- Search Menu
- Sign in through your institution
- Editor's Choice
- Author Guidelines
- Submission Site
- Open Access
- About Journal of Cybersecurity
- Editorial Board
- Advertising and Corporate Services
- Journals Career Network
- Self-Archiving Policy
- Journals on Oxford Academic
- Books on Oxford Academic


Article Contents
Exposure to cyberattacks and policy attitudes, the mediating role of threat perceptions, experimental method.
- < Previous
Cyberattacks, cyber threats, and attitudes toward cybersecurity policies

- Article contents
- Figures & tables
- Supplementary Data
Keren L G Snider, Ryan Shandler, Shay Zandani, Daphna Canetti, Cyberattacks, cyber threats, and attitudes toward cybersecurity policies, Journal of Cybersecurity , Volume 7, Issue 1, 2021, tyab019, https://doi.org/10.1093/cybsec/tyab019
- Permissions Icon Permissions
Does exposure to cyberattacks influence public support for intrusive cybersecurity policies? How do perceptions of cyber threats mediate this relationship? While past research has demonstrated how exposure to cyberattacks affects political attitudes, the mediating role played by threat perception has been overlooked. This study employs a controlled randomized survey experiment design to test the effect of exposure to lethal and nonlethal cyberattacks on support for different types of cybersecurity policies. One thousand twenty-two Israeli participants are exposed to scripted and simulated television reports of lethal or nonlethal cyberattacks against national infrastructure. Findings suggest that exposure to cyberattacks leads to greater support for stringent cybersecurity regulations, through a mechanism of threat perception. Results also indicate that different types of exposure relate to heightened support for different types of regulatory policies. People exposed to lethal cyberattacks tend to support cybersecurity policies that compel the government to alert citizens about cyberattacks. People who were exposed to nonlethal attacks, on the other hand, tend to support oversight policies at higher levels. More broadly, our research suggests that peoples’ willingness to accept government cybersecurity policies that limit personal civil liberties and privacy depends on the type of cyberattacks to which they were exposed and the perceptions associated with such exposure.
In recent years, the increase in civilian exposure to cyberattacks has been accompanied by heightened demands for governments to introduce comprehensive cybersecurity policies. These demands peaked in the aftermath of the 2021 Colonial Pipeline and SolarWinds cyberattacks, where the US government's lack of access to cybersecurity information in critical industries wrought havoc on the country's national and economic security. In the aftermath of these attacks, lawmakers and the public exhibited newfound enthusiasm for legislation that would mandate cyberattack reporting by private enterprises—accelerating a regulatory trend that has existed for several years [ 1 ]. In 2020, for example, 40 US states and territories introduced more than 280 cybersecurity related bills and resolutions [ 2 , 3 ]. A similar process has taken place in Europe [ 4 ] and in Israel [ 5 , 6 ].
The public willingness to accept government policies and regulations that limit personal civil liberties and privacy is part of a delicate tradeoff between security and privacy. In some ways, privacy is seen as an adequate cost of enhanced personal and societal security in the face of novel threats. However, the public has grown increasingly sensitive to the importance of online privacy, and is keenly aware of the ethical, political, legal, and rights-based dilemmas that revolve around government monitoring of online activity and communications [ 7 , 8 ].
The debate on digital surveillance centers on how and whether authorities should gain access to encrypted materials, and raise key questions concerning the extent of state interference in civic life, and the protection of civil rights in the context of security. Yet what lies at the heart of this willingness to accept government policies and regulations that limit personal civil liberties and privacy via increasing public demand for government intervention in cybersecurity? Does exposure to different types of cyberattacks lead to heightened support for different types of regulatory policies? And does the public differentiate between interventionist and regulatory forms of cybersecurity policies?
To test these questions, we ran a controlled randomized survey experiment that exposed 1022 Israeli participants to simulated video news reports of lethal and nonlethal cyberattacks. We argue that public support for governmental cybersecurity measures rises as a result of exposure to different forms of cyberattacks, and that perceived threat plays a mediating role in this relationship. More specifically, we propose that exposure to initial media reports about cyberattacks is a key to the exposure effect, since at this time the threat is magnified and the public has minimal information about the identity of the attacker and the type of cyberattack that was conducted. Past events show that in many cases, the public internalizes the details of an attack in its immediate aftermath when media reports are heaviest. While later reports in the days and weeks following an attack will include far more detailed information, the damage by this time has already been done and the public is already scared and alert.
Further to this, we suggest that the literature has erroneously pooled together all cyber regulatory policies under a single banner of cybersecurity. We propose that civilian exposure to different types of cyberattacks leads to increased support for different and specific cybersecurity policies. We therefore differentiate between support for policies that focus on alerting the public in cases of cyberattacks and others that call for oversight of cybersecurity. In examining how exposure to cyberattacks influences support for these specific policy positions, we distinguish between the outcome of cyberattacks—lethal attacks that cause lethal consequences as a first- or second-degree outcome of the attack, versus nonlethal attacks that merely involve financial consequences. This more nuanced breakdown of exposure types and policy options can help officials contend with certain policy debates without the need for a one-size-fits-all policy. For example, reservations expressed by conservative/libertarian scholars who are concerned about government intervention in the commercial marketplace need not disqualify all forms of cybersecurity policy [ 9 ]. Likewise, the reservations of those concerned with individual privacy violations need not lead to the denunciation of all policies [ 10 ].
To ground this analysis of how the public responds following exposure to both lethal and nonlethal cyberattacks, we apply theories associated with the literature on terrorism and political violence. These theories offer sophisticated mechanisms that explain how individual exposure to violence translates into political outcomes—including demands for government intervention and policymaking. This approach is especially applicable in the digital realm as cyberattacks track a middle ground between technological breakthroughs that constitute tactical developments and new strategic weapons [ 11 ]. The consequence of such ambiguity is that civilians who are exposed to digital political threats can only identify the outcomes of the attack—i.e. whether it is a lethal or nonlethal cyberattack—while the motivations and identities of attackers often remain veiled, or at least unsettled. In light of these attributional challenges, and reflecting the fact that the public typically operates in a low-information environment, we refrain from declaring that the cyberattacks that appear in our experimental manipulations are cybercrime, cyberterrorism, cyber-vandalism, or any other type of attack. Rather, we refer to all attacks under the general heading of "cyberattacks," leaving all respondents to react to the attacks in a way that they see as appropriate in light of the severity of the reported outcome.
The most common form of cyberattack is cybercrime. Reports of data breaches resulting from cyberattacks by criminal organizations show a growth of more than threefold between 2011 and 2018 [ 12 ]. In the first half of 2019 alone, the United States Treasury Department announced that there had been 3494 successful cyberattacks against financial institutions resulting in colossal financial losses and the capture of personal information relating to hundreds of millions of people [ 13 ]. Cyberattacks executed by terror organizations are a newer phenomenon, albeit one that has captured the popular imagination. While terror organizations predominantly make use of cyberspace for fundraising, propaganda, and recruitment [ 14 , 15 ], a recent development has been the next-generation capacity of cyber strikes to trigger lethal consequences, be it through first- or second-order effects. 1 We acknowledge that scholars have expressed some skepticism about the likelihood of impending destructive cyberterror incidents [ 16–18 ], yet national security officials have regularly predicted that lethal cyberattacks pose a "critical threat" [ 19 ]. In the last decade, the nature of this threat has evolved from the earlier depictions of an apocalyptic cyber "pearl harbor" that would ravage modern society from the shadows [ 20 ], to a more nuanced understanding that cyberattacks, while still posing a threat to critical infrastructure, are more likely to manifest through targeted strikes. For example, in April 2020, Israel narrowly averted a cyberattack targeting civilian water networks that would have killed scores of civilians by adding chlorine to the water supply [ 19 ]. Other physically destructive cyberattacks have caused explosive damage to critical infrastructure [ 21 ], while researchers have experimentally verified the ability of malicious digital actors to hack pacemakers and insulin pumps [ 22 ]. While the lethal stature of cyberattacks is still developing, these incidents establish the bona fides of this impending threat and the importance of understanding how the public responds to this type of event.
The discussion that follows has four parts. We begin by examining the theory of how exposure to violence translates into policy preferences, with a particular focus on the mediating role of threat perception. Second, we discuss the design of our controlled, randomized experiment that exposes participants to television news reports of lethal and nonlethal cyberattacks. Third, we present our main results and consider various mediation models that pertain to the different regulatory subsets. We conclude by discussing the implications of our findings for the study of cybersecurity and cyber threats more generally.
Civilians who are exposed to political violence often suffer from feelings of trauma, anxiety, and helplessness in the face of threatening external forces [ 23–25 ]. These emotional responses—whether caused by acts of cyber or conventional violence—are known to cause shifts in political attitudes. Research has shown how exposure to conventional terrorism, which targets civilians and disrupts their daily routines, has an impact on individuals’ support for attitudes toward peace and compromise with the other [ 26 ], political conservatism [ 27 ], exclusionism [ 28 ] and intragroup relations [ 29 ].
Despite the sizeable literature dealing with the effects of exposure to violence, few studies directly investigate the effects of exposure to destructive cyberattacks. This is despite the growing recognition that these threats have become a very tangible part of modern life. In a complex scenario described in the Tallinn Manual 2.0 on the International Law Applicable to Cyber Warfare, the authors contemplated how new forms of cyberattacks could be used to “acquire the credentials necessary to access the industrial control system of a nuclear power plant… with the intent of threatening to conduct cyber operations against the system in a manner that will cause significant damage or death…” [ 30 ]. Even more recently, reports have acknowledged how cyberterror attacks could immobilize a country's or region's electrical infrastructure [ 31 ], disable military defense systems [ 32 ], and even imperil nuclear stability [ 33 ]. While there is a difference between capability and intent, and we acknowledge that physically destructive cyber threats have remained scarce until now, understanding how civilians respond to such digital cyberattacks will become particularly important as the threat matures.
Studies that directly investigated exposure to digital political violence found that exposure had significant effects on political behavior and attitudes, akin to exposure to conventional political violence [ 34 , 35 ]. In a series of exploratory studies regarding the phenomena of cyberterrorism, Gross et al . [ 34 , 36 ] sought to empirically measure the effects of exposure to cyberterrorism under controlled experimental conditions. Their key finding was that exposure to cyberterrorism was severe enough to generate significant negative emotions and cognitive reactions (threat perceptions) at equivalent levels to those of conventional terror acts. Canetti et al . [ 37 ] found that victims of cyberattacks react by demanding government protection, with psychological distress explaining the relationship between exposure and the demand for government intervention. In a subsequent biologically focused experiment, Canetti et al . measured cortisol levels to show how participants who are exposed to cyberterror attacks and experience higher levels of stress are more likely to support hardline retaliatory policies [ 38 ].
Building on this foundation, other research has sought to refine a more precise psycho-political mechanism that understands how cyberattacks trigger shifts in political attitudes. Research by Shandler et al . [ 39 , 40 ], e.g. found that only lethal cyberattacks cause political consequences akin to conventional political violence, and that only the emotion of anger explained these shifts.
In the current paper, we aim to add to this emerging body of research by examining the topic of cybersecurity preferences in the aftermath of lethal and nonlethal cyberattacks. While one past study by Cheung-Blunden et al . [ 41 ] examined how emotional responses to cyber incidents sway cybersecurity preferences, no research has yet attempted to analyze how different types of cyberattacks affect different kinds of cybersecurity policies. As such, we add much needed nuance to the literature.
For the purpose of considering the effects of exposure to cyberattacks, this research focuses on the "outcome" of a cyberattack rather than the "identity" of the perpetrator or the "classification" of the attack. This is necessary for several reasons that relate to the specific characteristics of cyberspace. First, as introduced above, a new class of cyberattack exemplified by the ransomware epidemic has exhibited characteristics of both cybercrime and cyberterror operations, impeding the classification of cyber incidents into simple categories. Second, attribution in cyberspace is fraught with difficulty, and an age of manipulated information complicates the determination of provenance [ 42–44 ]. Sophisticated cyber operatives working from anywhere in the world can exploit the principle of anonymity that underlies the Internet infrastructure to hide their identity. Though authorities would be able to quickly identify the identity of an attacker behind any major cyberattack [ 42 ], this is essentially impossible for members of the public who are confronted with both structural and technical obstacles that prevent them from rendering an objective judgement about the attack source. This reality of publicly obscured cyber antagonists can be viewed in the timelines of several famous cyber incidents. It took between six months and three years for authorities and private actors to publicly reveal the actors behind the 2017 WannaCry attacks, the 2016 cyber intrusion into the Democratic National Committee's networks, and the 2016 cyberattack against the Bowman Dam in New York [ 45–47 ]. While each of these incidents were eventually attributed to an attack source, and the authorities may well have known the identity of the attacker from an early date, we can see that from the perspective of the public, there was a time lag of several months or years before a name was attached to any attack. Third, state involvement in cyberattacks—either as a direct attacker or via proxies—can add substantial background noise to the perception of an attack, raising the specter of interstate war. There is an interesting debate in the literature about whether states may be deemed capable of conducting cyberterrorism—or whether this is a label that can only be applied to nonstate actors. While the literature is still unsettled on this point, Macdonald, Jarvis and Nouri [ 48 ] found considerable expert support for the proposition that states can engage in cyberterrorism.
It is for these reasons that we choose to follow the lead of the scholars who are beginning to evaluate responses to cyber threats through the prism that is most readily available for the public—specifically, the outcome variable, or in other words, the lethality of the attack [ 33 ]. This focus on outcome rather than attacker is necessary in order to understand the factors that prompt emotional and political responses in the public. While these information asymmetries explain our focus on the outcome of the attack rather than the identity of the attacker, we acknowledge that the people draw inferences about the identity and motivations of attackers based on prior experiences and political orientation [ 49 ]. Liberman and Skitka's vicarious retribution theory [ 50 , 51 ] demonstrates how the public may impute responsibility to unrelated or symbolically related offenders when the identity of an attacker is unclear. Nonetheless, maintaining the highest standards of ecological validity demands that attribution and attack categorization is absent in initial public reports of cyber incidents.
Under this framework, we hypothesize that:
Hypothesis 1: Exposure to (i) lethal or (ii) nonlethal cyberattacks will lead to greater support for adopting cybersecurity policies compared with people who were not exposed to any cyberattack. In other words, exposure to cyberattacks—lethal (LC) or nonlethal (NLC)—will increase support for adopting cybersecurity policies, as compared with a control group.
Hypothesis 2: People who are exposed to lethal cyberattacks (LC) will exhibit to higher support for adopting cybersecurity policies than people who are exposed to nonlethal cyberattacks (NLC).
Civilians are notoriously weak at accurately assessing security threats—a fact that is amplified in the cyber realm due to low cybersecurity knowledge, general cognitive biases in calculating risk, and the distortion of cyber risks by the media, which focuses predominantly on spectacular yet low-likelihood attacks [ 52–54 ]. Perceived risk is partly reliant on the scope of the attack to which people are exposed. Victims of cybercrimes (identity theft and cyber bullying) report moderate or severe emotional distress such as anger, fear, anxiety, mistrust, and loss of confidence [ 55 ]. The effects of conventional terrorism include post-traumatic stress, depression, and anticipatory anxiety [ 56 , 29 ]. In both of these cases, threat perception is a common predictor of political attitudes and behavior. Indeed, the best predictor of hostile out-group attitudes is the perceived threat that out-group members will harm members of the in-group, whether physically, economically or symbolically [ 28 , 57 , 58 ]. In many of the studies cited above, threat perception was found to mediate the relationship between exposure to violence and support for harsh or restrictive policies, especially in conflict-related contexts [ 27 ]. Extending this empirical and theoretical evidence to digital political violence suggests that individuals are likely to respond similarly to cyber threats by supporting strong cybersecurity policies through the interceding influence of heightened threat perception.
A set of early studies compared the level of threat evoked by exposure to different forms of cyber threats, identifying key differences in the how cybercrime and cyberterrorism influenced attitudes toward government policy [ 34 , 36 ]. These studies concluded that direct exposure to cyberterrorism had no effect on support for hardline cybersecurity policies (increased digital surveillance, the introduction of intrusive new regulations), but threat perceptions relating to cyberterrorism successfully predicted support for these policies. Recognizing therefore that threat perception plays a central role in understanding the response to cyberattacks, we predict that
Hypothesis 3: Cyber threat perception will mediate the relationship between individual exposure to cyberattacks and support for cybersecurity policies.
To test our hypotheses, we conducted a controlled survey experiment that exposed respondents to simulated news reports about major cyberattacks. The experimental manipulation relied on professionally produced original video clips that broadcast feature news reports. The lethal treatment group viewed a feature report discussing several lethal cyberattacks that had taken place against Israeli targets, while the nonlethal treatment group broadcast a collection of stories pertaining to nonlethal cyber incidents (see below for additional details about each manipulation). The control group did not watch any news report.
We utilized the medium of video news reports for our experimental manipulation since experiments in recent years have shown how broadcast videos and media reports of major attacks arouse strong emotions among viewers, which in turn trigger reevaluations of policy positions and political attitudes related to issues of security [ 35 , 59 , 60 ]. The rationale behind these finding can be partly explained by Terror Management Theory, which explains how even indirect exposure to violent acts triggers potent emotional reactions as people confront threats to their mortality [ 61 , 62 ]. Just as importantly, news reports are a key avenue by which the public learns about major security incidents, and so this method maintains its ecological validity. Each of the groups completed a pre- and post-survey, answering a series of questions about their attitudes to cybersecurity along with relevant sociodemographic information.
Each of the television news reports was presented as an authentic feature story that appeared on Israeli channel 1 television station. The news reports described the global scale of cyber threats facing the public (i.e. two million malicious web sites launch each month and 60 000 new malware programs appear every day at an annual cost to the global economy of 500 billion dollars). The clips were screened in a feature format using on-camera interviews, voiceover and film footage to describe various cyberattacks. To increase the authenticity of the experience, the reports included interviews with well-known Israeli security experts. To mimic the challenges of cyber attribution, the perpetrators of the attacks described in the videos were not identified and were neutrally referred to as cyber operatives. Each video lasted approximately 3 min.
Lethal Cyber Condition—The television news report described various cyberattacks with lethal consequences that had targeted Israel during the previous years. For example, in one of the featured stories, an attack was revealed to have targeted the servers controlling Israel's electric power grid, cutting off electricity to a hospital and causing deaths. In another story, cyber operatives were said to have attacked a military navigation system, altering the course of a missile so that it killed three Israeli soldiers. A third story concerned the use of malware to infect the pacemaker of the Israeli Defense Minister, and a fourth involved the failure of an emergency call to 10 000 military reserve soldiers due to a cyberattack in which foreign agents changed the last digit of the soldiers’ telephone numbers in the military database. The video's interviews with well-known figures from Israel's security sector emphasized the life-threatening danger posed by cyberattacks.
Nonlethal Cyber Condition—The television news report revealed various nonlethal cyberattacks that had targeted Israel during recent years. For example, the broadcast explained how mobile phone users are made vulnerable to attackers by installing new games and applications, potentially introducing malware that can later access data like personal messages or financial details. Another example concerned the dangers posed by the Internet of Things and featured a story in which all the major credit cards companies suspended their customer support after hundreds of thousands of citizens were fraudulently charged for food purchases by their smart refrigerators. The Israeli experts in this video emphasized the potential financial damage from cyberattacks.
Participants
The online survey experiment was administered in Israel during September 2015 via the Midgam Survey Panel. One thousand twenty-two participants were randomly assigned to the three groups (lethal condition: N = 387; nonlethal condition: N = 374; control group: N = 361). The experimental sample represents a random cross-section of the Jewish Israeli population. The sample is largely representative of the wider population, and balance checks reveal that the treatment distribution is acceptable. We note that due to data collection constraints, the sample does not include ultra-orthodox (religious) respondents due to difficulties in accessing this subgroup through online methods. The mean age of the participants was 41 (SD = 14.81), and gender distribution of 49.96% male and 50.04% female. With respect to political orientation, 44.35% of the sample define themself as right-wing ( N = 452), 38.28% themselves as centrist ( N = 390), and 17.37% as left-wing ( N = 177) (this reflects the right-wing slant of the Israeli population that has been apparent in recent elections). The distribution of education and income levels was similar across the three groups (Education: F(2, 1120) = 0.20, P < 0.82; Income: F(2, 1045) = 0.63, P < 0.53). Sociodemographic characteristics of the participants are presented in Appendix A (Supporting Information), together with experimental balance checks.
The experiment incorporated three primary variables: the predictor variable (exposure to cyberattacks), the dependent variable (support for cybersecurity policies), and the mediator variable (threat perception). Sociodemographic measures were also collected.
Predictor variable—exposure to cyberattacks
Exposure to cyberattacks was operationalized by random assignment to one of the three experimental treatments described above—lethal cyberattacks/nonlethal cyberattacks/control condition.
Dependent variable: support for cybersecurity policies
Support for cybersecurity policies was examined using twelve questions taken from two scales developed by McCallister and Graves [ 63 , 64 ]. After separating out one item that reflected a unique form of cybersecurity policy, the remaining items were subjected to a principal component analysis (PCA), which highlighted different aspects of cybersecurity policy. Our criteria for the factor dimension extraction was an eigenvalue greater than one for number of dimensions, and factor loading greater than 0.35, for dimension assignment. We applied the PCA extraction method with the Varimax rotation to construct orthogonal factors [ 65 ]. This procedure gave rise to two clearly distinguishable cyber policy dimensions. Following this process, we combined the two remaining items that were excluded due to poor loadings (loading < 0.35) to create a third policy dimension with a high correlation between the items ( r = 0.617, P < 0.001) (see Appendix B in the Supporting Information for the PCA and complete list of the items used to construct each scale). The final three measures of cybersecurity policies reflected the breadth of available policy options, which emphasized different levels of government intervention and oversight strategies. The first of these is cybersecurity prevention policy (CPP); the second is cybersecurity alert policy (CAP); and the third is cybersecurity oversight policy (COP).
The cybersecurity prevention policy dimension (CPP) captures the idea that the state should mandate commercial companies to implement minimum levels of cybersecurity to prevent damage. Respondents were asked questions such as: “should the state compel business owners to protect themselves against cyberattacks?” Cronbach's α was within an acceptable range at 0.720.
The cybersecurity oversight policy dimension (COP) refers to the notion that the state should directly intervene to offer cyber protection to its citizens and businesses. Relevant questions for this dimension included “should the state protect its citizens from cyberattacks?” Cronbach's α was within an acceptable range at 0.737.
The cybersecurity alert policy dimension (CAP) relates to the state's presumed responsibility to ensure citizens are alerted when a hack of a cyberattack is discovered. For example, a related question would ask: “should the state alert citizens after a successful attack on critical infrastructure?” As opposed to the prevention policy dimension that relates to measures that must be taken before a cyberattack, the alert policy focuses on the measures to be taken after an attack. Cronbach's α was slightly below acceptable range at 0.632. All questions were measured on a scale ranging from 1 (“completely disagree”) to 6 (“completely agree”).
Mediator: perceptions of cybersecurity threats
Threat perception pertaining to cyber threats was gauged using a five-item scale based on studies conducted in the United States [ 66 ]. Respondents were asked how concerned they feel about the possibility of an actual threat to their security. Respondents answered questions including: “To what extent does the idea of a cyberattack on Israel affect your sense of personal security?” and “To what extent does a cyberattack on Israel threaten the country's critical infrastructure?,” and the answers ranged from 1 (“not at all”) to 6 (“to a very great degree”). The internal consistency of this measure was very high (Alpha = 0.913).
Control variables
Control variables collected included political ideology (assessed through a self-reported five-point scale ranging from 1 [very conservative] to 5 [very liberal]), age, gender, marital status, religiosity, education, and income.
We also measured and controlled for participants’ past exposure to cyberattacks. To measure this variable, we adapted a four-item scale used to measure exposure to terrorism and political violence [ 67 , 35 ]. Items included questions that asked the extent to which the respondents, their friends and their family had ever suffered harm or loss from a cyberattack. Similarly to past studies, we did not calculate the internal reliability for past exposure, given that one type of exposure does not necessarily portend another type.
Preliminary analyses
We begin our analysis by testing the variance between the treatment groups regarding attitudes toward cybersecurity policies, to establish that the experimental conditions produce at least minimal levels of differences in the dependent variables. Hence, we conducted a one-way univariate analysis of variance (ANOVA), in which the different cyber policies were the dependent variables. The results indicated differences between the three groups in support for policies regarding cybersecurity alerts (CAP: F(2, 1020) = 4.61, P < 0.010). No differences between groups were found in support for cybersecurity prevention policy or cybersecurity oversight policy (CPP: F(2, 1020) = 1.35, P < 0.259; COP: F(2, 1020) = 0.94, P < 0.39). We followed the CAP ANOVA analysis with pairwise comparisons using Bonferroni corrections, which revealed that the highest level of support for cybersecurity alerts was expressed by the group exposed to lethal cyberattacks on average, while the other two groups showed lower levels of support for this policy. These results support the conclusion that the differences in cybersecurity policy preferences between the three groups derive from the video stimulus, and not from differences in participants’ sociodemographic characteristics (see Appendix C in the Supporting Information for means and standard deviations of study variables, in all three manipulation groups).
In addition, we tested group differences regarding threat perceptions and found significant differences in threat perceptions between the three groups (F(2, 1020) = 21.68, P < 0.001). The follow up pairwise comparisons with Bonferroni corrections, revealed that participants in both experimental groups (LC and NLC) expressed higher levels of threat perceptions in comparison to participants in the control group. These analyses provide sufficient preliminary support to conduct more complex analyses that integrate multiple effects in this triangle of exposure to cyberattacks, cyber threat perception, and support for cybersecurity policies.
Mediation analysis
To test hypothesis 3, we ran a path analysis model, i.e. a structural equation modeling with observed indicators only. In this model, the exposure was divided into lethal vs control and nonlethal vs control. More specifically, with regard to the mediation effect, the model structure included two pathways from the experimental conditions to support for cybersecurity policies: From the lethal vs control, and from nonlethal vs control through threat perceptions. The latter variable was expected to mediate the effect condition effects on cyber policy positions as proposed in the theory section.
In order to further investigate the mediation mechanism, we constructed an integrative path analysis model [ 53 ]. Running this model enables us to identify direct and indirect effects among all the study variables. We provide modeling results in the following Table 1 and an illustration of the path analysis model in Fig. 1 .

Empirical model results—direct effects of exposure to lethal and nonlethal attack groups vs control group. * P < 0.05, ** P < 0.01, *** P < 0.001.
Path: analysis direct effects, standardized estimates
. | Threat (M) . | CAP (Y ) . | COP (Y ) . | CPP (Y ) . |
---|---|---|---|---|
. | Beta (S.E.) [95% CI] . | Beta (S.E.) [95% CI] . | Beta (S.E.) [95% CI] . | Beta (S.E.) [95% CI] . |
0.058***(0.035)[0.088, 0.262] | 0.249***(0.030) [0.151, 0.275] | 0.273*** (0.032)[0.193, 0.335] | ||
) | ||||
0.163***(0.034)[0.077, 0.221] | –0.070*(0.036)[–0.164, –0.012] | –0.073*(0.036)[–0.168, –0.017] | –0.043(0.037)[0.030, 0.077] | |
) | ||||
0.207***(0.033)[0.123, 0.258] | –0.140***(0.035)[–0.230, –0.080] | –0.024(0.034)[–0.105, 0.035] | –0.015(0.035)[–0.101, 0.046] | |
0.109***(0.031)[0.028, 0.164] | –0.012(0.030)[–0.088, 0.036] | –0.005(0.030)[–0.083, 0.035] | 0.016(0.030)[–0.063, 0.064] | |
0.200***(0.030)[0.125, 0.254] | 0.072*(0.032)[–0.011, 0.126] | 0.010(0.031)[–0.070, 0.060] | 0.050(0.031)[–0.030, 0.095] | |
0.034 (0.031)[–0.051, 0.085] | –0.044(0.036)[–0.137, 0.013] | 0.088**(0.031)[0.004, 0.137] | 0.015(0.033)[–0.075, 0.066] | |
–0.049 (0.032)[–0.131, 0.097] | –0.028(032)[–0.124, –0.028] | –0.027(0.031)[–0.104, 0.022] | –0.033(0.033)[–0.117, 0.017] | |
0.035 (0.033)[–0.045, 0.097] | 0.050(0.032)[–0.025, 0.106] | 0.027(0.033)[–0.063, 0.082] | –0.040(0.033)[–0.127, 0.024] | |
0.093***(0.017) | 0.047***(0.014) | 0.074***(0.015) | 0.087***(0.018) |
. | Threat (M) . | CAP (Y ) . | COP (Y ) . | CPP (Y ) . |
---|---|---|---|---|
. | Beta (S.E.) [95% CI] . | Beta (S.E.) [95% CI] . | Beta (S.E.) [95% CI] . | Beta (S.E.) [95% CI] . |
0.058***(0.035)[0.088, 0.262] | 0.249***(0.030) [0.151, 0.275] | 0.273*** (0.032)[0.193, 0.335] | ||
) | ||||
0.163***(0.034)[0.077, 0.221] | –0.070*(0.036)[–0.164, –0.012] | –0.073*(0.036)[–0.168, –0.017] | –0.043(0.037)[0.030, 0.077] | |
) | ||||
0.207***(0.033)[0.123, 0.258] | –0.140***(0.035)[–0.230, –0.080] | –0.024(0.034)[–0.105, 0.035] | –0.015(0.035)[–0.101, 0.046] | |
0.109***(0.031)[0.028, 0.164] | –0.012(0.030)[–0.088, 0.036] | –0.005(0.030)[–0.083, 0.035] | 0.016(0.030)[–0.063, 0.064] | |
0.200***(0.030)[0.125, 0.254] | 0.072*(0.032)[–0.011, 0.126] | 0.010(0.031)[–0.070, 0.060] | 0.050(0.031)[–0.030, 0.095] | |
0.034 (0.031)[–0.051, 0.085] | –0.044(0.036)[–0.137, 0.013] | 0.088**(0.031)[0.004, 0.137] | 0.015(0.033)[–0.075, 0.066] | |
–0.049 (0.032)[–0.131, 0.097] | –0.028(032)[–0.124, –0.028] | –0.027(0.031)[–0.104, 0.022] | –0.033(0.033)[–0.117, 0.017] | |
0.035 (0.033)[–0.045, 0.097] | 0.050(0.032)[–0.025, 0.106] | 0.027(0.033)[–0.063, 0.082] | –0.040(0.033)[–0.127, 0.024] | |
0.093***(0.017) | 0.047***(0.014) | 0.074***(0.015) | 0.087***(0.018) |
Standard error in parentheses; * P < 0.05, ** P < 0.01, *** P < 0.001. NLC = non-ethal cyberattack; LC = lethal cyberattack.
Direct effects
Table 1 presents the results of the standardized estimates (beta coefficients) of each experimental group vis-à-vis the control group (i.e. NLC vs control, and LC vs control), perceptions of threat, past exposure to cyberattacks and socio demographic variables—gender, religiosity, education and political ideology—with the three dimensions of cybersecurity policies as the dependent variables. In the pairwise comparison of the experimental groups, which compares the lethal and nonlethal conditions to the control group, we find a larger direct effect in the LC (lethal) group compared with the NLC (nonlethal) group in predicting support for CAP.
A follow-up that compared the two regression weights further confirmed the stronger relative effect of the lethal exposure over the nonlethal exposure (H 2 : NLC-LC = −0.21 (0.10), P = 0.047). This demonstrates support for our second hypothesis. People who were exposed to lethal cyberattacks tended to support cybersecurity policies that compel the government and security forces to alert citizens if they have evidence of citizens’ computers being hacked or if an act cyberattack is discovered (CAP) at higher levels than people who were exposed to nonlethal/economic cyberattacks compared with people in the control group.
Interestingly, this trend was reversed for the oversight policies (COP) form of cybersecurity regulation. Here, we identified a significant direct effect wherein exposure to nonlethal cyberattacks led to support for oversight policies (COP) at higher levels than respondents who were exposed to the lethal cyberattacks manipulation or the control group. However, the difference between the two treatment conditions was not significant (NLC-LC = 0.11(0.08), P = 0.16). This indicates that exposure to any kind of cyberattack, lethal or nonlethal, predicts greater support for oversight regulation policies (COP) to the same extent. No direct effect was found between exposure to cyberattacks and support for prevention regulation policies (CPP). By breaking apart this analysis into different dimensions of cybersecurity polices our results reveal how exposure to different forms of cyberattacks contribute to support for distinct types of policy that emphasize oversight or intervention.
Most importantly, results indicate a significant direct effect of threat perceptions on all three dimensions of cybersecurity policy and higher levels of threat perception in the lethal cyber manipulation group compared with the nonlethal cyber manipulation group and the control group.
Mediating effects
Table 2 presents the indirect effects of each of the two treatment conditions in comparison to the control group for the three dimensions of cybersecurity policies—with threat perception as a mediator. The indirect effects are pathways from the independent variable to the policy variables through threat perceptions. In the path analysis model, each dependent variable, i.e. support for particular cybersecurity policies, could have two potential paths, one from the nonlethal condition and the one from the lethal condition. Altogether, six mediation pathways were tested. These indirect outcomes are illustrated in Fig. 1 . In the LC group we see a complete mediation effect of threat perceptions and no significant direct effect of exposure on COP support. This means that for those participants who were exposed to the lethal condition, the actual exposure was not as strong a predictor of policy support as the threat perception associated with the attacks.
Path: analysis mediation effects, standardized estimates
. | Independent . | Mediation . | Outcome . | Indirect effect (coefficient; S.E. [95% CI]) . |
---|---|---|---|---|
Mediation 1 | NLC/Control (X ) | Threat | CAP | 0.026***; 0.008 [0.010, 0.042] |
Mediation 2 | LC/Control (X ) | Threat | CAP | 0.033***; 0.009 [0.015, 0.050] |
Mediation 3 | NLC/Control (X ) | Threat | COP | 0.041***; 0.010 [0.020, 0.060] |
Mediation 4 | LC/Control (X ) | Threat | COP | 0.052***; 0.011 [0.028, 0.071] |
Mediation 5 | NLC/Control (X ) | Threat | CPP | 0.045***; 0.011 [0.021. 0.066] |
Mediation 6 | LC/Control (X ) | Threat | CPP | 0.056***; 0.011 [0.030, 0.077] |
. | Independent . | Mediation . | Outcome . | Indirect effect (coefficient; S.E. [95% CI]) . |
---|---|---|---|---|
Mediation 1 | NLC/Control (X ) | Threat | CAP | 0.026***; 0.008 [0.010, 0.042] |
Mediation 2 | LC/Control (X ) | Threat | CAP | 0.033***; 0.009 [0.015, 0.050] |
Mediation 3 | NLC/Control (X ) | Threat | COP | 0.041***; 0.010 [0.020, 0.060] |
Mediation 4 | LC/Control (X ) | Threat | COP | 0.052***; 0.011 [0.028, 0.071] |
Mediation 5 | NLC/Control (X ) | Threat | CPP | 0.045***; 0.011 [0.021. 0.066] |
Mediation 6 | LC/Control (X ) | Threat | CPP | 0.056***; 0.011 [0.030, 0.077] |
Standard error in parentheses; * P < 0.05, ** P < 0.01, *** P < 0.001. In squared brackets 95% confidence interval with bias correction bootstrapping ( n = 2000).
In our models predicting CAP, we see a partial mediation effect for both treatment groups, in addition to the direct effect that we described above. We see a larger indirect effect in the LC group than in the NLC group and this was confirmed by a test of difference. This indicates that people who were exposed to lethal cyberattacks reported higher levels of cyber threat perception as compared with people who were exposed to the nonlethal condition, and this heightened threat perception in turn led to more support for various cybersecurity polices.
Support for CAP (i.e. cybersecurity policies whereby the government or relevant organizations are expected to alert citizens if they have evidence of citizens’ computers being hacked or an act of cyberattack being detected) was predicted both by a direct effect of level of exposure to cyberattacks (NLC, LC) and by the mediation of threat perceptions.
Yet our models predicting support for oversight polices (COP) showed a different picture. In the NLC group we see a partial mediation of threat perceptions in addition to the direct effect that we found in the models shown in Table 2 . Support for COP (i.e. cybersecurity policies whereby the state should protect the country, organizations, and citizens from cyberattacks through direct government action) was predicted by a direct effect of NLC exposure and by the mediation of threat perceptions in both LC and NLC groups. In the LC group versus the control group, support of COP was predicted only through the mediation perceptions of threat. These results support our third hypothesis regarding the mediating role played by threat perception in predicting COP.
Our models predicting support for prevention policies (CPP) showed a complete mediation effect of threat perception in both experimental treatment groups. No direct effect of exposure on CPP was found, indicating that the mediating mechanism is the best predictor for CPP. Support for CPP (i.e. cybersecurity policies whereby the state compels commercial enterprises to install minimum thresholds of cybersecurity) was predicted by the indirect effect of threat perception.
These results emphasize the central role played by threat perception in predicting support for adopting stringent cybersecurity policies. What is especially noteworthy is that threat perception overrides past experience as the full mediation models indicate. For example, we found that when people are exposed to destructive cyberattacks, the level of perceived threat predicted support for adopting cybersecurity policies that required the state to protect citizens and organizations (COP). Similarly, we found that when it comes to predicting support for prevention policies—threat is the driving force.
In order to complement the indirect effect analyses and test the relative strength of the mediation pathways, we contrasted the indirect effects of the various groups on each policy option. According to the outcome estimates in Table 2 , model 3 has a significantly larger mediation effect compared with model 1 (difference = –0.014; 0.024 P < 0.001) 2 , which indicates that within the NLC group, the mediation model is a stronger predictor of support for COP than CAP. In other words, participants who were exposed to the nonlethal condition were more likely to support oversight polices than alert policies.
Our findings draw on an experimental design that suggests that exposure to different types of cyberattacks intensifies perceptions of cyber threats and shifts political attitudes in support of stringent cybersecurity policies. We find that exposure to lethal cyberattacks affects individual-level political behavior in a manner akin to conventional terrorism [ 68–71 ]. This research was motivated by a desire to better understand what drives individuals to support strong or hardline cybersecurity policies, using Israel as a case study. The findings contribute to this research direction in a number of important ways.
First, exposure to lethal cyberattacks heightens perceptions of cyber threat to a greater degree than nonlethal/economic cyberattacks. Second, as a result of exposure to cyberattacks, respondents were willing to forfeit civil liberties and privacy in exchange for more security. Like conventional terrorism, cyberattacks with lethal consequences harden political attitudes, as individuals tend to support more government oversight, greater regulation of cybersecurity among commercial businesses, and the implementation of strategies to increase public awareness following cyberattacks. Third, our data suggest that in some cases the mere exposure to cyberattack, either lethal or nonlethal, affects the level of support for specific types of cybersecurity polices (stronger support of cybersecurity alert policies among participants in the lethal cyberattack manipulation, and stronger support of cybersecurity oversight policy among participants in the nonlethal cyberattack treatment group). In other cases, threat perception, rather than the exposure to the cyber-events themselves, drive the cognitive effects of cyberattacks on attitudes toward policy (A strong support for COP among the LC group was predicted only through the mediating role of threat perception, and support of CPP, in both manipulation groups was predicted only through a mediated pathway). Finally, we observed differences in the way our mediation model works in relation to different cybersecurity policies. The mediation model for the nonlethal condition group participants predicted greater support for cybersecurity policies focusing on oversight rather than policies focusing on alerting the public.
Our study examined public support for three distinct types of cybersecurity policies that we described as prevention policies, alert policies, and oversight policies. Each of these play a role in securing cyberspace, where the uncertainty regarding the form and nature of potential threats calls for a varied array of preventive actions [ 36 , 37 ]. Each of these policies raises questions about the delicate balancing act between privacy and security demands. In reality, policy approaches are likely to combine several of these elements—yet it behooves us to first consider each of them independently since very little is known about the public knowledge and familiarity with different cybersecurity policies. While preliminary research has looked at public support for cybersecurity preferences in general [ 41 ], these have yet to consider the varied approaches to cybersecurity. To that end, in the current paper we tried to simplify the different cybersecurity polices as much as possible based on real-world policies.
Overall, the study provides evidence that exposure to cyberattacks predicts support for cybersecurity policies through the mediating effect of threat perception. Yet our discovery of differential effects depending on the type of cybersecurity policy being proposed adds a new level of nuance that should be probed further in subsequent studies. More so, results indicate that the public worry and concern in the aftermath of cyberattacks leads directly to calls for governmental intervention. This information sheds light on public opinion processes and helps inform our understanding how individuals will likely respond to new cyber threats. It may also help policymakers understand the complex emotions and cognitions evoked by attacks, which can improve policy formulations that respond to the needs of the public.
Future studies should also investigate how fear appeals intervene in this mechanism, and how to motivate people to take cyber threats more seriously in a way that leads to positive behavioral change.
Participants who were exposed to the lethal manipulation supported cybersecurity policies that focus on alerting the public in cases of cyberattacks more than participants in the two other groups. On the other hand, participants who were exposed to the nonlethal manipulation tended to support cybersecurity policies that call for state oversight of cybersecurity. We found no evidence that any type of exposure has a direct effect on support for polices mandating minimum thresholds of cybersecurity in the commercial arena.
One possible explanation for these results is that thus far, cyberattacks have caused economic damage, but lethal cyberattacks that vividly resemble terrorism are a significantly rarer phenomenon. Hence, participants who were exposed to lethal terror cyberattacks supported cybersecurity policies that would alert them and keep them informed about impending cyber threats. Policies that focus on oversight are perceived as less important during violent terror attacks. On the other hand, exposure to nonlethal cyberattacks, which are typically focused on economic gain, is more common. The economic damage caused by cyberattacks is estimated to reach $6 trillion by 2021 [ 72 ]. As such, participants in the nonlethal manipulation may have regarded cyberattacks causing economic damage as more likely and therefore supported polices that will bolster digital protections.
We note a key condition about the temporal nature of these findings. In analyzing the effect of exposure to cyberattacks, this study focuses on people's immediate response following exposure to cyber threats. Assessing people's short-term responses is valuable as the responses speak to the direction of the political and psychological effects. Yet what is missing from this picture (and beyond the scope of our research design), is the longevity of the response, which speaks to the strength of the effect. If the measured distress and political outcomes swiftly dissipate, then the policy relevance of our findings comes into question.
The literature is split on the question of the temporal durability of attitudinal shifts in the aftermath of major attacks. There is one school of thought that holds that most political effects stemming from political violence or terrorism are fleeting, and that the public is broadly desensitized to political violence [ 73–75 ]. Yet a second school of thought suggests that exposure to attacks can trigger prolonged effects and lasting shifts in political and psychological attitudes. Brandon & Silke [ 76 ] assert that while the distress triggered by exposure dissipates over time, this is not an instantaneous process. Several longitudinal studies following the Oklahama bombing and 9/11 found lingering harms, with exposed individuals reporting elevated levels of psychological distress and altered political attitudes for months or years following the event [ 77–79 ].
In applying this to the case of cyberattacks, there is insufficient evidence to positively determine the longevity of the political and psychological effects that we identified in our study. We anticipate that the effects will be more than fleeting, since the novelty of cyber threats means that people have yet to undergo any cognitive or emotional desensitization to cyberattacks [ 80 ]. However, we acknowledge that this this position requires further empirical substantiation in future research.
A central conclusion of this study is that the implementation of cybersecurity regulations should take account of public perception of cyber threats and public exposure to cyberattacks. This position challenges two unspoken yet ubiquitous notions in the field of cybersecurity. First, the formulation of cybersecurity policies—in a manner akin to national security and espionage discussions—has typically taken place without public input due to the perception that it is a question best left to experts with engineering or national security expertise [ 81 ]. Scholars argue that this complete abdication of cybersecurity policy to specialists is a profound mistake, since excluding “the general public from any meaningful voice in cyber policymaking removes citizens from democratic governance in an area where our welfare is deeply implicated” [ 82 ]. Functional cybersecurity relies on good practices by the ordinary public, and the failure of cybersecurity awareness campaigns to effectively change behavior may well be linked to the lack of public input in its regulation [ 81 ]. Our findings indicate that growing civilian exposure to cyberattacks leads to more defined attitudes toward specific cybersecurity regulations through the mechanism of heightened threat perception. Governments will increasingly need to engage the public as one of the stakeholders in effecting new cyber regulations.
A second conceptual dilemma about the role of public exposure and opinion has to do with the question of whether cybersecurity is a public good deserving of government investment and regulation at all. Much of the field of cybersecurity is dominated by private enterprise, with government involvement taking place in limited ways. Support for government intervention in the realm of cybersecurity is premised on the astronomical public costs of cybercrime, the threat of cyberterror attacks, and the claim of a market failure in the provision of cybersecurity whose negative externalities in the absence of government involvement would cause substantial national damage [ 83 ]. A prominent counter-school of thought, resting on a belief that the private market is the most efficient system of allocating economic resources, claims that there is no need for government intervention in the cybersecurity market [ 84 ]. These proponents of private sector cybersecurity suggest that the private sector can more effectively achieve cybersecurity outcomes, an assertion that is backed up by the fact that private spending on cybersecurity in 2018 reached USD $96 billion [ 85 ]. This raises the question of how civilian exposure to cyberattacks and the subsequent support for cybersecurity regulation can translate to real outcomes if the market responds to both public and private interests, which take account of public opinion and civilian threat perception in different ways.
Seeing that cyber threats are continuously evolving, there are opportunities to expand and consolidate this research in future studies. In the current article, we focus on the effect of exposure to lethal and nonlethal cyberattacks on support for different types of cybersecurity policies among Israeli participants. Yet despite this singular geographic focus, the results offer lessons that can be applied widely. Like several other Western countries, Israel has been repeatedly exposed to publicly reported cyberattacks on critical infrastructure. And, similarly to American and some European countries, Israel has high levels of Internet penetration and publicly renowned levels of cybersecurity readiness to deal with such attacks. Past studies that examined public perceptions of cyber threats have replicated the findings across multiple countries. Shandler et al . [ 80 ] found that psychological responses to internalized reports of cyberattacks explains support for military retaliation, and that this mechanism applies similarly in Israel, the United States, and England. Though requiring additional research, the evidence suggests that cyber threats operate via an underlying psycho-political mechanism that transcends national borders. In fact, the effects of cyberattacks may prove weaker in Israel than elsewhere as the constant exposure among Israelis to political violence places digital violence in the context of a political struggle that has, in many ways, fixed and acceptable costs [ 34 ]. Therefore, we believe that an Israeli sample offers major advantages in understanding the effects of cyberattacks among other Western nations. Nonetheless, we encourage future studies to corroborate these findings in different settings.
A second area where our findings could benefit from additional research relates to the nature of the media exposure. In this study, we exposed respondents to "initial" media reports about major cyberattacks where there is minimal information pertaining to the identity of the attacker and the type of attack that was conducted. While this in many ways reflects the reality of media reports about cyberattacks, it does not discount that journalists will sometimes make inferences about the details of an attack, and that later reports in the days and weeks following an attack will include far more detailed information. More so, this article bears implications for a wide literature beyond the political violence discipline. The public discussion regarding digital privacy and surveillance has spurred crucial new research on the dynamics of digital insecurity. In communications and media studies, for example, scientists are focusing on information-age warfare via different social media platforms, and early results show that citizens are as active in correcting disinformation online as they are in spreading disinformation [ 86 , 87 ]. The debate in the field of business management is also developing as it focuses on consumer expectations surrounding information technology and big data, as well as on the roles and responsibilities of public and private actors in securing personal data [ 88 , 89 ].
Cyber threats are a critical and growing component of national security. As this threat continues to grow all over the world, both in its public perception and in the true scope of the threat, the need to implement strong cybersecurity regulations will grow as well. Our findings indicate that particular forms of exposure to cyberattacks can contribute to support for various types of cybersecurity legislation and contribute to their public legitimacy. This is especially important since the introduction of these regulations constitutes a sacrifice of civil liberties, a sacrifice that citizens are prone to support only under particular conditions.
Though a DDoS attack, e.g. may not trigger physical casualties, its crippling of emergency services and telecommunications could catastrophically amplify the second- and third-order damage during a physical attack; for more, see Catherine A. Theohary and John W. Rollins, Cyberwarfare and cyberterrorism: In brief (Washington, DC: Congressional Research Service, 2015).
We also see a marginal significant effect between mediation 1 and 5 and 2 and 6. The differences between mediation 1 and mediation 5 show mediation 5 (NLC/control-threat-CPP) has a marginal significant larger mediation effect compared with mediation 1 (NLC/control-threat-CAP) (difference = –0.035; 0.035 P = 0.073). This means that within the NLC group the mediation model predicts stronger predicting CPP than CAP. In other words, participants who were exposed to the nonlethal (NLC) condition were more likely to support CPP than CAP. We saw that the CAP is stronger in the LC group. Another marginal significant effect was found between mediation 2 and mediation 6. The differences between mediation 2 and mediation 6 show mediation 6 (LC/control-threat-CPP) has a marginal significant larger mediation effect compared with mediation 2 (LC/control-threat-CAP) (difference = −0.044; 0.024 P = 0.062). This means that within the LC group the mediation model predicts stronger predicting CPP than CAP. In other words, participants who were exposed to the lethal (LC) condition were more likely to support CPP than CAP. We saw a direct effect of LC on CAP.
Geller E , Matishak M . A federal government left ‘completely blind’ on cyberattacks looks to force reporting . Politico . 2021 . https://www.politico.com/news/2021/05/15/congress-colonial-pipeline-disclosure-488406 (10 August, 2021, date last accessed) .
Google Scholar
Cybersecurity legislation 2020. NCSL . https://www.ncsl.org/research/telecommunications-and-information-technology/cybersecurity-legislation-2020.aspx (17 October 2020, date last accessed).
US state cybersecurity regulation more than doubled in 2017, while federal regulation waned. BusinessWire . https://www.businesswire.com/news/home/20180129005238/en/State-Cybersecurity-Regulation-Doubled-2017-Federal-Regulation (29 January 2018, last accessed) .
Kasper A . EU cybersecurity governance: stakeholders and normative intentions towards integration . In: Harwood M , Moncada S , Pace R (eds). The Future of the European Union: Demisting the Debate . Msida : Institute for European Studies , 2020 , 166 – 85 .
Google Preview
Israel National Cyber Directorate (INCD) . https://www.gov.il/en/departments/about/newabout (1 February 2021, date last accessed) .
Ochoa CS , Gadinger F , Yildiz T . Surveillance under dispute: conceptualizing narrative legitimation politics . Eur J Int Secur . 2021 ; 6 : 210 – 32 ..
Flyverbom M , Deibert R , Matten D . The governance of digital technology, big data, and the internet: new roles and responsibilities for business . Bus Soc . 2019 ; 58 : 3 – 19 ..
Rosenzweig P . The alarming trend of cybersecurity breaches and failures in the U.S. government . The Heritage Foundation. https://www.heritage.org/defense/report/the-alarming-trend-cybersecurity-breaches-and-failures-the-us-government-continues (17 April 2020, last accessed) .
Lee JK , Chang Y , Kwon HY et al. Reconciliation of privacy with preventive cybersecurity: the bright internet approach . Inf Syst Front . 2020 ; 22 : 45 – 57 .
Nye JS . Nuclear lessons for cyber security? . Strateg Stud Q . 2011 ; 5 : 18 – 38 .
Annual number of data breaches and exposed records in the United States from 2005 to 2018 (in millions) . Statista . https://www.statista.com/statistics/273550/data-breaches-recorded-in-the-united-states-by-number-of-breaches-and-records-exposed (26 February 2019, last accessed) .
For big banks, it's an endless fight with hackers The Business Times , 30 July 2019 . https://www.businesstimes.com.sg/banking-finance/for-big-banks-it%E2%80%99s-an-endless-fight-with-hackers
Nye JS Jr . Cyber Power . Cambridge : Harvard Kennedy School, Belfer Center for Science and International Affairs , 2010 .
Stohl M . Cyber terrorism: a clear and present danger, the sum of all fears, breaking point or patriot games? . Crime Law Soc Change . 2006 ; 46 : 223 – 38 .
Lawson ST . Cybersecurity Discourse in the United States: Cyber-Doom Rhetoric and Beyond . New York : Routledge , 2019 .
Valeriano B , Maness RC . Cyber War Versus Cyber Realities: Cyber Conflict in the International System . New York : Oxford University Press , 2015 .
Lawson S . Beyond cyber-doom: Assessing the limits of hypothetical scenarios in the framing of cyber-threats . J Inf Technol Polit . 2013 ; 10 : 86 – 103 .
Israeli cyber chief: Major attack on water systems thwarted. Washington Post. https://www.washingtonpost.com/world/middle_east/israeli-cyber-chief-major-attack-on-water-systems-thwarted/2020/05/28/5a923fa0-a0b5-11ea-be06-af5514ee0385_story.html (28 May 2020, last accessed) .
Panetta warns of dire threat of cyberattack on U.S. New York Times. (October 11, 2012). https://www.nytimes.com/2012/10/12/world/panetta-warns-of-dire-threat-of-cyberattack.html
Choi SJ , Johnson ME , Lehmann CU . Data breach remediation efforts and their implications for hospital quality . Health Serv Res . 2019 ; 54 : 971 – 80 .
Zetter K . A cyber attack has caused confirmed physical damage for the second time ever . Wired . 2015 . http://www.wired.com/2015/01/german-steel-mill-hack-destruction . (April 2020, date last accessed) .
Hobfoll SE , Canetti-Nisim D , Johnson RJ . Exposure to terrorism, stress-related mental health symptoms, and defensive coping among Jews and Arabs in Israel . J Consult Clin Psychol . 2006 ; 74 : 207 – 18 .
Halperin E , Canetti-Nisim D , Hirsch-Hoefler S . The central role of group-based hatred as an emotional antecedent of political intolerance: Evidence from Israel . Polit Psychol . 2009 ; 30 : 93 – 123 .
Bar-Tal D , Halperin E , de Rivera J . Collective emotions in conflict situations: societal implications . J Soc Issues . 2007 ; 63 : 441 – 60 .
Hirsch-Hoefler S , Canetti D , Rapaport C et al. Conflict will harden your heart: exposure to violence, psychological distress, and peace barriers in Israel and Palestine . Br J Polit Sci . 2016 ; 46 : 845 – 59 .
Bonanno GA , Jost JT . Conservative shift among high-exposure survivors of the September 11th terrorist attacks . Basic Appl Soc Psychol . 2006 ; 28 : 311 – 23 .
Canetti-Nisim D , Ariely G , Halperin E . Life, pocketbook, or culture: the role of perceived security threats in promoting exclusionist political attitudes toward minorities in Israel . Polit Res Q . 2008 ; 61 : 90 – 103 .
Zeitzoff T . Anger, exposure to violence, and intragroup conflict: a “lab in the field” experiment in southern Israel . Polit Psychol . 2014 ; 35 : 309 – 35 .
Schmitt N . Tallinn Manual 2.0 on the International Law Applicable to Cyber Operations . Cambridge : Cambridge University Press , 2017 .
Russian hackers appear to shift focus to U.S. power grid. The New York Times, 27 July 2018 . 2018 ;
Aucsmith D . Disintermediation, Counterinsurgency, and Cyber Defense . 2016 , Available at SSRN 2836100 . doi: 10.1093/cybsec/tyw018 , (10 August, 2021 last accessed) .
Gartzke E , Lindsay JR . Thermonuclear cyberwar . J Cybersecur . 2017 ; 3 : 37 – 48 .
Gross ML , Canetti D , Vashdi DR . Cyberterrorism: its effects on psychological well-being, public confidence and political attitudes . J Cybersecur . 2017 ; 3 : 49 – 58 .
Backhaus S , Gross ML , Waismel-Manor I et al. A cyberterrorism effect? Emotional reactions to lethal attacks on critical infrastructure . Cyberpsychol Behav Soc Netw . 2020 ; 23 : 595 – 603 ..
Gross ML , Canetti D , Vashdi DR . The psychological effects of cyber-terrorism . Bull At Sci . 2016 ; 72 : 284 – 91 .
Canetti D , Gross ML , Waismel-Manor I . Immune from cyber-fire? The psychological & physiological effects of cyberwar . In: Allhoff F , Henschke A , Strawser BJ (eds). Binary Bullets: The Ethics of Cyberwarfare . Oxford : Oxford University Press , 2016 , 157 – 76 .
Canetti D , Gross ML , Waismel-Manor I et al. How cyberattacks terrorize: Cortisol and personal insecurity jump in the wake of cyberattacks . Cyberpsychol Behav Soc Netw . 2017 ; 20 : 72 – 7 .
Shandler R , Gross MG , Backhaus S et al. Cyber terrorism and public support for retaliation: a multi-country survey experiment . Br J Polit Sci . 1 – 19 ., 2021 . DOI: 10.1017/S0007123420000812 .
Rosenzweig P . Cybersecurity and public goods, The public/private ‘partnership’ . In: Berkowitz P (ed). Emerging Threats in National Security and Law . Stanford : Hoover Institution, Stanford University , 2011 , 1 – 36 .
Cheung-Blunden V , Cropper K , Panis A et al. Functional divergence of two threat-induced emotions: fear-based versus anxiety-based cybersecurity preferences . Emotion . 2017 ; 19 : 1353 – 65 .
Jardine E , Porter N . Pick your poison: the attribution paradox in cyberwar. 2020 , https://osf.io/preprints/socarxiv/etb72/ .
Rid T , Buchanan B . Attributing cyber attacks . J Strateg Stud . 2015 ; 38 : 4 – 37 .
Clark DD , Landau S . Untangling attribution . Harvard National Secur J . 2011 ; 2 : 323 – 52 .
Alraddadi W , Sarvotham H . A comprehensive analysis of WannaCry: technical analysis, reverse engineering, and motivation . https://docplayer.net/130787668-A-comprehensive-analysis-of-wannacry-technical-analysis-reverse-engineering-and-motivation.html , (17 April 2020, last accessed).
Romanosky S , Boudreaux B . Private-sector attribution of cyber incidents: benefits and risks to the US government . Int J Intell CounterIntelligence . 2020 ; 0 : 1 – 31 .
Baezner M . Iranian cyber-activities in the context of regional rivalries and international tensions . ETH Zurich . 2019 : 1 – 37 .
Macdonald S , Jarvis L , Nouri L . State cyberterrorism: a contradiction in terms? . J Terrorism Res . 2015 ; 6 : 62 – 75 .
Canetti D , Gubler J , Zeitzoff T . Motives don't matter? Motive attribution and counterterrorism policy . Polit Psychol . 2021 ; 42 : 483 – 99 .
Liberman P , Skitka LJ . Revenge in US public support for war against Iraq . Public Opin Q . 2017 ; 81 : 636 – 60 .
Liberman P , Skitka LJ . Vicarious retribution in US public support for war against Iraq . Secur Stud . 2019 ; 28 : 189 – 215 .
Kostyuk N , Wayne C . The microfoundations of state cybersecurity: cyber risk perceptions and the mass public . J Glob Secur Stud . 2021 ; 6 : ogz077 .
Gomez MA . Past behavior and future judgements: seizing and freezing in response to cyber operations . J Cybersecur . 2019 ; 5 : 1 – 19 .
Gomez MA , Villar EB . Fear, uncertainty, and dread: cognitive heuristics and cyber threats . Polit Gov . 2018 ; 6 : 61 – 72 .
Harrell E , Langton L . The Victims of Identity Theft, 2012 . US Department of Justice, Office of Justice Programs, Bureau of Justice Statistics , 2013 . https://www.bjs.gov/content/pub/pdf/vit12.pdf
Sinclair SJ , Antonius D . The Psychology of Terrorism Fears . Oxford : Oxford University Press , 2012 .
Quillian L . Prejudice as a response to perceived group threat: population composition and anti-immigrant and racial prejudice in Europe . Am Sociol Rev . 1995 ; 60 : 586 – 611 .
Ben-Nun Bloom P , Arikan G , Lahav G . The effect of perceived cultural and material threats on ethnic preferences in immigration attitudes . Ethn Racial Stud . 2015 ; 38 : 1760 – 78 .
Shoshani A , Slone M . The drama of media coverage of terrorism: emotional and attitudinal impact on the audience . Stud Confl Terror . 2008 ; 31 : 627 – 40 ..
Huddy L , Smirnov O , Snider KL et al. Anger, anxiety, and selective exposure to terrorist violence . J Confl Resolut . 2021 : 00220027211014937 .
Greenberg J , Pyszczynski T , Solomon S . The causes and consequences of a need for self-esteem: a terror management theory . In: Public Self and Private Self . New York, NY : Springer , 1986 , 212 – 189 .
Hall BJ , Hobfoll SE , Canetti D et al. The defensive nature of benefit finding during ongoing terrorism: an examination of a national sample of Israeli Jews . J Soc Clin Psychol . 2009 ; 28 : 993 – 1021 ..
Canetti D , Hall BJ , Rapaport C et al. Exposure to political violence and political extremism . Eur Psychol . 2013 ; 18 : 263 – 72 .
McCallister E . Guide to Protecting the Confidentiality of Personally Identifiable Information . Darby : Diane Publishing , 2010 .
Graves J , Acquisti A , Anderson R . Experimental measurement of attitudes regarding cybercrime . In: 13th Annual Workshop on the Economics of Information Security . 2014 ; Pennsylvania State University.
Huddy L , Feldman S , Capelos T et al. The consequences of terrorism: disentangling the effects of personal and national threat . Polit Psychol . 2002 ; 23 : 485 – 509 .
Hefetz A , Liberman G . The factor analysis procedure for exploration: a short guide with examples . Cult Educ . 2017 ; 29 : 526 – 62 .
Muthén LK , Muthén BO . MPlus: Statistical Analysis with Latent Variables: User's Guide . Muthén & Muthén , Los Angeles, CA , 2012 .
Galea S , Ahern J , Resnick H et al. Psychological sequelae of the September 11 terrorist attacks in New York City . N Engl J Med . 2002 ; 346 : 982 – 7 .
Canetti-Nisim D , Halperin E , Sharvit K et al. A new stress-based model of political extremism: personal exposure to terrorism, psychological distress, and exclusionist political attitudes . J Confl Res . 2009 ; 53 : 363 – 89 .
Canetti D , Snider KLG , Pedersen A et al. Threatened or threatening? How ideology shapes asylum seekers’ immigration policy attitudes in Israel and Australia . J Refug Stud . 2016 ; 29 : 583 – 606 .
Morgan S . Cybersecurity Ventures predicts cybercrime will cost the world in excess of $6 trillion annually by 2021. Cybercrime Magazine . 2017 ; https://cybersecurityventures.com/hackerpocalypse-cybercrime-report-2016/ (11 May 2020, date last accessed) .
Yakter A , Harsgor L . Long-term change in conflict attitudes: a dynamic approach . 2021 . http://liran.harsgor.com/wp-content/uploads/2021/07/YakterHarsgor_2021_Long-term-conflict.pdf
Brouard S , Vasilopoulos P , Foucault M . How terrorism affects political attitudes: France in the aftermath of the 2015–2016 attacks . West Eur Polit . 2018 ; 41 : 1073 – 99 .
Castanho Silva B . The (non)impact of the 2015 Paris terrorist attacks on political attitudes . Pers Soc Psychol Bull . 2018 ; 44 : 838 – 50 .
Brandon SE , Silke AP . Near- and long-term psychological effects of exposure to terrorist attacks . In: Bongar B , Brown LM , Beutler LE , al. et (eds). Psychology of Terrorism . Oxford: Oxford University Press 2007 , 175 – 93 .
Pfefferbaum B , Nixon SJ , Krug RS et al. Clinical needs assessment of middle and high school students following the 1995 Oklahoma City bombing . Am J Psychiatry . 1999 ; 156 : 1069 – 74 ..
Galea S , Vlahov D , Resnick H et al. Trends of probable post-traumatic stress disorder in New York City after the September 11 terrorist attacks . Am J Epidemiol . 2003 ; 158 : 514 – 24 ..
Landau MJ , Solomon S , Greenberg J et al. Deliver us from evil: the effects of mortality salience and reminders of 9/11 on support for President George W. Bush . Pers Soc Psychol Bull . 2004 ; 30 : 1136 – 50 ..
Nussio E . Attitudinal and emotional consequences of Islamist terrorism. Evidence from the Berlin attack . Polit Psychol . 2020 ; 41 : 1151 – 71 ..
Bada M , Sasse AM , Nurse JRC . Cyber security awareness campaigns: why do they fail to change behaviour? In: International Conference on Cyber Security for Sustainable Society , Global Cyber Security Capacity Centre. 2015 , 1 – 11 .
Shane PM . Cybersecurity policy as if ‘ordinary citizens’ mattered: the case for public participation in cyber policy making . SSRN Electron J . 2012 ; 8 : 433 – 62 .
Shandler R . White paper: Israel as a cyber power . 2019 , DOI: 10.13140/RG.2.2.15936.07681 .
Gartner forecasts worldwide security spending will reach $96 billion in 2018, up 8 percent from 2017. Gartner. https://www.gartner.com/newsroom/id/3836563 (1 August 2019, date last accessed) .
Shandler R , Gross ML , Canetti D . A fragile public preference for using cyber strikes: evidence from survey experiments in the United States, United Kingdom and Israel . Contemp Secur Policy . 2021 ; 42 : 135 – 62 .
Prier J . Commanding the trend: social media as information warfare . Strateg Stud Q . 2017 ; 11 : 50 – 85 ..
Golovchenko Y , Hartmann M , Adler-Nissen R . State, media and civil society in the information warfare over Ukraine: citizen curators of digital disinformation . Int Aff . 2018 ; 94 : 975 – 94 ..
Belk RW . Extended self in a digital world . J Consum Res . 2013 ; 40 : 477 – 500 .
West SM . Data capitalism: redefining the logics of surveillance and privacy . Bus Soc . 2019 ; 58 : 20 – 41 .
Cahane A . The new Israeli cyber draft bill: a preliminary overview . CSRCL . 2018 . https://csrcl.huji.ac.il/news/new-israeli-cyber-law-draft-bill . (10 August, 2021, date last accessed) .
Supplementary data
Month: | Total Views: |
---|---|
October 2021 | 1,060 |
November 2021 | 1,171 |
December 2021 | 742 |
January 2022 | 658 |
February 2022 | 455 |
March 2022 | 444 |
April 2022 | 527 |
May 2022 | 576 |
June 2022 | 512 |
July 2022 | 410 |
August 2022 | 416 |
September 2022 | 556 |
October 2022 | 669 |
November 2022 | 639 |
December 2022 | 443 |
January 2023 | 344 |
February 2023 | 372 |
March 2023 | 482 |
April 2023 | 464 |
May 2023 | 525 |
June 2023 | 375 |
July 2023 | 355 |
August 2023 | 318 |
September 2023 | 658 |
October 2023 | 1,190 |
November 2023 | 1,584 |
December 2023 | 1,169 |
January 2024 | 1,232 |
February 2024 | 1,266 |
March 2024 | 1,357 |
April 2024 | 1,519 |
May 2024 | 1,447 |
June 2024 | 1,021 |
July 2024 | 940 |
August 2024 | 1,038 |
Email alerts
Citing articles via, affiliations.
- Online ISSN 2057-2093
- Print ISSN 2057-2085
- Copyright © 2024 Oxford University Press
- About Oxford Academic
- Publish journals with us
- University press partners
- What we publish
- New features
- Open access
- Institutional account management
- Rights and permissions
- Get help with access
- Accessibility
- Advertising
- Media enquiries
- Oxford University Press
- Oxford Languages
- University of Oxford
Oxford University Press is a department of the University of Oxford. It furthers the University's objective of excellence in research, scholarship, and education by publishing worldwide
- Copyright © 2024 Oxford University Press
- Cookie settings
- Cookie policy
- Privacy policy
- Legal notice
This Feature Is Available To Subscribers Only
Sign In or Create an Account
This PDF is available to Subscribers Only
For full access to this pdf, sign in to an existing account, or purchase an annual subscription.
Cyber Security Threats and Vulnerabilities: A Systematic Mapping Study
- Research Article - Computer Engineering and Computer Science
- Published: 06 January 2020
- Volume 45 , pages 3171–3189, ( 2020 )
Cite this article
- Mamoona Humayun 1 ,
- Mahmood Niazi 2 ,
- NZ Jhanjhi ORCID: orcid.org/0000-0001-8116-4733 3 ,
- Mohammad Alshayeb 2 &
- Sajjad Mahmood 2
16k Accesses
143 Citations
Explore all metrics
There has been a tremendous increase in research in the area of cyber security to support cyber applications and to avoid key security threats faced by these applications. The goal of this study is to identify and analyze the common cyber security vulnerabilities. To achieve this goal, a systematic mapping study was conducted, and in total, 78 primary studies were identified and analyzed. After a detailed analysis of the selected studies, we identified the important security vulnerabilities and their frequency of occurrence. Data were also synthesized and analyzed to present the venue of publication, country of publication, key targeted infrastructures and applications. The results show that the security approaches mentioned so far only target security in general, and the solutions provided in these studies need more empirical validation and real implementation. In addition, our results show that most of the selected studies in this review targeted only a few common security vulnerabilities such as phishing, denial-of-service and malware. However, there is a need, in future research, to identify the key cyber security vulnerabilities, targeted/victimized applications, mitigation techniques and infrastructures, so that researchers and practitioners could get a better insight into it.
This is a preview of subscription content, log in via an institution to check access.
Access this article
Subscribe and save.
- Get 10 units per month
- Download Article/Chapter or eBook
- 1 Unit = 1 Article or 1 Chapter
- Cancel anytime
Price includes VAT (Russian Federation)
Instant access to the full article PDF.
Rent this article via DeepDyve
Institutional subscriptions
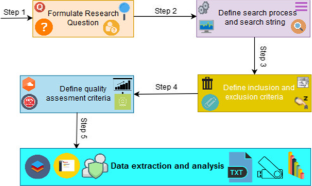
Similar content being viewed by others
Cybersecurity Vulnerabilities Assessment (A Systematic Review Approach)
A Systematic Literature Review on Information Security Leakage: Evaluating Security Threat
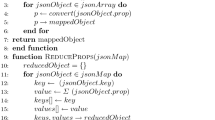
Threats on the horizon: understanding security threats in the era of cyber-physical systems
Explore related subjects.
- Artificial Intelligence
Lun, Y.Z.; et al.: Cyber-physical systems security: a systematic mapping study. arXiv:1605.09641 (2016)
Razzaq, A.; et al.: Cyber security: threats, reasons, challenges, methodologies and state of the art solutions for industrial applications. In: 2013 IEEE Eleventh International Symposium on Autonomous Decentralized Systems (ISADS). IEEE (2013)
Von Solms, R.; Van Niekerk, J.: From information security to cyber security. Comput. Secur. 38 , 97–102 (2013)
Article Google Scholar
Benson, V.; McAlaney, J.; Frumkin, L.A.: Emerging threats for the human element and countermeasures in current cyber security landscape. Psychological and Behavioral Examinations in Cyber Security, pp. 266–271. IGI Global, Hershey (2018)
Chapter Google Scholar
Bada, M.; Sasse, A.M.; Nurse, J.R.: Cyber security awareness campaigns: why do they fail to change behaviour? arXiv:1901.02672 (2019)
Floyd, D.H.; Shelton, J.W.; Bush, J.E.: Systems and methods for detecting a security breach in an aircraft network. Google Patents (2018)
Taha, A.F.; et al.: Risk mitigation for dynamic state estimation against cyber attacks and unknown inputs. IEEE Trans. Smart Grid 9 (2), 886–899 (2018)
Valeriano, B.; Maness, R.C.: International relations theory and cyber security. In: Brown, C., Eckersley, R. (eds.) The Oxford Handbook of International Political Theory, p. 259. Oxford University Press, Oxford (2018)
Google Scholar
von Solms, B.; von Solms, R.: Cybersecurity and information security—what goes where? Inf. Comput. Secur. 26 (1), 2–9 (2018)
Ron, M.: Situational status of global cybersecurity and cyber defense according to global indicators. Adaptation of a model for ecuador. In: Developments and Advances in Defense and Security: Proceedings of the Multidisciplinary International Conference of Research Applied to Defense and Security (MICRADS 2018). Springer (2018)
Al Mazari, A.; et al.: Cyber terrorism taxonomies: definition, targets, patterns, risk factors, and mitigation strategies. Cyber Security and Threats: Concepts, Methodologies, Tools, and Applications, pp. 608–621. IGI Global, Hershey (2018)
Hansen, L.; Nissenbaum, H.: Digital disaster, cyber security, and the Copenhagen School. Int. Stud. Q. 53 (4), 1155–1175 (2009)
Kuehl, D.T.: From cyberspace to cyberpower: Defining the problem. Cyberpower and National Security, vol. 30. National Defense University Press, Washington, D.C (2009)
Benedickt, M.: Cyberspace: First Steps. MIT Press, Cambridge (1991)
Gunkel, D.J.: Hacking Cyberspace. Routledge, Abingdon (2018)
Book Google Scholar
Abomhara, M.; Køien, G.M.: Cyber security and the internet of things: vulnerabilities, threats, intruders and attacks. J. Cyber Secur. 4 (1), 65–88 (2015)
Mittal, S.; et al.: Cybertwitter: using twitter to generate alerts for cybersecurity threats and vulnerabilities. In: Proceedings of the 2016 IEEE/ACM International Conference on Advances in Social Networks Analysis and Mining. IEEE Press (2016)
Johnson, C.; et al.: Guide to cyber threat information sharing. NIST Spec. Publ. 800 , 150 (2016)
Rid, T.; Buchanan, B.: Attributing cyber attacks. J. Strateg. Stud. 38 (1–2), 4–37 (2015)
Banks, W.C.: Cyber espionage and electronic surveillance: beyond the media coverage. Emory L. J. 66 , 513 (2016)
Zhang, H.; et al.: Optimal denial-of-service attack scheduling with energy constraint. IEEE Trans. Autom. Control 60 (11), 3023–3028 (2015)
Article MathSciNet Google Scholar
Kustarz, C.: et al.: System and method for denial of service attack mitigation using cloud services. Google Patents (2016)
Niemelä, J.; Hyppönen, M.; Kangas, S.: Malware protection. Google Patents (2016)
Choo, K.-K.R.: The cyber threat landscape: challenges and future research directions. Comput. Secur. 30 (8), 719–731 (2011)
Parmar, B.: Protecting against spear-phishing. Comput. Fraud Secur. 2012 (1), 8–11 (2012)
Dodge Jr., R.C.; Carver, C.; Ferguson, A.J.: Phishing for user security awareness. Comput. Secur. 26 (1), 73–80 (2007)
Sharma, P.; Johari, R.; Sarma, S.: Integrated approach to prevent SQL injection attack and reflected cross site scripting attack. Int. J. Syst. Assur. Eng. Manag. 3 (4), 343–351 (2012)
Choraś, M.; et al.: Correlation approach for SQL injection attacks detection. In: International Joint Conference CISIS’12-ICEUTE´12-SOCO´12 Special Sessions. Springer (2013)
Brar, H.S.; Kumar, G.: Cybercrimes: a proposed taxonomy and challenges. J. Comput. Netw. Commun. 2018 , Article ID 1798659 (2018)
Gill, R.S.; Smith, J.; Looi, M.H.; Clark, A.J.: Passive techniques for detecting session hijacking attacks in IEEE 802.11 wireless networks. In: Clark, A.J., Kerr, K., Mohay, G.M. (eds.) AusCERT Asia Pacific Information Technology Security Conference: Refereed R&D Stream, 22–26 May 2005, Gold Coast, Australia (2005)
Wassermann, G.; Su, Z.: Static detection of cross-site scripting vulnerabilities. In: Proceedings of the 30th International Conference on Software Engineering. ACM (2008)
Kieyzun, A.; et al.: Automatic creation of SQL injection and cross-site scripting attacks. In: Proceedings of the 31st International Conference on Software Engineering. IEEE Computer Society (2009)
Nguyen, P.H.; Ali, S.; Yue, T.: Model-based security engineering for cyber-physical systems: a systematic mapping study. Inf. Softw. Technol. 83 , 116–135 (2017)
Hydara, I.; et al.: Current state of research on cross-site scripting (XSS)—a systematic literature review. Inf. Softw. Technol. 58 , 170–186 (2015)
Muccini, H.; Sharaf, M.; Weyns, D.:. Self-adaptation for cyber-physical systems: a systematic literature review. In: Proceedings of the 11th International Symposium on Software Engineering for Adaptive and Self-managing Systems. ACM (2016)
Mishna, F.; et al.: Interventions to prevent and reduce cyber abuse of youth: a systematic review. Res. Soc. Work Pract. 21 (1), 5–14 (2011)
Lewis, G.; Lago, P.: Architectural tactics for cyber-foraging: results of a systematic literature review. J. Syst. Softw. 107 , 158–186 (2015)
Rahim, N.H.A.; et al.: A systematic review of approaches to assessing cybersecurity awareness. Kybernetes 44 (4), 606–622 (2015)
Enoch, S.Y.; et al.: A systematic evaluation of cybersecurity metrics for dynamic networks. Comput. Netw. 144 , 216–229 (2018)
Ramaki, A.A.; Rasoolzadegan, A.; Bafghi, A.G.: A systematic mapping study on intrusion alert analysis in intrusion detection systems. ACM Comput. Surv. (CSUR) 51 (3), 55 (2018)
Chockalingam, S.; et al.: Bayesian network models in cyber security: a systematic review. In: Nordic Conference on Secure IT Systems. Springer (2017)
Alguliyev, R.; Imamverdiyev, Y.; Sukhostat, L.: Cyber-physical systems and their security issues. Comput. Ind. 100 , 212–223 (2018)
Franke, U.; Brynielsson, J.: Cyber situational awareness—a systematic review of the literature. Comput. Secur. 46 , 18–31 (2014)
Budgen, D.; Brereton, P.: Performing systematic literature reviews in software engineering. In: Proceedings of the 28th International Conference on Software Engineering. ACM (2006)
Kitchenham, B.A.; Budgen, D.; Brereton, O.P.: The value of mapping studies-A participant-observer case study. In: EASE (2010)
Petersen, K.; Vakkalanka, S.; Kuzniarz, L.: Guidelines for conducting systematic mapping studies in software engineering: an update. Inf. Softw. Technol. 64 , 1–18 (2015)
Niazi, M.: Do systematic literature reviews outperform informal literature reviews in the software engineering domain? An initial case study. Arab. J. Sci. Eng. 40 (3), 845–855 (2015)
Chong, R.: Quick reference guide to endnote (2018)
Beecham, S.; et al.: Using an expert panel to validate a requirements process improvement model. J. Syst. Softw. 76 (3), 251–275 (2005)
Mohammed, N.M.; et al.: Exploring software security approaches in software development lifecycle: a systematic mapping study. Comput. Stand. Interfaces 50 , 107–115 (2017)
Mufti, Y.; et al.: A readiness model for security requirements engineering. IEEE Access 6 , 28611–28631 (2018)
Download references
Acknowledgements
The authors would like to acknowledge the support provided by the Deanship of Scientific Research via the project number IN161024 at King Fahd University of Petroleum and Minerals, Saudi Arabia. In addition, we are grateful to the participants who evaluated the proposed model and recommended improvements.
Author information
Authors and affiliations.
Department of Information systems, College of Computer and Information Sciences, Jouf University, Al-Jouf, Saudi Arabia
Mamoona Humayun
Information and Computer Science Department, King Fahd University of Petroleum and Minerals (KFUPM), Dhahran, Saudi Arabia
Mahmood Niazi, Mohammad Alshayeb & Sajjad Mahmood
SoCIT, Taylor’s University, Subang Jaya, Malaysia
You can also search for this author in PubMed Google Scholar
Corresponding author
Correspondence to NZ Jhanjhi .
Appendix A: Data Extraction Form
Section 1: Paper information | |
---|---|
Paper title: | |
Authors: | Year of publication: |
Reference type: Journal/Conference | Publisher: |
Country: |
Section 2: Quality assessment | |
---|---|
The findings and results of study are clearly stated? | Yes No |
The findings of the study are evaluated empirically? | Yes No |
The study has been published in a relevant journal or conference? | Very relevant Relevant Not relevant |
The study has been cited by other authors? | Yes Partially No |
Section 3: Data extraction | |
---|---|
Questions | Possible answers |
Which application is targeted for cybercrime in the given study? | Application name |
Which method is used to protect the application for cyber attack? | Method name |
Which cyber connection is used for committing cybercrime? | Connection name |
Who are the victims of cybercrimes in the given study? | Individual Organization |
Which cyber security vulnerability is discussed in the study? | Malware Phishing SQL injection attack Cross-site scripting (XSS) Denial-of-service (DoS) Session hijacking and man-in-the-middle attacks Credential reuse Others |
What is the severity of discussed cyber security vulnerability? | Critical High Medium Low |
Which technique is used in the study for detecting cyber threats? | Technique name |
What kind of data is used for validation? Data characteristics | Academia Industrial Government Mixed |
Which empirical validation methods are used in the proposed approach? | Case study Experiment Simulation Others |
Appendix B: Finally Selected Papers
Khandpur, Rupinder Paul, et al. “Crowdsourcing cybersecurity: Cyber attack detection using social media.” Proceedings of the 2017 ACM on Conference on Information and Knowledge Management. ACM, 2017.
Li, Zhen, Deqing Zou, Shouhuai Xu, Hai Jin, Hanchao Qi, and Jie Hu. “VulPecker: an automated vulnerability detection system based on code similarity analysis.” In Proceedings of the 32nd Annual Conference on Computer Security Applications , pp. 201–213. ACM, 2016.
Cheng, Maggie, Mariesa Crow, and Robert F. Erbacher. “Vulnerability analysis of a smart grid with monitoring and control system.” Proceedings of the Eighth Annual Cyber Security and Information Intelligence Research Workshop . ACM, 2013.
Zanero, Stefano. “Ulisse, a network intrusion detection system.” In Proceedings of the 4th annual workshop on Cyber security and information intelligence research: developing strategies to meet the cyber security and information intelligence challenges ahead , p. 20. ACM, 2008.
Werner, Gordon, Shanchieh Yang, and Katie McConky. “Time series forecasting of cyber attack intensity.” In Proceedings of the 12th Annual Conference on cyber and information security research , p. 18. ACM, 2017.
Masi, Denise, Martin J. Fischer, John F. Shortle, and Chun-Hung Chen. “Simulating network cyber attacks using splitting techniques. ACM” In Proceedings of the Winter Simulation Conference , pp. 3217–3228. Winter Simulation Conference, 2011.
Okutan, Ahmet, Shanchieh Jay Yang, and Katie McConky. “Predicting cyber attacks with bayesian networks using unconventional signals.” In Proceedings of the 12th Annual Conference on Cyber and Information Security Research , p. 13. ACM, 2017.
Farraj, Abdallah, Eman Hammad, and Deepa Kundur. “Impact of Cyber Attacks on Data Integrity in Transient Stability Control.” In Proceedings of the 2nd Workshop on Cyber - Physical Security and Resilience in Smart Grids , pp. 29–34. ACM, 2017.
Kuhl, Michael E., Jason Kistner, Kevin Costantini, and Moises Sudit. “Cyber attack modeling and simulation for network security analysis.” In Proceedings of the 39th Conference on Winter Simulation: 40 years! The best is yet to come , pp. 1180–1188. ACM Press, 2007.
Gudo, Munyaradzi, and Keshnee Padayachee. “SpotMal: A hybrid malware detection framework with privacy protection for BYOD.” In Proceedings of the 2015 Annual Research Conference on South African Institute of Computer Scientists and Information Technologists , p. 18. ACM, 2015.
Kim, Ikkyun, Daewon Kim, Byunggoo Kim, Yangseo Choi, Seongyong Yoon, Jintae Oh, and Jongsoo Jang. “A case study of unknown attack detection against Zero-day worm in the honeynet environment.” In 2009 11th International Conference on Advanced Communication Technology , vol. 3, pp. 1715–1720. IEEE, 2009.
Ahmadloo, Fatemeh, and Farzad Rajaei Salmasi. “A cyber-attack on communication link in distributed systems and detection scheme based on H-infinity filtering.” In 2017 IEEE International Conference on Industrial Technology (ICIT) , pp. 698–703. IEEE, 2017.
Aishwarya, R., and S. Malliga. “Intrusion detection system-An efficient way to thwart against Dos/DDos attack in the cloud environment.” In 2014 International Conference on Recent Trends in Information Technology , pp. 1–6. IEEE, 2014.
Al-Dabbagh, Ahmad W., Yuzhe Li, and Tongwen Chen. “An intrusion detection system for cyber attacks in wireless networked control systems.” IEEE Transactions on Circuits and Systems II: Express Briefs 65, no. 8 (2017): 1049–1053.
Alom, Md Zahangir, and Tarek M. Taha. “Network intrusion detection for cyber security on neuromorphic computing system.” In 2017 International Joint Conference on Neural Networks (IJCNN) , pp. 3830–3837. IEEE, 2017.
Aparicio-Navarro, Francisco J., Konstantinos G. Kyriakopoulos, Yu Gong, David J. Parish, and Jonathon A. Chambers. “Using pattern-of-life as contextual information for anomaly-based intrusion detection systems.” IEEE Access 5 (2017): 22177–22193.
Bhadre, Parvati, and Deepali Gothawal. “Detection and blocking of spammers using SPOT detection algorithm.” In 2014 First International Conference on Networks & Soft Computing (ICNSC2014) , pp. 97–101. IEEE, 2014.
Bottazzi, Giovanni, Emiliano Casalicchio, Davide Cingolani, Fabio Marturana, and Marco Piu. “MP-Shield: a framework for phishing detection in mobile devices.” In 2015 IEEE International Conference on Computer and Information Technology; Ubiquitous Computing and Communications; Dependable, Autonomic and Secure Computing; Pervasive Intelligence and Computing , pp. 1977–1983. IEEE, 2015.
Chen, Chia-Mei, Han-Wei Hsiao, Peng-Yu Yang, and Ya-Hui Ou. “Defending malicious attacks in cyber physical systems.” In 2013 IEEE 1st International Conference on Cyber - Physical Systems, Networks, and Applications (CPSNA) , pp. 13–18. IEEE, 2013.
Chen, Chia-Mei, Ya-Hui Ou, and Yu-Chou Tsai. “Web botnet detection based on flow information.” In 2010 International Computer Symposium (ICS2010) , pp. 381–384. IEEE, 2010.
Chonka, Ashley, and Jemal Abawajy. “Detecting and mitigating HX-DoS attacks against cloud web services.” In 2012 15th International Conference on Network - Based Information Systems , pp. 429–434. IEEE, 2012.
Devi, BS Kiruthika, G. Preetha, G. Selvaram, and S. Mercy Shalinie. “An impact analysis: Real time DDoS attack detection and mitigation using machine learning.” In 2014 International Conference on Recent Trends in Information Technology , pp. 1–7. IEEE, 2014.
Eslahi, Meisam, Habibah Hashim, and Nooritawati Md Tahir. “An efficient false alarm reduction approach in HTTP-based botnet detection.” In 2013 IEEE Symposium on Computers & Informatics (ISCI) , pp. 201–205. IEEE, 2013.
Gantsou, Dhavy. “On the use of security analytics for attack detection in vehicular ad hoc networks.” In 2015 International Conference on Cyber Security of Smart Cities, Industrial Control System and Communications (SSIC) , pp. 1–6. IEEE, 2015.
Hesar, Amin Danandeh, and Mahmoud Ahmadian Attari. “Simulating and analysis of cyber attacks on a BLPC network.” In 2014 Smart Grid Conference (SGC) , pp. 1–6. IEEE, 2014.
Hong, Junho, Chen-Ching Liu, and Manimaran Govindarasu. “Integrated anomaly detection for cyber security of the substations.” IEEE Transactions on Smart Grid 5, no. 4 (2014): 1643–1653.
Hu, Xin, Jiyong Jang, Marc Ph Stoecklin, Ting Wang, Douglas L. Schales, Dhilung Kirat, and Josyula R. Rao. “BAYWATCH: robust beaconing detection to identify infected hosts in large-scale enterprise networks.” In 2016 46th Annual IEEE/IFIP International Conference on Dependable Systems and Networks (DSN) , pp. 479–490. IEEE, 2016.
Ichise, Hikaru, Yong Jin, and Katsuyoshi Iida. “Analysis of via-resolver DNS TXT queries and detection possibility of botnet communications.” In 2015 IEEE Pacific Rim Conference on Communications, Computers and Signal Processing (PACRIM) , pp. 216–221. IEEE, 2015.
Indre, Ionut, and Camelia Lemnaru. “Detection and prevention system against cyber attacks and botnet malware for information systems and Internet of Things.” In 2016 IEEE 12th International Conference on Intelligent Computer Communication and Processing (ICCP) , pp. 175–182. IEEE, 2016.
Jakaria, A. H. M., Wei Yang, Bahman Rashidi, Carol Fung, and M. Ashiqur Rahman. “Vfence: A defense against distributed denial of service attacks using network function virtualization.” In 2016 IEEE 40th Annual Computer Software and Applications Conference (COMPSAC) , vol. 2, pp. 431–436. IEEE, 2016.
Jin, Guang, Fei Zhang, Yuan Li, Honghao Zhang, and Jiangbo Qian. “A Hash-based Path Identification Scheme for DDoS Attacks Defense.” In 2009 Ninth IEEE International Conference on Computer and Information Technology , vol. 2, pp. 219–224. IEEE, 2009.
Jing, Tao, Jun Li, and Rong Xing. “Research on malicious links detection system based on script text analysis.” In 2012 14th International Conference on Advanced Communication Technology (ICACT) , pp. 439–442. IEEE, 2012.
Khan, Mohiuddin Ali, Sateesh Kumar Pradhan, and Huda Fatima. “Applying data mining techniques in cyber crimes.” In 2017 2nd International Conference on Anti - Cyber Crimes (ICACC) , pp. 213–216. IEEE, 2017.
Khan, Muhammad Salman, Ken Ferens, and Witold Kinsner. “A chaotic measure for cognitive machine classification of distributed denial of service attacks.” In 2014 IEEE 13th International Conference on Cognitive Informatics and Cognitive Computing , pp. 100–108. IEEE, 2014.
Kong, Xinling, Yonghong Chen, Hui Tian, Tian Wang, Yiqiao Cai, and Xin Chen. “A novel botnet detection method based on preprocessing data packet by graph structure clustering.” In 2016 International Conference on Cyber - Enabled Distributed Computing and Knowledge Discovery (CyberC) , pp. 42–45. IEEE, 2016.
Misra, Sudip, P. Venkata Krishna, Harshit Agarwal, Antriksh Saxena, and Mohammad S. Obaidat. “A learning automata based solution for preventing distributed denial of service in internet of things.” In 2011 International Conference on Internet of Things and 4th International Conference on Cyber, Physical and Social Computing , pp. 114–122. IEEE, 2011.
Sanchez, Fernando, and Zhenhai Duan. “A sender-centric approach to detecting phishing emails.” In 2012 International Conference on Cyber Security , pp. 32–39. IEEE, 2012.
Shitharth, S., and D. Prince Winston. “A novel IDS technique to detect DDoS and sniffers in smart grid.” In 2016 World Conference on Futuristic Trends in Research and Innovation for Social Welfare (Startup Conclave) , pp. 1–6. IEEE, 2016.
Sun, Jia-Hao, Tzung-Han Jeng, Chien-Chih Chen, Hsiu-Chuan Huang, and Kuo-Sen Chou. “MD-Miner: Behavior-Based Tracking of Network Traffic for Malware-Control Domain Detection.” In 2017 IEEE Third International Conference on Big Data Computing Service and Applications (BigDataService) , pp. 96–105. IEEE, 2017.
Velauthapillai, Thaneswaran, Aaron Harwood, and Shanika Karunasekera. “Global detection of flooding-based DDoS attacks using a cooperative overlay network.” In 2010 Fourth International Conference on Network and System Security , pp. 357–364. IEEE, 2010.
Sun, Cong, Jiao Liu, Xinpeng Xu, and Jianfeng Ma. “A privacy-preserving mutual authentication resisting DoS attacks in VANETs.” IEEE Access 5 (2017): 24012–24022.
Fan, Lejun, Yuanzhuo Wang, Xueqi Cheng, and Shuyuan Jin. “Privacy Theft Malware Detection with Privacy Petri Net.” In 2012 13th International Conference on Parallel and Distributed Computing, Applications and Technologies , pp. 195–200. IEEE, 2012.
Cui, Helei, Yajin Zhou, Cong Wang, Qi Li, and Kui Ren. “Towards Privacy-Preserving Malware Detection Systems for Android.” In 2018 IEEE 24th International Conference on Parallel and Distributed Systems (ICPADS) , pp. 545–552. IEEE, 2018.
Xu, Lei, Chunxiao Jiang, Nengqiang He, Zhu Han, and Abderrahim Benslimane. “Trust-based collaborative privacy management in online social networks.” IEEE Transactions on Information Forensics and Security 14, no. 1 (2018): 48–60.
Shitharth, S., and D. Prince Winston. “A comparative analysis between two countermeasure techniques to detect DDoS with sniffers in a SCADA network.” Procedia Technology 21 (2015): 179–186. ScienceDirect.
Spyridopoulos, Theodoros, G. Karanikas, Theodore Tryfonas, and Georgios Oikonomou. “A game theoretic defence framework against DoS/DDoS cyber attacks.” Computers & Security 38 (2013): 39–50. ScienceDirect.
Shon, Taeshik, and Jongsub Moon. “A hybrid machine learning approach to network anomaly detection.” Information Sciences 177, no. 18 (2007): 3799–3821. ScienceDirect.
Wang, Fei, Hailong Wang, Xiaofeng Wang, and Jinshu Su. “A new multistage approach to detect subtle DDoS attacks.” Mathematical and Computer Modelling 55, no. 1–2 (2012): 198–213. ScienceDirect.
Varshney, Gaurav, Manoj Misra, and Pradeep K. Atrey. “A phish detector using lightweight search features.” Computers & Security 62 (2016): 213–228. ScienceDirect.
Liu, Ting, Yanan Sun, Yang Liu, Yuhong Gui, Yucheng Zhao, Dai Wang, and Chao Shen. “Abnormal traffic-indexed state estimation: A cyber–physical fusion approach for smart grid attack detection.” Future Generation Computer Systems 49 (2015): 94–103. ScienceDirect.
Qiu, Yue, Maode Ma, and Shuo Chen. “An anonymous authentication scheme for multi-domain machine-to-machine communication in cyber-physical systems.” Computer Networks 129 (2017): 306–318. ScienceDirect.
Kumara, Ajay, and C. D. Jaidhar. “Automated multi-level malware detection system based on reconstructed semantic view of executables using machine learning techniques at VMM.” Future Generation Computer Systems 79 (2018): 431–446. ScienceDirect.
Zhao, David, Issa Traore, Bassam Sayed, Wei Lu, Sherif Saad, Ali Ghorbani, and Dan Garant. “Botnet detection based on traffic behavior analysis and flow intervals.” Computers & Security 39 (2013): 2–16. ScienceDirect.
Noor, Muzzamil, Haider Abbas, and Waleed Bin Shahid. “Countering cyber threats for industrial applications: An automated approach for malware evasion detection and analysis.” Journal of Network and Computer Applications 103 (2018): 249–261. ScienceDirect.
Huda, Shamsul, Suruz Miah, Mohammad Mehedi Hassan, Rafiqul Islam, John Yearwood, Majed Alrubaian, and Ahmad Almogren. “Defending unknown attacks on cyber-physical systems by semi-supervised approach and available unlabeled data.” Information Sciences 379 (2017): 211–228. ScienceDirect.
Alajeely, Majeed, Robin Doss, and Vicky Mak-Hau. “Defense against packet collusion attacks in opportunistic networks.” Computers & Security 65 (2017): 269–282. ScienceDirect.
Maciá-Fernández, Gabriel, Rafael A. Rodríguez-Gómez, and Jesús E. Díaz-Verdejo. “Defense techniques for low-rate DoS attacks against application servers.” Computer Networks 54, no. 15 (2010): 2711–2727. ScienceDirect.
Kiss, Istvan, Piroska Haller, and Adela Bereş. “Denial of Service attack Detection in case of Tennessee Eastman challenge process.” Procedia Technology 19 (2015): 835–841. ScienceDirect.
Abbaspour, Alireza, Kang K. Yen, Shirin Noei, and Arman Sargolzaei. “Detection of fault data injection attack on uav using adaptive neural network.” Procedia computer science 95 (2016): 193–200. ScienceDirect.
Stevanovic, Dusan, Natalija Vlajic, and Aijun An. “Detection of malicious and non-malicious website visitors using unsupervised neural network learning.” Applied Soft Computing 13, no. 1 (2013): 698–708. ScienceDirect.
Li, Beibei, Rongxing Lu, Wei Wang, and Kim-Kwang Raymond Choo. “Distributed host-based collaborative detection for false data injection attacks in smart grid cyber-physical system.” Journal of Parallel and Distributed Computing 103 (2017): 32–41. ScienceDirect.
Yu, Wei, Sriram Chellappan, Xun Wang, and Dong Xuan. “Peer-to-peer system-based active worm attacks: Modeling, analysis and defense.” Computer Communications 31, no. 17 (2008): 4005–4017. ScienceDirect.
Abdelhamid, Neda, Aladdin Ayesh, and Fadi Thabtah. “Phishing detection based associative classification data mining.” Expert Systems with Applications 41, no. 13 (2014): 5948–5959. ScienceDirect.
Alazab, Mamoun. “Profiling and classifying the behavior of malicious codes.” Journal of Systems and Software 100 (2015): 91–102. ScienceDirect.
Song, Jungsuk, Hiroki Takakura, Yasuo Okabe, and Koji Nakao. “Toward a more practical unsupervised anomaly detection system.” Information Sciences 231 (2013): 4–14. ScienceDirect.
Saini, Anil, Manoj Singh Gaur, Vijay Laxmi, and Mauro Conti. “Colluding browser extension attack on user privacy and its implication for web browsers.” Computers & Security 63 (2016): 14–28. ScienceDirect.
Choi, Sang‐soo, Jungsuk Song, Seokhun Kim, and Sookyun Kim. “A model of analyzing cyber threats trend and tracing potential attackers based on darknet traffic.” Security and Communication Networks 7, no. 10 (2014): 1612–1621. Wiley.
Rubio‐Hernan, Jose, Luca De Cicco, and Joaquin Garcia‐Alfaro. “Adaptive control‐theoretic detection of integrity attacks against cyber‐physical industrial systems.” Transactions on Emerging Telecommunications Technologies 29, no. 7 (2018): e3209. Wiley.
Zhang, Jian, Phillip Porras, and Johannes Ullrich. “Gaussian process learning for cyber‐attack early warning.” Statistical Analysis and Data Mining: The ASA Data Science Journal 3, no. 1 (2010): 56–68. Wiley.
Fan, Lejun, Yuanzhuo Wang, Xueqi Cheng, Jinming Li, and Shuyuan Jin. “Privacy theft malware multi‐process collaboration analysis.” Security and Communication Networks 8, no. 1 (2015): 51–67. Wiley.
Wu, Yu-Sung, Vinita Apte, Saurabh Bagchi, Sachin Garg, and Navjot Singh. “Intrusion detection in voice over IP environments.” International Journal of Information Security 8, no. 3 (2009): 153–172. Springer.
Deepa, G., P. Santhi Thilagam, Furqan Ahmed Khan, Amit Praseed, Alwyn R. Pais, and Nushafreen Palsetia. “Black-box detection of XQuery injection and parameter tampering vulnerabilities in web applications.” International Journal of Information Security 17, no. 1 (2018): 105–120. Springer.
Gowtham, R., and Ilango Krishnamurthi. “PhishTackle—a web services architecture for antiphishing.” Cluster computing 17, no. 3 (2014): 1051–1068. Springer.
Saha, Sujoy, Subrata Nandi, Rohit Verma, Satadal Sengupta, Kartikeya Singh, Vivek Sinha, and Sajal K. Das. “Design of efficient lightweight strategies to combat DoS attack in delay tolerant network routing.” Wireless Networks 24, no. 1 (2018): 173–194. Springer.
Gupta, Shashank, and B. B. Gupta. “XSS-SAFE: a server-side approach to detect and mitigate cross-site scripting (XSS) attacks in JavaScript code.” Arabian Journal for Science and Engineering 41, no. 3 (2016): 897–920. Springer.
Jain, Ankit Kumar, and Brij B. Gupta. “A novel approach to protect against phishing attacks at client side using auto-updated white-list.” EURASIP Journal on Information Security 2016, no. 1 (2016): 9. Springer.
Ahmad, Farhan Habib, Komal Batool, and Azhar Javed. “Detection of Privacy Threat by Peculiar Feature Extraction in Malwares to Combat Targeted Cyber Attacks.” In Advanced Computer and Communication Engineering Technology , pp. 1237–1247. Springer, Cham, 2016.
Saini, Anil, Manoj Singh Gaur, Vijay Laxmi, Tushar Singhal, and Mauro Conti. “Privacy leakage attacks in browsers by colluding extensions.” In International Conference on Information Systems Security , pp. 257–276. Springer, Cham, 2014.
Rights and permissions
Reprints and permissions
About this article
Humayun, M., Niazi, M., Jhanjhi, N. et al. Cyber Security Threats and Vulnerabilities: A Systematic Mapping Study. Arab J Sci Eng 45 , 3171–3189 (2020). https://doi.org/10.1007/s13369-019-04319-2
Download citation
Received : 09 May 2019
Accepted : 26 December 2019
Published : 06 January 2020
Issue Date : April 2020
DOI : https://doi.org/10.1007/s13369-019-04319-2
Share this article
Anyone you share the following link with will be able to read this content:
Sorry, a shareable link is not currently available for this article.
Provided by the Springer Nature SharedIt content-sharing initiative
- Cyber security
- Vulnerabilities
- Find a journal
- Publish with us
- Track your research
Information
- Author Services
Initiatives
You are accessing a machine-readable page. In order to be human-readable, please install an RSS reader.
All articles published by MDPI are made immediately available worldwide under an open access license. No special permission is required to reuse all or part of the article published by MDPI, including figures and tables. For articles published under an open access Creative Common CC BY license, any part of the article may be reused without permission provided that the original article is clearly cited. For more information, please refer to https://www.mdpi.com/openaccess .
Feature papers represent the most advanced research with significant potential for high impact in the field. A Feature Paper should be a substantial original Article that involves several techniques or approaches, provides an outlook for future research directions and describes possible research applications.
Feature papers are submitted upon individual invitation or recommendation by the scientific editors and must receive positive feedback from the reviewers.
Editor’s Choice articles are based on recommendations by the scientific editors of MDPI journals from around the world. Editors select a small number of articles recently published in the journal that they believe will be particularly interesting to readers, or important in the respective research area. The aim is to provide a snapshot of some of the most exciting work published in the various research areas of the journal.
Original Submission Date Received: .
- Active Journals
- Find a Journal
- Proceedings Series
- For Authors
- For Reviewers
- For Editors
- For Librarians
- For Publishers
- For Societies
- For Conference Organizers
- Open Access Policy
- Institutional Open Access Program
- Special Issues Guidelines
- Editorial Process
- Research and Publication Ethics
- Article Processing Charges
- Testimonials
- Preprints.org
- SciProfiles
- Encyclopedia
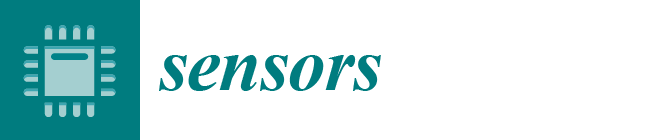
Article Menu

- Subscribe SciFeed
- Recommended Articles
- PubMed/Medline
- Google Scholar
- on Google Scholar
- Table of Contents
Find support for a specific problem in the support section of our website.
Please let us know what you think of our products and services.
Visit our dedicated information section to learn more about MDPI.
JSmol Viewer
A systematic literature review on cyber threat intelligence for organizational cybersecurity resilience.
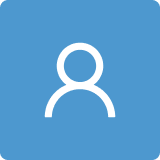
1. Introduction
2. materials and methods.
- The selected paper should focus on CTI in business organizations.
- The paper should be published between 2019 and 2023.
- All papers were journal articles or conference proceedings; any other publication type was excluded.
- Stage 1: The manuscript must be published in peer-reviewed journals or conference proceedings. Poster presentations, books, and blogs were left out due to quality concerns.
- Stage 2: The paper must be focused on the CTI domain that impacts organizations’ performance.
- Stage 3: The paper must be a case study, system application, or modeling implementation.
3.1. Detection Model
3.2. knowledge sharing and training, 4. discussion, 5. conclusions, author contributions, institutional review board statement, informed consent statement, data availability statement, acknowledgments, conflicts of interest.
- Lenka, A.; Goswami, M.; Singh, H.; Baskaran, H. Cybersecurity Disclosure and Corporate Reputation: Rising Popularity of Cybersecurity in the Business World. In Effective Cybersecurity Operations for Enterprise-Wide Systems ; IGI Global: Hershey, PA, USA, 2023; pp. 169–183. [ Google Scholar ]
- Kotsias, J.; Ahmad, A.; Scheepers, R. Adopting and integrating cyber-threat intelligence in a commercial organisation. Eur. J. Inf. Syst. 2023 , 32 , 35–51. [ Google Scholar ] [ CrossRef ]
- Gately, H. Russian Organised Crime and Ransomware as a Service: State Cultivated Cybercrime. Doctoral Dissertation, Macquarie University, Sydney, Australia, 2023. [ Google Scholar ]
- Abu, M.S.; Selamat, S.R.; Ariffin, A.; Yusof, R. CTI–issue and challenges. Indones. J. Electr. Eng. Comput. Sci. 2018 , 10 , 371–379. [ Google Scholar ]
- Webb, J.; Maynard, S.; Ahmad, A.; Shanks, G. Information security risk management: An intelligence-driven approach. Australas. J. Inf. Syst. 2014 , 18 , 391–404. [ Google Scholar ] [ CrossRef ]
- Webb, J.; Maynard, S.; Ahmad, A.; Shanks, G. Towards an intelligence-driven information security risk management process for organisations. In Proceedings of the ACIS 2013 Proceedings, 52, Niigata, Japan, 16–20 June 2013. [ Google Scholar ]
- Schlette, D.; Caselli, M.; Pernul, G. A comparative study on cyber threat intelligence: The security incident response perspective. IEEE Commun. Surv. Tutor. 2021 , 23 , 2525–2556. [ Google Scholar ] [ CrossRef ]
- Kitchenham, B.; Charters, S. Guidelines for Performing Systematic Literature Reviews in Software Engineering ; Technical Report, EBSE Technical Report EBSE-2007-0; Elsevier: London, UK, 2007. [ Google Scholar ]
- Page, M.J.; McKenzie, J.E.; Bossuyt, P.M.; Boutron, I.; Hoffmann, T.C.; Mulrow, C.D.; Moher, D. The PRISMA 2020 statement: An updated guideline for reporting systematic reviews. BMJ 2021 , 372 , n71. [ Google Scholar ] [ CrossRef ]
- Available online: https://ieeexplore.ieee.org/Xplore/home.jsp (accessed on 30 June 2023).
- Available online: https://dl.acm.org/ (accessed on 30 June 2023).
- Suryotrisongko, H.; Musashi, Y.; Tsuneda, A.; Sugitani, K. Robust botnet DGA detection: Blending XAI and OSINT for CTI sharing. IEEE Access 2022 , 10 , 34613–34624. [ Google Scholar ] [ CrossRef ]
- Moraliyage, H.; Sumanasena, V.; De Silva, D.; Nawaratne, R.; Sun, L.; Alahakoon, D. Multimodal classification of onion services for proactive CTI using explainable deep learning. IEEE Access 2022 , 10 , 56044–56056. [ Google Scholar ] [ CrossRef ]
- Irshad, E.; Siddiqui, A.B. Cyber threat attribution using unstructured reports in CTI. Egypt. Inform. J. 2023 , 24 , 43–59. [ Google Scholar ] [ CrossRef ]
- Zhang, H.; Shen, G.; Guo, C.; Cui, Y.; Jiang, C. Ex-action: Automatically extracting threat actions from CTI report based on multimodal learning. Secur. Commun. Netw. 2021 , 2021 , 1–12. [ Google Scholar ]
- Cha, J.; Singh, S.K.; Pan, Y.; Park, J.H. Blockchain-based CTI system architecture for sustainable computing. Sustainability 2020 , 12 , 6401. [ Google Scholar ] [ CrossRef ]
- Gong, S.; Lee, C. CTI framework for incident response in an energy cloud platform. Electronics 2021 , 10 , 239. [ Google Scholar ] [ CrossRef ]
- Ejaz, S.; Noor, U.; Rashid, Z. Visualizing Interesting Patterns in CTI Using Machine Learning Techniques. Cybern. Inf. Technol. 2022 , 22 , 96–113. [ Google Scholar ]
- Mendez Mena, D.; Yang, B. Decentralized actionable CTI for networks and the internet of things. IoT 2020 , 2 , 1–16. [ Google Scholar ] [ CrossRef ]
- Liu, J.; Yan, J.; Jiang, J.; He, Y.; Wang, X.; Jiang, Z.; Yang, P.; Li, N. TriCTI: An actionable CTI discovery system via trigger-enhanced neural network. Cybersecurity 2022 , 5 , 8. [ Google Scholar ] [ CrossRef ]
- Kiwia, D.; Dehghantanha, A.; Choo, K.K.R.; Slaughter, J. A cyber kill chain based taxonomy of banking Trojans for evolutionary computational intelligence. J. Comput. Sci. 2018 , 27 , 394–409. [ Google Scholar ] [ CrossRef ]
- Gong, S.; Lee, C. Blocis: Blockchain-based CTI sharing framework for sybil-resistance. Electronics 2020 , 9 , 521. [ Google Scholar ] [ CrossRef ]
- Borges Amaro, L.J.; Percilio Azevedo, B.W.; Lopes de Mendonca, F.L.; Giozza, W.F.; Albuquerque, R.D.O.; García Villalba, L.J. Methodological framework to collect, process, analyze and visualize CTI data. Appl. Sci. 2022 , 12 , 1205. [ Google Scholar ] [ CrossRef ]
- Al-Fawa’reh, M.; Al-Fayoumi, M.; Nashwan, S.; Fraihat, S. CTI using PCA-DNN model to detect abnormal network behavior. Egypt. Inform. J. 2022 , 23 , 173–185. [ Google Scholar ] [ CrossRef ]
- Sun, T.; Yang, P.; Li, M.; Liao, S. An automatic generation approach of the CTI records based on multi-source information fusion. Future Internet 2021 , 13 , 40. [ Google Scholar ] [ CrossRef ]
- Serketzis, N.; Katos, V.; Ilioudis, C.; Baltatzis, D.; Pangalos, G.J. Actionable threat intelligence for digital forensics readiness. Inf. Comput. Secur. 2019 , 27 , 273–291. [ Google Scholar ] [ CrossRef ]
- Raptis, G.E.; Katsini, C.; Alexakos, C.; Kalogeras, A.; Serpanos, D. CAVeCTIR: Matching CTI Reports on Connected and Autonomous Vehicles Using Machine Learning. Appl. Sci. 2022 , 12 , 11631. [ Google Scholar ] [ CrossRef ]
- Alsaedi, M.; Ghaleb, F.A.; Saeed, F.; Ahmad, J.; Alasli, M. CTI-based malicious url detection model using ensemble learning. Sensors 2022 , 22 , 3373. [ Google Scholar ] [ CrossRef ] [ PubMed ]
- Van Haastrecht, M.; Golpur, G.; Tzismadia, G.; Kab, R.; Priboi, C.; David, D.; Răcătăian, A.; Baumgartner, L.; Fricker, S.; Ruiz, J.F.; et al. A shared CTI solution for smes. Electronics 2021 , 10 , 2913. [ Google Scholar ] [ CrossRef ]
- Zhang, S.; Chen, P.; Bai, G.; Wang, S.; Zhang, M.; Li, S.; Zhao, C. An automatic assessment method of CTI combined with ATT&CK matrix. Wirel. Commun. Mob. Comput. 2022 , 7875910. [ Google Scholar ]
- Mishra, S.; Albarakati, A.; Sharma, S.K. CTI for IoT Using Machine Leamrning. Processes 2022 , 10 , 2673. [ Google Scholar ] [ CrossRef ]
- Chatziamanetoglou, D.; Rantos, K. Blockchain-Based CTI Sharing Using Proof-of-Quality Consensus. Secur. Commun. Netw. 2023 , 3303122. [ Google Scholar ]
- Li, Z.X.; Li, Y.J.; Liu, Y.W.; Liu, C.; Zhou, N.X. K-CTIAA: Automatic Analysis of CTI Based on a Knowledge Graph. Symmetry 2023 , 15 , 337. [ Google Scholar ] [ CrossRef ]
- Zhang, X.; Miao, X.; Xue, M. A Reputation-Based Approach Using Consortium Blockchain for CTI Sharing. Secur. Commun. Netw. 2022 , 7760509. [ Google Scholar ] [ CrossRef ]
- Serketzis, N.; Katos, V.; Ilioudis, C.; Baltatzis, D.; Pangalos, G. Improving forensic triage efficiency through CTI. Future Internet 2019 , 11 , 162. [ Google Scholar ] [ CrossRef ]
- Afzaliseresht, N.; Miao, Y.; Michalska, S.; Liu, Q.; Wang, H. From logs to stories: Human-centred data mining for CTI. IEEE Access 2020 , 8 , 19089–19099. [ Google Scholar ] [ CrossRef ]
- Riesco, R.; Larriva-Novo, X.; Villagrá, V.A. Cybersecurity threat intelligence knowledge exchange based on blockchain: Proposal of a new incentive model based on blockchain and Smart contracts to foster the cyber threat and risk intelligence exchange of information. Telecommun. Syst. 2020 , 73 , 259–288. [ Google Scholar ] [ CrossRef ]
- Rana, M.U.; Ellahi, O.; Alam, M.; Webber, J.L.; Mehbodniya, A.; Khan, S. Offensive Security: CTI Enrichment With Counterintelligence and Counterattack. IEEE Access 2022 , 10 , 108760–108774. [ Google Scholar ] [ CrossRef ]
- Samtani, S.; Li, W.; Benjamin, V.; Chen, H. Informing CTI through dark Web situational awareness: The AZSecure hacker assets portal. Digit. Threats Res. Pract. (DTRAP) 2021 , 2 , 1–10. [ Google Scholar ] [ CrossRef ]
- Koloveas, P.; Chantzios, T.; Tryfonopoulos, C.; Skiadopoulos, S. A crawler architecture for harvesting the clear, social, and dark web for IoT-related cyber-threat intelligence. In Proceedings of the 2019 IEEE World Congress on Services (SERVICES), Milan, Italy, 8–13 July 2019; Volume 2642, pp. 3–8. [ Google Scholar ]
- Basheer, R.; Alkhatib, B. Threats from the dark: A review over dark web investigation research for CTI. J. Comput. Netw. Commun. 2021 , 2021 , 1–21. [ Google Scholar ] [ CrossRef ]
- Mundt, M.; Baier, H. Threat-based Simulation of Data Exfiltration Towards Mitigating Multiple Ransomware Extortions. Digit. Threats Res. Pract. 2022 . [ Google Scholar ] [ CrossRef ]
- Sakellariou, G.; Fouliras, P.; Mavridis, I. SECDFAN: A CTI System for Discussion Forums Utilization. Eng 2023 , 4 , 615–634. [ Google Scholar ] [ CrossRef ]
- Sacher-Boldewin, D.; Leverett, E. The Intelligent Process Lifecycle of Active Cyber Defenders. Digit. Threats Res. Pract. (DTRAP) 2022 , 3 , 1–17. [ Google Scholar ] [ CrossRef ]
- Koloveas, P.; Chantzios, T.; Alevizopoulou, S.; Skiadopoulos, S.; Tryfonopoulos, C. Intime: A machine learning-based framework for gathering and leveraging web data to cyber-threat intelligence. Electronics 2021 , 10 , 818. [ Google Scholar ] [ CrossRef ]
- Riesco, R.; Villagrá, V.A. Leveraging CTI for a dynamic risk framework: Automation by using a semantic reasoner and a new combination of standards (STIX™, SWRL and OWL). Int. J. Inf. Secur. 2019 , 18 , 715–739. [ Google Scholar ] [ CrossRef ]
- Aljuhami, A.M.; Bamasoud, D.M. CTI in Risk Management. Int. J. Adv. Comput. Sci. Appl. 2021 , 12 , 156–164. [ Google Scholar ]
- Sakellariou, G.; Fouliras, P.; Mavridis, I.; Sarigiannidis, P. A reference model for CTI systems. Electronics 2022 , 11 , 1401. [ Google Scholar ] [ CrossRef ]
- Dulaunoy, A.; Huynen, J.L.; Thirion, A. Active and Passive Collection of SSH key material for CTI. Digit. Threats Res. Pract. (DTRAP) 2022 , 3 , 1–5. [ Google Scholar ] [ CrossRef ]
- Gao, P.; Liu, X.; Choi, E.; Soman, B.; Mishra, C.; Farris, K.; Song, D. A system for automated open-source threat intelligence gathering and management. In Proceedings of the 2021 International Conference on Management of Data, Xi’an, China, 20–25 June 2021; pp. 2716–2720. [ Google Scholar ]
- Al-Mohannadi, H.; Awan, I.; Al Hamar, J. Analysis of adversary activities using cloud-based web services to enhance CTI. Serv. Oriented Comput. Appl. 2020 , 14 , 175–187. [ Google Scholar ] [ CrossRef ]
- Sufi, F. A New Social Media-Driven CTI. Electronics 2023 , 12 , 1242. [ Google Scholar ] [ CrossRef ]
- Cristea, L.M. Risks Associated with Threats Related to Disruptive Technologies in the Current Financial Systems Context. Audit Financiar 2021 , 1 , 119–129. [ Google Scholar ] [ CrossRef ]
- Thach, N.N.; Hanh, H.T.; Huy, D.T.N.; Vu, Q.N. Technology quality management of the industry 4.0 and cybersecurity risk management on current banking activities in emerging markets-the case in Vietnam. Int. J. Qual. Res. 2021 , 15 , 840–856. [ Google Scholar ] [ CrossRef ]
- Tripodi, F.B. ReOpen demands as public health threat: A sociotechnical framework for understanding the stickiness of misinformation. Comput. Math. Organ. Theory 2022 , 28 , 321–334. [ Google Scholar ] [ CrossRef ]
- Odemis, M.; Yucel, C.; Koltuksuz, A. Detecting user behavior in CTI: Development of honeypsy system. Secur. Commun. Netw. arXiv 2022 , arXiv:2304.07411. [ Google Scholar ] [ CrossRef ]
- Vevera, A.V.; Cirnu, C.E.; Radulescu, C.Z. A Multi-Attribute Approach for CTI Product and Services Selection. Stud. Inform. Control 2022 , 31 , 13–23. [ Google Scholar ] [ CrossRef ]
- Du, L.; Fan, Y.; Zhang, L.; Wang, L.; Sun, T. A summary of the development of cyber security threat intelligence sharing. Int. J. Digit. Crime Forensics (IJDCF) 2020 , 12 , 54–67. [ Google Scholar ] [ CrossRef ]
- Westerlund, M. The emergence of deepfake technology: A review. Technol. Innov. Manag. Rev. 2019, 9. Available online: https://timreview.ca/article/1282 (accessed on 30 June 2023). [ CrossRef ]
- Sarhan, M.; Layeghy, S.; Moustafa, N.; Portmann, M. CTI sharing scheme based on federated learning for network intrusion detection. J. Netw. Syst. Manag. 2023 , 31 , 3. [ Google Scholar ] [ CrossRef ]
- Ramsdale, A.; Shiaeles, S.; Kolokotronis, N. A comparative analysis of cyber-threat intelligence sources, formats and languages. Electronics 2020 , 9 , 824. [ Google Scholar ] [ CrossRef ]
- Oosthoek, K.; Doerr, C. CTI: A product without a process? Int. J. Intell. CounterIntell. 2021 , 34 , 300–315. [ Google Scholar ] [ CrossRef ]
- de Melo e Silva, A.; Costa Gondim, J.J.; de Oliveira Albuquerque, R.; García Villalba, L.J. A methodology to evaluate standards and platforms within CTI. Future Internet 2020 , 12 , 108. [ Google Scholar ] [ CrossRef ]
- Al Obaidan, F.; Saeed, S. Digital transformation and cybersecurity challenges: A study of malware detection using machine learning techniques. In Handbook of Research on Advancing Cybersecurity for Digital Transformation ; IGI Global: Hershey, PA, USA, 2021; pp. 203–226. [ Google Scholar ]
- Saeed, S.; Bolívar, M.P.R.; Thurasamy, R. Pandemic, Lockdown, and Digital Transformation ; Springer International Publishing: Cham, Switzerland, 2021. [ Google Scholar ]
- Naeem, H.; Ullah, F.; Naeem, M.R.; Khalid, S.; Vasan, D.; Jabbar, S.; Saeed, S. Malware detection in industrial internet of things based on hybrid image visualization and deep learning model. Ad Hoc Netw. 2020 , 105 , 102154. [ Google Scholar ] [ CrossRef ]
- Mekala, S.H.; Baig, Z.; Anwar, A.; Zeadally, S. Cybersecurity for industrial IoT (IIoT): Threats, countermeasures, challenges and future directions. Comput. Commun. 2023 , 208 , 294–320. [ Google Scholar ] [ CrossRef ]
- Saeed, S. Education, Online Presence and Cybersecurity Implications: A Study of Information Security Practices of Computing Students in Saudi Arabia. Sustainability 2023 , 15 , 9426. [ Google Scholar ] [ CrossRef ]
- Saeed, S. Digital Workplaces and Information Security Behavior of Business Employees: An Empirical Study of Saudi Arabia. Sustainability 2023 , 15 , 6019. [ Google Scholar ] [ CrossRef ]
- Kont, K.R. Libraries and cyber security: The importance of the human factor in preventing cyber attacks. Libr. Hi Tech News 2023 . [ Google Scholar ] [ CrossRef ]
- Saeed, S. A Customer-Centric View of E-Commerce Security and Privacy. Appl. Sci. 2023 , 13 , 1020. [ Google Scholar ] [ CrossRef ]
- Gull, H.; Alabbad, D.A.; Saqib, M.; Iqbal, S.Z.; Nasir, T.; Saeed, S.; Almuhaideb, A.M. E-Commerce and Cybersecurity Challenges: Recent Advances and Future Trends. In Handbook of Research on Cybersecurity Issues and Challenges for Business and FinTech Applications ; IGI Global: Hershey, PA, USA, 2023; pp. 91–111. [ Google Scholar ]
Click here to enlarge figure
Ref. No. | Publication Year | Main Contribution | Technology/Method | Source of Data |
---|---|---|---|---|
[ ] | 2022 | The paper develops a model based on statistical characteristics to detect DGA-based traffic and explores the application of artificial intelligence/machine learning (AI/ML) in CTI. | Random forest algorithm (ML) | DNS query logs from a campus network |
[ ] | 2022 | The authors suggest a unique multimodal classification method based on understandable deep learning that categorizes onion services depending on their picture and text content. | Gradient-weighted Class Activation Mapping Convolutional Neural Network with a trained word-embedding algorithm with additive attention from Bahdanau | Dark web onion service images and texts |
[ ] | 2022 | The paper describes how unstructured CTI data may be used to gather cyber threat intelligence. The authors developed a novel model called “Attack2vec” that outperforms other models. The detailed feature set used in the model TTP tools, target company, virus, and application are all included. The usage of a comprehensive feature set improves classification outcomes. | Novel Attack2vec embedding model | Unstructured cyber threat intelligence reports |
[ ] | 2021 | In the research, an EX-Action framework for automatically extracting threat actions from natural language processing (NLP) technologies and a multimodal learning algorithm for creating CTI reports are developed. Utilizing a measure, the extracted activities were assessed for information completeness. The efficiency of the framework is compared to that of two state-of-the-art action extraction methods in terms of precision, recall, accuracy, and F1 score. In order to better defend against network threats, intelligence-based active defense sharing was improved. | NLP and multimodal learning algorithms | CTI reports consisting of sentences with complex structure |
[ ] | 2020 | The authors propose a blockchain-based intelligence on cyber threat system architecture for long-term computing to handle dependability, confidentiality, scalability, and sustainability challenges in collecting and analyzing data to identify potential threats. The model was proposed to work with multiple feeds, provide a trustworthy dataset, minimize network congestion, and stimulate participation by quantifying companies’ contributions. Additionally, through experimental study, the proposed model’s success was assessed using various metrics, including dependability, privacy, scalability, and sustainability. | NLP and multimodal learning algorithms | IP information, domains, URLs, network artifacts, and aggregation |
[ ] | 2022 | In order to build a cyber threat intelligence-based detection model, the article addresses a study that intends to improve the identification of hazardous URLs by applying two-stage ensemble learning. The suggested approach outperformed detection methods from prior research, improving accuracy by 7.8% and reducing false-positive rates by 6.7% when compared to conventional URL-based models. | Customized algorithm | Scholarly journal- malicious URL |
[ ] | 2023 | The report offers a systematic evaluation that contends that SMEs may profit from threat information-sharing platforms like MISP if shared intelligence is transformed into useful insights. In order to evaluate MISP data, rank cybersecurity hazards for SMEs, and provide personalized advice, a prototype application is developed. | - | - |
[ ] | 2022 | The authors of this paper used automatic classification based on feature extraction and integrated ATT&CK to identify attack methods associated with IOC. | CTI systems modeling | Community-sourced threat intelligence and open-source intelligence |
[ ] | 2022 | This paper focuses on potential network attack identification, and countermeasures are recommended utilizing simulated data. Anomalies in IoT networks are detected using message queuing telemetry transport (MQTT) and machine-learning algorithms. | Mixed methods using quantitative and qualitative approaches | Al-Kasassbeh dataset |
[ ] | 2023 | In this paper, the authors underscore the significance of acquiring advanced and in-depth information about cyber threats in Saudi universities. | Probabilistic approach | GitHub repository |
[ ] | 2023 | In this paper, the authors propose an automatic CTI analysis method called K-CTIAA to address the challenges of analyzing these threats. K-CTIAA pre-trained algorithms and knowledge graphs were used to obtain threat actions from unorganized CTI and achieved high automatic threat intelligence analysis performance. | K-CTIAA/BERT analysis | An open-source APT |
[ ] | 2018 | The authors of this paper present a collaborative cyber threat intelligence-sharing scheme to allow many enterprises to collaborate on the design, training, and evaluation of a powerful ML-based network intrusion detection system. | Consortium blockchain | CTI data |
[ ] | 2019 | The paper proposes a DFR model that combines CTI and forensic preparedness to help increase Digital Forensics Readiness and minimize the time and expense of response to incidents and investigations. The model achieved high accuracy, precision, and recall rates while reducing the amount of information that investigators must study, demonstrating the effectiveness of combining CTI and digital forensics processes. | Digital Forensic Readiness | Local log dataset |
[ ] | 2022 | This paper presents a unique technique for detecting commonalities amongst CTI reports describing harmful actions identified on CAVs. This unique model achieved 96% accuracy, 96.5% precision, 95.58% recall, and 95.75% F1 score, respectively. | Decision Tree, Random Forest, and Support Vector Machine are examples of machine-learning models. | Reports from the scientific community, security manufacturers, and a programmable Google search engine |
[ ] | 2020 | In this study, the authors created a blockchain-based CTI framework that can swiftly identify and reject false data in order to defend against a Sybil attack and increase confidence in the source and content of data. The suggested architecture collects CTI via a process certified by smart contracts and stores data meta-information on a blockchain network. | Blockchain-based open architecture for exchanging cyber threat intelligence (BLOCIS) | Utilizes open-sourced intelligence as a route for data acquisition |
[ ] | 2022 | The authors of this research created a platform to address situations in which a cybersecurity analyst may import threat data, analyze it, and generate a timeline to gain insight and properly contextualize a threat. The results demonstrate that knowledge is facilitated about the environment in which the threats are placed, making vulnerability mitigation more effective. | Timeline representation of danger details and analytical data insights | Multiple sources |
[ ] | 2021 | The methodology used in this study examines potential danger indications gathered by smart meters and proposes a method for acquiring cyber threat information that focuses on the energy cloud. Through a mechanism for exchanging and distributing knowledge about cyber threats across the Advanced Metering Infrastructure (AMI) and cloud tiers, this research also provides a method for quickly deploying a security framework to a significant energy cloud architecture. | A setting that models an attacker model and an energy cloud system | Every second, threats employing energy item data and 20,480 IoC data instances are broadcast from a prosumer device. |
[ ] | 2021 | This paper enhances IDS detection mechanisms by incorporating novel features for identifying threats based on two assumptions related to handling zero-day attacks with constrained computing power and resources, as well as a comprehensive approach for detection by combining DNN and principal component analysis (PCA) to enhance security and performance. | The accuracy rate of DNN using PCA and model was 98%. | Initial packet capture (PCAP) is a common method for collecting network traffic data. |
[ ] | 2022 | In this paper, the authors highlight the use of effective visualizations for CTI. A preliminary analysis of the data of CTI reports was carried out to unearth and depict relevant cyber threat trends, allowing security professionals to reduce vulnerabilities and proactively forecast cyber-attacks in their networks. | Based on machine-learning approaches, a system for visually analyzing CTI data is developed. | TTP dataset |
[ ] | 2021 | This article demonstrates a proof of concept (PoC) using blockchain technology to secure private networks, Internet of Things (IoT) devices, and internet service providers (ISPs). The findings back up the idea of decentralized cyber threat intelligence-sharing networks that are capable of protecting several stakeholders. | A proof of concept (PoC) using blockchain technology to secure home networks, Internet of Things (IoT) devices, and internet service providers | Data collected at the ISP and the customer premises, equipment (CPE) routers |
[ ] | 2022 | This paper analyzes CTI VirusTotal (VT) large-scale field data. The authors discovered that the threat intelligence given by VT is inefficient, and the proposed method can improve CTI. | TriCTI is a trigger-enhanced system that discovers actionable threat intelligence, conveying a fuller context of IOCs by disclosing their campaign phases. | DS-1 (2013–2020) and DS-2 (2021) datasets are used. |
[ ] | 2021 | This article offers an automated technique to produce CTI records by merging NLP, neural networks, and cyber threat intelligence expertise. | A method for utilizing cybersecurity threat intelligence data together with NLP, machine learning, and CTI records is automatically generated based on multi-type OSTIPs (GCO). | GCO was performed on the collected OSTIPs, yielding 24,835 articles published between 2010 and 2019. |
[ ] | 2018 | In this paper, the authors highlight that CTI has become a common practice for preventing or detecting security incidents, especially in the digital forensics (DF) domain. | Creation of a unique methodology for boosting the efficacy of current digital forensic readiness (DFR) schemes by exploiting cyber threat information-sharing capabilities | Local IoC database |
[ ] | 2017 | This article presents a banking Trojan feature taxonomy based on a cyber death chain. This danger intelligence-based taxonomy, which provides stage-by-stage operational knowledge of a cyber attack, can help security practitioners as well as aid in the construction of evolving artificial intelligence for Trojan detection and mitigation strategies. | A taxonomy that provides operational knowledge of a cyber-attack stage by stage | 127 financial transactions from the real world |
Ref. No. | Publication Year | Main Contribution | Technology/Method | Source of Data |
---|---|---|---|---|
[ ] | 2019 | Using storytelling approaches, the authors suggest a mechanism that creates insights into the natural language from security data. | Log-driven storytelling model using narrative techniques and human-centered data mining | Security logs |
[ ] | 2022 | The authors use novel methods for circumventing virtual private networks (VPN) and additional security measures to gather accurate source information. | The counterintelligence and counterattack approach employs an Elastic Sky X Integrated (ESXI) server in a data center, public and private pathways for accessing attacker logs, Cowrie and Windows honeypots with numerous open ports like Secure Shell (SSH) to confuse attackers, and a log server to store logs. | Attacker logs and Cowrie and Windows honeypots |
[ ] | 2022 | The main benefits of the system described in this article include the fact that it allows for the storage and retrieval of SSH connections used to collect historical forensic artifacts and provides a Representational State Transfer (REST) API to aid in incident investigations and infrastructure monitoring. | The program maintains fingerprints in a Redis-compatible backend and offers an API that uses REST to put information into a datastore and obtain signatures. | Server banners, key types, and IP addresses |
[ ] | 2021 | The primary goal of this study was to increase awareness of situations in cybersecurity by offering greater active inspection of possible dangers that are developing in cyberspace before an assault. The AZSecure Hacker Assets Portal (HAP) gathers, analyzes, and publishes on dark web data sources to provide a unique view of hackers and associated cybercriminal assets while adding CTI insights to increase awareness of the situation. | CTI, text, and data mining (key exchange algorithms, encryption algorithms, and message authentication code algorithms) | Dark web cyber-attack tools |
[ ] | 2022 | The primary goal of this work was to offer an idea to accomplish targeted automated data exfiltration mitigation along with a preliminary assessment. The authors propose using international approaches and the MITRE ATT&CK framework to automatically recognize and simulate the most relevant data exfiltration risks, strictly focusing on mitigating these threats. | Mapped Building Security in Maturity Model (BSIMM) and threat-based security concepts | Automatic procedures based on the framework would be network traffic and persistent data. |
[ ] | 2021 | This article describes SecurityKG, a system proposed to collect and manage open-source security threat intelligence (OSCTI) information. SecurityKG extracts high-fidelity information about threat behaviors using AI and machine learning and builds a security-knowing graph. | Artificial Intelligence and natural language processing techniques | OSCTI reports |
[ ] | 2022 | The major goal of this study was to demonstrate how false-positive occurrences can be identified methodically for all services and the ways in which this data can be utilized to suggest areas for development. The study also focuses on recognizing and recording issues that arise while detecting and analyzing vulnerabilities and whenever the security operations center (SOC) attempts to add an inventory source for continuous monitoring. | Systematic categorization of possible failure states and building these into existing security workflows and tools | False positives, incorrect states, and cyber defense operations issues |
[ ] | 2019 | The major goal of this work was to offer a novel CTI-sharing model that encourages all participants at all levels to communicate important information in real-time. The suggested solution uses the blockchain and guidelines such as Generalized Threat Information Transfer and World Wide Web Consortium (W3C) semantics web standards to allow for a workspace of information linked to behavioral threat intelligence patterns. This will aid in characterizing strategies, approaches, and processes while also rewarding CTI sharing via an Ethereum-based smart contract marketplace. | Ethereum blockchain smart contract marketplace | CTI data |
[ ] | 2021 | This article presents IN TIME, a machine-learning-based architecture that provides a complete platform for managing cyber threat intelligence. The framework may be used by security analysts to swiftly find, acquire, assess, extract, integrate, and distribute information on cyberthreats from diverse online sources. It also supports the whole threat lifecycle through open standards and user-friendly interfaces, allowing for the quick deployment of data collection services and the automatic grading of acquired information. | Machine-learning-based framework called IN TIME | Internet sources |
[ ] | 2019 | The article emphasizes the importance of risk management in organizations and the challenges they face in managing risks. It proposes a new architecture for dynamic risk assessment and management, which enables real-time risk management while ensuring ease of adoption by incorporating a mix of standards. The article demonstrates the effectiveness of the proposed framework in supporting decision making across different organizational levels using a leading cybersecurity organization. | Protegé | Semantic data model |
[ ] | 2023 | The paper covers the possible damage that cyber-attacks may do to the world economy and the necessity of comprehending the danger level to modify cybersecurity measures at various levels. It suggests a modern technique for analyzing the context of social media posts on cyber-attacks and electronic warfare using AI and NLP. Seventy-five daily cyber threat indices for six countries are produced due to the technique’s validation utilizing real-time Twitter feeds. | Twitter feed, AI, and NLP | Social media |
[ ] | 2021 | The article highlights the vulnerability of small- and medium-sized enterprises (SMEs) to cyber-attacks due to their lack of resources. It discusses the need to share CTI to assist SMEs in cybersecurity defense. However, existing shared intelligence approaches do not sufficiently meet SME requirements, and further investigation is necessary to enhance SME cybersecurity resilience. A prototype application was developed to process MISP data, prioritize cybersecurity threats for SMEs, and give customized recommendations. Future evaluations will refine the application and help SMEs to defend themselves against cyber-attacks more effectively. | The body of studies on intelligence draws on qualitative approaches used in social science. research. | Live social media feeds |
[ ] | 2021 | The paper explores how CTI can reduce cyber risks in Saudi universities by improving risk management. It examines CTI concepts, challenges, and risk management practices in higher education. It concludes that integrating CTI into risk management can enhance defenders’ capacity to mitigate the risk of cyber threats. | - | - |
[ ] | 2022 | In order to aid in the design of CTI systems, this study emphasizes a number of important CTI ideas and an eight-layer CTI reference model. A powerful ML-based network intrusion detection system may be designed, trained, and evaluated by several enterprises working together through a collaborative cyber threat intelligence-sharing scheme. | CTI model design methodology | Existing CTI platforms |
[ ] | 2023 | The authors of this paper propose a semantic schema for organizing collected data. The SECDFAN system is introduced as a comprehensive approach for creating CTI products by analyzing forum content. | SECDFAN’s CTI reference architecture | Repository data |
[ ] | 2023 | The authors present a strategy for collaborative cyber threat information sharing to allow several enterprises to collaborate on creating, training, and assessing an effective ML-based intrusion detection system. | Federated, centralized, and localized learning scenarios | Local data |
[ ] | 2020 | In this paper, researchers explore ways to assist SMEs in their cybersecurity defense through CTI sharing. Existing shared intelligence approaches do not meet SMEs’ requirements; further investigation is needed to enhance cybersecurity resilience. | Methods and platforms for sharing CTI | CTI data |
[ ] | 2021 | The article discusses the significance of analyzing dark web content for CTI to deter cybercrimes and understand criminal behavior. | The life cycle of CTI | Internal network data, external threat feeds, open-source intelligence (OSINT), human intelligence |
[ ] | 2020 | In this paper, the authors emphasize that shared intelligence needs to be translated into actionable insights to be effective. A prototype application is created to process MISP data, prioritize cybersecurity threats, and give customized recommendations. | 5W3H method | Open-source TI platform |
[ ] | 2022 | The writers of this work want to highlight the nuances of hackers’ personalities and competence to assist defense specialists of targeted institutions in developing cybersecurity tactics based on the hackers’ modus operandi. | The relationship between a hacker’s behavior/logs in a server and the hacker’s personality, skills, and psychology | Logs |
[ ] | 2022 | A case study is investigated based on user assessments and reviews of security threat intelligence providers. The effect of VIseKriterijumska Optimizacija I Kompromisno Resenje (VIKOR) user parameter modification on CTI provider ranking is investigated. The suggested approach is a tool to help security program executives decide which CTI providers to use. It also assists CTI service providers in improving the quality of their goods and services. | Support tools for security program executives confronted with the issue of which CTI providers to choose. It also assists CTI service providers in improving the quality of their goods and services. | Security threat intelligence internet platform |
[ ] | 2019 | To enable active threat intelligence, this study provides a threat intelligence framework for evaluating attack data acquired via a cloud-based web service. | Analyzing attack data acquired via cloud-based web services to provide active threat intelligence | Data cloud |
[ ] | 2020 | The significance and value of threat intelligence are covered in the opening paragraphs of this article. A threat intelligence analysis model is then presented. Next, the study compiles and organizes the suppliers of threat intelligence as well as the threat intelligence-sharing policies. | The existing threat intelligence-sharing method has several major flaws. | - |
[ ] | 2021 | The writers concentrate on the task of acquiring information in this study. They demonstrate a unique crawling architecture for openly gathering data from clear web security websites, social web security forums, and dark web hacker forums/marketplaces. The suggested architecture divides data collection into two phases. | Information-gathering task | - |
[ ] | 2019 | The research includes a complete assessment of deep fakes and economic potential for cybersecurity and AI businesses battling multimedia fraud and fake news. | A comprehensive review of deep fakes | - |
[ ] | 2021 | This article focuses on integrating, comparing, and examining disruptive technologies’ effects, presenting security threats and occurrences, and building risk management strategies. | Design measures to manage risk | _ |
[ ] | 2020 | As the important financial sector adapts to greater autonomy, there is a risk of increasing vulnerabilities and amplification of the impact of cybersecurity threats. As a result, companies must possess the flexibility to invest in ICT and cybersecurity expenditures to adjust to unanticipated conditions swiftly and efficiently for improved technology quality management. | Risk management plans | - |
[ ] | 2022 | This study provides a deeper knowledge of the main stories being shared by ReOpen members as well as the sources they use to back up their opinions. Members offered public safety solutions based on individualism and self-inquiry while trying to reinterpret data to reduce the danger of COVID. Members questioned the veracity of the fact checkers when the platform tried to uncover problematic content, highlighting the intimate connection between misinformation and epistemology. | It clarifies the primary narratives circulating among ReOpen members and the material they used to back up their claims. | Facebook groups |
The statements, opinions and data contained in all publications are solely those of the individual author(s) and contributor(s) and not of MDPI and/or the editor(s). MDPI and/or the editor(s) disclaim responsibility for any injury to people or property resulting from any ideas, methods, instructions or products referred to in the content. |
Share and Cite
Saeed, S.; Suayyid, S.A.; Al-Ghamdi, M.S.; Al-Muhaisen, H.; Almuhaideb, A.M. A Systematic Literature Review on Cyber Threat Intelligence for Organizational Cybersecurity Resilience. Sensors 2023 , 23 , 7273. https://doi.org/10.3390/s23167273
Saeed S, Suayyid SA, Al-Ghamdi MS, Al-Muhaisen H, Almuhaideb AM. A Systematic Literature Review on Cyber Threat Intelligence for Organizational Cybersecurity Resilience. Sensors . 2023; 23(16):7273. https://doi.org/10.3390/s23167273
Saeed, Saqib, Sarah A. Suayyid, Manal S. Al-Ghamdi, Hayfa Al-Muhaisen, and Abdullah M. Almuhaideb. 2023. "A Systematic Literature Review on Cyber Threat Intelligence for Organizational Cybersecurity Resilience" Sensors 23, no. 16: 7273. https://doi.org/10.3390/s23167273
Article Metrics
Article access statistics, further information, mdpi initiatives, follow mdpi.
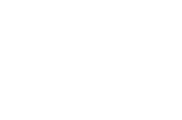
Subscribe to receive issue release notifications and newsletters from MDPI journals
REVIEW article
Phishing attacks: a recent comprehensive study and a new anatomy.

- Cardiff School of Technologies, Cardiff Metropolitan University, Cardiff, United Kingdom
With the significant growth of internet usage, people increasingly share their personal information online. As a result, an enormous amount of personal information and financial transactions become vulnerable to cybercriminals. Phishing is an example of a highly effective form of cybercrime that enables criminals to deceive users and steal important data. Since the first reported phishing attack in 1990, it has been evolved into a more sophisticated attack vector. At present, phishing is considered one of the most frequent examples of fraud activity on the Internet. Phishing attacks can lead to severe losses for their victims including sensitive information, identity theft, companies, and government secrets. This article aims to evaluate these attacks by identifying the current state of phishing and reviewing existing phishing techniques. Studies have classified phishing attacks according to fundamental phishing mechanisms and countermeasures discarding the importance of the end-to-end lifecycle of phishing. This article proposes a new detailed anatomy of phishing which involves attack phases, attacker’s types, vulnerabilities, threats, targets, attack mediums, and attacking techniques. Moreover, the proposed anatomy will help readers understand the process lifecycle of a phishing attack which in turn will increase the awareness of these phishing attacks and the techniques being used; also, it helps in developing a holistic anti-phishing system. Furthermore, some precautionary countermeasures are investigated, and new strategies are suggested.
Introduction
The digital world is rapidly expanding and evolving, and likewise, as are cybercriminals who have relied on the illegal use of digital assets—especially personal information—for inflicting damage to individuals. One of the most threatening crimes of all internet users is that of ‘identity theft’ ( Ramanathan and Wechsler, 2012 ) which is defined as impersonating the person’s identity to steal and use their personal information (i.e., bank details, social security number, or credit card numbers, etc.) by an attacker for the individuals’ own gain not just for stealing money but also for committing other crimes ( Arachchilage and Love, 2014 ). Cyber criminals have also developed their methods for stealing their information, but social-engineering-based attacks remain their favorite approach. One of the social engineering crimes that allow the attacker to perform identity theft is called a phishing attack. Phishing has been one of the biggest concerns as many internet users fall victim to it. It is a social engineering attack wherein a phisher attempts to lure the users to obtain their sensitive information by illegally utilizing a public or trustworthy organization in an automated pattern so that the internet user trusts the message, and reveals the victim’s sensitive information to the attacker ( Jakobsson and Myers, 2006 ). In phishing attacks, phishers use social engineering techniques to redirect users to malicious websites after receiving an email and following an embedded link ( Gupta et al., 2015 ). Alternatively, attackers could exploit other mediums to execute their attacks such as Voice over IP (VoIP), Short Message Service (SMS) and, Instant Messaging (IM) ( Gupta et al., 2015 ). Phishers have also turned from sending mass-email messages, which target unspecified victims, into more selective phishing by sending their emails to specific victims, a technique called “spear-phishing.”
Cybercriminals usually exploit users with a lack of digital/cyber ethics or who are poorly trained in addition to technical vulnerabilities to reach their goals. Susceptibility to phishing varies between individuals according to their attributes and awareness level, therefore, in most attacks, phishers exploit human nature for hacking, instead of utilising sophisticated technologies. Even though the weakness in the information security chain is attributed to humans more than the technology, there is a lack of understanding about which ring in this chain is first penetrated. Studies found that certain personal characteristics make some persons more receptive to various lures ( Iuga et al., 2016 ; Ovelgönne et al., 2017 ; Crane, 2019 ). For example, individuals who usually obey authorities more than others are more likely to fall victim to a Business Email Compromise (BEC) that is pretending to be from a financial institution and requests immediate action by seeing it as a legitimate email ( Barracuda, 2020 ). Greediness is another human weakness that could be used by an attacker, for example, emails that offering either great discounts, free gift cards, and others ( Workman, 2008 ).
Various channels are used by the attacker to lure the victim through a scam or through an indirect manner to deliver a payload for gaining sensitive and personal information from the victim ( Ollmann, 2004 ). However, phishing attacks have already led to damaging losses and could affect the victim not only through a financial context but could also have other serious consequences such as loss of reputation, or compromise of national security ( Ollmann, 2004 ; Herley and Florêncio, 2008 ). Cybercrime damages have been expected to cost the world $6 trillion annually by 2021, up from $3 trillion in 2015 according to Cybersecurity Ventures ( Morgan, 2019 ). Phishing attacks are the most common type of cybersecurity breaches as stated by the official statistics from the cybersecurity breaches survey 2020 in the United Kingdom ( GOV.UK, 2020 ). Although these attacks affect organizations and individuals alike, the loss for the organizations is significant, which includes the cost for recovery, the loss of reputation, fines from information laws/regulations, and reduced productivity ( Medvet et al., 2008 ).
Phishing is a field of study that merges social psychology, technical systems, security subjects, and politics. Phishing attacks are more prevalent: a recent study ( Proofpoint, 2020 ) found that nearly 90% of organizations faced targeted phishing attacks in 2019. From which 88% experienced spear-phishing attacks, 83% faced voice phishing (Vishing), 86% dealt with social media attacks, 84% reported SMS/text phishing (SMishing), and 81% reported malicious USB drops. The 2018 Proofpoint 1 annual report ( Proofpoint, 2019a ) has stated that phishing attacks jumped from 76% in 2017 to 83% in 2018, where all phishing types happened more frequently than in 2017. The number of phishing attacks identified in the second quarter of 2019 was notably higher than the number recorded in the previous three quarters. While in the first quarter of 2020, this number was higher than it was in the previous one according to a report from Anti-Phishing Working Group (APWG 2 ) ( APWG, 2018 ) which confirms that phishing attacks are on the rise. These findings have shown that phishing attacks have increased continuously in recent years and have become more sophisticated and have gained more attention from cyber researchers and developers to detect and mitigate their impact. This article aims to determine the severity of the phishing problem by providing detailed insights into the phishing phenomenon in terms of phishing definitions, current statistics, anatomy, and potential countermeasures.
The rest of the article is organized as follows. Phishing Definitions provides a number of phishing definitions as well as some real-world examples of phishing. The evolution and development of phishing attacks are discussed in Developing a Phishing Campaign . What Attributes Make Some People More Susceptible to Phishing Attacks Than Others explores the susceptibility to these attacks. The proposed phishing anatomy and types of phishing attacks are elaborated in Proposed Phishing Anatomy . In Countermeasures , various anti-phishing countermeasures are discussed. The conclusions of this study are drawn in Conclusion .
Phishing Definitions
Various definitions for the term “phishing” have been proposed and discussed by experts, researchers, and cybersecurity institutions. Although there is no established definition for the term “phishing” due to its continuous evolution, this term has been defined in numerous ways based on its use and context. The process of tricking the recipient to take the attacker’s desired action is considered the de facto definition of phishing attacks in general. Some definitions name websites as the only possible medium to conduct attacks. The study ( Merwe et al., 2005 , p. 1) defines phishing as “a fraudulent activity that involves the creation of a replica of an existing web page to fool a user into submitting personal, financial, or password data.” The above definition describes phishing as an attempt to scam the user into revealing sensitive information such as bank details and credit card numbers, by sending malicious links to the user that leads to the fake web establishment. Others name emails as the only attack vector. For instance, PishTank (2006) defines phishing as “a fraudulent attempt, usually made through email, to steal your personal information.” A description for phishing stated by ( Kirda and Kruegel, 2005 , p.1) defines phishing as “a form of online identity theft that aims to steal sensitive information such as online banking passwords and credit card information from users.” Some definitions highlight the usage of combined social and technical skills. For instance, APWG defines phishing as “a criminal mechanism employing both social engineering and technical subterfuge to steal consumers’ personal identity data and financial account credentials” ( APWG, 2018 , p. 1). Moreover, the definition from the United States Computer Emergency Readiness Team (US-CERT) states phishing as “a form of social engineering that uses email or malicious websites (among other channels) to solicit personal information from an individual or company by posing as a trustworthy organization or entity” ( CISA, 2018 ). A detailed definition has been presented in ( Jakobsson and Myers, 2006 , p. 1), which describes phishing as “a form of social engineering in which an attacker, also known as a phisher, attempts to fraudulently retrieve legitimate users’ confidential or sensitive credentials by mimicking electronic communications from a trustworthy or public organization in an automated fashion. Such communications are most frequently done through emails that direct users to fraudulent websites that in turn collect the credentials in question.”
In order to understand the anatomy of the phishing attack, there is a necessity for a clear and detailed definition that underpins previous existent definitions. Since a phishing attack constitutes a mix of technical and social engineering tactics, a new definition (i.e., Anatomy) has been proposed in this article, which describes the complete process of a phishing attack. This provides a better understanding for the readers as it covers phishing attacks in depth from a range of perspectives. Various angles and this might help beginner readers or researchers in this field. To this end, we define phishing as a socio-technical attack, in which the attacker targets specific valuables by exploiting an existing vulnerability to pass a specific threat via a selected medium into the victim’s system, utilizing social engineering tricks or some other techniques to convince the victim into taking a specific action that causes various types of damages.
Figure 1 depicts the general process flow for a phishing attack that contains four phases; these phases are elaborated in Proposed Phishing Anatomy . However, as shown in Figure 1 , in most attacks, the phishing process is initiated by gathering information about the target. Then the phisher decides which attack method is to be used in the attack as initial steps within the planning phase. The second phase is the preparation phase, in which the phisher starts to search for vulnerabilities through which he could trap the victim. The phisher conducts his attack in the third phase and waits for a response from the victim. In turn, the attacker could collect the spoils in the valuables acquisition phase, which is the last step in the phishing process. To elaborate the above phishing process using an example, an attacker may send a fraudulent email to an internet user pretending to be from the victim’s bank, requesting the user to confirm the bank account details, or else the account may be suspended. The user may think this email is legitimate since it uses the same graphic elements, trademarks, and colors of their legitimate bank. Submitted information will then be directly transmitted to the phisher who will use it for different malicious purposes such as money withdrawal, blackmailing, or committing further frauds.
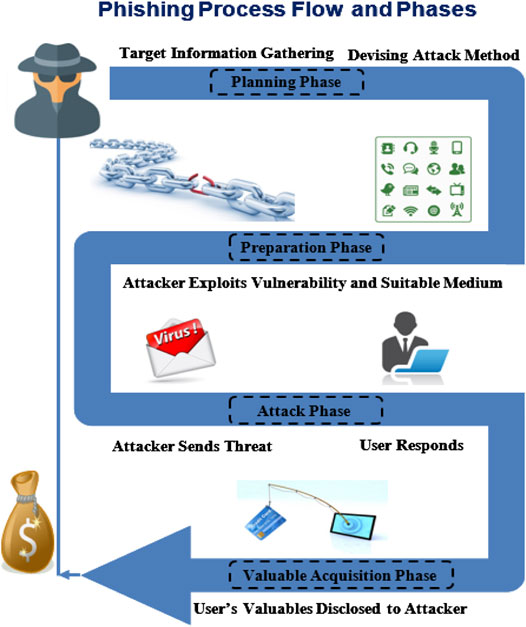
FIGURE 1 . General phishing attack process.
Real-World Phishing Examples
Some real-world examples of phishing attacks are discussed in this section to present the complexity of some recent phishing attacks. Figure 2 shows the screenshot of a suspicious phishing email that passed a University’s spam filters and reached the recipient mailbox. As shown in Figure 2 , the phisher uses the sense of importance or urgency in the subject through the word ‘important,’ so that the email can trigger a psychological reaction in the user to prompt them into clicking the button “View message.” The email contains a suspicious embedded button, indeed, when hovering over this embedded button, it does not match with Uniform Resource Locator (URL) in the status bar. Another clue in this example is that the sender's address is questionable and not known to the receiver. Clicking on the fake attachment button will result in either installation of a virus or worm onto the computer or handing over the user’s credentials by redirecting the victim onto a fake login page.
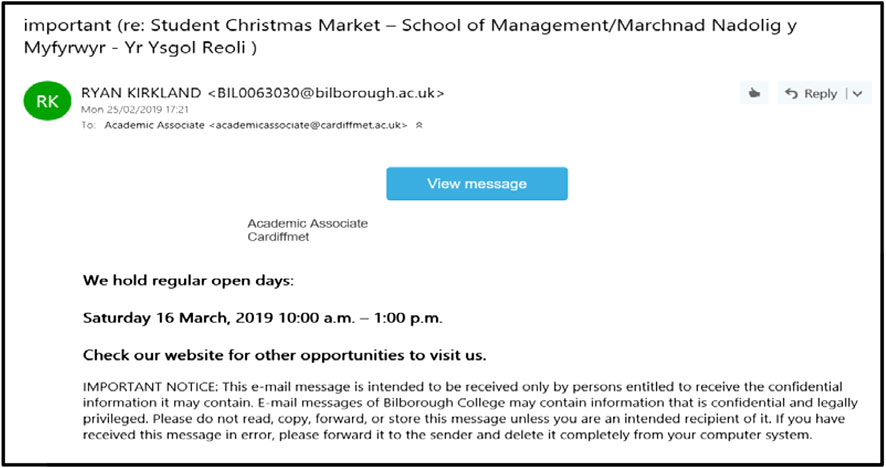
FIGURE 2 . Screenshot of a real suspicious phishing email received by the authors’ institution in February 2019.
More recently, phishers take advantage of the Coronavirus pandemic (COVID-19) to fool their prey. Many Coronavirus-themed scam messages sent by attackers exploited people’s fear of contracting COVID-19 and urgency to look for information related to Coronavirus (e.g., some of these attacks are related to Personal Protective Equipment (PPE) such as facemasks), the WHO stated that COVID-19 has created an Infodemic which is favorable for phishers ( Hewage, 2020 ). Cybercriminals also lured people to open attachments claiming that it contains information about people with Coronavirus within the local area.
Figure 3 shows an example of a phishing e-mail where the attacker claimed to be the recipient’s neighbor sending a message in which they pretended to be dying from the virus and threatening to infect the victim unless a ransom was paid ( Ksepersky, 2020 ).
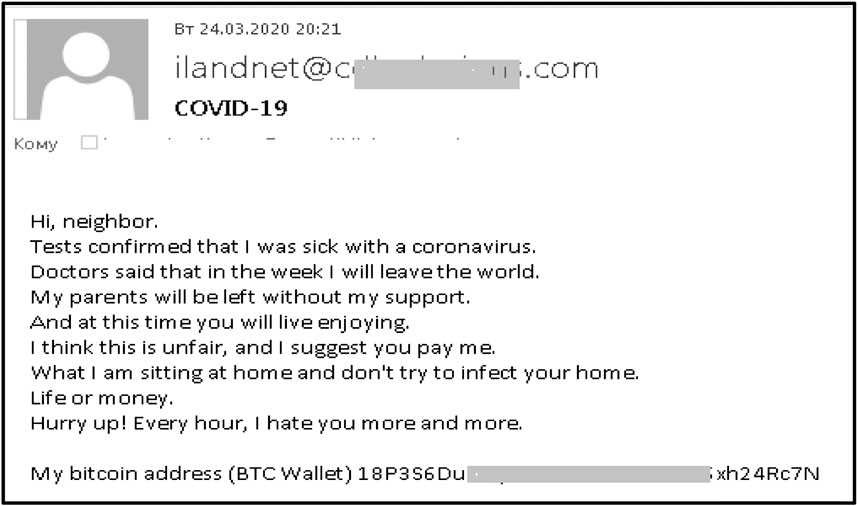
FIGURE 3 . Screenshot of a coronavirus related phishing email ( Ksepersky, 2020 ).
Another example is the phishing attack spotted by a security researcher at Akamai organization in January 2019. The attack attempted to use Google Translate to mask suspicious URLs, prefacing them with the legit-looking “ www.translate.google.com ” address to dupe users into logging in ( Rhett, 2019 ). That attack followed with Phishing scams asking for Netflix payment detail for example, or embedded in promoted tweets that redirect users to genuine-looking PayPal login pages. Although the tricky/bogus page was very well designed in the latter case, the lack of a Hypertext Transfer Protocol Secure (HTTPS) lock and misspellings in the URL were key red flags (or giveaways) that this was actually a phishing attempt ( Keck, 2018 ). Figure 4A shows a screenshot of a phishing email received by the Federal Trade Commission (FTC). The email promotes the user to update his payment method by clicking on a link, pretending that Netflix is having a problem with the user's billing information ( FTC, 2018 ).
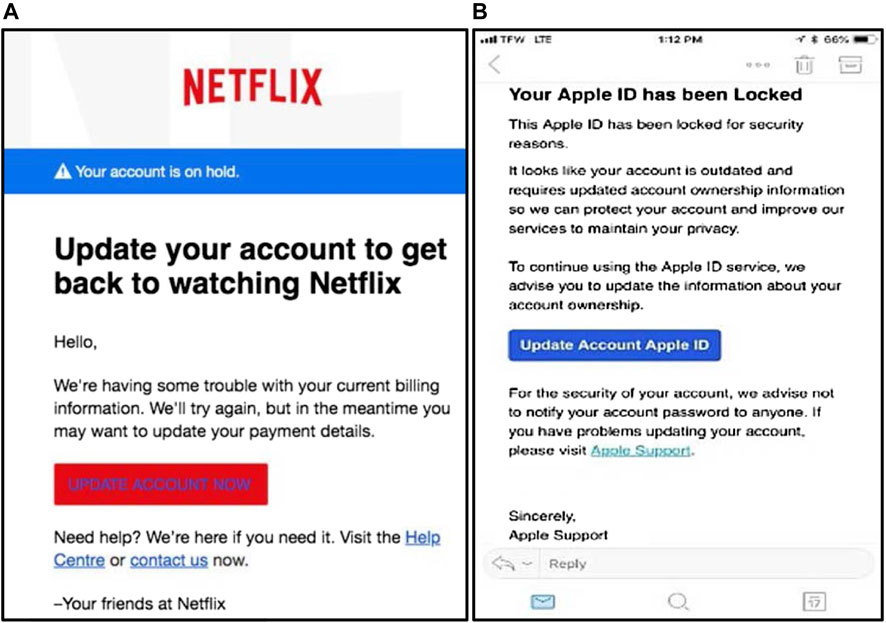
FIGURE 4 . Screenshot of the (A) Netflix scam email and (B) fraudulent text message (Apple) ( Keck, 2018 ; Rhett, 2019 )
Figure 4B shows a text message as another example of phishing that is difficult to spot as a fake text message ( Pompon et al., 2018 ). The text message shown appears to come from Apple asking the customer to update the victim’s account. A sense of urgency is used in the message as a lure to motivate the user to respond.
Developing a Phishing Campaign
Today, phishing is considered one of the most pressing cybersecurity threats for all internet users, regardless of their technical understanding and how cautious they are. These attacks are getting more sophisticated by the day and can cause severe losses to the victims. Although the attacker’s first motivation is stealing money, stolen sensitive data can be used for other malicious purposes such as infiltrating sensitive infrastructures for espionage purposes. Therefore, phishers keep on developing their techniques over time with the development of electronic media. The following sub-sections discuss phishing evolution and the latest statistics.
Historical Overview
Cybersecurity has been a major concern since the beginning of APRANET, which is considered to be the first wide-area packet-switching network with distributed control and one of the first networks to implement the TCP/IP protocol suite. The term “Phishing” which was also called carding or brand spoofing, was coined for the first time in 1996 when the hackers created randomized credit card numbers using an algorithm to steal users' passwords from America Online (AOL) ( Whitman and Mattord, 2012 ; Cui et al., 2017 ). Then phishers used instant messages or emails to reach users by posing as AOL employees to convince users to reveal their passwords. Attackers believed that requesting customers to update their account would be an effective way to disclose their sensitive information, thereafter, phishers started to target larger financial companies. The author in ( Ollmann, 2004 ) believes that the “ph” in phishing comes from the terminology “Phreaks” which was coined by John Draper, who was also known as Captain Crunch, and was used by early Internet criminals when they phreak telephone systems. Where the “f” in ‘fishing’ replaced with “ph” in “Phishing” as they both have the same meaning by phishing the passwords and sensitive information from the sea of internet users. Over time, phishers developed various and more advanced types of scams for launching their attack. Sometimes, the purpose of the attack is not limited to stealing sensitive information, but it could involve injecting viruses or downloading the malicious program into a victim's computer. Phishers make use of a trusted source (for instance a bank helpdesk) to deceive victims so that they disclose their sensitive information ( Ollmann, 2004 ).
Phishing attacks are rapidly evolving, and spoofing methods are continuously changing as a response to new corresponding countermeasures. Hackers take advantage of new tool-kits and technologies to exploit systems’ vulnerabilities and also use social engineering techniques to fool unsuspecting users. Therefore, phishing attacks continue to be one of the most successful cybercrime attacks.
The Latest Statistics of Phishing Attacks
Phishing attacks are becoming more common and they are significantly increasing in both sophistication and frequency. Lately, phishing attacks have appeared in various forms. Different channels and threats are exploited and used by the attackers to trap more victims. These channels could be social networks or VoIP, which could carry various types of threats such as malicious attachments, embedded links within an email, instant messages, scam calls, or other types. Criminals know that social engineering-based methods are effective and profitable; therefore, they keep focusing on social engineering attacks, as it is their favorite weapon, instead of concentrating on sophisticated techniques and toolkits. Phishing attacks have reached unprecedented levels especially with emerging technologies such as mobile and social media ( Marforio et al., 2015 ). For instance, from 2017 to 2020, phishing attacks have increased from 72 to 86% among businesses in the United Kingdom in which a large proportion of the attacks are originated from social media ( GOV.UK, 2020 ).
The APWG Phishing Activity Trends Report analyzes and measures the evolution, proliferation, and propagation of phishing attacks reported to the APWG. Figure 5 shows the growth in phishing attacks from 2015 to 2020 by quarters based on APWG annual reports ( APWG, 2020 ). As demonstrated in Figure 5 , in the third quarter of 2019, the number of phishing attacks rose to 266,387, which is the highest level in three years since late 2016. This was up 46% from the 182,465 for the second quarter, and almost double the 138,328 seen in the fourth quarter of 2018. The number of unique phishing e-mails reported to APWG in the same quarter was 118,260. Furthermore, it was found that the number of brands targeted by phishing campaigns was 1,283.
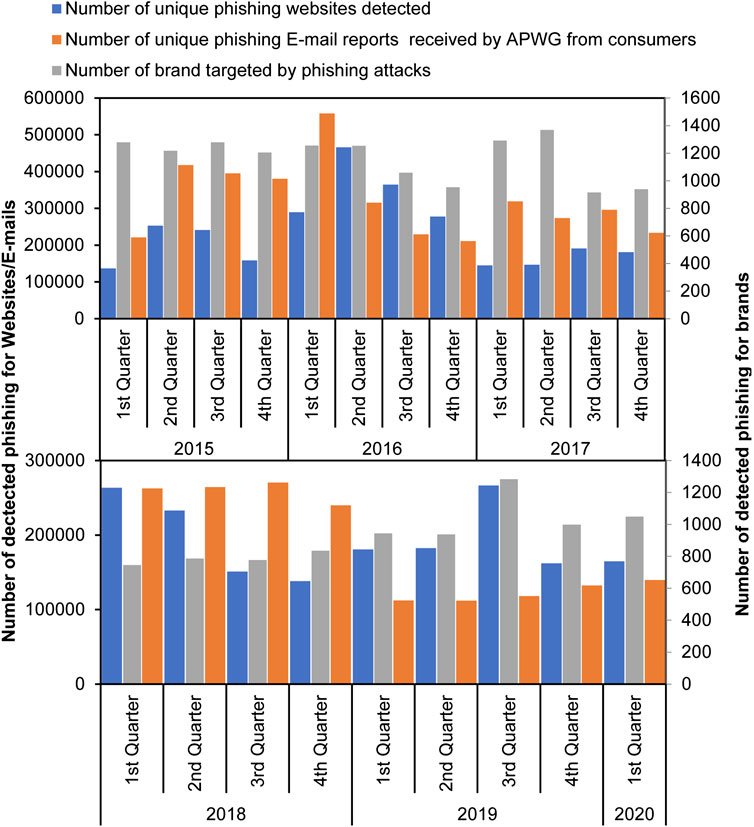
FIGURE 5 . The growth in phishing attacks 2015–2020 by quarters based on data collected from APWG annual reports.
Cybercriminals are always taking advantage of disasters and hot events for their own gains. With the beginning of the COVID-19 crisis, a variety of themed phishing and malware attacks have been launched by phishers against workers, healthcare facilities, and even the general public. A report from Microsoft ( Microsoft, 2020 ) showed that cyber-attacks related to COVID-19 had spiked to an unprecedented level in March, most of these scams are fake COVID-19 websites according to security company RiskIQ ( RISKIQ, 2020 ). However, the total number of phishing attacks observed by APWG in the first quarter of 2020 was 165,772, up from the 162,155 observed in the fourth quarter of 2019. The number of these unique phishing reports submitted to APWG during the first quarter of 2020 was 139,685, up from 132,553 in the fourth quarter of 2019, 122,359 in the third quarter of 2019, and 112,163 in the second quarter of 2019 ( APWG, 2020 ).
A study ( KeepnetLABS, 2018 ) confirmed that more than 91% of system breaches are caused by attacks initiated by email. Although cybercriminals use email as the main medium for leveraging their attacks, many organizations faced a high volume of different social engineering attacks in 2019 such as Social Media Attacks, Smishing Attacks, Vishing Attacks, USB-based Attacks (for example by hiding and delivering malware to smartphones via USB phone chargers and distributing malware-laden free USBs) ( Proofpoint, 2020 ). However, info-security professionals reported a higher frequency of all types of social engineering attacks year-on-year according to a report presented by Proofpoint. Spear phishing increased to 64% in 2018 from 53% in 2017, Vishing and/or SMishing increased to 49% from 45%, and USB attacks increased to 4% from 3%. The positive side shown in this study is that 59% of suspicious emails reported by end-users were classified as potential phishing, indicating that employees are being more security-aware, diligent, and thoughtful about the emails they receive ( Proofpoint, 2019a ). In all its forms, phishing can be one of the easiest cyber attacks to fall for. With the increasing levels of different phishing types, a survey was conducted by Proofpoint to identify the strengths and weaknesses of particular regions in terms of specific fundamental cybersecurity concepts. In this study, several questions were asked of 7,000 end-users about the identification of multiple terms like phishing, ransomware, SMishing, and Vishing across seven countries; the US, United Kingdom, France, Germany, Italy, Australia, and Japan. The response was different from country to country, where respondents from the United Kingdom recorded the highest knowledge with the term phishing at 70% and the same with the term ransomware at 60%. In contrast, the results showed that the United Kingdom recorded only 18% for each Vishing and SMishing ( Proofpoint, 2019a ), as shown in Table 1 .

TABLE 1 . Percentage of respondents understanding multiple cybersecurity terms from different countries.
On the other hand, a report by Wombat security reflects responses from more than 6,000 working adults about receiving fraudulent solicitation across six countries; the US, United Kingdom, Germany, France, Italy, and Australia ( Ksepersky, 2020 ). Respondents from the United Kingdom stated that they were recipients of fraudulent solicitations through the following sources: email 62%, phone call 27%, text message 16%, mailed letter 8%, social media 10%, and 17% confirmed that they been the victim of identity theft ( Ksepersky, 2020 ). However, the consequences of responding to phishing are serious and costly. For instance, the United Kingdom losses from financial fraud across payment cards, remote banking, and cheques totaled £768.8 million in 2016 ( Financial Fraud Action UK, 2017 ). Indeed, the losses resulting from phishing attacks are not limited to financial losses that might exceed millions of pounds, but also loss of customers and reputation. According to the 2020 state of phish report ( Proofpoint, 2020 ), damages from successful phishing attacks can range from lost productivity to cash outlay. The cost can include; lost hours from employees, remediation time for info security teams’ costs due to incident response, damage to reputation, lost intellectual property, direct monetary losses, compliance fines, lost customers, legal fees, etc.
There are many targets for phishing including end-user, business, financial services (i.e., banks, credit card companies, and PayPal), retail (i.e., eBay, Amazon) and, Internet Service Providers ( wombatsecurity.com, 2018 ). Affected organizations detected by Kaspersky Labs globally in the first quarter of 2020 are demonstrated in Figure 6 . As shown in the figure, online stores were at the top of the targeted list (18.12%) followed by global Internet portals (16.44%) and social networks in third place (13.07%) ( Ksepersky, 2020 ). While the most impersonated brands overall for the first quarter of 2020 were Apple, Netflix, Yahoo, WhatsApp, PayPal, Chase, Facebook, Microsoft eBay, and Amazon ( Checkpoint, 2020 ).
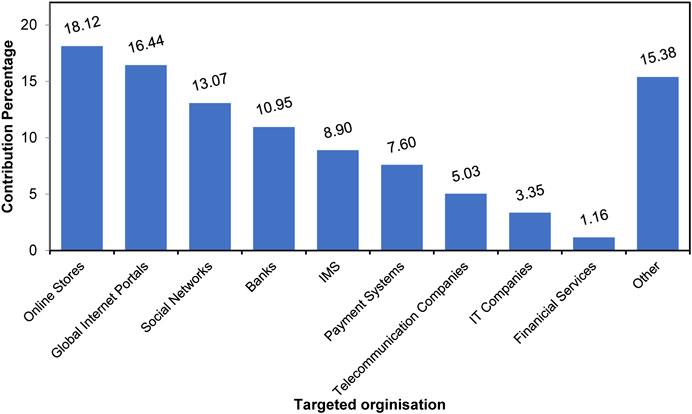
FIGURE 6 . Distribution of organizations affected by phishing attacks detected by Kaspersky in quarter one of 2020.
Phishing attacks can take a variety of forms to target people and steal sensitive information from them. Current data shows that phishing attacks are still effective, which indicates that the available existing countermeasures are not enough to detect and prevent these attacks especially on smart devices. The social engineering element of the phishing attack has been effective in bypassing the existing defenses to date. Therefore, it is essential to understand what makes people fall victim to phishing attacks. What Attributes Make Some People More Susceptible to Phishing Attacks Than Others discusses the human attributes that are exploited by the phishers.
What Attributes Make Some People More Susceptible to Phishing Attacks Than Others
Why do most existing defenses against phishing not work? What personal and contextual attributes make them more susceptible to phishing attacks than other users? Different studies have discussed those two questions and examined the factors affecting susceptibility to a phishing attack and the reasons behind why people get phished. Human nature is considered one of the most affecting factors in the process of phishing. Everyone is susceptible to phishing attacks because phishers play on an individual’s specific psychological/emotional triggers as well as technical vulnerabilities ( KeepnetLABS, 2018 ; Crane, 2019 ). For instance, individuals are likely to click on a link within an email when they see authority cues ( Furnell, 2007 ). In 2017, a report by PhishMe (2017) found that curiosity and urgency were the most common triggers that encourage people to respond to the attack, later these triggers were replaced by entertainment, social media, and reward/recognition as the top emotional motivators. However, in the context of a phishing attack, the psychological triggers often surpass people’s conscious decisions. For instance, when people are working under stress, they tend to make decisions without thinking of the possible consequences and options ( Lininger and Vines, 2005 ). Moreover, everyday stress can damage areas of the brain that weakens the control of their emotions ( Keinan, 1987 ). Several studies have addressed the association between susceptibility to phishing and demographic variables (e.g., age and gender) as an attempt to identify the reasons behind phishing success at different population groups. Although everyone is susceptible to phishing, studies showed that different age groups are more susceptible to certain lures than others are. For example, participants with an age range between 18 and 25 are more susceptible to phishing than other age groups ( Williams et al., 2018 ). The reason that younger adults are more likely to fall for phishing, is that younger adults are more trusting when it comes to online communication, and are also more likely to click on unsolicited e-mails ( Getsafeonline, 2017 ). Moreover, older participants are less susceptible because they tend to be less impulsive ( Arnsten et al., 2012 ). While some studies confirmed that women are more susceptible than men to phishing as they click on links in phishing emails and enter information into phishing websites more often than men do. The study published by Getsafeonline (2017) identifies a lack of technical know-how and experience among women than men as the main reason for this. In contrast, a survey conducted by antivirus company Avast found that men are more susceptible to smartphone malware attacks than women ( Ong, 2014 ). These findings confirmed the results from the study ( Hadlington, 2017 ) that found men are more susceptible to mobile phishing attacks than women. The main reason behind this according to Hadlington (2017) is that men are more comfortable and trusting when using mobile online services. The relationships between demographic characteristics of individualls and their ability to correctly detect a phishing attack have been studied in ( Iuga et al., 2016 ). The study showed that participants with high Personal Computer (PC) usage tend to identify phishing efforts more accurately and faster than other participants. Another study ( Hadlington, 2017 ) showed that internet addiction, attentional, and motor impulsivity were significant positive predictors for risky cybersecurity behaviors while a positive attitude toward cybersecurity in business was negatively related to risky cybersecurity behaviors. On the other hand, the trustworthiness of people in some web sites/platforms is one of the holes that the scammers or crackers exploit especially when it based on visual appearance that could fool the user ( Hadlington, 2017 ). For example, fraudsters take advantage of people’s trust in a website by replacing a letter from the legitimate site with a number such as goog1e.com instead of google.com . Another study ( Yeboah-Boateng and Amanor, 2014 ) demonstrates that although college students are unlikely to disclose personal information as a response to an email, nonetheless they could easily be tricked by other tactics, making them alarmingly susceptible to email phishing attacks. The reason for that is most college students do not have a basis in ICT especially in terms of security. Although security terms like viruses, online scams and worms are known by some end-users, these users could have no knowledge about Phishing, SMishing, and Vishing and others ( Lin et al., 2012 ). However, study ( Yeboah-Boateng and Amanor, 2014 ) shows that younger students are more susceptible than older students, and students who worked full-time were less likely to fall for phishing.
The study reported in ( Diaz et al., 2020 ) examines user click rates and demographics among undergraduates by sending phishing attacks to 1,350 randomly selected students. Students from various disciplines were involved in the test, from engineering and mathematics to arts and social sciences. The study observed that student susceptibility was affected by a range of factors such as phishing awareness, time spent on the computer, cyber training, age, academic year, and college affiliation. The most surprising finding is that those who have greater phishing knowledge are more susceptible to phishing scams. The authors consider two speculations for these unexpected findings. First, user’s awareness about phishing might have been increased with the continuous falling for phishing scams. Second, users who fell for the phish might have less knowledge about phishing than they claim. Other findings from this study agreed with findings from other studies that is, older students were more able to detect a phishing email, and engineering and IT majors had some of the lowest click rates as shown in Figure 7 , which shows that some academic disciplines are more susceptible to phishing than others ( Bailey et al., 2008 ).
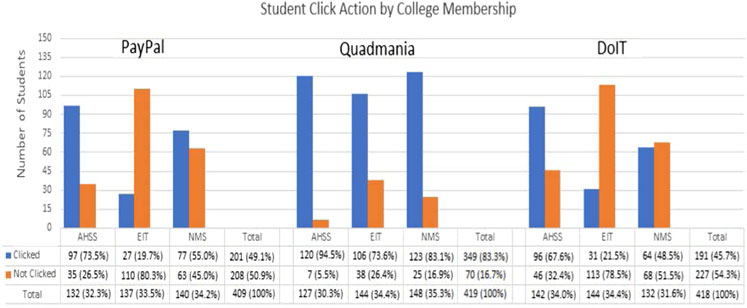
FIGURE 7 . The number of clicks on phishing emails by students in the College of Arts, Humanities, and Social Sciences (AHSS), the College of Engineering and Information Technology (EIT), and the College of Natural and Mathematical Sciences (NMS) at the University of Maryland, Baltimore County (UMBC) ( Diaz et al., 2020 ).
Psychological studies have also illustrated that the user’s ability to avoid phishing attacks affected by different factors such as browser security indicators and user's awareness of phishing. The author in ( Dhamija et al., 2006 ) conducted an experimental study using 22 participants to test the user’s ability to recognize phishing websites. The study shows that 90% of these participants became victims of phishing websites and 23% of them ignored security indexes such as the status and address bar. In 2015, another study was conducted for the same purpose, where a number of fake web pages was shown to the participants ( Alsharnouby et al., 2015 ). The results of this study showed that participants detected only 53% of phishing websites successfully. The authors also observed that the time spent on looking at browser elements affected the ability to detect phishing. Lack of knowledge or awareness and carelessness are common causes for making people fall for a phishing trap. Most people have unknowingly opened a suspicious attachment or clicked a fake link that could lead to different levels of compromise. Therefore, focusing on training and preparing users for dealing with such attacks are essential elements to minimize the impact of phishing attacks.
Given the above discussion, susceptibility to phishing varies according to different factors such as age, gender, education level, internet, and PC addiction, etc. Although for each person, there is a trigger that can be exploited by phishers, even people with high experience may fall prey to phishing due to the attack sophistication that makes it difficult to be recognized. Therefore, it is inequitable that the user has always been blamed for falling for these attacks, developers must improve the anti-phishing systems in a way that makes the attack invisible. Understanding the susceptibility of individuals to phishing attacks will help in better developing prevention and detection techniques and solutions.
Proposed Phishing Anatomy
Phishing process overview.
Generally, most of the phishing attacks start with an email ( Jagatic et al., 2007 ). The phishing mail could be sent randomly to potential users or it can be targeted to a specific group or individuals. Many other vectors can also be used to initiate the attack such as phone calls, instant messaging, or physical letters. However, phishing process steps have been discussed by many researchers due to the importance of understanding these steps in developing an anti-phishing solution. The author in the study ( Rouse, 2013 ) divides the phishing attack process into five phases which are planning, setup, attack, collection, and cash. A study ( Jakobsson and Myers, 2006 ) discusses the phishing process in detail and explained it as step-by-step phases. These phases include preparation for the attack, sending a malicious program using the selected vector, obtaining the user’s reaction to the attack, tricking a user to disclose their confidential information which will be transmitted to the phisher, and finally obtaining the targeted money. While the study ( Abad, 2005 ) describes a phishing attack in three phases: the early phase which includes initializing attack, creating the phishing email, and sending a phishing email to the victim. The second phase includes receiving an email by the victim and disclosing their information (in the case of the respondent) and the final phase in which the defrauding is successful. However, all phishing scams include three primary phases, the phisher requests sensitive valuables from the target, and the target gives away these valuables to a phisher, and phisher misuses these valuables for malicious purposes. These phases can be classified furthermore into its sub-processes according to phishing trends. Thus, a new anatomy for phishing attacks has been proposed in this article, which expands and integrates previous definitions to cover the full life cycle of a phishing attack. The proposed new anatomy, which consists of 4 phases, is shown in Figure 8 . This new anatomy provides a reference structure to look at phishing attacks in more detail and also to understand potential countermeasures to prevent them. The explanations for each phase and its components are presented as follows:
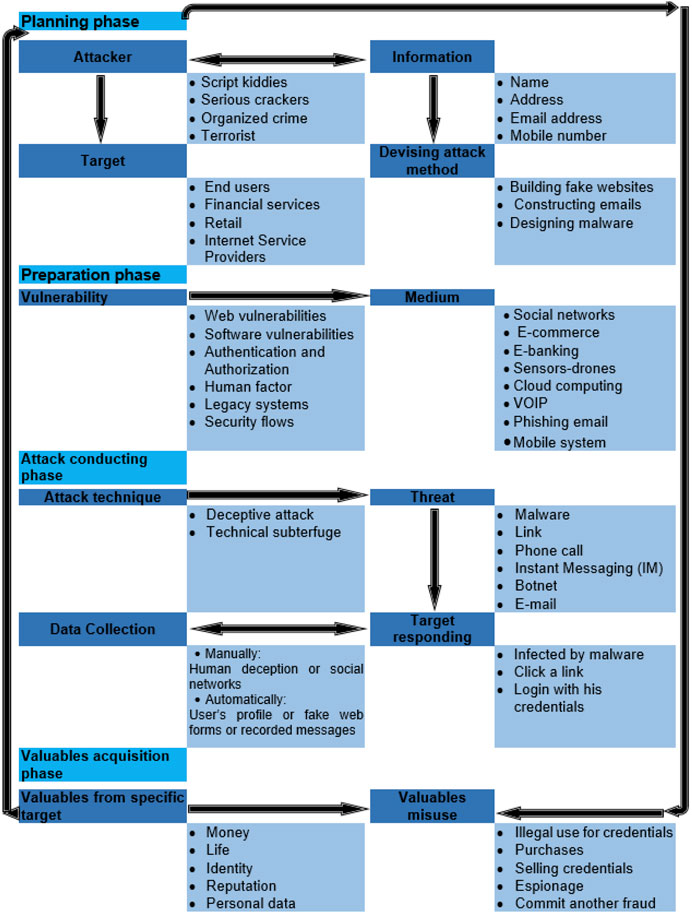
FIGURE 8 . The proposed anatomy of phishing was built upon the proposed phishing definition in this article, which concluded from our understanding of a phishing attack.
Figure 8 depicts the proposed anatomy of the phishing attack process, phases, and components drawn upon the proposed definition in this article. The proposed phishing anatomy explains in detail each phase of phishing phases including attackers and target types, examples about the information that could be collected by the attacker about the victim, and examples about attack methods. The anatomy, as shown in the figure, illustrates a set of vulnerabilities that the attacker can exploit and the mediums used to conduct the attack. Possible threats are also listed, as well as the data collection method for a further explanation and some examples about target responding types and types of spoils that the attacker could gain and how they can use the stolen valuables. This anatomy elaborates on phishing attacks in depth which helps people to better understand the complete phishing process (i.e., end to end Phishing life cycle) and boost awareness among readers. It also provides insights into potential solutions for phishing attacks we should focus on. Instead of always placing the user or human in an accusation ring as the only reason behind phishing success, developers must be focusing on solutions to mitigate the initiation of the attack by preventing the bait from reaching the user. For instance, to reach the target’s system, the threat has to pass through many layers of technology or defenses exploiting one or more vulnerabilities such as web and software vulnerabilities.
Planning Phase
This is the first stage of the attack, where a phisher makes a decision about the targets and starts gathering information about them (individuals or company). Phishers gather information about the victims to lure them based on psychological vulnerability. This information can be anything like name, e-mail addresses for individuals, or the customers of that company. Victims could also be selected randomly, by sending mass mailings or targeted by harvesting their information from social media, or any other source. Targets for phishing could be any user with a bank account and has a computer on the Internet. Phishers target businesses such as financial services, retail sectors such as eBay and Amazon, and internet service providers such as MSN/Hotmail, and Yahoo ( Ollmann, 2004 ; Ramzan and Wuest, 2007 ). This phase also includes devising attack methods such as building fake websites (sometimes phishers get a scam page that is already designed or used, designing malware, constructing phishing emails. The attacker can be categorized based on the attack motivation. There are four types of attackers as mentioned in studies ( Vishwanath, 2005 ; Okin, 2009 ; EDUCBA, 2017 ; APWG, 2020 ):
▪ Script kiddies: the term script kiddies represents an attacker with no technical background or knowledge about writing sophisticated programs or developing phishing tools but instead they use scripts developed by others in their phishing attack. Although the term comes from children that use available phishing kits to crack game codes by spreading malware using virus toolkits, it does not relate precisely to the actual age of the phisher. Script kiddies can get access to website administration privileges and commit a “Web cracking” attack. Moreover, they can use hacking tools to compromise remote computers so-called “botnet,” the single compromised computer called a “zombie computer.” These attackers are not limited to just sit back and enjoy phishing, they could cause serious damage such as stealing information or uploading Trojans or viruses. In February 2000, an attack launched by Canadian teen Mike Calce resulted in $1.7 million US Dollars (USD) damages from Distributed Denial of Service (DDoS) attacks on CNN, eBay, Dell, Yahoo, and Amazon ( Leyden, 2001 ).
▪ Serious Crackers: also known as Black Hats. These attackers can execute sophisticated attacks and develop worms and Trojans for their attack. They hijack people's accounts maliciously and steal credit card information, destroy important files, or sell compromised credentials for personal gains.
▪ Organized crime: this is the most organized and effective type of attacker and they can incur significant damage to victims. These people hire serious crackers for conducting phishing attacks. Moreover, they can thoroughly trash the victim's identity, and committing devastated frauds as they have the skills, tools, and manpower. An organized cybercrime group is a team of expert hackers who share their skills to build complex attacks and to launch phishing campaigns against individuals and organizations. These groups offer their work as ‘crime as a service’ and they can be hired by terrorist groups, organizations, or individuals.
▪ Terrorists: due to our dependency on the internet for most activities, terrorist groups can easily conduct acts of terror remotely which could have an adverse impact. These types of attacks are dangerous since they are not in fear of any aftermath, for instance going to jail. Terrorists could use the internet to the maximum effect to create fear and violence as it requires limited funds, resources, and efforts compared to, for example, buying bombs and weapons in a traditional attack. Often, terrorists use spear phishing to launch their attacks for different purposes such as inflicting damage, cyber espionage, gathering information, locating individuals, and other vandalism purposes. Cyber espionage has been used extensively by cyber terrorists to steal sensitive information on national security, commercial information, and trade secrets which can be used for terrorist activities. These types of crimes may target governments or organizations, or individuals.
Attack Preparation
After making a decision about the targets and gathering information about them, phishers start to set up the attack by scanning for the vulnerabilities to exploit. The following are some examples of vulnerabilities exploited by phishers. For example, the attacker might exploit buffer overflow vulnerability to take control of target applications, create a DoS attack, or compromise computers. Moreover, “zero-day” software vulnerabilities, which refer to newly discovered vulnerabilities in software programs or operating systems could be exploited directly before it is fixed ( Kayne, 2019 ). Another example is browser vulnerabilities, adding new features and updates to the browser might introduce new vulnerabilities to the browser software ( Ollmann, 2004 ). In 2005, attackers exploited a cross-domain vulnerability in Internet Explorer (IE) ( Symantic, 2019 ). The cross-domain used to separate content from different sources in Microsoft IE. Attackers exploited a flaw in the cross-domain that enables them to execute programs on a user's computer after running IE. According to US-CERT, hackers are actively exploiting this vulnerability. To carry out a phishing attack, attackers need a medium so that they can reach their target. Therefore, apart from planning the attack to exploit potential vulnerabilities, attackers choose the medium that will be used to deliver the threat to the victim and carry out the attack. These mediums could be the internet (social network, websites, emails, cloud computing, e-banking, mobile systems) or VoIP (phone call), or text messages. For example, one of the actively used mediums is Cloud Computing (CC). The CC has become one of the more promising technologies and has popularly replaced conventional computing technologies. Despite the considerable advantages produced by CC, the adoption of CC faces several controversial obstacles including privacy and security issues ( CVEdetails, 2005 ). Due to the fact that different customers could share the same recourses in the cloud, virtualization vulnerabilities may be exploited by a possible malicious customer to perform security attacks on other customers’ applications and data ( Zissis and Lekkas, 2012 ). For example, in September 2014, secret photos of some celebrities suddenly moved through the internet in one of the more terrible data breaches. The investigation revealed that the iCloud accounts of the celebrities were breached ( Lehman and Vajpayee, 2011 ). According to Proofpoint, in 2017, attackers used Microsoft SharePoint to infect hundreds of campaigns with malware through messages.
Attack Conducting Phase
This phase involves using attack techniques to deliver the threat to the victim as well as the victim’s interaction with the attack in terms of responding or not. After the victim's response, the system may be compromised by the attacker to collect user's information using techniques such as injecting client-side script into webpages ( Johnson, 2016 ). Phishers can compromise hosts without any technical knowledge by purchasing access from hackers ( Abad, 2005 ). A threat is a possible danger that that might exploit a vulnerability to compromise people’s security and privacy or cause possible harm to a computer system for malicious purposes. Threats could be malware, botnet, eavesdropping, unsolicited emails, and viral links. Several Phishing techniques are discussed in sub- Types and Techniques of Phishing Attacks .
Valuables Acquisition Phase
In this stage, the phisher collects information or valuables from victims and uses it illegally for purchasing, funding money without the user’s knowledge, or selling these credentials in the black market. Attackers target a wide range of valuables from their victims that range from money to people’s lives. For example, attacks on online medical systems may lead to loss of life. Victim’s data can be collected by phishers manually or through automated techniques ( Jakobsson et al., 2007 ).
The data collection can be conducted either during or after the victim’s interaction with the attacker. However, to collect data manually simple techniques are used wherein victims interact directly with the phisher depending on relationships within social networks or other human deception techniques ( Ollmann, 2004 ). Whereas in automated data collection, several techniques can be used such as fake web forms that are used in web spoofing ( Dhamija et al., 2006 ). Additionally, the victim’s public data such as the user’s profile in social networks can be used to collect the victim’s background information that is required to initialize social engineering attacks ( Wenyin et al., 2005 ). In VoIP attacks or phone attack techniques such as recorded messages are used to harvest user's data ( Huber et al., 2009 ).
Types and Techniques of Phishing Attacks
Phishers conduct their attack either by using psychological manipulation of individuals into disclosing personal information (i.e., deceptive attack as a form of social engineering) or using technical methods. Phishers, however, usually prefer deceptive attacks by exploiting human psychology rather than technical methods. Figure 9 illustrates the types of phishing and techniques used by phishers to conduct a phishing attack. Each type and technique is explained in subsequent sections and subsections.
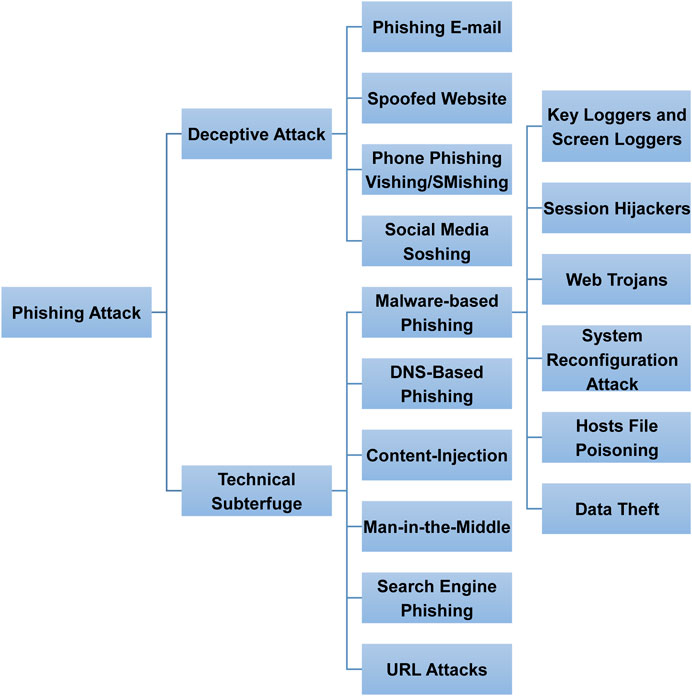
FIGURE 9 . Phishing attack types and techniques drawing upon existing phishing attacks.
Deceptive Phishing
Deceptive phishing is the most common type of phishing attack in which the attacker uses social engineering techniques to deceive victims. In this type of phishing, a phisher uses either social engineering tricks by making up scenarios (i.e., false account update, security upgrade), or technical methods (i.e., using legitimate trademarks, images, and logos) to lure the victim and convince them of the legitimacy of the forged email ( Jakobsson and Myers, 2006 ). By believing these scenarios, the user will fall prey and follow the given link, which leads to disclose his personal information to the phisher.
Deceptive phishing is performed through phishing emails; fake websites; phone phishing (Scam Call and IM); social media; and via many other mediums. The most common social phishing types are discussed below;
Phishing e-Mail
The most common threat derived by an attacker is deceiving people via email communications and this remains the most popular phishing type to date. A Phishing email or Spoofed email is a forged email sent from an untrusted source to thousands of victims randomly. These fake emails are claiming to be from a person or financial institution that the recipient trusts in order to convince recipients to take actions that lead them to disclose their sensitive information. A more organized phishing email that targets a particular group or individuals within the same organization is called spear phishing. In the above type, the attacker may gather information related to the victim such as name and address so that it appears to be credible emails from a trusted source ( Wang et al., 2008 ), and this is linked to the planning phase of the phishing anatomy proposed in this article. A more sophisticated form of spear phishing is called whaling, which targets high-rank people such as CEOs and CFOs. Some examples of spear-phishing attack victims in early 2016 are the phishing email that hacked the Clinton campaign chairman John Podesta’s Gmail account ( Parmar, 2012 ). Clone phishing is another type of email phishing, where the attacker clones a legitimate and previously delivered email by spoofing the email address and using information related to the recipient such as addresses from the legitimate email with replaced links or malicious attachments ( Krawchenko, 2016 ). The basic scenario for this attack is illustrated previously in Figure 4 and can be described in the following steps.
1. The phisher sets up a fraudulent email containing a link or an attachment (planning phase).
2. The phisher executes the attack by sending a phishing email to the potential victim using an appropriate medium (attack conducting phase).
3. The link (if clicked) directs the user to a fraudulent website, or to download malware in case of clicking the attachment (interaction phase).
4. The malicious website prompts users to provide confidential information or credentials, which are then collected by the attacker and used for fraudulent activities. (Valuables acquisition phase).
Often, the phisher does not use the credentials directly; instead, they resell the obtained credentials or information on a secondary market ( Jakobsson and Myers, 2006 ), for instance, script kiddies might sell the credentials on the dark web.
Spoofed Website
This is also called phishing websites, in which phishers forge a website that appears to be genuine and looks similar to the legitimate website. An unsuspicious user is redirected to this website after clicking a link embedded within an email or through an advertisement (clickjacking) or any other way. If the user continues to interact with the spoofed website, sensitive information will be disclosed and harvested by the phisher ( CSIOnsite, 2012 ).
Phone Phishing (Vishing and SMishing)
This type of phishing is conducted through phone calls or text messages, in which the attacker pretends to be someone the victim knows or any other trusted source the victim deals with. A user may receive a convincing security alert message from a bank convincing the victim to contact a given phone number with the aim to get the victim to share passwords or PIN numbers or any other Personally Identifiable Information (PII). The victim may be duped into clicking on an embedded link in the text message. The phisher then could take the credentials entered by the victim and use them to log in to the victims' instant messaging service to phish other people from the victim’s contact list. A phisher could also make use of Caller IDentification (CID) 3 spoofing to dupe the victim that the call is from a trusted source or by leveraging from an internet protocol private branch exchange (IP PBX) 4 tools which are open-source and software-based that support VoIP ( Aburrous et al., 2008 ). A new report from Fraud Watch International about phishing attack trends for 2019 anticipated an increase in SMishing where the text messages content is only viewable on a mobile device ( FraudWatchInternational, 2019 ).
Social Media Attack (Soshing, Social Media Phishing)
Social media is the new favorite medium for cybercriminals to conduct their phishing attacks. The threats of social media can be account hijacking, impersonation attacks, scams, and malware distributing. However, detecting and mitigating these threats requires a longer time than detecting traditional methods as social media exists outside of the network perimeter. For example, the nation-state threat actors conducted an extensive series of social media attacks on Microsoft in 2014. Multiple Twitter accounts were affected by these attacks and passwords and emails for dozens of Microsoft employees were revealed ( Ramzan, 2010 ). According to Kaspersky Lab’s, the number of phishing attempts to visit fraudulent social network pages in the first quarter of 2018 was more than 3.7 million attempts, of which 60% were fake Facebook pages ( Raggo, 2016 ).
The new report from predictive email defense company Vade Secure about phishers’ favorites for quarter 1 and quarter 2 of 2019, stated that Soshing primarily on Facebook and Instagram saw a 74.7% increase that is the highest quarter-over- quarter growth of any industry ( VadeSecure, 2021 ).
Technical Subterfuge
Technical subterfuge is the act of tricking individuals into disclosing their sensitive information through technical subterfuge by downloading malicious code into the victim's system. Technical subterfuge can be classified into the following types:
Malware-Based Phishing
As the name suggests, this is a type of phishing attack which is conducted by running malicious software on a user’s machine. The malware is downloaded to the victim’s machine, either by one of the social engineering tricks or technically by exploiting vulnerabilities in the security system (e.g., browser vulnerabilities) ( Jakobsson and Myers, 2006 ). Panda malware is one of the successful malware programs discovered by Fox-IT Company in 2016. This malware targets Windows Operating Systems (OS). It spreads through phishing campaigns and its main attack vectors include web injects, screenshots of user activity (up to 100 per mouse click), logging of keyboard input, Clipboard pastes (to grab passwords and paste them into form fields), and exploits to the Virtual Network Computing (VNC) desktop sharing system. In 2018, Panda malware expanded its targets to include cryptocurrency exchanges and social media sites ( F5Networks, 2018 ). There are many forms of Malware-based phishing attacks; some of them are discussed below:
Key Loggers and Screen Loggers
Loggers are the type of malware used by phishers and installed either through Trojan horse email attachments or through direct download to the user’s personal computer. This software monitors data and records user keystrokes and then sends it to the phisher. Phisher uses the key loggers to capture sensitive information related to victims, such as names, addresses, passwords, and other confidential data. Key loggers can also be used for non-phishing purposes such as to monitor a child's use of the internet. Key loggers can also be implemented in many other ways such as detecting URL changes and logs information as Browser Helper Object (BHO) that enables the attacker to take control of the features of all IE’s, monitoring keyboard and mouse input as a device driver and, monitoring users input and displays as a screen logger ( Jakobsson and Myers, 2006 ).
Viruses and Worms
A virus is a type of malware, which is a piece of code spreading in another application or program by making copies of itself in a self-automated manner ( Jakobsson and Myers, 2006 ; F5Networks, 2018 ). Worms are similar to viruses but they differ in the execution manner, as worms are executed by exploiting the operating systems vulnerability without the need to modify another program. Viruses transfer from one computer to another with the document that they are attached to, while worms transfer through the infected host file. Both viruses and worms can cause data and software damaging or Denial-of-Service (DoS) conditions ( F5Networks, 2018 ).
Spying software is a malicious code designed to track the websites visited by users in order to steal sensitive information and conduct a phishing attack. Spyware can be delivered through an email and, once it is installed on the computer, take control over the device and either change its settings or gather information such as passwords and credit card numbers or banking records which can be used for identity theft ( Jakobsson and Myers, 2006 ).
Adware is also known as advertising-supported software ( Jakobsson and Myers, 2006 ). Adware is a type of malware that shows the user an endless pop-up window with ads that could harm the performance of the device. Adware can be annoying but most of it is safe. Some of the adware could be used for malicious purposes such as tracking the internet sites the user visits or even recording the user's keystrokes ( cisco, 2018 ).
Ransomware is a type of malware that encrypts the user's data after they run an executable program on the device. In this type of attack, the decryption key is held until the user pays a ransom (cisco, 2018). Ransomware is responsible for tens of millions of dollars in extortion annually. Worse still, this is hard to detect with developing new variants, facilitating the evasion of many antivirus and intrusion detection systems ( Latto, 2020 ). Ransomware is usually delivered to the victim's device through phishing emails. According to a report ( PhishMe, 2016 ), 93% of all phishing emails contained encryption ransomware. Phishing, as a social engineering attack, convinces victims into executing actions without knowing about the malicious program.
A rootkit is a collection of programs, typically malicious, that enables access to a computer or computer network. These toolsets are used by intruders to hide their actions from system administrators by modifying the code of system calls and changing the functionality ( Belcic, 2020 ). The term “rootkit” has negative connotations through its association with malware, and it is used by the attacker to alert existing system tools to escape detection. These kits enable individuals with little or no knowledge to launch phishing exploits. It contains coding, mass emailing software (possibly with thousands of email addresses included), web development software, and graphic design tools. An example of rootkits is the Kernel kit. Kernel-Level Rootkits are created by replacing portions of the core operating system or adding new code via Loadable Kernel Modules in (Linux) or device drivers (in Windows) ( Jakobsson and Myers, 2006 ).
Session Hijackers
In this type, the attacker monitors the user’s activities by embedding malicious software within a browser component or via network sniffing. The monitoring aims to hijack the session, so that the attacker performs an unauthorized action with the hijacked session such as financial transferring, without the user's permission ( Jakobsson and Myers, 2006 ).
Web Trojans
Web Trojans are malicious programs that collect user’s credentials by popping up in a hidden way over the login screen ( Jakobsson and Myers, 2006 ). When the user enters the credentials, these programs capture and transmit the stolen credentials directly to the attacker ( Jakobsson et al., 2007 ).

Hosts File Poisoning
This is a way to trick a user into going to the phisher’s site by poisoning (changing) the host’s file. When the user types a particular website address in the URL bar, the web address will be translated into a numeric (IP) address before visiting the site. The attacker, to take the user to a fake website for phishing purposes, will modify this file (e.g., DNS cache). This type of phishing is hard to detect even by smart and perceptive users ( Ollmann, 2004 ).
System Reconfiguration Attack
In this format of the phishing attack, the phisher manipulates the settings on a user’s computer for malicious activities so that the information on this PC will be compromised. System reconfigurations can be changed using different methods such as reconfiguring the operating system and modifying the user’s Domain Name System (DNS) server address. The wireless evil twin is an example of a system reconfiguration attack in which all user’s traffic is monitored via a malicious wireless Access Point (AP) ( Jakobsson and Myers, 2006 ).
Data theft is an unauthorized accessing and stealing of confidential information for a business or individuals. Data theft can be performed by a phishing email that leads to the download of a malicious code to the user's computer which in turn steals confidential information stored in that computer directly ( Jakobsson and Myers, 2006 ). Stolen information such as passwords, social security numbers, credit card information, sensitive emails, and other personal data could be used directly by a phisher or indirectly by selling it for different purposes.
Domain Name System Based Phishing (Pharming)
Any form of phishing that interferes with the domain name system so that the user will be redirected to the malicious website by polluting the user's DNS cache with wrong information is called DNS-based phishing. Although the host’s file is not a part of the DNS, the host’s file poisoning is another form of DNS based phishing. On the other hand, by compromising the DNS server, the genuine IP addresses will be modified which results in taking the user unwillingly to a fake location. The user can fall prey to pharming even when clicking on a legitimate link because the website’s domain name system (DNS) could be hijacked by cybercriminals ( Jakobsson and Myers, 2006 ).
Content Injection Phishing
Content-Injection Phishing refers to inserting false content into a legitimate site. This malicious content could misdirect the user into fake websites, leading users into disclosing their sensitive information to the hacker or it can lead to downloading malware into the user's device ( Jakobsson and Myers, 2006 ). The malicious content could be injected into a legitimate site in three primary ways:
1. Hacker exploits a security vulnerability and compromises a web server.
2. Hacker exploits a Cross-Site Scripting (XSS) vulnerability that is a programming flaw that enables attackers to insert client-side scripts into web pages, which will be viewed by the visitors to the targeted site.
3. Hacker exploits Structured Query Language (SQL) injection vulnerability, which allows hackers to steal information from the website’s database by executing database commands on a remote server.
Man-In-The-Middle Phishing
The Man In The Middle attack (MITM) is a form of phishing, in which the phishers insert communications between two parties (i.e. the user and the legitimate website) and tries to obtain the information from both parties by intercepting the victim’s communications ( Ollmann, 2004 ). Such that the message is going to the attacker instead of going directly to the legitimate recipients. For a MITM, the attacker records the information and misuse it later. The MITM attack conducts by redirecting the user to a malicious server through several techniques such as Address Resolution Protocol (ARP) poisoning, DNS spoofing, Trojan key loggers, and URL Obfuscation ( Jakobsson and Myers, 2006 ).
Search Engine Phishing
In this phishing technique, the phisher creates malicious websites with attractive offers and use Search Engine Optimization (SEO) tactics to have them indexed legitimately such that it appears to the user when searching for products or services. This is also known as black hat SEO ( Jakobsson and Myers, 2006 ).
URL and HTML Obfuscation Attacks
In most of the phishing attacks, phishers aim to convince a user to click on a given link that connects the victim to a malicious phishing server instead of the destination server. This is the most popular technique used by today's phishers. This type of attack is performed by obfuscating the real link (URL) that the user intends to connect (an attempt from the attacker to make their web address look like the legitimate one). Bad Domain Names and Host Name Obfuscation are common methods used by attackers to fake an address ( Ollmann, 2004 ).
Countermeasures
A range of solutions are being discussed and proposed by the researchers to overcome the problems of phishing, but still, there is no single solution that can be trusted or capable of mitigating these attacks ( Hong, 2012 ; Boddy, 2018 ; Chanti and Chithralekha, 2020 ). The proposed phishing countermeasures in the literature can be categorized into three major defense strategies. The first line of defense is human-based solutions by educating end-users to recognize phishing and avoid taking the bait. The second line of defense is technical solutions that involve preventing the attack at early stages such as at the vulnerability level to prevent the threat from materializing at the user's device, which means decreasing the human exposure, and detecting the attack once it is launched through the network level or at the end-user device. This also includes applying specific techniques to track down the source of the attack (for example these could include identification of new domains registered that are closely matched with well-known domain names). The third line of defense is the use of law enforcement as a deterrent control. These approaches can be combined to create much stronger anti-phishing solutions. The above solutions are discussed in detail below.
Human Education (Improving User Awareness About Phishing)
Human education is by far an effective countermeasure to avoid and prevent phishing attacks. Awareness and human training are the first defense approach in the proposed methodology for fighting against phishing even though it does not assume complete protection ( Hong, 2012 ). End-user education reduces user's susceptibility to phishing attacks and compliments other technical solutions. According to the analysis carried out in ( Bailey et al., 2008 ), 95% of phishing attacks are caused due to human errors; nonetheless, existing phishing detection training is not enough for combating current sophisticated attacks. In the study presented by Khonji et al. (2013) , security experts contradict the effectiveness and usability of user education. Furthermore, some security experts claim that user education is not effective as security is not the main goal for users and users do not have a motivation to educate themselves about phishing ( Scaife et al., 2016 ), while others confirm that user education could be effective if designed properly ( Evers, 2006 ; Whitman and Mattord, 2012 ). Moreover, user training has been mentioned by many researchers as an effective way to protect users when they are using online services ( Dodge et al., 2007 ; Salem et al., 2010 ; Chanti and Chithralekha, 2020 ). To detect and avoid phishing emails, a combined training approach was proposed by authors in the study ( Salem et al., 2010 ). The proposed solution uses a combination of tools and human learning, wherein a security awareness program is introduced to the user as a first step. The second step is using an intelligent system that detects the attacks at the email level. After that, the emails are classified by a fuzzy logic-based expert system. The main critic of this method is that the study chooses only limited characteristics of the emails as distinguishing features ( Kumaraguru et al., 2010 ; CybintCyberSolutions, 2018 ). Moreover, the majority of phishing training programs focus on how to recognize and avoid phishing emails and websites while other threatening phishing types receive less attention such as voice phishing and malware or adware phishing. The authors in ( Salem et al., 2010 ) found that the most used solutions in educating people are not useful if they ignore the notifications/warnings about fake websites. Training users should involve three major directions: the first one is awareness training through holding seminars or online courses for both employees within organizations or individuals. The second one is using mock phishing attacks to attack people to test users’ vulnerability and allow them to assess their own knowledge about phishing. However, only 38% of global organizations claim they are prepared to handle a sophisticated cyber-attack ( Kumaraguru et al., 2010 ). Wombat Security’s State of the Phish™ Report 2018 showed that approximately two-fifths of American companies use computer-based online awareness training and simulated phishing attacks as educating tools on a monthly basis, while just 15% of United Kingdom firms do so ( CybintCyberSolutions, 2018 ). The third direction is educating people by developing games to teach people about phishing. The game developer should take into consideration different aspects before designing the game such as audience age and gender, because people's susceptibility to phishing is varying. Authors in the study ( Sheng et al., 2007 ) developed a game to train users so that they can identify phishing attacks called Anti-Phishing Phil that teaches about phishing web pages, and then tests users about the efficiency and effectiveness of the game. The results from the study showed that the game participants improve their ability to identify phishing by 61% indicating that interactive games might turn out to be a joyful way of educating people. Although, user’s education and training can be very effective to mitigate security threats, phishing is becoming more complex and cybercriminals can fool even the security experts by creating convincing spear phishing emails via social media. Therefore, individual users and employees must have at least basic knowledge about dealing with suspicious emails and report it to IT staff and specific authorities. In addition, phishers change their strategies continuously, which makes it harder for organizations, especially small/medium enterprises to afford the cost of their employee education. With millions of people logging on to their social media accounts every day, social media phishing is phishers' favorite medium to deceive their victims. For example, phishers are taking advantage of the pervasiveness of Facebook to set up creative phishing attacks utilizing the Facebook Login feature that enables the phisher to compromise all the user's accounts with the same credentials (VadeSecure). Some countermeasures are taken by Social networks to reduce suspicious activities on social media such as Two-Factor authentication for logging in, that is required by Facebook, and machine-learning techniques used by Snapchat to detect and prevent suspicious links sent within the app ( Corrata, 2018 ). However, countermeasures to control Soshing and phone phishing attacks might include:
• Install anti-virus, anti-spam software as a first action and keep it up to date to detect and prevent any unauthorized access.
• Educate yourself about recent information on phishing, the latest trends, and countermeasures.
• Never click on hyperlinks attached to a suspicious email, post, tweet, direct message.
• Never trust social media, do not give any sensitive information over the phone or non-trusted account. Do not accept friend requests from people you do not know.
• Use a unique password for each account.
Training and educating users is an effective anti-phishing countermeasure and has already shown promising initial results. The main downside of this solution is that it demands high costs ( Dodge et al., 2007 ). Moreover, this solution requires basic knowledge in computer security among trained users.
Technical Solutions
The proposed technical solutions for detecting and blocking phishing attacks can be divided into two major approaches: non-content based solutions and content-based solutions ( Le et al., 2006 ; Bin et al., 2010 ; Boddy, 2018 ). Both approaches are briefly described in this section. Non-content based methods include blacklists and whitelists that classify the fake emails or webpages based on the information that is not part of the email or the webpage such as URL and domain name features ( Dodge et al., 2007 ; Ma et al., 2009 ; Bin et al., 2010 ; Salem et al., 2010 ). Stopping the phishing sites using blacklist and whitelist approaches, wherein a list of known URLs and sites is maintained, the website under scrutiny is checked against such a list in order to be classified as a phishing or legitimate site. The downside of this approach is that it will not identify all phishing websites. Because once a phishing site is taken down, the phisher can easily register a new domain ( Miyamoto et al., 2009 ). Content-based methods classify the page or the email relying on the information within its content such as texts, images, and also HTML, java scripts, and Cascading Style Sheets (CSS) codes ( Zhang et al., 2007 ; Maurer and Herzner, 2012 ). Content-based solutions involve Machine Learning (ML), heuristics, visual similarity, and image processing methods ( Miyamoto et al., 2009 ; Chanti and Chithralekha, 2020 ). and finally, multifaceted methods, which apply a combination of the previous approaches to detect and prevent phishing attacks ( Afroz and Greenstadt, 2009 ). For email filtering, ML techniques are commonly used for example in 2007, the first email phishing filter was developed by authors in ( Fette et al., 2007 ). This technique uses a set of features such as URLs that use different domain names. Spam filtering techniques ( Cormack et al., 2011 ) and statistical classifiers ( Bergholz et al., 2010 ) are also used to identify a phishing email. Authentication and verification technologies are also used in spam email filtering as an alternative to heuristics methods. For example, the Sender Policy Framework (SPF) verifies whether a sender is valid when accepting mail from a remote mail server or email client ( Deshmukh and raddha Popat, 2017 ).
The technical solutions for Anti-phishing are available at different levels of the delivery chain such as mail servers and clients, Internet Service Providers (ISPs), and web browser tools. Drawing from the proposed anatomy for phishing attacks in Proposed Phishing Anatomy , authors categorize technical solutions into the following approaches:
1. Techniques to detect the attack after it has been launched. Such as by scanning the web to find fake websites. For example, content-based phishing detection approaches are heavily deployed on the Internet. The features from the website elements such as Image, URL, and text content are analyzed using Rule-based approaches and Machine Learning that examine the presence of special characters (@), IP addresses instead of the domain name, prefix/suffix, HTTPS in domain part and other features ( Jeeva and Rajsingh, 2016 ). Fuzzy Logic (FL) has also been used as an anti-phishing model to help classify websites into legitimate or ‘phishy’ as this model deals with intervals rather than specific numeric values ( Aburrous et al., 2008 ).
2. Techniques to prevent the attack from reaching the user's system. Phishing prevention is an important step to defend against phishing by blocking a user from seeing and dealing with the attack. In email phishing, anti-spam software tools can block suspicious emails. Phishers usually send a genuine look-alike email that dupes the user to open an attachment or click on a link. Some of these emails pass the spam filter because phishers use misspelled words. Therefore, techniques that detect fake emails by checking the spelling and grammar correction are increasingly used, so that it can prevent the email from reaching the user's mailbox. Authors in the study ( Fette et al., 2007 ) have developed a new classification algorithm based on the Random Forest algorithm after exploring email phishing utilizing the C4.5 decision tree generator algorithm. The developed method is called "Phishing Identification by Learning on Features of Email Received" (PILFER), which can classify phishing email depending on various features such as IP based URLs, the number of links in the HTML part(s) of an email, the number of domains, the number of dots, nonmatching URLs, and availability of JavaScripts. The developed method showed high accuracy in detecting phishing emails ( Afroz and Greenstadt, 2009 ).
3. Corrective techniques that can take down the compromised website, by requesting the website's Internet Service Provider (ISP) to shut down the fake website in order to prevent more users from falling victims to phishing ( Moore and Clayton, 2007 ; Chanti and Chithralekha, 2020 ). ISPs are responsible for taking down fake websites. Removing the compromised and illegal websites is a complex process; many entities are involved in this process from private companies, self-regulatory bodies, government agencies, volunteer organizations, law enforcement, and service providers. Usually, illegal websites are taken down by Takedown Orders, which are issued by courts or in some jurisdictions by law enforcement. On the other hand, these can be voluntarily taken down by the providers themselves as a result of issued takedown notices ( Moore and Clayton, 2007 ; Hutchings et al., 2016 ). According to PHISHLABS ( PhishLabs, 2019 ) report, taking down phishing sites is helpful but it is not completely effective as these sites can still be alive for days stealing customers' credentials before detecting the attack.
4. Warning tools or security indicators that embedded into the web browser to inform the user after detecting the attack. For example, eBay Toolbar and Account Guard ( eBay Toolbar and Account Guard, 2009 ) protect customer’s eBay and PayPal passwords respectively by alerting the users about the authenticity of the sites that users try to type the password in. Numerous anti-phishing solutions rely mainly on warnings that are displayed on the security toolbar. In addition, some toolbars block suspicious sites to warn about it such as McAfee and Netscape. A study presented in ( Robichaux and Ganger, 2006 ) conducted a test to evaluate the performance of eight anti-phishing solutions, including Microsoft Internet Explorer 7, EarthLink, eBay, McAfee, GeoTrust, Google using Firefox, Netscape, and Netcraft. These tools are warning and blocking tools that allow legitimate sites while block and warn about known phishing sites. The study also found that Internet Explorer and Netcraft Toolbar showed the most effective results than other anti-phishing tools. However, security toolbars are still failing to avoid people falling victim to phishing despite these toolbars improving internet security in general ( Abu-Nimeh and Nair, 2008 ).
5. Authentication ( Moore and Clayton, 2007 ) and authorization ( Hutchings et al., 2016 ) techniques that provide protection from phishing by verifying the identity of the legitimate person. This prevents phishers from accessing a protected resource and conducting their attack. There are three types of authentication; single-factor authentication requires only username and password. The second type is two-factor authentication that requires additional information in addition to the username and password such as an OTP (One-Time Password) which is sent to the user’s email id or phone. The third type is multi-factor authentication using more than one form of identity (i.e., a combination of something you know, something you are, and something you have). Some widely used methods in the authorization process are API authorization and OAuth 2.0 that allow the previously generated API to access the system.
However, the progressive increase in phishing attacks shows that previous methods do not provide the required protection against most existing phishing attacks. Because no single solution or technology could prevent all phishing attacks. An effective anti-phishing solution should be based on a combination of technical solutions and increased user awareness ( Boddy, 2018 ).
Solutions Provided by Legislations as a Deterrent Control
A cyber-attack is considered a crime when an individual intentionally accesses personal information on a computer without permission, even if the individual does not steal information or damage the system ( Mince-Didier, 2020 ). Since the sole objective of almost all phishing attacks is to obtain sensitive information by knowingly intending to commit identity theft, and while there are currently no federal laws in the United States aimed specifically at phishing, therefore, phishing crimes are usually covered under identity theft laws. Phishing is considered a crime even if the victim does not actually fall for the phishing scam, the punishments depend on circumstances and usually include jail, fines, restitution, probation ( Nathan, 2020 ). Phishing attacks are causing different levels of damages to the victims such as financial and reputational losses. Therefore, law enforcement authorities should track down these attacks in order to punish the criminal as with real-world crimes. As a complement to technical solutions and human education, the support provided by applicable laws and regulations can play a vital role as a deterrent control. Increasingly authorities around the world have created several regulations in order to mitigate the increase of phishing attacks and their impact. The first anti-phishing laws were enacted by the United States, where the FTC in the US added the phishing attacks to the computer crime list in January 2004. A year later, the ‘‘Anti-Phishing Act’’ was introduced in the US Congress in March 2005 ( Mohammad et al., 2014 ). Meanwhile, in the United Kingdom, the law legislation is gradually conforming to address phishing and other forms of cyber-crime. In 2006, the United Kingdom government improved the Computer Misuse Act 1990 intending to bring it up to date with developments in computer crime and to increase penalties for breach enacted penalties of up to 10 years ( eBay Toolbar and Account Guard, 2009 ; PhishLabs, 2019 ). In this regard, a student in the United Kingdom who made hundreds of thousands of pounds blackmailing pornography website users was jailed in April 2019 for six years and five months. According to the National Crime Agency (NCA), this attacker was the most prolific cybercriminal to be sentenced in the United Kingdom ( Casciani, 2019 ). Moreover, the organizations bear part of the responsibility in protecting personal information as stated in the Data Protection Act 2018 and EU General Data Protection Regulation (GDPR). Phishing websites also can be taken down through Law enforcement agencies' conduct. In the United Kingdom, websites can be taken down by the National Crime Agency (NCA), which includes the National Cyber Crime Unit, and by the City of London Police, which includes the Police Intellectual Property Crime Unit (PIPCU) and the National Fraud Intelligence Bureau (NFIB) ( Hutchings et al., 2016 ).
However, anti-phishing law enforcement is still facing numerous challenges and limitations. Firstly, after perpetrating the phishing attack, the phisher can vanish in cyberspace making it difficult to prove the guilt attributed to the offender and to recover the damages caused by the attack, limiting the effectiveness of the law enforcement role. Secondly, even if the attacker’s identity is disclosed in the case of international attackers, it will be difficult to bring this attacker to justice because of the differences in countries' legislations (e.g., exchange treaties). Also, the attack could be conducted within a short time span, for instance, the average lifetime for a phishing web site is about 54 h as stated by the APWG, therefore, there must be a quick response from the government and the authorities to detect, control and identify the perpetrators of the attack ( Ollmann, 2004 ).
Phishing attacks remain one of the major threats to individuals and organizations to date. As highlighted in the article, this is mainly driven by human involvement in the phishing cycle. Often phishers exploit human vulnerabilities in addition to favoring technological conditions (i.e., technical vulnerabilities). It has been identified that age, gender, internet addiction, user stress, and many other attributes affect the susceptibility to phishing between people. In addition to traditional phishing channels (e.g., email and web), new types of phishing mediums such as voice and SMS phishing are on the increase. Furthermore, the use of social media-based phishing has increased in use in parallel with the growth of social media. Concomitantly, phishing has developed beyond obtaining sensitive information and financial crimes to cyber terrorism, hacktivism, damaging reputations, espionage, and nation-state attacks. Research has been conducted to identify the motivations and techniques and countermeasures to these new crimes, however, there is no single solution for the phishing problem due to the heterogeneous nature of the attack vector. This article has investigated problems presented by phishing and proposed a new anatomy, which describes the complete life cycle of phishing attacks. This anatomy provides a wider outlook for phishing attacks and provides an accurate definition covering end-to-end exclusion and realization of the attack.
Although human education is the most effective defense for phishing, it is difficult to remove the threat completely due to the sophistication of the attacks and social engineering elements. Although, continual security awareness training is the key to avoid phishing attacks and to reduce its impact, developing efficient anti-phishing techniques that prevent users from being exposed to the attack is an essential step in mitigating these attacks. To this end, this article discussed the importance of developing anti-phishing techniques that detect/block the attack. Furthermore, the importance of techniques to determine the source of the attack could provide a stronger anti-phishing solution as discussed in this article.
Furthermore, this article identified the importance of law enforcement as a deterrent mechanism. Further investigations and research are necessary as discussed below.
1. Further research is necessary to study and investigate susceptibility to phishing among users, which would assist in designing stronger and self-learning anti-phishing security systems.
2. Research on social media-based phishing, Voice Phishing, and SMS Phishing is sparse and these emerging threats are predicted to be significantly increased over the next years.
3. Laws and legislations that apply for phishing are still at their infant stage, in fact, there are no specific phishing laws in many countries. Most of the phishing attacks are covered under traditional criminal laws such as identity theft and computer crimes. Therefore, drafting of specific laws for phishing is an important step in mitigating these attacks in a time where these crimes are becoming more common.
4. Determining the source of the attack before the end of the phishing lifecycle and enforcing law legislation on the offender could help in restricting phishing attacks drastically and would benefit from further research.
It can be observed that the mediums used for phishing attacks have changed from traditional emails to social media-based phishing. There is a clear lag between sophisticated phishing attacks and existing countermeasures. The emerging countermeasures should be multidimensional to tackle both human and technical elements of the attack. This article provides valuable information about current phishing attacks and countermeasures whilst the proposed anatomy provides a clear taxonomy to understand the complete life cycle of phishing.
Author Contributions
This work is by our PhD student ZA supported by her Supervisory Team.
Conflict of Interest
The authors declare that the research was conducted in the absence of any commercial or financial relationships that could be construed as a potential conflict of interest.
AOL America Online
APWG Anti Phishing Working Group Advanced
APRANET Advanced Research Projects Agency Network.
ARP address resolution protocol.
BHO Browser Helper Object
BEC business email compromise
COVID-19 Coronavirus disease 2019
CSS cascading style sheets
DDoS distributed denial of service
DNS Domain Name System
DoS Denial of Service
FTC Federal Trade Commission
FL Fuzzy Logic
HTTPS Hypertext Transfer Protocol Secure
IE Internet Explorer
ICT Information and Communications Technology
IM Instant Message
IT Information Technology
IP Internet Protocol
MITM Man-in-the-Middle
NCA National Crime Agency
NFIB National Fraud Intelligence Bureau
PIPCU Police Intellectual Property Crime Unit
OS Operating Systems
PBX Private Branch Exchange
SMishing Text Message Phishing
SPF Sender Policy Framework
SMTP Simple Mail Transfer Protocol
SMS Short Message Service
Soshing Social Media Phishing
SQL structured query language
URL Uniform Resource Locator
UK United Kingdom
US United States
USB Universal Serial Bus
US-CERT United States Computer Emergency Readiness Team.
Vishing Voice Phishing
VNC Virtual Network Computing
VoIP Voice over Internet Protocol
XSS Cross-Site Scripting
1 Proofpoint is “a leading cybersecurity company that protects organizations’ greatest assets and biggest risks: their people. With an integrated suite of cloud-based solutions”( Proofpoint, 2019b ).
2 APWG Is “the international coalition unifying the global response to cybercrime across industry, government and law-enforcement sectors and NGO communities” ( APWG, 2020 ).
3 CalleR ID is “a telephone facility that displays a caller’s phone number on the recipient's phone device before the call is answered” ( Techpedia, 2021 ).
4 An IPPBX is “a telephone switching system within an enterprise that switches calls between VoIP users on local lines while allowing all users to share a certain number of external phone lines” ( Margaret, 2008 ).
Abad, C. (2005). The economy of phishing: a survey of the operations of the phishing market. First Monday 10, 1–11. doi:10.5210/fm.v10i9.1272
CrossRef Full Text | Google Scholar
Abu-Nimeh, S., and Nair, S. (2008). “Bypassing security toolbars and phishing filters via dns poisoning,” in IEEE GLOBECOM 2008–2008 IEEE global telecommunications conference , New Orleans, LA , November 30–December 2, 2008 ( IEEE) , 1–6. doi:10.1109/GLOCOM.2008.ECP.386
Aburrous, M., Hossain, M. A., Thabatah, F., and Dahal, K. (2008). “Intelligent phishing website detection system using fuzzy techniques,” in 2008 3rd international conference on information and communication technologies: from theory to applications (New York, NY: IEEE , 1–6. doi:10.1109/ICTTA.2008.4530019
Afroz, S., and Greenstadt, R. (2009). “Phishzoo: an automated web phishing detection approach based on profiling and fuzzy matching,” in Proceeding 5th IEEE international conference semantic computing (ICSC) , 1–11.
Google Scholar
Alsharnouby, M., Alaca, F., and Chiasson, S. (2015). Why phishing still works: user strategies for combating phishing attacks. Int. J. Human-Computer Stud. 82, 69–82. doi:10.1016/j.ijhcs.2015.05.005
APWG (2018). Phishing activity trends report 3rd quarter 2018 . US. 1–11.
APWG (2020). APWG phishing attack trends reports. 2020 anti-phishing work. Group, Inc Available at: https://apwg.org/trendsreports/ (Accessed September 20, 2020).
Arachchilage, N. A. G., and Love, S. (2014). Security awareness of computer users: a phishing threat avoidance perspective. Comput. Hum. Behav. 38, 304–312. doi:10.1016/j.chb.2014.05.046
Arnsten, B. A., Mazure, C. M., and April, R. S. (2012). Everyday stress can shut down the brain’s chief command center. Sci. Am. 306, 1–6. Available at: https://www.scientificamerican.com/article/this-is-your-brain-in-meltdown/ (Accessed October 15, 2019).
Bailey, J. L., Mitchell, R. B., and Jensen, B. k. (2008). “Analysis of student vulnerabilities to phishing,” in 14th americas conference on information systems, AMCIS 2008 , 75–84. Available at: https://aisel.aisnet.org/amcis2008/271 .
Barracuda (2020). Business email compromise (BEC). Available at: https://www.barracuda.com/glossary/business-email-compromise (Accessed November 15, 2020).
Belcic, I. (2020). Rootkits defined: what they do, how they work, and how to remove them. Available at: https://www.avast.com/c-rootkit (Accessed November 7, 2020).
Bergholz, A., De Beer, J., Glahn, S., Moens, M.-F., Paaß, G., and Strobel, S. (2010). New filtering approaches for phishing email. JCS 18, 7–35. doi:10.3233/JCS-2010-0371
Bin, S., Qiaoyan, W., and Xiaoying, L. (2010). “A DNS based anti-phishing approach.” in 2010 second international conference on networks security, wireless communications and trusted computing , Wuhan, China , April 24–25, 2010 . ( IEEE ), 262–265. doi:10.1109/NSWCTC.2010.196
Boddy, M. (2018). Phishing 2.0: the new evolution in cybercrime. Comput. Fraud Secur. 2018, 8–10. doi:10.1016/S1361-3723(18)30108-8
Casciani, D. (2019). Zain Qaiser: student jailed for blackmailing porn users worldwide. Available at: https://www.bbc.co.uk/news/uk-47800378 (Accessed April 9, 2019).
Chanti, S., and Chithralekha, T. (2020). Classification of anti-phishing solutions. SN Comput. Sci. 1, 11. doi:10.1007/s42979-019-0011-2
Checkpoint (2020). Check point research’s Q1 2020 brand phishing report. Available at: https://www.checkpoint.com/press/2020/apple-is-most-imitated-brand-for-phishing-attempts-check-point-researchs-q1-2020-brand-phishing-report/ (Accessed August 6, 2020).
cisco (2018). What is the difference: viruses, worms, Trojans, and bots? Available at: https://www.cisco.com/c/en/us/about/security-center/virus-differences.html (Accessed January 20, 2020).
CISA (2018). What is phishing. Available at: https://www.us-cert.gov/report-phishing (Accessed June 10, 2019).
Cormack, G. V., Smucker, M. D., and Clarke, C. L. A. (2011). Efficient and effective spam filtering and re-ranking for large web datasets. Inf. Retrieval 14, 441–465. doi:10.1007/s10791-011-9162-z
Corrata (2018). The rising threat of social media phishing attacks. Available at: https://corrata.com/the-rising-threat-of-social-media-phishing-attacks/%0D (Accessed October 29, 2019).
Crane, C. (2019). The dirty dozen: the 12 most costly phishing attack examples. Available at: https://www.thesslstore.com/blog/the-dirty-dozen-the-12-most-costly-phishing-attack-examples/#:∼:text=At some level%2C everyone is susceptible to phishing,outright trick you into performing a particular task (Accessed August 2, 2020).
CSI Onsite (2012). Phishing. Available at: http://csionsite.com/2012/phishing/ (Accessed May 8, 2019).
Cui, Q., Jourdan, G.-V., Bochmann, G. V., Couturier, R., and Onut, I.-V. (2017). Tracking phishing attacks over time. Proc. 26th Int. Conf. World Wide Web - WWW ’17 , Republic and Canton of Geneva, Switzerland: International World Wide Web Conferences Steering Committee . 667–676. doi:10.1145/3038912.3052654
CVEdetails (2005). Vulnerability in microsoft internet explorer. Available at: https://www.cvedetails.com/cve/CVE-2005-4089/ (Accessed August 20, 2019).
Cybint Cyber Solutions (2018). 13 alarming cyber security facts and stats. Available at: https://www.cybintsolutions.com/cyber-security-facts-stats/ (Accessed July 20, 2019).
Deshmukh, M., and raddha Popat, S. (2017). Different techniques for detection of phishing attack. Int. J. Eng. Sci. Comput. 7, 10201–10204. Available at: http://ijesc.org/ .
Dhamija, R., Tygar, J. D., and Hearst, M. (2006). “Why phishing works,” in Proceedings of the SIGCHI conference on human factors in computing systems - CHI ’06 , Montréal Québec, Canada , (New York, NY: ACM Press ), 581. doi:10.1145/1124772.1124861
Diaz, A., Sherman, A. T., and Joshi, A. (2020). Phishing in an academic community: a study of user susceptibility and behavior. Cryptologia 44, 53–67. doi:10.1080/01611194.2019.1623343
Dodge, R. C., Carver, C., and Ferguson, A. J. (2007). Phishing for user security awareness. Comput. Security 26, 73–80. doi:10.1016/j.cose.2006.10.009
eBay Toolbar and Account Guard (2009). Available at: https://download.cnet.com/eBay-Toolbar/3000-12512_4-10153544.html (Accessed August 7, 2020).
EDUCBA (2017). Hackers vs crackers: easy to understand exclusive difference. Available at: https://www.educba.com/hackers-vs-crackers/ (Accessed July 17, 2019).
Evers, J. (2006). Security expert: user education is pointless. Available at: https://www.cnet.com/news/security-expert-user-education-is-pointless/ (Accessed June 25, 2019).
F5Networks (2018). Panda malware broadens targets to cryptocurrency exchanges and social media. Available at: https://www.f5.com/labs/articles/threat-intelligence/panda-malware-broadens-targets-to-cryptocurrency-exchanges-and-social-media (Accessed April 23, 2019).
Fette, I., Sadeh, N., and Tomasic, A. (2007). “Learning to detect phishing emails,” in Proceedings of the 16th international conference on world wide web - WWW ’07 , Banff Alberta, Canada , (New York, NY: ACM Press) , 649–656. doi:10.1145/1242572.1242660
Financial Fraud Action UK (2017). Fraud the facts 2017: the definitive overview of payment industry fraud. London. Available at: https://www.financialfraudaction.org.uk/fraudfacts17/assets/fraud_the_facts.pdf .
Fraud Watch International (2019). Phishing attack trends for 2019. Available at: https://fraudwatchinternational.com/phishing/phishing-attack-trends-for-2019/ (Accessed October 29, 2019).
FTC (2018). Netflix scam email. Available at: https://www.ftc.gov/tips-advice/business-center/small-businesses/cybersecurity/phishing (Accessed May 8, 2019).
Furnell, S. (2007). An assessment of website password practices). Comput. Secur. 26, 445–451. doi:10.1016/j.cose.2007.09.001
Getsafeonline (2017). Caught on the net. Available at: https://www.getsafeonline.org/news/caught-on-the-net/%0D (Accessed August 1, 2020).
GOV.UK (2020). Cyber security breaches survey 2020. Available at: https://www.gov.uk/government/publications/cyber-security-breaches-survey-2020/cyber-security-breaches-survey-2020 (Accessed August 6, 2020).
Gupta, P., Srinivasan, B., Balasubramaniyan, V., and Ahamad, M. (2015). “Phoneypot: data-driven understanding of telephony threats,” in Proceedings 2015 network and distributed system security symposium , (Reston, VA: Internet Society ), 8–11. doi:10.14722/ndss.2015.23176
Hadlington, L. (2017). Human factors in cybersecurity; examining the link between internet addiction, impulsivity, attitudes towards cybersecurity, and risky cybersecurity behaviours. Heliyon 3, e00346-18. doi:10.1016/j.heliyon.2017.e00346
Herley, C., and Florêncio, D. (2008). “A profitless endeavor,” in New security paradigms workshop (NSPW ’08) , New Hampshire, United States , October 25–28, 2021 , 1–12. doi:10.1145/1595676.1595686
Hewage, C. (2020). Coronavirus pandemic has unleashed a wave of cyber attacks – here’s how to protect yourself. Conversat . Available at: https://theconversation.com/coronavirus-pandemic-has-unleashed-a-wave-of-cyber-attacks-heres-how-to-protect-yourself-135057 (Accessed November 16, 2020).
Hong, J. (2012). The state of phishing attacks. Commun. ACM 55, 74–81. doi:10.1145/2063176.2063197
Huber, M., Kowalski, S., Nohlberg, M., and Tjoa, S. (2009). “Towards automating social engineering using social networking sites,” in 2009 international conference on computational science and engineering , Vancouver, BC , August 29–31, 2009 ( IEEE , 117–124. doi:10.1109/CSE.2009.205
Hutchings, A., Clayton, R., and Anderson, R. (2016). “Taking down websites to prevent crime,” in 2016 APWG symposium on electronic crime research (eCrime) ( IEEE ), 1–10. doi:10.1109/ECRIME.2016.7487947
Iuga, C., Nurse, J. R. C., and Erola, A. (2016). Baiting the hook: factors impacting susceptibility to phishing attacks. Hum. Cent. Comput. Inf. Sci. 6, 8. doi:10.1186/s13673-016-0065-2
Jagatic, T. N., Johnson, N. A., Jakobsson, M., and Menczer, F. (2007). Social phishing. Commun. ACM 50, 94–100. doi:10.1145/1290958.1290968
Jakobsson, M., and Myers, S. (2006). Phishing and countermeasures: understanding the increasing problems of electronic identity theft . New Jersey: John Wiley and Sons .
Jakobsson, M., Tsow, A., Shah, A., Blevis, E., and Lim, Y. K. (2007). “What instills trust? A qualitative study of phishing,” in Lecture notes in computer science (including subseries lecture notes in artificial intelligence and lecture notes in bioinformatics) , (Berlin, Heidelberg: Springer ), 356–361. doi:10.1007/978-3-540-77366-5_32
Jeeva, S. C., and Rajsingh, E. B. (2016). Intelligent phishing url detection using association rule mining. Hum. Cent. Comput. Inf. Sci. 6, 10. doi:10.1186/s13673-016-0064-3
Johnson, A. (2016). Almost 600 accounts breached in “celebgate” nude photo hack, FBI says. Available at: http://www.cnbc.com/id/102747765 (Accessed: February 17, 2020).
Kayne, R. (2019). What are script kiddies? Wisegeek. Available at: https://www.wisegeek.com/what-are-script-kiddies.htm V V February 19, 2020).
Keck, C. (2018). FTC warns of sketchy Netflix phishing scam asking for payment details. Available at: https://gizmodo.com/ftc-warns-of-sketchy-netflix-phishing-scam-asking-for-p-1831372416 (Accessed April 23, 2019).
Keepnet LABS (2018). Statistical analysis of 126,000 phishing simulations carried out in 128 companies around the world. USA, France. Available at: www.keepnetlabs.com .
Keinan, G. (1987). Decision making under stress: scanning of alternatives under controllable and uncontrollable threats. J. Personal. Soc. Psychol. 52, 639–644. doi:10.1037/0022-3514.52.3.639
Khonji, M., Iraqi, Y., and Jones, A. (2013). Phishing detection: a literature survey. IEEE Commun. Surv. Tutorials 15, 2091–2121. doi:10.1109/SURV.2013.032213.00009
Kirda, E., and Kruegel, C. (2005). Protecting users against phishing attacks with AntiPhish. Proc. - Int. Comput. Softw. Appl. Conf. 1, 517–524. doi:10.1109/COMPSAC.2005.126
Krawchenko, K. (2016). The phishing email that hacked the account of John Podesta. CBSNEWS Available at: https://www.cbsnews.com/news/the-phishing-email-that-hacked-the-account-of-john-podesta/ (Accessed April 13, 2019).
Ksepersky (2020). Spam and phishing in Q1 2020. Available at: https://securelist.com/spam-and-phishing-in-q1-2020/97091/ (Accessed July 27, 2020).
Kumaraguru, P., Sheng, S., Acquisti, A., Cranor, L. F., and Hong, J. (2010). Teaching Johnny not to fall for phish. ACM Trans. Internet Technol. 10, 1–31. doi:10.1145/1754393.1754396
Latto, N. (2020). What is adware and how can you prevent it? Avast. Available at: https://www.avast.com/c-adware (Accessed May 8, 2020).
Le, D., Fu, X., and Hogrefe, D. (2006). A review of mobility support paradigms for the internet. IEEE Commun. Surv. Tutorials 8, 38–51. doi:10.1109/COMST.2006.323441
Lehman, T. J., and Vajpayee, S. (2011). “We’ve looked at clouds from both sides now,” in 2011 annual SRII global conference , San Jose, CA , March 20–April 2, 2011 , ( IEEE , 342–348. doi:10.1109/SRII.2011.46
Leyden, J. (2001). Virus toolkits are s’kiddie menace. Regist . Available at: https://www.theregister.co.uk/2001/02/21/virus_toolkits_are_skiddie_menace/%0D (Accessed June 15, 2019).
Lin, J., Sadeh, N., Amini, S., Lindqvist, J., Hong, J. I., and Zhang, J. (2012). “Expectation and purpose,” in Proceedings of the 2012 ACM conference on ubiquitous computing - UbiComp ’12 (New York, New York, USA: ACM Press ), 1625. doi:10.1145/2370216.2370290
Lininger, R., and Vines, D. R. (2005). Phishing: cutting the identity theft line. Print book . Indiana: Wiley Publishing, Inc .
Ma, J., Saul, L. K., Savage, S., and Voelker, G. M. (2009). “Identifying suspicious URLs.” in Proceedings of the 26th annual international conference on machine learning - ICML ’09 (New York, NY: ACM Press ), 1–8. doi:10.1145/1553374.1553462
Marforio, C., Masti, R. J., Soriente, C., Kostiainen, K., and Capkun, S. (2015). Personalized security indicators to detect application phishing attacks in mobile platforms. Available at: http://arxiv.org/abs/1502.06824 .
Margaret, R. I. P. (2008). PBX (private branch exchange). Available at: https://searchunifiedcommunications.techtarget.com/definition/IP-PBX (Accessed June 19, 2019).
Maurer, M.-E., and Herzner, D. (2012). Using visual website similarity for phishing detection and reporting. 1625–1630. doi:10.1145/2212776.2223683
Medvet, E., Kirda, E., and Kruegel, C. (2008). “Visual-similarity-based phishing detection,” in Proceedings of the 4th international conference on Security and privacy in communication netowrks - SecureComm ’08 (New York, NY: ACM Press ), 1. doi:10.1145/1460877.1460905
Merwe, A. v. d., Marianne, L., and Marek, D. (2005). “Characteristics and responsibilities involved in a Phishing attack, in WISICT ’05: proceedings of the 4th international symposium on information and communication technologies . Trinity College Dublin , 249–254.
Microsoft (2020). Exploiting a crisis: how cybercriminals behaved during the outbreak. Available at: https://www.microsoft.com/security/blog/2020/06/16/exploiting-a-crisis-how-cybercriminals-behaved-during-the-outbreak/ (Accessed August 1, 2020).
Mince-Didier, A. (2020). Hacking a computer or computer network. Available at: https://www.criminaldefenselawyer.com/resources/hacking-computer.html (Accessed August 7, 2020).
Miyamoto, D., Hazeyama, H., and Kadobayashi, Y. (2009). “An evaluation of machine learning-based methods for detection of phishing sites,” in international conference on neural information processing ICONIP 2008: advances in neuro-information processing lecture notes in computer science . Editors M. Köppen, N. Kasabov, and G. Coghill (Berlin, Heidelberg: Springer Berlin Heidelberg ), 539–546. doi:10.1007/978-3-642-02490-0_66
Mohammad, R. M., Thabtah, F., and McCluskey, L. (2014). Predicting phishing websites based on self-structuring neural network. Neural Comput. Applic 25, 443–458. doi:10.1007/s00521-013-1490-z
Moore, T., and Clayton, R. (2007). “Examining the impact of website take-down on phishing,” in Proceedings of the anti-phishing working groups 2nd annual eCrime researchers summit on - eCrime ’07 (New York, NY: ACM Press ), 1–13. doi:10.1145/1299015.1299016
Morgan, S. (2019). 2019 official annual cybercrime report. USA, UK, Canada. Available at: https://www.herjavecgroup.com/wp-content/uploads/2018/12/CV-HG-2019-Official-Annual-Cybercrime-Report.pdf .
Nathan, G. (2020). What is phishing? + laws, charges & statute of limitations. Available at: https://www.federalcharges.com/phishing-laws-charges/ (Accessed August 7, 2020).
Okin, S. (2009). From script kiddies to organised cybercrime. Available at: https://comsecglobal.com/from-script-kiddies-to-organised-cybercrime-things-are-getting-nasty-out-there/ (Accessed August 12, 2019).
Ollmann, G. (2004). The phishing guide understanding & preventing phishing attacks abstract. USA. Available at: http://www.ngsconsulting.com .
Ong, S. (2014). Avast survey shows men more susceptible to mobile malware. Available at: https://www.mirekusoft.com/avast-survey-shows-men-more-susceptible-to-mobile-malware/ (Accessed November 5, 2020).
Ovelgönne, M., Dumitraş, T., Prakash, B. A., Subrahmanian, V. S., and Wang, B. (2017). Understanding the relationship between human behavior and susceptibility to cyber attacks. ACM Trans. Intell. Syst. Technol. 8, 1–25. doi:10.1080/00207284.1985.11491413
Parmar, B. (2012). Protecting against spear-phishing. Computer Fraud Security , 2012, 8–11. doi:10.1016/S1361-3723(12)70007-6
Phish Labs (2019). 2019 phishing trends and intelligence report the growing social engineering threat. Available at: https://info.phishlabs.com/hubfs/2019 PTI Report/2019 Phishing Trends and Intelligence Report.pdf .
PhishMe (2016). Q1 2016 malware review. Available at: WWW.PHISHME.COM .
PhishMe (2017). Human phishing defense enterprise phishing resiliency and defense report 2017 analysis of susceptibility, resiliency and defense against simulated and real phishing attacks. Available at: https://cofense.com/wp-content/uploads/2017/11/Enterprise-Phishing-Resiliency-and-Defense-Report-2017.pdf .
PishTank (2006). What is phishing. Available at: http://www.phishtank.com/what_is_phishing.php?view=website&annotated=true (Accessed June 19, 2019).
Pompon, A. R., Walkowski, D., and Boddy, S. (2018). Phishing and Fraud Report attacks peak during the holidays. US .
Proofpoint (2019a). State of the phish 2019 report. Sport Mark. Q. 14, 4. doi:10.1038/sj.jp.7211019
Proofpoint (2019b). What is Proofpoint. Available at: https://www.proofpoint.com/us/company/about (Accessed September 25, 2019).
Proofpoint (2020). 2020 state of the phish. Available at: https://www.proofpoint.com/sites/default/files/gtd-pfpt-us-tr-state-of-the-phish-2020.pdf .
Raggo, M. (2016). Anatomy of a social media attack. Available at: https://www.darkreading.com/analytics/anatomy-of-a-social-media-attack/a/d-id/1326680 (Accessed March 14, 2019).
Ramanathan, V., and Wechsler, H. (2012). PhishGILLNET-phishing detection methodology using probabilistic latent semantic analysis, AdaBoost, and co-training. EURASIP J. Info. Secur. 2012, 1–22. doi:10.1186/1687-417X-2012-1
Ramzan, Z. (2010). “Phishing attacks and countermeasures,” in Handbook of Information and communication security (Berlin, Heidelberg: Springer Berlin Heidelberg ), 433–448. doi:10.1007/978-3-642-04117-4_23
Ramzan, Z., and Wuest, C. (2007). “Phishing Attacks: analyzing trends in 2006,” in Fourth conference on email and anti-Spam (Mountain View , ( California, United States ).
Rhett, J. (2019). Don’t fall for this new Google translate phishing attack. Available at: https://www.gizmodo.co.uk/2019/02/dont-fall-for-this-new-google-translate-phishing-attack/ (Accessed April 23, 2019). doi:10.5040/9781350073272
RISKIQ (2020). Investigate | COVID-19 cybercrime weekly update. Available at: https://www.riskiq.com/blog/analyst/covid19-cybercrime-update/%0D (Accessed August 1, 2020).
Robichaux, P., and Ganger, D. L. (2006). Gone phishing: evaluating anti-phishing tools for windows. Available at: http://www.3sharp.com/projects/antiphishing/gonephishing.pdf .
Rouse, M. (2013). Phishing defintion. Available at: https://searchsecurity.techtarget.com/definition/phishing (Accessed April 10, 2019).
Salem, O., Hossain, A., and Kamala, M. (2010). “Awareness program and AI based tool to reduce risk of phishing attacks,” in 2010 10th IEEE international conference on computer and information technology (IEEE) , Bradford, United Kingdom , June 29–July 1, 2010, 2001 ( IEEE ), 1418–1423. doi:10.1109/CIT.2010.254
Scaife, N., Carter, H., Traynor, P., and Butler, K. R. B. (2016). “Crypto lock (and drop it): stopping ransomware attacks on user data,” in 2016 IEEE 36th international conference on distributed computing systems (ICDCS) ( IEEE , 303–312. doi:10.1109/ICDCS.2016.46
Sheng, S., Magnien, B., Kumaraguru, P., Acquisti, A., Cranor, L. F., Hong, J., et al. (2007). “Anti-Phishing Phil: the design and evaluation of a game that teaches people not to fall for phish,” in Proceedings of the 3rd symposium on usable privacy and security - SOUPS ’07 (New York, NY: ACM Press ), 88–99. doi:10.1145/1280680.1280692
Symantic, (2019). Internet security threat report volume 24|February 2019 . USA.
Techpedia (2021). Caller ID. Available at: https://www.techopedia.com/definition/24222/caller-id (Accessed June 19, 2019).
VadeSecure (2021). Phishers favorites 2019. Available at: https://www.vadesecure.com/en/ (Accessed October 29, 2019).
Vishwanath, A. (2005). “Spear phishing: the tip of the spear used by cyber terrorists,” in deconstruction machines (United States: University of Minnesota Press ), 469–484. doi:10.4018/978-1-5225-0156-5.ch023
Wang, X., Zhang, R., Yang, X., Jiang, X., and Wijesekera, D. (2008). “Voice pharming attack and the trust of VoIP,” in Proceedings of the 4th international conference on security and privacy in communication networks, SecureComm’08 , 1–11. doi:10.1145/1460877.1460908
Wenyin, L., Huang, G., Xiaoyue, L., Min, Z., and Deng, X. (2005). “Detection of phishing webpages based on visual similarity,” in 14th international world wide web conference, WWW2005 , Chiba, Japan , May 10–14, 2005 , 1060–1061. doi:10.1145/1062745.1062868
Whitman, M. E., and Mattord, H. J. (2012). Principles of information security. Course Technol. 1–617. doi:10.1016/B978-0-12-381972-7.00002-6
Williams, E. J., Hinds, J., and Joinson, A. N. (2018). Exploring susceptibility to phishing in the workplace. Int. J. Human-Computer Stud. 120, 1–13. doi:10.1016/j.ijhcs.2018.06.004
wombatsecurity.com (2018). Wombat security user risk report. USA. Available at: https://info.wombatsecurity.com/hubfs/WombatProofpoint-UserRiskSurveyReport2018_US.pdf .
Workman, M. (2008). Wisecrackers: a theory-grounded investigation of phishing and pretext social engineering threats to information security. J. Am. Soc. Inf. Sci. 59 (4), 662–674. doi:10.1002/asi.20779
Yeboah-Boateng, E. O., and Amanor, P. M. (2014). Phishing , SMiShing & vishing: an assessment of threats against mobile devices. J. Emerg. Trends Comput. Inf. Sci. 5 (4), 297–307.
Zhang, Y., Hong, J. I., and Cranor, L. F. (2007). “Cantina,” in Proceedings of the 16th international conference on World Wide Web - WWW ’07 (New York, NY: ACM Press ), 639. doi:10.1145/1242572.1242659
Zissis, D., and Lekkas, D. (2012). Addressing cloud computing security issues. Future Generat. Comput. Syst. 28, 583–592. doi:10.1016/j.future.2010.12.006
Keywords: phishing anatomy, precautionary countermeasures, phishing targets, phishing attack mediums, phishing attacks, attack phases, phishing techniques
Citation: Alkhalil Z, Hewage C, Nawaf L and Khan I (2021) Phishing Attacks: A Recent Comprehensive Study and a New Anatomy. Front. Comput. Sci. 3:563060. doi: 10.3389/fcomp.2021.563060
Received: 17 May 2020; Accepted: 18 January 2021; Published: 09 March 2021.
Reviewed by:
Copyright © 2021 Alkhalil, Hewage, Nawaf and Khan. This is an open-access article distributed under the terms of the Creative Commons Attribution License (CC BY). The use, distribution or reproduction in other forums is permitted, provided the original author(s) and the copyright owner(s) are credited and that the original publication in this journal is cited, in accordance with accepted academic practice. No use, distribution or reproduction is permitted which does not comply with these terms.
*Correspondence: Chaminda Hewage, [email protected]
Disclaimer: All claims expressed in this article are solely those of the authors and do not necessarily represent those of their affiliated organizations, or those of the publisher, the editors and the reviewers. Any product that may be evaluated in this article or claim that may be made by its manufacturer is not guaranteed or endorsed by the publisher.
IEEE Account
- Change Username/Password
- Update Address
Purchase Details
- Payment Options
- Order History
- View Purchased Documents
Profile Information
- Communications Preferences
- Profession and Education
- Technical Interests
- US & Canada: +1 800 678 4333
- Worldwide: +1 732 981 0060
- Contact & Support
- About IEEE Xplore
- Accessibility
- Terms of Use
- Nondiscrimination Policy
- Privacy & Opting Out of Cookies
A not-for-profit organization, IEEE is the world's largest technical professional organization dedicated to advancing technology for the benefit of humanity. © Copyright 2024 IEEE - All rights reserved. Use of this web site signifies your agreement to the terms and conditions.

An official website of the United States government
The .gov means it’s official. Federal government websites often end in .gov or .mil. Before sharing sensitive information, make sure you’re on a federal government site.
The site is secure. The https:// ensures that you are connecting to the official website and that any information you provide is encrypted and transmitted securely.
- Publications
- Account settings
Preview improvements coming to the PMC website in October 2024. Learn More or Try it out now .
- Advanced Search
- Journal List
- Springer Nature - PMC COVID-19 Collection

Cyber risk and cybersecurity: a systematic review of data availability
Frank cremer.
1 University of Limerick, Limerick, Ireland
Barry Sheehan
Michael fortmann.
2 TH Köln University of Applied Sciences, Cologne, Germany
Arash N. Kia
Martin mullins, finbarr murphy, stefan materne, associated data.
Cybercrime is estimated to have cost the global economy just under USD 1 trillion in 2020, indicating an increase of more than 50% since 2018. With the average cyber insurance claim rising from USD 145,000 in 2019 to USD 359,000 in 2020, there is a growing necessity for better cyber information sources, standardised databases, mandatory reporting and public awareness. This research analyses the extant academic and industry literature on cybersecurity and cyber risk management with a particular focus on data availability. From a preliminary search resulting in 5219 cyber peer-reviewed studies, the application of the systematic methodology resulted in 79 unique datasets. We posit that the lack of available data on cyber risk poses a serious problem for stakeholders seeking to tackle this issue. In particular, we identify a lacuna in open databases that undermine collective endeavours to better manage this set of risks. The resulting data evaluation and categorisation will support cybersecurity researchers and the insurance industry in their efforts to comprehend, metricise and manage cyber risks.
Supplementary Information
The online version contains supplementary material available at 10.1057/s41288-022-00266-6.
Introduction
Globalisation, digitalisation and smart technologies have escalated the propensity and severity of cybercrime. Whilst it is an emerging field of research and industry, the importance of robust cybersecurity defence systems has been highlighted at the corporate, national and supranational levels. The impacts of inadequate cybersecurity are estimated to have cost the global economy USD 945 billion in 2020 (Maleks Smith et al. 2020 ). Cyber vulnerabilities pose significant corporate risks, including business interruption, breach of privacy and financial losses (Sheehan et al. 2019 ). Despite the increasing relevance for the international economy, the availability of data on cyber risks remains limited. The reasons for this are many. Firstly, it is an emerging and evolving risk; therefore, historical data sources are limited (Biener et al. 2015 ). It could also be due to the fact that, in general, institutions that have been hacked do not publish the incidents (Eling and Schnell 2016 ). The lack of data poses challenges for many areas, such as research, risk management and cybersecurity (Falco et al. 2019 ). The importance of this topic is demonstrated by the announcement of the European Council in April 2021 that a centre of excellence for cybersecurity will be established to pool investments in research, technology and industrial development. The goal of this centre is to increase the security of the internet and other critical network and information systems (European Council 2021 ).
This research takes a risk management perspective, focusing on cyber risk and considering the role of cybersecurity and cyber insurance in risk mitigation and risk transfer. The study reviews the existing literature and open data sources related to cybersecurity and cyber risk. This is the first systematic review of data availability in the general context of cyber risk and cybersecurity. By identifying and critically analysing the available datasets, this paper supports the research community by aggregating, summarising and categorising all available open datasets. In addition, further information on datasets is attached to provide deeper insights and support stakeholders engaged in cyber risk control and cybersecurity. Finally, this research paper highlights the need for open access to cyber-specific data, without price or permission barriers.
The identified open data can support cyber insurers in their efforts on sustainable product development. To date, traditional risk assessment methods have been untenable for insurance companies due to the absence of historical claims data (Sheehan et al. 2021 ). These high levels of uncertainty mean that cyber insurers are more inclined to overprice cyber risk cover (Kshetri 2018 ). Combining external data with insurance portfolio data therefore seems to be essential to improve the evaluation of the risk and thus lead to risk-adjusted pricing (Bessy-Roland et al. 2021 ). This argument is also supported by the fact that some re/insurers reported that they are working to improve their cyber pricing models (e.g. by creating or purchasing databases from external providers) (EIOPA 2018 ). Figure 1 provides an overview of pricing tools and factors considered in the estimation of cyber insurance based on the findings of EIOPA ( 2018 ) and the research of Romanosky et al. ( 2019 ). The term cyber risk refers to all cyber risks and their potential impact.

An overview of the current cyber insurance informational and methodological landscape, adapted from EIOPA ( 2018 ) and Romanosky et al. ( 2019 )
Besides the advantage of risk-adjusted pricing, the availability of open datasets helps companies benchmark their internal cyber posture and cybersecurity measures. The research can also help to improve risk awareness and corporate behaviour. Many companies still underestimate their cyber risk (Leong and Chen 2020 ). For policymakers, this research offers starting points for a comprehensive recording of cyber risks. Although in many countries, companies are obliged to report data breaches to the respective supervisory authority, this information is usually not accessible to the research community. Furthermore, the economic impact of these breaches is usually unclear.
As well as the cyber risk management community, this research also supports cybersecurity stakeholders. Researchers are provided with an up-to-date, peer-reviewed literature of available datasets showing where these datasets have been used. For example, this includes datasets that have been used to evaluate the effectiveness of countermeasures in simulated cyberattacks or to test intrusion detection systems. This reduces a time-consuming search for suitable datasets and ensures a comprehensive review of those available. Through the dataset descriptions, researchers and industry stakeholders can compare and select the most suitable datasets for their purposes. In addition, it is possible to combine the datasets from one source in the context of cybersecurity or cyber risk. This supports efficient and timely progress in cyber risk research and is beneficial given the dynamic nature of cyber risks.
Cyber risks are defined as “operational risks to information and technology assets that have consequences affecting the confidentiality, availability, and/or integrity of information or information systems” (Cebula et al. 2014 ). Prominent cyber risk events include data breaches and cyberattacks (Agrafiotis et al. 2018 ). The increasing exposure and potential impact of cyber risk have been highlighted in recent industry reports (e.g. Allianz 2021 ; World Economic Forum 2020 ). Cyberattacks on critical infrastructures are ranked 5th in the World Economic Forum's Global Risk Report. Ransomware, malware and distributed denial-of-service (DDoS) are examples of the evolving modes of a cyberattack. One example is the ransomware attack on the Colonial Pipeline, which shut down the 5500 mile pipeline system that delivers 2.5 million barrels of fuel per day and critical liquid fuel infrastructure from oil refineries to states along the U.S. East Coast (Brower and McCormick 2021 ). These and other cyber incidents have led the U.S. to strengthen its cybersecurity and introduce, among other things, a public body to analyse major cyber incidents and make recommendations to prevent a recurrence (Murphey 2021a ). Another example of the scope of cyberattacks is the ransomware NotPetya in 2017. The damage amounted to USD 10 billion, as the ransomware exploited a vulnerability in the windows system, allowing it to spread independently worldwide in the network (GAO 2021 ). In the same year, the ransomware WannaCry was launched by cybercriminals. The cyberattack on Windows software took user data hostage in exchange for Bitcoin cryptocurrency (Smart 2018 ). The victims included the National Health Service in Great Britain. As a result, ambulances were redirected to other hospitals because of information technology (IT) systems failing, leaving people in need of urgent assistance waiting. It has been estimated that 19,000 cancelled treatment appointments resulted from losses of GBP 92 million (Field 2018 ). Throughout the COVID-19 pandemic, ransomware attacks increased significantly, as working from home arrangements increased vulnerability (Murphey 2021b ).
Besides cyberattacks, data breaches can also cause high costs. Under the General Data Protection Regulation (GDPR), companies are obliged to protect personal data and safeguard the data protection rights of all individuals in the EU area. The GDPR allows data protection authorities in each country to impose sanctions and fines on organisations they find in breach. “For data breaches, the maximum fine can be €20 million or 4% of global turnover, whichever is higher” (GDPR.EU 2021 ). Data breaches often involve a large amount of sensitive data that has been accessed, unauthorised, by external parties, and are therefore considered important for information security due to their far-reaching impact (Goode et al. 2017 ). A data breach is defined as a “security incident in which sensitive, protected, or confidential data are copied, transmitted, viewed, stolen, or used by an unauthorized individual” (Freeha et al. 2021 ). Depending on the amount of data, the extent of the damage caused by a data breach can be significant, with the average cost being USD 392 million 1 (IBM Security 2020 ).
This research paper reviews the existing literature and open data sources related to cybersecurity and cyber risk, focusing on the datasets used to improve academic understanding and advance the current state-of-the-art in cybersecurity. Furthermore, important information about the available datasets is presented (e.g. use cases), and a plea is made for open data and the standardisation of cyber risk data for academic comparability and replication. The remainder of the paper is structured as follows. The next section describes the related work regarding cybersecurity and cyber risks. The third section outlines the review method used in this work and the process. The fourth section details the results of the identified literature. Further discussion is presented in the penultimate section and the final section concludes.
Related work
Due to the significance of cyber risks, several literature reviews have been conducted in this field. Eling ( 2020 ) reviewed the existing academic literature on the topic of cyber risk and cyber insurance from an economic perspective. A total of 217 papers with the term ‘cyber risk’ were identified and classified in different categories. As a result, open research questions are identified, showing that research on cyber risks is still in its infancy because of their dynamic and emerging nature. Furthermore, the author highlights that particular focus should be placed on the exchange of information between public and private actors. An improved information flow could help to measure the risk more accurately and thus make cyber risks more insurable and help risk managers to determine the right level of cyber risk for their company. In the context of cyber insurance data, Romanosky et al. ( 2019 ) analysed the underwriting process for cyber insurance and revealed how cyber insurers understand and assess cyber risks. For this research, they examined 235 American cyber insurance policies that were publicly available and looked at three components (coverage, application questionnaires and pricing). The authors state in their findings that many of the insurers used very simple, flat-rate pricing (based on a single calculation of expected loss), while others used more parameters such as the asset value of the company (or company revenue) or standard insurance metrics (e.g. deductible, limits), and the industry in the calculation. This is in keeping with Eling ( 2020 ), who states that an increased amount of data could help to make cyber risk more accurately measured and thus more insurable. Similar research on cyber insurance and data was conducted by Nurse et al. ( 2020 ). The authors examined cyber insurance practitioners' perceptions and the challenges they face in collecting and using data. In addition, gaps were identified during the research where further data is needed. The authors concluded that cyber insurance is still in its infancy, and there are still several unanswered questions (for example, cyber valuation, risk calculation and recovery). They also pointed out that a better understanding of data collection and use in cyber insurance would be invaluable for future research and practice. Bessy-Roland et al. ( 2021 ) come to a similar conclusion. They proposed a multivariate Hawkes framework to model and predict the frequency of cyberattacks. They used a public dataset with characteristics of data breaches affecting the U.S. industry. In the conclusion, the authors make the argument that an insurer has a better knowledge of cyber losses, but that it is based on a small dataset and therefore combination with external data sources seems essential to improve the assessment of cyber risks.
Several systematic reviews have been published in the area of cybersecurity (Kruse et al. 2017 ; Lee et al. 2020 ; Loukas et al. 2013 ; Ulven and Wangen 2021 ). In these papers, the authors concentrated on a specific area or sector in the context of cybersecurity. This paper adds to this extant literature by focusing on data availability and its importance to risk management and insurance stakeholders. With a priority on healthcare and cybersecurity, Kruse et al. ( 2017 ) conducted a systematic literature review. The authors identified 472 articles with the keywords ‘cybersecurity and healthcare’ or ‘ransomware’ in the databases Cumulative Index of Nursing and Allied Health Literature, PubMed and Proquest. Articles were eligible for this review if they satisfied three criteria: (1) they were published between 2006 and 2016, (2) the full-text version of the article was available, and (3) the publication is a peer-reviewed or scholarly journal. The authors found that technological development and federal policies (in the U.S.) are the main factors exposing the health sector to cyber risks. Loukas et al. ( 2013 ) conducted a review with a focus on cyber risks and cybersecurity in emergency management. The authors provided an overview of cyber risks in communication, sensor, information management and vehicle technologies used in emergency management and showed areas for which there is still no solution in the literature. Similarly, Ulven and Wangen ( 2021 ) reviewed the literature on cybersecurity risks in higher education institutions. For the literature review, the authors used the keywords ‘cyber’, ‘information threats’ or ‘vulnerability’ in connection with the terms ‘higher education, ‘university’ or ‘academia’. A similar literature review with a focus on Internet of Things (IoT) cybersecurity was conducted by Lee et al. ( 2020 ). The review revealed that qualitative approaches focus on high-level frameworks, and quantitative approaches to cybersecurity risk management focus on risk assessment and quantification of cyberattacks and impacts. In addition, the findings presented a four-step IoT cyber risk management framework that identifies, quantifies and prioritises cyber risks.
Datasets are an essential part of cybersecurity research, underlined by the following works. Ilhan Firat et al. ( 2021 ) examined various cybersecurity datasets in detail. The study was motivated by the fact that with the proliferation of the internet and smart technologies, the mode of cyberattacks is also evolving. However, in order to prevent such attacks, they must first be detected; the dissemination and further development of cybersecurity datasets is therefore critical. In their work, the authors observed studies of datasets used in intrusion detection systems. Khraisat et al. ( 2019 ) also identified a need for new datasets in the context of cybersecurity. The researchers presented a taxonomy of current intrusion detection systems, a comprehensive review of notable recent work, and an overview of the datasets commonly used for assessment purposes. In their conclusion, the authors noted that new datasets are needed because most machine-learning techniques are trained and evaluated on the knowledge of old datasets. These datasets do not contain new and comprehensive information and are partly derived from datasets from 1999. The authors noted that the core of this issue is the availability of new public datasets as well as their quality. The availability of data, how it is used, created and shared was also investigated by Zheng et al. ( 2018 ). The researchers analysed 965 cybersecurity research papers published between 2012 and 2016. They created a taxonomy of the types of data that are created and shared and then analysed the data collected via datasets. The researchers concluded that while datasets are recognised as valuable for cybersecurity research, the proportion of publicly available datasets is limited.
The main contributions of this review and what differentiates it from previous studies can be summarised as follows. First, as far as we can tell, it is the first work to summarise all available datasets on cyber risk and cybersecurity in the context of a systematic review and present them to the scientific community and cyber insurance and cybersecurity stakeholders. Second, we investigated, analysed, and made available the datasets to support efficient and timely progress in cyber risk research. And third, we enable comparability of datasets so that the appropriate dataset can be selected depending on the research area.
Methodology
Process and eligibility criteria.
The structure of this systematic review is inspired by the Preferred Reporting Items for Systematic Reviews and Meta-Analyses (PRISMA) framework (Page et al. 2021 ), and the search was conducted from 3 to 10 May 2021. Due to the continuous development of cyber risks and their countermeasures, only articles published in the last 10 years were considered. In addition, only articles published in peer-reviewed journals written in English were included. As a final criterion, only articles that make use of one or more cybersecurity or cyber risk datasets met the inclusion criteria. Specifically, these studies presented new or existing datasets, used them for methods, or used them to verify new results, as well as analysed them in an economic context and pointed out their effects. The criterion was fulfilled if it was clearly stated in the abstract that one or more datasets were used. A detailed explanation of this selection criterion can be found in the ‘Study selection’ section.
Information sources
In order to cover a complete spectrum of literature, various databases were queried to collect relevant literature on the topic of cybersecurity and cyber risks. Due to the spread of related articles across multiple databases, the literature search was limited to the following four databases for simplicity: IEEE Xplore, Scopus, SpringerLink and Web of Science. This is similar to other literature reviews addressing cyber risks or cybersecurity, including Sardi et al. ( 2021 ), Franke and Brynielsson ( 2014 ), Lagerström (2019), Eling and Schnell ( 2016 ) and Eling ( 2020 ). In this paper, all databases used in the aforementioned works were considered. However, only two studies also used all the databases listed. The IEEE Xplore database contains electrical engineering, computer science, and electronics work from over 200 journals and three million conference papers (IEEE 2021 ). Scopus includes 23,400 peer-reviewed journals from more than 5000 international publishers in the areas of science, engineering, medicine, social sciences and humanities (Scopus 2021 ). SpringerLink contains 3742 journals and indexes over 10 million scientific documents (SpringerLink 2021 ). Finally, Web of Science indexes over 9200 journals in different scientific disciplines (Science 2021 ).
A search string was created and applied to all databases. To make the search efficient and reproducible, the following search string with Boolean operator was used in all databases: cybersecurity OR cyber risk AND dataset OR database. To ensure uniformity of the search across all databases, some adjustments had to be made for the respective search engines. In Scopus, for example, the Advanced Search was used, and the field code ‘Title-ABS-KEY’ was integrated into the search string. For IEEE Xplore, the search was carried out with the Search String in the Command Search and ‘All Metadata’. In the Web of Science database, the Advanced Search was used. The special feature of this search was that it had to be carried out in individual steps. The first search was carried out with the terms cybersecurity OR cyber risk with the field tag Topic (T.S. =) and the second search with dataset OR database. Subsequently, these searches were combined, which then delivered the searched articles for review. For SpringerLink, the search string was used in the Advanced Search under the category ‘Find the resources with all of the words’. After conducting this search string, 5219 studies could be found. According to the eligibility criteria (period, language and only scientific journals), 1581 studies were identified in the databases:
- Scopus: 135
- Springer Link: 548
- Web of Science: 534
An overview of the process is given in Fig. 2 . Combined with the results from the four databases, 854 articles without duplicates were identified.

Literature search process and categorisation of the studies
Study selection
In the final step of the selection process, the articles were screened for relevance. Due to a large number of results, the abstracts were analysed in the first step of the process. The aim was to determine whether the article was relevant for the systematic review. An article fulfilled the criterion if it was recognisable in the abstract that it had made a contribution to datasets or databases with regard to cyber risks or cybersecurity. Specifically, the criterion was considered to be met if the abstract used datasets that address the causes or impacts of cyber risks, and measures in the area of cybersecurity. In this process, the number of articles was reduced to 288. The articles were then read in their entirety, and an expert panel of six people decided whether they should be used. This led to a final number of 255 articles. The years in which the articles were published and the exact number can be seen in Fig. 3 .

Distribution of studies
Data collection process and synthesis of the results
For the data collection process, various data were extracted from the studies, including the names of the respective creators, the name of the dataset or database and the corresponding reference. It was also determined where the data came from. In the context of accessibility, it was determined whether access is free, controlled, available for purchase or not available. It was also determined when the datasets were created and the time period referenced. The application type and domain characteristics of the datasets were identified.
This section analyses the results of the systematic literature review. The previously identified studies are divided into three categories: datasets on the causes of cyber risks, datasets on the effects of cyber risks and datasets on cybersecurity. The classification is based on the intended use of the studies. This system of classification makes it easier for stakeholders to find the appropriate datasets. The categories are evaluated individually. Although complete information is available for a large proportion of datasets, this is not true for all of them. Accordingly, the abbreviation N/A has been inserted in the respective characters to indicate that this information could not be determined by the time of submission. The term ‘use cases in the literature’ in the following and supplementary tables refers to the application areas in which the corresponding datasets were used in the literature. The areas listed there refer to the topic area on which the researchers conducted their research. Since some datasets were used interdisciplinarily, the listed use cases in the literature are correspondingly longer. Before discussing each category in the next sections, Fig. 4 provides an overview of the number of datasets found and their year of creation. Figure 5 then shows the relationship between studies and datasets in the period under consideration. Figure 6 shows the distribution of studies, their use of datasets and their creation date. The number of datasets used is higher than the number of studies because the studies often used several datasets (Table (Table1). 1 ).

Distribution of dataset results

Correlation between the studies and the datasets

Distribution of studies and their use of datasets
Percentage contribution of datasets for each place of origin
Rank | Place of origin | Percentage of datasets |
---|---|---|
1 | U.S. | 58.2 |
2 | Canada | 11.3 |
3 | Australia | 5 |
4 | Germany | 3.7 |
5 | U.K. | 3.7 |
6 | France | 2.5 |
7 | Italy | 2.5 |
8 | Spain | 2.5 |
9 | China | 1.2 |
10 | Czech Republic | 1.2 |
11 | Greece | 1.2 |
12 | Japan | 1.2 |
13 | Lithuania | 1.2 |
14 | Luxembourg | 1.2 |
15 | Netherlands | 1.2 |
16 | Republic of Korea | 1.2 |
17 | Turkey | 1.2 |
Most of the datasets are generated in the U.S. (up to 58.2%). Canada and Australia rank next, with 11.3% and 5% of all the reviewed datasets, respectively.
Additionally, to create value for the datasets for the cyber insurance industry, an assessment of the applicability of each dataset has been provided for cyber insurers. This ‘Use Case Assessment’ includes the use of the data in the context of different analyses, calculation of cyber insurance premiums, and use of the information for the design of cyber insurance contracts or for additional customer services. To reasonably account for the transition of direct hyperlinks in the future, references were directed to the main websites for longevity (nearest resource point). In addition, the links to the main pages contain further information on the datasets and different versions related to the operating systems. The references were chosen in such a way that practitioners get the best overview of the respective datasets.
Case datasets
This section presents selected articles that use the datasets to analyse the causes of cyber risks. The datasets help identify emerging trends and allow pattern discovery in cyber risks. This information gives cybersecurity experts and cyber insurers the data to make better predictions and take appropriate action. For example, if certain vulnerabilities are not adequately protected, cyber insurers will demand a risk surcharge leading to an improvement in the risk-adjusted premium. Due to the capricious nature of cyber risks, existing data must be supplemented with new data sources (for example, new events, new methods or security vulnerabilities) to determine prevailing cyber exposure. The datasets of cyber risk causes could be combined with existing portfolio data from cyber insurers and integrated into existing pricing tools and factors to improve the valuation of cyber risks.
A portion of these datasets consists of several taxonomies and classifications of cyber risks. Aassal et al. ( 2020 ) propose a new taxonomy of phishing characteristics based on the interpretation and purpose of each characteristic. In comparison, Hindy et al. ( 2020 ) presented a taxonomy of network threats and the impact of current datasets on intrusion detection systems. A similar taxonomy was suggested by Kiwia et al. ( 2018 ). The authors presented a cyber kill chain-based taxonomy of banking Trojans features. The taxonomy built on a real-world dataset of 127 banking Trojans collected from December 2014 to January 2016 by a major U.K.-based financial organisation.
In the context of classification, Aamir et al. ( 2021 ) showed the benefits of machine learning for classifying port scans and DDoS attacks in a mixture of normal and attack traffic. Guo et al. ( 2020 ) presented a new method to improve malware classification based on entropy sequence features. The evaluation of this new method was conducted on different malware datasets.
To reconstruct attack scenarios and draw conclusions based on the evidence in the alert stream, Barzegar and Shajari ( 2018 ) use the DARPA2000 and MACCDC 2012 dataset for their research. Giudici and Raffinetti ( 2020 ) proposed a rank-based statistical model aimed at predicting the severity levels of cyber risk. The model used cyber risk data from the University of Milan. In contrast to the previous datasets, Skrjanc et al. ( 2018 ) used the older dataset KDD99 to monitor large-scale cyberattacks using a cauchy clustering method.
Amin et al. ( 2021 ) used a cyberattack dataset from the Canadian Institute for Cybersecurity to identify spatial clusters of countries with high rates of cyberattacks. In the context of cybercrime, Junger et al. ( 2020 ) examined crime scripts, key characteristics of the target company and the relationship between criminal effort and financial benefit. For their study, the authors analysed 300 cases of fraudulent activities against Dutch companies. With a similar focus on cybercrime, Mireles et al. ( 2019 ) proposed a metric framework to measure the effectiveness of the dynamic evolution of cyberattacks and defensive measures. To validate its usefulness, they used the DEFCON dataset.
Due to the rapidly changing nature of cyber risks, it is often impossible to obtain all information on them. Kim and Kim ( 2019 ) proposed an automated dataset generation system called CTIMiner that collects threat data from publicly available security reports and malware repositories. They released a dataset to the public containing about 640,000 records from 612 security reports published between January 2008 and 2019. A similar approach is proposed by Kim et al. ( 2020 ), using a named entity recognition system to extract core information from cyber threat reports automatically. They created a 498,000-tag dataset during their research (Ulven and Wangen 2021 ).
Within the framework of vulnerabilities and cybersecurity issues, Ulven and Wangen ( 2021 ) proposed an overview of mission-critical assets and everyday threat events, suggested a generic threat model, and summarised common cybersecurity vulnerabilities. With a focus on hospitality, Chen and Fiscus ( 2018 ) proposed several issues related to cybersecurity in this sector. They analysed 76 security incidents from the Privacy Rights Clearinghouse database. Supplementary Table 1 lists all findings that belong to the cyber causes dataset.
Impact datasets
This section outlines selected findings of the cyber impact dataset. For cyber insurers, these datasets can form an important basis for information, as they can be used to calculate cyber insurance premiums, evaluate specific cyber risks, formulate inclusions and exclusions in cyber wordings, and re-evaluate as well as supplement the data collected so far on cyber risks. For example, information on financial losses can help to better assess the loss potential of cyber risks. Furthermore, the datasets can provide insight into the frequency of occurrence of these cyber risks. The new datasets can be used to close any data gaps that were previously based on very approximate estimates or to find new results.
Eight studies addressed the costs of data breaches. For instance, Eling and Jung ( 2018 ) reviewed 3327 data breach events from 2005 to 2016 and identified an asymmetric dependence of monthly losses by breach type and industry. The authors used datasets from the Privacy Rights Clearinghouse for analysis. The Privacy Rights Clearinghouse datasets and the Breach level index database were also used by De Giovanni et al. ( 2020 ) to describe relationships between data breaches and bitcoin-related variables using the cointegration methodology. The data were obtained from the Department of Health and Human Services of healthcare facilities reporting data breaches and a national database of technical and organisational infrastructure information. Also in the context of data breaches, Algarni et al. ( 2021 ) developed a comprehensive, formal model that estimates the two components of security risks: breach cost and the likelihood of a data breach within 12 months. For their survey, the authors used two industrial reports from the Ponemon institute and VERIZON. To illustrate the scope of data breaches, Neto et al. ( 2021 ) identified 430 major data breach incidents among more than 10,000 incidents. The database created is available and covers the period 2018 to 2019.
With a direct focus on insurance, Biener et al. ( 2015 ) analysed 994 cyber loss cases from an operational risk database and investigated the insurability of cyber risks based on predefined criteria. For their study, they used data from the company SAS OpRisk Global Data. Similarly, Eling and Wirfs ( 2019 ) looked at a wide range of cyber risk events and actual cost data using the same database. They identified cyber losses and analysed them using methods from statistics and actuarial science. Using a similar reference, Farkas et al. ( 2021 ) proposed a method for analysing cyber claims based on regression trees to identify criteria for classifying and evaluating claims. Similar to Chen and Fiscus ( 2018 ), the dataset used was the Privacy Rights Clearinghouse database. Within the framework of reinsurance, Moro ( 2020 ) analysed cyber index-based information technology activity to see if index-parametric reinsurance coverage could suggest its cedant using data from a Symantec dataset.
Paté-Cornell et al. ( 2018 ) presented a general probabilistic risk analysis framework for cybersecurity in an organisation to be specified. The results are distributions of losses to cyberattacks, with and without considered countermeasures in support of risk management decisions based both on past data and anticipated incidents. The data used were from The Common Vulnerability and Exposures database and via confidential access to a database of cyberattacks on a large, U.S.-based organisation. A different conceptual framework for cyber risk classification and assessment was proposed by Sheehan et al. ( 2021 ). This framework showed the importance of proactive and reactive barriers in reducing companies’ exposure to cyber risk and quantifying the risk. Another approach to cyber risk assessment and mitigation was proposed by Mukhopadhyay et al. ( 2019 ). They estimated the probability of an attack using generalised linear models, predicted the security technology required to reduce the probability of cyberattacks, and used gamma and exponential distributions to best approximate the average loss data for each malicious attack. They also calculated the expected loss due to cyberattacks, calculated the net premium that would need to be charged by a cyber insurer, and suggested cyber insurance as a strategy to minimise losses. They used the CSI-FBI survey (1997–2010) to conduct their research.
In order to highlight the lack of data on cyber risks, Eling ( 2020 ) conducted a literature review in the areas of cyber risk and cyber insurance. Available information on the frequency, severity, and dependency structure of cyber risks was filtered out. In addition, open questions for future cyber risk research were set up. Another example of data collection on the impact of cyberattacks is provided by Sornette et al. ( 2013 ), who use a database of newspaper articles, press reports and other media to provide a predictive method to identify triggering events and potential accident scenarios and estimate their severity and frequency. A similar approach to data collection was used by Arcuri et al. ( 2020 ) to gather an original sample of global cyberattacks from newspaper reports sourced from the LexisNexis database. This collection is also used and applied to the fields of dynamic communication and cyber risk perception by Fang et al. ( 2021 ). To create a dataset of cyber incidents and disputes, Valeriano and Maness ( 2014 ) collected information on cyber interactions between rival states.
To assess trends and the scale of economic cybercrime, Levi ( 2017 ) examined datasets from different countries and their impact on crime policy. Pooser et al. ( 2018 ) investigated the trend in cyber risk identification from 2006 to 2015 and company characteristics related to cyber risk perception. The authors used a dataset of various reports from cyber insurers for their study. Walker-Roberts et al. ( 2020 ) investigated the spectrum of risk of a cybersecurity incident taking place in the cyber-physical-enabled world using the VERIS Community Database. The datasets of impacts identified are presented below. Due to overlap, some may also appear in the causes dataset (Supplementary Table 2).
Cybersecurity datasets
General intrusion detection.
General intrusion detection systems account for the largest share of countermeasure datasets. For companies or researchers focused on cybersecurity, the datasets can be used to test their own countermeasures or obtain information about potential vulnerabilities. For example, Al-Omari et al. ( 2021 ) proposed an intelligent intrusion detection model for predicting and detecting attacks in cyberspace, which was applied to dataset UNSW-NB 15. A similar approach was taken by Choras and Kozik ( 2015 ), who used machine learning to detect cyberattacks on web applications. To evaluate their method, they used the HTTP dataset CSIC 2010. For the identification of unknown attacks on web servers, Kamarudin et al. ( 2017 ) proposed an anomaly-based intrusion detection system using an ensemble classification approach. Ganeshan and Rodrigues ( 2020 ) showed an intrusion detection system approach, which clusters the database into several groups and detects the presence of intrusion in the clusters. In comparison, AlKadi et al. ( 2019 ) used a localisation-based model to discover abnormal patterns in network traffic. Hybrid models have been recommended by Bhattacharya et al. ( 2020 ) and Agrawal et al. ( 2019 ); the former is a machine-learning model based on principal component analysis for the classification of intrusion detection system datasets, while the latter is a hybrid ensemble intrusion detection system for anomaly detection using different datasets to detect patterns in network traffic that deviate from normal behaviour.
Agarwal et al. ( 2021 ) used three different machine learning algorithms in their research to find the most suitable for efficiently identifying patterns of suspicious network activity. The UNSW-NB15 dataset was used for this purpose. Kasongo and Sun ( 2020 ), Feed-Forward Deep Neural Network (FFDNN), Keshk et al. ( 2021 ), the privacy-preserving anomaly detection framework, and others also use the UNSW-NB 15 dataset as part of intrusion detection systems. The same dataset and others were used by Binbusayyis and Vaiyapuri ( 2019 ) to identify and compare key features for cyber intrusion detection. Atefinia and Ahmadi ( 2021 ) proposed a deep neural network model to reduce the false positive rate of an anomaly-based intrusion detection system. Fossaceca et al. ( 2015 ) focused in their research on the development of a framework that combined the outputs of multiple learners in order to improve the efficacy of network intrusion, and Gauthama Raman et al. ( 2020 ) presented a search algorithm based on Support Vector machine to improve the performance of the detection and false alarm rate to improve intrusion detection techniques. Ahmad and Alsemmeari ( 2020 ) targeted extreme learning machine techniques due to their good capabilities in classification problems and handling huge data. They used the NSL-KDD dataset as a benchmark.
With reference to prediction, Bakdash et al. ( 2018 ) used datasets from the U.S. Department of Defence to predict cyberattacks by malware. This dataset consists of weekly counts of cyber events over approximately seven years. Another prediction method was presented by Fan et al. ( 2018 ), which showed an improved integrated cybersecurity prediction method based on spatial-time analysis. Also, with reference to prediction, Ashtiani and Azgomi ( 2014 ) proposed a framework for the distributed simulation of cyberattacks based on high-level architecture. Kirubavathi and Anitha ( 2016 ) recommended an approach to detect botnets, irrespective of their structures, based on network traffic flow behaviour analysis and machine-learning techniques. Dwivedi et al. ( 2021 ) introduced a multi-parallel adaptive technique to utilise an adaption mechanism in the group of swarms for network intrusion detection. AlEroud and Karabatis ( 2018 ) presented an approach that used contextual information to automatically identify and query possible semantic links between different types of suspicious activities extracted from network flows.
Intrusion detection systems with a focus on IoT
In addition to general intrusion detection systems, a proportion of studies focused on IoT. Habib et al. ( 2020 ) presented an approach for converting traditional intrusion detection systems into smart intrusion detection systems for IoT networks. To enhance the process of diagnostic detection of possible vulnerabilities with an IoT system, Georgescu et al. ( 2019 ) introduced a method that uses a named entity recognition-based solution. With regard to IoT in the smart home sector, Heartfield et al. ( 2021 ) presented a detection system that is able to autonomously adjust the decision function of its underlying anomaly classification models to a smart home’s changing condition. Another intrusion detection system was suggested by Keserwani et al. ( 2021 ), which combined Grey Wolf Optimization and Particle Swam Optimization to identify various attacks for IoT networks. They used the KDD Cup 99, NSL-KDD and CICIDS-2017 to evaluate their model. Abu Al-Haija and Zein-Sabatto ( 2020 ) provide a comprehensive development of a new intelligent and autonomous deep-learning-based detection and classification system for cyberattacks in IoT communication networks that leverage the power of convolutional neural networks, abbreviated as IoT-IDCS-CNN (IoT-based Intrusion Detection and Classification System using Convolutional Neural Network). To evaluate the development, the authors used the NSL-KDD dataset. Biswas and Roy ( 2021 ) recommended a model that identifies malicious botnet traffic using novel deep-learning approaches like artificial neural networks gutted recurrent units and long- or short-term memory models. They tested their model with the Bot-IoT dataset.
With a more forensic background, Koroniotis et al. ( 2020 ) submitted a network forensic framework, which described the digital investigation phases for identifying and tracing attack behaviours in IoT networks. The suggested work was evaluated with the Bot-IoT and UINSW-NB15 datasets. With a focus on big data and IoT, Chhabra et al. ( 2020 ) presented a cyber forensic framework for big data analytics in an IoT environment using machine learning. Furthermore, the authors mentioned different publicly available datasets for machine-learning models.
A stronger focus on a mobile phones was exhibited by Alazab et al. ( 2020 ), which presented a classification model that combined permission requests and application programme interface calls. The model was tested with a malware dataset containing 27,891 Android apps. A similar approach was taken by Li et al. ( 2019a , b ), who proposed a reliable classifier for Android malware detection based on factorisation machine architecture and extraction of Android app features from manifest files and source code.
Literature reviews
In addition to the different methods and models for intrusion detection systems, various literature reviews on the methods and datasets were also found. Liu and Lang ( 2019 ) proposed a taxonomy of intrusion detection systems that uses data objects as the main dimension to classify and summarise machine learning and deep learning-based intrusion detection literature. They also presented four different benchmark datasets for machine-learning detection systems. Ahmed et al. ( 2016 ) presented an in-depth analysis of four major categories of anomaly detection techniques, which include classification, statistical, information theory and clustering. Hajj et al. ( 2021 ) gave a comprehensive overview of anomaly-based intrusion detection systems. Their article gives an overview of the requirements, methods, measurements and datasets that are used in an intrusion detection system.
Within the framework of machine learning, Chattopadhyay et al. ( 2018 ) conducted a comprehensive review and meta-analysis on the application of machine-learning techniques in intrusion detection systems. They also compared different machine learning techniques in different datasets and summarised the performance. Vidros et al. ( 2017 ) presented an overview of characteristics and methods in automatic detection of online recruitment fraud. They also published an available dataset of 17,880 annotated job ads, retrieved from the use of a real-life system. An empirical study of different unsupervised learning algorithms used in the detection of unknown attacks was presented by Meira et al. ( 2020 ).
New datasets
Kilincer et al. ( 2021 ) reviewed different intrusion detection system datasets in detail. They had a closer look at the UNS-NB15, ISCX-2012, NSL-KDD and CIDDS-001 datasets. Stojanovic et al. ( 2020 ) also provided a review on datasets and their creation for use in advanced persistent threat detection in the literature. Another review of datasets was provided by Sarker et al. ( 2020 ), who focused on cybersecurity data science as part of their research and provided an overview from a machine-learning perspective. Avila et al. ( 2021 ) conducted a systematic literature review on the use of security logs for data leak detection. They recommended a new classification of information leak, which uses the GDPR principles, identified the most widely publicly available dataset for threat detection, described the attack types in the datasets and the algorithms used for data leak detection. Tuncer et al. ( 2020 ) presented a bytecode-based detection method consisting of feature extraction using local neighbourhood binary patterns. They chose a byte-based malware dataset to investigate the performance of the proposed local neighbourhood binary pattern-based detection method. With a different focus, Mauro et al. ( 2020 ) gave an experimental overview of neural-based techniques relevant to intrusion detection. They assessed the value of neural networks using the Bot-IoT and UNSW-DB15 datasets.
Another category of results in the context of countermeasure datasets is those that were presented as new. Moreno et al. ( 2018 ) developed a database of 300 security-related accidents from European and American sources. The database contained cybersecurity-related events in the chemical and process industry. Damasevicius et al. ( 2020 ) proposed a new dataset (LITNET-2020) for network intrusion detection. The dataset is a new annotated network benchmark dataset obtained from the real-world academic network. It presents real-world examples of normal and under-attack network traffic. With a focus on IoT intrusion detection systems, Alsaedi et al. ( 2020 ) proposed a new benchmark IoT/IIot datasets for assessing intrusion detection system-enabled IoT systems. Also in the context of IoT, Vaccari et al. ( 2020 ) proposed a dataset focusing on message queue telemetry transport protocols, which can be used to train machine-learning models. To evaluate the performance of machine-learning classifiers, Mahfouz et al. ( 2020 ) created a dataset called Game Theory and Cybersecurity (GTCS). A dataset containing 22,000 malware and benign samples was constructed by Martin et al. ( 2019 ). The dataset can be used as a benchmark to test the algorithm for Android malware classification and clustering techniques. In addition, Laso et al. ( 2017 ) presented a dataset created to investigate how data and information quality estimates enable the detection of anomalies and malicious acts in cyber-physical systems. The dataset contained various cyberattacks and is publicly available.
In addition to the results described above, several other studies were found that fit into the category of countermeasures. Johnson et al. ( 2016 ) examined the time between vulnerability disclosures. Using another vulnerabilities database, Common Vulnerabilities and Exposures (CVE), Subroto and Apriyana ( 2019 ) presented an algorithm model that uses big data analysis of social media and statistical machine learning to predict cyber risks. A similar databank but with a different focus, Common Vulnerability Scoring System, was used by Chatterjee and Thekdi ( 2020 ) to present an iterative data-driven learning approach to vulnerability assessment and management for complex systems. Using the CICIDS2017 dataset to evaluate the performance, Malik et al. ( 2020 ) proposed a control plane-based orchestration for varied, sophisticated threats and attacks. The same dataset was used in another study by Lee et al. ( 2019 ), who developed an artificial security information event management system based on a combination of event profiling for data processing and different artificial network methods. To exploit the interdependence between multiple series, Fang et al. ( 2021 ) proposed a statistical framework. In order to validate the framework, the authors applied it to a dataset of enterprise-level security breaches from the Privacy Rights Clearinghouse and Identity Theft Center database. Another framework with a defensive aspect was recommended by Li et al. ( 2021 ) to increase the robustness of deep neural networks against adversarial malware evasion attacks. Sarabi et al. ( 2016 ) investigated whether and to what extent business details can help assess an organisation's risk of data breaches and the distribution of risk across different types of incidents to create policies for protection, detection and recovery from different forms of security incidents. They used data from the VERIS Community Database.
Datasets that have been classified into the cybersecurity category are detailed in Supplementary Table 3. Due to overlap, records from the previous tables may also be included.
This paper presented a systematic literature review of studies on cyber risk and cybersecurity that used datasets. Within this framework, 255 studies were fully reviewed and then classified into three different categories. Then, 79 datasets were consolidated from these studies. These datasets were subsequently analysed, and important information was selected through a process of filtering out. This information was recorded in a table and enhanced with further information as part of the literature analysis. This made it possible to create a comprehensive overview of the datasets. For example, each dataset contains a description of where the data came from and how the data has been used to date. This allows different datasets to be compared and the appropriate dataset for the use case to be selected. This research certainly has limitations, so our selection of datasets cannot necessarily be taken as a representation of all available datasets related to cyber risks and cybersecurity. For example, literature searches were conducted in four academic databases and only found datasets that were used in the literature. Many research projects also used old datasets that may no longer consider current developments. In addition, the data are often focused on only one observation and are limited in scope. For example, the datasets can only be applied to specific contexts and are also subject to further limitations (e.g. region, industry, operating system). In the context of the applicability of the datasets, it is unfortunately not possible to make a clear statement on the extent to which they can be integrated into academic or practical areas of application or how great this effort is. Finally, it remains to be pointed out that this is an overview of currently available datasets, which are subject to constant change.
Due to the lack of datasets on cyber risks in the academic literature, additional datasets on cyber risks were integrated as part of a further search. The search was conducted on the Google Dataset search portal. The search term used was ‘cyber risk datasets’. Over 100 results were found. However, due to the low significance and verifiability, only 20 selected datasets were included. These can be found in Table 2 in the “ Appendix ”.
Summary of Google datasets
No | Dataset creator | Name of the dataset | Data availability | Year of creation/start year | Description |
---|---|---|---|---|---|
1 | ActionFraud | Cyber Crime Dashboard | Public | 2020 | Shows cybercrime and fraud reported in the U.K.. |
2 | Carlos E. Jimenez-Gomez | Data Breaches 2004–2017 | Public | 2018 | Includes 270 records and 11 variables of data breaches. The data breaches happened between 2004–2017. Only data breaches with over 30,000 records are considered. |
3 | Chubb | Chubb Cyber Index | Public | 2007 | Shows cyber claims for more than two decades. In this dashboard, there is the possibility to get information about different areas regarding claims cost. Furthermore, it is possible to get an overview of claims of different years. |
4 | CMS | DGDPR Enforcement Tracker | Public | 2018 | An overview of fines and penalties, which data protection authorities within the EU have imposed under the EU GDPR. |
5 | DSGVO Portal | DSGVO—Portal | Public | 2014 | Fines for violations of the GDPR and other data protection laws. |
6 | Federal Bureau of Investigation | Internet Crime Report 2020 | Public | 2021 | Includes the cyber risk impact situation in the U.S.. |
7 | Government of Canada | No name | Public | 2017 | Percentage of enterprises impacted by specific types of cybersecurity incidents by the North American Industry Classification System (NAICS) and size of the enterprise. |
8 | Hiscox | Hisco Cyber Readiness Report 2020 | Public | 2020 | The average cost of all cyberattacks to firms from Europe and the U.S. in 2020, by size, in USD. |
9 | IBM Security | Cost of a Data Breach Report 2020 | Public | 2020 | Includes the cost of data breaches from 2014 to 2020. |
10 | Information is beautiful | World's Biggest Data Breaches & Hacks | Public | 2004 | Selected events over 30,000 records. |
11 | Ipsos Mori | Cyber Security Breaches Survey | Public | 2020 | Displays the share of businesses that have had certain outcomes after experiencing a cybersecurity breach or attack in the last 12 months in the U.K. in 2020 |
12 | Kaspersky | Damage Control: The Cost of Security Breaches | Public | 2020 | Analyses the different data of Kaspersky |
13 | Marsch—Mircosoft—Global Cyber Risk Perception Survey | Marsch—Mircosoft—Global Cyber Risk Perception Survey | Public | 2018 | Presents the greatest potential imp.acts to an organisation due to cyber loss scenarios, according to senior executives |
14 | Mendeley Data | California Data Breach Notification Data | Public | 2019 | An empirical study of security breach notifications filed in California during 2012–2016. |
15 | Norton | 2019 Cyber Safety Insights Report | Public | 2020 | A survey of internet users who have experienced an internet crime. |
16 | Paolo Passeri | Hackmageddon | Access controlled | 2011 | Overview of collected timelines with a focus on cyberattacks. |
17 | Pierangelo and Theo | Data Breach Dataset | Public | 2020 | Consists of 506 data breaches and associated characteristics that affected U.S.-listed companies over a 10-year period from April 2005 to March 2015. The dataset was gathered from the Privacy Rights Clearinghouse (PRC) and then augmented with manual data collection. |
18 | PwC | 2015 Information Security Breaches Survey | Public | 2015 | Illustrates the ranking of what made a particular security breach incident the worst of the year in the U.K. in 2015. |
19 | Spy Cloud | Spy Cloud | Private | - | - |
20 | Willis Towers Watson | Cyber claims analysis report | Public | 2020 | Uses analysed claims data of Willis Towers Watson to provide specific insight. |
The results of the literature review and datasets also showed that there continues to be a lack of available, open cyber datasets. This lack of data is reflected in cyber insurance, for example, as it is difficult to find a risk-based premium without a sufficient database (Nurse et al. 2020 ). The global cyber insurance market was estimated at USD 5.5 billion in 2020 (Dyson 2020 ). When compared to the USD 1 trillion global losses from cybercrime (Maleks Smith et al. 2020 ), it is clear that there exists a significant cyber risk awareness challenge for both the insurance industry and international commerce. Without comprehensive and qualitative data on cyber losses, it can be difficult to estimate potential losses from cyberattacks and price cyber insurance accordingly (GAO 2021 ). For instance, the average cyber insurance loss increased from USD 145,000 in 2019 to USD 359,000 in 2020 (FitchRatings 2021 ). Cyber insurance is an important risk management tool to mitigate the financial impact of cybercrime. This is particularly evident in the impact of different industries. In the Energy & Commodities financial markets, a ransomware attack on the Colonial Pipeline led to a substantial impact on the U.S. economy. As a result of the attack, about 45% of the U.S. East Coast was temporarily unable to obtain supplies of diesel, petrol and jet fuel. This caused the average price in the U.S. to rise 7 cents to USD 3.04 per gallon, the highest in seven years (Garber 2021 ). In addition, Colonial Pipeline confirmed that it paid a USD 4.4 million ransom to a hacker gang after the attack. Another ransomware attack occurred in the healthcare and government sector. The victim of this attack was the Irish Health Service Executive (HSE). A ransom payment of USD 20 million was demanded from the Irish government to restore services after the hack (Tidy 2021 ). In the car manufacturing sector, Miller and Valasek ( 2015 ) initiated a cyberattack that resulted in the recall of 1.4 million vehicles and cost manufacturers EUR 761 million. The risk that arises in the context of these events is the potential for the accumulation of cyber losses, which is why cyber insurers are not expanding their capacity. An example of this accumulation of cyber risks is the NotPetya malware attack, which originated in Russia, struck in Ukraine, and rapidly spread around the world, causing at least USD 10 billion in damage (GAO 2021 ). These events highlight the importance of proper cyber risk management.
This research provides cyber insurance stakeholders with an overview of cyber datasets. Cyber insurers can use the open datasets to improve their understanding and assessment of cyber risks. For example, the impact datasets can be used to better measure financial impacts and their frequencies. These data could be combined with existing portfolio data from cyber insurers and integrated with existing pricing tools and factors to better assess cyber risk valuation. Although most cyber insurers have sparse historical cyber policy and claims data, they remain too small at present for accurate prediction (Bessy-Roland et al. 2021 ). A combination of portfolio data and external datasets would support risk-adjusted pricing for cyber insurance, which would also benefit policyholders. In addition, cyber insurance stakeholders can use the datasets to identify patterns and make better predictions, which would benefit sustainable cyber insurance coverage. In terms of cyber risk cause datasets, cyber insurers can use the data to review their insurance products. For example, the data could provide information on which cyber risks have not been sufficiently considered in product design or where improvements are needed. A combination of cyber cause and cybersecurity datasets can help establish uniform definitions to provide greater transparency and clarity. Consistent terminology could lead to a more sustainable cyber market, where cyber insurers make informed decisions about the level of coverage and policyholders understand their coverage (The Geneva Association 2020).
In addition to the cyber insurance community, this research also supports cybersecurity stakeholders. The reviewed literature can be used to provide a contemporary, contextual and categorised summary of available datasets. This supports efficient and timely progress in cyber risk research and is beneficial given the dynamic nature of cyber risks. With the help of the described cybersecurity datasets and the identified information, a comparison of different datasets is possible. The datasets can be used to evaluate the effectiveness of countermeasures in simulated cyberattacks or to test intrusion detection systems.
In this paper, we conducted a systematic review of studies on cyber risk and cybersecurity databases. We found that most of the datasets are in the field of intrusion detection and machine learning and are used for technical cybersecurity aspects. The available datasets on cyber risks were relatively less represented. Due to the dynamic nature and lack of historical data, assessing and understanding cyber risk is a major challenge for cyber insurance stakeholders. To address this challenge, a greater density of cyber data is needed to support cyber insurers in risk management and researchers with cyber risk-related topics. With reference to ‘Open Science’ FAIR data (Jacobsen et al. 2020 ), mandatory reporting of cyber incidents could help improve cyber understanding, awareness and loss prevention among companies and insurers. Through greater availability of data, cyber risks can be better understood, enabling researchers to conduct more in-depth research into these risks. Companies could incorporate this new knowledge into their corporate culture to reduce cyber risks. For insurance companies, this would have the advantage that all insurers would have the same understanding of cyber risks, which would support sustainable risk-based pricing. In addition, common definitions of cyber risks could be derived from new data.
The cybersecurity databases summarised and categorised in this research could provide a different perspective on cyber risks that would enable the formulation of common definitions in cyber policies. The datasets can help companies addressing cybersecurity and cyber risk as part of risk management assess their internal cyber posture and cybersecurity measures. The paper can also help improve risk awareness and corporate behaviour, and provides the research community with a comprehensive overview of peer-reviewed datasets and other available datasets in the area of cyber risk and cybersecurity. This approach is intended to support the free availability of data for research. The complete tabulated review of the literature is included in the Supplementary Material.
This work provides directions for several paths of future work. First, there are currently few publicly available datasets for cyber risk and cybersecurity. The older datasets that are still widely used no longer reflect today's technical environment. Moreover, they can often only be used in one context, and the scope of the samples is very limited. It would be of great value if more datasets were publicly available that reflect current environmental conditions. This could help intrusion detection systems to consider current events and thus lead to a higher success rate. It could also compensate for the disadvantages of older datasets by collecting larger quantities of samples and making this contextualisation more widespread. Another area of research may be the integratability and adaptability of cybersecurity and cyber risk datasets. For example, it is often unclear to what extent datasets can be integrated or adapted to existing data. For cyber risks and cybersecurity, it would be helpful to know what requirements need to be met or what is needed to use the datasets appropriately. In addition, it would certainly be helpful to know whether datasets can be modified to be used for cyber risks or cybersecurity. Finally, the ability for stakeholders to identify machine-readable cybersecurity datasets would be useful because it would allow for even clearer delineations or comparisons between datasets. Due to the lack of publicly available datasets, concrete benchmarks often cannot be applied.
Below is the link to the electronic supplementary material.
Biographies
is a PhD student at the Kemmy Business School, University of Limerick, as part of the Emerging Risk Group (ERG). He is researching in joint cooperation with the Institute for Insurance Studies (ivwKöln), TH Köln, where he is working as a Research Assistant at the Cologne Research Centre for Reinsurance. His current research interests include cyber risks, cyber insurance and cybersecurity. Frank is a Fellow of the Chartered Insurance Institute (FCII) and a member of the German Association for Insurance Studies (DVfVW).
is a Lecturer in Risk and Finance at the Kemmy Business School at the University of Limerick. In his research, Dr Sheehan investigates novel risk metrication and machine learning methodologies in the context of insurance and finance, attentive to a changing private and public emerging risk environment. He is a researcher with significant insurance industry and academic experience. With a professional background in actuarial science, his research uses machine-learning techniques to estimate the changing risk profile produced by emerging technologies. He is a senior member of the Emerging Risk Group (ERG) at the University of Limerick, which has long-established expertise in insurance and risk management and has continued success within large research consortia including a number of SFI, FP7 and EU H2020 research projects. In particular, he contributed to the successful completion of three Horizon 2020 EU-funded projects, including PROTECT, Vision Inspired Driver Assistance Systems (VI-DAS) and Cloud Large Scale Video Analysis (Cloud-LSVA).
is a Professor at the Institute of Insurance at the Technical University of Cologne. His activities include teaching and research in insurance law and liability insurance. His research focuses include D&O, corporate liability, fidelity and cyber insurance. In addition, he heads the Master’s degree programme in insurance law and is the Academic Director of the Automotive Insurance Manager and Cyber Insurance Manager certificate programmes. He is also chairman of the examination board at the Institute of Insurance Studies.
Arash Negahdari Kia
is a postdoctoral Marie Cuire scholar and Research Fellow at the Kemmy Business School (KBS), University of Limerick (UL), a member of the Lero Software Research Center and Emerging Risk Group (ERG). He researches the cybersecurity risks of autonomous vehicles using machine-learning algorithms in a team supervised by Dr Finbarr Murphy at KBS, UL. For his PhD, he developed two graph-based, semi-supervised algorithms for multivariate time series for global stock market indices prediction. For his Master’s, he developed neural network models for Forex market prediction. Arash’s other research interests include text mining, graph mining and bioinformatics.
is a Professor in Risk and Insurance at the Kemmy Business School, University of Limerick. He worked on a number of insurance-related research projects, including four EU Commission-funded projects around emerging technologies and risk transfer. Prof. Mullins maintains strong links with the international insurance industry and works closely with Lloyd’s of London and XL Catlin on emerging risk. His work also encompasses the area of applied ethics as it pertains to new technologies. In the field of applied ethics, Dr Mullins works closely with the insurance industry and lectures on cultural and technological breakthroughs of high societal relevance. In that respect, Dr Martin Mullins has been appointed to a European expert group to advise EIOPA on the development of digital responsibility principles in insurance.
is Executive Dean Kemmy Business School. A computer engineering graduate, Finbarr worked for over 10 years in investment banking before returning to academia and completing his PhD in 2010. Finbarr has authored or co-authored over 70 refereed journal papers, edited books and book chapters. His research has been published in leading research journals in his discipline, such as Nature Nanotechnology, Small, Transportation Research A-F and the Review of Derivatives Research. A former Fulbright Scholar and Erasmus Mundus Exchange Scholar, Finbarr has delivered numerous guest lectures in America, mainland Europe, Israel, Russia, China and Vietnam. His research interests include quantitative finance and, more recently, emerging technological risk. Finbarr is currently engaged in several EU H2020 projects and with the Irish Science Foundation Ireland.
(FCII) has held the Chair of Reinsurance at the Institute of Insurance of TH Köln since 1998, focusing on the efficiency of reinsurance, industrial insurance and alternative risk transfer (ART). He studied mathematics and computer science with a focus on artificial intelligence and researched from 1988 to 1991 at the Fraunhofer Institute for Autonomous Intelligent Systems (AiS) in Schloß Birlinghoven. From 1991 to 2004, Prof. Materne worked for Gen Re (formerly Cologne Re) in various management positions in Germany and abroad, and from 2001 to 2003, he served as General Manager of Cologne Re of Dublin in Ireland. In 2008, Prof. Materne founded the Cologne Reinsurance Research Centre, of which he is the Director. Current issues in reinsurance and related fields are analysed and discussed with practitioners, with valuable contacts through the ‘Förderkreis Rückversicherung’ and the organisation of the annual Cologne Reinsurance Symposium. Prof. Materne holds various international supervisory boards, board of directors and advisory board mandates at insurance and reinsurance companies, captives, InsurTechs, EIOPA, as well as at insurance-scientific institutions. He also acts as an arbitrator and party representative in arbitration proceedings.
Open Access funding provided by the IReL Consortium.
Declarations
On behalf of all authors, the corresponding author states that there is no conflict of interest.
1 Average cost of a breach of more than 50 million records.
Publisher's Note
Springer Nature remains neutral with regard to jurisdictional claims in published maps and institutional affiliations.
- Aamir M, Rizvi SSH, Hashmani MA, Zubair M, Ahmad J. Machine learning classification of port scanning and DDoS attacks: A comparative analysis. Mehran University Research Journal of Engineering and Technology. 2021; 40 (1):215–229. doi: 10.22581/muet1982.2101.19. [ CrossRef ] [ Google Scholar ]
- Aamir M, Zaidi SMA. DDoS attack detection with feature engineering and machine learning: The framework and performance evaluation. International Journal of Information Security. 2019; 18 (6):761–785. doi: 10.1007/s10207-019-00434-1. [ CrossRef ] [ Google Scholar ]
- Aassal A, El S, Baki A. Das, Verma RM. An in-depth benchmarking and evaluation of phishing detection research for security needs. IEEE Access. 2020; 8 :22170–22192. doi: 10.1109/ACCESS.2020.2969780. [ CrossRef ] [ Google Scholar ]
- Abu Al-Haija Q, Zein-Sabatto S. An efficient deep-learning-based detection and classification system for cyber-attacks in IoT communication networks. Electronics. 2020; 9 (12):26. doi: 10.3390/electronics9122152. [ CrossRef ] [ Google Scholar ]
- Adhikari U, Morris TH, Pan SY. Applying Hoeffding adaptive trees for real-time cyber-power event and intrusion classification. IEEE Transactions on Smart Grid. 2018; 9 (5):4049–4060. doi: 10.1109/tsg.2017.2647778. [ CrossRef ] [ Google Scholar ]
- Agarwal A, Sharma P, Alshehri M, Mohamed AA, Alfarraj O. Classification model for accuracy and intrusion detection using machine learning approach. PeerJ Computer Science. 2021 doi: 10.7717/peerj-cs.437. [ PMC free article ] [ PubMed ] [ CrossRef ] [ Google Scholar ]
- Agrafiotis Ioannis, Nurse Jason R.C., Goldsmith M, Creese S, Upton D. A taxonomy of cyber-harms: Defining the impacts of cyber-attacks and understanding how they propagate. Journal of Cybersecurity. 2018; 4 :tyy006. doi: 10.1093/cybsec/tyy006. [ CrossRef ] [ Google Scholar ]
- Agrawal A, Mohammed S, Fiaidhi J. Ensemble technique for intruder detection in network traffic. International Journal of Security and Its Applications. 2019; 13 (3):1–8. doi: 10.33832/ijsia.2019.13.3.01. [ CrossRef ] [ Google Scholar ]
- Ahmad, I., and R.A. Alsemmeari. 2020. Towards improving the intrusion detection through ELM (extreme learning machine). CMC Computers Materials & Continua 65 (2): 1097–1111. 10.32604/cmc.2020.011732.
- Ahmed M, Mahmood AN, Hu JK. A survey of network anomaly detection techniques. Journal of Network and Computer Applications. 2016; 60 :19–31. doi: 10.1016/j.jnca.2015.11.016. [ CrossRef ] [ Google Scholar ]
- Al-Jarrah OY, Alhussein O, Yoo PD, Muhaidat S, Taha K, Kim K. Data randomization and cluster-based partitioning for Botnet intrusion detection. IEEE Transactions on Cybernetics. 2016; 46 (8):1796–1806. doi: 10.1109/TCYB.2015.2490802. [ PubMed ] [ CrossRef ] [ Google Scholar ]
- Al-Mhiqani MN, Ahmad R, Abidin ZZ, Yassin W, Hassan A, Abdulkareem KH, Ali NS, Yunos Z. A review of insider threat detection: Classification, machine learning techniques, datasets, open challenges, and recommendations. Applied Sciences—Basel. 2020; 10 (15):41. doi: 10.3390/app10155208. [ CrossRef ] [ Google Scholar ]
- Al-Omari M, Rawashdeh M, Qutaishat F, Alshira'H M, Ababneh N. An intelligent tree-based intrusion detection model for cyber security. Journal of Network and Systems Management. 2021; 29 (2):18. doi: 10.1007/s10922-021-09591-y. [ CrossRef ] [ Google Scholar ]
- Alabdallah A, Awad M. Using weighted Support Vector Machine to address the imbalanced classes problem of Intrusion Detection System. KSII Transactions on Internet and Information Systems. 2018; 12 (10):5143–5158. doi: 10.3837/tiis.2018.10.027. [ CrossRef ] [ Google Scholar ]
- Alazab M, Alazab M, Shalaginov A, Mesleh A, Awajan A. Intelligent mobile malware detection using permission requests and API calls. Future Generation Computer Systems—the International Journal of eScience. 2020; 107 :509–521. doi: 10.1016/j.future.2020.02.002. [ CrossRef ] [ Google Scholar ]
- Albahar MA, Al-Falluji RA, Binsawad M. An empirical comparison on malicious activity detection using different neural network-based models. IEEE Access. 2020; 8 :61549–61564. doi: 10.1109/ACCESS.2020.2984157. [ CrossRef ] [ Google Scholar ]
- AlEroud AF, Karabatis G. Queryable semantics to detect cyber-attacks: A flow-based detection approach. IEEE Transactions on Systems, Man, and Cybernetics: Systems. 2018; 48 (2):207–223. doi: 10.1109/TSMC.2016.2600405. [ CrossRef ] [ Google Scholar ]
- Algarni AM, Thayananthan V, Malaiya YK. Quantitative assessment of cybersecurity risks for mitigating data breaches in business systems. Applied Sciences (switzerland) 2021 doi: 10.3390/app11083678. [ CrossRef ] [ Google Scholar ]
- Alhowaide A, Alsmadi I, Tang J. Towards the design of real-time autonomous IoT NIDS. Cluster Computing—the Journal of Networks Software Tools and Applications. 2021 doi: 10.1007/s10586-021-03231-5. [ CrossRef ] [ Google Scholar ]
- Ali S, Li Y. Learning multilevel auto-encoders for DDoS attack detection in smart grid network. IEEE Access. 2019; 7 :108647–108659. doi: 10.1109/ACCESS.2019.2933304. [ CrossRef ] [ Google Scholar ]
- AlKadi O, Moustafa N, Turnbull B, Choo KKR. Mixture localization-based outliers models for securing data migration in cloud centers. IEEE Access. 2019; 7 :114607–114618. doi: 10.1109/ACCESS.2019.2935142. [ CrossRef ] [ Google Scholar ]
- Allianz. 2021. Allianz Risk Barometer. https://www.agcs.allianz.com/content/dam/onemarketing/agcs/agcs/reports/Allianz-Risk-Barometer-2021.pdf . Accessed 15 May 2021.
- Almiani Muder, AbuGhazleh Alia, Al-Rahayfeh Amer, Atiewi Saleh, Razaque Abdul. Deep recurrent neural network for IoT intrusion detection system. Simulation Modelling Practice and Theory. 2020; 101 :102031. doi: 10.1016/j.simpat.2019.102031. [ CrossRef ] [ Google Scholar ]
- Alsaedi A, Moustafa N, Tari Z, Mahmood A, Anwar A. TON_IoT telemetry dataset: A new generation dataset of IoT and IIoT for data-driven intrusion detection systems. IEEE Access. 2020; 8 :165130–165150. doi: 10.1109/access.2020.3022862. [ CrossRef ] [ Google Scholar ]
- Alsamiri J, Alsubhi K. Internet of Things cyber attacks detection using machine learning. International Journal of Advanced Computer Science and Applications. 2019; 10 (12):627–634. doi: 10.14569/IJACSA.2019.0101280. [ CrossRef ] [ Google Scholar ]
- Alsharafat W. Applying artificial neural network and eXtended classifier system for network intrusion detection. International Arab Journal of Information Technology. 2013; 10 (3):230–238. [ Google Scholar ]
- Amin RW, Sevil HE, Kocak S, Francia G, III, Hoover P. The spatial analysis of the malicious uniform resource locators (URLs): 2016 dataset case study. Information (switzerland) 2021; 12 (1):1–18. doi: 10.3390/info12010002. [ CrossRef ] [ Google Scholar ]
- Arcuri MC, Gai LZ, Ielasi F, Ventisette E. Cyber attacks on hospitality sector: Stock market reaction. Journal of Hospitality and Tourism Technology. 2020; 11 (2):277–290. doi: 10.1108/jhtt-05-2019-0080. [ CrossRef ] [ Google Scholar ]
- Arp Daniel, Spreitzenbarth Michael, Hubner Malte, Rieck Konrad, et al. Drebin: Effective and explainable detection of android malware in your pocket. NDSS Conference. 2014; 14 :23–26. [ Google Scholar ]
- Ashtiani M, Azgomi MA. A distributed simulation framework for modeling cyber attacks and the evaluation of security measures. Simulation—Transactions of the Society for Modeling and Simulation International. 2014; 90 (9):1071–1102. doi: 10.1177/0037549714540221. [ CrossRef ] [ Google Scholar ]
- Atefinia R, Ahmadi M. Network intrusion detection using multi-architectural modular deep neural network. Journal of Supercomputing. 2021; 77 (4):3571–3593. doi: 10.1007/s11227-020-03410-y. [ CrossRef ] [ Google Scholar ]
- Avila R, Khoury R, Khoury R, Petrillo F. Use of security logs for data leak detection: A systematic literature review. Security and Communication Networks. 2021; 2021 :29. doi: 10.1155/2021/6615899. [ CrossRef ] [ Google Scholar ]
- Azeez NA, Ayemobola TJ, Misra S, Maskeliunas R, Damasevicius R. Network Intrusion Detection with a Hashing Based Apriori Algorithm Using Hadoop MapReduce. Computers. 2019; 8 (4):15. doi: 10.3390/computers8040086. [ CrossRef ] [ Google Scholar ]
- Bakdash JZ, Hutchinson S, Zaroukian EG, Marusich LR, Thirumuruganathan S, Sample C, Hoffman B, Das G. Malware in the future forecasting of analyst detection of cyber events. Journal of Cybersecurity. 2018 doi: 10.1093/cybsec/tyy007. [ CrossRef ] [ Google Scholar ]
- Barletta VS, Caivano D, Nannavecchia A, Scalera M. Intrusion detection for in-vehicle communication networks: An unsupervised Kohonen SOM approach. Future Internet. 2020 doi: 10.3390/FI12070119. [ CrossRef ] [ Google Scholar ]
- Barzegar M, Shajari M. Attack scenario reconstruction using intrusion semantics. Expert Systems with Applications. 2018; 108 :119–133. doi: 10.1016/j.eswa.2018.04.030. [ CrossRef ] [ Google Scholar ]
- Bessy-Roland Yannick, Boumezoued Alexandre, Hillairet Caroline. Multivariate Hawkes process for cyber insurance. Annals of Actuarial Science. 2021; 15 (1):14–39. doi: 10.1017/S1748499520000093. [ CrossRef ] [ Google Scholar ]
- Bhardwaj A, Mangat V, Vig R. Hyperband tuned deep neural network with well posed stacked sparse AutoEncoder for detection of DDoS attacks in cloud. IEEE Access. 2020; 8 :181916–181929. doi: 10.1109/ACCESS.2020.3028690. [ CrossRef ] [ Google Scholar ]
- Bhati BS, Rai CS, Balamurugan B, Al-Turjman F. An intrusion detection scheme based on the ensemble of discriminant classifiers. Computers & Electrical Engineering. 2020; 86 :9. doi: 10.1016/j.compeleceng.2020.106742. [ CrossRef ] [ Google Scholar ]
- Bhattacharya S, Krishnan SSR, Maddikunta PKR, Kaluri R, Singh S, Gadekallu TR, Alazab M, Tariq U. A novel PCA-firefly based XGBoost classification model for intrusion detection in networks using GPU. Electronics. 2020; 9 (2):16. doi: 10.3390/electronics9020219. [ CrossRef ] [ Google Scholar ]
- Bibi I, Akhunzada A, Malik J, Iqbal J, Musaddiq A, Kim S. A dynamic DL-driven architecture to combat sophisticated android malware. IEEE Access. 2020; 8 :129600–129612. doi: 10.1109/ACCESS.2020.3009819. [ CrossRef ] [ Google Scholar ]
- Biener C, Eling M, Wirfs JH. Insurability of cyber risk: An empirical analysis. Geneva Papers on Risk and Insurance: Issues and Practice. 2015; 40 (1):131–158. doi: 10.1057/gpp.2014.19. [ CrossRef ] [ Google Scholar ]
- Binbusayyis A, Vaiyapuri T. Identifying and benchmarking key features for cyber intrusion detection: An ensemble approach. IEEE Access. 2019; 7 :106495–106513. doi: 10.1109/ACCESS.2019.2929487. [ CrossRef ] [ Google Scholar ]
- Biswas R, Roy S. Botnet traffic identification using neural networks. Multimedia Tools and Applications. 2021 doi: 10.1007/s11042-021-10765-8. [ CrossRef ] [ Google Scholar ]
- Bouyeddou B, Harrou F, Kadri B, Sun Y. Detecting network cyber-attacks using an integrated statistical approach. Cluster Computing—the Journal of Networks Software Tools and Applications. 2021; 24 (2):1435–1453. doi: 10.1007/s10586-020-03203-1. [ CrossRef ] [ Google Scholar ]
- Bozkir AS, Aydos M. LogoSENSE: A companion HOG based logo detection scheme for phishing web page and E-mail brand recognition. Computers & Security. 2020; 95 :18. doi: 10.1016/j.cose.2020.101855. [ CrossRef ] [ Google Scholar ]
- Brower, D., and M. McCormick. 2021. Colonial pipeline resumes operations following ransomware attack. Financial Times .
- Cai H, Zhang F, Levi A. An unsupervised method for detecting shilling attacks in recommender systems by mining item relationship and identifying target items. The Computer Journal. 2019; 62 (4):579–597. doi: 10.1093/comjnl/bxy124. [ CrossRef ] [ Google Scholar ]
- Cebula, J.J., M.E. Popeck, and L.R. Young. 2014. A Taxonomy of Operational Cyber Security Risks Version 2 .
- Chadza T, Kyriakopoulos KG, Lambotharan S. Learning to learn sequential network attacks using hidden Markov models. IEEE Access. 2020; 8 :134480–134497. doi: 10.1109/ACCESS.2020.3011293. [ CrossRef ] [ Google Scholar ]
- Chatterjee S, Thekdi S. An iterative learning and inference approach to managing dynamic cyber vulnerabilities of complex systems. Reliability Engineering and System Safety. 2020 doi: 10.1016/j.ress.2019.106664. [ CrossRef ] [ Google Scholar ]
- Chattopadhyay M, Sen R, Gupta S. A comprehensive review and meta-analysis on applications of machine learning techniques in intrusion detection. Australasian Journal of Information Systems. 2018; 22 :27. doi: 10.3127/ajis.v22i0.1667. [ CrossRef ] [ Google Scholar ]
- Chen HS, Fiscus J. The inhospitable vulnerability: A need for cybersecurity risk assessment in the hospitality industry. Journal of Hospitality and Tourism Technology. 2018; 9 (2):223–234. doi: 10.1108/JHTT-07-2017-0044. [ CrossRef ] [ Google Scholar ]
- Chhabra GS, Singh VP, Singh M. Cyber forensics framework for big data analytics in IoT environment using machine learning. Multimedia Tools and Applications. 2020; 79 (23–24):15881–15900. doi: 10.1007/s11042-018-6338-1. [ CrossRef ] [ Google Scholar ]
- Chiba Z, Abghour N, Moussaid K, Elomri A, Rida M. Intelligent approach to build a Deep Neural Network based IDS for cloud environment using combination of machine learning algorithms. Computers and Security. 2019; 86 :291–317. doi: 10.1016/j.cose.2019.06.013. [ CrossRef ] [ Google Scholar ]
- Choras M, Kozik R. Machine learning techniques applied to detect cyber attacks on web applications. Logic Journal of the IGPL. 2015; 23 (1):45–56. doi: 10.1093/jigpal/jzu038. [ CrossRef ] [ Google Scholar ]
- Chowdhury Sudipta, Khanzadeh Mojtaba, Akula Ravi, Zhang Fangyan, Zhang Song, Medal Hugh, Marufuzzaman Mohammad, Bian Linkan. Botnet detection using graph-based feature clustering. Journal of Big Data. 2017; 4 (1):14. doi: 10.1186/s40537-017-0074-7. [ CrossRef ] [ Google Scholar ]
- Cost Of A Cyber Incident: Systematic Review And Cross-Validation, Cybersecurity & Infrastructure Agency , 1, https://www.cisa.gov/sites/default/files/publications/CISA-OCE_Cost_of_Cyber_Incidents_Study-FINAL_508.pdf (2020).
- D'Hooge L, Wauters T, Volckaert B, De Turck F. Classification hardness for supervised learners on 20 years of intrusion detection data. IEEE Access. 2019; 7 :167455–167469. doi: 10.1109/access.2019.2953451. [ CrossRef ] [ Google Scholar ]
- Damasevicius R, Venckauskas A, Grigaliunas S, Toldinas J, Morkevicius N, Aleliunas T, Smuikys P. LITNET-2020: An annotated real-world network flow dataset for network intrusion detection. Electronics. 2020; 9 (5):23. doi: 10.3390/electronics9050800. [ CrossRef ] [ Google Scholar ]
- Giovanni De, Domenico Arturo Leccadito, Pirra Marco. On the determinants of data breaches: A cointegration analysis. Decisions in Economics and Finance. 2020 doi: 10.1007/s10203-020-00301-y. [ CrossRef ] [ Google Scholar ]
- Deng Lianbing, Li Daming, Yao Xiang, Wang Haoxiang. Retracted Article: Mobile network intrusion detection for IoT system based on transfer learning algorithm. Cluster Computing. 2019; 22 (4):9889–9904. doi: 10.1007/s10586-018-1847-2. [ CrossRef ] [ Google Scholar ]
- Donkal G, Verma GK. A multimodal fusion based framework to reinforce IDS for securing Big Data environment using Spark. Journal of Information Security and Applications. 2018; 43 :1–11. doi: 10.1016/j.jisa.2018.10.001. [ CrossRef ] [ Google Scholar ]
- Dunn C, Moustafa N, Turnbull B. Robustness evaluations of sustainable machine learning models against data Poisoning attacks in the Internet of Things. Sustainability. 2020; 12 (16):17. doi: 10.3390/su12166434. [ CrossRef ] [ Google Scholar ]
- Dwivedi S, Vardhan M, Tripathi S. Multi-parallel adaptive grasshopper optimization technique for detecting anonymous attacks in wireless networks. Wireless Personal Communications. 2021 doi: 10.1007/s11277-021-08368-5. [ CrossRef ] [ Google Scholar ]
- Dyson, B. 2020. COVID-19 crisis could be ‘watershed’ for cyber insurance, says Swiss Re exec. https://www.spglobal.com/marketintelligence/en/news-insights/latest-news-headlines/covid-19-crisis-could-be-watershed-for-cyber-insurance-says-swiss-re-exec-59197154 . Accessed 7 May 2020.
- EIOPA. 2018. Understanding cyber insurance—a structured dialogue with insurance companies. https://www.eiopa.europa.eu/sites/default/files/publications/reports/eiopa_understanding_cyber_insurance.pdf . Accessed 28 May 2018
- Elijah AV, Abdullah A, JhanJhi NZ, Supramaniam M, Abdullateef OB. Ensemble and deep-learning methods for two-class and multi-attack anomaly intrusion detection: An empirical study. International Journal of Advanced Computer Science and Applications. 2019; 10 (9):520–528. doi: 10.14569/IJACSA.2019.0100969. [ CrossRef ] [ Google Scholar ]
- Eling M, Jung K. Copula approaches for modeling cross-sectional dependence of data breach losses. Insurance Mathematics & Economics. 2018; 82 :167–180. doi: 10.1016/j.insmatheco.2018.07.003. [ CrossRef ] [ Google Scholar ]
- Eling M, Schnell W. What do we know about cyber risk and cyber risk insurance? Journal of Risk Finance. 2016; 17 (5):474–491. doi: 10.1108/jrf-09-2016-0122. [ CrossRef ] [ Google Scholar ]
- Eling M, Wirfs J. What are the actual costs of cyber risk events? European Journal of Operational Research. 2019; 272 (3):1109–1119. doi: 10.1016/j.ejor.2018.07.021. [ CrossRef ] [ Google Scholar ]
- Eling Martin. Cyber risk research in business and actuarial science. European Actuarial Journal. 2020; 10 (2):303–333. doi: 10.1007/s13385-020-00250-1. [ CrossRef ] [ Google Scholar ]
- Elmasry W, Akbulut A, Zaim AH. Empirical study on multiclass classification-based network intrusion detection. Computational Intelligence. 2019; 35 (4):919–954. doi: 10.1111/coin.12220. [ CrossRef ] [ Google Scholar ]
- Elsaid Shaimaa Ahmed, Albatati Nouf Saleh. An optimized collaborative intrusion detection system for wireless sensor networks. Soft Computing. 2020; 24 (16):12553–12567. doi: 10.1007/s00500-020-04695-0. [ CrossRef ] [ Google Scholar ]
- Estepa R, Díaz-Verdejo JE, Estepa A, Madinabeitia G. How much training data is enough? A case study for HTTP anomaly-based intrusion detection. IEEE Access. 2020; 8 :44410–44425. doi: 10.1109/ACCESS.2020.2977591. [ CrossRef ] [ Google Scholar ]
- European Council. 2021. Cybersecurity: how the EU tackles cyber threats. https://www.consilium.europa.eu/en/policies/cybersecurity/ . Accessed 10 May 2021
- Falco Gregory, Eling Martin, Jablanski Danielle, Weber Matthias, Miller Virginia, Gordon Lawrence A, Wang Shaun Shuxun, Schmit Joan, Thomas Russell, Elvedi Mauro, Maillart Thomas, Donavan Emy, Dejung Simon, Durand Eric, Nutter Franklin, Scheffer Uzi, Arazi Gil, Ohana Gilbert, Lin Herbert. Cyber risk research impeded by disciplinary barriers. Science (american Association for the Advancement of Science) 2019; 366 (6469):1066–1069. doi: 10.1126/science.aaz4795. [ PubMed ] [ CrossRef ] [ Google Scholar ]
- Fan ZJ, Tan ZP, Tan CX, Li X. An improved integrated prediction method of cyber security situation based on spatial-time analysis. Journal of Internet Technology. 2018; 19 (6):1789–1800. doi: 10.3966/160792642018111906015. [ CrossRef ] [ Google Scholar ]
- Fang ZJ, Xu MC, Xu SH, Hu TZ. A framework for predicting data breach risk: Leveraging dependence to cope with sparsity. IEEE Transactions on Information Forensics and Security. 2021; 16 :2186–2201. doi: 10.1109/tifs.2021.3051804. [ CrossRef ] [ Google Scholar ]
- Farkas S, Lopez O, Thomas M. Cyber claim analysis using Generalized Pareto regression trees with applications to insurance. Insurance: Mathematics and Economics. 2021; 98 :92–105. doi: 10.1016/j.insmatheco.2021.02.009. [ CrossRef ] [ Google Scholar ]
- Farsi H, Fanian A, Taghiyarrenani Z. A novel online state-based anomaly detection system for process control networks. International Journal of Critical Infrastructure Protection. 2019; 27 :11. doi: 10.1016/j.ijcip.2019.100323. [ CrossRef ] [ Google Scholar ]
- Ferrag MA, Maglaras L, Moschoyiannis S, Janicke H. Deep learning for cyber security intrusion detection: Approaches, datasets, and comparative study. Journal of Information Security and Applications. 2020; 50 :19. doi: 10.1016/j.jisa.2019.102419. [ CrossRef ] [ Google Scholar ]
- Field, M. 2018. WannaCry cyber attack cost the NHS £92m as 19,000 appointments cancelled. https://www.telegraph.co.uk/technology/2018/10/11/wannacry-cyber-attack-cost-nhs-92m-19000-appointments-cancelled/ . Accessed 9 May 2018.
- FitchRatings. 2021. U.S. Cyber Insurance Market Update (Spike in Claims Leads to Decline in 2020 Underwriting Performance). https://www.fitchratings.com/research/insurance/us-cyber-insurance-market-update-spike-in-claims-leads-to-decline-in-2020-underwriting-performance-26-05-2021 .
- Fossaceca JM, Mazzuchi TA, Sarkani S. MARK-ELM: Application of a novel Multiple Kernel Learning framework for improving the robustness of network intrusion detection. Expert Systems with Applications. 2015; 42 (8):4062–4080. doi: 10.1016/j.eswa.2014.12.040. [ CrossRef ] [ Google Scholar ]
- Franke Ulrik, Brynielsson Joel. Cyber situational awareness – A systematic review of the literature. Computers & Security. 2014; 46 :18–31. doi: 10.1016/j.cose.2014.06.008. [ CrossRef ] [ Google Scholar ]
- Freeha Khan, Hwan Kim Jung, Lars Mathiassen, Robin Moore. Data breach management: An integrated risk model. Information & Management. 2021; 58 (1):103392. doi: 10.1016/j.im.2020.103392. [ CrossRef ] [ Google Scholar ]
- Ganeshan R, Rodrigues Paul. Crow-AFL: Crow based adaptive fractional lion optimization approach for the intrusion detection. Wireless Personal Communications. 2020; 111 (4):2065–2089. doi: 10.1007/s11277-019-06972-0. [ CrossRef ] [ Google Scholar ]
- GAO. 2021. CYBER INSURANCE—Insurers and policyholders face challenges in an evolving market. https://www.gao.gov/assets/gao-21-477.pdf . Accessed 16 May 2021.
- Garber, J. 2021. Colonial Pipeline fiasco foreshadows impact of Biden energy policy. https://www.foxbusiness.com/markets/colonial-pipeline-fiasco-foreshadows-impact-of-biden-energy-policy . Accessed 4 May 2021.
- Gauthama Raman MR, Somu Nivethitha, Jagarapu Sahruday, Manghnani Tina, Selvam Thirumaran, Krithivasan Kannan, Shankar Sriram VS. An efficient intrusion detection technique based on support vector machine and improved binary gravitational search algorithm. Artificial Intelligence Review. 2020; 53 (5):3255–3286. doi: 10.1007/s10462-019-09762-z. [ CrossRef ] [ Google Scholar ]
- Gavel S, Raghuvanshi AS, Tiwari S. Distributed intrusion detection scheme using dual-axis dimensionality reduction for Internet of things (IoT) Journal of Supercomputing. 2021 doi: 10.1007/s11227-021-03697-5. [ CrossRef ] [ Google Scholar ]
- GDPR.EU. 2021. FAQ. https://gdpr.eu/faq/ . Accessed 10 May 2021.
- Georgescu TM, Iancu B, Zurini M. Named-entity-recognition-based automated system for diagnosing cybersecurity situations in IoT networks. Sensors (switzerland) 2019 doi: 10.3390/s19153380. [ PMC free article ] [ PubMed ] [ CrossRef ] [ Google Scholar ]
- Giudici Paolo, Raffinetti Emanuela. Cyber risk ordering with rank-based statistical models. AStA Advances in Statistical Analysis. 2020 doi: 10.1007/s10182-020-00387-0. [ CrossRef ] [ Google Scholar ]
- Goh, J., S. Adepu, K.N. Junejo, and A. Mathur. 2016. A dataset to support research in the design of secure water treatment systems. In CRITIS.
- Gong XY, Lu JL, Zhou YF, Qiu H, He R. Model uncertainty based annotation error fixing for web attack detection. Journal of Signal Processing Systems for Signal Image and Video Technology. 2021; 93 (2–3):187–199. doi: 10.1007/s11265-019-01494-1. [ CrossRef ] [ Google Scholar ]
- Goode Sigi, Hoehle Hartmut, Venkatesh Viswanath, Brown Susan A. USER compensation as a data breach recovery action: An investigation of the sony playstation network breach. MIS Quarterly. 2017; 41 (3):703–727. doi: 10.25300/MISQ/2017/41.3.03. [ CrossRef ] [ Google Scholar ]
- Guo H, Huang S, Huang C, Pan Z, Zhang M, Shi F. File entropy signal analysis combined with wavelet decomposition for malware classification. IEEE Access. 2020; 8 :158961–158971. doi: 10.1109/ACCESS.2020.3020330. [ CrossRef ] [ Google Scholar ]
- Habib Maria, Aljarah Ibrahim, Faris Hossam. A Modified multi-objective particle swarm optimizer-based Lévy flight: An approach toward intrusion detection in Internet of Things. Arabian Journal for Science and Engineering. 2020; 45 (8):6081–6108. doi: 10.1007/s13369-020-04476-9. [ CrossRef ] [ Google Scholar ]
- Hajj S, El Sibai R, Abdo JB, Demerjian J, Makhoul A, Guyeux C. Anomaly-based intrusion detection systems: The requirements, methods, measurements, and datasets. Transactions on Emerging Telecommunications Technologies. 2021; 32 (4):36. doi: 10.1002/ett.4240. [ CrossRef ] [ Google Scholar ]
- Heartfield R, Loukas G, Bezemskij A, Panaousis E. Self-configurable cyber-physical intrusion detection for smart homes using reinforcement learning. IEEE Transactions on Information Forensics and Security. 2021; 16 :1720–1735. doi: 10.1109/tifs.2020.3042049. [ CrossRef ] [ Google Scholar ]
- Hemo, B., T. Gafni, K. Cohen, and Q. Zhao. 2020. Searching for anomalies over composite hypotheses. IEEE Transactions on Signal Processing 68: 1181–1196. 10.1109/TSP.2020.2971438
- Hindy H, Brosset D, Bayne E, Seeam AK, Tachtatzis C, Atkinson R, Bellekens X. A taxonomy of network threats and the effect of current datasets on intrusion detection systems. IEEE Access. 2020; 8 :104650–104675. doi: 10.1109/ACCESS.2020.3000179. [ CrossRef ] [ Google Scholar ]
- Hong W, Huang D, Chen C, Lee J. Towards accurate and efficient classification of power system contingencies and cyber-attacks using recurrent neural networks. IEEE Access. 2020; 8 :123297–123309. doi: 10.1109/ACCESS.2020.3007609. [ CrossRef ] [ Google Scholar ]
- Husák Martin, Zádník M, Bartos V, Sokol P. Dataset of intrusion detection alerts from a sharing platform. Data in Brief. 2020; 33 :106530. doi: 10.1016/j.dib.2020.106530. [ PMC free article ] [ PubMed ] [ CrossRef ] [ Google Scholar ]
- IBM Security. 2020. Cost of a Data breach Report. https://www.capita.com/sites/g/files/nginej291/files/2020-08/Ponemon-Global-Cost-of-Data-Breach-Study-2020.pdf . Accessed 19 May 2021.
- IEEE. 2021. IEEE Quick Facts. https://www.ieee.org/about/at-a-glance.html . Accessed 11 May 2021.
- Firat Ilhan, Kilincer Ertam Fatih, Abdulkadir Sengur. Machine learning methods for cyber security intrusion detection: Datasets and comparative study. Computer Networks. 2021; 188 :107840. doi: 10.1016/j.comnet.2021.107840. [ CrossRef ] [ Google Scholar ]
- Jaber AN, Ul Rehman S. FCM-SVM based intrusion detection system for cloud computing environment. Cluster Computing—the Journal of Networks Software Tools and Applications. 2020; 23 (4):3221–3231. doi: 10.1007/s10586-020-03082-6. [ CrossRef ] [ Google Scholar ]
- Jacobs, J., S. Romanosky, B. Edwards, M. Roytman, and I. Adjerid. 2019. Exploit prediction scoring system (epss). arXiv:1908.04856
- Jacobsen Annika, de Miranda Ricardo, Azevedo Nick Juty, Batista Dominique, Coles Simon, Cornet Ronald, Courtot Mélanie, Crosas Mercè, Dumontier Michel, Evelo Chris T, Goble Carole, Guizzardi Giancarlo, Hansen Karsten Kryger, Hasnain Ali, Hettne Kristina, Heringa Jaap, Hooft Rob W.W., Imming Melanie, Jeffery Keith G, Kaliyaperumal Rajaram, Kersloot Martijn G, Kirkpatrick Christine R, Kuhn Tobias, Labastida Ignasi, Magagna Barbara, McQuilton Peter, Meyers Natalie, Montesanti Annalisa, van Reisen Mirjam, Rocca-Serra Philippe, Pergl Robert, Sansone Susanna-Assunta, da Silva Luiz Olavo Bonino, Santos Juliane Schneider, Strawn George, Thompson Mark, Waagmeester Andra, Weigel Tobias, Wilkinson Mark D, Willighagen Egon L, Wittenburg Peter, Roos Marco, Mons Barend, Schultes Erik. FAIR principles: Interpretations and implementation considerations. Data Intelligence. 2020; 2 (1–2):10–29. doi: 10.1162/dint_r_00024. [ CrossRef ] [ Google Scholar ]
- Jahromi AN, Hashemi S, Dehghantanha A, Parizi RM, Choo KKR. An enhanced stacked LSTM method with no random initialization for malware threat hunting in safety and time-critical systems. IEEE Transactions on Emerging Topics in Computational Intelligence. 2020; 4 (5):630–640. doi: 10.1109/TETCI.2019.2910243. [ CrossRef ] [ Google Scholar ]
- Jang S, Li S, Sung Y. FastText-based local feature visualization algorithm for merged image-based malware classification framework for cyber security and cyber defense. Mathematics. 2020; 8 (3):13. doi: 10.3390/math8030460. [ CrossRef ] [ Google Scholar ]
- Javeed D, Gao TH, Khan MT. SDN-enabled hybrid DL-driven framework for the detection of emerging cyber threats in IoT. Electronics. 2021; 10 (8):16. doi: 10.3390/electronics10080918. [ CrossRef ] [ Google Scholar ]
- Johnson P, Gorton D, Lagerstrom R, Ekstedt M. Time between vulnerability disclosures: A measure of software product vulnerability. Computers & Security. 2016; 62 :278–295. doi: 10.1016/j.cose.2016.08.004. [ CrossRef ] [ Google Scholar ]
- Johnson P, Lagerström R, Ekstedt M, Franke U. Can the common vulnerability scoring system be trusted? A Bayesian analysis. IEEE Transactions on Dependable and Secure Computing. 2018; 15 (6):1002–1015. doi: 10.1109/TDSC.2016.2644614. [ CrossRef ] [ Google Scholar ]
- Junger Marianne, Wang Victoria, Schlömer Marleen. Fraud against businesses both online and offline: Crime scripts, business characteristics, efforts, and benefits. Crime Science. 2020; 9 (1):13. doi: 10.1186/s40163-020-00119-4. [ CrossRef ] [ Google Scholar ]
- Kalutarage Harsha Kumara, Nguyen Hoang Nga, Shaikh Siraj Ahmed. Towards a threat assessment framework for apps collusion. Telecommunication Systems. 2017; 66 (3):417–430. doi: 10.1007/s11235-017-0296-1. [ PMC free article ] [ PubMed ] [ CrossRef ] [ Google Scholar ]
- Kamarudin MH, Maple C, Watson T, Safa NS. A LogitBoost-based algorithm for detecting known and unknown web attacks. IEEE Access. 2017; 5 :26190–26200. doi: 10.1109/ACCESS.2017.2766844. [ CrossRef ] [ Google Scholar ]
- Kasongo SM, Sun YX. A deep learning method with wrapper based feature extraction for wireless intrusion detection system. Computers & Security. 2020; 92 :15. doi: 10.1016/j.cose.2020.101752. [ CrossRef ] [ Google Scholar ]
- Keserwani Pankaj Kumar, Govil Mahesh Chandra, Pilli Emmanuel S, Govil Prajjval. A smart anomaly-based intrusion detection system for the Internet of Things (IoT) network using GWO–PSO–RF model. Journal of Reliable Intelligent Environments. 2021; 7 (1):3–21. doi: 10.1007/s40860-020-00126-x. [ CrossRef ] [ Google Scholar ]
- Keshk M, Sitnikova E, Moustafa N, Hu J, Khalil I. An integrated framework for privacy-preserving based anomaly detection for cyber-physical systems. IEEE Transactions on Sustainable Computing. 2021; 6 (1):66–79. doi: 10.1109/TSUSC.2019.2906657. [ CrossRef ] [ Google Scholar ]
- Khan IA, Pi DC, Bhatia AK, Khan N, Haider W, Wahab A. Generating realistic IoT-based IDS dataset centred on fuzzy qualitative modelling for cyber-physical systems. Electronics Letters. 2020; 56 (9):441–443. doi: 10.1049/el.2019.4158. [ CrossRef ] [ Google Scholar ]
- Khraisat A, Gondal I, Vamplew P, Kamruzzaman J, Alazab A. Hybrid intrusion detection system based on the stacking ensemble of C5 decision tree classifier and one class support vector machine. Electronics. 2020; 9 (1):18. doi: 10.3390/electronics9010173. [ CrossRef ] [ Google Scholar ]
- Khraisat Ansam, Gondal Iqbal, Vamplew Peter, Kamruzzaman Joarder. Survey of intrusion detection systems: Techniques, datasets and challenges. Cybersecurity. 2019; 2 (1):20. doi: 10.1186/s42400-019-0038-7. [ CrossRef ] [ Google Scholar ]
- Kilincer IF, Ertam F, Sengur A. Machine learning methods for cyber security intrusion detection: Datasets and comparative study. Computer Networks. 2021; 188 :16. doi: 10.1016/j.comnet.2021.107840. [ CrossRef ] [ Google Scholar ]
- Kim D, Kim HK. Automated dataset generation system for collaborative research of cyber threat analysis. Security and Communication Networks. 2019; 2019 :10. doi: 10.1155/2019/6268476. [ CrossRef ] [ Google Scholar ]
- Kim Gyeongmin, Lee Chanhee, Jo Jaechoon, Lim Heuiseok. Automatic extraction of named entities of cyber threats using a deep Bi-LSTM-CRF network. International Journal of Machine Learning and Cybernetics. 2020; 11 (10):2341–2355. doi: 10.1007/s13042-020-01122-6. [ CrossRef ] [ Google Scholar ]
- Kirubavathi G, Anitha R. Botnet detection via mining of traffic flow characteristics. Computers & Electrical Engineering. 2016; 50 :91–101. doi: 10.1016/j.compeleceng.2016.01.012. [ CrossRef ] [ Google Scholar ]
- Kiwia D, Dehghantanha A, Choo KKR, Slaughter J. A cyber kill chain based taxonomy of banking Trojans for evolutionary computational intelligence. Journal of Computational Science. 2018; 27 :394–409. doi: 10.1016/j.jocs.2017.10.020. [ CrossRef ] [ Google Scholar ]
- Koroniotis N, Moustafa N, Sitnikova E. A new network forensic framework based on deep learning for Internet of Things networks: A particle deep framework. Future Generation Computer Systems. 2020; 110 :91–106. doi: 10.1016/j.future.2020.03.042. [ CrossRef ] [ Google Scholar ]
- Kruse Clemens Scott, Frederick Benjamin, Jacobson Taylor, Kyle Monticone D. Cybersecurity in healthcare: A systematic review of modern threats and trends. Technology and Health Care. 2017; 25 (1):1–10. doi: 10.3233/THC-161263. [ PubMed ] [ CrossRef ] [ Google Scholar ]
- Kshetri N. The economics of cyber-insurance. IT Professional. 2018; 20 (6):9–14. doi: 10.1109/MITP.2018.2874210. [ CrossRef ] [ Google Scholar ]
- Kumar R, Kumar P, Tripathi R, Gupta GP, Gadekallu TR, Srivastava G. SP2F: A secured privacy-preserving framework for smart agricultural Unmanned Aerial Vehicles. Computer Networks. 2021 doi: 10.1016/j.comnet.2021.107819. [ CrossRef ] [ Google Scholar ]
- Kumar R, Tripathi R. DBTP2SF: A deep blockchain-based trustworthy privacy-preserving secured framework in industrial internet of things systems. Transactions on Emerging Telecommunications Technologies. 2021; 32 (4):27. doi: 10.1002/ett.4222. [ CrossRef ] [ Google Scholar ]
- Laso PM, Brosset D, Puentes J. Dataset of anomalies and malicious acts in a cyber-physical subsystem. Data in Brief. 2017; 14 :186–191. doi: 10.1016/j.dib.2017.07.038. [ PMC free article ] [ PubMed ] [ CrossRef ] [ Google Scholar ]
- Lee J, Kim J, Kim I, Han K. Cyber threat detection based on artificial neural networks using event profiles. IEEE Access. 2019; 7 :165607–165626. doi: 10.1109/ACCESS.2019.2953095. [ CrossRef ] [ Google Scholar ]
- Lee SJ, Yoo PD, Asyhari AT, Jhi Y, Chermak L, Yeun CY, Taha K. IMPACT: Impersonation attack detection via edge computing using deep Autoencoder and feature abstraction. IEEE Access. 2020; 8 :65520–65529. doi: 10.1109/ACCESS.2020.2985089. [ CrossRef ] [ Google Scholar ]
- Leong Yin-Yee, Chen Yen-Chih. Cyber risk cost and management in IoT devices-linked health insurance. The Geneva Papers on Risk and Insurance—Issues and Practice. 2020; 45 (4):737–759. doi: 10.1057/s41288-020-00169-4. [ CrossRef ] [ Google Scholar ]
- Levi, M. 2017. Assessing the trends, scale and nature of economic cybercrimes: overview and Issues: In Cybercrimes, cybercriminals and their policing, in crime, law and social change. Crime, Law and Social Change 67 (1): 3–20. 10.1007/s10611-016-9645-3.
- Li C, Mills K, Niu D, Zhu R, Zhang H, Kinawi H. Android malware detection based on factorization machine. IEEE Access. 2019; 7 :184008–184019. doi: 10.1109/ACCESS.2019.2958927. [ CrossRef ] [ Google Scholar ]
- Li DQ, Li QM. Adversarial deep ensemble: evasion attacks and defenses for malware detection. IEEE Transactions on Information Forensics and Security. 2020; 15 :3886–3900. doi: 10.1109/tifs.2020.3003571. [ CrossRef ] [ Google Scholar ]
- Li DQ, Li QM, Ye YF, Xu SH. A framework for enhancing deep neural networks against adversarial malware. IEEE Transactions on Network Science and Engineering. 2021; 8 (1):736–750. doi: 10.1109/tnse.2021.3051354. [ CrossRef ] [ Google Scholar ]
- Li RH, Zhang C, Feng C, Zhang X, Tang CJ. Locating vulnerability in binaries using deep neural networks. IEEE Access. 2019; 7 :134660–134676. doi: 10.1109/access.2019.2942043. [ CrossRef ] [ Google Scholar ]
- Li X, Xu M, Vijayakumar P, Kumar N, Liu X. Detection of low-frequency and multi-stage attacks in industrial Internet of Things. IEEE Transactions on Vehicular Technology. 2020; 69 (8):8820–8831. doi: 10.1109/TVT.2020.2995133. [ CrossRef ] [ Google Scholar ]
- Liu HY, Lang B. Machine learning and deep learning methods for intrusion detection systems: A survey. Applied Sciences—Basel. 2019; 9 (20):28. doi: 10.3390/app9204396. [ CrossRef ] [ Google Scholar ]
- Lopez-Martin M, Carro B, Sanchez-Esguevillas A. Application of deep reinforcement learning to intrusion detection for supervised problems. Expert Systems with Applications. 2020 doi: 10.1016/j.eswa.2019.112963. [ CrossRef ] [ Google Scholar ]
- Loukas G, Gan D, Vuong Tuan. A review of cyber threats and defence approaches in emergency management. Future Internet. 2013; 5 :205–236. doi: 10.3390/fi5020205. [ CrossRef ] [ Google Scholar ]
- Luo CC, Su S, Sun YB, Tan QJ, Han M, Tian ZH. A convolution-based system for malicious URLs detection. CMC—Computers Materials Continua. 2020; 62 (1):399–411. doi: 10.32604/cmc.2020.06507. [ CrossRef ] [ Google Scholar ]
- Mahbooba B, Timilsina M, Sahal R, Serrano M. Explainable artificial intelligence (XAI) to enhance trust management in intrusion detection systems using decision tree model. Complexity. 2021; 2021 :11. doi: 10.1155/2021/6634811. [ CrossRef ] [ Google Scholar ]
- Mahdavifar S, Ghorbani AA. DeNNeS: Deep embedded neural network expert system for detecting cyber attacks. Neural Computing & Applications. 2020; 32 (18):14753–14780. doi: 10.1007/s00521-020-04830-w. [ CrossRef ] [ Google Scholar ]
- Mahfouz A, Abuhussein A, Venugopal D, Shiva S. Ensemble classifiers for network intrusion detection using a novel network attack dataset. Future Internet. 2020; 12 (11):1–19. doi: 10.3390/fi12110180. [ CrossRef ] [ Google Scholar ]
- Maleks Smith, Z., E. Lostri, and J.A. Lewis. 2020. The hidden costs of cybercrime. https://www.mcafee.com/enterprise/en-us/assets/reports/rp-hidden-costs-of-cybercrime.pdf . Accessed 16 May 2021.
- Malik J, Akhunzada A, Bibi I, Imran M, Musaddiq A, Kim SW. Hybrid deep learning: An efficient reconnaissance and surveillance detection mechanism in SDN. IEEE Access. 2020; 8 :134695–134706. doi: 10.1109/ACCESS.2020.3009849. [ CrossRef ] [ Google Scholar ]
- Manimurugan S. IoT-Fog-Cloud model for anomaly detection using improved Naive Bayes and principal component analysis. Journal of Ambient Intelligence and Humanized Computing. 2020 doi: 10.1007/s12652-020-02723-3. [ CrossRef ] [ Google Scholar ]
- Martin A, Lara-Cabrera R, Camacho D. Android malware detection through hybrid features fusion and ensemble classifiers: The AndroPyTool framework and the OmniDroid dataset. Information Fusion. 2019; 52 :128–142. doi: 10.1016/j.inffus.2018.12.006. [ CrossRef ] [ Google Scholar ]
- Mauro MD, Galatro G, Liotta A. Experimental review of neural-based approaches for network intrusion management. IEEE Transactions on Network and Service Management. 2020; 17 (4):2480–2495. doi: 10.1109/TNSM.2020.3024225. [ CrossRef ] [ Google Scholar ]
- McLeod A, Dolezel D. Cyber-analytics: Modeling factors associated with healthcare data breaches. Decision Support Systems. 2018; 108 :57–68. doi: 10.1016/j.dss.2018.02.007. [ CrossRef ] [ Google Scholar ]
- Meira J, Andrade R, Praca I, Carneiro J, Bolon-Canedo V, Alonso-Betanzos A, Marreiros G. Performance evaluation of unsupervised techniques in cyber-attack anomaly detection. Journal of Ambient Intelligence and Humanized Computing. 2020; 11 (11):4477–4489. doi: 10.1007/s12652-019-01417-9. [ CrossRef ] [ Google Scholar ]
- Miao Y, Ma J, Liu X, Weng J, Li H, Li H. Lightweight fine-grained search over encrypted data in Fog computing. IEEE Transactions on Services Computing. 2019; 12 (5):772–785. doi: 10.1109/TSC.2018.2823309. [ CrossRef ] [ Google Scholar ]
- Miller, C., and C. Valasek. 2015. Remote exploitation of an unaltered passenger vehicle. Black Hat USA 2015 (S 91).
- Mireles JD, Ficke E, Cho JH, Hurley P, Xu SH. Metrics towards measuring cyber agility. IEEE Transactions on Information Forensics and Security. 2019; 14 (12):3217–3232. doi: 10.1109/tifs.2019.2912551. [ CrossRef ] [ Google Scholar ]
- Mishra N, Pandya S. Internet of Things applications, security challenges, attacks, intrusion detection, and future visions: A systematic review. IEEE Access. 2021 doi: 10.1109/ACCESS.2021.3073408. [ CrossRef ] [ Google Scholar ]
- Monshizadeh M, Khatri V, Atli BG, Kantola R, Yan Z. Performance evaluation of a combined anomaly detection platform. IEEE Access. 2019; 7 :100964–100978. doi: 10.1109/ACCESS.2019.2930832. [ CrossRef ] [ Google Scholar ]
- Moreno VC, Reniers G, Salzano E, Cozzani V. Analysis of physical and cyber security-related events in the chemical and process industry. Process Safety and Environmental Protection. 2018; 116 :621–631. doi: 10.1016/j.psep.2018.03.026. [ CrossRef ] [ Google Scholar ]
- Moro ED. Towards an economic cyber loss index for parametric cover based on IT security indicator: A preliminary analysis. Risks. 2020 doi: 10.3390/risks8020045. [ CrossRef ] [ Google Scholar ]
- Moustafa N, Adi E, Turnbull B, Hu J. A new threat intelligence scheme for safeguarding industry 4.0 systems. IEEE Access. 2018; 6 :32910–32924. doi: 10.1109/ACCESS.2018.2844794. [ CrossRef ] [ Google Scholar ]
- Moustakidis S, Karlsson P. A novel feature extraction methodology using Siamese convolutional neural networks for intrusion detection. Cybersecurity. 2020 doi: 10.1186/s42400-020-00056-4. [ CrossRef ] [ Google Scholar ]
- Mukhopadhyay Arunabha, Chatterjee Samir, Bagchi Kallol K, Kirs Peteer J, Shukla Girja K. Cyber Risk Assessment and Mitigation (CRAM) framework using Logit and Probit models for cyber insurance. Information Systems Frontiers. 2019; 21 (5):997–1018. doi: 10.1007/s10796-017-9808-5. [ CrossRef ] [ Google Scholar ]
- Murphey, H. 2021a. Biden signs executive order to strengthen US cyber security. https://www.ft.com/content/4d808359-b504-4014-85f6-68e7a2851bf1?accessToken=zwAAAXl0_ifgkc9NgINZtQRAFNOF9mjnooUb8Q.MEYCIQDw46SFWsMn1iyuz3kvgAmn6mxc0rIVfw10Lg1ovJSfJwIhAK2X2URzfSqHwIS7ddRCvSt2nGC2DcdoiDTG49-4TeEt&sharetype=gift?token=fbcd6323-1ecf-4fc3-b136-b5b0dd6a8756 . Accessed 7 May 2021.
- Murphey, H. 2021b. Millions of connected devices have security flaws, study shows. https://www.ft.com/content/0bf92003-926d-4dee-87d7-b01f7c3e9621?accessToken=zwAAAXnA7f2Ikc8L-SADkm1N7tOH17AffD6WIQ.MEQCIDjBuROvhmYV0Mx3iB0cEV7m5oND1uaCICxJu0mzxM0PAiBam98q9zfHiTB6hKGr1gGl0Azt85yazdpX9K5sI8se3Q&sharetype=gift?token=2538218d-77d9-4dd3-9649-3cb556a34e51 . Accessed 6 May 2021.
- Murugesan V, Shalinie M, Yang MH. Design and analysis of hybrid single packet IP traceback scheme. IET Networks. 2018; 7 (3):141–151. doi: 10.1049/iet-net.2017.0115. [ CrossRef ] [ Google Scholar ]
- Mwitondi KS, Zargari SA. An iterative multiple sampling method for intrusion detection. Information Security Journal. 2018; 27 (4):230–239. doi: 10.1080/19393555.2018.1539790. [ CrossRef ] [ Google Scholar ]
- Neto NN, Madnick S, De Paula AMG, Borges NM. Developing a global data breach database and the challenges encountered. ACM Journal of Data and Information Quality. 2021; 13 (1):33. doi: 10.1145/3439873. [ CrossRef ] [ Google Scholar ]
- Nurse, J.R.C., L. Axon, A. Erola, I. Agrafiotis, M. Goldsmith, and S. Creese. 2020. The data that drives cyber insurance: A study into the underwriting and claims processes. In 2020 International conference on cyber situational awareness, data analytics and assessment (CyberSA), 15–19 June 2020.
- Oliveira N, Praca I, Maia E, Sousa O. Intelligent cyber attack detection and classification for network-based intrusion detection systems. Applied Sciences—Basel. 2021; 11 (4):21. doi: 10.3390/app11041674. [ CrossRef ] [ Google Scholar ]
- Page Matthew J, McKenzie Joanne E, Bossuyt Patrick M, Boutron Isabelle, Hoffmann Tammy C, Mulrow Cynthia D, Shamseer Larissa, Tetzlaff Jennifer M, Akl Elie A, Brennan Sue E, Chou Roger, Glanville Julie, Grimshaw Jeremy M, Hróbjartsson Asbjørn, Lalu Manoj M, Li Tianjing, Loder Elizabeth W, Mayo-Wilson Evan, McDonald Steve, McGuinness Luke A, Stewart Lesley A, Thomas James, Tricco Andrea C, Welch Vivian A, Whiting Penny, Moher David. The PRISMA 2020 statement: An updated guideline for reporting systematic reviews. Systematic Reviews. 2021; 10 (1):89. doi: 10.1186/s13643-021-01626-4. [ PMC free article ] [ PubMed ] [ CrossRef ] [ Google Scholar ]
- Pajouh HH, Javidan R, Khayami R, Dehghantanha A, Choo KR. A two-layer dimension reduction and two-tier classification model for anomaly-based intrusion detection in IoT backbone networks. IEEE Transactions on Emerging Topics in Computing. 2019; 7 (2):314–323. doi: 10.1109/TETC.2016.2633228. [ CrossRef ] [ Google Scholar ]
- Parra GD, Rad P, Choo KKR, Beebe N. Detecting Internet of Things attacks using distributed deep learning. Journal of Network and Computer Applications. 2020; 163 :13. doi: 10.1016/j.jnca.2020.102662. [ CrossRef ] [ Google Scholar ]
- Paté-Cornell ME, Kuypers M, Smith M, Keller P. Cyber risk management for critical infrastructure: A risk analysis model and three case studies. Risk Analysis. 2018; 38 (2):226–241. doi: 10.1111/risa.12844. [ PubMed ] [ CrossRef ] [ Google Scholar ]
- Pooser, D.M., M.J. Browne, and O. Arkhangelska. 2018. Growth in the perception of cyber risk: evidence from U.S. P&C Insurers. The Geneva Papers on Risk and Insurance—Issues and Practice 43 (2): 208–223. 10.1057/s41288-017-0077-9.
- Pu, G., L. Wang, J. Shen, and F. Dong. 2021. A hybrid unsupervised clustering-based anomaly detection method. Tsinghua Science and Technology 26 (2): 146–153. 10.26599/TST.2019.9010051.
- Qiu J, Luo W, Pan L, Tai Y, Zhang J, Xiang Y. Predicting the impact of android malicious samples via machine learning. IEEE Access. 2019; 7 :66304–66316. doi: 10.1109/ACCESS.2019.2914311. [ CrossRef ] [ Google Scholar ]
- Qu X, Yang L, Guo K, Sun M, Ma L, Feng T, Ren S, Li K, Ma X. Direct batch growth hierarchical self-organizing mapping based on statistics for efficient network intrusion detection. IEEE Access. 2020; 8 :42251–42260. doi: 10.1109/ACCESS.2020.2976810. [ CrossRef ] [ Google Scholar ]
- Shafiur Rahman, Md, Sajal Halder Md, Uddin Ashraf, Acharjee Uzzal Kumar. An efficient hybrid system for anomaly detection in social networks. Cybersecurity. 2021; 4 (1):10. doi: 10.1186/s42400-021-00074-w. [ CrossRef ] [ Google Scholar ]
- Ramaiah M, Chandrasekaran V, Ravi V, Kumar N. An intrusion detection system using optimized deep neural network architecture. Transactions on Emerging Telecommunications Technologies. 2021; 32 (4):17. doi: 10.1002/ett.4221. [ CrossRef ] [ Google Scholar ]
- Raman, M.R.G., K. Kannan, S.K. Pal, and V.S.S. Sriram. 2016. Rough set-hypergraph-based feature selection approach for intrusion detection systems. Defence Science Journal 66 (6): 612–617. 10.14429/dsj.66.10802.
- Rathore, S., J.H. Park. 2018. Semi-supervised learning based distributed attack detection framework for IoT. Applied Soft Computing 72: 79–89. 10.1016/j.asoc.2018.05.049.
- Romanosky Sasha, Ablon Lillian, Kuehn Andreas, Jones Therese. Content analysis of cyber insurance policies: How do carriers price cyber risk? Journal of Cybersecurity (oxford) 2019; 5 (1):tyz002. [ Google Scholar ]
- Sarabi A, Naghizadeh P, Liu Y, Liu M. Risky business: Fine-grained data breach prediction using business profiles. Journal of Cybersecurity. 2016; 2 (1):15–28. doi: 10.1093/cybsec/tyw004. [ CrossRef ] [ Google Scholar ]
- Sardi Alberto, Rizzi Alessandro, Sorano Enrico, Guerrieri Anna. Cyber risk in health facilities: A systematic literature review. Sustainability. 2021; 12 (17):7002. doi: 10.3390/su12177002. [ CrossRef ] [ Google Scholar ]
- Sarker Iqbal H, Kayes ASM, Badsha Shahriar, Alqahtani Hamed, Watters Paul, Ng Alex. Cybersecurity data science: An overview from machine learning perspective. Journal of Big Data. 2020; 7 (1):41. doi: 10.1186/s40537-020-00318-5. [ CrossRef ] [ Google Scholar ]
- Scopus. 2021. Factsheet. https://www.elsevier.com/__data/assets/pdf_file/0017/114533/Scopus_GlobalResearch_Factsheet2019_FINAL_WEB.pdf . Accessed 11 May 2021.
- Sentuna A, Alsadoon A, Prasad PWC, Saadeh M, Alsadoon OH. A novel Enhanced Naïve Bayes Posterior Probability (ENBPP) using machine learning: Cyber threat analysis. Neural Processing Letters. 2021; 53 (1):177–209. doi: 10.1007/s11063-020-10381-x. [ CrossRef ] [ Google Scholar ]
- Shaukat K, Luo SH, Varadharajan V, Hameed IA, Chen S, Liu DX, Li JM. Performance comparison and current challenges of using machine learning techniques in cybersecurity. Energies. 2020; 13 (10):27. doi: 10.3390/en13102509. [ CrossRef ] [ Google Scholar ]
- Sheehan B, Murphy F, Mullins M, Ryan C. Connected and autonomous vehicles: A cyber-risk classification framework. Transportation Research Part a: Policy and Practice. 2019; 124 :523–536. doi: 10.1016/j.tra.2018.06.033. [ CrossRef ] [ Google Scholar ]
- Sheehan Barry, Murphy Finbarr, Kia Arash N, Kiely Ronan. A quantitative bow-tie cyber risk classification and assessment framework. Journal of Risk Research. 2021; 24 (12):1619–1638. doi: 10.1080/13669877.2021.1900337. [ CrossRef ] [ Google Scholar ]
- Shlomo A, Kalech M, Moskovitch R. Temporal pattern-based malicious activity detection in SCADA systems. Computers & Security. 2021; 102 :17. doi: 10.1016/j.cose.2020.102153. [ CrossRef ] [ Google Scholar ]
- Singh KJ, De T. Efficient classification of DDoS attacks using an ensemble feature selection algorithm. Journal of Intelligent Systems. 2020; 29 (1):71–83. doi: 10.1515/jisys-2017-0472. [ CrossRef ] [ Google Scholar ]
- Skrjanc I, Ozawa S, Ban T, Dovzan D. Large-scale cyber attacks monitoring using Evolving Cauchy Possibilistic Clustering. Applied Soft Computing. 2018; 62 :592–601. doi: 10.1016/j.asoc.2017.11.008. [ CrossRef ] [ Google Scholar ]
- Smart, W. 2018. Lessons learned review of the WannaCry Ransomware Cyber Attack. https://www.england.nhs.uk/wp-content/uploads/2018/02/lessons-learned-review-wannacry-ransomware-cyber-attack-cio-review.pdf . Accessed 7 May 2021.
- Sornette D, Maillart T, Kröger W. Exploring the limits of safety analysis in complex technological systems. International Journal of Disaster Risk Reduction. 2013; 6 :59–66. doi: 10.1016/j.ijdrr.2013.04.002. [ CrossRef ] [ Google Scholar ]
- Sovacool Benjamin K. The costs of failure: A preliminary assessment of major energy accidents, 1907–2007. Energy Policy. 2008; 36 (5):1802–1820. doi: 10.1016/j.enpol.2008.01.040. [ CrossRef ] [ Google Scholar ]
- SpringerLink. 2021. Journal Search. https://rd.springer.com/search?facet-content-type=%22Journal%22 . Accessed 11 May 2021.
- Stojanovic B, Hofer-Schmitz K, Kleb U. APT datasets and attack modeling for automated detection methods: A review. Computers & Security. 2020; 92 :19. doi: 10.1016/j.cose.2020.101734. [ CrossRef ] [ Google Scholar ]
- Subroto A, Apriyana A. Cyber risk prediction through social media big data analytics and statistical machine learning. Journal of Big Data. 2019 doi: 10.1186/s40537-019-0216-1. [ CrossRef ] [ Google Scholar ]
- Tan Z, Jamdagni A, He X, Nanda P, Liu RP, Hu J. Detection of denial-of-service attacks based on computer vision techniques. IEEE Transactions on Computers. 2015; 64 (9):2519–2533. doi: 10.1109/TC.2014.2375218. [ CrossRef ] [ Google Scholar ]
- Tidy, J. 2021. Irish cyber-attack: Hackers bail out Irish health service for free. https://www.bbc.com/news/world-europe-57197688 . Accessed 6 May 2021.
- Tuncer T, Ertam F, Dogan S. Automated malware recognition method based on local neighborhood binary pattern. Multimedia Tools and Applications. 2020; 79 (37–38):27815–27832. doi: 10.1007/s11042-020-09376-6. [ CrossRef ] [ Google Scholar ]
- Uhm Y, Pak W. Service-aware two-level partitioning for machine learning-based network intrusion detection with high performance and high scalability. IEEE Access. 2021; 9 :6608–6622. doi: 10.1109/ACCESS.2020.3048900. [ CrossRef ] [ Google Scholar ]
- Ulven JB, Wangen G. A systematic review of cybersecurity risks in higher education. Future Internet. 2021; 13 (2):1–40. doi: 10.3390/fi13020039. [ CrossRef ] [ Google Scholar ]
- Vaccari I, Chiola G, Aiello M, Mongelli M, Cambiaso E. MQTTset, a new dataset for machine learning techniques on MQTT. Sensors. 2020; 20 (22):17. doi: 10.3390/s20226578. [ PMC free article ] [ PubMed ] [ CrossRef ] [ Google Scholar ]
- Valeriano B, Maness RC. The dynamics of cyber conflict between rival antagonists, 2001–11. Journal of Peace Research. 2014; 51 (3):347–360. doi: 10.1177/0022343313518940. [ CrossRef ] [ Google Scholar ]
- Varghese JE, Muniyal B. An Efficient IDS framework for DDoS attacks in SDN environment. IEEE Access. 2021; 9 :69680–69699. doi: 10.1109/ACCESS.2021.3078065. [ CrossRef ] [ Google Scholar ]
- Varsha M. V., Vinod P., Dhanya K. A. Identification of malicious android app using manifest and opcode features. Journal of Computer Virology and Hacking Techniques. 2017; 13 (2):125–138. doi: 10.1007/s11416-016-0277-z. [ CrossRef ] [ Google Scholar ]
- Velliangiri S, Pandey HM. Fuzzy-Taylor-elephant herd optimization inspired Deep Belief Network for DDoS attack detection and comparison with state-of-the-arts algorithms. Future Generation Computer Systems—the International Journal of Escience. 2020; 110 :80–90. doi: 10.1016/j.future.2020.03.049. [ CrossRef ] [ Google Scholar ]
- Verma A, Ranga V. Machine learning based intrusion detection systems for IoT applications. Wireless Personal Communications. 2020; 111 (4):2287–2310. doi: 10.1007/s11277-019-06986-8. [ CrossRef ] [ Google Scholar ]
- Vidros S, Kolias C, Kambourakis G, Akoglu L. Automatic detection of online recruitment frauds: Characteristics, methods, and a public dataset. Future Internet. 2017; 9 (1):19. doi: 10.3390/fi9010006. [ CrossRef ] [ Google Scholar ]
- Vinayakumar R, Alazab M, Soman KP, Poornachandran P, Al-Nemrat A, Venkatraman S. Deep learning approach for intelligent intrusion detection system. IEEE Access. 2019; 7 :41525–41550. doi: 10.1109/access.2019.2895334. [ CrossRef ] [ Google Scholar ]
- Walker-Roberts S, Hammoudeh M, Aldabbas O, Aydin M, Dehghantanha A. Threats on the horizon: Understanding security threats in the era of cyber-physical systems. Journal of Supercomputing. 2020; 76 (4):2643–2664. doi: 10.1007/s11227-019-03028-9. [ CrossRef ] [ Google Scholar ]
- Web of Science. 2021. Web of Science: Science Citation Index Expanded. https://clarivate.com/webofsciencegroup/solutions/webofscience-scie/ . Accessed 11 May 2021.
- World Economic Forum. 2020. WEF Global Risk Report. http://www3.weforum.org/docs/WEF_Global_Risk_Report_2020.pdf . Accessed 13 May 2020.
- Xin Y, Kong L, Liu Z, Chen Y, Li Y, Zhu H, Gao M, Hou H, Wang C. Machine learning and deep learning methods for cybersecurity. IEEE Access. 2018; 6 :35365–35381. doi: 10.1109/ACCESS.2018.2836950. [ CrossRef ] [ Google Scholar ]
- Xu, C., J. Zhang, K. Chang, and C. Long. 2013. Uncovering collusive spammers in Chinese review websites. In Proceedings of the 22nd ACM international conference on Information & Knowledge Management.
- Yang J, Li T, Liang G, He W, Zhao Y. A Simple recurrent unit model based intrusion detection system with DCGAN. IEEE Access. 2019; 7 :83286–83296. doi: 10.1109/ACCESS.2019.2922692. [ CrossRef ] [ Google Scholar ]
- Yuan BG, Wang JF, Liu D, Guo W, Wu P, Bao XH. Byte-level malware classification based on Markov images and deep learning. Computers & Security. 2020; 92 :12. doi: 10.1016/j.cose.2020.101740. [ CrossRef ] [ Google Scholar ]
- Zhang S, Ou XM, Caragea D. Predicting cyber risks through national vulnerability database. Information Security Journal. 2015; 24 (4–6):194–206. doi: 10.1080/19393555.2015.1111961. [ CrossRef ] [ Google Scholar ]
- Zhang Ying, Li Peisong, Wang Xinheng. Intrusion detection for IoT based on improved genetic algorithm and deep belief network. IEEE Access. 2019; 7 :31711–31722. doi: 10.1109/ACCESS.2019.2903723. [ CrossRef ] [ Google Scholar ]
- Zheng, Muwei, Hannah Robbins, Zimo Chai, Prakash Thapa, and Tyler Moore. 2018. Cybersecurity research datasets: taxonomy and empirical analysis. In 11th {USENIX} workshop on cyber security experimentation and test ({CSET} 18).
- Zhou X, Liang W, Shimizu S, Ma J, Jin Q. Siamese neural network based few-shot learning for anomaly detection in industrial cyber-physical systems. IEEE Transactions on Industrial Informatics. 2021; 17 (8):5790–5798. doi: 10.1109/TII.2020.3047675. [ CrossRef ] [ Google Scholar ]
- Zhou YY, Cheng G, Jiang SQ, Dai M. Building an efficient intrusion detection system based on feature selection and ensemble classifier. Computer Networks. 2020; 174 :17. doi: 10.1016/j.comnet.2020.107247. [ CrossRef ] [ Google Scholar ]
Thank you for visiting nature.com. You are using a browser version with limited support for CSS. To obtain the best experience, we recommend you use a more up to date browser (or turn off compatibility mode in Internet Explorer). In the meantime, to ensure continued support, we are displaying the site without styles and JavaScript.
- View all journals
- Explore content
- About the journal
- Publish with us
- Sign up for alerts
- Open access
- Published: 05 September 2024
An intelligent dynamic cyber physical system threat detection system for ensuring secured communication in 6G autonomous vehicle networks
- Shanthalakshmi M ORCID: orcid.org/0009-0003-4234-7125 1 &
- Ponmagal R S ORCID: orcid.org/0000-0002-7228-1310 1
Scientific Reports volume 14 , Article number: 20795 ( 2024 ) Cite this article
Metrics details
- Engineering
- Mathematics and computing
Smart cities have developed advanced technology that improves people’s lives. A collaboration of smart cities with autonomous vehicles shows the development towards a more advanced future. Cyber-physical system (CPS) are used blend the cyber and physical world, combined with electronic and mechanical systems, Autonomous vehicles (AVs) provide an ideal model of CPS. The integration of 6G technology with Autonomous Vehicles (AVs) marks a significant advancement in Intelligent Transportation Systems (ITS), offering enhanced self-sufficiency, intelligence, and effectiveness. Autonomous vehicles rely on a complex network of sensors, cameras, and software to operate. A cyber-attack could interfere with these systems, leading to accidents, injuries, or fatalities. Autonomous vehicles are often connected to broader transportation networks and infrastructure. A successful cyber-attack could disrupt not only individual vehicles but also public transportation systems, causing widespread chaos and economic damage. Autonomous vehicles communicate with other vehicles (V2V) and infrastructure (V2I) for safe and efficient operation. If these communication channels are compromised, it could lead to collisions, traffic jams, or other dangerous situations. So we present a novel approach to mitigating these security risks by leveraging pre-trained Convolutional Neural Network (CNN) models for dynamic cyber-attack detection within the cyber-physical systems (CPS) framework of AVs. The proposed Intelligent Intrusion Detection System (IIDS) employs a combination of advanced learning techniques, including Data Fusion, One-Class Support Vector Machine, Random Forest, and k-Nearest Neighbor, to improve detection accuracy. The study demonstrates that the EfficientNet model achieves superior performance with an accuracy of up to 99.97%, highlighting its potential to significantly enhance the security of AV networks. This research contributes to the development of intelligent cyber-security models that align with 6G standards, ultimately supporting the safe and efficient integration of AVs into smart cities.
Introduction
The rapid progress of 6G technology holds the potential to improve Intelligent Transportation Systems (ITS) making them more self-sufficient, smart and effective. Traditional ITS technologies have been widely adopted globally leading to enhancements, in vehicle capabilities. The economy’s rapid expansion and urbanization’s speed up have resulted in an increase in car ownership. By connecting transportation tools to traffic assets, Intelligent Transportation Systems (ITS) are a promising solution for traffic control that addresses the needs of linking people with other services 3 .
Today, with the advent of urbanization, there is an increasing demand for intelligent ITS systems that can tackle problems like gridlock, boost traffic movement, minimize accidents and reduce energy usage and environmental pollution.6G will outdo personalized communications through full integration of the Internet of Everything (IoE) concept which connects people, vehicles, computing resources, wearable and sensors devices even robotic entities among others 36 . These challenges encompass resource management, privacy protection, security issues, learning and communication difficulties, standardized protocols, and the deployment of machine learning capabilities on IoT sensors 46 .
The Space Air Ground Underwater Network (SAGUN) is expected to form the main architecture for the upcoming 6G network so as to facilitate fast and seamless connectivity 24 , 25 . Apart from improving communication metrics other researchers consider Artificial Intelligence (AI) as shaping 6G too. Advanced machine learning technologies are viewed as good tools for addressing complex challenges effectively 26 , 51 .
Modern sensors, computation and network technologies come together in a cyber-physical system (CPS) to blend the cyber and physical world. On the other hand, if combined with electronic and mechanical systems, autonomous vehicles (AVs) provide an ideal model of CPS. AV components can be connected through sensors, ECUs and actuators in various vehicle network systems. Some common examples of such network systems are: FlexRay, Local Interconnect Networks (LIN) Controller Area Networks (CAN) and Time-Triggered CAN (TTCAN).
More research presents a layer-based classification of cyberattacks, grading them by integrity, availability, confidentiality, and accountability. It emphasizes the need for new approaches to address security issues in smart grids without reducing efficiency 45 . Addressing these speed and security issues through effective regulations and best practices is essential for safe and efficient integration into urban environments 47 , 56 .
The development of internet-based smart appliances is a result of remarkable advancements in IoT as well as embedded systems. This has seen traditional cars transformed into “smart” fully functional machines that offer convenience while in transit. The ground-breaking features and possibilities provided by modern technology are the foundation for intelligent vehicles. Daily affairs have necessitated people to purchase these intelligent cars because they value comfort and safety above all else 35 . They possess attributes such as eco-friendly consciousness, online capability, and adherence to traffic provisions, self-piloting capacity, rapid decision-making abilities, passenger and pedestrian safety including parking among others. These autonomous vehicles are popularly known as driverless cars and mark the peak of smart vehicle inventions today. Autonomous driving is being considered the next disruptive innovation. Among image processing techniques involving neural networks, convolutional neural networks (CNNs) have been used because of their high performance in extracting information from images using convolution. Process 2D inputs through several layers for extraction of complicated features and find out useful patterns on a picture based on how pixels are arranged spatially. No preprocessing is required for CNNs since they are simple to implement.
The advantages to integrating ML throughout the network, there are still security issues, mostly with regard to authentication and data integrity. Notwithstanding its drawbacks, machine learning (ML) exhibits potential in domains such as personal identification and health data analysis; however, confidentiality and vulnerability management are critical 48 . To enhance IDS, researchers incorporated a feature selection and classification mechanism, which prioritizes essential information by removing unnecessary attributes from datasets 8 . Through their study, the researchers found that Decision Trees were the most effective ML technique for defending AVs against IDS attacks due to their reliability and consistency. This highlights the importance of using appropriate ML algorithms to enhance IDS performance in identifying and mitigating cyber-attacks in AVs.
Figure 1 represents the design of autonomous vehicle system. We can deploy VANET to meet your needs by accessing resources and connecting to the Internet while on the move. Additionally, other potential applications in health and safety, intelligent transportation systems, military systems, etc. were also expected. VANET allows you to group vehicles based on route, mobility, and on-road behavior, allowing you to maintain an adaptive approach to traffic monitoring and pollution density. The AV also uses this information to make adaptive decisions regarding route selection. Once the government approves vehicle-to-vehicle communication and all cars adapt to it, it will become clear why it is so important to use VANET for tracking, navigation, routing or communication.
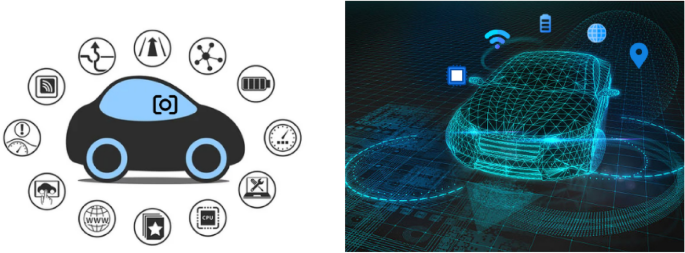
Autonomous vehicle design.
Generation | History | Advantages | Disadvantages |
---|---|---|---|
1G (Analog) | Late 1970s—Early 1980s | Pioneered mobile wireless communication Enabled widespread adoption of mobile phones | Poor voice quality and security Limited capacity and coverage High susceptibility to interference and eavesdropping |
2G (Digital) | Early 1990s | Improved voice quality and capacity Introduction of SMS and basic data services Enhanced security and encryption | Limited data transmission rates Early 2G networks had slow data services compared to later generations |
3G (Mobile broadband) | Early 2000s | High-speed data transmission, supporting video calls and mobile internet Enhanced capacity and coverage Support for a wide range of multimedia services | Higher costs for network deployment and maintenance Power consumption concerns for mobile devices |
4G (High-speed mobile internet) | Late 2000s | Significantly higher data rates and capacity Improved spectral efficiency Support for HD video streaming, VoIP, and enhanced gaming experiences | Expensive infrastructure upgrades Potential for network congestion with increased data usage Battery life concerns for mobile devices |
5G (Ultra-Fast, Low latency) | Late 2010s | Extremely high data rates and low latency Enhanced capacity for IoT devices and smart cities Support for autonomous vehicles and advanced industrial applications | High deployment costs and complexity Limited initial coverage, especially in rural areas Potential health concerns over higher frequency bands |
6G (Future vision) | Expected around 2030 | Projected to offer even higher data rates, ultra-low latency, and greater reliability Integration of AI for network optimization and autonomous operations Enhanced support for holographic communications and advanced VR/AR applications | Anticipated high costs for development and deployment Potential challenges in spectrum allocation and management Privacy and security concerns with highly connected environments |
Objectives and motivation
In this work, we emphasize that all adopted regulations influence the vulnerabilities of devices used in AV, and these are directly linked to the intelligence level of the vehicle. Additionally, operational regulations with practical restrictions should govern the features of these AV devices. Motivated by these considerations, our objectives include: improving the reliability of the devices to prevent unnecessary vulnerabilities; safeguarding the services of all devices integrated into AVs; and developing an intelligent cybersecurity model that influences the policies adopted for AVs and their devices.
The widespread adoption of AVs has driven the development of numerous internal and external devices, such as sensors. As the number of 6G users increases, so does the connectivity, mobility, and vulnerability of the devices integrated into AVs. This leads to more interactions and a higher volume of unsecured communications. This research aims to mitigate these issues, including the associated costs and overall energy consumption. A system becomes vulnerable and a target for hackers when accurate policies are not provided promptly, leaving the system defenseless. It is crucial for the intelligent decision-makers within these systems to deliver policies promptly; otherwise, services, such as those of banks, will be susceptible to attacks. Timely delivery means considering all aspects, including clients, servers, and all interface links and communications.
Major contribution of the proposed work
In our proposed approach, we utilized policies derived from 6G guidelines. Intelligent cyber-security aims to enhance the cyber-security solutions of AV services by implementing policies based on 6G requirements and intelligence levels, which are directly related to the robustness of the policies. As the strength of the policies increases, the intelligence level in intelligent cyber-security solutions also rises.
This paper makes the following contributions:
Development of a technique that utilizes ConvNet (CNN) models to detect cyber-attacks on the interlinked components of AVs via the Controller Area Network (CAN).
Addressing the vulnerabilities of the CAN communication protocol within 6G vehicle networks, highlighting its lack of encryption and authentication, which poses risks to network security and the safety of individuals.
Utilization of pre-trained CNN models for the detection of cyber-attacks based on the Interactions and Interdependent Data Structures (IIDS) mechanism.
Creation of the IIDS mechanism, which enhances cyber-attack detection capabilities and Integration of Multiple Learning Techniques: Employment of four distinct learning techniques within the IIDS mechanism: Data Fusion, One-Class Support Vector Machine, Random Forest, and k-Nearest Neighbor.
The following topics are addressed later in this paper. Sect. “ Related work ” discusses the various research studies conducted recently and the available approaches of detecting CPS cyber-attacks. The discussion about the proposed solution with the implementation of AV-CPS is in sect. “ Methodology ”. Its efficiency has been determined through result analysis contained in sect. “ Experimentation and result discussion ”, while it has been proved to be more accurate than any usual approach suggested in sect. “ Experimentation and result discussion ”. In sect. “ Conclusion ”, conclusion is given.
Related work
Smart cities and their applications and services are at a higher development pace due to the rapid advances in artificial intelligence, communications, and remote sensing. To enhance living standards of smart cities, various smart services in such areas as communications, cyber security, smart grids, healthcare, and transportation systems are emerging and being industrialized. The main attention was focused on intelligent mobility and intelligent transportation systems that include autonomous cars. It is predicted that within a few years more than 300 million AV’s will be connected to roadside units. Nevertheless, despite the tremendous growth of the industry of self-driving cars these vehicles still remain susceptible to multiple cyber-attacks with consequences ranging from minor disruptions to severe threats against life and health of individuals 2 . There is a broad scope for possibilities. Therefore multiple researches have been done on this issue as well as numerous systems created to study, recognize and diminish cyber-attacks or threats occurring in the autonomous vehicle system Most of these studies integrate diverse machine learning (ML) techniques with cyber security measures to build security and defense systems for them.
In this regard, it will be significant to note that a new intelligent intrusion detection method has been proposed towards safeguarding autonomous vehicle’s external communications 9 . This mechanism exploits a blend of hybrid intelligent intrusion system comprising overlapping criteria proportional score (POS) techniques, multilayer perceptron (MLPs), and fuzzy sets that detect activity in connected and communicating self-driving cars 11 . To spot denial of service attacks (DoS), we apply the hybrid IDS back propagation neural network. Experimental evaluation results have shown that this detection system is very effective in identifying DoS attacks in autonomous vehicles. However, these models are related with increased interpretation costs because of computational processing by different sub-systems such as pre-processing sub-system, feature extraction sub-system with POS module and fuzzification sub-system. They have given a technique to detect wrong insertion of data in self-driven cars 19 , 20 , 21 .
Guo et al. 57 proposed a two-tiered security framework. The first component is a reinforcement learning model designed to evaluate message credibility. To enhance the accuracy of these evaluations, a context-aware trust management model is introduced. This second component selects the most appropriate evaluation method, thereby improving the overall precision of the framework 57 .
This is where he has his three subsystems. False Data Insertion (FDI) Subsystem imitates attacks on self-driving cars. The Cyber Attack Dataset Collection (CDC) Subsystem. Normal and attack mode simulation models for creating and collecting of data 23 . A deep long-term short term memory (LSTM) network is used by the Intrusion Detection Mechanism (IDM) Subsystem to detect types of cyber-attacks such as FDI attacks on vehicle control systems 30 . The system labels data samples as normal or abnormal. Results from their experimental evaluation show that their model worked well with high detection rates compared to other state-of-the-art methods 33 , 39 . However, their proposed system was only tested against simulated data sets without incorporating any control communication system in the self-driven car.
The authors of 49 developed a novel auto encoder-based detection framework for identifying attacks in Industrial IoT (IIoT) networks, utilizing Recurrent Neural Networks (LSTM) and Convolutional Neural Networks (CNN). The key advantage of this framework is its ability to detect both novel (zero-day) and conventional IIoT attacks by combining LSTM and CNN 54 . Additionally, each prediction made by the CNN-LSTM model is accompanied by a local explanation using the LIME technique. Similarly, Khan et al. 50 introduced an unsupervised anomaly detection system aimed at identifying IP-based attacks such as denial-of-service (DoS), reconnaissance, exploits, fuzzes, and generic attacks. The system operates in two phases. The first phase employs two models based on the conventional state-based approach, while the second phase utilizes a bidirectional LSTM-based technique 53 . These models were implemented at the gateway of the connected vehicle. The system was evaluated using the UNSW-NB15 dataset, and its effectiveness was measured using accuracy, recall, precision, and F1-score.
Smart transportation systems, particularly those reliant on autonomous vehicles, face significant security and privacy risks. Despite their potential to improve traffic flow, safety, and environmental impact, these complex systems are vulnerable to cyber- attacks. To address these challenges, innovative network structures like Space-Air-Ground Integrated Vehicular Networks (SAGIVNs) are being explored. However, even these advanced systems require robust security measures to protect against threats that could compromise user privacy and system integrity. Ultimately, the successful implementation of smart transportation hinges on developing effective solutions that safeguard both privacy and security 52 .
Smart transportation systems, particularly those involving AVs, face significant cyber physical risks. The increasing reliance on interconnected devices (IoT) creates vulnerabilities similar to those seen in other sectors like utilities and manufacturing. Protecting these systems requires a comprehensive approach beyond traditional digital security. The complex interplay of digital and physical components in these systems demands new security measures. Existing digital security practices are insufficient to protect against the evolving threats in this domain.
Essentially, the integration of numerous sensors and actuators in autonomous vehicles, while enabling advanced features, also creates a larger attack surface. To safeguard these systems, a holistic security strategy is crucial, considering both digital and physical vulnerabilities.
Methodology
In smart cities, Autonomous Vehicles (AVs) are a typical example of how Cyber-Physical Systems (CPS) principles work and they raise the standards of urban life through curbing energy consumption and air pollution. For instance, cloud computing is very important in AV-CPS especially when it comes to IT integration and communication processes which should be scalable for real-time data processing, analysis and storage as well as promoting smooth operation of such systems in smart urban areas. Moreover, AVs are prone to cyber-attacks such as key fob cloning, telematics service disruptions radar interference sensor spoofing ultrasonic sensor tampering camera sensor attacks lidar sensor interference etcetera including emergent threats e.g. ransomware vehicle theft among others. As a result, this article proposes a technique that employs pre-trained ConvNet models to expose the cyberattacks targeted at the interlinked mechanical components of motor vehicles using the CAN communication protocol.
Simulation of AV systems
Simulation is a computerized model used for assessing the performance of a prototype before it moves to production. Using simulation models rather than physical models has several advantages including cost effectiveness, ease of implementation, testing and maintenance. The self-driving car system research employs a simulation model of an autonomous vehicle system which consists of front vehicle and self-propelled vehicle (self-driving car). The self-propelled vehicle should at all times maintain its distance from the front vehicle using the ACC under perfect conditions. Hence, it is necessary for the two-wheeler on the rear side to keep constant track of where its leading counterpart is situated. In this case, we will focus on three important components that make up the self-propelled vehicle: Velocity sensor, position sensor and ACC as indicated in Fig. 2 .The velocity sensor is used to measure the speed while the position sensor detects how far away from both cars are from each other. ACC receives these readings and subsequently adjusts the speed so as to match that of the front car.
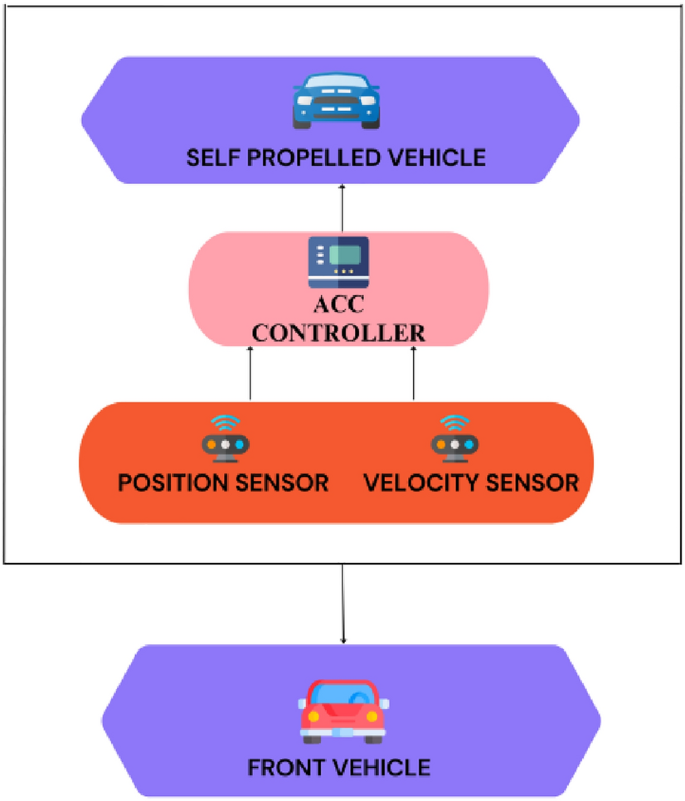
Simulation of AV systems.
CAN communication network
To reduce the increasing number of wires in cars that hampered their reliability, Bosch developed CAN protocol in 1985. Although it processes only a limited amount of real-time sensor data, CAN is a standard communication protocol for managing vehicle control and sensor data 18 . Furthermore, to collect data flows from all major automotive core control systems: engine transmission body systems among others into CAN bus broadcasting each piece of information to the CAN bus. There is always an open network at any node that implies that any CAN network node in a vehicle can be attacked by harmful internal or external sources. As more sensing and communication equipment is required for independent operation of AVs, security risks increase with autonomy levels.
CAN Network serves as a utilized communication protocol in 6G vehicle networks; however its lack of encryption and authentication exposes vulnerabilities to attacks and misconduct, by vehicle users posing risks to network security and the safety of vehicle occupants. The most used communication protocol in 6G vehicle networks is CAN. This can make the network vulnerable to attacks and harmful acts by motorists that could put the lives of passengers at risk because it does not have encryption and authentication measures to protect against such events 10 . Consequently, there is a pressing need for better intrusion detection systems in 6G vehicular networks that allow the network to modify its environment in order to meet different application requirements and service types as fast as possible. Figure 3 represents CAN data transfer system.
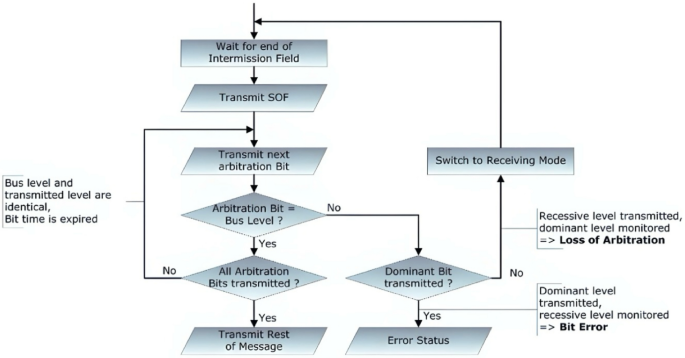
CAN data transfer structure.
The CAN bus acts as a broadcast system, allowing all nodes to receive all transmissions. It’s not possible to target a message to a specific node; all nodes inevitably capture all traffic. However, the CAN hardware offers local filtering, enabling nodes to respond solely to pertinent messages 43 . CAN employ brief messages with a maximum payload of 94 bits. Messages do not contain explicit addresses; rather, they are content-addressed, meaning the content implicitly defines their destination.
Autonomous Vehicle—Cyber Physical Systems
Cyber-Physical Systems (CPS) combines computation, verbal exchange, and physical techniques, ranging from small-scale wearable clinical gadgets to massive-scale countrywide energy grids 15 . The middle concept of CPS is the seamless integration of cyber components and physical elements, with applications in car, avionics, robotics, healthcare, and energy grids 1 . CPS design historically separates manage set of rules concerns (handling bodily dynamics) from cyber implementation structures (data processing and networking).
Autonomous Vehicles (AVs), alternatively, are able to navigating and making choices without human input. They utilize sensors (like lidar, radar, and cameras), on-board computers, and control algorithms to sense their environment and make riding selections. AVs’ levels of autonomy range from driver assistance structures (such as adaptive cruise manage) to finish autonomy (no human intervention) 4 . Figure 4 represents autonomous vehicle systems in collaboration with cyber physical systems.
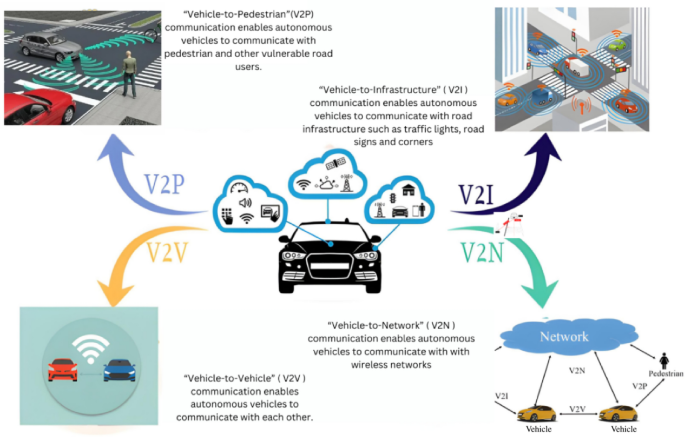
AV in collaboration with CPS module.
Relationship between Autonomous Vehicles and Cyber-Physical Systems:
1. Physical aspect:—AVs interact with the bodily global, such as roads, traffic, pedestrians, and weather conditions.—Their movements (steering, braking, and acceleration) have direct environmental effects.
2. Cyber aspect:—AVs rely upon cyber components:—Sensor Fusion: AVs integrate records from a couple of sensors (cyber) to form a entire environmental photograph (physical).—Control Algorithms: AVs employ manage algorithms (cyber) to handle physical dynamics (ex, lane upkeep, obstacle avoidance).—Communication Networks: AVs use wireless networks (cyber) to talk with different vehicles (V2V) and infrastructure (V2I).—Safety–Critical Systems: AVs’ dependable and secure operation includes cyber additives.—Machine Learning: AVs follow system getting to know algorithms (cyber) to beautify belief.
The self-driving motorcar—cyber physical system is made up of the following subsystems: Sensors, actuators, two CAN nodes with names Node A and B and a controller. Nodes A&B communicates with each other through the signals as shown in Fig. 4 and the same is simulated with the activity of the CAN communication within the AV-CPS. Therefore, node A will get these signals.
The current location of the self-propelled vehicle.
The current position of the front vehicle
The current speed of the self-propelled vehicle
The current speed of the front vehicle
The temporal interval
The Target velocity
The position of the vehicle is measured in meters and velocity in m/s. The time interval between the car ahead and the self-propelled vehicle is 1.4 s. Next, the target speed is 30 m/s Upon receipt of such signals by ACC from Output, it will produce a control signal for adjusting the speed of self-propelled vehicle to meet up with a target velocity or front vehicle’s position. In turn, nodes B send these commands to actuators which convert them into physical motion of the vehicle.
Dataset generation
Let us assume that the implementation of AV-CPS is done with various components like sensors,ACC controller,Node A,Node B,actuators as shown in Fig. 5 . There is a possibility that an attacker may insert any malicious data through the attacked Node A which causes data spoofing/data tampering. The ACC received erroneous data regarding the self-propelled vehicle’s position, leading to the generation of flawed control signals. Figure 5 represents the ordinary status of AV-CPS which has zero cyber-attack. Figure 6 depicts the scenario of the unusual condition wherein the attacker targets Node A. The raw dataset consists of one dimension and comprises 80,000 data points. The dataset is evenly balanced, with 40,000 data points labeled as normal and the remaining labeled as attack data. Basically, these four characteristics make up the dataset:
1. The current position of a self-propelled vehicle.
2. The present position of a front vehicle.
3. The speed at which the self-propelled vehicle is going at that particular time.
4. The rate at which the front vehicle is moving.

Implementation of AV-CPS (self-propelled vehicle).
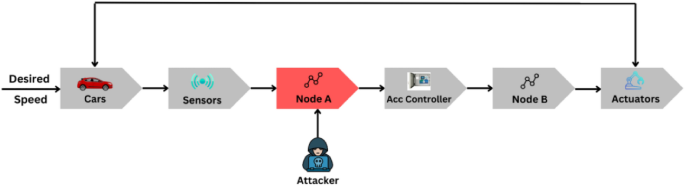
Cyber attack in AV-CPS.
Intelligent Intrusion Detection Systems
Intrusion Detection Systems are systems that identify unauthorized access by hackers and malicious actors. These systems are usually implemented in places where frequent monitoring for any suspicious access to any secure data to ensure data integrity and security 7 , 22 . It checks for any behavioral anomalies and notifies the user about the malicious activity if found. This intelligent system is very promising when it is almost impossible to monitor an important system all the time, especially in the cases of monitoring in distant lands 16 , 17 .
IIDS in Autonomous Vehicles
For self-using cars, there may be a want for coordinated Intrusion Detection Systems (IDS). These structures are incorporated into the automobile`s state-of-the-art structure to check and examine facts flows, perceive any uncommon structures or capability protection dangers and observe non-stop data and gadget mastering strategies to quick perceive unique functions in actual time, permitting quicker responses to cyber threats and unauthorized tries. The complexity and interconnectedness of superior automobile structures poses brilliant demanding situations for IDS in self-sustaining cars 5 .
The complicated provisioning of sensors, manage structures, and network affords a huge spectrum of assaults that may be abused via way of means of malicious actors. A key undertaking is securing controller region management (CAN) communication, a important meeting in cars that calls for strong protection publicity to keep away from vulnerabilities 12 .
The modern evolution of cyber threats poses a hazard to self-sustaining cars. These assaults goal flaws inside the gadget configuration, the communications network, or interference inside the outside drive, in all likelihood compromising its capability and protection data-pushed assaults, monitor quite a few demanding situations, and attackers manage facts to misdirect the gadget considering the calculations of self-sustaining cars, using to incorrect selections and sports.To keep away from getting self-sustaining cars need to be customer-centric facts safety comes first 13 . The IDS needs to paintings with actual-time vertical reaction to differentiate and save you from cyber threats, guard the application of the automobile and make certain occupant protection. Managing those demanding situations calls for a complete method that consists of a robust cyber security strategy, relentless checking out and refinement of protection platforms, collaboration with enterprise friends mounted cyber security suggestions for self-sustaining cars, and the continuing pursuit of systematic size to enhance IDS abilities towards superior cyber threat ranges can assist flow forward, that are intermediate operations that assure their protection.
As the technology develops rapidly, newer ML models are found and are used to improve the levels of Autonomous Vehicles. These new models ensure that IDS systems are more accurate to avoid any malicious attacks. Models like EfficientNet, DenseNet, InceptionV3, Inception ResNet V2, VGGNet, NasNet, and ResNet are some of the most powerful, current advanced ML models that are used in many autonomous vehicles.
ML with IIDS
Machine Learning and Intelligent Intrusion Detection are core building blocks of synthetic intelligence, both immediately concerned with data analysis, pattern reputation and selection making. ML is the sphere of look at granted for the development of structures that may analyze from statistics to discover samples and make predictions autonomously 31 , 32 . In contrast, IIDS is a complex record systems machine in which entities interact, even though forming complex relationships challenging to examine through traditional methods. The interplay between IIDS and ML algorithms enhances both my understanding and the functioning of such algorithms. In IIDS, Machine Mastery tech mainly performs well in data mining insights from the connectivity available in big datasets 34 . By applying to IIDS machine learning techniques like neural networks, decision trees or clustering that consist of graphs, relational databases and know-how graphs we can reveal hidden patterns, relationships or trends.
Integrating machine learning (ML) with intelligent and intrusion detection (IIDS) has several advantages like Analyzing structured data for complex relationships between entities. For example, nodes can be detected by using social network analysis based on ML. This can help you predict your network user’s actions based on their interactions within it. ML applied to genetics can find relations between organic objects through genetic traits 40 , 41 .
In addition to that, having partnership between ML and IIDS facilitates innovation in areas such as Natural language processing, Image recognition, Recommendation systems and Self-driving cars. Through these approach researchers working within these domains would use ML algorithms for processing his/her related data concerning IIDS helping him/her to enhance language models, increase accuracy while reflecting adjustments according to user preferences and improve the system’s decision making ability 42 .
Transfer Learning
Transfer learning in machine learning is also a technique that allows knowledge from one task to be used to achieve better results on another task. This entails reusing knowledge acquired from previous tasks so as to enhance efficiency and speed up the training of new tasks 14 . This comes in handy when there are insufficient labeled instances from target domain hence models can rely on their experience with other similar domains as expertise. Transfer learning involves several types such as instance-based transfer, parameter transfer, feature-representation transfer and relational-knowledge transfer, each one focusing on different aspects of transferring knowledge across domains. When there are minor dissimilarities between the two domains or limited labeled data available for the target domain, marginal distributions adaptation, learned structure transfer or weights reassignment is an effective way to optimize model performance through transfer learning. In neural network models that involve Transfer Learning, what occurs instead is using pertained representations of features in place of starting over again with a fresh model. By doing this, it is possible to solve problems associated with small datasets by learning from past experience and applying them in future related tasks through this approach 44 .
Experimentation and result discussion
The experiment was carried out with the help of MATLAB and Simulink. MATLAB is a powerful programming approach used by programmers for the analysis and design of products and systems that can take technology to the next level, while Simulink is a graphical programming platform that works on a MATLAB model for modeling and simulating. The AV animation demonstration was devised by MathWorks making use of MATLAB and Simulink. This study carried out the CAN component assembly utilizing the Simulink organize tool kit, later programmed in the AV rest using MATLAB to accomplish and examine the pre-trained CNNs looked at.
We developed a preparation test system by implementing a digit system using a Design preparing unit (GPU) to minimize the processing duration, and optimize the execution of the test. To fix the input of the pre-trained neural structures, we need to convert 1-D information to 2-D information model. Figure 7 represents the conversion of 1D image to 2D image. In the first phase, AV-CPS interests are developed. Step 2 records the responses to the points as previously identified as statistical 1-dimensional information in a chart. At that point, step 3 changes the information leading it from a 1D grid to a 2D structure. Step 4 includes storing the 2D presentation as an image. Each image has a measurement area of 4 × 81. Since we got four points, our computation is 4 × 81, and the animation runs 81 s each time. In the end, stage 5 collects and saves the resultant normality and abnormality images in separate folders.
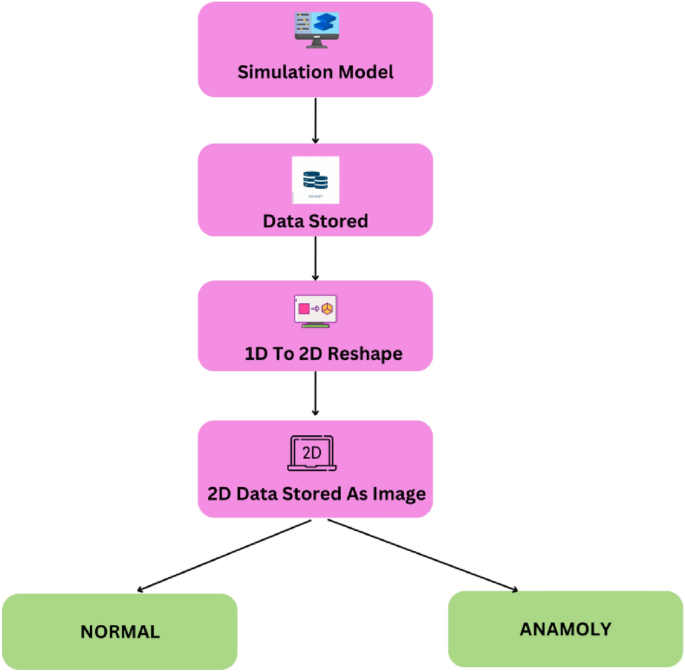
Steps involved converting 1-D image to 2-D image.
Algorithm 1 shows the algorithm that how the information in part 1 was converted into two parts. First, ‘image index’ will be initialized with 0. Then decide it can be reshaped or not. ’current_row’ and ‘end_row’ factors are utilized as counters since we propose to integrate all four data set lines. Therefore, ordinary information and attack information has been collected for use. The ‘normal’ catalog comprises images with specific information, and the ‘attack’ registry is designed to keep attack images. Where the circle begins with one and goes the length of the information source, whether specific or attack. So a few thousand copies are made of which halves of them were listed as ordinary and the rest as attack. Each image will be compressed in jpg format.
The following mentioned models are various current, powerful ML models used in Autonomous Vehicles along with their accuracies 6 . These have been billed as an integral part of sophisticated study paradigms in Intelligent Intrusion Detection in Autonomous Vehicles. Figure 8 illustrates the resizing of images for various models. EfficientNet, DenseNet, InceptionV3, InceptionResNet V2, VGG Net, NasNet, and ResNet 18 are published free of charge for their important parts in solving critical vehicle decision statistics 27 , 28 . Figure 9 represents the working model of intelligent intrusion detection system.
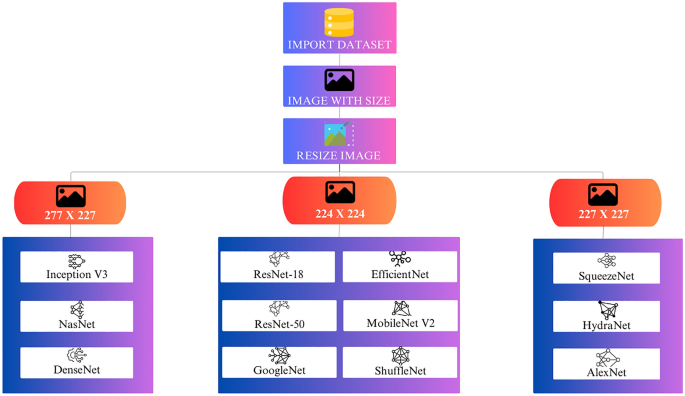
Resizing of images for various models.
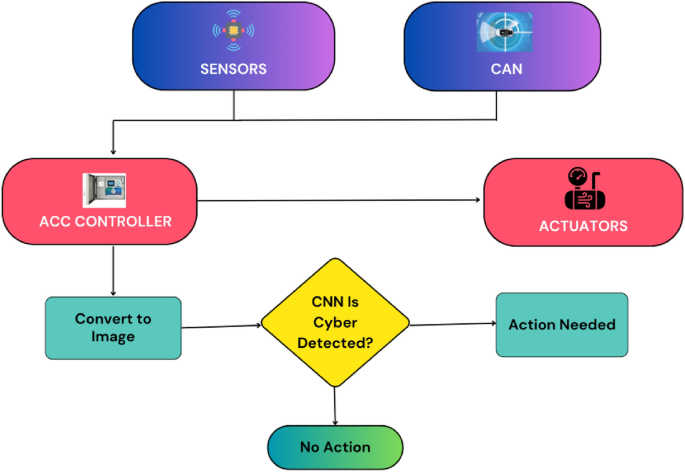
Working principle of IIDS.
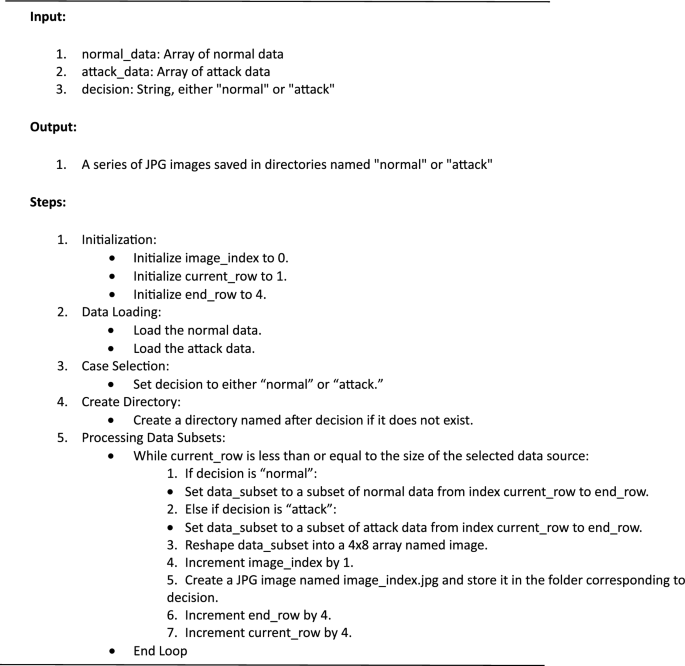
To convert 1D image to 2D image.
The experiment was first started with two ML models ResNet—18 and NasNet 55 . After training and testing, their accuracy values were not up to the mark as they gave accuracy values of 97.65% and 98.18% respectively. Due to its susceptibility to over fitting, ResNet–18 provided lower accuracy, while due to its potential computational complexity and resource requirements, which may hinder its efficiency in real-time intrusion detection tasks, especially in dynamic and complex environments, NasNet provided lower accuracy. Hence we went for other advanced models namely ShuffleNet, MobileNet V2, and HydraNet. HydraNet is a strong ML model used in Tesla’s present models like Tesla Model 3, Tesla Model S, etc. The aforementioned models provided a good accuracy of 99.30, 99.30, 99.33% respectively. Even though they are significantly higher than the previous models, they are still very far from reaching a 100% accuracy rate 29 , 37 .
The potential challenge in capturing complex and intricate patterns present in the data related to network intrusions in dynamic and evolving environments made ShuffleNet to provide lesser accuracy while the lightweight design of MobileNet V2 optimized for efficiency, may compromise its ability to capture and analyze complex and intricate patterns and HydraNet’s reliance on statistical trust-based methods and weak hidden Markov models made these models to provide lesser accuracy rate. This in turn made us move on with higher and more powerful models like GoogleNet, InceptionV3, DenseNet, and EfficientNet. Of those aforementioned ML models, GoogleNet is currently the strongest model with an astonishing accuracy rate of 99.47%. Even though it is almost close to being 100%, Inception V3’s 99.6%, DenseNet’s 99.8%, and EfficientNet’s excellent, mind-blowing 99.97% made it more clear that there exists many stronger models than present GoogleNet. For sure, we went for the best in the industry, EfficientNet. EfficientNet’s exceptional execution, with system accuracy of up to 99.97%, and DenseNet’s basic test accuracy of 99.8% highlight their plethora of basic techniques in fully classifying images and real-time data 38 .
Metrics like Precision, Recall, F1-Score, and Accuracy are calculated using the formulae,
•TP—True positive.
•TN—True negative.
•FP—False positive.
•FN—False negative.
Confusion matrix can be used to find out the above mentioned terms. TP stands for true positive that represents the number of normal images classified correctly. FP refers to false positives that refer to normal images classified incorrectly. FN stands for false negative indicating normal images misclassified as abnormal ones. Finally, TN is called true negatives meaning there are abnormal images recognized correctly. Precision is a measure of how well the classifier separates positives from negatives which can be calculated using Eq. ( 1 ). Precision values for various models are represented in Fig. 9 .Recall on the other hand can be determined by following Eq. ( 2 ). Recall values for several models are shown in Fig. 10 .The F1 score combines precision and recall as in Eq. ( 3 ), whereas accuracy classification was determined using Eq. ( 4 ). Figures 11 , 12 represent the F1 score and accuracy for various models accordingly. On comparison of various accuracy values provided by the aforementioned ML models, EfficientNet emerges as the best model with an incredible accuracy of up to 99.97%. Since the accuracy is almost close to 100%, this model is more promising in vehicles safety and protection against any malicious attacks. As shown in Fig. 13 , this research also proves that EfficientNet – B7 works better than GoogleNet (accuracy—99%), which is currently the most powerful and secure model. Figure 14 represents a comparative analysis of the proposed model, EfficientNet-B7, with existing machine learning models. Figure 15 provides the training and validation loss & training and validation accuracy of ResNet-18 model. Figure 16 represents the training and validation loss & training and validation accuracy of ResNet-50. The training and validation loss & training and validation accuracy of AlexNet is represented in Fig. 17 . Figure 18 provides the training and validation loss & training and validation accuracy of InceptionV3. The training and validation loss & training and validation accuracy of DenseNet is represented in Fig. 19 . Figure 20 represents the training and validation loss & training and validation accuracy of proposed system—EfficientNet. From the analysis of these graphs it is cleared that the proposed system has better accuracy when it is compared with the existing systems. The reason for the improvement is that the proposed system employs EfficientNet which provides the efficient classification thereby it improves learning in the network.
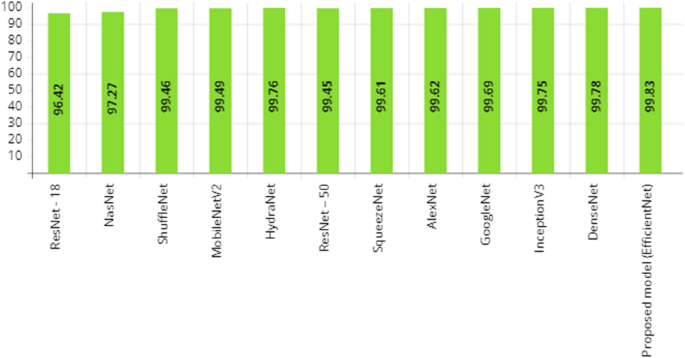
Precision analysis.
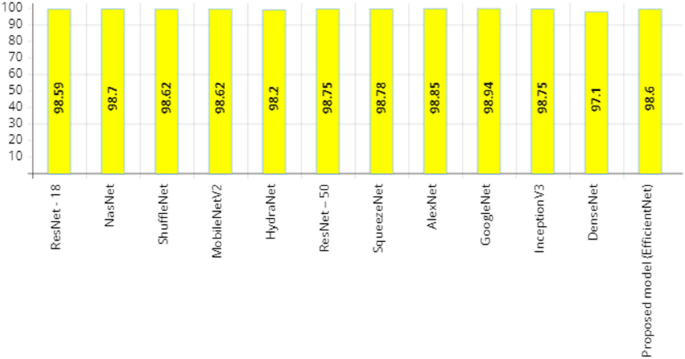
Recall analysis.
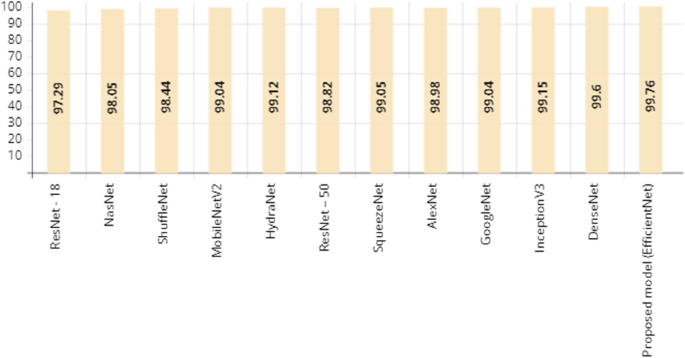
F1 Score analysis.
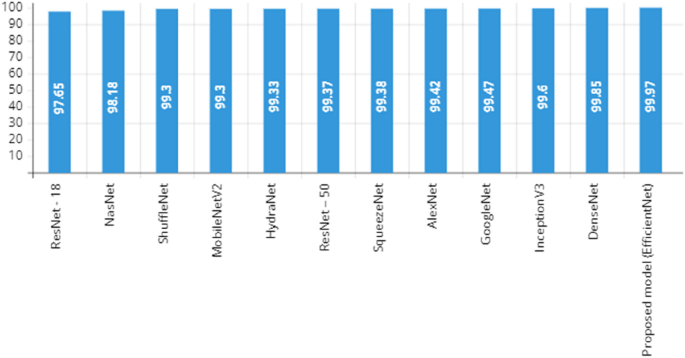
Accuracy analysis.

Comparison with existing ML models.
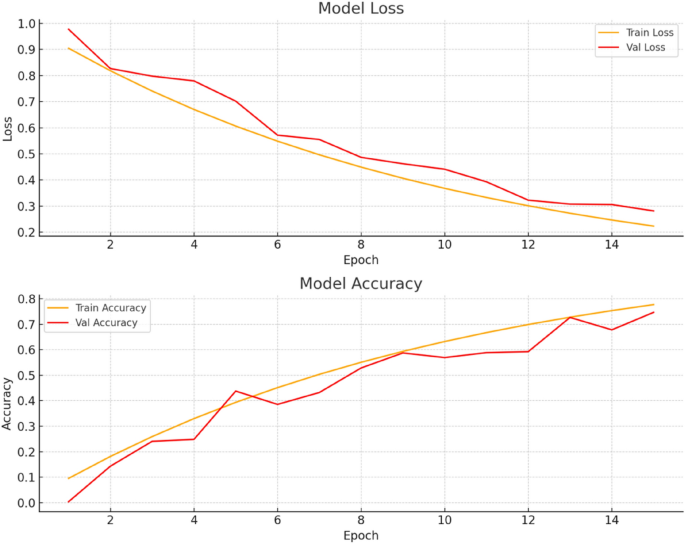
Training and validation loss & Training and validation accuracy of ResNet-18.
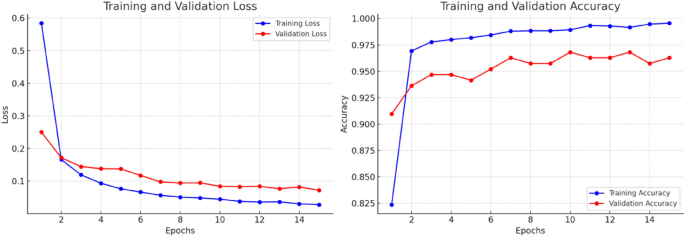
Training and validation loss & Training and validation accuracy of ResNet-50.
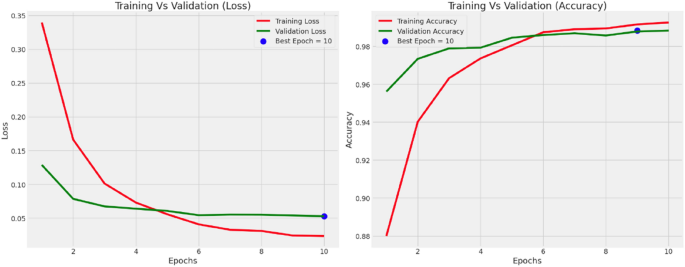
Training and validation loss & Training and validation accuracy of AlexNet.
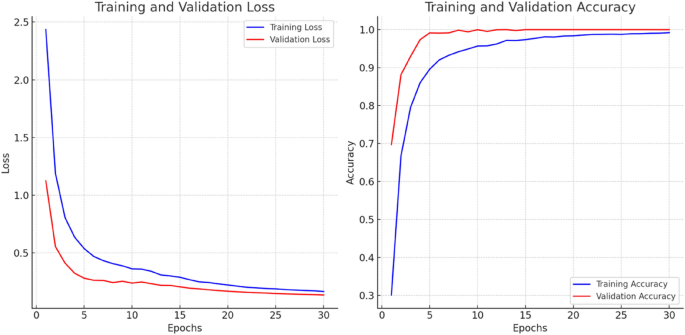
Training and validation loss & Training and validation accuracy of InceptionV3.
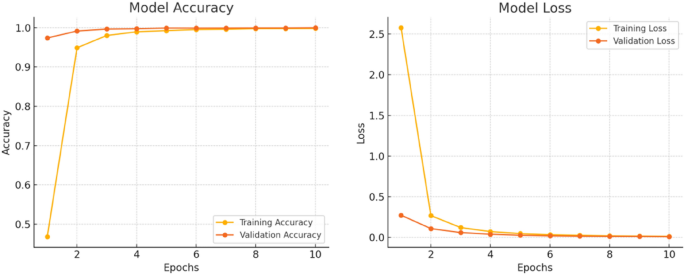
Training and validation loss & Training and validation accuracy of DenseNet.
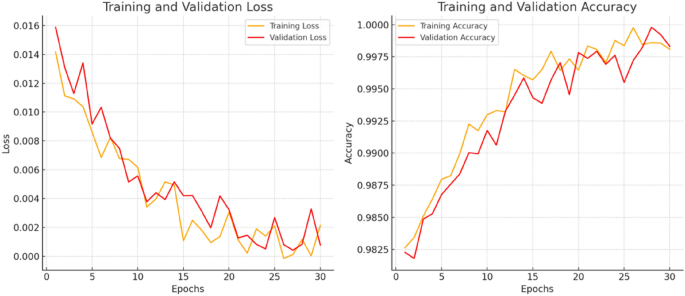
Training and validation loss & Training and validation accuracy of EfficientNet (Proposed Model).
Looking at Table 1 , we can see the calculation of the accuracy of pre-trained models that focuses on four metrics with regard to accuracy analysis. We compared the performance outcomes of this research with recent IDS methods employed in AV systems to validate and verify our work. The cost analysis of all models is provided in Table 1 . Due to the complexity of the proposed EfficientNet model’s architecture, it requires 12 GPU hours for computation. Despite its complexity, this model achieves higher accuracy compared to the other models, while also having a lower computation cost than the DenseNet model. Table 2 gives details about the performance of each method.
Smart cities have integrated advanced technology and data analytics to maximize urban operations, promote sustainability, and improve people’s lives. In this ecosystem, self-driving cars play a critical role because they are effective, secure, and environmentally friendly means of transportation. The development of 6G technology highlights their potential to transform autonomous vehicle systems. The integration of an intelligent dynamic threat detection system within 6G-enabled autonomous vehicle networks represents a critical advancement in securing communication in cyber-physical systems. The proposed system, which leverages advanced machine learning techniques and real-time data processing, offers a robust solution to detect and neutralize cyber threats that could compromise the safety and functionality of autonomous vehicles. After analysis and comparisons it was found that EfficientNet stands out as the model providing improved performance and reliability necessary for the safety focused tasks of autonomous vehicles.
Thus this research proves that EfficientNet emerges as the best model with an incredible accuracy of up to 99.97%.By ensuring secure communication channels and proactively addressing potential vulnerabilities, this approach not only enhances the reliability of autonomous vehicles but also sets a new standard for security in next-generation vehicular networks.
Future work
The proposed work can be further improved by employing federated learning along with smart grids for efficient identification of intrusion in CPS and 6G cellular networks. Moreover, the scalability of the proposed work can be improved by using heterogeneous devices which provides seamless connectivity of all the devices that are connected in 6G network.
Data availability
The data that supports the findings of this research work are available from the corresponding author upon reasonable request.
Abbreviations
Cyber-physical system
Autonomous vehicles
Convolutional neural networks
Controller area network
Intelligent intrusion detection system
Transfer learning
Intrusion detection system
Intelligent transportation systems
Internet of everything
Internet of things
Space air ground underwater network
Artificial intelligence
Machine learning
Vehicular ad-hoc network
Denial of services
Multilayer perceptron
False Data insertion
Cyber attack dataset collection
Long-term short term memory
Intrusion detection mechanism
Adaptive cruise control
Al-Haija QA, Smadi MA, Zein-Sabatto S (2020) Multi-class weather classification using resnet-18 CNN for autonomous IOT and CPS applications. International Conference Computational Science and Computational Intelligence (CSCI) 2020:1586–1591. https:// doi.org/ https://doi.org/10.1109/CSCI51800.2020.00293 .
AlOmari AA, Smadi AA, Johnson BK, Feilat EA. Combined approach of LST-ANN for discrimination between transformer inrush current and internal fault. 2020 52nd North American Power Symposium (NAPS), Tempe, p 1–6. https://doi.org/10.1109/NAPS50074.2021.9449768 .
International Conference on Intelligent Computing and Control Systems (ICICCS) , Madurai, India, 2023, pp. 1252-1257, https://doi.org/10.1109/ICICCS56967.2023.10142687 .
Bizon, N., Dascalescu, L., Tabatabaei, M. & Naser.,. Autonomous Vehicles: Intelligent Transport Systems and Smart Technologies (Nova Science Publishers Inc, Series, 2014).
Google Scholar
Shi, Y., Lv, L., Yu, H., Yu, L. & Zhang, Z. A center-rule-based neighborhood search algorithm for roadside units deployment in emergency scenarios. Mathematics 8 , 1734. https://doi.org/10.3390/math8101734 (2020).
Article Google Scholar
Natheeswari, N., Sivaranjani, P., Vijay, K. & Vijayakumar, R. Efficient data migration method in distributed systems environment. Adv. Parallel Comput. 37 , 533–537 (2020).
Ali Alheeti, K. M. & McDonald-Maier, K. Intelligent intrusion detection in external communication systems for autonomous vehicles. Syst. Sci. Control Eng. 6 (1), 48–56 (2018).
Mahmoud, O. et al. A feature selection method for classifcation within functional genomics experiments based on the proportional overlapping score. BMC Bioinform. 15 , 274. https://doi.org/10.1186/1471-2105-15-274 (2014).
Article CAS Google Scholar
F. A. Fauzi, E. Mulyana, R. Mardiati, and A. Eko Setiawan, “Fuzzy Logic Control for Avoiding Static Obstacle in Autonomous Vehicle Robot,” 2021 7th International Conference on Wireless and Telematics (ICWT) , 2021. 1–5, https://doi.org/10.1109/ICWT52862.2021.9678436 .
Alsulami, A. A., Abu Al-Haija, Q., Alqahtani, A. & Alsini, R. Symmetrical simulation scheme for anomaly detection in autonomous vehicles based on LSTM model. Symmetry 14 , 1450. https://doi.org/10.3390/sym14071450 (2022).
Article ADS Google Scholar
Philipsen SG, Andersen B, Singh B (2021) Threats and Attacks to Modern Vehicles. In: IEEE International Conference Internet Things and Intelligent Systems (IoTaIS) 2021:22–27. https://doi.org/10.1109/IoTaIS53735.2021.9628576 .
Negi N, Jelassi O, Clemencon S, Fischmeister S (2019) A LSTM approach to detection of autonomous vehicle hijacking. In: Proceedings of the 5th International Conference on Vehicle Technology and Intelligent Transport Systems - VEHITS . SciTePress, p 475–482. https://doi.org/10.5220/00077 26004750482.
D. Kosmanos et al., “Intrusion Detection System for Platooning Connected Autonomous Vehicles,” 2019 4th South-East Europe Design Automation, Computer Engineering, Computer Networks and Social Media Conference (SEEDA-CECNSM) , 2019. 1–9, https://doi.org/10.1109/SEEDA-CECNSM.2019.8908528 .
Shanthalakshmi, M., Jananee, V., Perumal, P. N. & Jayakar, S. M. September). Identification of casting product surface quality using alexnet and lenet CNN Models. J. Phys. Conf. Ser. https://doi.org/10.1088/1742-6596/2335/1/012031 (2022).
Yang, L., Moubayed, A. & Shami, A. MTH-IDS: a multitiered hybrid intrusion detection system for the internet of vehicles. IEEE Int. Things J. 9 (1), 616–632. https://doi.org/10.1109/JIOT.2021.3084796 (2022).
Omar Minawi, Jason Whelan, Abdulaziz Almehmadi, and Khalil ElKhatib. 2020. Machine Learning-Based Intrusion Detection System for Controller Area Networks. In Proceedings of the 10th ACM Symposium on Design and Analysis of Intelligent Vehicular Networks and Applications (DIVANet ’20) . Association for Computing Machinery, New York, NY, USA, 41–47. https://doi.org/10.1145/3416014.3424581
Alfardus A, Rawat DB, “Intrusion detection system for can bus in vehicle network based on machine learning algorithms,” 2021 IEEE 12th Annual Ubiquitous Computing, Electronics & Mobile Communication Conference (UEMCON), 2021. 0944-0949, 10.1109/ UEMCON53757.2021.9666745
Yang L, Shami A (2022) A transfer learning and optimized CNN based intrusion detection system for internet of vehicles. ICC 2022 - IEEE International Conference on Communications . Seoul, Korea, p 2774–2779. https://doi.org/10.1109/ICC45855.2022.9838780
Liu, Y. et al. Interaction-enhanced and time-aware graph convolutional network for successive point-of-interest recommendation in traveling enterprises. IEEE Trans. Ind. Inf. 19 (1), 635–643. https://doi.org/10.1109/TII.2022.3200067 (2023).
Anbalagan, S., Raja, G., Gurumoorthy, S., Suresh, R. D. & Dev, K. IIDS: Intelligent intrusion detection system for sustainable development in autonomous vehicles. IEEE Trans. Intell. Transp. Syst. https://doi.org/10.1109/TITS.2023.3271768 (2023).
CPSRC - UCSC. (2018, May 23). What are cyber physical systems? [Video] . YouTube. https://www.youtube.com/watch?v=C6q88zJwq2g
Simplilearn. (2022b, May 27). What is Intrusion Detection System? | Intrusion Detection System (IDS) | Cyber Security |Simplilearn [Video]. YouTube. https://www.youtube.com/watch?v=dfVAi87BSEs
India Science. (2023, April 8). Cyber Physical system [Video]. YouTube. https://www.youtube.com/watch?v=VhtFv6TtWBo
Journal, I. (2021). Intrusion Detection System: an approach to Autonomous vehicles . www.academia.edu . https://www.academia.edu/64696486/Intrusion_Detection_System_An_Approach_to_Autonomous_Vehicles
Alheeti, K. M. A. & McDonald-Maier, K. Intelligent intrusion detection in external communication systems for autonomous vehicles. Syst. Sci. Control Eng. 6 (1), 48–56. https://doi.org/10.1080/21642583.2018.1440260 (2018).
Aloqaily, M., Otoum, S., Ridhawi, I. A. & Jararweh, Y. An intrusion detection system for connected vehicles in smart cities. Ad Hoc Netw. 90 , 101842. https://doi.org/10.1016/j.adhoc.2019.02.001 (2019).
Birkinshaw, C., Rouka, E. & Vassilakis, V. G. Implementing an intrusion detection and prevention system using software-defined networking: Defending against port-scanning and denial-of-service attacks. J. Netw. Comput. Appl. 136 , 71–85. https://doi.org/10.1016/j.jnca.2019.03.005 (2019).
Vijayakumar, R., Vijay, K., Sivaranjani, P. & Priya, V. Detection of network attacks based on multiprocessing and trace back methods. Adv. Parallel Comput. 38 , 608–613 (2021).
Mathew, Dennise, G. Kirubasri, K. Vijay, I. Eugene Berna, and K. R. Sowmia. “System for Detecting Intrusions using Raspberry PI.” In: 2023 International Conference on Computer Communication and Informatics (ICCCI), pp. 1–6. IEEE, 2023.
Shanthalakshmi, M., Gogoi, D., Chhabra, M., Rana, S. & Thakur, S. “A distributed malicious attack detection and prevention approach using honeypots in ad-hoc network “was published in SSRG International Journal of Computer Science and Engineering – (2’ICEIS-2017) –Special Issue–2017.
Sadaf, M. et al. A novel framework for detection and prevention of denial of service attacks on autonomous vehicles using fuzzy logic. Veh. Commun. 46 , 100741. https://doi.org/10.1016/j.vehcom.2024.100741 (2024).
Dr, A., George, S., Dr, T. & Baskar, & Dr.P. Balaji Srikaanth.,. Securing the self-driving future: Cybersecurity challenges and solutions for autonomous vehicles. Partn. Univers. Innov. Res. Publ. (PUIRP) 01 (02), 137–156. https://doi.org/10.5281/zenodo.10246882 (2023).
Dr, A. S., George, A. S., George, H. & Baskar, T. Wi-Fi 7: The next frontier in wireless connectivity. Partn. Univers. Int. Innov. J. https://doi.org/10.5281/zenodo.8266217 (2023).
Vinayagam, J., Murugan, S., Mishra, S., Samuel, L. J., Prabakar, R., & Shalini, M. (2023, August). An approach for devising stenography application using cross modal attention. In AIP Conference Proceedings (Vol. 2790, No. 1). AIP Publishing.
Cao Y, Xiao C, Cyr B, Zhou Y, Park W, Rampazzi S et al (2019) Adversarial sensor attack on LiDAR-based perception in autonomous driving. In: Proceedings of the 2019 ACM SIGSAC Conference on Computer and Communications Security (CCS ‘19) . Association for Computing Machinery, New York, p 2267–2281. https://doi.org/10.1145/3319535.3339815 .
S. K. D et al., “Implementation of Smart Vehicle Accident Detection using Raspberry PI in Smart Cities,” 2022 4th International Conference on Inventive Research in Computing Applications (ICIRCA) , Coimbatore, India, 2022, pp. 1611-1614, https://doi.org/10.1109/ICIRCA54612.2022.998576
Vinayagam, J., Murugan, S., Jesu, S. G., Vaidhya, G. K., Narayanan, N. S., & Thayil, N. B. (2023, August). Detection of diabetic retinopathy using AlexNet and lenet CNN models. In AIP Conference Proceedings (Vol. 2790, No. 1). AIP Publishing.
Cao Y, Xiao C, Cyr B, Zhou Y, Park W, Rampazzi S et al (2019) Adversarial sensor attack on LiDAR-based perception in autonomous driving. In: Proceedings of the 2019 ACM SIGSAC Conference on Computer and Communications Security (CCS ‘19 ). Association for Computing Machinery, New York, p 2267–2281. https://doi.org/10.1145/3319535.3339815 .
Zhang, L. & Ma, D. A hybrid approach toward efficient and accurate intrusion detection for in-vehicle networks. IEEE Access 10 , 10852–10866. https://doi.org/10.1109/ACCESS.2022.3145007 (2022).
Intrusion detection system using machine learning for vehicular ad hoc networks based on TON-IoT dataset. (2021). IEEE Journals & Magazine | IEEE Xplore. https://ieeexplore.ieee.org/abstract/document/9576115/
Zhu, Z., Hu, Z., Dai, W., Chen, H. & Lv, Z. Deep learning for autonomous vehicle and pedestrian interaction safety. Saf. Sci. 145 , 105479. https://doi.org/10.1016/j.ssci.2021.105479 (2022).
Song, H. M., Woo, J. & Kim, H. K. In-vehicle network intrusion detection using deep convolutional neural network. Veh. Commun. 21 , 100198. https://doi.org/10.1016/j.vehcom.2019.100198 (2020).
Koonce, B. Convolutional neural networks with swift for tensorflow. In Apress eBooks (ed. Koonce, B.) (Springer, 2021). https://doi.org/10.1007/978-1-4842-6168-2 .
Chapter Google Scholar
AlEisa, H. N. et al . Transforming transportation: Safe and secure vehicular communication and anomaly detection with intelligent cyber–physical system and deep learning. IEEE Trans. Consum. Electron. 70 (1), 1736–1746. https://doi.org/10.1109/TCE.2023.3325827 (2024).
Mazhar, T. et al. Analysis of cyber security attacks and its solutions for the smart grid using machine learning and blockchain methods. Future Internet 15 (2), 83 (2023).
Ghadi, Y. Y. et al. Integration of federated learning with IoT for smart cities applications, challenges, and solutions. PeerJ Comput. Sci. 9 , e1657 (2023).
Article PubMed PubMed Central Google Scholar
Shah, S. F. A. et al. Applications, challenges, and solutions of unmanned aerial vehicles in smart city using blockchain. PeerJ Comput. Sci. 10 , e1776 (2024).
Ghadi, Y. Y. et al. Machine learning solution for the security of wireless sensor network. IEEE Access https://doi.org/10.1109/ACCESS.2024.3355312 (2024).
Khan, I. A. et al. A new explainable deep learning framework for cyber threat discovery in industrial IoT networks. IEEE Internet Things J. 9 (13), 11604–11613 (2021).
Khan, I. A. et al. An enhanced multi-stage deep learning framework for detecting malicious activities from autonomous vehicles. IEEE Trans. Intell. Transp. Syst. 23 (12), 25469–25478 (2021).
Khan, I. A. et al. A privacy-conserving framework based intrusion detection method for detecting and recognizing malicious behaviours in cyber-physical power networks. Appl. Intell. https://doi.org/10.1007/s10489-021-02222-8 (2021).
Liu, Z. et al . Establishing trustworthy and privacy-preserving SAGIVNs in 6G: architectures, requirements, and solutions. IEEE Netw . 38 (2), 141–147. https://doi.org/10.1109/MNET.2023.3335974 (2023).
J. Manikandan, S. R. Devakadacham, M. Shanthalakshmi, Y. Arockia Raj and K. Vijay, (2023)”An Efficient Technique for the Better Recognition of Oral Cancer using Support Vector Machine,” 2023 7th International Conference on Intelligent Computing and Control Systems (ICICCS), Madurai, India, 2023, pp. 1252-1257.
Anandhi, S., Devakadacham, S.R., Manikandan, J., Shanthalakshmi, M.(2024), Enhancing Lung Disease Diagnosis through Meta Learning: A Framework Utilizing Few-Shot Learning Techniques, Proceedings - 3rd International Conference on Advances in Computing, Communication and Applied Informatics, ACCAI 2024.
Keerthana, S., Deepika, N., Pooja, E., Shanthalakshmi, M., Khanaghavalle, G.R.(2024), An effective approach for detecting deepfake videos using Long Short-Term Memory and ResNet, 2024 International Conference on Communication, Computing and Internet of Things, IC3IoT 2024 - Proceedings, 2024.
Liu, Z. et al. PPRU: A privacy-preserving reputation updating scheme for cloud-assisted vehicular networks. IEEE Trans. Veh. Technol. https://doi.org/10.1109/TVT.2023.3340723 (2023).
Guo, J. et al. TROVE: A context-awareness trust model for VANETs using reinforcement learning. IEEE Internet Things J. 7 (7), 6647–6662 (2020).
Download references
Author information
Authors and affiliations.
Department of Computing Technologies, SRM Institute of Science and Technology, Kattankulathur, Chennai, 603203, Tamil Nadu, India
Shanthalakshmi M & Ponmagal R S
You can also search for this author in PubMed Google Scholar
Contributions
M.S confirms sole responsibility for the following: study conception and design, data collection, analysis and interpretation of results, and manuscript preparation. R.S.P reviewed the results and approved the final version of the manuscript.
Corresponding authors
Correspondence to Shanthalakshmi M or Ponmagal R S .
Ethics declarations
Competing interests.
The authors declare no competing interests.
Additional information
Publisher's note.
Springer Nature remains neutral with regard to jurisdictional claims in published maps and institutional affiliations.
Rights and permissions
Open Access This article is licensed under a Creative Commons Attribution-NonCommercial-NoDerivatives 4.0 International License, which permits any non-commercial use, sharing, distribution and reproduction in any medium or format, as long as you give appropriate credit to the original author(s) and the source, provide a link to the Creative Commons licence, and indicate if you modified the licensed material. You do not have permission under this licence to share adapted material derived from this article or parts of it. The images or other third party material in this article are included in the article’s Creative Commons licence, unless indicated otherwise in a credit line to the material. If material is not included in the article’s Creative Commons licence and your intended use is not permitted by statutory regulation or exceeds the permitted use, you will need to obtain permission directly from the copyright holder. To view a copy of this licence, visit http://creativecommons.org/licenses/by-nc-nd/4.0/ .
Reprints and permissions
About this article
Cite this article.
M, S., R S, P. An intelligent dynamic cyber physical system threat detection system for ensuring secured communication in 6G autonomous vehicle networks. Sci Rep 14 , 20795 (2024). https://doi.org/10.1038/s41598-024-70835-3
Download citation
Received : 09 June 2024
Accepted : 21 August 2024
Published : 05 September 2024
DOI : https://doi.org/10.1038/s41598-024-70835-3
Share this article
Anyone you share the following link with will be able to read this content:
Sorry, a shareable link is not currently available for this article.
Provided by the Springer Nature SharedIt content-sharing initiative
- Transfer learning (TL)
- Intrusion detection system (IDS)
- Autonomous Vehicles (AV)
- Cyber-physical system (CPS)
- Controller area network (CAN)
By submitting a comment you agree to abide by our Terms and Community Guidelines . If you find something abusive or that does not comply with our terms or guidelines please flag it as inappropriate.
Quick links
- Explore articles by subject
- Guide to authors
- Editorial policies
Sign up for the Nature Briefing: AI and Robotics newsletter — what matters in AI and robotics research, free to your inbox weekly.

Cyber attacks: A literature Survey

- Michael Okpara University of Agriculture, Umudike

- Spiritan University Nneochi
Discover the world's research
- 25+ million members
- 160+ million publication pages
- 2.3+ billion citations

- Karan Singh
- Surbhi Singh
- Mehar Vohra
- Ravi Shankar Jha
- Harmandeep Singh Brar

- Jong Hyuk Park
- Comput Law Secur Rep

- Evelyne Studer
- COMPUT SECUR
- Rossouw von Solms

- Syed Taha Ali
- Vijay Sivaraman
- Adam Radford

- Int Stud Rev

- Recruit researchers
- Join for free
- Login Email Tip: Most researchers use their institutional email address as their ResearchGate login Password Forgot password? Keep me logged in Log in or Continue with Google Welcome back! Please log in. Email · Hint Tip: Most researchers use their institutional email address as their ResearchGate login Password Forgot password? Keep me logged in Log in or Continue with Google No account? Sign up

IMAGES
COMMENTS
In addition, five scenarios can be considered for cyber warfare: (1) Government-sponsored cyber espionage to gather information to plan future cyber-attacks, (2) a cyber-attack aimed at laying the groundwork for any unrest and popular uprising, (3) Cyber-attack aimed at disabling equipment and facilitating physical aggression, (4) Cyber-attack as a complement to physical aggression, and (5 ...
Abstract. Cyber security has become a very critical concern that needs the attention of researchers, academicians, and organizations to confidentially ensure the protection and security of information systems. Due to the increasing demand for digitalization, every individual and organization faces continually shifting cyber threats.
Abstract. There has been a tremendous increase in research in the area of cyber security to support cyber applications and to avoid key security threats faced by these applications. The goal of ...
Firstly, over the span of a decade, from 2011 to 2020, 50 significant cyber incidents have served as pivotal studies in the realm of cyber threats and security. Examining these case studies ...
Recent research has introduced effective Machine Learning (ML) models for cyber-attack detection, promising to automate the task of detecting, tracking and blocking malware and intruders.
Internet usage has grown exponentially, with individuals and companies performing multiple daily transactions in cyberspace rather than in the real world. The coronavirus (COVID-19) pandemic has accelerated this process. As a result of the widespread usage of the digital environment, traditional crimes have also shifted to the digital space. Emerging technologies such as cloud computing, the ...
Cybercrime is estimated to have cost the global economy just under USD 1 trillion in 2020, indicating an increase of more than 50% since 2018. With the average cyber insurance claim rising from USD 145,000 in 2019 to USD 359,000 in 2020, there is a growing necessity for better cyber information sources, standardised databases, mandatory reporting and public awareness. This research analyses ...
The article is a full research paper (i.e., not a presentation or supplement to a poster). ... These five cybersecurity functions cover the use of AI tasks from the prevention of the security attack to the more complex mechanism of actively looking for new threats and counterattack. The functions cope with different aspects of the cybersecurity ...
ABSTRACT. Cyber attacks are increasing rapidly due to advanced digital technologies used by hackers. In addition, cybercriminals are conducting cyber attacks, making cyber security a rapidly growing field. Although machine learning techniques worked well in solving large-scale cybersecurity problems, an emerging concept of deep learning (DL ...
The consequences of these emerging AI-driven attack techniques could be life-threatening and highly destructive. Hence, this study investigates the emerging threat of AI-driven attacks and reviews the negative impacts of this sophisticated cyber weaponry in cyberspace. The paper is divided into five parts.
Civilians are notoriously weak at accurately assessing security threats—a fact that is amplified in the cyber realm due to low cybersecurity knowledge, general cognitive biases in calculating risk, and the distortion of cyber risks by the media, which focuses predominantly on spectacular yet low-likelihood attacks . Perceived risk is partly ...
There has been a tremendous increase in research in the area of cyber security to support cyber applications and to avoid key security threats faced by these applications. The goal of this study is to identify and analyze the common cyber security vulnerabilities. To achieve this goal, a systematic mapping study was conducted, and in total, 78 primary studies were identified and analyzed ...
Cyber security is a set of technologies, processes, and practices aimed at preventing attacks, damage, and illegal access to networks, computers, programmes, and data. The primary goal of this ...
Cybersecurity is a significant concern for businesses worldwide, as cybercriminals target business data and system resources. Cyber threat intelligence (CTI) enhances organizational cybersecurity resilience by obtaining, processing, evaluating, and disseminating information about potential risks and opportunities inside the cyber domain. This research investigates how companies can employ CTI ...
The paper first explains what cyber space and cyber security is. Then the costs and impact of cyber security are discussed. The causes of security vulnerabilities in an organization and the challenging factors of protecting an organization from cybercrimes are discussed in brief. ... Then a few common cyber-attacks and the ways to protect from ...
However, info-security professionals reported a higher frequency of all types of social engineering attacks year-on-year according to a report presented by Proofpoint. Spear phishing increased to 64% in 2018 from 53% in 2017, Vishing and/or SMishing increased to 49% from 45%, and USB attacks increased to 4% from 3%.
This paper focuses on comprehensive analysis of various cyber-attacks. The analysis of cyber-attack is done using kali Linux. Many tools that are present in the kali Linux are being explored in this paper. Published in: 2021 IEEE Mysore Sub Section International Conference (MysuruCon) Article #: Date of Conference: 24-25 October 2021.
The research was based on attacks identified and traced among the last three years. ... Procedia Economics and Finance 28 ( 2015 ) 24 â€" 31 Fig. 1. Cyber-attacks per industry sector 5. Security countermeasures 5.1. ... cyber-crime and regulatory decisions and facts meant to support the cyber-security. Acknowledgements This paper was co ...
Cybersecurity attacks are exponentially increasing, making existing detection mechanisms insufficient and enhancing the necessity to design more relevant prediction models and approaches.
The researchers analysed 965 cybersecurity research papers published between 2012 and 2016. They created a taxonomy of the types of data that are created and shared and then analysed the data collected via datasets. ... Ashtiani M, Azgomi MA. A distributed simulation framework for modeling cyber attacks and the evaluation of security measures ...
So we present a novel approach to mitigating these security risks by leveraging pre-trained Convolutional Neural Network (CNN) models for dynamic cyber-attack detection within the cyber-physical ...
Cyber-attacks - trends, patterns and security countermeasures. Andreea Bendovschi. doi: 10.1016/S2212-5671 (15)01077-1. Abstract. Technology is ra pidly evolving in a world driven by social ...
This paper mainly focuses on challenges faced by cyber security on the latest technologies .It also focuses on latest about the cyber security techniques, ethics and the trends changing the face of cyber security. Keywords: cyber security, cyber crime, cyber ethics, social media, cloud computing, android apps. 1. INTRODUCTION.
Abstract. Cyber attacks refer to those attacks launched on. unsuspecting online users either using a computer as the. object of the crime (hacking, phishing, spamming etc.), or. as a tool to ...