PDF) Survey as a Quantitative Research Method
Survey Descriptive Research: Design & Examples
When are surveys qualitative or quantitative research
Quantitative Research Excellence: Study Design and ...
actions, or opinions of a large group of people". three distinguishing characteristics of survey research. First, survey research is used to quantitatively describe specific. aspects of a given ...
Types of Quantitative Research Methods and Designs
Survey data plays a crucial role in decision-making for businesses, governments, and researchers. Here are some key reasons why survey data is important: Market Research: Businesses use surveys to understand customer needs, preferences, and satisfaction levels, which helps them develop products and services that better meet market demands.
Following this, the quantitative online survey solely examines the practice-level perspective of policy implementation. The mixed-method study's findings are ultimately presented using a triangulation approach to combine the results from the qualitative and quantitative perspectives and address the research questions in a holistic matter.
A Practical Guide to Writing Quantitative and Qualitative ...
The research design may be quantitative, qualitative, or a mixed methods design. The focus of this overview is quantitative methods. The general purpose of quantitative research is to explain, predict, investigate relationships, describe current conditions, or to examine possible impacts or influences on designated outcomes.
Debris flows and floods generated by rainfall runoff occur in rocky mountainous landscapes and burned steeplands. Flow type is commonly identified post-event through interpretation of depositional structures, but these may be poorly preserved or misinterpreted. Prior research indicates that discharge magnitude is commonly amplified in debris flows relative to floods due to volumetric bulking and i
Next-generation sequencing (NGS) approaches have revolutionized gut microbiome research and can provide strain-level resolution, but these techniques have limitations in that they are only semi-quantitative, suffer from high detection limits, and generate data that is compositional. The present study aimed to systematically compare quantitative PCR (qPCR) and droplet digital PCR (ddPCR) for ...