Have a thesis expert improve your writing
Check your thesis for plagiarism in 10 minutes, generate your apa citations for free.
- Knowledge Base
- Null and Alternative Hypotheses | Definitions & Examples

Null and Alternative Hypotheses | Definitions & Examples
Published on 5 October 2022 by Shaun Turney . Revised on 6 December 2022.
The null and alternative hypotheses are two competing claims that researchers weigh evidence for and against using a statistical test :
- Null hypothesis (H 0 ): There’s no effect in the population .
- Alternative hypothesis (H A ): There’s an effect in the population.
The effect is usually the effect of the independent variable on the dependent variable .
Table of contents
Answering your research question with hypotheses, what is a null hypothesis, what is an alternative hypothesis, differences between null and alternative hypotheses, how to write null and alternative hypotheses, frequently asked questions about null and alternative hypotheses.
The null and alternative hypotheses offer competing answers to your research question . When the research question asks “Does the independent variable affect the dependent variable?”, the null hypothesis (H 0 ) answers “No, there’s no effect in the population.” On the other hand, the alternative hypothesis (H A ) answers “Yes, there is an effect in the population.”
The null and alternative are always claims about the population. That’s because the goal of hypothesis testing is to make inferences about a population based on a sample . Often, we infer whether there’s an effect in the population by looking at differences between groups or relationships between variables in the sample.
You can use a statistical test to decide whether the evidence favors the null or alternative hypothesis. Each type of statistical test comes with a specific way of phrasing the null and alternative hypothesis. However, the hypotheses can also be phrased in a general way that applies to any test.
The null hypothesis is the claim that there’s no effect in the population.
If the sample provides enough evidence against the claim that there’s no effect in the population ( p ≤ α), then we can reject the null hypothesis . Otherwise, we fail to reject the null hypothesis.
Although “fail to reject” may sound awkward, it’s the only wording that statisticians accept. Be careful not to say you “prove” or “accept” the null hypothesis.
Null hypotheses often include phrases such as “no effect”, “no difference”, or “no relationship”. When written in mathematical terms, they always include an equality (usually =, but sometimes ≥ or ≤).
Examples of null hypotheses
The table below gives examples of research questions and null hypotheses. There’s always more than one way to answer a research question, but these null hypotheses can help you get started.
( ) | ||
Does tooth flossing affect the number of cavities? | Tooth flossing has on the number of cavities. | test: The mean number of cavities per person does not differ between the flossing group (µ ) and the non-flossing group (µ ) in the population; µ = µ . |
Does the amount of text highlighted in the textbook affect exam scores? | The amount of text highlighted in the textbook has on exam scores. | : There is no relationship between the amount of text highlighted and exam scores in the population; β = 0. |
Does daily meditation decrease the incidence of depression? | Daily meditation the incidence of depression.* | test: The proportion of people with depression in the daily-meditation group ( ) is greater than or equal to the no-meditation group ( ) in the population; ≥ . |
*Note that some researchers prefer to always write the null hypothesis in terms of “no effect” and “=”. It would be fine to say that daily meditation has no effect on the incidence of depression and p 1 = p 2 .
The alternative hypothesis (H A ) is the other answer to your research question . It claims that there’s an effect in the population.
Often, your alternative hypothesis is the same as your research hypothesis. In other words, it’s the claim that you expect or hope will be true.
The alternative hypothesis is the complement to the null hypothesis. Null and alternative hypotheses are exhaustive, meaning that together they cover every possible outcome. They are also mutually exclusive, meaning that only one can be true at a time.
Alternative hypotheses often include phrases such as “an effect”, “a difference”, or “a relationship”. When alternative hypotheses are written in mathematical terms, they always include an inequality (usually ≠, but sometimes > or <). As with null hypotheses, there are many acceptable ways to phrase an alternative hypothesis.
Examples of alternative hypotheses
The table below gives examples of research questions and alternative hypotheses to help you get started with formulating your own.
Does tooth flossing affect the number of cavities? | Tooth flossing has an on the number of cavities. | test: The mean number of cavities per person differs between the flossing group (µ ) and the non-flossing group (µ ) in the population; µ ≠ µ . |
Does the amount of text highlighted in a textbook affect exam scores? | The amount of text highlighted in the textbook has an on exam scores. | : There is a relationship between the amount of text highlighted and exam scores in the population; β ≠ 0. |
Does daily meditation decrease the incidence of depression? | Daily meditation the incidence of depression. | test: The proportion of people with depression in the daily-meditation group ( ) is less than the no-meditation group ( ) in the population; < . |
Null and alternative hypotheses are similar in some ways:
- They’re both answers to the research question
- They both make claims about the population
- They’re both evaluated by statistical tests.
However, there are important differences between the two types of hypotheses, summarized in the following table.
A claim that there is in the population. | A claim that there is in the population. | |
| ||
Equality symbol (=, ≥, or ≤) | Inequality symbol (≠, <, or >) | |
Rejected | Supported | |
Failed to reject | Not supported |
To help you write your hypotheses, you can use the template sentences below. If you know which statistical test you’re going to use, you can use the test-specific template sentences. Otherwise, you can use the general template sentences.
The only thing you need to know to use these general template sentences are your dependent and independent variables. To write your research question, null hypothesis, and alternative hypothesis, fill in the following sentences with your variables:
Does independent variable affect dependent variable ?
- Null hypothesis (H 0 ): Independent variable does not affect dependent variable .
- Alternative hypothesis (H A ): Independent variable affects dependent variable .
Test-specific
Once you know the statistical test you’ll be using, you can write your hypotheses in a more precise and mathematical way specific to the test you chose. The table below provides template sentences for common statistical tests.
( ) | ||
test
with two groups | The mean dependent variable does not differ between group 1 (µ ) and group 2 (µ ) in the population; µ = µ . | The mean dependent variable differs between group 1 (µ ) and group 2 (µ ) in the population; µ ≠ µ . |
with three groups | The mean dependent variable does not differ between group 1 (µ ), group 2 (µ ), and group 3 (µ ) in the population; µ = µ = µ . | The mean dependent variable of group 1 (µ ), group 2 (µ ), and group 3 (µ ) are not all equal in the population. |
There is no correlation between independent variable and dependent variable in the population; ρ = 0. | There is a correlation between independent variable and dependent variable in the population; ρ ≠ 0. | |
There is no relationship between independent variable and dependent variable in the population; β = 0. | There is a relationship between independent variable and dependent variable in the population; β ≠ 0. | |
Two-proportions test | The dependent variable expressed as a proportion does not differ between group 1 ( ) and group 2 ( ) in the population; = . | The dependent variable expressed as a proportion differs between group 1 ( ) and group 2 ( ) in the population; ≠ . |
Note: The template sentences above assume that you’re performing one-tailed tests . One-tailed tests are appropriate for most studies.
The null hypothesis is often abbreviated as H 0 . When the null hypothesis is written using mathematical symbols, it always includes an equality symbol (usually =, but sometimes ≥ or ≤).
The alternative hypothesis is often abbreviated as H a or H 1 . When the alternative hypothesis is written using mathematical symbols, it always includes an inequality symbol (usually ≠, but sometimes < or >).
A research hypothesis is your proposed answer to your research question. The research hypothesis usually includes an explanation (‘ x affects y because …’).
A statistical hypothesis, on the other hand, is a mathematical statement about a population parameter. Statistical hypotheses always come in pairs: the null and alternative hypotheses. In a well-designed study , the statistical hypotheses correspond logically to the research hypothesis.
Cite this Scribbr article
If you want to cite this source, you can copy and paste the citation or click the ‘Cite this Scribbr article’ button to automatically add the citation to our free Reference Generator.
Turney, S. (2022, December 06). Null and Alternative Hypotheses | Definitions & Examples. Scribbr. Retrieved 3 September 2024, from https://www.scribbr.co.uk/stats/null-and-alternative-hypothesis/
Is this article helpful?
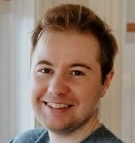
Shaun Turney
Other students also liked, levels of measurement: nominal, ordinal, interval, ratio, the standard normal distribution | calculator, examples & uses, types of variables in research | definitions & examples.
9.1 Null and Alternative Hypotheses
The actual test begins by considering two hypotheses . They are called the null hypothesis and the alternative hypothesis . These hypotheses contain opposing viewpoints.
H 0 , the — null hypothesis: a statement of no difference between sample means or proportions or no difference between a sample mean or proportion and a population mean or proportion. In other words, the difference equals 0.
H a —, the alternative hypothesis: a claim about the population that is contradictory to H 0 and what we conclude when we reject H 0 .
Since the null and alternative hypotheses are contradictory, you must examine evidence to decide if you have enough evidence to reject the null hypothesis or not. The evidence is in the form of sample data.
After you have determined which hypothesis the sample supports, you make a decision. There are two options for a decision. They are reject H 0 if the sample information favors the alternative hypothesis or do not reject H 0 or decline to reject H 0 if the sample information is insufficient to reject the null hypothesis.
Mathematical Symbols Used in H 0 and H a :
equal (=) | not equal (≠) greater than (>) less than (<) |
greater than or equal to (≥) | less than (<) |
less than or equal to (≤) | more than (>) |
H 0 always has a symbol with an equal in it. H a never has a symbol with an equal in it. The choice of symbol depends on the wording of the hypothesis test. However, be aware that many researchers use = in the null hypothesis, even with > or < as the symbol in the alternative hypothesis. This practice is acceptable because we only make the decision to reject or not reject the null hypothesis.
Example 9.1
H 0 : No more than 30 percent of the registered voters in Santa Clara County voted in the primary election. p ≤ 30 H a : More than 30 percent of the registered voters in Santa Clara County voted in the primary election. p > 30
A medical trial is conducted to test whether or not a new medicine reduces cholesterol by 25 percent. State the null and alternative hypotheses.
Example 9.2
We want to test whether the mean GPA of students in American colleges is different from 2.0 (out of 4.0). The null and alternative hypotheses are the following: H 0 : μ = 2.0 H a : μ ≠ 2.0
We want to test whether the mean height of eighth graders is 66 inches. State the null and alternative hypotheses. Fill in the correct symbol (=, ≠, ≥, <, ≤, >) for the null and alternative hypotheses.
- H 0 : μ __ 66
- H a : μ __ 66
Example 9.3
We want to test if college students take fewer than five years to graduate from college, on the average. The null and alternative hypotheses are the following: H 0 : μ ≥ 5 H a : μ < 5
We want to test if it takes fewer than 45 minutes to teach a lesson plan. State the null and alternative hypotheses. Fill in the correct symbol ( =, ≠, ≥, <, ≤, >) for the null and alternative hypotheses.
- H 0 : μ __ 45
- H a : μ __ 45
Example 9.4
An article on school standards stated that about half of all students in France, Germany, and Israel take advanced placement exams and a third of the students pass. The same article stated that 6.6 percent of U.S. students take advanced placement exams and 4.4 percent pass. Test if the percentage of U.S. students who take advanced placement exams is more than 6.6 percent. State the null and alternative hypotheses. H 0 : p ≤ 0.066 H a : p > 0.066
On a state driver’s test, about 40 percent pass the test on the first try. We want to test if more than 40 percent pass on the first try. Fill in the correct symbol (=, ≠, ≥, <, ≤, >) for the null and alternative hypotheses.
- H 0 : p __ 0.40
- H a : p __ 0.40
Collaborative Exercise
Bring to class a newspaper, some news magazines, and some internet articles. In groups, find articles from which your group can write null and alternative hypotheses. Discuss your hypotheses with the rest of the class.
This book may not be used in the training of large language models or otherwise be ingested into large language models or generative AI offerings without OpenStax's permission.
Want to cite, share, or modify this book? This book uses the Creative Commons Attribution License and you must attribute Texas Education Agency (TEA). The original material is available at: https://www.texasgateway.org/book/tea-statistics . Changes were made to the original material, including updates to art, structure, and other content updates.
Access for free at https://openstax.org/books/statistics/pages/1-introduction
- Authors: Barbara Illowsky, Susan Dean
- Publisher/website: OpenStax
- Book title: Statistics
- Publication date: Mar 27, 2020
- Location: Houston, Texas
- Book URL: https://openstax.org/books/statistics/pages/1-introduction
- Section URL: https://openstax.org/books/statistics/pages/9-1-null-and-alternative-hypotheses
© Apr 16, 2024 Texas Education Agency (TEA). The OpenStax name, OpenStax logo, OpenStax book covers, OpenStax CNX name, and OpenStax CNX logo are not subject to the Creative Commons license and may not be reproduced without the prior and express written consent of Rice University.
- Key Differences
Know the Differences & Comparisons
Difference Between Null and Alternative Hypothesis
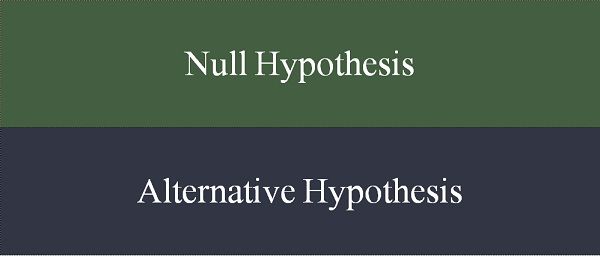
Null hypothesis implies a statement that expects no difference or effect. On the contrary, an alternative hypothesis is one that expects some difference or effect. Null hypothesis This article excerpt shed light on the fundamental differences between null and alternative hypothesis.
Content: Null Hypothesis Vs Alternative Hypothesis
Comparison chart.
Basis for Comparison | Null Hypothesis | Alternative Hypothesis |
---|---|---|
Meaning | A null hypothesis is a statement, in which there is no relationship between two variables. | An alternative hypothesis is statement in which there is some statistical significance between two measured phenomenon. |
Represents | No observed effect | Some observed effect |
What is it? | It is what the researcher tries to disprove. | It is what the researcher tries to prove. |
Acceptance | No changes in opinions or actions | Changes in opinions or actions |
Testing | Indirect and implicit | Direct and explicit |
Observations | Result of chance | Result of real effect |
Denoted by | H-zero | H-one |
Mathematical formulation | Equal sign | Unequal sign |
Definition of Null Hypothesis
A null hypothesis is a statistical hypothesis in which there is no significant difference exist between the set of variables. It is the original or default statement, with no effect, often represented by H 0 (H-zero). It is always the hypothesis that is tested. It denotes the certain value of population parameter such as µ, s, p. A null hypothesis can be rejected, but it cannot be accepted just on the basis of a single test.
Definition of Alternative Hypothesis
A statistical hypothesis used in hypothesis testing, which states that there is a significant difference between the set of variables. It is often referred to as the hypothesis other than the null hypothesis, often denoted by H 1 (H-one). It is what the researcher seeks to prove in an indirect way, by using the test. It refers to a certain value of sample statistic, e.g., x¯, s, p
The acceptance of alternative hypothesis depends on the rejection of the null hypothesis i.e. until and unless null hypothesis is rejected, an alternative hypothesis cannot be accepted.
Key Differences Between Null and Alternative Hypothesis
The important points of differences between null and alternative hypothesis are explained as under:
- A null hypothesis is a statement, in which there is no relationship between two variables. An alternative hypothesis is a statement; that is simply the inverse of the null hypothesis, i.e. there is some statistical significance between two measured phenomenon.
- A null hypothesis is what, the researcher tries to disprove whereas an alternative hypothesis is what the researcher wants to prove.
- A null hypothesis represents, no observed effect whereas an alternative hypothesis reflects, some observed effect.
- If the null hypothesis is accepted, no changes will be made in the opinions or actions. Conversely, if the alternative hypothesis is accepted, it will result in the changes in the opinions or actions.
- As null hypothesis refers to population parameter, the testing is indirect and implicit. On the other hand, the alternative hypothesis indicates sample statistic, wherein, the testing is direct and explicit.
- A null hypothesis is labelled as H 0 (H-zero) while an alternative hypothesis is represented by H 1 (H-one).
- The mathematical formulation of a null hypothesis is an equal sign but for an alternative hypothesis is not equal to sign.
- In null hypothesis, the observations are the outcome of chance whereas, in the case of the alternative hypothesis, the observations are an outcome of real effect.
There are two outcomes of a statistical test, i.e. first, a null hypothesis is rejected and alternative hypothesis is accepted, second, null hypothesis is accepted, on the basis of the evidence. In simple terms, a null hypothesis is just opposite of alternative hypothesis.
You Might Also Like:
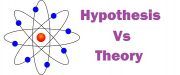
Zipporah Thuo says
February 22, 2018 at 6:06 pm
The comparisons between the two hypothesis i.e Null hypothesis and the Alternative hypothesis are the best.Thank you.
Getu Gamo says
March 4, 2019 at 3:42 am
Thank you so much for the detail explanation on two hypotheses. Now I understood both very well, including their differences.
Jyoti Bhardwaj says
May 28, 2019 at 6:26 am
Thanks, Surbhi! Appreciate the clarity and precision of this content.
January 9, 2020 at 6:16 am
John Jenstad says
July 20, 2020 at 2:52 am
Thanks very much, Surbhi, for your clear explanation!!
Navita says
July 2, 2021 at 11:48 am
Thanks for the Comparison chart! it clears much of my doubt.
GURU UPPALA says
July 21, 2022 at 8:36 pm
Thanks for the Comparison chart!
Enock kipkoech says
September 22, 2022 at 1:57 pm
What are the examples of null hypothesis and substantive hypothesis
Leave a Reply Cancel reply
Your email address will not be published. Required fields are marked *
Save my name, email, and website in this browser for the next time I comment.
If you could change one thing about college, what would it be?
Graduate faster
Better quality online classes
Flexible schedule
Access to top-rated instructors
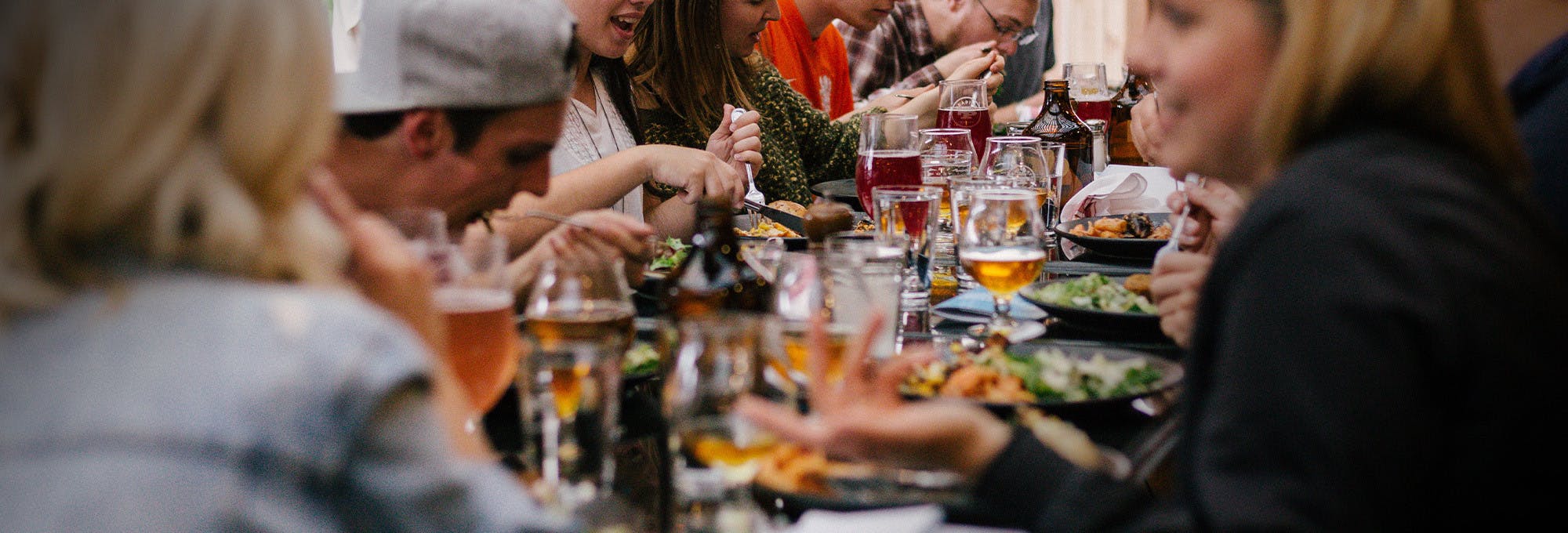
Null vs. Alternative Hypothesis
04.28.2023 • 5 min read
Sarah Thomas
Subject Matter Expert
Learn about a null versus alternative hypothesis and what they show with examples for each. Also go over the main differences and similarities between them.
In This Article
What Is a Null Hypothesis?
What is an alternative hypothesis, outcomes of a hypothesis test.
Main Differences Between Null & Alternative Hypothesis
Similarities Between Null & Alternative Hypothesis
Hypothesis Testing & Errors
In statistics, you’ll draw insights or “inferences” about population parameters using data from a sample. This process is called inferential statistics.
To make statistical inferences, you need to determine if you have enough evidence to support a certain hypothesis about the population. This is where null and alternative hypotheses come into play!
In this article, we’ll explain the differences between these two types of hypotheses, and we’ll explain the role they play in hypothesis testing.
Imagine you want to know what percent of Americans are vegetarians. You find a Gallup poll claiming 5% of the population was vegetarian in 2018, but your intuition tells you vegetarianism is on the rise and that far more than 5% of Americans are vegetarian today.
To investigate further, you collect your own sample data by surveying 1,000 randomly selected Americans. You’ll use this random sample to determine whether it’s likely the true population proportion of vegetarians is, in fact, 5% (as the Gallup data suggests) or whether it could be the case that the percentage of vegetarians is now higher.
Notice that your investigation involves two rival hypotheses about the population. One hypothesis is that the proportion of vegetarians is 5%. The other hypothesis is that the proportion of vegetarians is greater than 5%. In statistics, we would call the first hypothesis the null hypothesis, and the second hypothesis the alternative hypothesis. The null hypothesis ( H 0 H_0 H 0 ) represents the status quo or what is assumed to be true about the population at the start of your investigation.
Null Hypothesis
In hypothesis testing, the null hypothesis ( H 0 H_0 H 0 ) is the default hypothesis.
It's what the status quo assumes to be true about the population.
The alternative hypothesis ( H a H_a H a or H 1 H_1 H 1 ) is the hypothesis that stands contrary to the null hypothesis. The alternative hypothesis represents the research hypothesis—what you as the statistician are trying to prove with your data .
In medical studies, where scientists are trying to demonstrate whether a treatment has a significant effect on patient outcomes, the alternative hypothesis represents the hypothesis that the treatment does have an effect, while the null hypothesis represents the assumption that the treatment has no effect.
Alternative Hypothesis
The alternative hypothesis ( H a H_a H a or H 1 H_1 H 1 ) is the hypothesis being proposed in opposition to the null hypothesis.
Examples of Null and Alternative Hypotheses
In a hypothesis test, the null and alternative hypotheses must be mutually exclusive statements, meaning both hypotheses cannot be true at the same time. For example, if the null hypothesis includes an equal sign, the alternative hypothesis must state that the values being mentioned are “not equal” in some way.
Your hypotheses will also depend on the formulation of your test—are you running a one-sample T-test, a two-sample T-test, F-test for ANOVA , or a Chi-squared test? It also matters whether you are conducting a directional one-tailed test or a nondirectional two-tailed test.
Example 1: Two-Tailed T-test
Null Hypothesis: The population mean is equal to some number, x. 𝝁 = x
Alternative Hypothesis: The population mean is not equal to x. 𝝁 ≠ x
Example 2: One-tailed T-test (Right-Tailed)
Null Hypothesis: The population mean is less than or equal to some number, x. 𝝁 ≤ x Alternative Hypothesis: The population mean is greater than x. 𝝁 > x
Example 3: One-tailed T-test (Left-Tailed)
Null Hypothesis: The population mean is greater than or equal to some number, x. 𝝁 ≥ x
Alternative Hypothesis: The population mean is less than x. 𝝁 < x
By the end of a hypothesis test, you will have reached one of two conclusions.
You will run into either 2 outcomes:
Fail to reject the null hypothesis on the grounds that there's insufficient evidence to move away from the null hypothesis
Reject the null hypothesis in favor of the alternative.
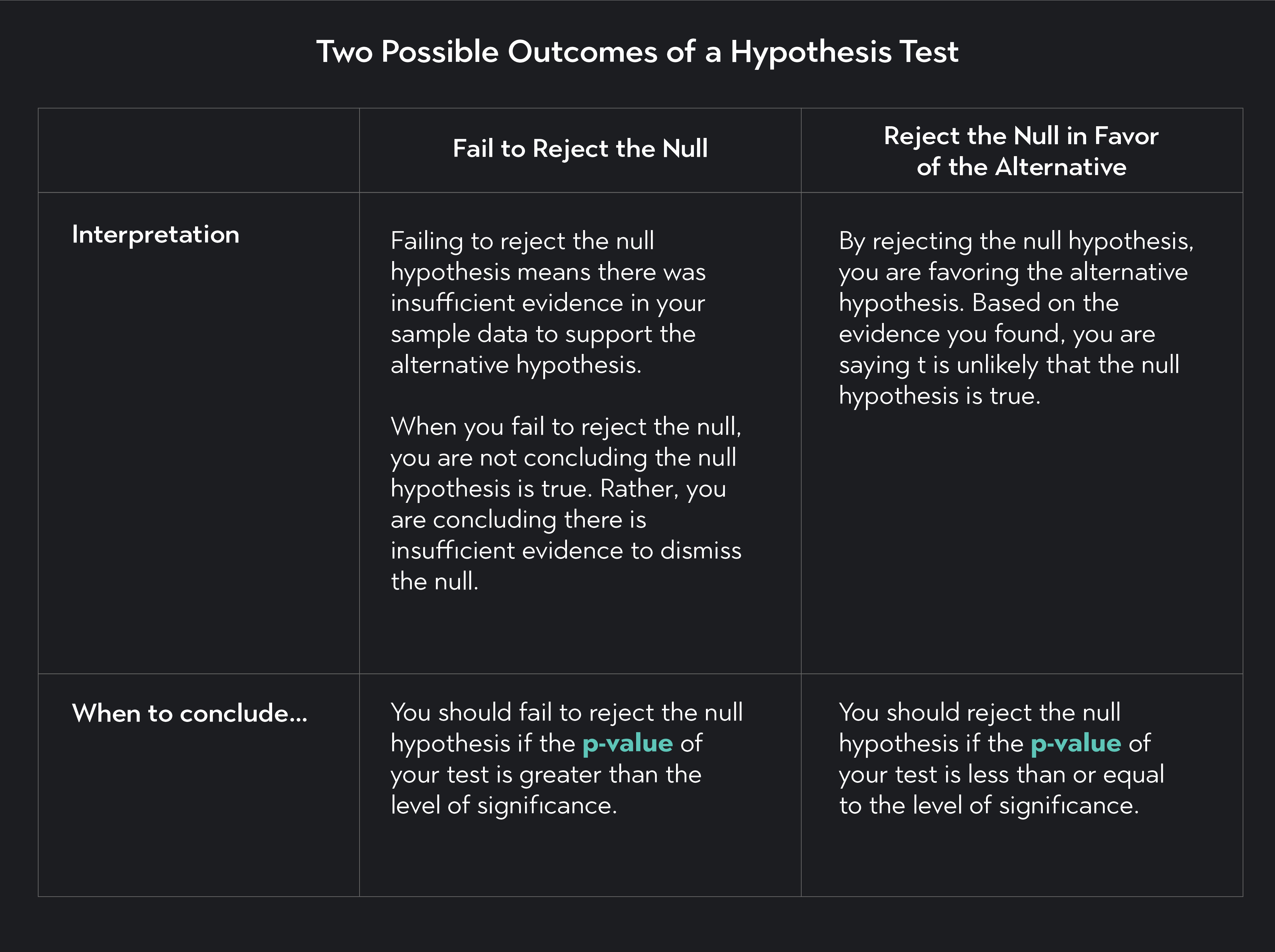
If you’re confused about the outcomes of a hypothesis test, a good analogy is a jury trial. In a jury trial, the defendant is innocent until proven guilty. To reach a verdict of guilt, the jury must find strong evidence (beyond a reasonable doubt) that the defendant committed the crime.
This is analogous to a statistician who must assume the null hypothesis is true unless they can uncover strong evidence ( a p-value less than or equal to the significance level) in support of the alternative hypothesis.
Notice also, that a jury never concludes a defendant is innocent—only that the defendant is guilty or not guilty. This is similar to how we never conclude that the null hypothesis is true. In a hypothesis test, we never conclude that the null hypothesis is true. We can only “reject” the null hypothesis or “fail to reject” it.
In this video, let’s look at the jury example again, the reasoning behind hypothesis testing, and how to form a test. It starts by stating your null and alternative hypotheses.
Main Differences Between Null and Alternative Hypothesis
Here is a summary of the key differences between the null and the alternative hypothesis test.
The null hypothesis represents the status quo; the alternative hypothesis represents an alternative statement about the population.
The null and the alternative are mutually exclusive statements, meaning both statements cannot be true at the same time.
In a medical study, the null hypothesis represents the assumption that a treatment has no statistically significant effect on the outcome being studied. The alternative hypothesis represents the belief that the treatment does have an effect.
The null hypothesis is denoted by H_0 ; the alternative hypothesis is denoted by H_a H_1
You “fail to reject” the null hypothesis when the p-value is larger than the significance level. You “reject” the null hypothesis in favor of the alternative hypothesis when the p-value is less than or equal to your test’s significance level.
Similarities Between Null and Alternative Hypothesis
The similarities between the null and alternative hypotheses are as follows.
Both the null and the alternative are statements about the same underlying data.
Both statements provide a possible answer to a statistician’s research question.
The same hypothesis test will provide evidence for or against the null and alternative hypotheses.
Hypothesis Testing and Errors
Always remember that statistical inference provides you with inferences based on probability rather than hard truths. Anytime you conduct a hypothesis test, there is a chance that you’ll reach the wrong conclusion about your data.
In statistics, we categorize these wrong conclusions into two types of errors:
Type I Errors
Type II Errors
Type I Error (ɑ)
A Type I error occurs when you reject the null hypothesis when, in fact, the null hypothesis is true. This is sometimes called a false positive and is analogous to a jury that falsely convicts an innocent defendant. The probability of making this type of error is represented by alpha, ɑ.
Type II Error (ꞵ)
A Type II error occurs when you fail to reject the null hypothesis when, in fact, the null hypothesis is false. This is sometimes called a false negative and is analogous to a jury that reaches a verdict of “not guilty,” when, in fact, the defendant has committed the crime. The probability of making this type of error is represented by beta, ꞵ.
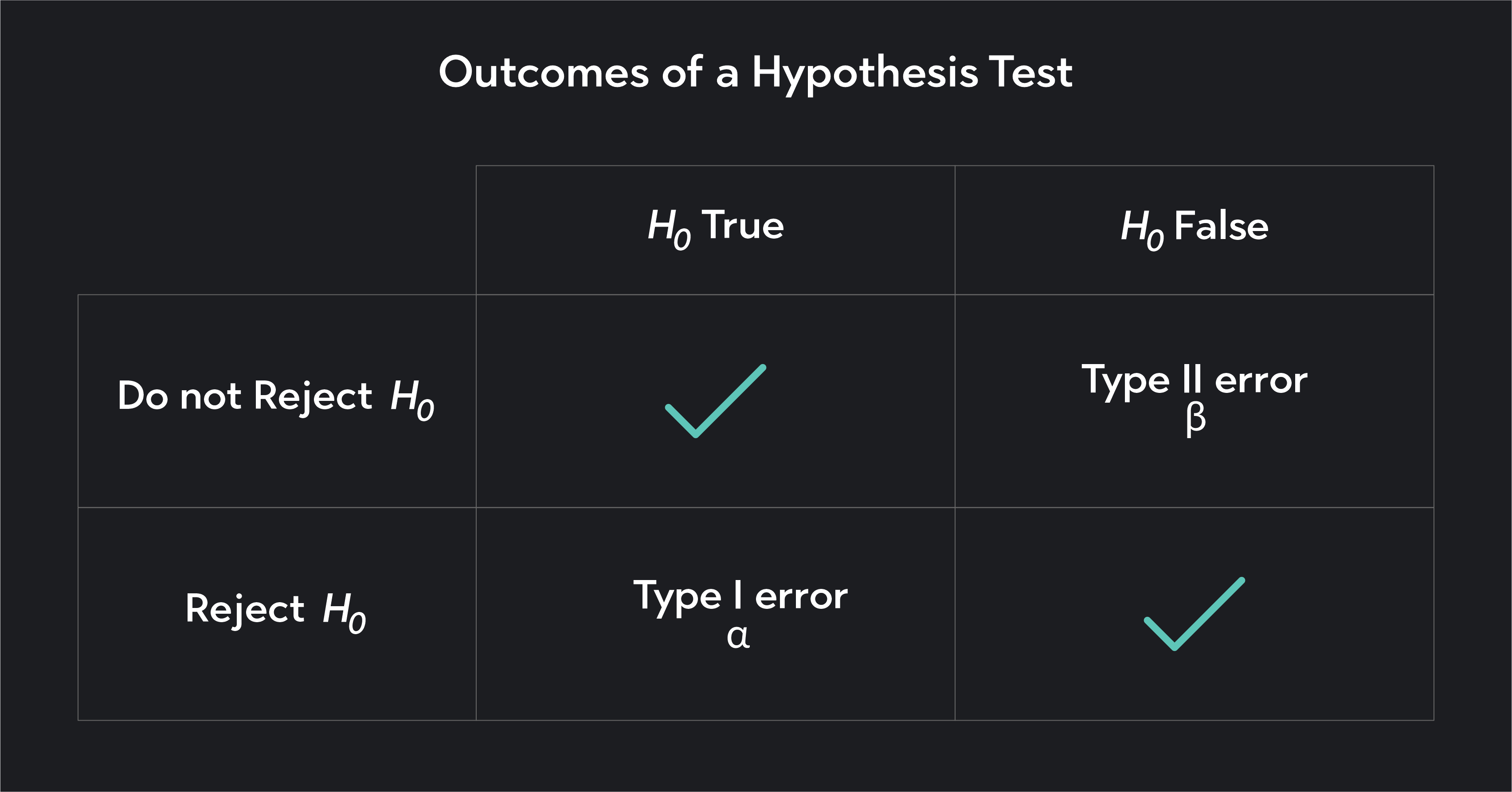
Explore Outlier's Award-Winning For-Credit Courses
Outlier (from the co-founder of MasterClass) has brought together some of the world's best instructors, game designers, and filmmakers to create the future of online college.
Check out these related courses:
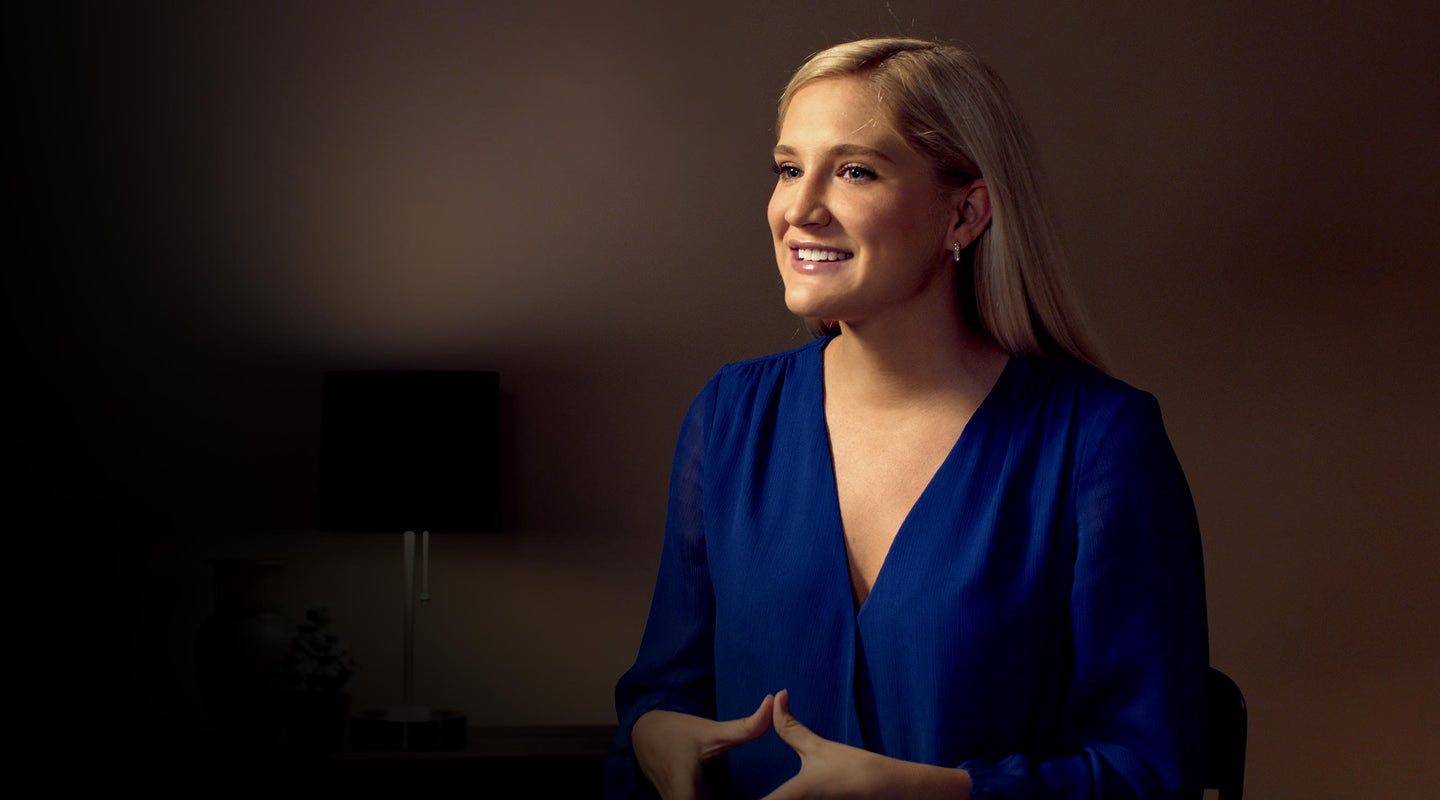
Intro to Statistics
How data describes our world.
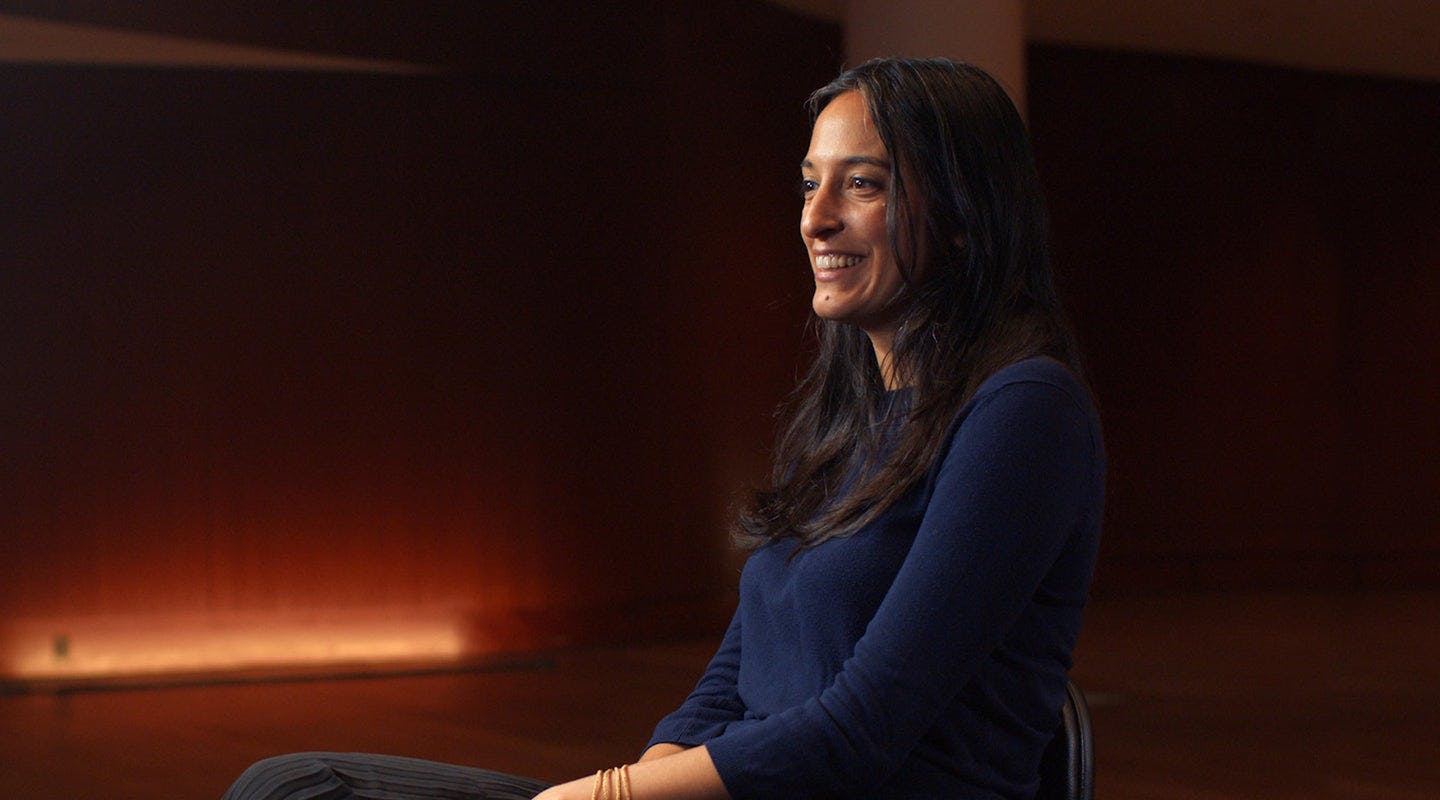
Intro to Microeconomics
Why small choices have big impact.
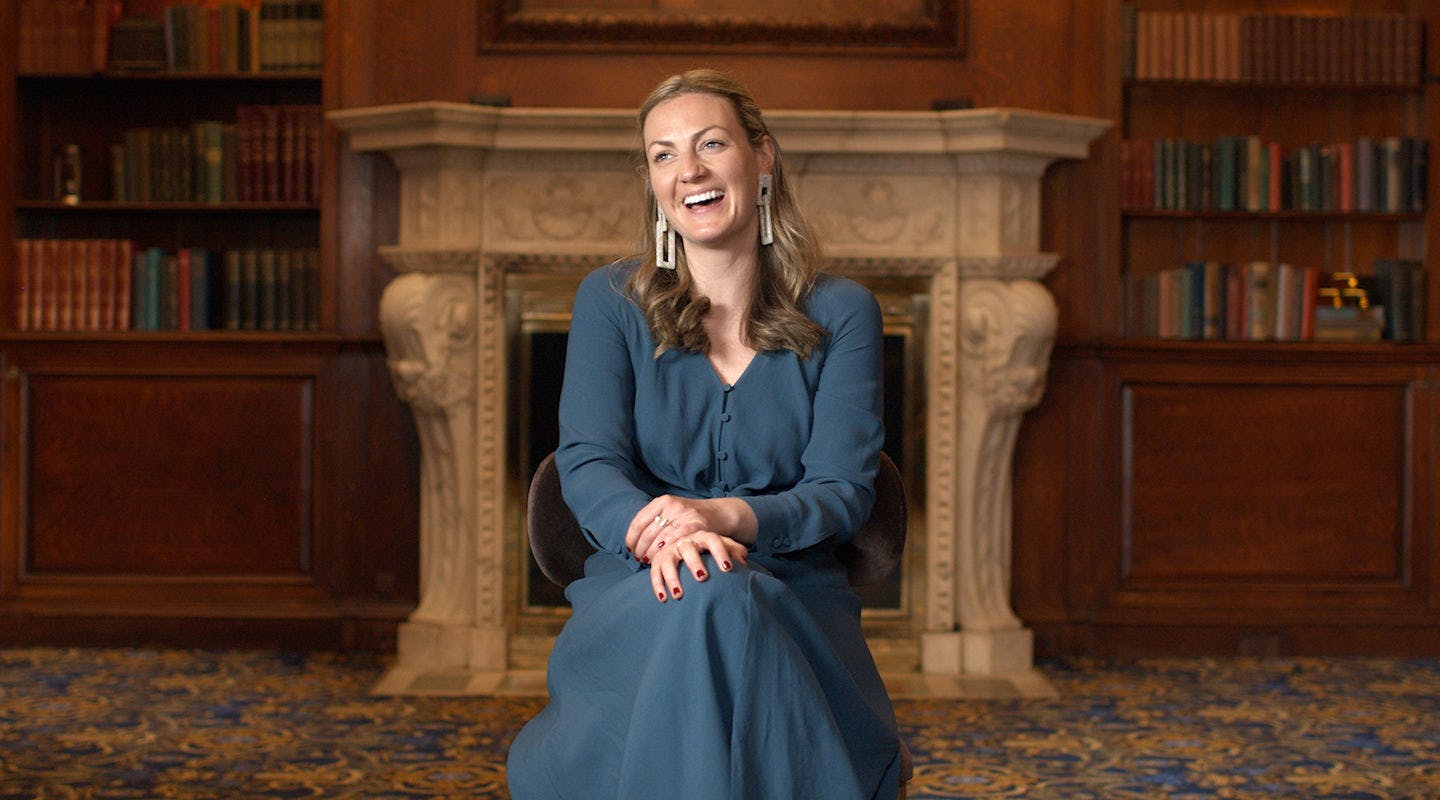
Intro to Macroeconomics
How money moves our world.

Intro to Psychology
The science of the mind.
Related Articles
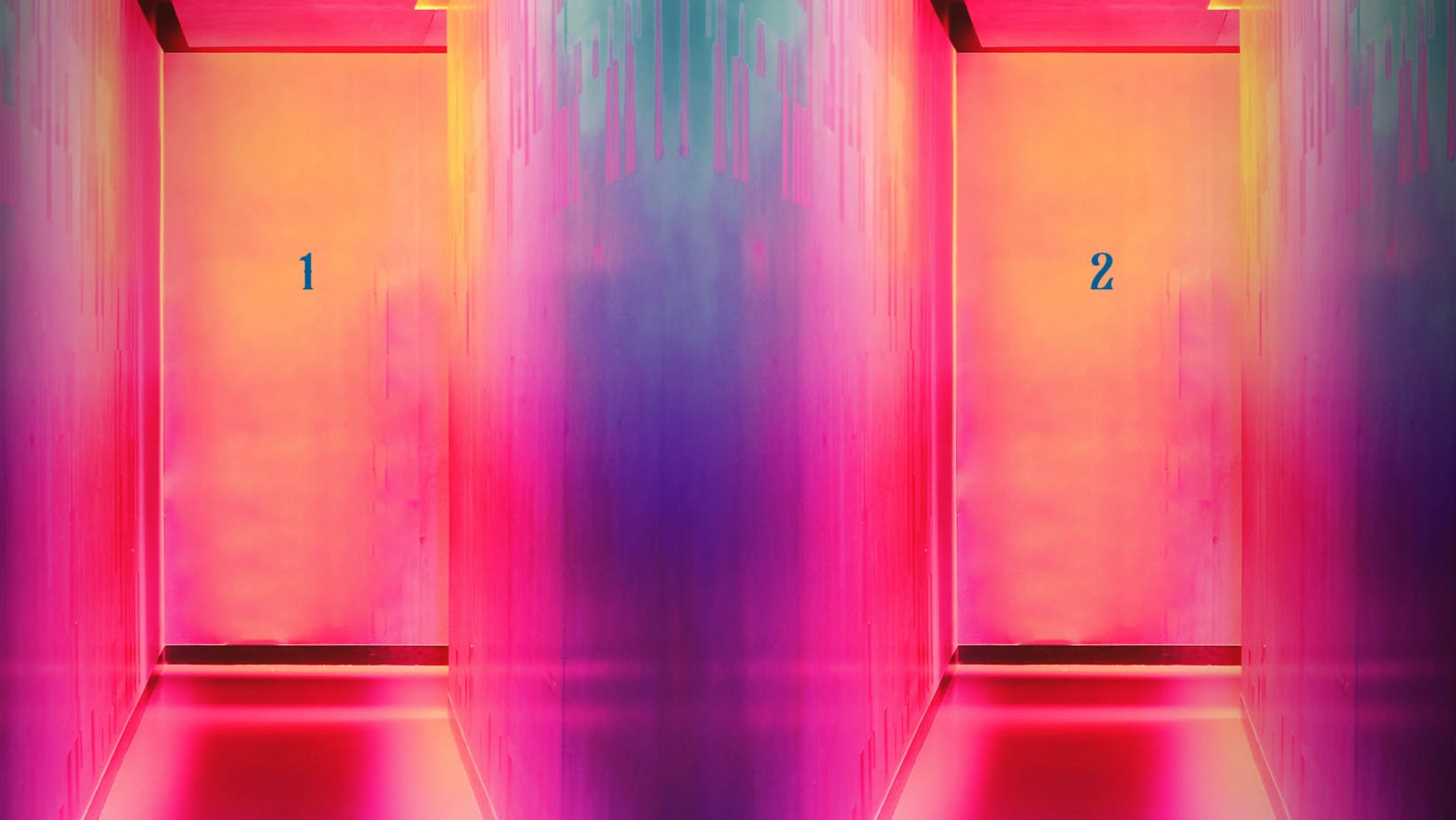
Degrees of Freedom In Statistics
Explore degrees of freedom. Learn about their importance, calculation methods, and two test types. Plus dive into solved examples for better understanding.
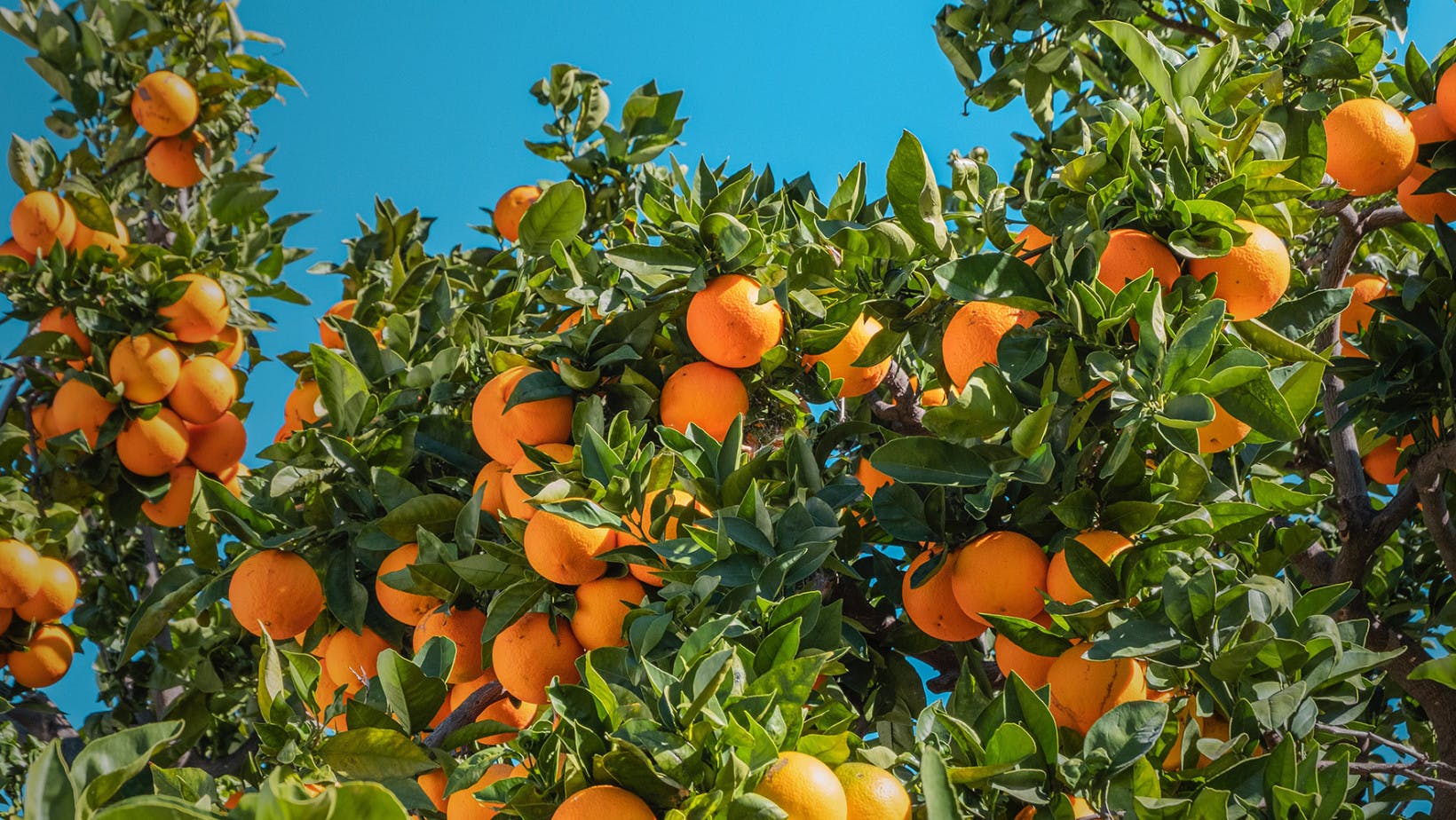
What Is Standard Error? Statistics Calculation and Overview
Learn what is standard error in statistics. This overview explains the definition, the process, the difference with standard deviation, and includes examples.
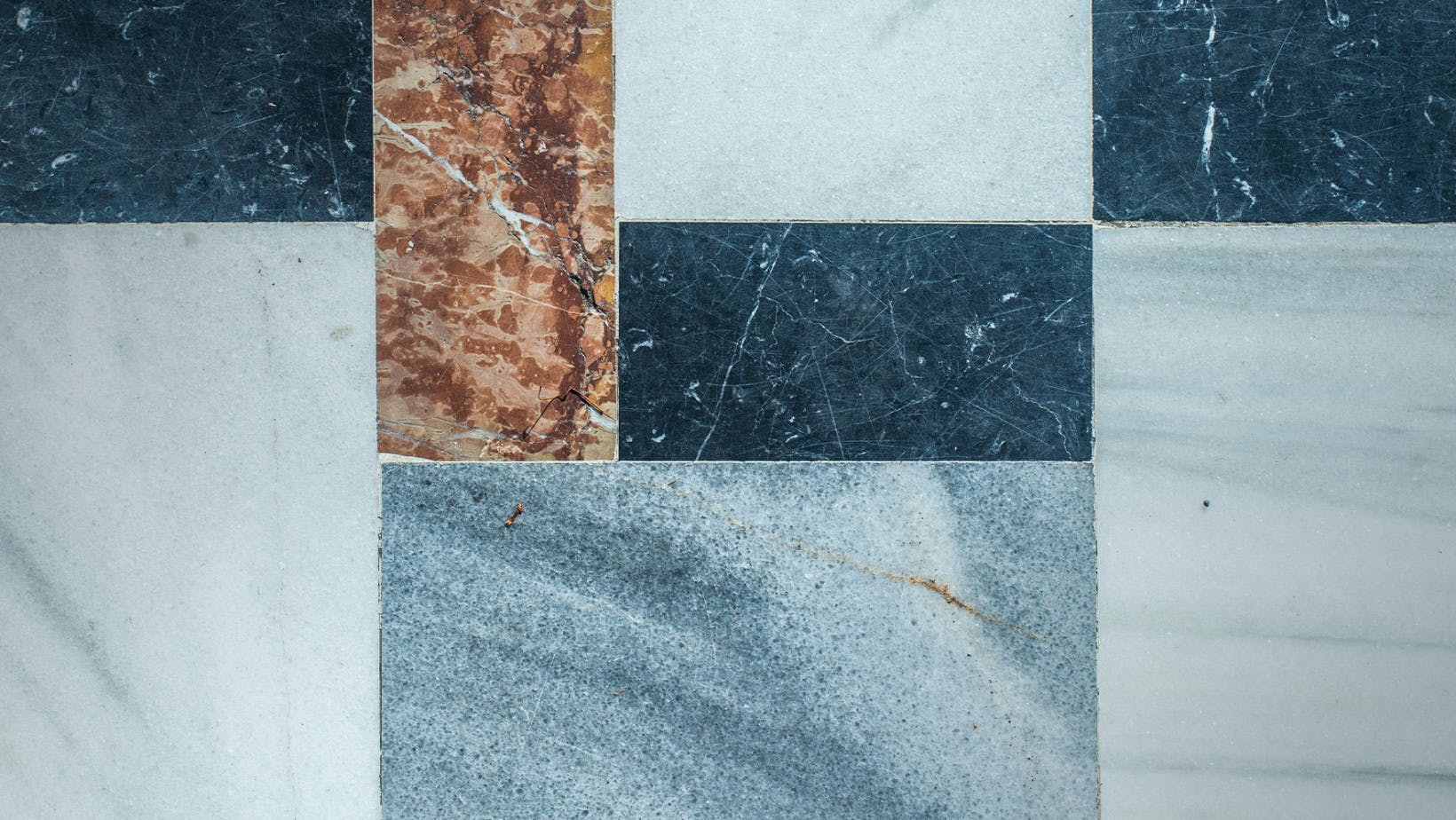
What Are Quartiles? Statistics 101
Learn what quartiles are and how they work in statistics. Understand how to calculate them and why even learn them.
Further Reading
What is the second derivative test [full guide], how to find derivatives in 3 steps, test statistics: definition, formulas & examples, what is the product rule [with examples], understanding math probability - definition, formula & how to find it, what is a residual in stats.
Null vs. Alternative Hypothesis: Key Differences and Examples Explained
In this article you will learn difference between Null and Alternative Hypothesis with examples.
The null and alternative hypotheses are two opposing assertions that researchers use a statistical test to analyze evidence for and against:
The alternative and null hypotheses provide conflicting answers to your research question.
Null Hypothesis
Alternative hypothesis, null hypothesis and alternative hypothesis.
Does following a healthy lifestyle ensure happiness? | A healthy lifestyle has no effect on happiness. | A healthy lifestyle has an effect on happiness. |
Does the attendance of student affect his performance in exams? | The attendance of student has in his performance in exams. | The attendance of student in the textbook has an in his performance in exams. |
Does daily exercise decrease the panic attacks? | Daily exercise the incidence of panic attacks. | Daily exercise the panic attacks. |
Difference between Null and Alternative Hypothesis
No relationship between two variables | There is a relationship between two variables |
Researcher tries to disprove | Researcher tries to prove |
H-null, H-zero, or H | H or H |
=, ≤, ≥ | ≠,<,> |
No effect, No difference, No relationship, No change, Does not increase, Does not decrease | effect, difference, relationship, change, increases, decreases |
Accepted- results of study become insignificant | Accepted- results of study become significant |
Indirect and implicit | Direct and explicit |
P-value is greater than the level of significance | P-value is smaller than the level of significance |
✔ The null hypothesis is a broad statement stating that there is no relationship between two variables in a study or that no association exists between two groups. ✔ An alternate hypothesis , on the other hand, is a statement that describes the existence of a relationship between two variables in a study.
✔ Null Hypothesis is followed by a ‘equals to’ sign in a mathematical expression. ✔ The sign not equals to, ‘less than,’ or ‘greater than’ follows Alternate Hypothesis .
✔ The null hypothesis is accepted if the p-value is greater than the level of significance. ✔ if the p-value is less than the level of significance, the al ternative hypothesis is accepted.
Examples of null hypothesis and an alternative hypothesis
There is a difference in work ethic values between American and Asian employees. μAM denotes the mean work ethic value of Americans, and μAS denotes the mean work ethic value of Asians.
Similarities between Null Hypothesis and an Alternative Hypothesis
✔ Both are answers to the research question. ✔ They make population-related assertions. ✔ Statistical tests are used to examine null and alternative hypothesis.
Other articles
Related posts, types of research questions, correlational research | example, types, inductive vs deductive approach: which is more effective, 8 types of validity in research | examples, nominal, ordinal, interval, and ratio scales | measurement of scale, operationalization of variables in research | examples | benefits, types of quasi experimental design, difference between experimental and non-experimental research, 10 types of variables in research: definitions and examples, types of descriptive research: methods and examples.
Hypothesis Testing (cont...)
Hypothesis testing, the null and alternative hypothesis.
In order to undertake hypothesis testing you need to express your research hypothesis as a null and alternative hypothesis. The null hypothesis and alternative hypothesis are statements regarding the differences or effects that occur in the population. You will use your sample to test which statement (i.e., the null hypothesis or alternative hypothesis) is most likely (although technically, you test the evidence against the null hypothesis). So, with respect to our teaching example, the null and alternative hypothesis will reflect statements about all statistics students on graduate management courses.
The null hypothesis is essentially the "devil's advocate" position. That is, it assumes that whatever you are trying to prove did not happen ( hint: it usually states that something equals zero). For example, the two different teaching methods did not result in different exam performances (i.e., zero difference). Another example might be that there is no relationship between anxiety and athletic performance (i.e., the slope is zero). The alternative hypothesis states the opposite and is usually the hypothesis you are trying to prove (e.g., the two different teaching methods did result in different exam performances). Initially, you can state these hypotheses in more general terms (e.g., using terms like "effect", "relationship", etc.), as shown below for the teaching methods example:
Null Hypotheses (H ): | Undertaking seminar classes has no effect on students' performance. |
Alternative Hypothesis (H ): | Undertaking seminar class has a positive effect on students' performance. |
Depending on how you want to "summarize" the exam performances will determine how you might want to write a more specific null and alternative hypothesis. For example, you could compare the mean exam performance of each group (i.e., the "seminar" group and the "lectures-only" group). This is what we will demonstrate here, but other options include comparing the distributions , medians , amongst other things. As such, we can state:
Null Hypotheses (H ): | The mean exam mark for the "seminar" and "lecture-only" teaching methods is the same in the population. |
Alternative Hypothesis (H ): | The mean exam mark for the "seminar" and "lecture-only" teaching methods is not the same in the population. |
Now that you have identified the null and alternative hypotheses, you need to find evidence and develop a strategy for declaring your "support" for either the null or alternative hypothesis. We can do this using some statistical theory and some arbitrary cut-off points. Both these issues are dealt with next.
Significance levels
The level of statistical significance is often expressed as the so-called p -value . Depending on the statistical test you have chosen, you will calculate a probability (i.e., the p -value) of observing your sample results (or more extreme) given that the null hypothesis is true . Another way of phrasing this is to consider the probability that a difference in a mean score (or other statistic) could have arisen based on the assumption that there really is no difference. Let us consider this statement with respect to our example where we are interested in the difference in mean exam performance between two different teaching methods. If there really is no difference between the two teaching methods in the population (i.e., given that the null hypothesis is true), how likely would it be to see a difference in the mean exam performance between the two teaching methods as large as (or larger than) that which has been observed in your sample?
So, you might get a p -value such as 0.03 (i.e., p = .03). This means that there is a 3% chance of finding a difference as large as (or larger than) the one in your study given that the null hypothesis is true. However, you want to know whether this is "statistically significant". Typically, if there was a 5% or less chance (5 times in 100 or less) that the difference in the mean exam performance between the two teaching methods (or whatever statistic you are using) is as different as observed given the null hypothesis is true, you would reject the null hypothesis and accept the alternative hypothesis. Alternately, if the chance was greater than 5% (5 times in 100 or more), you would fail to reject the null hypothesis and would not accept the alternative hypothesis. As such, in this example where p = .03, we would reject the null hypothesis and accept the alternative hypothesis. We reject it because at a significance level of 0.03 (i.e., less than a 5% chance), the result we obtained could happen too frequently for us to be confident that it was the two teaching methods that had an effect on exam performance.
Whilst there is relatively little justification why a significance level of 0.05 is used rather than 0.01 or 0.10, for example, it is widely used in academic research. However, if you want to be particularly confident in your results, you can set a more stringent level of 0.01 (a 1% chance or less; 1 in 100 chance or less).
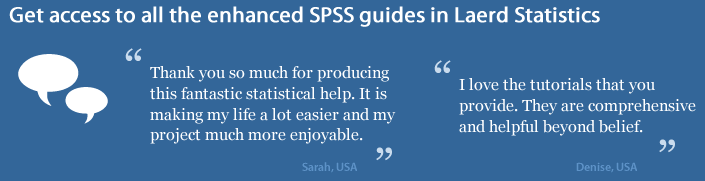
One- and two-tailed predictions
When considering whether we reject the null hypothesis and accept the alternative hypothesis, we need to consider the direction of the alternative hypothesis statement. For example, the alternative hypothesis that was stated earlier is:
Alternative Hypothesis (H ): | Undertaking seminar classes has a positive effect on students' performance. |
The alternative hypothesis tells us two things. First, what predictions did we make about the effect of the independent variable(s) on the dependent variable(s)? Second, what was the predicted direction of this effect? Let's use our example to highlight these two points.
Sarah predicted that her teaching method (independent variable: teaching method), whereby she not only required her students to attend lectures, but also seminars, would have a positive effect (that is, increased) students' performance (dependent variable: exam marks). If an alternative hypothesis has a direction (and this is how you want to test it), the hypothesis is one-tailed. That is, it predicts direction of the effect. If the alternative hypothesis has stated that the effect was expected to be negative, this is also a one-tailed hypothesis.
Alternatively, a two-tailed prediction means that we do not make a choice over the direction that the effect of the experiment takes. Rather, it simply implies that the effect could be negative or positive. If Sarah had made a two-tailed prediction, the alternative hypothesis might have been:
Alternative Hypothesis (H ): | Undertaking seminar classes has an effect on students' performance. |
In other words, we simply take out the word "positive", which implies the direction of our effect. In our example, making a two-tailed prediction may seem strange. After all, it would be logical to expect that "extra" tuition (going to seminar classes as well as lectures) would either have a positive effect on students' performance or no effect at all, but certainly not a negative effect. However, this is just our opinion (and hope) and certainly does not mean that we will get the effect we expect. Generally speaking, making a one-tail prediction (i.e., and testing for it this way) is frowned upon as it usually reflects the hope of a researcher rather than any certainty that it will happen. Notable exceptions to this rule are when there is only one possible way in which a change could occur. This can happen, for example, when biological activity/presence in measured. That is, a protein might be "dormant" and the stimulus you are using can only possibly "wake it up" (i.e., it cannot possibly reduce the activity of a "dormant" protein). In addition, for some statistical tests, one-tailed tests are not possible.
Rejecting or failing to reject the null hypothesis
Let's return finally to the question of whether we reject or fail to reject the null hypothesis.
If our statistical analysis shows that the significance level is below the cut-off value we have set (e.g., either 0.05 or 0.01), we reject the null hypothesis and accept the alternative hypothesis. Alternatively, if the significance level is above the cut-off value, we fail to reject the null hypothesis and cannot accept the alternative hypothesis. You should note that you cannot accept the null hypothesis, but only find evidence against it.
Null Hypothesis and Alternative Hypothesis
- Inferential Statistics
- Statistics Tutorials
- Probability & Games
- Descriptive Statistics
- Applications Of Statistics
- Math Tutorials
- Pre Algebra & Algebra
- Exponential Decay
- Worksheets By Grade
- Ph.D., Mathematics, Purdue University
- M.S., Mathematics, Purdue University
- B.A., Mathematics, Physics, and Chemistry, Anderson University
Hypothesis testing involves the careful construction of two statements: the null hypothesis and the alternative hypothesis. These hypotheses can look very similar but are actually different.
How do we know which hypothesis is the null and which one is the alternative? We will see that there are a few ways to tell the difference.
The Null Hypothesis
The null hypothesis reflects that there will be no observed effect in our experiment. In a mathematical formulation of the null hypothesis, there will typically be an equal sign. This hypothesis is denoted by H 0 .
The null hypothesis is what we attempt to find evidence against in our hypothesis test. We hope to obtain a small enough p-value that it is lower than our level of significance alpha and we are justified in rejecting the null hypothesis. If our p-value is greater than alpha, then we fail to reject the null hypothesis.
If the null hypothesis is not rejected, then we must be careful to say what this means. The thinking on this is similar to a legal verdict. Just because a person has been declared "not guilty", it does not mean that he is innocent. In the same way, just because we failed to reject a null hypothesis it does not mean that the statement is true.
For example, we may want to investigate the claim that despite what convention has told us, the mean adult body temperature is not the accepted value of 98.6 degrees Fahrenheit . The null hypothesis for an experiment to investigate this is “The mean adult body temperature for healthy individuals is 98.6 degrees Fahrenheit.” If we fail to reject the null hypothesis, then our working hypothesis remains that the average adult who is healthy has a temperature of 98.6 degrees. We do not prove that this is true.
If we are studying a new treatment, the null hypothesis is that our treatment will not change our subjects in any meaningful way. In other words, the treatment will not produce any effect in our subjects.
The Alternative Hypothesis
The alternative or experimental hypothesis reflects that there will be an observed effect for our experiment. In a mathematical formulation of the alternative hypothesis, there will typically be an inequality, or not equal to symbol. This hypothesis is denoted by either H a or by H 1 .
The alternative hypothesis is what we are attempting to demonstrate in an indirect way by the use of our hypothesis test. If the null hypothesis is rejected, then we accept the alternative hypothesis. If the null hypothesis is not rejected, then we do not accept the alternative hypothesis. Going back to the above example of mean human body temperature, the alternative hypothesis is “The average adult human body temperature is not 98.6 degrees Fahrenheit.”
If we are studying a new treatment, then the alternative hypothesis is that our treatment does, in fact, change our subjects in a meaningful and measurable way.
The following set of negations may help when you are forming your null and alternative hypotheses. Most technical papers rely on just the first formulation, even though you may see some of the others in a statistics textbook.
- Null hypothesis: “ x is equal to y .” Alternative hypothesis “ x is not equal to y .”
- Null hypothesis: “ x is at least y .” Alternative hypothesis “ x is less than y .”
- Null hypothesis: “ x is at most y .” Alternative hypothesis “ x is greater than y .”
- What 'Fail to Reject' Means in a Hypothesis Test
- Type I and Type II Errors in Statistics
- An Example of a Hypothesis Test
- The Runs Test for Random Sequences
- An Example of Chi-Square Test for a Multinomial Experiment
- The Difference Between Type I and Type II Errors in Hypothesis Testing
- What Level of Alpha Determines Statistical Significance?
- What Is the Difference Between Alpha and P-Values?
- What Is ANOVA?
- How to Find Critical Values with a Chi-Square Table
- Example of a Permutation Test
- Degrees of Freedom for Independence of Variables in Two-Way Table
- Example of an ANOVA Calculation
- How to Find Degrees of Freedom in Statistics
- How to Construct a Confidence Interval for a Population Proportion
- Degrees of Freedom in Statistics and Mathematics
Our websites may use cookies to personalize and enhance your experience. By continuing without changing your cookie settings, you agree to this collection. For more information, please see our University Websites Privacy Notice .
Neag School of Education
Educational Research Basics by Del Siegle
Null and alternative hypotheses.
Converting research questions to hypothesis is a simple task. Take the questions and make it a positive statement that says a relationship exists (correlation studies) or a difference exists between the groups (experiment study) and you have the alternative hypothesis. Write the statement such that a relationship does not exist or a difference does not exist and you have the null hypothesis. You can reverse the process if you have a hypothesis and wish to write a research question.
When you are comparing two groups, the groups are the independent variable. When you are testing whether something affects something else, the cause is the independent variable. The independent variable is the one you manipulate.
Teachers given higher pay will have more positive attitudes toward children than teachers given lower pay. The first step is to ask yourself “Are there two or more groups being compared?” The answer is “Yes.” What are the groups? Teachers who are given higher pay and teachers who are given lower pay. The independent variable is teacher pay. The dependent variable (the outcome) is attitude towards school.
You could also approach is another way. “Is something causing something else?” The answer is “Yes.” What is causing what? Teacher pay is causing attitude towards school. Therefore, teacher pay is the independent variable (cause) and attitude towards school is the dependent variable (outcome).
By tradition, we try to disprove (reject) the null hypothesis. We can never prove a null hypothesis, because it is impossible to prove something does not exist. We can disprove something does not exist by finding an example of it. Therefore, in research we try to disprove the null hypothesis. When we do find that a relationship (or difference) exists then we reject the null and accept the alternative. If we do not find that a relationship (or difference) exists, we fail to reject the null hypothesis (and go with it). We never say we accept the null hypothesis because it is never possible to prove something does not exist. That is why we say that we failed to reject the null hypothesis, rather than we accepted it.
Del Siegle, Ph.D. Neag School of Education – University of Connecticut [email protected] www.delsiegle.com
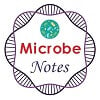
Microbe Notes
Null hypothesis and alternative hypothesis with 9 differences
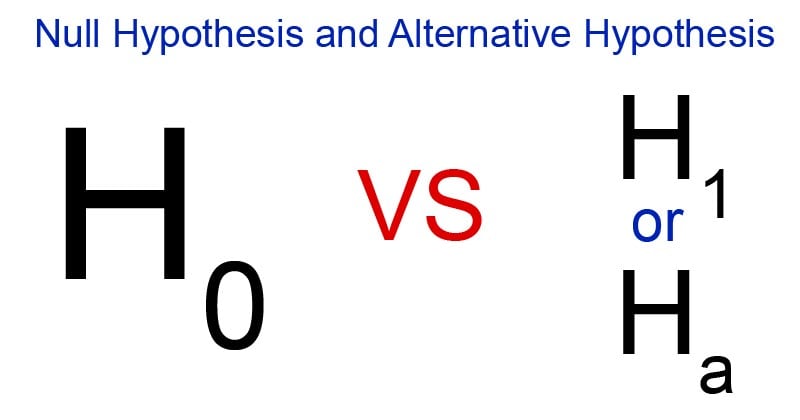
Table of Contents
Interesting Science Videos
Null hypothesis definition
The null hypothesis is a general statement that states that there is no relationship between two phenomenons under consideration or that there is no association between two groups.
- A hypothesis, in general, is an assumption that is yet to be proved with sufficient pieces of evidence. A null hypothesis thus is the hypothesis a researcher is trying to disprove.
- A null hypothesis is a hypothesis capable of being objectively verified, tested, and even rejected.
- If a study is to compare method A with method B about their relationship, and if the study is preceded on the assumption that both methods are equally good, then this assumption is termed as the null hypothesis.
- The null hypothesis should always be a specific hypothesis, i.e., it should not state about or approximately a certain value.
Null hypothesis symbol
- The symbol for the null hypothesis is H 0, and it is read as H-null, H-zero, or H-naught.
- The null hypothesis is usually associated with just ‘equals to’ sign as a null hypothesis can either be accepted or rejected.
Null hypothesis purpose
- The main purpose of a null hypothesis is to verify/ disprove the proposed statistical assumptions.
- Some scientific null hypothesis help to advance a theory.
- The null hypothesis is also used to verify the consistent results of multiple experiments. For e.g., the null hypothesis stating that there is no relation between some medication and age of the patients supports the general effectiveness conclusion, and allows recommendations.
Null hypothesis principle
- The principle of the null hypothesis is collecting the data and determining the chances of the collected data in the study of a random sample, proving that the null hypothesis is true.
- In situations or studies where the collected data doesn’t complete the expectation of the null hypothesis, it is concluded that the data doesn’t provide sufficient or reliable pieces of evidence to support the null hypothesis and thus, it is rejected.
- The data collected is tested through some statistical tool which is designed to measure the extent of departure of the date from the null hypothesis.
- The procedure decides whether the observed departure obtained from the statistical tool is larger than a defined value so that the probability of occurrence of a high departure value is very small under the null hypothesis.
- However, some data might not contradict the null hypothesis which explains that only a weak conclusion can be made and that the data doesn’t provide strong pieces of evidence against the null hypothesis and the null hypothesis might or might not be true.
- Under some other conditions, if the data collected is sufficient and is capable of providing enough evidence, the null hypothesis can be considered valid, indicating no relationship between the phenomena.
When to reject null hypothesis?
- When the p-value of the data is less than the significant level of the test, the null hypothesis is rejected, indicating the test results are significant.
- However, if the p-value is higher than the significant value, the null hypothesis is not rejected, and the results are considered not significant.
- The level of significance is an important concept while hypothesis testing as it determines the percentage risk of rejecting the null hypothesis when H 0 might happen to be true.
- In other words, if we take the level of significance at 5%, it means that the researcher is willing to take as much as a 5 percent risk of rejecting the null hypothesis when it (H 0 ) happens to be true.
- The null hypothesis cannot be accepted because the lack of evidence only means that the relationship is not proven. It doesn’t prove that something doesn’t exist, but it just means that there are not enough shreds of evidence and the study might have missed it.
Null hypothesis examples
The following are some examples of null hypothesis:
- If the hypothesis is that “the consumption of a particular medicine reduces the chances of heart arrest”, the null hypothesis will be “the consumption of the medicine doesn’t reduce the chances of heart arrest.”
- If the hypothesis is that, “If random test scores are collected from men and women, does the score of one group differ from the other?” a possible null hypothesis will be that the mean test score of men is the same as that of the women.
H 0 : µ 1 = µ 2
H 0 = null hypothesis µ 1 = mean score of men µ 2 = mean score of women
Alternative hypothesis definition
An alternative hypothesis is a statement that describes that there is a relationship between two selected variables in a study.
- An alternative hypothesis is usually used to state that a new theory is preferable to the old one (null hypothesis).
- This hypothesis can be simply termed as an alternative to the null hypothesis.
- The alternative hypothesis is the hypothesis that is to be proved that indicates that the results of a study are significant and that the sample observation is not results just from chance but from some non-random cause.
- If a study is to compare method A with method B about their relationship and we assume that the method A is superior or the method B is inferior, then such a statement is termed as an alternative hypothesis.
- Alternative hypotheses should be clearly stated, considering the nature of the research problem.
Alternative hypothesis symbol
- The symbol of the alternative hypothesis is either H 1 or H a while using less than, greater than or not equal signs.
Alternative hypothesis purpose
- An alternative hypothesis provides the researchers with some specific restatements and clarifications of the research problem.
- An alternative hypothesis provides a direction to the study, which then can be utilized by the researcher to obtain the desired results.
- Since the alternative hypothesis is selected before conducting the study, it allows the test to prove that the study is supported by evidence, separating it from the researchers’ desires and values.
- An alternative hypothesis provides a chance of discovering new theories that can disprove an existing one that might not be supported by evidence.
- The alternative hypothesis is important as they prove that a relationship exists between two variables selected and that the results of the study conducted are relevant and significant.
Alternative hypothesis principle
- The principle behind the alternative hypothesis is similar to that of the null hypothesis.
- The alternative hypothesis is based on the concept that when sufficient evidence is collected from the data of random sample, it provides a basis for proving the assumption made by the researcher regarding the study.
- Like in the null hypothesis, the data collected from a random sample is passed through a statistical tool that measures the extent of departure of the data from the null hypothesis.
- If the departure is small under the selected level of significance, the alternative hypothesis is accepted, and the null hypothesis is rejected.
- If the data collected don’t have chances of being in the study of the random sample and are instead decided by the relationship within the sample of the study, an alternative hypothesis stands true.
Alternative hypothesis examples
The following are some examples of alternative hypothesis:
1. If a researcher is assuming that the bearing capacity of a bridge is more than 10 tons, then the hypothesis under this study will be:
Null hypothesis H 0 : µ= 10 tons Alternative hypothesis H a : µ>10 tons
2. Under another study that is trying to test whether there is a significant difference between the effectiveness of medicine against heart arrest, the alternative hypothesis will be that there is a relationship between the medicine and chances of heart arrest.
Null hypothesis vs Alternative hypothesis
The null hypothesis is a general statement that states that there is no relationship between two phenomenons under consideration or that there is no association between two groups. | An alternative hypothesis is a statement that describes that there is a relationship between two selected variables in a study. | |
It is denoted by H . | It is denoted by H or H . | |
It is followed by ‘equals to’ sign. | It is followed by not equals to, ‘less than’ or ‘greater than’ sign. | |
The null hypothesis believes that the results are observed as a result of chance. | The alternative hypothesis believes that the results are observed as a result of some real causes. | |
It is the hypothesis that the researcher tries to disprove. | It is a hypothesis that the researcher tries to prove. | |
The result of the null hypothesis indicates no changes in opinions or actions. | The result of an alternative hypothesis causes changes in opinions and actions. | |
If the null hypothesis is accepted, the results of the study become insignificant. | If an alternative hypothesis is accepted, the results of the study become significant. | |
If the p-value is greater than the level of significance, the null hypothesis is accepted. | If the p-value is smaller than the level of significance, an alternative hypothesis is accepted. | |
The null hypothesis allows the acceptance of correct existing theories and the consistency of multiple experiments. | Alternative hypothesis are important as it establishes a relationship between two variables, resulting in new improved theories. |
- R. Kothari (1990) Research Methodology. Vishwa Prakasan. India.
- https://www.statisticssolutions.com/null-hypothesis-and-alternative-hypothesis/
- https://byjus.com/maths/null-hypothesis/
- https://en.wikipedia.org/wiki/Null_hypothesis
- https://keydifferences.com/difference-between-null-and-alternative-hypothesis.html
- 5% – https://en.wikipedia.org/wiki/Null_hypothesis
- 3% – https://keydifferences.com/difference-between-null-and-alternative-hypothesis.html
- 2% – https://byjus.com/maths/null-hypothesis/
- 1% – https://www.wisdomjobs.com/e-university/research-methodology-tutorial-355/procedure-for-hypothesis-testing-11525.html
- 1% – https://www.thoughtco.com/definition-of-null-hypothesis-and-examples-605436
- 1% – https://www.quora.com/What-are-the-different-types-of-hypothesis-and-what-are-some-examples-of-them
- 1% – https://www.dummies.com/education/math/statistics/what-a-p-value-tells-you-about-statistical-data/
- 1% – https://www.coursehero.com/file/p7jfbal5/These-are-hypotheses-capable-of-being-objectively-verified-and-tested-Thus-we/
- 1% – https://support.minitab.com/en-us/minitab/18/help-and-how-to/modeling-statistics/anova/how-to/one-way-anova/interpret-the-results/all-statistics-and-graphs/methods/
- 1% – https://stats.stackexchange.com/questions/105319/test-whether-there-is-a-significant-difference-between-two-groups
- 1% – https://statisticsbyjim.com/hypothesis-testing/failing-reject-null-hypothesis/
- 1% – https://quizlet.com/45299306/statistics-flash-cards/
- <1% – https://www.thoughtco.com/significance-level-in-hypothesis-testing-1147177
- <1% – https://www.thoughtco.com/null-hypothesis-vs-alternative-hypothesis-3126413
- <1% – https://www.sagepub.com/sites/default/files/upm-binaries/40007_Chapter8.pdf
- <1% – https://www.differencebetween.com/difference-between-hypothesis-and-vs-assumption/
- <1% – https://www.coursehero.com/file/18076181/introduction-to-hypothesis/
- <1% – https://statisticsbyjim.com/glossary/significance-level/
- <1% – https://quizlet.com/164755799/research-methods-midterm-2-flash-cards/
- <1% – https://online.stat.psu.edu/statprogram/reviews/statistical-concepts/hypothesis-testing/p-value-approach
About Author
Anupama Sapkota
Leave a Comment Cancel reply
Save my name, email, and website in this browser for the next time I comment.
Null hypothesis and Alternative Hypothesis
A hypothesis is a proposed explanation for a phenomenon, based on observation, reasoning, or scientific theory, awaiting verification or falsification through experimentation and data analysis. It serves as a starting point for investigation, guiding the research process by suggesting what outcomes to expect. In the realm of statistics and scientific research, hypotheses are crucial for designing experiments, analyzing results, and advancing knowledge.
The null hypothesis and alternative hypothesis are required to be fragmented properly before the data collection and interpretation phase in the research. Well fragmented hypotheses indicate that the researcher has adequate knowledge in that particular area and is thus able to take the investigation further because they can use a much more systematic system. It gives direction to the researcher on his/her collection and interpretation of data.
The null hypothesis and alternative hypothesis are useful only if they state the expected relationship between the variables or if they are consistent with the existing body of knowledge. They should be expressed as simply and concisely as possible. They are useful if they have explanatory power.
The purpose and importance of the null hypothesis and alternative hypothesis are that they provide an approximate description of the phenomena. The purpose is to provide the researcher or an investigator with a relational statement that is directly tested in a research study. The purpose is to provide the framework for reporting the inferences of the study. The purpose is to behave as a working instrument of the theory. The purpose is to prove whether or not the test is supported, which is separated from the investigator’s own values and decisions. They also provide direction to the research.
Need help with your analysis?
Schedule a time to speak with an expert using the calendar below.
The null hypothesis is generally denoted as H0. It states the exact opposite of what an investigator or an experimenter predicts or expects. It basically defines the statement which states that there is no exact or actual relationship between the variables.
The alternative hypothesis is generally denoted as H1. It makes a statement that suggests or advises a potential result or an outcome that an investigator or the researcher may expect. It has been categorized into two categories: directional alternative hypothesis and non directional alternative hypothesis.
The directional hypothesis is a kind that explains the direction of the expected findings. Sometimes this type of alternative hypothesis is developed to examine the relationship among the variables rather than a comparison between the groups.
The non directional hypothesis is a kind that has no definite direction of the expected findings being specified.
Related Pages:
Hypothesis Testing
Research Hypotheses
Ready to test your hypothesis? Check out Intellectus Statistics , the easy to use statistics software for the non-statistician.

What is The Null Hypothesis & When Do You Reject The Null Hypothesis
Julia Simkus
Editor at Simply Psychology
BA (Hons) Psychology, Princeton University
Julia Simkus is a graduate of Princeton University with a Bachelor of Arts in Psychology. She is currently studying for a Master's Degree in Counseling for Mental Health and Wellness in September 2023. Julia's research has been published in peer reviewed journals.
Learn about our Editorial Process
Saul McLeod, PhD
Editor-in-Chief for Simply Psychology
BSc (Hons) Psychology, MRes, PhD, University of Manchester
Saul McLeod, PhD., is a qualified psychology teacher with over 18 years of experience in further and higher education. He has been published in peer-reviewed journals, including the Journal of Clinical Psychology.
Olivia Guy-Evans, MSc
Associate Editor for Simply Psychology
BSc (Hons) Psychology, MSc Psychology of Education
Olivia Guy-Evans is a writer and associate editor for Simply Psychology. She has previously worked in healthcare and educational sectors.
On This Page:
A null hypothesis is a statistical concept suggesting no significant difference or relationship between measured variables. It’s the default assumption unless empirical evidence proves otherwise.
The null hypothesis states no relationship exists between the two variables being studied (i.e., one variable does not affect the other).
The null hypothesis is the statement that a researcher or an investigator wants to disprove.
Testing the null hypothesis can tell you whether your results are due to the effects of manipulating the dependent variable or due to random chance.
How to Write a Null Hypothesis
Null hypotheses (H0) start as research questions that the investigator rephrases as statements indicating no effect or relationship between the independent and dependent variables.
It is a default position that your research aims to challenge or confirm.
For example, if studying the impact of exercise on weight loss, your null hypothesis might be:
There is no significant difference in weight loss between individuals who exercise daily and those who do not.
Examples of Null Hypotheses
Research Question | Null Hypothesis |
---|---|
Do teenagers use cell phones more than adults? | Teenagers and adults use cell phones the same amount. |
Do tomato plants exhibit a higher rate of growth when planted in compost rather than in soil? | Tomato plants show no difference in growth rates when planted in compost rather than soil. |
Does daily meditation decrease the incidence of depression? | Daily meditation does not decrease the incidence of depression. |
Does daily exercise increase test performance? | There is no relationship between daily exercise time and test performance. |
Does the new vaccine prevent infections? | The vaccine does not affect the infection rate. |
Does flossing your teeth affect the number of cavities? | Flossing your teeth has no effect on the number of cavities. |
When Do We Reject The Null Hypothesis?
We reject the null hypothesis when the data provide strong enough evidence to conclude that it is likely incorrect. This often occurs when the p-value (probability of observing the data given the null hypothesis is true) is below a predetermined significance level.
If the collected data does not meet the expectation of the null hypothesis, a researcher can conclude that the data lacks sufficient evidence to back up the null hypothesis, and thus the null hypothesis is rejected.
Rejecting the null hypothesis means that a relationship does exist between a set of variables and the effect is statistically significant ( p > 0.05).
If the data collected from the random sample is not statistically significance , then the null hypothesis will be accepted, and the researchers can conclude that there is no relationship between the variables.
You need to perform a statistical test on your data in order to evaluate how consistent it is with the null hypothesis. A p-value is one statistical measurement used to validate a hypothesis against observed data.
Calculating the p-value is a critical part of null-hypothesis significance testing because it quantifies how strongly the sample data contradicts the null hypothesis.
The level of statistical significance is often expressed as a p -value between 0 and 1. The smaller the p-value, the stronger the evidence that you should reject the null hypothesis.
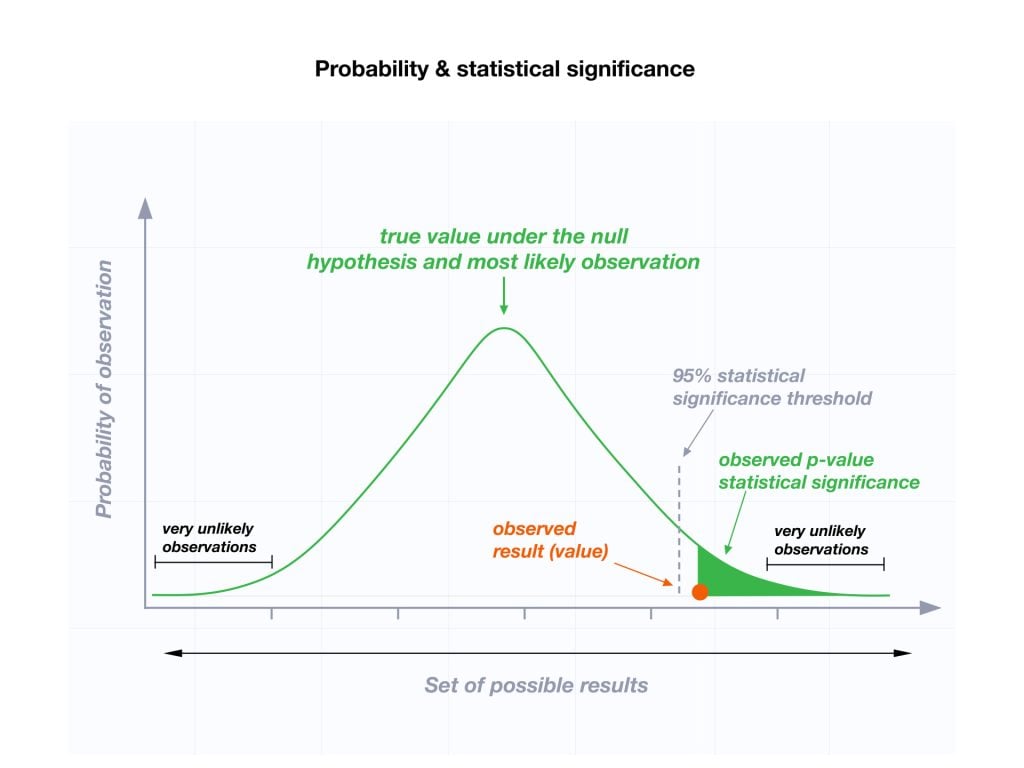
Usually, a researcher uses a confidence level of 95% or 99% (p-value of 0.05 or 0.01) as general guidelines to decide if you should reject or keep the null.
When your p-value is less than or equal to your significance level, you reject the null hypothesis.
In other words, smaller p-values are taken as stronger evidence against the null hypothesis. Conversely, when the p-value is greater than your significance level, you fail to reject the null hypothesis.
In this case, the sample data provides insufficient data to conclude that the effect exists in the population.
Because you can never know with complete certainty whether there is an effect in the population, your inferences about a population will sometimes be incorrect.
When you incorrectly reject the null hypothesis, it’s called a type I error. When you incorrectly fail to reject it, it’s called a type II error.
Why Do We Never Accept The Null Hypothesis?
The reason we do not say “accept the null” is because we are always assuming the null hypothesis is true and then conducting a study to see if there is evidence against it. And, even if we don’t find evidence against it, a null hypothesis is not accepted.
A lack of evidence only means that you haven’t proven that something exists. It does not prove that something doesn’t exist.
It is risky to conclude that the null hypothesis is true merely because we did not find evidence to reject it. It is always possible that researchers elsewhere have disproved the null hypothesis, so we cannot accept it as true, but instead, we state that we failed to reject the null.
One can either reject the null hypothesis, or fail to reject it, but can never accept it.
Why Do We Use The Null Hypothesis?
We can never prove with 100% certainty that a hypothesis is true; We can only collect evidence that supports a theory. However, testing a hypothesis can set the stage for rejecting or accepting this hypothesis within a certain confidence level.
The null hypothesis is useful because it can tell us whether the results of our study are due to random chance or the manipulation of a variable (with a certain level of confidence).
A null hypothesis is rejected if the measured data is significantly unlikely to have occurred and a null hypothesis is accepted if the observed outcome is consistent with the position held by the null hypothesis.
Rejecting the null hypothesis sets the stage for further experimentation to see if a relationship between two variables exists.
Hypothesis testing is a critical part of the scientific method as it helps decide whether the results of a research study support a particular theory about a given population. Hypothesis testing is a systematic way of backing up researchers’ predictions with statistical analysis.
It helps provide sufficient statistical evidence that either favors or rejects a certain hypothesis about the population parameter.
Purpose of a Null Hypothesis
- The primary purpose of the null hypothesis is to disprove an assumption.
- Whether rejected or accepted, the null hypothesis can help further progress a theory in many scientific cases.
- A null hypothesis can be used to ascertain how consistent the outcomes of multiple studies are.
Do you always need both a Null Hypothesis and an Alternative Hypothesis?
The null (H0) and alternative (Ha or H1) hypotheses are two competing claims that describe the effect of the independent variable on the dependent variable. They are mutually exclusive, which means that only one of the two hypotheses can be true.
While the null hypothesis states that there is no effect in the population, an alternative hypothesis states that there is statistical significance between two variables.
The goal of hypothesis testing is to make inferences about a population based on a sample. In order to undertake hypothesis testing, you must express your research hypothesis as a null and alternative hypothesis. Both hypotheses are required to cover every possible outcome of the study.
What is the difference between a null hypothesis and an alternative hypothesis?
The alternative hypothesis is the complement to the null hypothesis. The null hypothesis states that there is no effect or no relationship between variables, while the alternative hypothesis claims that there is an effect or relationship in the population.
It is the claim that you expect or hope will be true. The null hypothesis and the alternative hypothesis are always mutually exclusive, meaning that only one can be true at a time.
What are some problems with the null hypothesis?
One major problem with the null hypothesis is that researchers typically will assume that accepting the null is a failure of the experiment. However, accepting or rejecting any hypothesis is a positive result. Even if the null is not refuted, the researchers will still learn something new.
Why can a null hypothesis not be accepted?
We can either reject or fail to reject a null hypothesis, but never accept it. If your test fails to detect an effect, this is not proof that the effect doesn’t exist. It just means that your sample did not have enough evidence to conclude that it exists.
We can’t accept a null hypothesis because a lack of evidence does not prove something that does not exist. Instead, we fail to reject it.
Failing to reject the null indicates that the sample did not provide sufficient enough evidence to conclude that an effect exists.
If the p-value is greater than the significance level, then you fail to reject the null hypothesis.
Is a null hypothesis directional or non-directional?
A hypothesis test can either contain an alternative directional hypothesis or a non-directional alternative hypothesis. A directional hypothesis is one that contains the less than (“<“) or greater than (“>”) sign.
A nondirectional hypothesis contains the not equal sign (“≠”). However, a null hypothesis is neither directional nor non-directional.
A null hypothesis is a prediction that there will be no change, relationship, or difference between two variables.
The directional hypothesis or nondirectional hypothesis would then be considered alternative hypotheses to the null hypothesis.
Gill, J. (1999). The insignificance of null hypothesis significance testing. Political research quarterly , 52 (3), 647-674.
Krueger, J. (2001). Null hypothesis significance testing: On the survival of a flawed method. American Psychologist , 56 (1), 16.
Masson, M. E. (2011). A tutorial on a practical Bayesian alternative to null-hypothesis significance testing. Behavior research methods , 43 , 679-690.
Nickerson, R. S. (2000). Null hypothesis significance testing: a review of an old and continuing controversy. Psychological methods , 5 (2), 241.
Rozeboom, W. W. (1960). The fallacy of the null-hypothesis significance test. Psychological bulletin , 57 (5), 416.

Stack Exchange Network
Stack Exchange network consists of 183 Q&A communities including Stack Overflow , the largest, most trusted online community for developers to learn, share their knowledge, and build their careers.
Q&A for work
Connect and share knowledge within a single location that is structured and easy to search.
Should the alternative hypothesis always be the research hypothesis?
Let's say part of the mainstream believes that a drug X is more effective than drug Y . Another part of the mainstream believes that drug x is less effective than drug Y . A scientist appears who wants to challenge it and show that the drug X is equivalent to the drug Y , generating the same result and the same side effects, since both act exactly by the same mechanism.
I know the example seems a little forced, but it is just to provoke the classic definition that always aligns the alternative hypothesis with the research hypothesis.
In this case there should be the equality (expressed as a small interval) the alternative hypothesis and therefore the difference (outside of cited small interval) as an null hypothesis?
Previously Gavin Simpson thinks in his answer for that question , that the alternative hypothesis would be possible to use equality, but I would like to see more opinions.
In statistics there are tests of equivalence as well as the more common test the Null and decide if sufficient evidence against it. The equivalence test turn this on its head and posits that effects are different as the Null and we determine if there is sufficient evidence against this Null. I'm not clear on your drug example. If the response is a value/indicator of the effect, then an effect of 0 would indicate not effective. One would set that as the Null and evaluate the evidence against this. If the effect is sufficiently different from zero we would conclude that the no-effectiveness hypothesis is inconsistent with the data. A two-tailed test would count sufficiently negative values of effect as evidence against the Null. A one tailed test, the effect is positive and sufficiently different from zero, might be a more interesting test. If you want to test if the effect is 0, then we'd need to flip this around and use an equivalence test where the H0 is the effect is not equal to zero, and the alternative is that H1 = the effect = 0. That would evaluate the evidence against the idea that effect was different from 0.
ChatGPT has agreed with the cited user, and gave me the following answer to the same question (Don't be upset with me. I quote this answer because it raised reflections on me) :
Yes, in this case, the alternative hypothesis would be that the two drugs, X and Y, are equivalent in terms of effectiveness and side effects. The null hypothesis would be that there is a significant difference between the two drugs in terms of effectiveness or side effects. The scientist's aim would be to gather evidence that supports the alternative hypothesis and rejects the null hypothesis. It's worth noting that the null hypothesis is often stated as the opposite of the research hypothesis in order to provide a clear statement of what the scientist is trying to disprove. However, in this case, the null hypothesis does not necessarily contradict the mainstream beliefs that you mentioned. It simply states that there is a difference between the drugs, whereas the mainstream beliefs disagree on the direction of that difference.
- hypothesis-testing
- 9 $\begingroup$ ChatgPT has agreed with the cited user .... I am reading the post till above. $\endgroup$ – User1865345 Commented Apr 16, 2023 at 15:32
- 1 $\begingroup$ Also it would be appreciable to cite the comment/post where Gavin asserted so for the sake of completion of the post. $\endgroup$ – User1865345 Commented Apr 16, 2023 at 15:38
- 4 $\begingroup$ Paulo, What I and others would suggest strongly is not to consider even a minuscule of assertions provided by ChatGPT; it's not a stat guru sadly. $\endgroup$ – User1865345 Commented Apr 16, 2023 at 15:39
- 7 $\begingroup$ @PauloBuchsbaum welcome to the site, Paulo, and congrats on what I view as a very interesting question! But as this thread has demonstrated, a citation of ChatGPT is more likely to sidetrack the debate than to enrich it... $\endgroup$ – John Madden Commented Apr 16, 2023 at 15:46
- 3 $\begingroup$ @PauloBuchsbaum Answering is always a learning experience here, quite often people will offer constructive criticism. I find that the majority of my own answers were improved by these interactions $\endgroup$ – Firebug Commented Apr 16, 2023 at 19:39
5 Answers 5
I would say that the "alternative hypothesis" is usually NOT a "proposed hypothesis".
You do not define "proposed hypothesis" and it is not a common phrase. Presumably you mean that it is either a statistical hypothesis or it is a scientific hypothesis. They are usually quite different things.
A scientific hypothesis usually concerns a something to do with the true state of the real world, whereas a statistical hypothesis concerns only conditions within a statistical model. It is very common for the real world to be more complicated and less well-defined than a statistical model and so inferences regarding a statistical hypothesis will need to be thoughtfully extrapolated to become relevant to a scientific hypothesis.
For your example a scientific hypothesis concerning the two drugs in question might be something like 'drug x can be substituted for drug y without any noticeable change in results experienced by the patients'. A relevant statistical hypothesis would be much more restricted along the lines of 'drug x and drug y have similar potencies' or that 'drug x and drug y have similar durations of action' or maybe 'doses of drug x and drug y can be found where they have similar effects'. Of course, the required degree of similarity and the assays used for evaluation of the statistical hypothesis will have to be defined. Apart from the enormous differences in scope of the scientific and potential statistical hypotheses, the first may require several or all of the others to be true.
If you want to know if a hypothesis is a statistical hypothesis then if it concerns the value of a parameter within a statistical model or can be restated as being about a parameter value, then it is.
Now, the "alternative hypothesis". For the hypothesis testing framework there are two things that are commonly called 'alternative hypotheses'. The first is an arbitrary effect size that is used in the pre-data calculation of test power (usually for sample size determination). That alternative hypothesis is ONLY relevant before the data are in hand. Once you have the data the arbitrarily specified effect size loses its relevance because the observed effect size is known. When you perform the hypothesis test the effective alternative becomes nothing more than 'not the null'.
It is a bad mistake to assume that a rejection of the null hypothesis in a hypothesis test leads to the acceptance of the pre-data alternative hypothesis, and it is just about as bad to assume that it leads to the acceptance of the observed effect size as a true hypothesis.
Of course, the hypothesis test framework is not the only statistical approach, and I would argue, it is not even the most relevant to the majority of scientific endeavours. If you use a likelihood ratio test then you can compare the data support for two specified parameter values within the statistical model and that means that you can do the same within a Bayesian framework.
- $\begingroup$ I never say that reject the null hypothesis is equivalent to a proof of alternative hypothesis. $\endgroup$ – Paulo Buchsbaum Commented Apr 17, 2023 at 0:13
- $\begingroup$ Michel Lew I will change the term "proposed hypothesis" for "research hypothesis". I don't want to dive in tecnical jargon here (Bayes or likehood ratio test), I want a general picture. Here an excerpt of information on the hypothesis test: "Research hypothesis: this is the hypothesis that you propose, also known as the alternative hypothesis" $\endgroup$ – Paulo Buchsbaum Commented Apr 17, 2023 at 0:20
- $\begingroup$ All my life reading papers it's only hypothesis pairs: null and alternative. Perhaps um paper can includes more than one hypothesis pair, but I always see the alternative hypothesis as complementary to the null hypothesis. $\endgroup$ – Paulo Buchsbaum Commented Apr 17, 2023 at 0:22
- $\begingroup$ @PauloBuchsbaum Seems to me that to say that likelihood and Bayes approaches are "technical jargon" is more than a bit weird. Also, if you change from "proposed hypothesis" to "research hypothesis"you will need to think about my comments on hypotheses relating to the real world and those that relate to statistical models... $\endgroup$ – Michael Lew Commented Apr 17, 2023 at 7:22
- $\begingroup$ A relevant statistical hypothesis... is followed by reference to the real world rather than a statistical model. So in that sense it is not yet a statistical hypothesis, I think. $\endgroup$ – Richard Hardy Commented Apr 17, 2023 at 8:32
The principle of statistical hypothesis tests, by definition, treats the null hypothesis H0 and the alternative H1 asymmetrically. This always needs to be taken into account. A test is able to tell you whether there is evidence against the null hypothesis in the direction of the alternative.
It will never tell you that there is evidence against the alternative.
The choice of the H0 determines what the test can do; it determines what the test can indicate against.
I share @Michael Lew's reservations against a formal use of the term "proposed hypothesis", however let's assume for the following that you can translate your scientific research hypothesis into certain parameter values for a specified statistical model. Let's call this R.
If you choose R as H0, you can find evidence against it, but not in its favour. This may not be what you want - although it isn't out of question. You may well wonder whether certain data contradict your R, in which case you can use it as H0, however this has no potential, even in case of non-rejection, to convince other people that R is correct.
There is however a very reasonable scientific justification for using R as H0, which is that according to Popper in order to corroborate a scientific theory, you should try to falsify it, and the best corroboration comes from repeated attempts to falsify it (in a way in which it seems likely that the theory will be rejected if it is in fact false, which is what Mayo's "severity" concept is about). Apart from statistical error probabilities, this is what testing R as H0 actually allows to do, so there is a good reason for using R as H0.
If you choose R as H1, you can find evidence against the H0, which is not normally quite what you want, unless you interpret evidence against H0 as evidence in favour of your H1, which isn't necessarily granted (model assumptions may be violated for both H0 and H1, so they may both technically be wrong, and rejecting H0 doesn't "statistically prove" H1), although many would interpret a test in this way. It has value only to the extent that somebody who opposes your R argues that H0 might be true (as in "your hypothesised real effect does not exist, it's all just due to random variation"). In this case a test with R as H1 has at least the potential to indicate strongly against that H0. You can even go on and say it'll give you evidence that H0 is violated "in the direction of H1", but as said before there may be other explanations for this than that H1 is actually true. Also, "the direction of H1" is rather imprecise and doesn't amount to any specific parameter value or it's surroundings. It may depend on the application area how important that is. A homeopath may be happy enough to significantly show that homeopathy does something good rather than having its effect explained by random variation only, regardless of how much good it actually does, however precise numerical theories in physics/engineering, say, can hardly be backed up by just rejecting a random variation alternative.
The "equivalence testing" idea would amount to specifying a rather precise R (specific parameter value and small neighbourhood) as H1 and potentially rejecting a much bigger part of the parameter space on both sides of R. This would then be more informative, but has still the same issue with model assumptions, i.e., H0 and H1 may both be wrong. (Obviously model assumption diagnoses may help to some extent. Also even if neither H0 nor H1 is true, arguably some more distributions can be seen as "interpretatively equivalent" with them, e.g., two equal non-normal distributions in a two-sample setup where a normality-based test is applied, and actually may work well due to the Central Limit Theorem even for many non-normal distributions.)
So basically you need to choose what kind of statement you want to allow your test to back up. Choose R as H0 and the data can only reject it. Choose R as H1 and the data can reject the H0, and how valuable that is depends on the situation (particularly on how realistic the H0 looks as a competitor; i.e., how informative it actually is to reject it). The equivalence test setup is special by allowing you to use a rather precise R as H1 and reject a big H0, so the difference between this and rejecting a "random variation/no effect" H0 regards the precise or imprecise nature of the research hypothesis R to be tested.
In this case there should be the equality as an alternative hypothesis and therefore the difference as an null hypothesis?
Hypothesis testing works well when a particular hypothesis makes a precise prediction. Like the observed value is likely equal or above/below some value. Hypothesis testing is about making predictions based on a theory and observing whether those predictions come true.
If you would have a hypothesis that drug X and Y are unequal, then you would have an untestable theory. Given some differences between the populations of X and Y, whether that difference is zero or not, in nearly any circumstances the observations of samples from X and Y will be different anyway, independent from the hypothesis whether or not X and Y similar distributions.
(and even when samples from X and Y are observed to be equal, then this might not be significant as the difference can be as small as we like, making the observation of equal samples not anything special that falsifies the hypothesis)
Test of equivalence
However what would be possible is a test of equivalence , which relates to a hypothesis that the mean difference between X and Y is between some small range. Then, observing a larger range could falsify that hypothesis.
So the 'alternative hypothesis' can be used as the null hypothesis. But, it needs to be expressed in a form that restricts the observations. The hypothesis 'X ≠ Y' doesn't do that. However a limit on the difference '|X-Y| < a' is a testible theory/hypothesis.
Confidence interval
Another popular alternative to null hypothesis testing is to present confidence intervals. The confidence interval can be seen as the range of hypothetical parameter values that pass a null hypothesis test where the hypothetical value is the null hypothesis.
Why are standard frequentist hypotheses so uninteresting?

@Dave gave me a light about the question and told me about the equivalence test, explained here .
The hypothesis test for equivalence can be written as follows:
H0 : The difference between the two group means is outside the equivalence interval H1 : The difference between the two group means is inside the equivalence interval
However, I study this question deeply in the last days in many textbooks and other sources. There is no consensus of all statistics on this issue. I found several divergent opinions.
The divergence happens more relative to the debate if the alternative hypothesis is always complementary to the null hypothesis, or is not. I, for my part, find it much more logical to consider the null and alternative hypothesis as perfectly complementary. This , this and this (sources linked to universities) agree with me.
As stated in the referred link, if there is a gray zone, which is neither included in the alternative hypothesis nor in the zero hypothesis.In this case, rejecting the null hypothesis no longer serves conceptually to help highlight the alternative hypothesis, it may merely mean that we are in the gray zone. For me there is no point in thinking that way.
However, almost all experts agrees that the null hypothesis always should contain '=' operator ("<=", "=" or ">="). See, for instance, here , here and here , sources linked to universities, adopt this line of thought.
And I understand why. Always have '=' in the null hypothesis, it creates a certain standardization of statistical methodologies that could reject this equality. It would be very confusing to have to deal with an alternative hypothesis that could contain equality.
If we accept that null and the alternative hypothesis is the complement (">","≠" or "<", respectively) in a exhaustive way.
Sometimes the researcher believes in the alternative hypothesis, sometimes don't.
This applies to the hypothetical situation that I've created, if we suppose the research hypothesis does not necessarily align with the researcher's beliefs.
In this context, the alternative hypothesis it would be the hypothesis that "challenges" the null hypothesis, in the sense of finding out if the study has statistically evidence regarded as sufficient to reject it.
Addendum: 2 textbooks as an example
1) Understandable Statistics - Brase, Brase -10th edition - 2012
...Any hypothesis that differs from the null hypothesis is called an alternate hypothesis...
In statistical testing, the null hypothesis H0 always contains the equals symbol.
It agrees with this 2 claims (null hypothesis should contains '=' and null hypothesis and alternative hypothesis are complementary)
2) Statistics - James McClave, Terry Sincich - 13th edition - 2018
...While alternative hypotheses are always specified as strict inequalities, such as μ < 2,400, μ > 2,400, or μ ≠ 2,400, null hypotheses are usually specified as equalities, such as μ = 2,400. Even when the null hypothesis is an inequality, such as μ ≤ 2,400, we specify H0: μ = 2,400, reasoning that if sufficient evidence exists to show that Ha:μ > 2,400 is true when tested against H0: m = 2,400, then surely sufficient evidence exists to reject μ < 2,400 as well...
It agrees with that Ho should contain '=' operator, but works with a "hole" in hypothesis space. However, looking at the text, it's clear that it's irrelevant.
- 8 $\begingroup$ What makes $H_0$ a null hypothesis is that it determines the distribution of the test statistic. That is fundamental; it would be impossible to conduct a classical null hypothesis test otherwise. $\endgroup$ – whuber ♦ Commented Apr 16, 2023 at 19:14
- 3 $\begingroup$ Welcome! Good question and answer. The linked equivalence testing article explains the idea well except that it contains a basic misunderstanding about hypothesis test outcomes in general: "rejection of the null hypothesis (in which case we believe the alternate hypothesis is true at the specified confidence level)". The bracketed section is wrong. Rejecting a null at the 5% level does not imply confidence of 95% in the alternative hypothesis. The author repeats the error a number of times. $\endgroup$ – Graham Bornholt Commented Apr 16, 2023 at 19:37
- $\begingroup$ Thank you, Graham Bornholt . In fact, the linked article has mixed significance (alpha=5%) with 95% confidence. It's a mess! $\endgroup$ – Paulo Buchsbaum Commented Apr 17, 2023 at 0:04
- $\begingroup$ I don't see how the word "always" is in any way justified by this answer. The equivalence test specifies a particular situation in which the "hypothesis of research" is the H1, fair enough. But there may be reasons to test it as H0, see my answer. $\endgroup$ – Christian Hennig Commented Apr 17, 2023 at 20:35
- $\begingroup$ @whuber - Isn't that the answer, sweet and simple? I'm new to this community, but in some stackexchange sites we'd say "Make that an answer". $\endgroup$ – JonathanZ Commented Apr 18, 2023 at 15:40
We generally assume as the null hypotheses as an old orthodox belief as true even though we do not have sufficient proof of its truth.
and Alternate Hypothesis H1 as a new radical belief that is challenging our old system of belief.
So we need a great level of effort to reject our old belief H0. We will need a high degree of confidence in H1 to dismantle our old traditional beliefs.
Lets understand this by example.
Aristotle's view of solar system
For nearly 1,000 years, Aristotle’s view of a stationary Earth at the center of a revolving universe dominated natural philosophy
In old times we believed that sun revolves around earth consider this as our old traditional orthodox belief => Null Hypothesis {We will try very hard to stick to this belief}
“We revolve around the Sun like any other planet.” —Nicolaus Copernicus
Our new radical claim is that earth revolves around the sun. => Alternate Hypothesis H1.
So whichever drug company you work in X or Y you will assume your company's drug(H0) is better than the competitor(H1)
Reference NASA
Reference Georgia Tech course EDX.org
Note:- Please correct me if I am wrong I am just a student trying to learn Thanks

- 1 $\begingroup$ Welcome to CV, Banarasi. I think this answer confuses philosophy with statistical theory and thereby provides a misleading account of the statistical theory. "Old orthodox" and "radical new" are inappropriate (and meaningless) concepts in this context, and insisting that we "believe as true" a null hypothesis is going too far, because a hypothesis is just that: a set of assumptions erected to investigate their consequences in light of the data under analysis. $\endgroup$ – whuber ♦ Commented Apr 18, 2023 at 15:35
- $\begingroup$ Thanks for suggesting correction, I do not have any formal degree so I am not aware about the formal jargons used can you please suggest me some books or blogs to strengthen my knowledge about this . Thanks $\endgroup$ – Banarasi Vaibhav Commented Apr 19, 2023 at 6:13
- 1 $\begingroup$ You could start by browsing this site for highly upvoted threads . Add keywords to narrow the search, such as hypothesis . $\endgroup$ – whuber ♦ Commented Apr 19, 2023 at 13:22
Your Answer
Sign up or log in, post as a guest.
Required, but never shown
By clicking “Post Your Answer”, you agree to our terms of service and acknowledge you have read our privacy policy .
Not the answer you're looking for? Browse other questions tagged hypothesis-testing or ask your own question .
- Featured on Meta
- Announcing a change to the data-dump process
- Bringing clarity to status tag usage on meta sites
Hot Network Questions
- How can I copy a clip in Ableton Live so that we need to make a change to the clip, all copies of the clip are changed accordingly?
- Should I install our toilet on top of the subfloor or on top of the PVT?
- Identifications in differential geometry
- How can I align this figure with the page numbering?
- Is it a date format of YYMMDD, MMDDYY, and/or DDMMYY?
- Best way to explain the thinking steps from x² = 9 to x=±3
- Replicating Econometrics Research
- What did Wittgenstein mean by ”contradiction is the outer limit of propositions”?
- How to prevent my frozen dessert from going solid?
- Is this a 'standard' elliptic integral?
- Download facebook on ubuntu
- When a star becomes a black hole do the neutrons that are squeezed together release binding energy and if so does this energy escape from the hole?
- What is inside the SPIKE Essential battery?
- Can it be acceptable to take over CTRL + F shortcut in web app
- Find and delete files from unix directory of multiple patterns
- What is Zion's depth in the Matrix?
- If Starliner returns safely on autopilot, can this still prove that it's safe? Could it be launched back up to the ISS again to complete its mission?
- Is it possible to travel to USA with legal cannabis?
- Do eternal ordinances such as the festival of unleavened bread pose a biblical contradiction?
- Decomposable maps of half-smash products
- Driveway electric run using existing service poles
- If you have two probabilities, how do you describe how much more likely one is than the other?
- Self-descriptive
- Would it be Balanced to Give Everyone Warlock Slots for Casting Racial Spells?
- Alternative vs Null Hypothesis: Pros, Cons, Uses & Examples

All research starts with a problem that needs to be solved. From this problem, hypotheses are developed to provide the researcher with a clear statement of the problem.
To understand alternative hypotheses also known as alternate hypotheses, you must first understand what the hypothesis is .
When you hear the word hypothesis it means the accurate explanations in relation to a set of facts that can be analyzed when studied, using some specific method of research.
There are primarily two types of hypothesis which are null hypothesis and alternative hypothesis.
When you think about the word “null” what should come to mind is something that can not change, what you expect is what you get, unlike alternate hypotheses which can change.
Now, the research problems or questions which could be in the form of null hypothesis or alternative hypothesis are expressed as the relationship that exists between two or more variables. The process for this states that the questions should be what expresses the relationship between two variables that can be measured.
Both null hypotheses and alternative hypotheses are used by statisticians and researchers to conduct research in various industries or fields such as mathematics, psychology, science, medicine, and technology.
We are going to discuss alternative hypotheses and null hypotheses in this post and how they work in research.
What is an Alternative Hypothesis?
Alternative hypothesis simply put is another viable option to the null hypothesis. It means looking for a substantial change or option that can allow you to reject the null hypothesis.
It is an opposing theory to a null hypothesis.
If you develop a null hypothesis, you make an informed guess on whether a thing is true or whether there is a relationship between that thing and another variable. An alternate hypothesis will always take an opposite stand against a null hypothesis. So if according to a null hypothesis something is correct to an alternate hypothesis that same thing will be incorrect.
For example, let’s assume that you develop a null hypothesis that states “I”m going to be $500 richer” the alternate hypothesis will be “I’m going to get $500 or be richer”
When you are trying to disprove a null hypothesis, that is when you test an alternate hypothesis. If there is enough data to back up the alternative hypothesis then you can dispose of the null hypothesis.
Get Answers: What is Empirical Research Study? [Examples & Method]
What is a Null Hypothesis?
The null hypothesis is best explained as the statement showing that no relationship exists between two variables that are being considered or that two groups are not related. As we have earlier established, a hypothesis is an assumed statement that has not been proven with sufficient data that could serve as a piece of evidence.
The null hypothesis is now the statement that a researcher or an investigator wants to disprove. The null hypothesis is capable of being tested, being verifiable, and also capable of being rejected.
For example, if you want to conduct a study that will compare the relationship between project A and project B if the study is based on the assumption that both projects are of equal standard, the assumption is referred to as the null hypothesis.
This is because the null hypothesis should be specific at all times.
Learn: Hypothesis Testing in Research: Definition, Procedure, Uses, Limitations + Examples
Advantages of the Alternative Hypothesis
- Alternative hypothesis gives a researcher specific clarifications on the research questions or problems .
- It provides a study with the direction that can be used to collect data and obtain results of interest by the researcher.
- An alternative hypothesis is always selected before commencing the studies which gives the researcher the opportunity to prove that the restatement is backed up by evidence and not just from the researcher’s ideas or values.
- Another good thing about alternative hypotheses is that it provides the opportunity to discover new theories that a researcher can use to disprove an existing theory that may not have been backed up by evidence .
- An alternate hypothesis is also useful to prove that there is a relationship between two selected variables and the outcomes of the conducted study are relevant.
Principles of the Alternative Hypothesis
- Alternative hypotheses will be accepted if the amount of data that is gone is insignificant within the significance level. This means that the null hypothesis will be rejected.
- Another principle of the alternative hypothesis is that the data gathered from random samples go through a statistical tool that analyzes the effect of the amount of data leaving the null hypothesis.
For the curious: Sampling Bias: Definition, Types + [Examples]
Purpose of the Null Hypothesis
Here are the purposes of the null hypothesis in an experiment or study:
- The primary purpose of a null hypothesis is to disprove an assumption.
- Null hypotheses can help to further progress a theory in some scientific cases.
- You can also use a null hypothesis to ascertain how consistent the outcomes of multiple studies are.
Principle of the Null Hypothesis
Now, these are the principles of the null hypothesis:
1. The primary principle of the null hypothesis is to prove that the assumed statement is true. This is done by collecting data and analyzing in the study , what chance the collected data has in the random sample.
2. If the collected data does not meet the expectation of the null hypothesis, it is determined that the data lacks sufficient evidence to back up the null hypothesis therefore the null hypothesis statement is rejected.
Just as in the case of the alternative hypothesis the collected data in a null hypothesis is analyzed using some statistical tools that are made to measure the extent to which data left the null hypothesis.
The process will determine whether the data that left the null hypothesis is larger than a set value. If the data collected from the random sample is enough to serve as evidence to prove the null hypothesis then the null hypothesis will be accepted as true. And also defined that it has no relationship with other variables .
Learn About: Research Reports: Definition, Types + [Writing Guide]
Types of Alternative Hypothesis (Advantages of Each and When to Use)
There are four types of alternative hypotheses, and we will briefly discuss them below.
- One-tailed directional: For one of the sampling distributions one tail, this type of alternative hypothesis focuses on the rejected part only.
- Two-tailed directional: In an alternative hypothesis, a two-tailed directional focus on the two parts or directions that were rejected in the sampling distribution.
- Point: Point is another alternate hypothesis. It occurs in hypothesis testing when the sample population in the alternate hypothesis has been completely defined in a distribution. If there are no known parameters, the hypothesis will serve no interest. They are, however, important to the foundation of the statistical inferences.
- Non-directional: In an alternate hypothesis, a non-directional does not focus on the two directions of rejection. The only focus of the nondirectional alternative hypothesis is to prove that the null hypothesis is incorrect.
Read: Type I vs Type II Errors: Definition, Examples & Prevention
Difference between Null Hypothesis and Alternative Hypothesis
We are going to look at the differences between the alternate hypothesis and the null hypothesis based on these six factors which are:
- Mathematical expression
- Observation
- Acceptance criteria
- The difference in Mathematical expression
Null hypothesis is followed by an ‘equals to’ (=) sign. While the Alternative hypothesis is followed by these three signs;
- The difference in how they are observed
In the null hypothesis, it is believed that the results that are observed are as a result of chance. While In the alternative hypothesis, it is believed that the observed results are the outcome of some real causes.
- Differences in results
The result of the null hypothesis always shows that there have been no changes in statements or opinions. While the result of the alternative hypothesis shows that there have been significant changes in statements and opinions.
- Differences in Acceptance criteria
If the p-value in a null hypothesis is greater than the significance level, then the null hypothesis is accepted.
If the p-value in an alternate hypothesis is smaller than the significance level, then the alternative hypothesis is accepted.
- Differences in importance
The null hypothesis accepts true existing theories and also if there has been consistency in multiple experiments of similar hypotheses.
The alternative hypothesis establishes whether a relationship exists between two variables, and the result will then lead to new improved theories.
Read: T-testing: Definition, Formula & Interpretations
Examples of an Alternative Hypothesis and Null Hypothesis
Here are some examples of the alternative hypothesis:.
A researcher assumes that a bridge’s bearing capacity is over 10 tons, the researcher will then develop an hypothesis to support this study. The hypothesis will be:
For the null hypothesis H0: µ= 10 tons
For the alternate hypothesis Ha: µ>10 tons
In another study being conducted, the researcher wants to find out whether there is a noticeable difference or change in a patient’s heart arrest medicine and the patient’s heart condition.
For the alternate hypothesis: The hypothesis is that there might indeed be a relationship between the new medicine and the frequency or chances of heart arrest in a patient.
Here are the examples of the null hypothesis
The hypothesis from example 2 in the alternate hypothesis implies that the use of one specific medicine can reduce the frequency and chances of heart arrest.
For the null hypothesis: The hypothesis will be that the use of that particular medicine cannot reduce the chance and frequency of heart arrest in a patient.
An alternate hypothesis states that the random exam scores are collected from both men and women. But are the scores of the two groups (men and women) the same or are they different?
For the null hypothesis: The hypothesis will state that the calculated mean of the men’s exam score is equal to the exam score of the women.
This is represented as
H0= The null hypothesis
µ1= The calculated mean score of men
µ2= The calculated mean score of women
Read: What is Empirical Research Study? [Examples & Method]
- Can you reject an alternative hypothesis?
It is quite inappropriate to say or report that an alternate hypothesis was rejected. It is much better to use the phrase “the alternate hypothesis was rather not supported”.
The reason behind this use of words is that only the null hypothesis is designed to be rejected in a study. The alternative hypothesis is designed to prove the null hypothesis incorrect, to introduce new facts that can disprove the null hypothesis but it is not designed to be rejected.
It can either be accepted or not supported.
- How do you identify alternative hypotheses?
A researcher can use this formula to identify the alternate hypothesis in a study or experiment.
H0 and Ha are in contrast.
Therefore, if Ho has:
Equal to (=)
Greater than or equal to (≥)
Less than or equal to (≤)
And then Ha has:
Not equal (≠)
Greater than (>) or less than (
Less than ( )
If in a study, α ≤ p-value, then the researcher should not reject H0.
If in a study, α > p-value, then the researcher should reject H0.
α is preconceived. The value of α is determined even before the hypothesis test is conducted. While the p-value is derived from the calculation in the data.
- Which is better in formulating hypotheses of your study alternative or null?
The study a researcher wants to conduct will determine what hypothesis should be developed. However, the researcher should keep in mind what the purpose of the null and alternative two hypotheses are while developing the study hypothesis. So while the null hypothesis will accept existing theories that it found to be true or correct, and measure the consistency of multiple experiments, alternative hypotheses will find the relationship that exists (if any) between two phenomena and may lead to the development of a new and improved theory.
In this article, it has been clearly defined the relationship that exists between the null hypothesis and the alternative hypothesis. While the null hypothesis is always an assumption that needs to be proven with evidence for it to be accepted, the alternative hypothesis puts in all the effort to make sure the null hypothesis is disproved.
Researchers should note that for every null hypothesis, one or more alternate hypotheses can be developed.
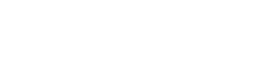
Connect to Formplus, Get Started Now - It's Free!
- acceptance criteria
- alternate hypotheses
- alternative hypothesis
- alternative hypothesis types
- alternative vs null hypothesis
- null hypothese
- busayo.longe
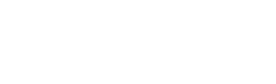
You may also like:
Type I vs Type II Errors: Causes, Examples & Prevention
This article will discuss the two different types of errors in hypothesis testing and how you can prevent them from occurring in your research

Hypothesis Testing: Definition, Uses, Limitations + Examples
The process of research validation involves testing and it is in this context that we will explore hypothesis testing.
Extrapolation in Statistical Research: Definition, Examples, Types, Applications
In this article we’ll look at the different types and characteristics of extrapolation, plus how it contrasts to interpolation.
Acceptance Sampling: Meaning, Examples, When to Use
In this post, we will discuss extensively what acceptance sampling is and when it is applied.
Formplus - For Seamless Data Collection
Collect data the right way with a versatile data collection tool. try formplus and transform your work productivity today..
Frequently asked questions
What’s the difference between a research hypothesis and a statistical hypothesis.
A research hypothesis is your proposed answer to your research question. The research hypothesis usually includes an explanation (“ x affects y because …”).
A statistical hypothesis, on the other hand, is a mathematical statement about a population parameter. Statistical hypotheses always come in pairs: the null and alternative hypotheses . In a well-designed study , the statistical hypotheses correspond logically to the research hypothesis.
Frequently asked questions: Statistics
As the degrees of freedom increase, Student’s t distribution becomes less leptokurtic , meaning that the probability of extreme values decreases. The distribution becomes more and more similar to a standard normal distribution .
The three categories of kurtosis are:
- Mesokurtosis : An excess kurtosis of 0. Normal distributions are mesokurtic.
- Platykurtosis : A negative excess kurtosis. Platykurtic distributions are thin-tailed, meaning that they have few outliers .
- Leptokurtosis : A positive excess kurtosis. Leptokurtic distributions are fat-tailed, meaning that they have many outliers.
Probability distributions belong to two broad categories: discrete probability distributions and continuous probability distributions . Within each category, there are many types of probability distributions.
Probability is the relative frequency over an infinite number of trials.
For example, the probability of a coin landing on heads is .5, meaning that if you flip the coin an infinite number of times, it will land on heads half the time.
Since doing something an infinite number of times is impossible, relative frequency is often used as an estimate of probability. If you flip a coin 1000 times and get 507 heads, the relative frequency, .507, is a good estimate of the probability.
Categorical variables can be described by a frequency distribution. Quantitative variables can also be described by a frequency distribution, but first they need to be grouped into interval classes .
A histogram is an effective way to tell if a frequency distribution appears to have a normal distribution .
Plot a histogram and look at the shape of the bars. If the bars roughly follow a symmetrical bell or hill shape, like the example below, then the distribution is approximately normally distributed.
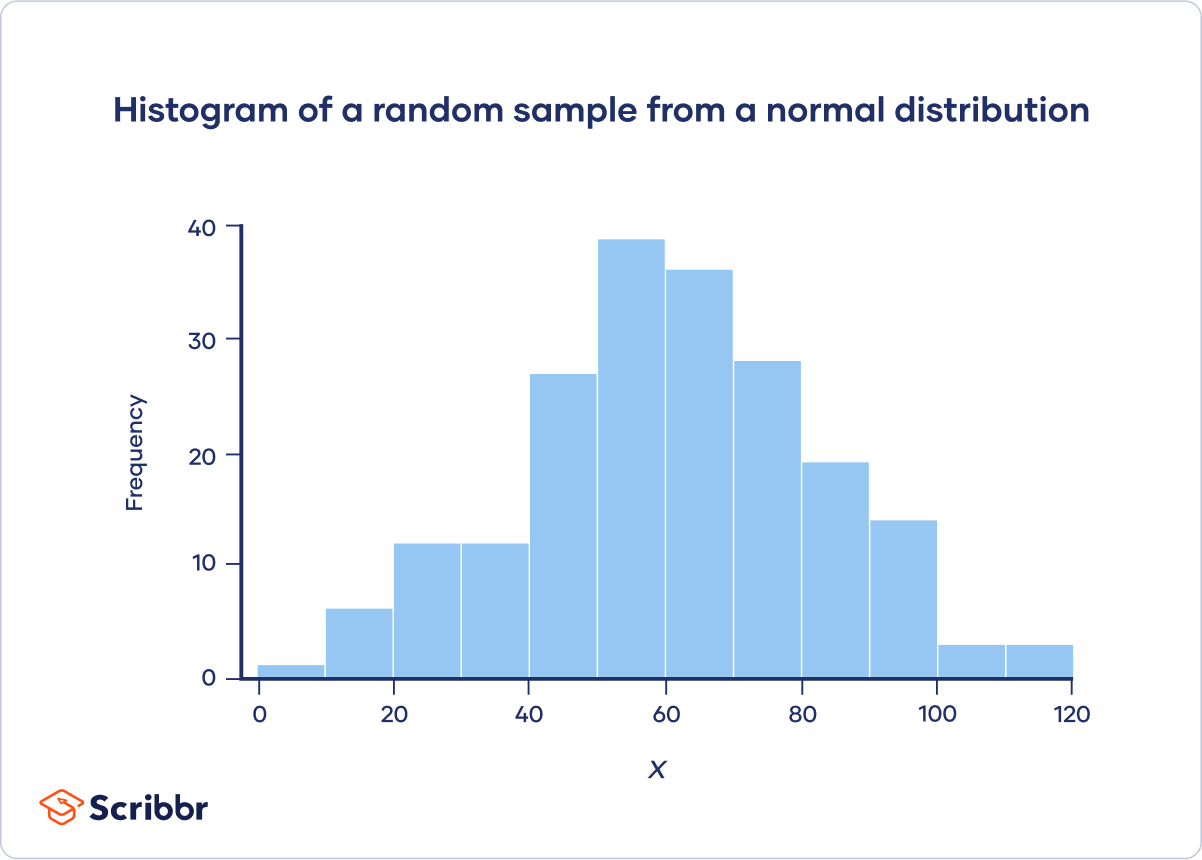
You can use the CHISQ.INV.RT() function to find a chi-square critical value in Excel.
For example, to calculate the chi-square critical value for a test with df = 22 and α = .05, click any blank cell and type:
=CHISQ.INV.RT(0.05,22)
You can use the qchisq() function to find a chi-square critical value in R.
For example, to calculate the chi-square critical value for a test with df = 22 and α = .05:
qchisq(p = .05, df = 22, lower.tail = FALSE)
You can use the chisq.test() function to perform a chi-square test of independence in R. Give the contingency table as a matrix for the “x” argument. For example:
m = matrix(data = c(89, 84, 86, 9, 8, 24), nrow = 3, ncol = 2)
chisq.test(x = m)
You can use the CHISQ.TEST() function to perform a chi-square test of independence in Excel. It takes two arguments, CHISQ.TEST(observed_range, expected_range), and returns the p value.
Chi-square goodness of fit tests are often used in genetics. One common application is to check if two genes are linked (i.e., if the assortment is independent). When genes are linked, the allele inherited for one gene affects the allele inherited for another gene.
Suppose that you want to know if the genes for pea texture (R = round, r = wrinkled) and color (Y = yellow, y = green) are linked. You perform a dihybrid cross between two heterozygous ( RY / ry ) pea plants. The hypotheses you’re testing with your experiment are:
- This would suggest that the genes are unlinked.
- This would suggest that the genes are linked.
You observe 100 peas:
- 78 round and yellow peas
- 6 round and green peas
- 4 wrinkled and yellow peas
- 12 wrinkled and green peas
Step 1: Calculate the expected frequencies
To calculate the expected values, you can make a Punnett square. If the two genes are unlinked, the probability of each genotypic combination is equal.
RRYY | RrYy | RRYy | RrYY | |
RrYy | rryy | Rryy | rrYy | |
RRYy | Rryy | RRyy | RrYy | |
RrYY | rrYy | RrYy | rrYY |
The expected phenotypic ratios are therefore 9 round and yellow: 3 round and green: 3 wrinkled and yellow: 1 wrinkled and green.
From this, you can calculate the expected phenotypic frequencies for 100 peas:
Round and yellow | 78 | 100 * (9/16) = 56.25 |
Round and green | 6 | 100 * (3/16) = 18.75 |
Wrinkled and yellow | 4 | 100 * (3/16) = 18.75 |
Wrinkled and green | 12 | 100 * (1/16) = 6.21 |
Step 2: Calculate chi-square
− | − | ||||
Round and yellow | 78 | 56.25 | 21.75 | 473.06 | 8.41 |
Round and green | 6 | 18.75 | −12.75 | 162.56 | 8.67 |
Wrinkled and yellow | 4 | 18.75 | −14.75 | 217.56 | 11.6 |
Wrinkled and green | 12 | 6.21 | 5.79 | 33.52 | 5.4 |
Χ 2 = 8.41 + 8.67 + 11.6 + 5.4 = 34.08
Step 3: Find the critical chi-square value
Since there are four groups (round and yellow, round and green, wrinkled and yellow, wrinkled and green), there are three degrees of freedom .
For a test of significance at α = .05 and df = 3, the Χ 2 critical value is 7.82.
Step 4: Compare the chi-square value to the critical value
Χ 2 = 34.08
Critical value = 7.82
The Χ 2 value is greater than the critical value .
Step 5: Decide whether the reject the null hypothesis
The Χ 2 value is greater than the critical value, so we reject the null hypothesis that the population of offspring have an equal probability of inheriting all possible genotypic combinations. There is a significant difference between the observed and expected genotypic frequencies ( p < .05).
The data supports the alternative hypothesis that the offspring do not have an equal probability of inheriting all possible genotypic combinations, which suggests that the genes are linked
You can use the chisq.test() function to perform a chi-square goodness of fit test in R. Give the observed values in the “x” argument, give the expected values in the “p” argument, and set “rescale.p” to true. For example:
chisq.test(x = c(22,30,23), p = c(25,25,25), rescale.p = TRUE)
You can use the CHISQ.TEST() function to perform a chi-square goodness of fit test in Excel. It takes two arguments, CHISQ.TEST(observed_range, expected_range), and returns the p value .
Both correlations and chi-square tests can test for relationships between two variables. However, a correlation is used when you have two quantitative variables and a chi-square test of independence is used when you have two categorical variables.
Both chi-square tests and t tests can test for differences between two groups. However, a t test is used when you have a dependent quantitative variable and an independent categorical variable (with two groups). A chi-square test of independence is used when you have two categorical variables.
The two main chi-square tests are the chi-square goodness of fit test and the chi-square test of independence .
A chi-square distribution is a continuous probability distribution . The shape of a chi-square distribution depends on its degrees of freedom , k . The mean of a chi-square distribution is equal to its degrees of freedom ( k ) and the variance is 2 k . The range is 0 to ∞.
As the degrees of freedom ( k ) increases, the chi-square distribution goes from a downward curve to a hump shape. As the degrees of freedom increases further, the hump goes from being strongly right-skewed to being approximately normal.
To find the quartiles of a probability distribution, you can use the distribution’s quantile function.
You can use the quantile() function to find quartiles in R. If your data is called “data”, then “quantile(data, prob=c(.25,.5,.75), type=1)” will return the three quartiles.
You can use the QUARTILE() function to find quartiles in Excel. If your data is in column A, then click any blank cell and type “=QUARTILE(A:A,1)” for the first quartile, “=QUARTILE(A:A,2)” for the second quartile, and “=QUARTILE(A:A,3)” for the third quartile.
You can use the PEARSON() function to calculate the Pearson correlation coefficient in Excel. If your variables are in columns A and B, then click any blank cell and type “PEARSON(A:A,B:B)”.
There is no function to directly test the significance of the correlation.
You can use the cor() function to calculate the Pearson correlation coefficient in R. To test the significance of the correlation, you can use the cor.test() function.
You should use the Pearson correlation coefficient when (1) the relationship is linear and (2) both variables are quantitative and (3) normally distributed and (4) have no outliers.
The Pearson correlation coefficient ( r ) is the most common way of measuring a linear correlation. It is a number between –1 and 1 that measures the strength and direction of the relationship between two variables.
This table summarizes the most important differences between normal distributions and Poisson distributions :
Characteristic | Normal | Poisson |
---|---|---|
Continuous | ||
Mean (µ) and standard deviation (σ) | Lambda (λ) | |
Shape | Bell-shaped | Depends on λ |
Symmetrical | Asymmetrical (right-skewed). As λ increases, the asymmetry decreases. | |
Range | −∞ to ∞ | 0 to ∞ |
When the mean of a Poisson distribution is large (>10), it can be approximated by a normal distribution.
In the Poisson distribution formula, lambda (λ) is the mean number of events within a given interval of time or space. For example, λ = 0.748 floods per year.
The e in the Poisson distribution formula stands for the number 2.718. This number is called Euler’s constant. You can simply substitute e with 2.718 when you’re calculating a Poisson probability. Euler’s constant is a very useful number and is especially important in calculus.
The three types of skewness are:
- Right skew (also called positive skew ) . A right-skewed distribution is longer on the right side of its peak than on its left.
- Left skew (also called negative skew). A left-skewed distribution is longer on the left side of its peak than on its right.
- Zero skew. It is symmetrical and its left and right sides are mirror images.
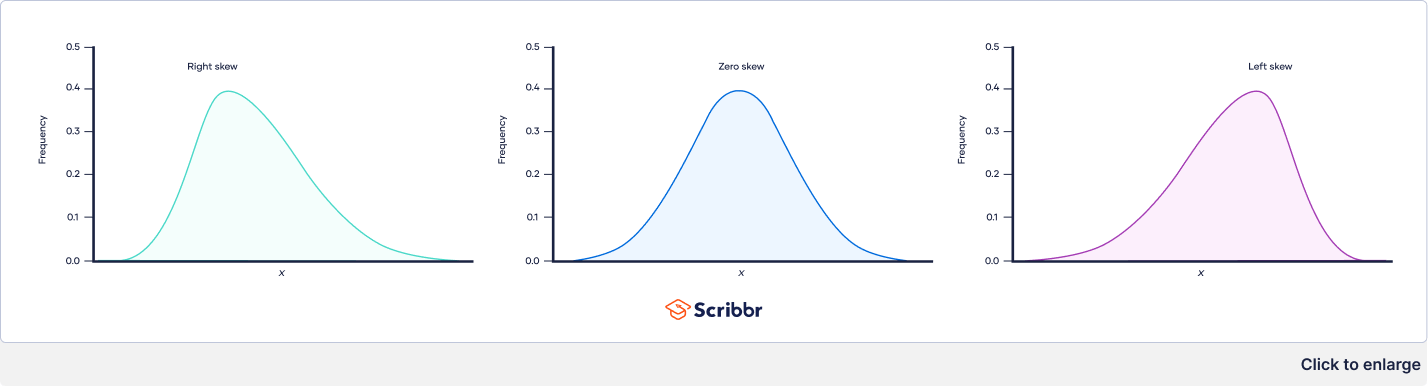
Skewness and kurtosis are both important measures of a distribution’s shape.
- Skewness measures the asymmetry of a distribution.
- Kurtosis measures the heaviness of a distribution’s tails relative to a normal distribution .
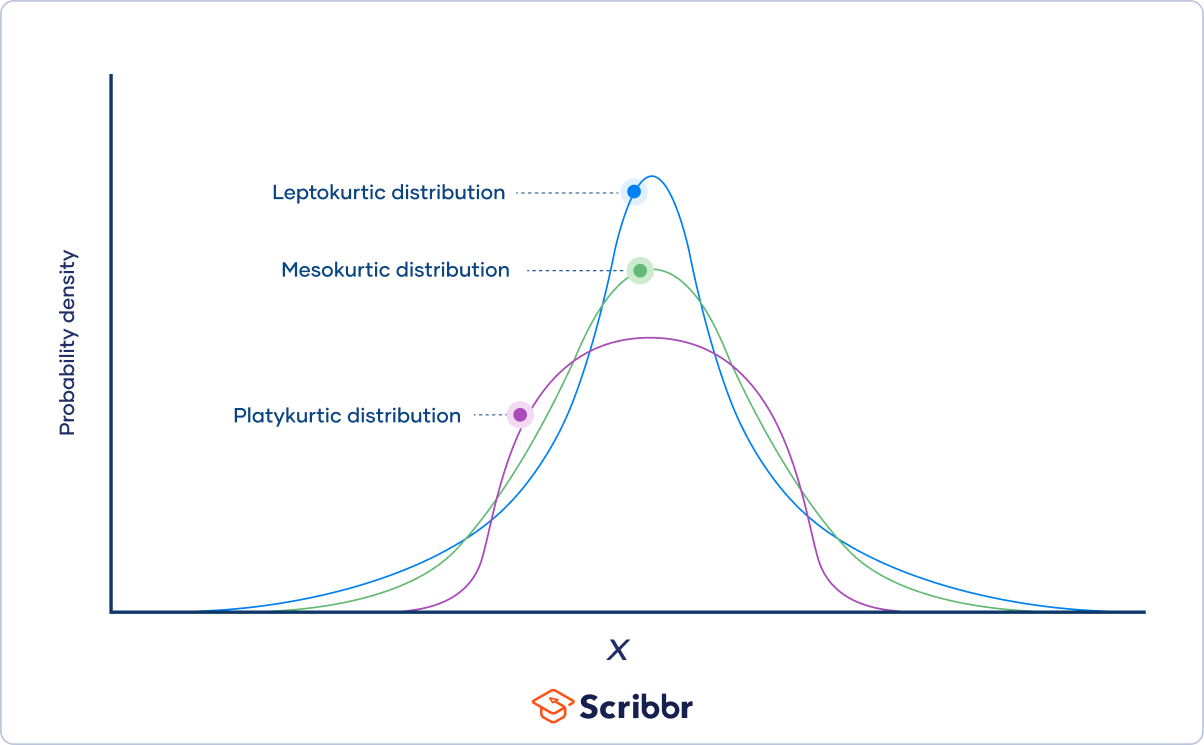
The alternative hypothesis is often abbreviated as H a or H 1 . When the alternative hypothesis is written using mathematical symbols, it always includes an inequality symbol (usually ≠, but sometimes < or >).
The null hypothesis is often abbreviated as H 0 . When the null hypothesis is written using mathematical symbols, it always includes an equality symbol (usually =, but sometimes ≥ or ≤).
The t distribution was first described by statistician William Sealy Gosset under the pseudonym “Student.”
To calculate a confidence interval of a mean using the critical value of t , follow these four steps:
- Choose the significance level based on your desired confidence level. The most common confidence level is 95%, which corresponds to α = .05 in the two-tailed t table .
- Find the critical value of t in the two-tailed t table.
- Multiply the critical value of t by s / √ n .
- Add this value to the mean to calculate the upper limit of the confidence interval, and subtract this value from the mean to calculate the lower limit.
To test a hypothesis using the critical value of t , follow these four steps:
- Calculate the t value for your sample.
- Find the critical value of t in the t table .
- Determine if the (absolute) t value is greater than the critical value of t .
- Reject the null hypothesis if the sample’s t value is greater than the critical value of t . Otherwise, don’t reject the null hypothesis .
You can use the T.INV() function to find the critical value of t for one-tailed tests in Excel, and you can use the T.INV.2T() function for two-tailed tests.
You can use the qt() function to find the critical value of t in R. The function gives the critical value of t for the one-tailed test. If you want the critical value of t for a two-tailed test, divide the significance level by two.
You can use the RSQ() function to calculate R² in Excel. If your dependent variable is in column A and your independent variable is in column B, then click any blank cell and type “RSQ(A:A,B:B)”.
You can use the summary() function to view the R² of a linear model in R. You will see the “R-squared” near the bottom of the output.
There are two formulas you can use to calculate the coefficient of determination (R²) of a simple linear regression .

The coefficient of determination (R²) is a number between 0 and 1 that measures how well a statistical model predicts an outcome. You can interpret the R² as the proportion of variation in the dependent variable that is predicted by the statistical model.
There are three main types of missing data .
Missing completely at random (MCAR) data are randomly distributed across the variable and unrelated to other variables .
Missing at random (MAR) data are not randomly distributed but they are accounted for by other observed variables.
Missing not at random (MNAR) data systematically differ from the observed values.
To tidy up your missing data , your options usually include accepting, removing, or recreating the missing data.
- Acceptance: You leave your data as is
- Listwise or pairwise deletion: You delete all cases (participants) with missing data from analyses
- Imputation: You use other data to fill in the missing data
Missing data are important because, depending on the type, they can sometimes bias your results. This means your results may not be generalizable outside of your study because your data come from an unrepresentative sample .
Missing data , or missing values, occur when you don’t have data stored for certain variables or participants.
In any dataset, there’s usually some missing data. In quantitative research , missing values appear as blank cells in your spreadsheet.
There are two steps to calculating the geometric mean :
- Multiply all values together to get their product.
- Find the n th root of the product ( n is the number of values).
Before calculating the geometric mean, note that:
- The geometric mean can only be found for positive values.
- If any value in the data set is zero, the geometric mean is zero.
The arithmetic mean is the most commonly used type of mean and is often referred to simply as “the mean.” While the arithmetic mean is based on adding and dividing values, the geometric mean multiplies and finds the root of values.
Even though the geometric mean is a less common measure of central tendency , it’s more accurate than the arithmetic mean for percentage change and positively skewed data. The geometric mean is often reported for financial indices and population growth rates.
The geometric mean is an average that multiplies all values and finds a root of the number. For a dataset with n numbers, you find the n th root of their product.
Outliers are extreme values that differ from most values in the dataset. You find outliers at the extreme ends of your dataset.
It’s best to remove outliers only when you have a sound reason for doing so.
Some outliers represent natural variations in the population , and they should be left as is in your dataset. These are called true outliers.
Other outliers are problematic and should be removed because they represent measurement errors , data entry or processing errors, or poor sampling.
You can choose from four main ways to detect outliers :
- Sorting your values from low to high and checking minimum and maximum values
- Visualizing your data with a box plot and looking for outliers
- Using the interquartile range to create fences for your data
- Using statistical procedures to identify extreme values
Outliers can have a big impact on your statistical analyses and skew the results of any hypothesis test if they are inaccurate.
These extreme values can impact your statistical power as well, making it hard to detect a true effect if there is one.
No, the steepness or slope of the line isn’t related to the correlation coefficient value. The correlation coefficient only tells you how closely your data fit on a line, so two datasets with the same correlation coefficient can have very different slopes.
To find the slope of the line, you’ll need to perform a regression analysis .
Correlation coefficients always range between -1 and 1.
The sign of the coefficient tells you the direction of the relationship: a positive value means the variables change together in the same direction, while a negative value means they change together in opposite directions.
The absolute value of a number is equal to the number without its sign. The absolute value of a correlation coefficient tells you the magnitude of the correlation: the greater the absolute value, the stronger the correlation.
These are the assumptions your data must meet if you want to use Pearson’s r :
- Both variables are on an interval or ratio level of measurement
- Data from both variables follow normal distributions
- Your data have no outliers
- Your data is from a random or representative sample
- You expect a linear relationship between the two variables
A correlation coefficient is a single number that describes the strength and direction of the relationship between your variables.
Different types of correlation coefficients might be appropriate for your data based on their levels of measurement and distributions . The Pearson product-moment correlation coefficient (Pearson’s r ) is commonly used to assess a linear relationship between two quantitative variables.
There are various ways to improve power:
- Increase the potential effect size by manipulating your independent variable more strongly,
- Increase sample size,
- Increase the significance level (alpha),
- Reduce measurement error by increasing the precision and accuracy of your measurement devices and procedures,
- Use a one-tailed test instead of a two-tailed test for t tests and z tests.
A power analysis is a calculation that helps you determine a minimum sample size for your study. It’s made up of four main components. If you know or have estimates for any three of these, you can calculate the fourth component.
- Statistical power : the likelihood that a test will detect an effect of a certain size if there is one, usually set at 80% or higher.
- Sample size : the minimum number of observations needed to observe an effect of a certain size with a given power level.
- Significance level (alpha) : the maximum risk of rejecting a true null hypothesis that you are willing to take, usually set at 5%.
- Expected effect size : a standardized way of expressing the magnitude of the expected result of your study, usually based on similar studies or a pilot study.
Null and alternative hypotheses are used in statistical hypothesis testing . The null hypothesis of a test always predicts no effect or no relationship between variables, while the alternative hypothesis states your research prediction of an effect or relationship.
Statistical analysis is the main method for analyzing quantitative research data . It uses probabilities and models to test predictions about a population from sample data.
The risk of making a Type II error is inversely related to the statistical power of a test. Power is the extent to which a test can correctly detect a real effect when there is one.
To (indirectly) reduce the risk of a Type II error, you can increase the sample size or the significance level to increase statistical power.
The risk of making a Type I error is the significance level (or alpha) that you choose. That’s a value that you set at the beginning of your study to assess the statistical probability of obtaining your results ( p value ).
The significance level is usually set at 0.05 or 5%. This means that your results only have a 5% chance of occurring, or less, if the null hypothesis is actually true.
To reduce the Type I error probability, you can set a lower significance level.
In statistics, a Type I error means rejecting the null hypothesis when it’s actually true, while a Type II error means failing to reject the null hypothesis when it’s actually false.
In statistics, power refers to the likelihood of a hypothesis test detecting a true effect if there is one. A statistically powerful test is more likely to reject a false negative (a Type II error).
If you don’t ensure enough power in your study, you may not be able to detect a statistically significant result even when it has practical significance. Your study might not have the ability to answer your research question.
While statistical significance shows that an effect exists in a study, practical significance shows that the effect is large enough to be meaningful in the real world.
Statistical significance is denoted by p -values whereas practical significance is represented by effect sizes .
There are dozens of measures of effect sizes . The most common effect sizes are Cohen’s d and Pearson’s r . Cohen’s d measures the size of the difference between two groups while Pearson’s r measures the strength of the relationship between two variables .
Effect size tells you how meaningful the relationship between variables or the difference between groups is.
A large effect size means that a research finding has practical significance, while a small effect size indicates limited practical applications.
Using descriptive and inferential statistics , you can make two types of estimates about the population : point estimates and interval estimates.
- A point estimate is a single value estimate of a parameter . For instance, a sample mean is a point estimate of a population mean.
- An interval estimate gives you a range of values where the parameter is expected to lie. A confidence interval is the most common type of interval estimate.
Both types of estimates are important for gathering a clear idea of where a parameter is likely to lie.
Standard error and standard deviation are both measures of variability . The standard deviation reflects variability within a sample, while the standard error estimates the variability across samples of a population.
The standard error of the mean , or simply standard error , indicates how different the population mean is likely to be from a sample mean. It tells you how much the sample mean would vary if you were to repeat a study using new samples from within a single population.
To figure out whether a given number is a parameter or a statistic , ask yourself the following:
- Does the number describe a whole, complete population where every member can be reached for data collection ?
- Is it possible to collect data for this number from every member of the population in a reasonable time frame?
If the answer is yes to both questions, the number is likely to be a parameter. For small populations, data can be collected from the whole population and summarized in parameters.
If the answer is no to either of the questions, then the number is more likely to be a statistic.
The arithmetic mean is the most commonly used mean. It’s often simply called the mean or the average. But there are some other types of means you can calculate depending on your research purposes:
- Weighted mean: some values contribute more to the mean than others.
- Geometric mean : values are multiplied rather than summed up.
- Harmonic mean: reciprocals of values are used instead of the values themselves.
You can find the mean , or average, of a data set in two simple steps:
- Find the sum of the values by adding them all up.
- Divide the sum by the number of values in the data set.
This method is the same whether you are dealing with sample or population data or positive or negative numbers.
The median is the most informative measure of central tendency for skewed distributions or distributions with outliers. For example, the median is often used as a measure of central tendency for income distributions, which are generally highly skewed.
Because the median only uses one or two values, it’s unaffected by extreme outliers or non-symmetric distributions of scores. In contrast, the mean and mode can vary in skewed distributions.
To find the median , first order your data. Then calculate the middle position based on n , the number of values in your data set.

A data set can often have no mode, one mode or more than one mode – it all depends on how many different values repeat most frequently.
Your data can be:
- without any mode
- unimodal, with one mode,
- bimodal, with two modes,
- trimodal, with three modes, or
- multimodal, with four or more modes.
To find the mode :
- If your data is numerical or quantitative, order the values from low to high.
- If it is categorical, sort the values by group, in any order.
Then you simply need to identify the most frequently occurring value.
The interquartile range is the best measure of variability for skewed distributions or data sets with outliers. Because it’s based on values that come from the middle half of the distribution, it’s unlikely to be influenced by outliers .
The two most common methods for calculating interquartile range are the exclusive and inclusive methods.
The exclusive method excludes the median when identifying Q1 and Q3, while the inclusive method includes the median as a value in the data set in identifying the quartiles.
For each of these methods, you’ll need different procedures for finding the median, Q1 and Q3 depending on whether your sample size is even- or odd-numbered. The exclusive method works best for even-numbered sample sizes, while the inclusive method is often used with odd-numbered sample sizes.
While the range gives you the spread of the whole data set, the interquartile range gives you the spread of the middle half of a data set.
Homoscedasticity, or homogeneity of variances, is an assumption of equal or similar variances in different groups being compared.
This is an important assumption of parametric statistical tests because they are sensitive to any dissimilarities. Uneven variances in samples result in biased and skewed test results.
Statistical tests such as variance tests or the analysis of variance (ANOVA) use sample variance to assess group differences of populations. They use the variances of the samples to assess whether the populations they come from significantly differ from each other.
Variance is the average squared deviations from the mean, while standard deviation is the square root of this number. Both measures reflect variability in a distribution, but their units differ:
- Standard deviation is expressed in the same units as the original values (e.g., minutes or meters).
- Variance is expressed in much larger units (e.g., meters squared).
Although the units of variance are harder to intuitively understand, variance is important in statistical tests .
The empirical rule, or the 68-95-99.7 rule, tells you where most of the values lie in a normal distribution :
- Around 68% of values are within 1 standard deviation of the mean.
- Around 95% of values are within 2 standard deviations of the mean.
- Around 99.7% of values are within 3 standard deviations of the mean.
The empirical rule is a quick way to get an overview of your data and check for any outliers or extreme values that don’t follow this pattern.
In a normal distribution , data are symmetrically distributed with no skew. Most values cluster around a central region, with values tapering off as they go further away from the center.
The measures of central tendency (mean, mode, and median) are exactly the same in a normal distribution.
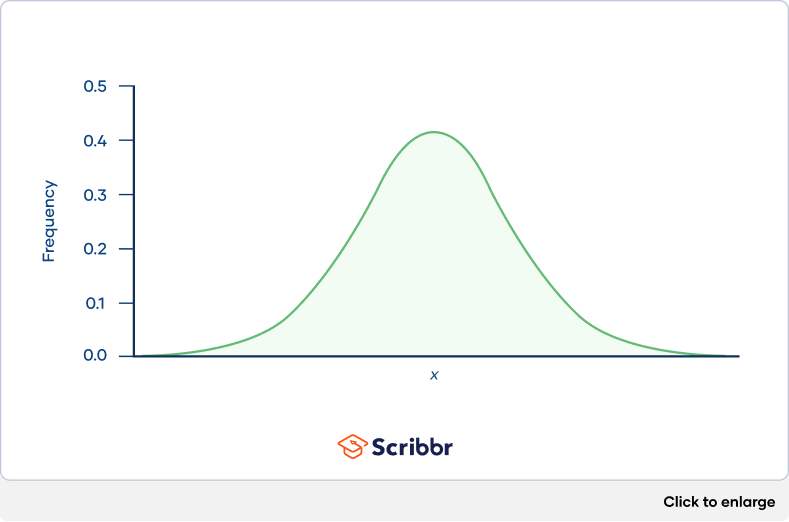
The standard deviation is the average amount of variability in your data set. It tells you, on average, how far each score lies from the mean .
In normal distributions, a high standard deviation means that values are generally far from the mean, while a low standard deviation indicates that values are clustered close to the mean.
No. Because the range formula subtracts the lowest number from the highest number, the range is always zero or a positive number.
In statistics, the range is the spread of your data from the lowest to the highest value in the distribution. It is the simplest measure of variability .
While central tendency tells you where most of your data points lie, variability summarizes how far apart your points from each other.
Data sets can have the same central tendency but different levels of variability or vice versa . Together, they give you a complete picture of your data.
Variability is most commonly measured with the following descriptive statistics :
- Range : the difference between the highest and lowest values
- Interquartile range : the range of the middle half of a distribution
- Standard deviation : average distance from the mean
- Variance : average of squared distances from the mean
Variability tells you how far apart points lie from each other and from the center of a distribution or a data set.
Variability is also referred to as spread, scatter or dispersion.
While interval and ratio data can both be categorized, ranked, and have equal spacing between adjacent values, only ratio scales have a true zero.
For example, temperature in Celsius or Fahrenheit is at an interval scale because zero is not the lowest possible temperature. In the Kelvin scale, a ratio scale, zero represents a total lack of thermal energy.
A critical value is the value of the test statistic which defines the upper and lower bounds of a confidence interval , or which defines the threshold of statistical significance in a statistical test. It describes how far from the mean of the distribution you have to go to cover a certain amount of the total variation in the data (i.e. 90%, 95%, 99%).
If you are constructing a 95% confidence interval and are using a threshold of statistical significance of p = 0.05, then your critical value will be identical in both cases.
The t -distribution gives more probability to observations in the tails of the distribution than the standard normal distribution (a.k.a. the z -distribution).
In this way, the t -distribution is more conservative than the standard normal distribution: to reach the same level of confidence or statistical significance , you will need to include a wider range of the data.
A t -score (a.k.a. a t -value) is equivalent to the number of standard deviations away from the mean of the t -distribution .
The t -score is the test statistic used in t -tests and regression tests. It can also be used to describe how far from the mean an observation is when the data follow a t -distribution.
The t -distribution is a way of describing a set of observations where most observations fall close to the mean , and the rest of the observations make up the tails on either side. It is a type of normal distribution used for smaller sample sizes, where the variance in the data is unknown.
The t -distribution forms a bell curve when plotted on a graph. It can be described mathematically using the mean and the standard deviation .
In statistics, ordinal and nominal variables are both considered categorical variables .
Even though ordinal data can sometimes be numerical, not all mathematical operations can be performed on them.
Ordinal data has two characteristics:
- The data can be classified into different categories within a variable.
- The categories have a natural ranked order.
However, unlike with interval data, the distances between the categories are uneven or unknown.
Nominal and ordinal are two of the four levels of measurement . Nominal level data can only be classified, while ordinal level data can be classified and ordered.
Nominal data is data that can be labelled or classified into mutually exclusive categories within a variable. These categories cannot be ordered in a meaningful way.
For example, for the nominal variable of preferred mode of transportation, you may have the categories of car, bus, train, tram or bicycle.
If your confidence interval for a difference between groups includes zero, that means that if you run your experiment again you have a good chance of finding no difference between groups.
If your confidence interval for a correlation or regression includes zero, that means that if you run your experiment again there is a good chance of finding no correlation in your data.
In both of these cases, you will also find a high p -value when you run your statistical test, meaning that your results could have occurred under the null hypothesis of no relationship between variables or no difference between groups.
If you want to calculate a confidence interval around the mean of data that is not normally distributed , you have two choices:
- Find a distribution that matches the shape of your data and use that distribution to calculate the confidence interval.
- Perform a transformation on your data to make it fit a normal distribution, and then find the confidence interval for the transformed data.
The standard normal distribution , also called the z -distribution, is a special normal distribution where the mean is 0 and the standard deviation is 1.
Any normal distribution can be converted into the standard normal distribution by turning the individual values into z -scores. In a z -distribution, z -scores tell you how many standard deviations away from the mean each value lies.
The z -score and t -score (aka z -value and t -value) show how many standard deviations away from the mean of the distribution you are, assuming your data follow a z -distribution or a t -distribution .
These scores are used in statistical tests to show how far from the mean of the predicted distribution your statistical estimate is. If your test produces a z -score of 2.5, this means that your estimate is 2.5 standard deviations from the predicted mean.
The predicted mean and distribution of your estimate are generated by the null hypothesis of the statistical test you are using. The more standard deviations away from the predicted mean your estimate is, the less likely it is that the estimate could have occurred under the null hypothesis .
To calculate the confidence interval , you need to know:
- The point estimate you are constructing the confidence interval for
- The critical values for the test statistic
- The standard deviation of the sample
- The sample size
Then you can plug these components into the confidence interval formula that corresponds to your data. The formula depends on the type of estimate (e.g. a mean or a proportion) and on the distribution of your data.
The confidence level is the percentage of times you expect to get close to the same estimate if you run your experiment again or resample the population in the same way.
The confidence interval consists of the upper and lower bounds of the estimate you expect to find at a given level of confidence.
For example, if you are estimating a 95% confidence interval around the mean proportion of female babies born every year based on a random sample of babies, you might find an upper bound of 0.56 and a lower bound of 0.48. These are the upper and lower bounds of the confidence interval. The confidence level is 95%.
The mean is the most frequently used measure of central tendency because it uses all values in the data set to give you an average.
For data from skewed distributions, the median is better than the mean because it isn’t influenced by extremely large values.
The mode is the only measure you can use for nominal or categorical data that can’t be ordered.
The measures of central tendency you can use depends on the level of measurement of your data.
- For a nominal level, you can only use the mode to find the most frequent value.
- For an ordinal level or ranked data, you can also use the median to find the value in the middle of your data set.
- For interval or ratio levels, in addition to the mode and median, you can use the mean to find the average value.
Measures of central tendency help you find the middle, or the average, of a data set.
The 3 most common measures of central tendency are the mean, median and mode.
- The mode is the most frequent value.
- The median is the middle number in an ordered data set.
- The mean is the sum of all values divided by the total number of values.
Some variables have fixed levels. For example, gender and ethnicity are always nominal level data because they cannot be ranked.
However, for other variables, you can choose the level of measurement . For example, income is a variable that can be recorded on an ordinal or a ratio scale:
- At an ordinal level , you could create 5 income groupings and code the incomes that fall within them from 1–5.
- At a ratio level , you would record exact numbers for income.
If you have a choice, the ratio level is always preferable because you can analyze data in more ways. The higher the level of measurement, the more precise your data is.
The level at which you measure a variable determines how you can analyze your data.
Depending on the level of measurement , you can perform different descriptive statistics to get an overall summary of your data and inferential statistics to see if your results support or refute your hypothesis .
Levels of measurement tell you how precisely variables are recorded. There are 4 levels of measurement, which can be ranked from low to high:
- Nominal : the data can only be categorized.
- Ordinal : the data can be categorized and ranked.
- Interval : the data can be categorized and ranked, and evenly spaced.
- Ratio : the data can be categorized, ranked, evenly spaced and has a natural zero.
No. The p -value only tells you how likely the data you have observed is to have occurred under the null hypothesis .
If the p -value is below your threshold of significance (typically p < 0.05), then you can reject the null hypothesis, but this does not necessarily mean that your alternative hypothesis is true.
The alpha value, or the threshold for statistical significance , is arbitrary – which value you use depends on your field of study.
In most cases, researchers use an alpha of 0.05, which means that there is a less than 5% chance that the data being tested could have occurred under the null hypothesis.
P -values are usually automatically calculated by the program you use to perform your statistical test. They can also be estimated using p -value tables for the relevant test statistic .
P -values are calculated from the null distribution of the test statistic. They tell you how often a test statistic is expected to occur under the null hypothesis of the statistical test, based on where it falls in the null distribution.
If the test statistic is far from the mean of the null distribution, then the p -value will be small, showing that the test statistic is not likely to have occurred under the null hypothesis.
A p -value , or probability value, is a number describing how likely it is that your data would have occurred under the null hypothesis of your statistical test .
The test statistic you use will be determined by the statistical test.
You can choose the right statistical test by looking at what type of data you have collected and what type of relationship you want to test.
The test statistic will change based on the number of observations in your data, how variable your observations are, and how strong the underlying patterns in the data are.
For example, if one data set has higher variability while another has lower variability, the first data set will produce a test statistic closer to the null hypothesis , even if the true correlation between two variables is the same in either data set.
The formula for the test statistic depends on the statistical test being used.
Generally, the test statistic is calculated as the pattern in your data (i.e. the correlation between variables or difference between groups) divided by the variance in the data (i.e. the standard deviation ).
- Univariate statistics summarize only one variable at a time.
- Bivariate statistics compare two variables .
- Multivariate statistics compare more than two variables .
The 3 main types of descriptive statistics concern the frequency distribution, central tendency, and variability of a dataset.
- Distribution refers to the frequencies of different responses.
- Measures of central tendency give you the average for each response.
- Measures of variability show you the spread or dispersion of your dataset.
Descriptive statistics summarize the characteristics of a data set. Inferential statistics allow you to test a hypothesis or assess whether your data is generalizable to the broader population.
In statistics, model selection is a process researchers use to compare the relative value of different statistical models and determine which one is the best fit for the observed data.
The Akaike information criterion is one of the most common methods of model selection. AIC weights the ability of the model to predict the observed data against the number of parameters the model requires to reach that level of precision.
AIC model selection can help researchers find a model that explains the observed variation in their data while avoiding overfitting.
In statistics, a model is the collection of one or more independent variables and their predicted interactions that researchers use to try to explain variation in their dependent variable.
You can test a model using a statistical test . To compare how well different models fit your data, you can use Akaike’s information criterion for model selection.
The Akaike information criterion is calculated from the maximum log-likelihood of the model and the number of parameters (K) used to reach that likelihood. The AIC function is 2K – 2(log-likelihood) .
Lower AIC values indicate a better-fit model, and a model with a delta-AIC (the difference between the two AIC values being compared) of more than -2 is considered significantly better than the model it is being compared to.
The Akaike information criterion is a mathematical test used to evaluate how well a model fits the data it is meant to describe. It penalizes models which use more independent variables (parameters) as a way to avoid over-fitting.
AIC is most often used to compare the relative goodness-of-fit among different models under consideration and to then choose the model that best fits the data.
A factorial ANOVA is any ANOVA that uses more than one categorical independent variable . A two-way ANOVA is a type of factorial ANOVA.
Some examples of factorial ANOVAs include:
- Testing the combined effects of vaccination (vaccinated or not vaccinated) and health status (healthy or pre-existing condition) on the rate of flu infection in a population.
- Testing the effects of marital status (married, single, divorced, widowed), job status (employed, self-employed, unemployed, retired), and family history (no family history, some family history) on the incidence of depression in a population.
- Testing the effects of feed type (type A, B, or C) and barn crowding (not crowded, somewhat crowded, very crowded) on the final weight of chickens in a commercial farming operation.
In ANOVA, the null hypothesis is that there is no difference among group means. If any group differs significantly from the overall group mean, then the ANOVA will report a statistically significant result.
Significant differences among group means are calculated using the F statistic, which is the ratio of the mean sum of squares (the variance explained by the independent variable) to the mean square error (the variance left over).
If the F statistic is higher than the critical value (the value of F that corresponds with your alpha value, usually 0.05), then the difference among groups is deemed statistically significant.
The only difference between one-way and two-way ANOVA is the number of independent variables . A one-way ANOVA has one independent variable, while a two-way ANOVA has two.
- One-way ANOVA : Testing the relationship between shoe brand (Nike, Adidas, Saucony, Hoka) and race finish times in a marathon.
- Two-way ANOVA : Testing the relationship between shoe brand (Nike, Adidas, Saucony, Hoka), runner age group (junior, senior, master’s), and race finishing times in a marathon.
All ANOVAs are designed to test for differences among three or more groups. If you are only testing for a difference between two groups, use a t-test instead.
Multiple linear regression is a regression model that estimates the relationship between a quantitative dependent variable and two or more independent variables using a straight line.
Linear regression most often uses mean-square error (MSE) to calculate the error of the model. MSE is calculated by:
- measuring the distance of the observed y-values from the predicted y-values at each value of x;
- squaring each of these distances;
- calculating the mean of each of the squared distances.
Linear regression fits a line to the data by finding the regression coefficient that results in the smallest MSE.
Simple linear regression is a regression model that estimates the relationship between one independent variable and one dependent variable using a straight line. Both variables should be quantitative.
For example, the relationship between temperature and the expansion of mercury in a thermometer can be modeled using a straight line: as temperature increases, the mercury expands. This linear relationship is so certain that we can use mercury thermometers to measure temperature.
A regression model is a statistical model that estimates the relationship between one dependent variable and one or more independent variables using a line (or a plane in the case of two or more independent variables).
A regression model can be used when the dependent variable is quantitative, except in the case of logistic regression, where the dependent variable is binary.
A t-test should not be used to measure differences among more than two groups, because the error structure for a t-test will underestimate the actual error when many groups are being compared.
If you want to compare the means of several groups at once, it’s best to use another statistical test such as ANOVA or a post-hoc test.
A one-sample t-test is used to compare a single population to a standard value (for example, to determine whether the average lifespan of a specific town is different from the country average).
A paired t-test is used to compare a single population before and after some experimental intervention or at two different points in time (for example, measuring student performance on a test before and after being taught the material).
A t-test measures the difference in group means divided by the pooled standard error of the two group means.
In this way, it calculates a number (the t-value) illustrating the magnitude of the difference between the two group means being compared, and estimates the likelihood that this difference exists purely by chance (p-value).
Your choice of t-test depends on whether you are studying one group or two groups, and whether you care about the direction of the difference in group means.
If you are studying one group, use a paired t-test to compare the group mean over time or after an intervention, or use a one-sample t-test to compare the group mean to a standard value. If you are studying two groups, use a two-sample t-test .
If you want to know only whether a difference exists, use a two-tailed test . If you want to know if one group mean is greater or less than the other, use a left-tailed or right-tailed one-tailed test .
A t-test is a statistical test that compares the means of two samples . It is used in hypothesis testing , with a null hypothesis that the difference in group means is zero and an alternate hypothesis that the difference in group means is different from zero.
Statistical significance is a term used by researchers to state that it is unlikely their observations could have occurred under the null hypothesis of a statistical test . Significance is usually denoted by a p -value , or probability value.
Statistical significance is arbitrary – it depends on the threshold, or alpha value, chosen by the researcher. The most common threshold is p < 0.05, which means that the data is likely to occur less than 5% of the time under the null hypothesis .
When the p -value falls below the chosen alpha value, then we say the result of the test is statistically significant.
A test statistic is a number calculated by a statistical test . It describes how far your observed data is from the null hypothesis of no relationship between variables or no difference among sample groups.
The test statistic tells you how different two or more groups are from the overall population mean , or how different a linear slope is from the slope predicted by a null hypothesis . Different test statistics are used in different statistical tests.
Statistical tests commonly assume that:
- the data are normally distributed
- the groups that are being compared have similar variance
- the data are independent
If your data does not meet these assumptions you might still be able to use a nonparametric statistical test , which have fewer requirements but also make weaker inferences.
Ask our team
Want to contact us directly? No problem. We are always here for you.
- Email [email protected]
- Start live chat
- Call +1 (510) 822-8066
- WhatsApp +31 20 261 6040
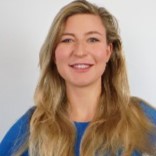
Our team helps students graduate by offering:
- A world-class citation generator
- Plagiarism Checker software powered by Turnitin
- Innovative Citation Checker software
- Professional proofreading services
- Over 300 helpful articles about academic writing, citing sources, plagiarism, and more
Scribbr specializes in editing study-related documents . We proofread:
- PhD dissertations
- Research proposals
- Personal statements
- Admission essays
- Motivation letters
- Reflection papers
- Journal articles
- Capstone projects
Scribbr’s Plagiarism Checker is powered by elements of Turnitin’s Similarity Checker , namely the plagiarism detection software and the Internet Archive and Premium Scholarly Publications content databases .
The add-on AI detector is powered by Scribbr’s proprietary software.
The Scribbr Citation Generator is developed using the open-source Citation Style Language (CSL) project and Frank Bennett’s citeproc-js . It’s the same technology used by dozens of other popular citation tools, including Mendeley and Zotero.
You can find all the citation styles and locales used in the Scribbr Citation Generator in our publicly accessible repository on Github .
- Trending Now
- Foundational Courses
- Data Science
- Practice Problem
- Machine Learning
- System Design
- DevOps Tutorial
Difference between Null and Alternate Hypothesis
Hypothesis is a statement or an assumption that may be true or false. There are six types of hypotheses mainly the Simple hypothesis, Complex hypothesis, Directional hypothesis, Associative hypothesis, and Null hypothesis. Usually, the hypothesis is the start point of any scientific investigation, It gives the right direction to the process of investigation. It avoids the blind search and gives direction to the search. It acts as a compass in the process.
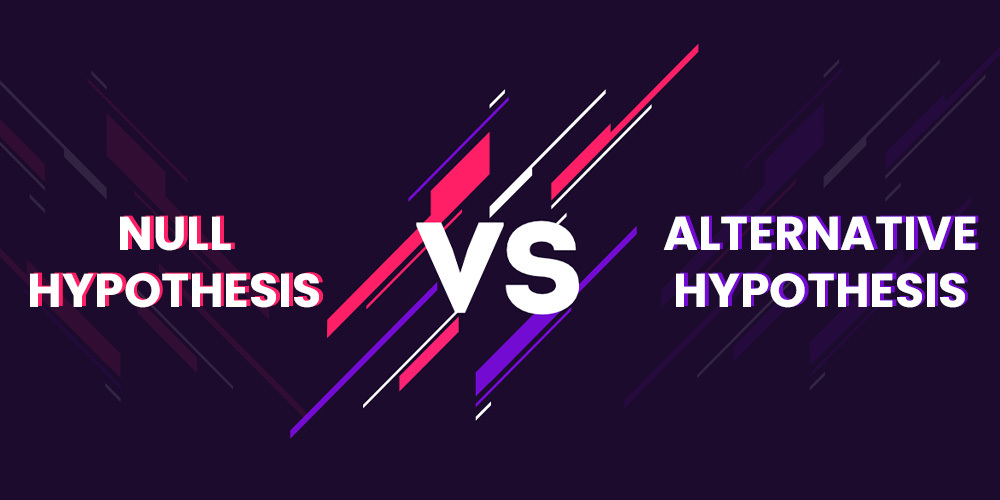
Null hypothesis suggests that there is no relationship between the two variables. Null hypothesis is also exactly the opposite of the alternative hypothesis. Null hypothesis is generally what researchers or scientists try to disprove and if the null hypothesis gets accepted then we have to make changes in our opinion i.e. we have to make changes in our original opinion or statement in order to match null hypothesis. Null hypothesis is represented as H0. If my alternative hypothesis is that 55% of boys in my town are taller than girls then my alternative hypothesis will be that 55% of boys in my town are not taller than girls.
Alternative hypothesis is a method for reaching a conclusion and making inferences and judgements about certain facts or a statement. This is done on the basis of the data which is available. Usually, the statement which we check regarding the null hypothesis is commonly known as the alternative hypothesis. Most of the times alternative hypothesis is exactly the opposite of the null hypothesis. This is what generally researchers or scientists try to approve. Alternative hypothesis is represented as Ha or H1. If my null hypothesis is that 55% of boys in my town are not taller than girls then my alternative hypothesis will be that 55% of boys in my town are taller than girls.
Following is the difference between the null hypothesis and alternate hypothesis:
|
| |
1. | In the null hypothesis, there is no relationship between the two variables. | In the alternative hypothesis, there is some relationship between the two variables i.e. They are dependent upon each other. |
2. | Generally, researchers and scientists try to reject or disprove the null hypothesis. | Generally, researchers and scientists try to accept or approve the null hypothesis |
3. | If the null hypothesis is accepted researchers have to make changes in their opinions and statements. | If the alternative hypothesis gets accepted researchers do not have to make changes in their opinions and statements. |
4. | Here no effect can be observed i.e. it does not affect output. | Here effect can be observed i.e. it affects the output. |
5. | Here the testing process is implicit and indirect. | Here the testing process is explicit and direct. |
6. | This hypothesis is denoted by H0. | This hypothesis is denoted by Ha or H1. |
7. | It is generally used when we reject the null hypothesis. | It gets accepted if we fail to reject the null hypothesis. |
8. | In this hypothesis, the p-value is smaller than the significance level. | In this hypothesis, the p-value is greater than the significance level. |
Please Login to comment...
Similar reads.
- Difference Between
- How to Delete Discord Servers: Step by Step Guide
- Google increases YouTube Premium price in India: Check our the latest plans
- California Lawmakers Pass Bill to Limit AI Replicas
- Best 10 IPTV Service Providers in Germany
- 15 Most Important Aptitude Topics For Placements [2024]
Improve your Coding Skills with Practice
What kind of Experience do you want to share?
- Open access
- Published: 30 August 2024
Assessment of MMP levels in reversible and irreversible pulpitis and a randomized controlled trial comparing clinical success of two different calcium-silicate cements in pulpotomy treatment of primary molars with an 18-month follow-up
- Hazal Ezgi Gerihan ORCID: orcid.org/0000-0002-7059-2326 1 nAff4 ,
- Dilşah Çoğulu ORCID: orcid.org/0000-0002-3156-9801 1 ,
- Özant Önçağ ORCID: orcid.org/0000-0001-7913-6928 1 ,
- Asude Durmaz ORCID: orcid.org/0000-0002-4109-9401 2 &
- Elif Hasibe Kuru ORCID: orcid.org/0000-0002-0396-7677 3
BMC Oral Health volume 24 , Article number: 1020 ( 2024 ) Cite this article
Matrix metalloproteinases (MMPs) are critical enzymes involved in the remodeling and defense mechanisms of dental pulp tissue. While their role in permanent teeth has been extensively studied, research focusing on MMPs in primary teeth remains limited. This gap highlights the need for further investigations to understand the specific contributions of MMPs to pulpal defense in primary teeth. Moreover, the clinical efficacy of Biodentine as a pulpotomy material in primary teeth warrants further exploration through well-designed studies to establish its success and long-term outcomes in pediatric dentistry.
This study aims to compare the expression levels of MMP-2, MMP-8, and MMP-9 in cases of reversible and irreversible pulpitis. Additionally, it seeks to evaluate the clinical success of Mineral Trioxide Aggregate (MTA) and Biodentine when used as pulpotomy agents in primary molars. By analyzing the differential expression of these MMPs, the study will contribute to a better understanding of their role in pulpal inflammation and the potential therapeutic outcomes of MTA and Biodentine in primary molars.
In this parallel randomized controlled trial, 63 mandibular primary second molars were assigned to two main groups: Group 1, consisting of 42 teeth diagnosed with reversible pulpitis, and Group 2, consisting of 21 teeth diagnosed with irreversible pulpitis. Group 1 was further divided into two randomized subgroups, each containing 21 teeth. The expression levels of MMP-2, MMP-8, and MMP-9 were evaluated in all samples. Pulpotomy treatments were performed using MTA and Biodentine in Group 1. Clinical and radiographic evaluations were conducted over an 18-month follow-up period. Statistical analyses were carried out using The Kolmogorov-Smirnov test, t-test and Fisher’s exact test ( p < 0.05).
The study revealed that MMP-2 and MMP-9 expression levels were significantly elevated in specimens with irreversible pulpitis ( p = 0.01), indicating a potential correlation between these matrix metalloproteinases and the severity of pulpal inflammation. However, no significant difference was observed in the clinical success rates of pulpotomies performed with MTA and Biodentine, suggesting that both materials are equally effective in the treatment of primary molars with reversible pulpitis.
Conclusions
The expression of MMP-2 and MMP-9 in pulpal blood presents a promising biomarker for assessing the degree of pulpal inflammation in primary teeth, offering a potentially valuable diagnostic tool. Additionally, the clinical success of Biodentine in pulpotomy procedures supports its viability as an effective alternative to MTA, providing a reliable option.
Clinical Trial Registration ID
The study protocol has been registered with an ID: NCT05145686. Registration Date: 9th November 2021.
Peer Review reports
Accurate assessment of pulp condition is critical to the success of pulp therapy in primary teeth [ 1 ]. Primary teeth, characterized by thinner hard tissue, wider dentinal tubules, and larger pulp chambers compared to permanent teeth, are more susceptible to pulp involvement [ 2 ]. Diagnosing pulpal diseases in children generally relies on the evaluation of clinical symptoms and radiographic findings. However, primary teeth do not possess a fully developed Raschkow plexus within the pulp-dentin complex, rendering thermal and electric pulp tests unreliable. Additionally, conducting proper radiological and clinical examinations can be particularly challenging in anxious pediatric patients or children with special needs, as their responses may lead to false positives [ 3 , 4 ].
Treatment for these patients is often performed under general anesthesia, with pulpal diagnosis mainly relying on operative findings where pulp bleeding control is managed [ 1 , 2 ]. The clinician is now at a point where they have to choose between two options, root canal treatment and pulpotomy. Particularly in cases of partial pulpal necrosis, it is challenging to determine the extent of necrosis by clinical observation. Moreover, during the late stages of primary teeth, bleeding control may not be enough for a safe diagnosis in some cases [ 3 , 4 ]. In this instance, there is a need for a diagnostic tool that uses biomarkers from pulpal bleeding.
Various biomarkers have been studied for assessing pulpal inflammation, such as interleukins, matrix metalloproteinases (MMPs), tissue growth factors, neurokinins, vasoactive intestinal peptides, the receptor for advanced glycation end products [ 5 , 6 , 7 ]. Recently, there has been a growing interest in investigating matrix metalloproteinases (MMPs), a subgroup of calcium and zinc-dependent endopeptidases, for their potential role in diagnosing dental inflammatory processes [ 8 ].
MMPs are proteolytic enzymes involved in various pathophysiological conditions including inflammatory processes [ 9 ]. It is believed that MMP-2, MMP-8, and MMP-9 are specifically involved in dental pathologies. Additionally, they are suspected to be involved in extracellular matrix (ECM) degradation and growth factor release in pulpal inflammation [ 10 , 11 , 12 ]. These MMPs are essential for tissue remodeling as they destroy protein components of the ECM [ 13 ].
Current literature indicates that pathological pulp tissue in permanent teeth exhibits significantly higher levels of MMP-1 and MMP-2 compared to healthy pulp tissue [ 12 , 18 , 19 ]. However, there is a significant lack of studies investigating the potential changes in intrapulpal MMP expression in primary teeth. Prospective assessment of pulpal inflammation based on these inflammatory biomarkers could enhance clinicians’ ability to formulate more accurate treatment plans, thereby improving prognosis, reducing the likelihood of treatment failures, and preventing the need for recurrent interventions.
Preserving the vitality of primary teeth affected by inflammation of the coronal pulp is commonly achieved through a vital pulpotomy. This procedure is only used when the radicular pulp is vital and not affected [ 4 , 14 ]. Different methods and medications are used to treat the surface of the remaining vital pulp tissue. These include formocresol, ferric sulfate, calcium hydroxide, glutaraldehyde, sodium hypochlorite, bone morphogenic proteins, dentin bonding agents, enamel matrix derivatives, growth factors, MTA (Mineral Trioxide Aggregate), Biodentine, electrosurgery, and lasers [ 15 , 16 , 17 , 18 , 19 ].
Among the materials described before, MTA first appeared in dentistry literature in 1993 as a calcium silicate cement [ 20 ]. This material has several beneficial properties, including biocompatibility, bioactivity, hydrophilicity, radiopacity, sealing properties, and low solubility [ 21 , 22 ]. Yet, due to its suboptimal manipulation qualities and cost-intensive nature, novel calcium silicate-based materials with enhanced attributes were subsequently introduced [ 16 , 23 ].
Biodentine, a novel calcium-silicate cement was first introduced in 2009, specifically designed as a dentin replacement material [ 24 ]. Leveraging the MTA-based cement technology, Biodentine refines certain characteristics, including its physical attributes and ease of manipulation. Moreover, Biodentine exhibits resistance to microleakage and exerts an antimicrobial influence. In comparison to MTA, it stands as a relatively more user-friendly material for clinicians [ 25 , 26 ]. In a systematic review in 2021 [ 27 ] and a meta-analysis in 2019 [ 28 ], Biodentine was found to be as effective as MTA in mature permanent teeth. While MTA is considered as the gold standard material for pulpotomy, more studies with long-term results are needed for Biodentine in pulpotomies in primary teeth.
Therefore, this two-step in vivo study aimed to compare the expression levels of MMP-2, -8, and -9 in primary molars with reversible and irreversible pulpitis and to compare the two different pulpotomy agents (MTA and Biodentine) clinically and radiographically for 18 months. According to our null hypothesis, neither medicament type has a significant difference in terms of clinical success; and similar MMP-2, -8, and -9 expressions are present in primary molars with both reversible and irreversible pulpitis.
This single-center, single-blinded, parallel randomized controlled trial was conducted according to Consolidated Standards of Reporting Trials (CONSORT) 2010 guidelines [ 29 ]. The flowchart of the study is presented in Fig. 1 . The registration number of the study protocol is NCT05145686. The study received approval from the University’s Ethical Committee of the Medical School (Reference No: 16-7/14). Comprehensive information about the treatment protocol was provided to both the children and their parents, and written informed consent was obtained.
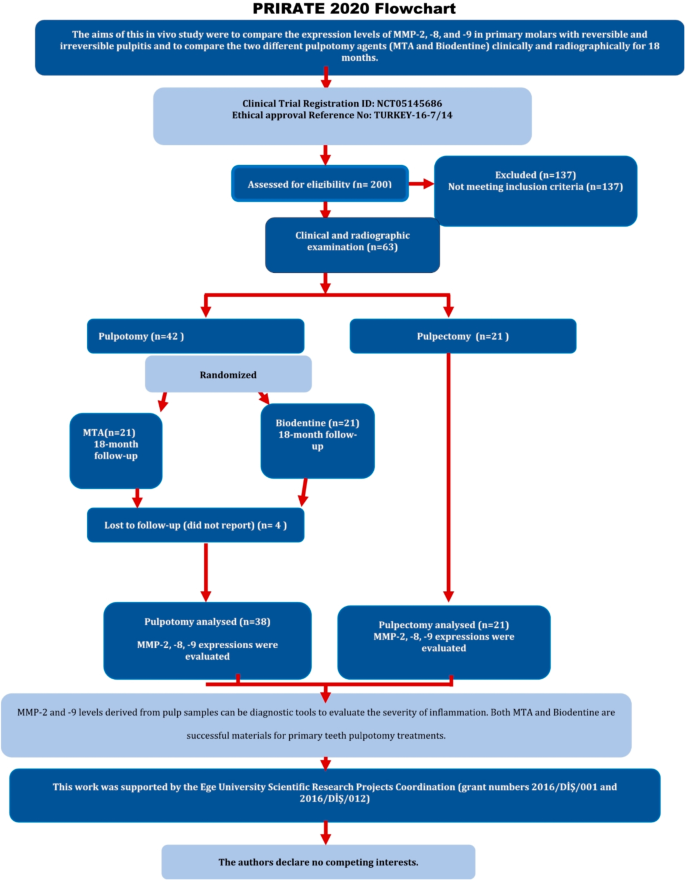
The PRIRATE flowchart of the study [ 34 ]
The primary second molar teeth of patients aged between 5 and 10 years were evaluated. Preceding any interventions, periapical radiographs were obtained to establish a baseline. Teeth exhibiting clinical or radiographical evidence of a profound carious lesion, extending close to or infiltrating the pulp chamber, were identified as potential candidates for inclusion in the study.
Inclusion criteria
Cooperative, healthy pediatric patients within the age range of 5 to 10 years.
A profound carious lesion without the existence of pathological root resorption and/or bone defect in the mandibular primary second molars.
The participants were not administered any anti-inflammatory medication within three months before the commencement of the study.
Restorable coronal structure.
Positive parental informed consent.
The sample size determination indicated that a total of 63 teeth would be required to achieve 80% statistical power to detect differences at a 5% significance level, assuming an effect size of 0.4 [ 35 ]. This sample size includes 21 teeth in the irreversible pulpitis group and 21 teeth in each of the reversible pulpitis subgroups treated with MTA and Biodentine.
A total of 63 mandibular primary second molar teeth from 57 healthy and cooperative children, aged between 5 and 10 years, who visited the pediatric dentistry department were included in this study. The teeth were assigned to different groups according to the clinical diagnosis. When the dental pulp was exposed during caries removal, the following clinical and radiographic diagnostic criteria were applied:
Group I (teeth with reversible pulpitis): mild clinical symptoms of minor intensity, slightly exaggerated reaction to cold or sweet stimuli, no history of spontaneous pain, no sensitivity to chewing or percussion, bleeding time from the exposed pulp tissue less than 5 min, and no widening of the periodontal ligament space. In the pulpotomy group teeth with pulpal exposure smaller than a pinpoint, teeth with purulent/viscous, dark-colored exudate detected at the exposure site, and necrotic teeth were excluded.
Group II (teeth with irreversible pulpitis): Spontaneous pain, sensitivity to chewing or percussion, bleeding time from the exposed pulp tissue of more than 5 min, and widening of the periodontal ligament space, periapical lesion.
For ethical reasons, there was no control group with blood samples from healthy, caries-free teeth. All primary molars were distributed between the two treatment groups as pulpotomy (Group I, n = 42) and pulpectomy (Group II, n = 21).
The pulpotomy procedures and all sampling were performed by the same operator (H.E.G.) following the procedure as follows: local anesthesia (2% lidocaine (1:000,000)- Jetokain Simplex, Adeka, Turkey) was administered, and teeth were isolated by a rubberdam (Roeko Dental Dam, Coltane, Whaledent, Germany). The carious dentin was removed from the cavity, working from the periphery toward the center using a low-speed round bur (no:4). The roof of the pulp chamber was removed, and coronal pulp tissue was taken using a sharp excavator. All pulp samples were placed in 1 ml Tri Pure Reagent transport medium in pre-weighed Eppendorf tubes. The samples were weighed again and their weights were noted before they were stored at -80 °C until the laboratory procedures. In the pulpectomy group (Group II), all sampling procedures were performed as same in the pulpotomy group.
In Group I, after removal of the coronal pulp, the cavity was irrigated with sterile saline, and initial bleeding was controlled by placing sterile cotton pellets of moisturizer with saline over the radicular pulp stump exerting slight pressure, and waiting for 5 min. The teeth for which hemostasis at the canal orifices could be achieved in 5 min were included in this group. The randomization was performed in two groups in a 1:1 ratio by using free online software ( http://www.random.org ). Following the randomization procedure, patients were assigned sequential numbers in the order of enrollment and the operator was informed about the material, either with MTA ( n = 21) or with Biodentine ( n = 21).
According to the manufacturer’s instructions, MTA (Pro Root White MTA, Dentsply, Tulsa Dental Specialties, USA) powder was mixed with sterile water in a 3:1 powder/water ratio to obtain a thick creamy paste, then placed on the floor of the pulp chamber. The MTA mixture was covered with a moistened cotton pellet and then temporarily sealed using glass ionomer cement (GIC-Ketac Molar Easymix, 3 M Espe, Seefeld, Germany) until the second visit.
Biodentine group
Biodentine (Septodont, Saint-Maur-des-Fossés, France) capsule was gently tapped on a hard surface to diffuse powder, five drops of liquid from the single dose dispenser were poured into the capsule and then mixed with 4200 rpm 30 s and placed over the pulp stumps using an appropriate spatula advised by the manufacturer. After allowing Biodentine to set for 12 min, the teeth were temporarily sealed with GIC as same in the MTA group. After 24 h, the teeth in both groups were restored with preformed stainless-steel crowns (3 M Espe, Stainless Steel Crowns, Seefeld, Germany).
Group II was treated with pulpectomy. A single dentist (H.E.G) treated each involved molar over two visits. During the first appointment, the molar was isolated with a rubber dam after administering local anesthesia. The pulp chamber was accessed by removing all carious tooth structures, and pulpal debris was cleared using barbed broaches. The working length was determined by Electronic Apex Locator (Root ZX mini) and a Mani K files (MANI Inc., Tochigi, Japan). Cleaning and shaping of the root canals were performed using Mani K files (MANI Inc., Tochigi, Japan) in a pullback direction, with sizes ranging from 35 to 40. Continuous irrigation with 2.5% sodium hypochlorite was conducted throughout the procedure, and sterile paper points were used to dry the root canals. Calcium hydroxide (Multi-Cal, Pulpdent Corporation, Watertown, MA, USA) was then injected into the root canal, and a sterile cotton ball was placed in the pulp chamber, which was sealed with Cavit (3 M ESPE, St. Paul, MN, USA) as a temporary sealing material. At the second visit, typically occurring two weeks later, the root canal was irrigated again with 2.5% sodium hypochlorite and dried with sterile paper points. The prepared molars were then randomly allocated to Vitapex (Neo Dental Chemical Products Co. Ltd., Tokyo, Japan), SSC restoration will be performed, and finally, a post-treatment radiograph will be taken.
The participants were recalled at 6, 12, and 18 months after the treatment. At each follow-up visit, clinical and radiographic evaluations were performed by two calibrated blinded examiners. All teeth were evaluated clinically and radiographically according to the criteria: (1) absence of spontaneous pain and/or sensitivity to pressure, (2) absence of sinus, fistula, edema, and/or abnormal mobility, (3) absence of radiolucency at the inter radicular and/or periapical regions, (4) absence of internal or external root resorption. The absence of spontaneous pain, pathologic mobility, tenderness to percussion, swelling, fistula, and gingival inflammation was considered a clinical success, whereas the absence of internal/external root resorption and periapical/furcal radiolucency was considered a radiographic success. Calcific metamorphosis of the pulp was not considered a failure.
Pulp tissue samples obtained from the patients were stored at -80 o C until RNA extraction. 50–100 mg of each sample was used for RNA extraction using RNAiso Plus (TaKaRa Bio, Shiga, Japan). RNA concentration and quality were evaluated by A260/A280 and A230/A280 absorbance ratios using the Nanodrop (Thermo Fisher Scientific, Waltham, MA). cDNA was synthesized from 400 ng of total RNA using the PrimeScript RT reagent kit (TaKaRa Bio, Otsu, Japan) according to the manufacturer’s instructions. One-tenth of the cDNA obtained was added for a final volume of 20 µL for each reaction containing SYBR Green I (TaKaRa Bio, Shiga, Japan). The primers for MMP-2, MMP-8, and MMP-9 were as follows (sense/antisense):
MMP-2: 5′-ATC CTG GCT TTC CCA AGC TC-3′/3′-CAC CCT TGA AGA AGT AGC TGT G-5′. MMP-8: 5′-CTGTTGAAGGCCTAGAGCTGCTGCTCC3′/5′CATCTTCTCTTCAAACTCTACCC-3′.
MMP-9: 5′-GGG CTT AGA TCA TTC CTC AGT G-3′/3′-GCC ATT CAC GTC GTC CTT AT-5′.
Reactions were run on an ABI Step One Real-Time PCR system (Applied Biosystems, Foster City, CA, USA) with the following program: 45 cycles of 95 °C for 10 s, 60 °C for 15 s, and 72 °C for 20s. The expression levels of each mRNA were normalized by the expression level of internal control gene G6PDH using primers 5′-ATGGGGAAGGTGAAGGTCG-3′ (forward) and 5′-GGGGTCATTGATGGCAACAATA-3′ (reverse). For relative comparisons of each gene, we analyzed the threshold cycle (Ct) value of the real-time PCR data using the 2−ΔΔCt method (Fig. 2 ) [ 12 , 30 , 31 ].
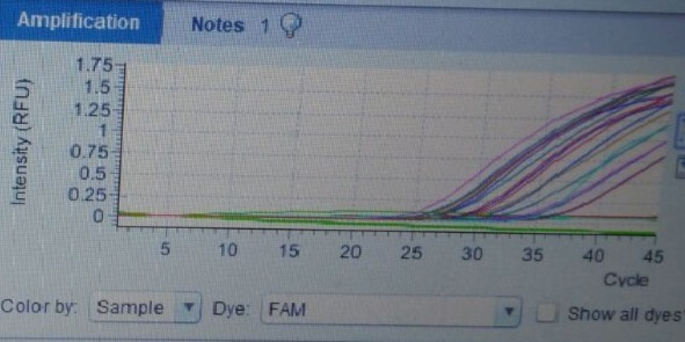
Representative graphical presentation of RT-qPCR results
Data analysis and statistical methods
The statistical analysis was performed by an independent statistician using SPSS for windows version 25.0 (SPSS, Chicago, IL, USA). A p-value of <0.05 was considered statistically significant. The Kolmogorov-Smirnov test was used to assess the normality of the data for MMP-2, MMP-8, and MMP-9 expression levels in each group. Since MMP-2, MMP-8, and MMP-9 expression levels were found to be normally distributed within each group, an independent samples t-test was used to compare the results. The clinical, and radiographic results were analyzed by the Fisher’s exact test ( p < 0.05).
The study was conducted on a total of 63 mandibular primary second molars in 57 children (29 males and 28 females) with a mean age of 6.53 ± 1.32 years. The reversible pulpitis group included 42 teeth and the irreversible group included 21 teeth. In the reversible pulpitis group, a total of 21 mandibular primary second molar teeth were subjected to vital pulpotomy using mineral trioxide aggregate (MTA), whereas an additional 21 teeth underwent the same procedure applying Biodentine. A total of 18 left and 24 right mandibular primary second molars were treated in the reversible pulpitis group.
The mean MMP-2, -8, and -9 expression levels according to the reversible and irreversible pulpitis groups are shown in Table 1 . Irreversible pulpitis specimens exhibited significantly higher MMP-2 and MMP-9 mRNA gene expression than the reversible pulpitis group ( p = 0.01). No significant difference was found between the MMP-8 expression level and the treatment groups ( p > 0.05).
Evaluation of clinical and radiographic success
The clinical success outcomes for MTA and Biodentine at the 6, 12, and 18-month follow-up intervals are outlined in Table 2 . At the 6-month follow-up, 40 out of 42 teeth were assessed for the reversible pulpitis group (2 dropouts), while the count was 38 for both groups at the 12 and 18-month follow-ups (4 dropouts). The clinical success rate stood at 100% for both MTA and Biodentine at the 6 and 12-month assessments, declining to 95% for MTA and 94.4% for Biodentine at the 18-month assessment.
Regarding radiographic success (Table 3 ), MTA showed 100% success rate at the 6-month follow-up, while Biodentine exhibited a rate of 94.7%. At the 12-month and 18-month follow-ups, MTA maintained a radiographic success rate of 100% and 95%, respectively. However, Biodentine’s radiographic success rate was 83.3% for both the 12 and 18-month assessments. An example of radiographs taken at baseline and 18 months after pulpotomies are shown in (Fig. 3 ).
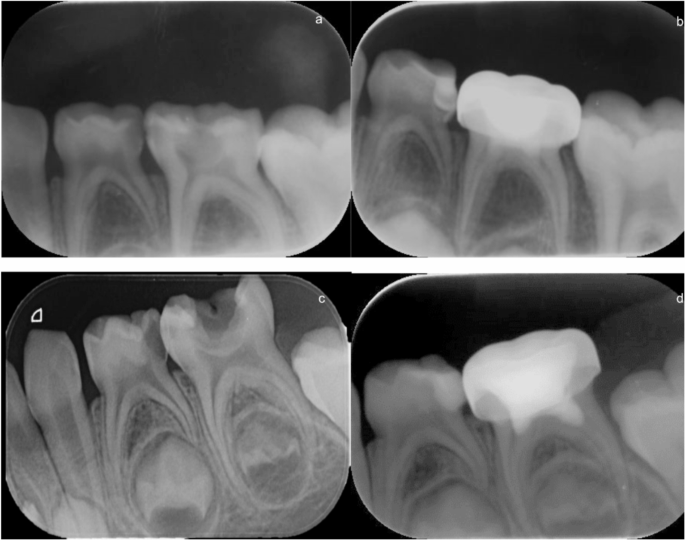
Periapical radiographs of primary second molars before ( a , c ) and 18 months after ( b , d ) pulpotomy treatments. ( b : pulpotomy with Biodentine, d : pulpotomy with MTA)
Analysis of total success rate and MMP expression
Comparing the overall success rate from the start of treatment to the 18-month mark, no significant difference found between the two material groups ( p > 0.05). Radiographic failure was notably more prevalent than clinical failure in both material groups. Although the radiographic failure rate was higher in the Biodentine group compared to MTA at 12 and 18 months, this difference did not attain statistical significance ( p > 0.05).
In terms of MMP-2 and -9 expression levels, clinically and radiographically unsuccessful pulpotomy treatments exhibited notably higher levels compared to the successful treatment group, regardless of the material applied ( p = 0.01). Conversely, no significant difference was observed in MMP-8 expression levels concerning the unsuccessful pulpotomy treatments ( p > 0.05) (Tables 4 and 5 ).
In the present study, in the reversible pulpitis group, there was no significant difference between MTA and Biodentine pulpotomy treatments in clinical and radiologic evaluation with 18-month follow-up. Thus, the first hypothesis was accepted. Also, MMP-2 and -9 expressions were significantly higher in the irreversible pulpitis group than the reversible pulpitis group; therefore, the second hypothesis was rejected. Based on our findings, MMP-2 and -9 levels were successful in determining the pulp inflammation status in primary teeth, and Biodentine showed similar success as MTA in pulpotomy treatments in primary teeth.
Matrix metalloproteinases (MMPs) are a family of 24 zinc-dependent endopeptidases involved in the degradation of extracellular matrix (ECM) components, essential for both normal tissue remodeling and pathological processes [ 7 , 32 ]. In the context of dental pulp tissue, Pulp tissue destruction is partially regulated by MMPs and tissue inhibitors of MMPs (TIMPs) [ 32 ]. MMP family proteins have dual roles in inflammation pathogenesis, stimulation of protective innate and adaptive immune functions and tissue destruction [ 33 ]. These enzymes are secreted by various cell types, including neutrophils, odontoblasts, and other cells within the dental pulp. During pulpal inflammation, MMPs, such as MMP-2, MMP-8, and particularly MMP-9, are activated and participate in the breakdown of ECM components like collagen, leading to pulp tissue degradation [ 7 ].
Our findings indicate that MMP-2 and MMP-9 expressions were significantly higher in the irreversible pulpitis group and in cases of pulpotomy failure, consistent with previous research showing increased MMP-9 levels in inflamed dental pulp tissues [ 34 , 35 , 36 ]. However, there was no significant difference in MMP-8 levels between the two groups. In contrast, Wahlgren et al. identified a role for MMP-8 in pulpal and periapical inflammation, contributing to ECM degradation and tissue remodeling [ 11 ]. This discrepancy in findings may be due to differences in methodologies, with Wahlgren’s study analyzing periapical exudates from permanent teeth undergoing root canal treatment (RCT), while our study focused on pulp samples from primary molars.
Despite the methodological differences, our findings align with the broader understanding of MMPs in pulp diseases. Notably, there is a lack of studies on MMP activity in primary teeth compared to permanent teeth. However, existing evidence in permanent teeth suggests that MMP-9, a member of the gelatinase family, is predominantly secreted by neutrophils, which are more abundant in inflamed pulp tissues than healthy pulp tissues [ 34 , 37 ]. Additionally, cytokines such as IL-8 and TNF-α, associated with neutrophil activity, are also present in primary tooth pulp inflammation, where they induce the rapid release of MMP-9 zymogen in human blood [ 38 ]. Scheffel et al. observed that while primary and permanent tooth tissues. initially exhibit similar MMP activity, collagen degradation occurs more rapidly and significantly in primary dentin over time [ 39 ]. These findings indicate that elevated levels of MMP-2 and MMP-9 could therefore serve as valuable diagnostic biomarkers for assessing pulpal status in primary teeth, though further studies are necessary to clarify these responses in pediatric populations.
In clinical part of the study, similar success rate of MTA and Biodentine can be attributed to the bioactive nature and odontoblast-promoting ability of calcium-silicate cements (CSC) [ 40 ]. Since its introduction in dentistry, MTA has mostly been used for endodontic applications, owing to its extended setting time and discoloration. On the other hand, Biodentine™ is a type of CSC with shorter setting time and color stability, which distinguishes it from the other CSC proposed for use as both endodontic and coronal restoration materials [ 41 , 42 , 43 ]. Similar results with different follow-up time were found in previous in-vivo studies [ 44 , 45 , 46 ]. While MTA is often considered the gold standard material for pulpotomies, Biodentine has similar success rates to other materials but offers a shorter setting time and better color stability, making it a viable alternative for primary teeth for both pulpotomy and dentin restorations.
The other factor in pulpotomy success is final coronal restoration. In the present study, in both groups, the final restorative material was stainless steel crowns (SSC). SSCs are more enduring and reliable restorations for endodontically treated primary molars [ 31 ] and recommended by American Association of Pediatric Dentistry [ 47 ].
Even though there was no statistically significant difference between the groups, low success rate over time in Biodentine group suggested that the follow-up period’s extension might have had an impact on the success rate differences. This is especially true given that numerous clinical studies have indicated that variations in follow-up duration affect success rates [ 38 , 48 , 49 , 50 ]. In our study, we determined our follow-up period as 18 months to observe the pulpal healing.
Our study has several strengths, as it is one of the few recent randomized clinical trials with MMP expression in the primary tooth with both irreversible and reversible pulpitis, as well as clinical and radiographic evaluation of Biodentine and MTA as pulpotomy materials with 18-month follow-up. Combining immunologic markers with clinical and radiologic evaluation led us to better understanding of material success and pulp healing mechanisms together. This clinical trial might be a groundwork for more research with more participants and longer follow–up times.
The present study has some limitations, such as restricted timeframe for follow up and strict criteria for participant selection. Operator blinding was unfeasible due to the materials requiring distinct manipulations, possibly introducing cognitive bias during procedures, although it did not affect follow-up assessments.
In conclusion, MMP-2 and -9 expression in pulpal blood shows potential for assessing pulpal inflammation and distinguishing between reversible and irreversible pulpitis in primary teeth. Furthermore, our study found similar success rates for pulpotomy treatments with MTA and Biodentine in primary molars over an 18-month follow-up. Biodentine’s clinical success and improved properties make it a viable alternative for pulpotomy and dentin restorations. Accurate biomarker-based diagnostics may reduce unnecessary RCTs by providing clinicians with better prognostic certainty for pulpotomy treatments in primary teeth.
Clinical relevance
MMP-2 and MMP-9 expression levels are significantly higher in primary teeth with irreversible pulpitis than reversible pulpitis.
MMP-2 and MMP-9 expressions are important to assess pulpal inflammation severity and can be used as prospective pulpal diagnosis tools in primary molars.
Biodentine is a successful pulpotomy material for carious primary teeth.
Data availability
The datasets used and/or analysed during the current study are available from the corresponding author on reasonable request.
Abbreviations
Matrix-metalloproteinases
Mineral Trioxide Aggregate
Glass ionomer cement
Extracellular matrix
Interleukin
Sevekar S, Jha MN, Avanti A. Characteristics and Comparison of Dental Treatment under General Anesthesia in Healthy Children and Children with Special Healthcare needs: a retrospective study. Int J Clin Pediatr Dent. 2021;14(Suppl 2):S157–61.
PubMed PubMed Central Google Scholar
Bergenholtz G. Inflammatory response of the dental pulp to bacterial irritation. J Endod. 1981;7(3):100–4.
Article CAS PubMed Google Scholar
Monteiro J, Day P, Duggal M, Morgan C, Rodd H. Pulpal status of human primary teeth with physiological root resorption. Int J Paediatr Dent. 2009;19(1):16–25.
Article PubMed Google Scholar
American Academy of Pediatric Dentistry. Pulp therapy for primary and immature permanent teeth. Pediatr Dent. 2017;39(6):325–33.
Google Scholar
Palosaari H, Wahlgren J, Larmas M, Rönkä H, Sorsa T, Salo T, et al. The expression of MMP-8 in human odontoblasts and dental pulp cells is down-regulated by TGF-beta1. J Dent Res. 2000;79(1):77–84.
Rechenberg D-K, Galicia JC, Peters OA, Zanini M, Meyer E, Simon S. Biological markers for Pulpal inflammation: a systematic review. PLoS ONE. 2016;11(11):e0167289.
Article PubMed PubMed Central Google Scholar
Zanini M, Meyer E, Simon S. Pulp inflammation diagnosis from clinical to Inflammatory mediators: a systematic review. J Endod. 2017;43(7):1033–51.
Jain A, Bahuguna R. Role of matrix metalloproteinases in dental caries, pulp and periapical inflammation: an overview. J oral Biol Craniofac Res. 2015;5(3):212–8.
Zheng L, Amano K, Iohara K, Ito M, Imabayashi K, Into T, et al. Matrix metalloproteinase-3 accelerates wound healing following dental pulp injury. Am J Pathol. 2009;175(5):1905–14.
Article CAS PubMed PubMed Central Google Scholar
Chang YC, Yang SF, Hsieh YS. Regulation of matrix metalloproteinase-2 production by cytokines and pharmacological agents in human pulp cell cultures. J Endod. 2001;27(11):679–82.
Wahlgren J, Salo T, Teronen O, Luoto H, Sorsa T, Tjäderhane L. Matrix metalloproteinase-8 (MMP-8) in pulpal and periapical inflammation and periapical root-canal exudates. Int Endod J. 2002;35(11):897–904.
Tsai C-H, Chen Y-J, Huang F-M, Su Y-F, Chang Y-C. The upregulation of matrix metalloproteinase-9 in inflamed human dental pulps. J Endod. 2005;31(12):860–2.
Lu P, Takai K, Weaver VM, Werb Z. Extracellular matrix degradation and remodeling in development and disease. Cold Spring Harb Perspect Biol. 2011;3(12).
Schröder U, Szpringer-Nodzak M, Janicha J, Wacińska M, Budny J, Mlosek K. A one-year follow‐up of partial pulpotomy and calcium hydroxide capping in primary molars. Dent Traumatol. 2006;3:304–6.
Article Google Scholar
Rivera N, Reyes E, Mazzaoui S, Morón A. Pulpal therapy for primary teeth: formocresol vs electrosurgery: a clinical study. J Dent Child (Chic). 2003;70(1):71–3.
PubMed Google Scholar
Vilella-Pastor S, Sáez S, Veloso A, Guinot-Jimeno F, Mercadé M. Long-term evaluation of primary teeth molar pulpotomies with Biodentine and MTA: a CONSORT randomized clinical trial. Eur Arch Paediatr Dent off J Eur Acad Paediatr Dent. 2021;22(4):685–92.
Article CAS Google Scholar
Torabinejad M, Chivian N. Clinical applications of mineral trioxide aggregate. J Endod. 1999;25(3):197–205.
Satyarth S, Alkhamis AM, Almunahi HF, Abdulaziz Alsuhaymi MO, Vadde HB, Senapathi SN, et al. Comparative evaluation of Mineral Trioxide Aggregate Pulpotomy and laser-assisted Mineral Trioxide Aggregate Pulpotomy: an Original Research Article. J Microsc Ultrastruct. 2021;9(1):7–11.
Ranly DM. Pulpotomy therapy in primary teeth: new modalities for old rationales. Pediatr Dent. 1994;16(6):403–9.
CAS PubMed Google Scholar
Camilleri J, Pitt Ford TR. Mineral trioxide aggregate: a review of the constituents and biological properties of the material. Int Endod J. 2006;39(10):747–54.
Parirokh M, Torabinejad M. Mineral trioxide aggregate: a comprehensive literature review–part III: clinical applications, drawbacks, and mechanism of action. J Endod. 2010;36(3):400–13.
Rao A, Rao A, Shenoy R. Mineral trioxide aggregate–a review. J Clin Pediatr Dent. 2009;34(1):1–7.
Simancas-Pallares M-A, Díaz-Caballero A-J, Luna-Ricardo L-M. Mineral trioxide aggregate in primary teeth pulpotomy. A systematic literature review. Med Oral Patol Oral Cir Bucal. 2010;15(6):e942–6.
de Lima SPR, Santos GL, Dos, Ferelle A, Ramos S, de Pessan P, Dezan-Garbelini JP. Clinical and radiographic evaluation of a new stain-free tricalcium silicate cement in pulpotomies. Braz Oral Res. 2020;34:e102.
Wong BJ, Fu E, Mathu-Muju KR. Thirty-Month outcomes of Biodentine ® Pulpotomies in primary molars: a retrospective review. Pediatr Dent. 2020;42(4):293–9.
Ahuja S, Surabhi K, Gandhi K, Kapoor R, Malhotra R, Kumar D. Comparative evaluation of success of Biodentine and Mineral Trioxide Aggregate with Formocresol as Pulpotomy medicaments in primary molars: an in vivo study. Int J Clin Pediatr Dent. 2020;13(2):167–73.
Cushley S, Duncan HF, Lappin MJ, Tomson PL, Lundy FT, Cooper P, et al. Pulpotomy for mature carious teeth with symptoms of irreversible pulpitis: a systematic review. J Dent. 2019;88:103158.
Mahmoud SH, El-Negoly SA, Zaen El-Din AM, El-Zekrid MH, Grawish LM, Grawish HM, et al. Biodentine versus mineral trioxide aggregate as a direct pulp capping material for human mature permanent teeth - A systematic review. J Conserv Dent. 2018;21(5):466–73.
Schulz KF, Altman DG, Moher D. CONSORT 2010 Statement: updated guidelines for reporting parallel group randomized trials. Open Med. 2010;4(1):e60–8.
Gusman H, Santana RB, Zehnder M. Matrix metalloproteinase levels and gelatinolytic activity in clinically healthy and inflamed human dental pulps. Eur J Oral Sci. 2002;110(5):353–7.
Soxman J. Stainless steel crown and pulpotomy: procedure and technique for primary molars. Gen Dent. 2000;48:294–7.
Nagase H, Woessner JFJ. Matrix metalloproteinases. J Biol Chem. 1999;274(31):21491–4.
Le NTV, Xue M, Castelnoble LA, Jackson CJ. The dual personalities of matrix metalloproteinases in inflammation. Front Biosci. 2007;12:1475–87.
Sharma R, Kumar V, Logani A, Chawla A, Mir RA, Sharma S, et al. Association between concentration of active MMP-9 in pulpal blood and pulpotomy outcome in permanent mature teeth with irreversible pulpitis – a preliminary study. Int Endod J. 2021;54(4):479–89.
Mente J, Petrovic J, Gehrig H, Rampf S, Michel A, Schürz A, et al. A prospective clinical pilot study on the level of Matrix Metalloproteinase-9 in Dental Pulpal blood as a marker for the state of inflammation in the pulp tissue. J Endod. 2016;42(2):190–7.
Petrovic J, Sekundo C, Gehrig H, Rampf S, El-Sayed S, Ritz A, et al. Outcome after partial pulpotomy: long-term results of the prospective clinical MMP-9 study. Oral Heal Prev Dent. 2023;21:331–8.
Chibinski ACR, Gomes JR, Camargo K, Reis A, Wambier DS. Bone sialoprotein, matrix metalloproteinases and type I collagen expression after sealing infected caries dentin in primary teeth. Caries Res. 2014;48(4):312–9.
Ozdemir Y, Kutukculer N, Topaloglu-Ak A, Kose T, Eronat C. Comparative evaluation of pro-inflammatory cytokine levels in pulpotomized primary molars. J Oral Sci. 2015;57(2):145–50.
Scheffel DLS, Cury JA, Tenuta LMA, Scheffel RH, Perez C, Soares DG, et al. Proteolytic activity, degradation, and dissolution of primary and permanent teeth. Int J Paediatr Dent. 2020;30(5):650–9.
Camilleri J. The biocompatibility of modified experimental Portland cements with potential for use in dentistry. Int Endod J. 2008;41(12):1107–14.
Carti O, Oznurhan F. Evaluation and comparison of mineral trioxide aggregate and biodentine in primary tooth pulpotomy: clinical and radiographic study. Niger J Clin Pract. 2017;20(12):1604–9.
Togaru H, Muppa R, Srinivas Nc, Naveen K, Reddy VK, Rebecca VC. Clinical and radiographic evaluation of success of two commercially available Pulpotomy agents in primary teeth: an in vivo study. J Contemp Dent Pract. 2016;17(7):557–63.
Cuadros-Fernández C, Lorente Rodríguez AI, Sáez-Martínez S, García-Binimelis J, About I, Mercadé M. Short-term treatment outcome of pulpotomies in primary molars using mineral trioxide aggregate and Biodentine: a randomized clinical trial. Clin Oral Investig. 2016;20(7):1639–45.
Bani M, Aktaş N, Çınar Ç, Odabaş ME. The clinical and radiographic success of primary molar pulpotomy using Biodentine™ and Mineral Trioxide Aggregate: a 24-Month Randomized Clinical Trial. Pediatr Dent. 2017;39(4):284–8.
Rajasekharan S, Martens LC, Vandenbulcke J, Jacquet W, Bottenberg P, Cauwels RGEC. Efficacy of three different pulpotomy agents in primary molars: a randomized control trial. Int Endod J. 2017;50(3):215–28.
Juneja P, Kulkarni S. Clinical and radiographic comparison of biodentine, mineral trioxide aggregate and formocresol as pulpotomy agents in primary molars. Eur Arch Paediatr Dent off J Eur Acad Paediatr Dent. 2017;18(4):271–8.
American Academy of Pediatric Dentistry. Pediatric Restorative Dentistry. The reference Manual of Pediatric Dentistry. Chicago, Ill. 2022.
Akcay M, Sari S, Duruturk L, Gunhan O. Effects of sodium hypoclorite as disinfectant material previous to pulpotomies in primary teeth. Clin Oral Investig. 2015;19(4):803–11.
Akcay M, Sari S. The effect of sodium hypochlorite application on the success of calcium hydroxide and mineral trioxide aggregate pulpotomies in primary teeth. Pediatr Dent. 2014;36(4):316–21.
Sönmez D, Durutürk L. Ca(OH)2 pulpotomy in primary teeth. Part I: internal resorption as a complication following pulpotomy. Oral Surg Oral Med Oral Pathol Oral Radiol Endod. 2008;106(2):e94–8.
Download references
Acknowledgements
Not applicable.
This work was supported by the Ege University Scientific Research Projects Coordination (grant numbers 2016/DİŞ/001 and 2016/DİŞ/012).
Author information
Hazal Ezgi Gerihan
Present address: Avrupadent Dental Clinic, İzmir, Turkey
Authors and Affiliations
Department of Pediatric Dentistry, Ege University School of Dentistry, İzmir, Turkey
Hazal Ezgi Gerihan, Dilşah Çoğulu & Özant Önçağ
Department of Medical Genetics, Ege University School of Medicine, İzmir, Turkey
Asude Durmaz
Department of Pediatric Dentistry, Usak University School of Dentistry, Usak, Turkey
Elif Hasibe Kuru
You can also search for this author in PubMed Google Scholar
Contributions
H.E.G. and D.C. conceived the ideas; D.C., H.E.G and E.K collected the data; A.D and Ö.Ö. analyzed the data, and E.K. and D.C. led the writing.
Corresponding author
Correspondence to Dilşah Çoğulu .
Ethics declarations
Ethics approval and consent to participate.
The Ethical Committee of the Medical Faculty of Ege University approved the study (Reference No: 16-7/14). All participants were comprehensively informed of the research objectives, and detailed information about the treatment regimen was provided to both the children and their parents. Written informed consent was obtained, ensuring all individuals’ voluntary and consensual participation. Data will be used exclusively for research purposes, maintaining strict confidentiality and privacy.
Consent for publication
Informed written consent was obtained from the patients to publish the medical history and radiographs.
Permission to reproduce material from other sources
This work is an original contribution, and no material has been reproduced from other sources.
Competing interests
The authors declare no competing interests.
Additional information
Publisher’s note.
Springer Nature remains neutral with regard to jurisdictional claims in published maps and institutional affiliations.
Electronic supplementary material
Below is the link to the electronic supplementary material.
Supplementary Material 1
Rights and permissions.
Open Access This article is licensed under a Creative Commons Attribution-NonCommercial-NoDerivatives 4.0 International License, which permits any non-commercial use, sharing, distribution and reproduction in any medium or format, as long as you give appropriate credit to the original author(s) and the source, provide a link to the Creative Commons licence, and indicate if you modified the licensed material. You do not have permission under this licence to share adapted material derived from this article or parts of it. The images or other third party material in this article are included in the article’s Creative Commons licence, unless indicated otherwise in a credit line to the material. If material is not included in the article’s Creative Commons licence and your intended use is not permitted by statutory regulation or exceeds the permitted use, you will need to obtain permission directly from the copyright holder. To view a copy of this licence, visit http://creativecommons.org/licenses/by-nc-nd/4.0/ .
Reprints and permissions
About this article
Cite this article.
Gerihan, H.E., Çoğulu, D., Önçağ, Ö. et al. Assessment of MMP levels in reversible and irreversible pulpitis and a randomized controlled trial comparing clinical success of two different calcium-silicate cements in pulpotomy treatment of primary molars with an 18-month follow-up. BMC Oral Health 24 , 1020 (2024). https://doi.org/10.1186/s12903-024-04795-5
Download citation
Received : 23 May 2024
Accepted : 22 August 2024
Published : 30 August 2024
DOI : https://doi.org/10.1186/s12903-024-04795-5
Share this article
Anyone you share the following link with will be able to read this content:
Sorry, a shareable link is not currently available for this article.
Provided by the Springer Nature SharedIt content-sharing initiative
- Irreversible pulpitis
- Matrix metalloproteinase
- Reversible pulpitis
BMC Oral Health
ISSN: 1472-6831
- General enquiries: [email protected]

COMMENTS
The null hypothesis (H0) answers "No, there's no effect in the population.". The alternative hypothesis (Ha) answers "Yes, there is an effect in the population.". The null and alternative are always claims about the population. That's because the goal of hypothesis testing is to make inferences about a population based on a sample.
The null and alternative hypotheses are two competing claims that researchers weigh evidence for and against using a statistical test: Null hypothesis (H0): There's no effect in the population. Alternative hypothesis (HA): There's an effect in the population. The effect is usually the effect of the independent variable on the dependent ...
The actual test begins by considering two hypotheses.They are called the null hypothesis and the alternative hypothesis.These hypotheses contain opposing viewpoints. H 0, the —null hypothesis: a statement of no difference between sample means or proportions or no difference between a sample mean or proportion and a population mean or proportion. In other words, the difference equals 0.
The research hypothesis is often called the alternative or experimental hypothesis in experimental research. It typically suggests a potential relationship between two key variables: the independent variable, which the researcher manipulates, and the dependent variable, which is measured based on those changes.
A null hypothesis is what, the researcher tries to disprove whereas an alternative hypothesis is what the researcher wants to prove. A null hypothesis represents, no observed effect whereas an alternative hypothesis reflects, some observed effect. If the null hypothesis is accepted, no changes will be made in the opinions or actions.
Here is a summary of the key differences between the null and the alternative hypothesis test. The null hypothesis represents the status quo; the alternative hypothesis represents an alternative statement about the population. The null and the alternative are mutually exclusive statements, meaning both statements cannot be true at the same time.
Null hypothesis: µ ≥ 70 inches. Alternative hypothesis: µ < 70 inches. A two-tailed hypothesis involves making an "equal to" or "not equal to" statement. For example, suppose we assume the mean height of a male in the U.S. is equal to 70 inches. The null and alternative hypotheses in this case would be: Null hypothesis: µ = 70 inches.
The alternative hypothesis and null hypothesis are types of conjectures used in statistical tests, which are formal methods of reaching conclusions or making judgments on the basis of data. In statistical hypothesis testing, the null hypothesis and alternative hypothesis are two mutually exclusive statements. "The statement being tested in a test of statistical significance is called the null ...
The actual test begins by considering two hypotheses.They are called the null hypothesis and the alternative hypothesis.These hypotheses contain opposing viewpoints. \(H_0\): The null hypothesis: It is a statement of no difference between the variables—they are not related. This can often be considered the status quo and as a result if you cannot accept the null it requires some action.
An alternate hypothesis, on the other hand, is a statement that describes the existence of a relationship between two variables in a study. A null hypothesis is the hypothesis that a researcher is attempting to refute. The alternative hypothesi s, on the other hand, is the hypothesis that the researcher attempts to confirm.
The null and alternative hypothesis. In order to undertake hypothesis testing you need to express your research hypothesis as a null and alternative hypothesis. The null hypothesis and alternative hypothesis are statements regarding the differences or effects that occur in the population. ... If there really is no difference between the two ...
Most technical papers rely on just the first formulation, even though you may see some of the others in a statistics textbook. Null hypothesis: " x is equal to y.". Alternative hypothesis " x is not equal to y.". Null hypothesis: " x is at least y.". Alternative hypothesis " x is less than y.". Null hypothesis: " x is at most ...
Converting research questions to hypothesis is a simple task. Take the questions and make it a positive statement that says a relationship exists (correlation studies) or a difference exists between the groups (experiment study) and you have the alternative hypothesis.
The null hypothesis is a general statement that states that there is no relationship between two phenomenons under consideration or that there is no association between two groups. An alternative hypothesis is a statement that describes that there is a relationship between two selected variables in a study. Symbol. It is denoted by H 0.
The null hypothesis is generally denoted as H0. It states the exact opposite of what an investigator or an experimenter predicts or expects. It basically defines the statement which states that there is no exact or actual relationship between the variables. The alternative hypothesis is generally denoted as H1.
This is opposed by the alternative hypothesis, also known as the research hypothesis, defined as the prediction that there is a measurable interaction between variables. The symbol for the ...
The alternative hypothesis is the complement to the null hypothesis. The null hypothesis states that there is no effect or no relationship between variables, while the alternative hypothesis claims that there is an effect or relationship in the population. It is the claim that you expect or hope will be true.
14. The rule for the proper formulation of a hypothesis test is that the alternative or research hypothesis is the statement that, if true, is strongly supported by the evidence furnished by the data. The null hypothesis is generally the complement of the alternative hypothesis. Frequently, it is (or contains) the assumption that you are making ...
Yes, in this case, the alternative hypothesis would be that the two drugs, X and Y, are equivalent in terms of effectiveness and side effects. The null hypothesis would be that there is a significant difference between the two drugs in terms of effectiveness or side effects. The scientist's aim would be to gather evidence that supports the ...
Here are some examples of the alternative hypothesis: Example 1. A researcher assumes that a bridge's bearing capacity is over 10 tons, the researcher will then develop an hypothesis to support this study. The hypothesis will be: For the null hypothesis H0: µ= 10 tons. For the alternate hypothesis Ha: µ>10 tons.
The research hypothesis usually includes an explanation (" x affects y because …"). A statistical hypothesis, on the other hand, is a mathematical statement about a population parameter. Statistical hypotheses always come in pairs: the null and alternative hypotheses. In a well-designed study, the statistical hypotheses correspond ...
1. In the null hypothesis, there is no relationship between the two variables. In the alternative hypothesis, there is some relationship between the two variables i.e. They are dependent upon each other. 2. Generally, researchers and scientists try to reject or disprove the null hypothesis.
They are called the null hypothesis and the alternative hypothesis. These hypotheses contain opposing viewpoints. \(H_0\): The null hypothesis: It is a statement of no difference between the variables—they are not related. This can often be considered the status quo and as a result if you cannot accept the null it requires some action.
Background Matrix metalloproteinases (MMPs) are critical enzymes involved in the remodeling and defense mechanisms of dental pulp tissue. While their role in permanent teeth has been extensively studied, research focusing on MMPs in primary teeth remains limited. This gap highlights the need for further investigations to understand the specific contributions of MMPs to pulpal defense in ...