Qualitative Research: Characteristics, Design, Methods & Examples
Lauren McCall
MSc Health Psychology Graduate
MSc, Health Psychology, University of Nottingham
Lauren obtained an MSc in Health Psychology from The University of Nottingham with a distinction classification.
Learn about our Editorial Process
Saul McLeod, PhD
Editor-in-Chief for Simply Psychology
BSc (Hons) Psychology, MRes, PhD, University of Manchester
Saul McLeod, PhD., is a qualified psychology teacher with over 18 years of experience in further and higher education. He has been published in peer-reviewed journals, including the Journal of Clinical Psychology.
Olivia Guy-Evans, MSc
Associate Editor for Simply Psychology
BSc (Hons) Psychology, MSc Psychology of Education
Olivia Guy-Evans is a writer and associate editor for Simply Psychology. She has previously worked in healthcare and educational sectors.
On This Page:
Qualitative research is a type of research methodology that focuses on gathering and analyzing non-numerical data to gain a deeper understanding of human behavior, experiences, and perspectives.
It aims to explore the “why” and “how” of a phenomenon rather than the “what,” “where,” and “when” typically addressed by quantitative research.
Unlike quantitative research, which focuses on gathering and analyzing numerical data for statistical analysis, qualitative research involves researchers interpreting data to identify themes, patterns, and meanings.

Qualitative research can be used to:
- Gain deep contextual understandings of the subjective social reality of individuals
- To answer questions about experience and meaning from the participant’s perspective
- To design hypotheses, theory must be researched using qualitative methods to determine what is important before research can begin.
Examples of qualitative research questions include:
- How does stress influence young adults’ behavior?
- What factors influence students’ school attendance rates in developed countries?
- How do adults interpret binge drinking in the UK?
- What are the psychological impacts of cervical cancer screening in women?
- How can mental health lessons be integrated into the school curriculum?
Characteristics
Naturalistic setting.
Individuals are studied in their natural setting to gain a deeper understanding of how people experience the world. This enables the researcher to understand a phenomenon close to how participants experience it.
Naturalistic settings provide valuable contextual information to help researchers better understand and interpret the data they collect.
The environment, social interactions, and cultural factors can all influence behavior and experiences, and these elements are more easily observed in real-world settings.
Reality is socially constructed
Qualitative research aims to understand how participants make meaning of their experiences – individually or in social contexts. It assumes there is no objective reality and that the social world is interpreted (Yilmaz, 2013).
The primacy of subject matter
The primary aim of qualitative research is to understand the perspectives, experiences, and beliefs of individuals who have experienced the phenomenon selected for research rather than the average experiences of groups of people (Minichiello, 1990).
An in-depth understanding is attained since qualitative techniques allow participants to freely disclose their experiences, thoughts, and feelings without constraint (Tenny et al., 2022).
Variables are complex, interwoven, and difficult to measure
Factors such as experiences, behaviors, and attitudes are complex and interwoven, so they cannot be reduced to isolated variables , making them difficult to measure quantitatively.
However, a qualitative approach enables participants to describe what, why, or how they were thinking/ feeling during a phenomenon being studied (Yilmaz, 2013).
Emic (insider’s point of view)
The phenomenon being studied is centered on the participants’ point of view (Minichiello, 1990).
Emic is used to describe how participants interact, communicate, and behave in the research setting (Scarduzio, 2017).
Interpretive analysis
In qualitative research, interpretive analysis is crucial in making sense of the collected data.
This process involves examining the raw data, such as interview transcripts, field notes, or documents, and identifying the underlying themes, patterns, and meanings that emerge from the participants’ experiences and perspectives.
Collecting Qualitative Data
There are four main research design methods used to collect qualitative data: observations, interviews, focus groups, and ethnography.
Observations
This method involves watching and recording phenomena as they occur in nature. Observation can be divided into two types: participant and non-participant observation.
In participant observation, the researcher actively participates in the situation/events being observed.
In non-participant observation, the researcher is not an active part of the observation and tries not to influence the behaviors they are observing (Busetto et al., 2020).
Observations can be covert (participants are unaware that a researcher is observing them) or overt (participants are aware of the researcher’s presence and know they are being observed).
However, awareness of an observer’s presence may influence participants’ behavior.
Interviews give researchers a window into the world of a participant by seeking their account of an event, situation, or phenomenon. They are usually conducted on a one-to-one basis and can be distinguished according to the level at which they are structured (Punch, 2013).
Structured interviews involve predetermined questions and sequences to ensure replicability and comparability. However, they are unable to explore emerging issues.
Informal interviews consist of spontaneous, casual conversations which are closer to the truth of a phenomenon. However, information is gathered using quick notes made by the researcher and is therefore subject to recall bias.
Semi-structured interviews have a flexible structure, phrasing, and placement so emerging issues can be explored (Denny & Weckesser, 2022).
The use of probing questions and clarification can lead to a detailed understanding, but semi-structured interviews can be time-consuming and subject to interviewer bias.
Focus groups
Similar to interviews, focus groups elicit a rich and detailed account of an experience. However, focus groups are more dynamic since participants with shared characteristics construct this account together (Denny & Weckesser, 2022).
A shared narrative is built between participants to capture a group experience shaped by a shared context.
The researcher takes on the role of a moderator, who will establish ground rules and guide the discussion by following a topic guide to focus the group discussions.
Typically, focus groups have 4-10 participants as a discussion can be difficult to facilitate with more than this, and this number allows everyone the time to speak.
Ethnography
Ethnography is a methodology used to study a group of people’s behaviors and social interactions in their environment (Reeves et al., 2008).
Data are collected using methods such as observations, field notes, or structured/ unstructured interviews.
The aim of ethnography is to provide detailed, holistic insights into people’s behavior and perspectives within their natural setting. In order to achieve this, researchers immerse themselves in a community or organization.
Due to the flexibility and real-world focus of ethnography, researchers are able to gather an in-depth, nuanced understanding of people’s experiences, knowledge and perspectives that are influenced by culture and society.
In order to develop a representative picture of a particular culture/ context, researchers must conduct extensive field work.
This can be time-consuming as researchers may need to immerse themselves into a community/ culture for a few days, or possibly a few years.
Qualitative Data Analysis Methods
Different methods can be used for analyzing qualitative data. The researcher chooses based on the objectives of their study.
The researcher plays a key role in the interpretation of data, making decisions about the coding, theming, decontextualizing, and recontextualizing of data (Starks & Trinidad, 2007).
Grounded theory
Grounded theory is a qualitative method specifically designed to inductively generate theory from data. It was developed by Glaser and Strauss in 1967 (Glaser & Strauss, 2017).
This methodology aims to develop theories (rather than test hypotheses) that explain a social process, action, or interaction (Petty et al., 2012). To inform the developing theory, data collection and analysis run simultaneously.
There are three key types of coding used in grounded theory: initial (open), intermediate (axial), and advanced (selective) coding.
Throughout the analysis, memos should be created to document methodological and theoretical ideas about the data. Data should be collected and analyzed until data saturation is reached and a theory is developed.
Content analysis
Content analysis was first used in the early twentieth century to analyze textual materials such as newspapers and political speeches.
Content analysis is a research method used to identify and analyze the presence and patterns of themes, concepts, or words in data (Vaismoradi et al., 2013).
This research method can be used to analyze data in different formats, which can be written, oral, or visual.
The goal of content analysis is to develop themes that capture the underlying meanings of data (Schreier, 2012).
Qualitative content analysis can be used to validate existing theories, support the development of new models and theories, and provide in-depth descriptions of particular settings or experiences.
The following six steps provide a guideline for how to conduct qualitative content analysis.
- Define a Research Question : To start content analysis, a clear research question should be developed.
- Identify and Collect Data : Establish the inclusion criteria for your data. Find the relevant sources to analyze.
- Define the Unit or Theme of Analysis : Categorize the content into themes. Themes can be a word, phrase, or sentence.
- Develop Rules for Coding your Data : Define a set of coding rules to ensure that all data are coded consistently.
- Code the Data : Follow the coding rules to categorize data into themes.
- Analyze the Results and Draw Conclusions : Examine the data to identify patterns and draw conclusions in relation to your research question.
Discourse analysis
Discourse analysis is a research method used to study written/ spoken language in relation to its social context (Wood & Kroger, 2000).
In discourse analysis, the researcher interprets details of language materials and the context in which it is situated.
Discourse analysis aims to understand the functions of language (how language is used in real life) and how meaning is conveyed by language in different contexts. Researchers use discourse analysis to investigate social groups and how language is used to achieve specific communication goals.
Different methods of discourse analysis can be used depending on the aims and objectives of a study. However, the following steps provide a guideline on how to conduct discourse analysis.
- Define the Research Question : Develop a relevant research question to frame the analysis.
- Gather Data and Establish the Context : Collect research materials (e.g., interview transcripts, documents). Gather factual details and review the literature to construct a theory about the social and historical context of your study.
- Analyze the Content : Closely examine various components of the text, such as the vocabulary, sentences, paragraphs, and structure of the text. Identify patterns relevant to the research question to create codes, then group these into themes.
- Review the Results : Reflect on the findings to examine the function of the language, and the meaning and context of the discourse.
Thematic analysis
Thematic analysis is a method used to identify, interpret, and report patterns in data, such as commonalities or contrasts.
Although the origin of thematic analysis can be traced back to the early twentieth century, understanding and clarity of thematic analysis is attributed to Braun and Clarke (2006).
Thematic analysis aims to develop themes (patterns of meaning) across a dataset to address a research question.
In thematic analysis, qualitative data is gathered using techniques such as interviews, focus groups, and questionnaires. Audio recordings are transcribed. The dataset is then explored and interpreted by a researcher to identify patterns.
This occurs through the rigorous process of data familiarisation, coding, theme development, and revision. These identified patterns provide a summary of the dataset and can be used to address a research question.
Themes are developed by exploring the implicit and explicit meanings within the data. Two different approaches are used to generate themes: inductive and deductive.
An inductive approach allows themes to emerge from the data. In contrast, a deductive approach uses existing theories or knowledge to apply preconceived ideas to the data.
Phases of Thematic Analysis
Braun and Clarke (2006) provide a guide of the six phases of thematic analysis. These phases can be applied flexibly to fit research questions and data.
Phase | |
---|---|
1. Gather and transcribe data | Gather raw data, for example interviews or focus groups, and transcribe audio recordings fully |
2. Familiarization with data | Read and reread all your data from beginning to end; note down initial ideas |
3. Create initial codes | Start identifying preliminary codes which highlight important features of the data and may be relevant to the research question |
4. Create new codes which encapsulate potential themes | Review initial codes and explore any similarities, differences, or contradictions to uncover underlying themes; create a map to visualize identified themes |
5. Take a break then return to the data | Take a break and then return later to review themes |
6. Evaluate themes for good fit | Last opportunity for analysis; check themes are supported and saturated with data |
Template analysis
Template analysis refers to a specific method of thematic analysis which uses hierarchical coding (Brooks et al., 2014).
Template analysis is used to analyze textual data, for example, interview transcripts or open-ended responses on a written questionnaire.
To conduct template analysis, a coding template must be developed (usually from a subset of the data) and subsequently revised and refined. This template represents the themes identified by researchers as important in the dataset.
Codes are ordered hierarchically within the template, with the highest-level codes demonstrating overarching themes in the data and lower-level codes representing constituent themes with a narrower focus.
A guideline for the main procedural steps for conducting template analysis is outlined below.
- Familiarization with the Data : Read (and reread) the dataset in full. Engage, reflect, and take notes on data that may be relevant to the research question.
- Preliminary Coding : Identify initial codes using guidance from the a priori codes, identified before the analysis as likely to be beneficial and relevant to the analysis.
- Organize Themes : Organize themes into meaningful clusters. Consider the relationships between the themes both within and between clusters.
- Produce an Initial Template : Develop an initial template. This may be based on a subset of the data.
- Apply and Develop the Template : Apply the initial template to further data and make any necessary modifications. Refinements of the template may include adding themes, removing themes, or changing the scope/title of themes.
- Finalize Template : Finalize the template, then apply it to the entire dataset.
Frame analysis
Frame analysis is a comparative form of thematic analysis which systematically analyzes data using a matrix output.
Ritchie and Spencer (1994) developed this set of techniques to analyze qualitative data in applied policy research. Frame analysis aims to generate theory from data.
Frame analysis encourages researchers to organize and manage their data using summarization.
This results in a flexible and unique matrix output, in which individual participants (or cases) are represented by rows and themes are represented by columns.
Each intersecting cell is used to summarize findings relating to the corresponding participant and theme.
Frame analysis has five distinct phases which are interrelated, forming a methodical and rigorous framework.
- Familiarization with the Data : Familiarize yourself with all the transcripts. Immerse yourself in the details of each transcript and start to note recurring themes.
- Develop a Theoretical Framework : Identify recurrent/ important themes and add them to a chart. Provide a framework/ structure for the analysis.
- Indexing : Apply the framework systematically to the entire study data.
- Summarize Data in Analytical Framework : Reduce the data into brief summaries of participants’ accounts.
- Mapping and Interpretation : Compare themes and subthemes and check against the original transcripts. Group the data into categories and provide an explanation for them.
Preventing Bias in Qualitative Research
To evaluate qualitative studies, the CASP (Critical Appraisal Skills Programme) checklist for qualitative studies can be used to ensure all aspects of a study have been considered (CASP, 2018).
The quality of research can be enhanced and assessed using criteria such as checklists, reflexivity, co-coding, and member-checking.
Co-coding
Relying on only one researcher to interpret rich and complex data may risk key insights and alternative viewpoints being missed. Therefore, coding is often performed by multiple researchers.
A common strategy must be defined at the beginning of the coding process (Busetto et al., 2020). This includes establishing a useful coding list and finding a common definition of individual codes.
Transcripts are initially coded independently by researchers and then compared and consolidated to minimize error or bias and to bring confirmation of findings.
Member checking
Member checking (or respondent validation) involves checking back with participants to see if the research resonates with their experiences (Russell & Gregory, 2003).
Data can be returned to participants after data collection or when results are first available. For example, participants may be provided with their interview transcript and asked to verify whether this is a complete and accurate representation of their views.
Participants may then clarify or elaborate on their responses to ensure they align with their views (Shenton, 2004).
This feedback becomes part of data collection and ensures accurate descriptions/ interpretations of phenomena (Mays & Pope, 2000).
Reflexivity in qualitative research
Reflexivity typically involves examining your own judgments, practices, and belief systems during data collection and analysis. It aims to identify any personal beliefs which may affect the research.
Reflexivity is essential in qualitative research to ensure methodological transparency and complete reporting. This enables readers to understand how the interaction between the researcher and participant shapes the data.
Depending on the research question and population being researched, factors that need to be considered include the experience of the researcher, how the contact was established and maintained, age, gender, and ethnicity.
These details are important because, in qualitative research, the researcher is a dynamic part of the research process and actively influences the outcome of the research (Boeije, 2014).
Reflexivity Example
Who you are and your characteristics influence how you collect and analyze data. Here is an example of a reflexivity statement for research on smoking. I am a 30-year-old white female from a middle-class background. I live in the southwest of England and have been educated to master’s level. I have been involved in two research projects on oral health. I have never smoked, but I have witnessed how smoking can cause ill health from my volunteering in a smoking cessation clinic. My research aspirations are to help to develop interventions to help smokers quit.
Establishing Trustworthiness in Qualitative Research
Trustworthiness is a concept used to assess the quality and rigor of qualitative research. Four criteria are used to assess a study’s trustworthiness: credibility, transferability, dependability, and confirmability.
1. Credibility in Qualitative Research
Credibility refers to how accurately the results represent the reality and viewpoints of the participants.
To establish credibility in research, participants’ views and the researcher’s representation of their views need to align (Tobin & Begley, 2004).
To increase the credibility of findings, researchers may use data source triangulation, investigator triangulation, peer debriefing, or member checking (Lincoln & Guba, 1985).
2. Transferability in Qualitative Research
Transferability refers to how generalizable the findings are: whether the findings may be applied to another context, setting, or group (Tobin & Begley, 2004).
Transferability can be enhanced by giving thorough and in-depth descriptions of the research setting, sample, and methods (Nowell et al., 2017).
3. Dependability in Qualitative Research
Dependability is the extent to which the study could be replicated under similar conditions and the findings would be consistent.
Researchers can establish dependability using methods such as audit trails so readers can see the research process is logical and traceable (Koch, 1994).
4. Confirmability in Qualitative Research
Confirmability is concerned with establishing that there is a clear link between the researcher’s interpretations/ findings and the data.
Researchers can achieve confirmability by demonstrating how conclusions and interpretations were arrived at (Nowell et al., 2017).
This enables readers to understand the reasoning behind the decisions made.
Audit Trails in Qualitative Research
An audit trail provides evidence of the decisions made by the researcher regarding theory, research design, and data collection, as well as the steps they have chosen to manage, analyze, and report data.
The researcher must provide a clear rationale to demonstrate how conclusions were reached in their study.
A clear description of the research path must be provided to enable readers to trace through the researcher’s logic (Halpren, 1983).
Researchers should maintain records of the raw data, field notes, transcripts, and a reflective journal in order to provide a clear audit trail.
Discovery of unexpected data
Open-ended questions in qualitative research mean the researcher can probe an interview topic and enable the participant to elaborate on responses in an unrestricted manner.
This allows unexpected data to emerge, which can lead to further research into that topic.
The exploratory nature of qualitative research helps generate hypotheses that can be tested quantitatively (Busetto et al., 2020).
Flexibility
Data collection and analysis can be modified and adapted to take the research in a different direction if new ideas or patterns emerge in the data.
This enables researchers to investigate new opportunities while firmly maintaining their research goals.
Naturalistic settings
The behaviors of participants are recorded in real-world settings. Studies that use real-world settings have high ecological validity since participants behave more authentically.
Limitations
Time-consuming .
Qualitative research results in large amounts of data which often need to be transcribed and analyzed manually.
Even when software is used, transcription can be inaccurate, and using software for analysis can result in many codes which need to be condensed into themes.
Subjectivity
The researcher has an integral role in collecting and interpreting qualitative data. Therefore, the conclusions reached are from their perspective and experience.
Consequently, interpretations of data from another researcher may vary greatly.
Limited generalizability
The aim of qualitative research is to provide a detailed, contextualized understanding of an aspect of the human experience from a relatively small sample size.
Despite rigorous analysis procedures, conclusions drawn cannot be generalized to the wider population since data may be biased or unrepresentative.
Therefore, results are only applicable to a small group of the population.
While individual qualitative studies are often limited in their generalizability due to factors such as sample size and context, metasynthesis enables researchers to synthesize findings from multiple studies, potentially leading to more generalizable conclusions.
By integrating findings from studies conducted in diverse settings and with different populations, metasynthesis can provide broader insights into the phenomenon of interest.
Extraneous variables
Qualitative research is often conducted in real-world settings. This may cause results to be unreliable since extraneous variables may affect the data, for example:
- Situational variables : different environmental conditions may influence participants’ behavior in a study. The random variation in factors (such as noise or lighting) may be difficult to control in real-world settings.
- Participant characteristics : this includes any characteristics that may influence how a participant answers/ behaves in a study. This may include a participant’s mood, gender, age, ethnicity, sexual identity, IQ, etc.
- Experimenter effect : experimenter effect refers to how a researcher’s unintentional influence can change the outcome of a study. This occurs when (i) their interactions with participants unintentionally change participants’ behaviors or (ii) due to errors in observation, interpretation, or analysis.
What sample size should qualitative research be?
The sample size for qualitative studies has been recommended to include a minimum of 12 participants to reach data saturation (Braun, 2013).
Are surveys qualitative or quantitative?
Surveys can be used to gather information from a sample qualitatively or quantitatively. Qualitative surveys use open-ended questions to gather detailed information from a large sample using free text responses.
The use of open-ended questions allows for unrestricted responses where participants use their own words, enabling the collection of more in-depth information than closed-ended questions.
In contrast, quantitative surveys consist of closed-ended questions with multiple-choice answer options. Quantitative surveys are ideal to gather a statistical representation of a population.
What are the ethical considerations of qualitative research?
Before conducting a study, you must think about any risks that could occur and take steps to prevent them. Participant Protection : Researchers must protect participants from physical and mental harm. This means you must not embarrass, frighten, offend, or harm participants. Transparency : Researchers are obligated to clearly communicate how they will collect, store, analyze, use, and share the data. Confidentiality : You need to consider how to maintain the confidentiality and anonymity of participants’ data.
What is triangulation in qualitative research?
Triangulation refers to the use of several approaches in a study to comprehensively understand phenomena. This method helps to increase the validity and credibility of research findings.
Types of triangulation include method triangulation (using multiple methods to gather data); investigator triangulation (multiple researchers for collecting/ analyzing data), theory triangulation (comparing several theoretical perspectives to explain a phenomenon), and data source triangulation (using data from various times, locations, and people; Carter et al., 2014).
Why is qualitative research important?
Qualitative research allows researchers to describe and explain the social world. The exploratory nature of qualitative research helps to generate hypotheses that can then be tested quantitatively.
In qualitative research, participants are able to express their thoughts, experiences, and feelings without constraint.
Additionally, researchers are able to follow up on participants’ answers in real-time, generating valuable discussion around a topic. This enables researchers to gain a nuanced understanding of phenomena which is difficult to attain using quantitative methods.
What is coding data in qualitative research?
Coding data is a qualitative data analysis strategy in which a section of text is assigned with a label that describes its content.
These labels may be words or phrases which represent important (and recurring) patterns in the data.
This process enables researchers to identify related content across the dataset. Codes can then be used to group similar types of data to generate themes.
What is the difference between qualitative and quantitative research?
Qualitative research involves the collection and analysis of non-numerical data in order to understand experiences and meanings from the participant’s perspective.
This can provide rich, in-depth insights on complicated phenomena. Qualitative data may be collected using interviews, focus groups, or observations.
In contrast, quantitative research involves the collection and analysis of numerical data to measure the frequency, magnitude, or relationships of variables. This can provide objective and reliable evidence that can be generalized to the wider population.
Quantitative data may be collected using closed-ended questionnaires or experiments.
What is trustworthiness in qualitative research?
Trustworthiness is a concept used to assess the quality and rigor of qualitative research. Four criteria are used to assess a study’s trustworthiness: credibility, transferability, dependability, and confirmability.
Credibility refers to how accurately the results represent the reality and viewpoints of the participants. Transferability refers to whether the findings may be applied to another context, setting, or group.
Dependability is the extent to which the findings are consistent and reliable. Confirmability refers to the objectivity of findings (not influenced by the bias or assumptions of researchers).
What is data saturation in qualitative research?
Data saturation is a methodological principle used to guide the sample size of a qualitative research study.
Data saturation is proposed as a necessary methodological component in qualitative research (Saunders et al., 2018) as it is a vital criterion for discontinuing data collection and/or analysis.
The intention of data saturation is to find “no new data, no new themes, no new coding, and ability to replicate the study” (Guest et al., 2006). Therefore, enough data has been gathered to make conclusions.
Why is sampling in qualitative research important?
In quantitative research, large sample sizes are used to provide statistically significant quantitative estimates.
This is because quantitative research aims to provide generalizable conclusions that represent populations.
However, the aim of sampling in qualitative research is to gather data that will help the researcher understand the depth, complexity, variation, or context of a phenomenon. The small sample sizes in qualitative studies support the depth of case-oriented analysis.
What is narrative analysis?
Narrative analysis is a qualitative research method used to understand how individuals create stories from their personal experiences.
There is an emphasis on understanding the context in which a narrative is constructed, recognizing the influence of historical, cultural, and social factors on storytelling.
Researchers can use different methods together to explore a research question.
Some narrative researchers focus on the content of what is said, using thematic narrative analysis, while others focus on the structure, such as holistic-form or categorical-form structural narrative analysis. Others focus on how the narrative is produced and performed.
Boeije, H. (2014). Analysis in qualitative research. Sage.
Braun, V., & Clarke, V. (2006). Using thematic analysis in psychology. Qualitative research in psychology , 3 (2), 77-101. https://doi.org/10.1191/1478088706qp063oa
Brooks, J., McCluskey, S., Turley, E., & King, N. (2014). The utility of template analysis in qualitative psychology research. Qualitative Research in Psychology , 12 (2), 202–222. https://doi.org/10.1080/14780887.2014.955224
Busetto, L., Wick, W., & Gumbinger, C. (2020). How to use and assess qualitative research methods. Neurological research and practice , 2 (1), 14-14. https://doi.org/10.1186/s42466-020-00059-z
Carter, N., Bryant-Lukosius, D., DiCenso, A., Blythe, J., & Neville, A. J. (2014). The use of triangulation in qualitative research. Oncology nursing forum , 41 (5), 545–547. https://doi.org/10.1188/14.ONF.545-547
Critical Appraisal Skills Programme. (2018). CASP Checklist: 10 questions to help you make sense of a Qualitative research. https://casp-uk.net/images/checklist/documents/CASP-Qualitative-Studies-Checklist/CASP-Qualitative-Checklist-2018_fillable_form.pdf Accessed: March 15 2023
Clarke, V., & Braun, V. (2013). Successful qualitative research: A practical guide for beginners. Successful Qualitative Research , 1-400.
Denny, E., & Weckesser, A. (2022). How to do qualitative research?: Qualitative research methods. BJOG : an international journal of obstetrics and gynaecology , 129 (7), 1166-1167. https://doi.org/10.1111/1471-0528.17150
Glaser, B. G., & Strauss, A. L. (2017). The discovery of grounded theory. The Discovery of Grounded Theory , 1–18. https://doi.org/10.4324/9780203793206-1
Guest, G., Bunce, A., & Johnson, L. (2006). How many interviews are enough? An experiment with data saturation and variability. Field Methods, 18 (1), 59-82. doi:10.1177/1525822X05279903
Halpren, E. S. (1983). Auditing naturalistic inquiries: The development and application of a model (Unpublished doctoral dissertation). Indiana University, Bloomington.
Hammarberg, K., Kirkman, M., & de Lacey, S. (2016). Qualitative research methods: When to use them and how to judge them. Human Reproduction , 31 (3), 498–501. https://doi.org/10.1093/humrep/dev334
Koch, T. (1994). Establishing rigour in qualitative research: The decision trail. Journal of Advanced Nursing, 19, 976–986. doi:10.1111/ j.1365-2648.1994.tb01177.x
Lincoln, Y., & Guba, E. G. (1985). Naturalistic inquiry. Newbury Park, CA: Sage.
Mays, N., & Pope, C. (2000). Assessing quality in qualitative research. BMJ, 320(7226), 50–52.
Minichiello, V. (1990). In-Depth Interviewing: Researching People. Longman Cheshire.
Nowell, L. S., Norris, J. M., White, D. E., & Moules, N. J. (2017). Thematic Analysis: Striving to Meet the Trustworthiness Criteria. International Journal of Qualitative Methods, 16 (1). https://doi.org/10.1177/1609406917733847
Petty, N. J., Thomson, O. P., & Stew, G. (2012). Ready for a paradigm shift? part 2: Introducing qualitative research methodologies and methods. Manual Therapy , 17 (5), 378–384. https://doi.org/10.1016/j.math.2012.03.004
Punch, K. F. (2013). Introduction to social research: Quantitative and qualitative approaches. London: Sage
Reeves, S., Kuper, A., & Hodges, B. D. (2008). Qualitative research methodologies: Ethnography. BMJ , 337 (aug07 3). https://doi.org/10.1136/bmj.a1020
Russell, C. K., & Gregory, D. M. (2003). Evaluation of qualitative research studies. Evidence Based Nursing, 6 (2), 36–40.
Saunders, B., Sim, J., Kingstone, T., Baker, S., Waterfield, J., Bartlam, B., Burroughs, H., & Jinks, C. (2018). Saturation in qualitative research: exploring its conceptualization and operationalization. Quality & quantity , 52 (4), 1893–1907. https://doi.org/10.1007/s11135-017-0574-8
Scarduzio, J. A. (2017). Emic approach to qualitative research. The International Encyclopedia of Communication Research Methods, 1–2 . https://doi.org/10.1002/9781118901731.iecrm0082
Schreier, M. (2012). Qualitative content analysis in practice / Margrit Schreier.
Shenton, A. K. (2004). Strategies for ensuring trustworthiness in qualitative research projects. Education for Information, 22 , 63–75.
Starks, H., & Trinidad, S. B. (2007). Choose your method: a comparison of phenomenology, discourse analysis, and grounded theory. Qualitative health research , 17 (10), 1372–1380. https://doi.org/10.1177/1049732307307031
Tenny, S., Brannan, J. M., & Brannan, G. D. (2022). Qualitative Study. In StatPearls. StatPearls Publishing.
Tobin, G. A., & Begley, C. M. (2004). Methodological rigour within a qualitative framework. Journal of Advanced Nursing, 48, 388–396. doi:10.1111/j.1365-2648.2004.03207.x
Vaismoradi, M., Turunen, H., & Bondas, T. (2013). Content analysis and thematic analysis: Implications for conducting a qualitative descriptive study. Nursing & health sciences , 15 (3), 398-405. https://doi.org/10.1111/nhs.12048
Wood L. A., Kroger R. O. (2000). Doing discourse analysis: Methods for studying action in talk and text. Sage.
Yilmaz, K. (2013). Comparison of Quantitative and Qualitative Research Traditions: epistemological, theoretical, and methodological differences. European journal of education , 48 (2), 311-325. https://doi.org/10.1111/ejed.12014

Have a language expert improve your writing
Run a free plagiarism check in 10 minutes, generate accurate citations for free.
- Knowledge Base
Methodology
- What Is Qualitative Research? | Methods & Examples
What Is Qualitative Research? | Methods & Examples
Published on June 19, 2020 by Pritha Bhandari . Revised on June 22, 2023.
Qualitative research involves collecting and analyzing non-numerical data (e.g., text, video, or audio) to understand concepts, opinions, or experiences. It can be used to gather in-depth insights into a problem or generate new ideas for research.
Qualitative research is the opposite of quantitative research , which involves collecting and analyzing numerical data for statistical analysis.
Qualitative research is commonly used in the humanities and social sciences, in subjects such as anthropology, sociology, education, health sciences, history, etc.
- How does social media shape body image in teenagers?
- How do children and adults interpret healthy eating in the UK?
- What factors influence employee retention in a large organization?
- How is anxiety experienced around the world?
- How can teachers integrate social issues into science curriculums?
Table of contents
Approaches to qualitative research, qualitative research methods, qualitative data analysis, advantages of qualitative research, disadvantages of qualitative research, other interesting articles, frequently asked questions about qualitative research.
Qualitative research is used to understand how people experience the world. While there are many approaches to qualitative research, they tend to be flexible and focus on retaining rich meaning when interpreting data.
Common approaches include grounded theory, ethnography , action research , phenomenological research, and narrative research. They share some similarities, but emphasize different aims and perspectives.
Approach | What does it involve? |
---|---|
Grounded theory | Researchers collect rich data on a topic of interest and develop theories . |
Researchers immerse themselves in groups or organizations to understand their cultures. | |
Action research | Researchers and participants collaboratively link theory to practice to drive social change. |
Phenomenological research | Researchers investigate a phenomenon or event by describing and interpreting participants’ lived experiences. |
Narrative research | Researchers examine how stories are told to understand how participants perceive and make sense of their experiences. |
Note that qualitative research is at risk for certain research biases including the Hawthorne effect , observer bias , recall bias , and social desirability bias . While not always totally avoidable, awareness of potential biases as you collect and analyze your data can prevent them from impacting your work too much.
Here's why students love Scribbr's proofreading services
Discover proofreading & editing
Each of the research approaches involve using one or more data collection methods . These are some of the most common qualitative methods:
- Observations: recording what you have seen, heard, or encountered in detailed field notes.
- Interviews: personally asking people questions in one-on-one conversations.
- Focus groups: asking questions and generating discussion among a group of people.
- Surveys : distributing questionnaires with open-ended questions.
- Secondary research: collecting existing data in the form of texts, images, audio or video recordings, etc.
- You take field notes with observations and reflect on your own experiences of the company culture.
- You distribute open-ended surveys to employees across all the company’s offices by email to find out if the culture varies across locations.
- You conduct in-depth interviews with employees in your office to learn about their experiences and perspectives in greater detail.
Qualitative researchers often consider themselves “instruments” in research because all observations, interpretations and analyses are filtered through their own personal lens.
For this reason, when writing up your methodology for qualitative research, it’s important to reflect on your approach and to thoroughly explain the choices you made in collecting and analyzing the data.
Qualitative data can take the form of texts, photos, videos and audio. For example, you might be working with interview transcripts, survey responses, fieldnotes, or recordings from natural settings.
Most types of qualitative data analysis share the same five steps:
- Prepare and organize your data. This may mean transcribing interviews or typing up fieldnotes.
- Review and explore your data. Examine the data for patterns or repeated ideas that emerge.
- Develop a data coding system. Based on your initial ideas, establish a set of codes that you can apply to categorize your data.
- Assign codes to the data. For example, in qualitative survey analysis, this may mean going through each participant’s responses and tagging them with codes in a spreadsheet. As you go through your data, you can create new codes to add to your system if necessary.
- Identify recurring themes. Link codes together into cohesive, overarching themes.
There are several specific approaches to analyzing qualitative data. Although these methods share similar processes, they emphasize different concepts.
Approach | When to use | Example |
---|---|---|
To describe and categorize common words, phrases, and ideas in qualitative data. | A market researcher could perform content analysis to find out what kind of language is used in descriptions of therapeutic apps. | |
To identify and interpret patterns and themes in qualitative data. | A psychologist could apply thematic analysis to travel blogs to explore how tourism shapes self-identity. | |
To examine the content, structure, and design of texts. | A media researcher could use textual analysis to understand how news coverage of celebrities has changed in the past decade. | |
To study communication and how language is used to achieve effects in specific contexts. | A political scientist could use discourse analysis to study how politicians generate trust in election campaigns. |
Qualitative research often tries to preserve the voice and perspective of participants and can be adjusted as new research questions arise. Qualitative research is good for:
- Flexibility
The data collection and analysis process can be adapted as new ideas or patterns emerge. They are not rigidly decided beforehand.
- Natural settings
Data collection occurs in real-world contexts or in naturalistic ways.
- Meaningful insights
Detailed descriptions of people’s experiences, feelings and perceptions can be used in designing, testing or improving systems or products.
- Generation of new ideas
Open-ended responses mean that researchers can uncover novel problems or opportunities that they wouldn’t have thought of otherwise.
Prevent plagiarism. Run a free check.
Researchers must consider practical and theoretical limitations in analyzing and interpreting their data. Qualitative research suffers from:
- Unreliability
The real-world setting often makes qualitative research unreliable because of uncontrolled factors that affect the data.
- Subjectivity
Due to the researcher’s primary role in analyzing and interpreting data, qualitative research cannot be replicated . The researcher decides what is important and what is irrelevant in data analysis, so interpretations of the same data can vary greatly.
- Limited generalizability
Small samples are often used to gather detailed data about specific contexts. Despite rigorous analysis procedures, it is difficult to draw generalizable conclusions because the data may be biased and unrepresentative of the wider population .
- Labor-intensive
Although software can be used to manage and record large amounts of text, data analysis often has to be checked or performed manually.
If you want to know more about statistics , methodology , or research bias , make sure to check out some of our other articles with explanations and examples.
- Chi square goodness of fit test
- Degrees of freedom
- Null hypothesis
- Discourse analysis
- Control groups
- Mixed methods research
- Non-probability sampling
- Quantitative research
- Inclusion and exclusion criteria
Research bias
- Rosenthal effect
- Implicit bias
- Cognitive bias
- Selection bias
- Negativity bias
- Status quo bias
Quantitative research deals with numbers and statistics, while qualitative research deals with words and meanings.
Quantitative methods allow you to systematically measure variables and test hypotheses . Qualitative methods allow you to explore concepts and experiences in more detail.
There are five common approaches to qualitative research :
- Grounded theory involves collecting data in order to develop new theories.
- Ethnography involves immersing yourself in a group or organization to understand its culture.
- Narrative research involves interpreting stories to understand how people make sense of their experiences and perceptions.
- Phenomenological research involves investigating phenomena through people’s lived experiences.
- Action research links theory and practice in several cycles to drive innovative changes.
Data collection is the systematic process by which observations or measurements are gathered in research. It is used in many different contexts by academics, governments, businesses, and other organizations.
There are various approaches to qualitative data analysis , but they all share five steps in common:
- Prepare and organize your data.
- Review and explore your data.
- Develop a data coding system.
- Assign codes to the data.
- Identify recurring themes.
The specifics of each step depend on the focus of the analysis. Some common approaches include textual analysis , thematic analysis , and discourse analysis .
Cite this Scribbr article
If you want to cite this source, you can copy and paste the citation or click the “Cite this Scribbr article” button to automatically add the citation to our free Citation Generator.
Bhandari, P. (2023, June 22). What Is Qualitative Research? | Methods & Examples. Scribbr. Retrieved September 2, 2024, from https://www.scribbr.com/methodology/qualitative-research/
Is this article helpful?
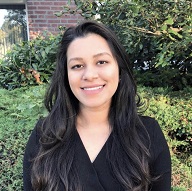
Pritha Bhandari
Other students also liked, qualitative vs. quantitative research | differences, examples & methods, how to do thematic analysis | step-by-step guide & examples, get unlimited documents corrected.
✔ Free APA citation check included ✔ Unlimited document corrections ✔ Specialized in correcting academic texts
- Privacy Policy

Home » Qualitative Research – Methods, Analysis Types and Guide
Qualitative Research – Methods, Analysis Types and Guide
Table of Contents

Qualitative Research
Qualitative research is a type of research methodology that focuses on exploring and understanding people’s beliefs, attitudes, behaviors, and experiences through the collection and analysis of non-numerical data. It seeks to answer research questions through the examination of subjective data, such as interviews, focus groups, observations, and textual analysis.
Qualitative research aims to uncover the meaning and significance of social phenomena, and it typically involves a more flexible and iterative approach to data collection and analysis compared to quantitative research. Qualitative research is often used in fields such as sociology, anthropology, psychology, and education.
Qualitative Research Methods

Qualitative Research Methods are as follows:
One-to-One Interview
This method involves conducting an interview with a single participant to gain a detailed understanding of their experiences, attitudes, and beliefs. One-to-one interviews can be conducted in-person, over the phone, or through video conferencing. The interviewer typically uses open-ended questions to encourage the participant to share their thoughts and feelings. One-to-one interviews are useful for gaining detailed insights into individual experiences.
Focus Groups
This method involves bringing together a group of people to discuss a specific topic in a structured setting. The focus group is led by a moderator who guides the discussion and encourages participants to share their thoughts and opinions. Focus groups are useful for generating ideas and insights, exploring social norms and attitudes, and understanding group dynamics.
Ethnographic Studies
This method involves immersing oneself in a culture or community to gain a deep understanding of its norms, beliefs, and practices. Ethnographic studies typically involve long-term fieldwork and observation, as well as interviews and document analysis. Ethnographic studies are useful for understanding the cultural context of social phenomena and for gaining a holistic understanding of complex social processes.
Text Analysis
This method involves analyzing written or spoken language to identify patterns and themes. Text analysis can be quantitative or qualitative. Qualitative text analysis involves close reading and interpretation of texts to identify recurring themes, concepts, and patterns. Text analysis is useful for understanding media messages, public discourse, and cultural trends.
This method involves an in-depth examination of a single person, group, or event to gain an understanding of complex phenomena. Case studies typically involve a combination of data collection methods, such as interviews, observations, and document analysis, to provide a comprehensive understanding of the case. Case studies are useful for exploring unique or rare cases, and for generating hypotheses for further research.
Process of Observation
This method involves systematically observing and recording behaviors and interactions in natural settings. The observer may take notes, use audio or video recordings, or use other methods to document what they see. Process of observation is useful for understanding social interactions, cultural practices, and the context in which behaviors occur.
Record Keeping
This method involves keeping detailed records of observations, interviews, and other data collected during the research process. Record keeping is essential for ensuring the accuracy and reliability of the data, and for providing a basis for analysis and interpretation.
This method involves collecting data from a large sample of participants through a structured questionnaire. Surveys can be conducted in person, over the phone, through mail, or online. Surveys are useful for collecting data on attitudes, beliefs, and behaviors, and for identifying patterns and trends in a population.
Qualitative data analysis is a process of turning unstructured data into meaningful insights. It involves extracting and organizing information from sources like interviews, focus groups, and surveys. The goal is to understand people’s attitudes, behaviors, and motivations
Qualitative Research Analysis Methods
Qualitative Research analysis methods involve a systematic approach to interpreting and making sense of the data collected in qualitative research. Here are some common qualitative data analysis methods:
Thematic Analysis
This method involves identifying patterns or themes in the data that are relevant to the research question. The researcher reviews the data, identifies keywords or phrases, and groups them into categories or themes. Thematic analysis is useful for identifying patterns across multiple data sources and for generating new insights into the research topic.
Content Analysis
This method involves analyzing the content of written or spoken language to identify key themes or concepts. Content analysis can be quantitative or qualitative. Qualitative content analysis involves close reading and interpretation of texts to identify recurring themes, concepts, and patterns. Content analysis is useful for identifying patterns in media messages, public discourse, and cultural trends.
Discourse Analysis
This method involves analyzing language to understand how it constructs meaning and shapes social interactions. Discourse analysis can involve a variety of methods, such as conversation analysis, critical discourse analysis, and narrative analysis. Discourse analysis is useful for understanding how language shapes social interactions, cultural norms, and power relationships.
Grounded Theory Analysis
This method involves developing a theory or explanation based on the data collected. Grounded theory analysis starts with the data and uses an iterative process of coding and analysis to identify patterns and themes in the data. The theory or explanation that emerges is grounded in the data, rather than preconceived hypotheses. Grounded theory analysis is useful for understanding complex social phenomena and for generating new theoretical insights.
Narrative Analysis
This method involves analyzing the stories or narratives that participants share to gain insights into their experiences, attitudes, and beliefs. Narrative analysis can involve a variety of methods, such as structural analysis, thematic analysis, and discourse analysis. Narrative analysis is useful for understanding how individuals construct their identities, make sense of their experiences, and communicate their values and beliefs.
Phenomenological Analysis
This method involves analyzing how individuals make sense of their experiences and the meanings they attach to them. Phenomenological analysis typically involves in-depth interviews with participants to explore their experiences in detail. Phenomenological analysis is useful for understanding subjective experiences and for developing a rich understanding of human consciousness.
Comparative Analysis
This method involves comparing and contrasting data across different cases or groups to identify similarities and differences. Comparative analysis can be used to identify patterns or themes that are common across multiple cases, as well as to identify unique or distinctive features of individual cases. Comparative analysis is useful for understanding how social phenomena vary across different contexts and groups.
Applications of Qualitative Research
Qualitative research has many applications across different fields and industries. Here are some examples of how qualitative research is used:
- Market Research: Qualitative research is often used in market research to understand consumer attitudes, behaviors, and preferences. Researchers conduct focus groups and one-on-one interviews with consumers to gather insights into their experiences and perceptions of products and services.
- Health Care: Qualitative research is used in health care to explore patient experiences and perspectives on health and illness. Researchers conduct in-depth interviews with patients and their families to gather information on their experiences with different health care providers and treatments.
- Education: Qualitative research is used in education to understand student experiences and to develop effective teaching strategies. Researchers conduct classroom observations and interviews with students and teachers to gather insights into classroom dynamics and instructional practices.
- Social Work : Qualitative research is used in social work to explore social problems and to develop interventions to address them. Researchers conduct in-depth interviews with individuals and families to understand their experiences with poverty, discrimination, and other social problems.
- Anthropology : Qualitative research is used in anthropology to understand different cultures and societies. Researchers conduct ethnographic studies and observe and interview members of different cultural groups to gain insights into their beliefs, practices, and social structures.
- Psychology : Qualitative research is used in psychology to understand human behavior and mental processes. Researchers conduct in-depth interviews with individuals to explore their thoughts, feelings, and experiences.
- Public Policy : Qualitative research is used in public policy to explore public attitudes and to inform policy decisions. Researchers conduct focus groups and one-on-one interviews with members of the public to gather insights into their perspectives on different policy issues.
How to Conduct Qualitative Research
Here are some general steps for conducting qualitative research:
- Identify your research question: Qualitative research starts with a research question or set of questions that you want to explore. This question should be focused and specific, but also broad enough to allow for exploration and discovery.
- Select your research design: There are different types of qualitative research designs, including ethnography, case study, grounded theory, and phenomenology. You should select a design that aligns with your research question and that will allow you to gather the data you need to answer your research question.
- Recruit participants: Once you have your research question and design, you need to recruit participants. The number of participants you need will depend on your research design and the scope of your research. You can recruit participants through advertisements, social media, or through personal networks.
- Collect data: There are different methods for collecting qualitative data, including interviews, focus groups, observation, and document analysis. You should select the method or methods that align with your research design and that will allow you to gather the data you need to answer your research question.
- Analyze data: Once you have collected your data, you need to analyze it. This involves reviewing your data, identifying patterns and themes, and developing codes to organize your data. You can use different software programs to help you analyze your data, or you can do it manually.
- Interpret data: Once you have analyzed your data, you need to interpret it. This involves making sense of the patterns and themes you have identified, and developing insights and conclusions that answer your research question. You should be guided by your research question and use your data to support your conclusions.
- Communicate results: Once you have interpreted your data, you need to communicate your results. This can be done through academic papers, presentations, or reports. You should be clear and concise in your communication, and use examples and quotes from your data to support your findings.
Examples of Qualitative Research
Here are some real-time examples of qualitative research:
- Customer Feedback: A company may conduct qualitative research to understand the feedback and experiences of its customers. This may involve conducting focus groups or one-on-one interviews with customers to gather insights into their attitudes, behaviors, and preferences.
- Healthcare : A healthcare provider may conduct qualitative research to explore patient experiences and perspectives on health and illness. This may involve conducting in-depth interviews with patients and their families to gather information on their experiences with different health care providers and treatments.
- Education : An educational institution may conduct qualitative research to understand student experiences and to develop effective teaching strategies. This may involve conducting classroom observations and interviews with students and teachers to gather insights into classroom dynamics and instructional practices.
- Social Work: A social worker may conduct qualitative research to explore social problems and to develop interventions to address them. This may involve conducting in-depth interviews with individuals and families to understand their experiences with poverty, discrimination, and other social problems.
- Anthropology : An anthropologist may conduct qualitative research to understand different cultures and societies. This may involve conducting ethnographic studies and observing and interviewing members of different cultural groups to gain insights into their beliefs, practices, and social structures.
- Psychology : A psychologist may conduct qualitative research to understand human behavior and mental processes. This may involve conducting in-depth interviews with individuals to explore their thoughts, feelings, and experiences.
- Public Policy: A government agency or non-profit organization may conduct qualitative research to explore public attitudes and to inform policy decisions. This may involve conducting focus groups and one-on-one interviews with members of the public to gather insights into their perspectives on different policy issues.
Purpose of Qualitative Research
The purpose of qualitative research is to explore and understand the subjective experiences, behaviors, and perspectives of individuals or groups in a particular context. Unlike quantitative research, which focuses on numerical data and statistical analysis, qualitative research aims to provide in-depth, descriptive information that can help researchers develop insights and theories about complex social phenomena.
Qualitative research can serve multiple purposes, including:
- Exploring new or emerging phenomena : Qualitative research can be useful for exploring new or emerging phenomena, such as new technologies or social trends. This type of research can help researchers develop a deeper understanding of these phenomena and identify potential areas for further study.
- Understanding complex social phenomena : Qualitative research can be useful for exploring complex social phenomena, such as cultural beliefs, social norms, or political processes. This type of research can help researchers develop a more nuanced understanding of these phenomena and identify factors that may influence them.
- Generating new theories or hypotheses: Qualitative research can be useful for generating new theories or hypotheses about social phenomena. By gathering rich, detailed data about individuals’ experiences and perspectives, researchers can develop insights that may challenge existing theories or lead to new lines of inquiry.
- Providing context for quantitative data: Qualitative research can be useful for providing context for quantitative data. By gathering qualitative data alongside quantitative data, researchers can develop a more complete understanding of complex social phenomena and identify potential explanations for quantitative findings.
When to use Qualitative Research
Here are some situations where qualitative research may be appropriate:
- Exploring a new area: If little is known about a particular topic, qualitative research can help to identify key issues, generate hypotheses, and develop new theories.
- Understanding complex phenomena: Qualitative research can be used to investigate complex social, cultural, or organizational phenomena that are difficult to measure quantitatively.
- Investigating subjective experiences: Qualitative research is particularly useful for investigating the subjective experiences of individuals or groups, such as their attitudes, beliefs, values, or emotions.
- Conducting formative research: Qualitative research can be used in the early stages of a research project to develop research questions, identify potential research participants, and refine research methods.
- Evaluating interventions or programs: Qualitative research can be used to evaluate the effectiveness of interventions or programs by collecting data on participants’ experiences, attitudes, and behaviors.
Characteristics of Qualitative Research
Qualitative research is characterized by several key features, including:
- Focus on subjective experience: Qualitative research is concerned with understanding the subjective experiences, beliefs, and perspectives of individuals or groups in a particular context. Researchers aim to explore the meanings that people attach to their experiences and to understand the social and cultural factors that shape these meanings.
- Use of open-ended questions: Qualitative research relies on open-ended questions that allow participants to provide detailed, in-depth responses. Researchers seek to elicit rich, descriptive data that can provide insights into participants’ experiences and perspectives.
- Sampling-based on purpose and diversity: Qualitative research often involves purposive sampling, in which participants are selected based on specific criteria related to the research question. Researchers may also seek to include participants with diverse experiences and perspectives to capture a range of viewpoints.
- Data collection through multiple methods: Qualitative research typically involves the use of multiple data collection methods, such as in-depth interviews, focus groups, and observation. This allows researchers to gather rich, detailed data from multiple sources, which can provide a more complete picture of participants’ experiences and perspectives.
- Inductive data analysis: Qualitative research relies on inductive data analysis, in which researchers develop theories and insights based on the data rather than testing pre-existing hypotheses. Researchers use coding and thematic analysis to identify patterns and themes in the data and to develop theories and explanations based on these patterns.
- Emphasis on researcher reflexivity: Qualitative research recognizes the importance of the researcher’s role in shaping the research process and outcomes. Researchers are encouraged to reflect on their own biases and assumptions and to be transparent about their role in the research process.
Advantages of Qualitative Research
Qualitative research offers several advantages over other research methods, including:
- Depth and detail: Qualitative research allows researchers to gather rich, detailed data that provides a deeper understanding of complex social phenomena. Through in-depth interviews, focus groups, and observation, researchers can gather detailed information about participants’ experiences and perspectives that may be missed by other research methods.
- Flexibility : Qualitative research is a flexible approach that allows researchers to adapt their methods to the research question and context. Researchers can adjust their research methods in real-time to gather more information or explore unexpected findings.
- Contextual understanding: Qualitative research is well-suited to exploring the social and cultural context in which individuals or groups are situated. Researchers can gather information about cultural norms, social structures, and historical events that may influence participants’ experiences and perspectives.
- Participant perspective : Qualitative research prioritizes the perspective of participants, allowing researchers to explore subjective experiences and understand the meanings that participants attach to their experiences.
- Theory development: Qualitative research can contribute to the development of new theories and insights about complex social phenomena. By gathering rich, detailed data and using inductive data analysis, researchers can develop new theories and explanations that may challenge existing understandings.
- Validity : Qualitative research can offer high validity by using multiple data collection methods, purposive and diverse sampling, and researcher reflexivity. This can help ensure that findings are credible and trustworthy.
Limitations of Qualitative Research
Qualitative research also has some limitations, including:
- Subjectivity : Qualitative research relies on the subjective interpretation of researchers, which can introduce bias into the research process. The researcher’s perspective, beliefs, and experiences can influence the way data is collected, analyzed, and interpreted.
- Limited generalizability: Qualitative research typically involves small, purposive samples that may not be representative of larger populations. This limits the generalizability of findings to other contexts or populations.
- Time-consuming: Qualitative research can be a time-consuming process, requiring significant resources for data collection, analysis, and interpretation.
- Resource-intensive: Qualitative research may require more resources than other research methods, including specialized training for researchers, specialized software for data analysis, and transcription services.
- Limited reliability: Qualitative research may be less reliable than quantitative research, as it relies on the subjective interpretation of researchers. This can make it difficult to replicate findings or compare results across different studies.
- Ethics and confidentiality: Qualitative research involves collecting sensitive information from participants, which raises ethical concerns about confidentiality and informed consent. Researchers must take care to protect the privacy and confidentiality of participants and obtain informed consent.
Also see Research Methods
About the author
Muhammad Hassan
Researcher, Academic Writer, Web developer
You may also like

Ethnographic Research -Types, Methods and Guide

Applied Research – Types, Methods and Examples

Focus Groups – Steps, Examples and Guide

Experimental Design – Types, Methods, Guide

Case Study – Methods, Examples and Guide

Qualitative Research Methods
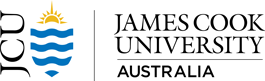
Want to create or adapt books like this? Learn more about how Pressbooks supports open publishing practices.
4.2 Definitions and Characteristics of Qualitative Research
Qualitative research aims to uncover the meaning and understanding of phenomena that cannot be broken down into measurable elements. It is based on naturalistic, interpretative and humanistic notions. 5 This research method seeks to discover, explore, identify or describe subjective human experiences using non-statistical methods and develops themes from the study participants’ stories. 5 Figure 4.1 depicts major features/ characteristics of qualitative research. It utilises exploratory open-ended questions and observations to search for patterns of meaning in collected data (e.g. observation, verbal/written narrative data, photographs, etc.) and uses inductive thinking (from specific observations to more general rules) to interpret meaning. 6 Participants’ voice is evident through quotations and description of the work. 6 The context/ setting of the study and the researcher’s reflexivity (i.e. “reflection on and awareness of their bias”, the effect of the researcher’s experience on the data and interpretations) are very important and described as part of data collection. 6 Analysis of collected data is complex, often involves inductive data analysis (exploration, contrasts, specific to general) and requires multiple coding and development of themes from participant stories. 6
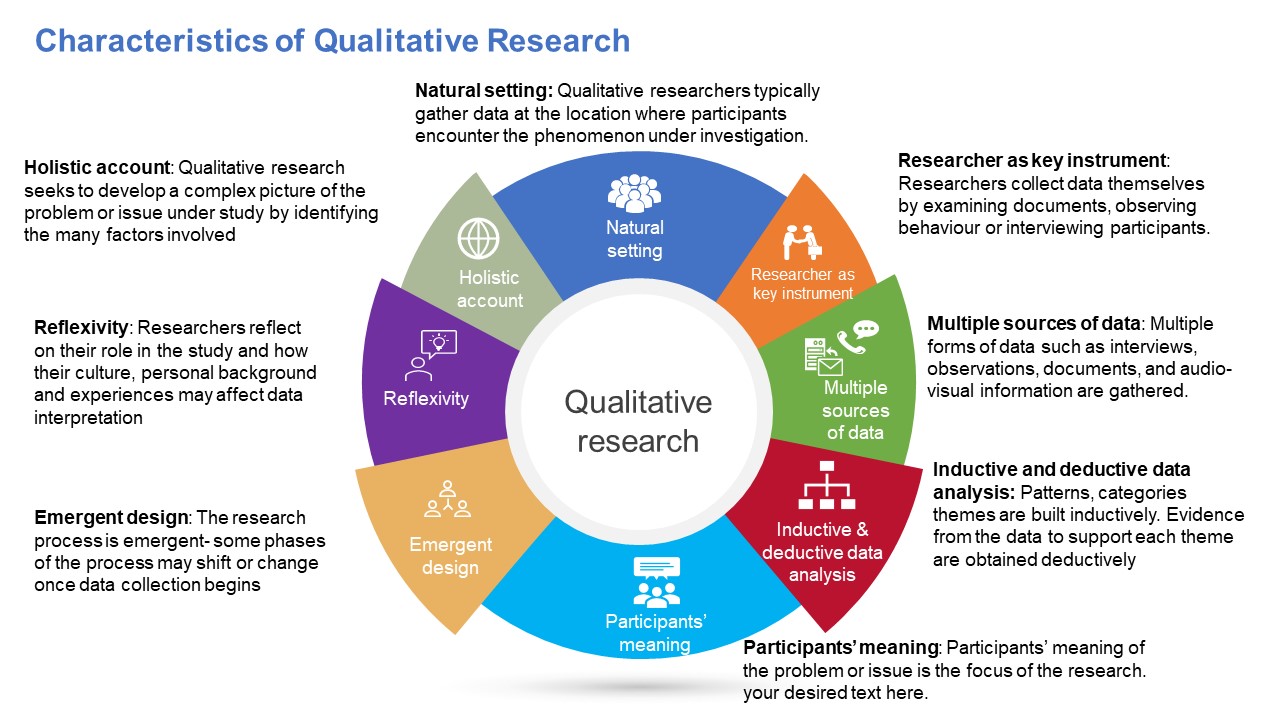
Reflexivity- avoiding bias/Role of the qualitative researcher
Qualitative researchers generally begin their work with the recognition that their position (or worldview) has a significant impact on the overall research process. 7 Researcher worldview shapes the way the research is conducted, i.e., how the questions are formulated, methods are chosen, data are collected and analysed, and results are reported. Therefore, it is essential for qualitative researchers to acknowledge, articulate, reflect on and clarify their own underlying biases and assumptions before embarking on any research project. 7 Reflexivity helps to ensure that the researcher’s own experiences, values, and beliefs do not unintentionally bias the data collection, analysis, and interpretation. 7 It is the gold standard for establishing trustworthiness and has been established as one of the ways qualitative researchers should ensure rigour and quality in their work. 8 The following questions in Table 4.1 may help you begin the reflective process. 9
Table 4.1: Questions to aid the reflection process
What piques my interest in this subject? | You need to consider what motivates your excitement, energy, and interest in investigating this topic to answer this question |
What exactly do I believe the solution is? | Asking this question allows you to detect any biases by honestly reflecting on what you anticipate finding. The assumptions can be grouped/classified to allow the participants’ opinions to be heard. |
What exactly am I getting out of this? | In many circumstances, the “pressure to publish” reduces research to nothing more than a job necessity. What effect does this have on your interest in the subject and its results? To what extent are you willing to go to find information? |
What do my colleagues think of this project—and me? | You will not work in a vacuum as a researcher; you will be part of a social and interpersonal world. These outside factors will impact your perceptions of yourself and your job. Recognising this impact and its possible implications on human behaviour will allow for more self-reflection during the study process. |
Philosophical underpinnings to qualitative research
Qualitative research uses an inductive approach and stems from interpretivism or constructivism and assumes that realities are multiple, socially constructed, and holistic. 10 According to this philosophical viewpoint, humans build reality through their interactions with the world around them. 10 As a result, qualitative research aims to comprehend how individuals make sense of their experiences and build meaning in their lives. 10 Because reality is complex/nuanced and context-bound, participants constantly construct it depending on their understanding. Thus, the interactions between the researcher and the participants are considered necessary to offer a rich description of the concept and provide an in-depth understanding of the phenomenon under investigation. 11
An Introduction to Research Methods for Undergraduate Health Profession Students Copyright © 2023 by Faith Alele and Bunmi Malau-Aduli is licensed under a Creative Commons Attribution-NonCommercial 4.0 International License , except where otherwise noted.
Qualitative Research : Definition
Qualitative research is the naturalistic study of social meanings and processes, using interviews, observations, and the analysis of texts and images. In contrast to quantitative researchers, whose statistical methods enable broad generalizations about populations (for example, comparisons of the percentages of U.S. demographic groups who vote in particular ways), qualitative researchers use in-depth studies of the social world to analyze how and why groups think and act in particular ways (for instance, case studies of the experiences that shape political views).
Events and Workshops
- Introduction to NVivo Have you just collected your data and wondered what to do next? Come join us for an introductory session on utilizing NVivo to support your analytical process. This session will only cover features of the software and how to import your records. Please feel free to attend any of the following sessions below: April 25th, 2024 12:30 pm - 1:45 pm Green Library - SVA Conference Room 125 May 9th, 2024 12:30 pm - 1:45 pm Green Library - SVA Conference Room 125
- Next: Choose an approach >>
- Choose an approach
- Find studies
- Learn methods
- Getting Started
- Get software
- Get data for secondary analysis
- Network with researchers

- Last Updated: Aug 9, 2024 2:09 PM
- URL: https://guides.library.stanford.edu/qualitative_research
Have a language expert improve your writing
Run a free plagiarism check in 10 minutes, automatically generate references for free.
- Knowledge Base
- Methodology
- What Is Qualitative Research? | Methods & Examples
What Is Qualitative Research? | Methods & Examples
Published on 4 April 2022 by Pritha Bhandari . Revised on 30 January 2023.
Qualitative research involves collecting and analysing non-numerical data (e.g., text, video, or audio) to understand concepts, opinions, or experiences. It can be used to gather in-depth insights into a problem or generate new ideas for research.
Qualitative research is the opposite of quantitative research , which involves collecting and analysing numerical data for statistical analysis.
Qualitative research is commonly used in the humanities and social sciences, in subjects such as anthropology, sociology, education, health sciences, and history.
- How does social media shape body image in teenagers?
- How do children and adults interpret healthy eating in the UK?
- What factors influence employee retention in a large organisation?
- How is anxiety experienced around the world?
- How can teachers integrate social issues into science curriculums?
Table of contents
Approaches to qualitative research, qualitative research methods, qualitative data analysis, advantages of qualitative research, disadvantages of qualitative research, frequently asked questions about qualitative research.
Qualitative research is used to understand how people experience the world. While there are many approaches to qualitative research, they tend to be flexible and focus on retaining rich meaning when interpreting data.
Common approaches include grounded theory, ethnography, action research, phenomenological research, and narrative research. They share some similarities, but emphasise different aims and perspectives.
Approach | What does it involve? |
---|---|
Grounded theory | Researchers collect rich data on a topic of interest and develop theories . |
Researchers immerse themselves in groups or organisations to understand their cultures. | |
Researchers and participants collaboratively link theory to practice to drive social change. | |
Phenomenological research | Researchers investigate a phenomenon or event by describing and interpreting participants’ lived experiences. |
Narrative research | Researchers examine how stories are told to understand how participants perceive and make sense of their experiences. |
Prevent plagiarism, run a free check.
Each of the research approaches involve using one or more data collection methods . These are some of the most common qualitative methods:
- Observations: recording what you have seen, heard, or encountered in detailed field notes.
- Interviews: personally asking people questions in one-on-one conversations.
- Focus groups: asking questions and generating discussion among a group of people.
- Surveys : distributing questionnaires with open-ended questions.
- Secondary research: collecting existing data in the form of texts, images, audio or video recordings, etc.
- You take field notes with observations and reflect on your own experiences of the company culture.
- You distribute open-ended surveys to employees across all the company’s offices by email to find out if the culture varies across locations.
- You conduct in-depth interviews with employees in your office to learn about their experiences and perspectives in greater detail.
Qualitative researchers often consider themselves ‘instruments’ in research because all observations, interpretations and analyses are filtered through their own personal lens.
For this reason, when writing up your methodology for qualitative research, it’s important to reflect on your approach and to thoroughly explain the choices you made in collecting and analysing the data.
Qualitative data can take the form of texts, photos, videos and audio. For example, you might be working with interview transcripts, survey responses, fieldnotes, or recordings from natural settings.
Most types of qualitative data analysis share the same five steps:
- Prepare and organise your data. This may mean transcribing interviews or typing up fieldnotes.
- Review and explore your data. Examine the data for patterns or repeated ideas that emerge.
- Develop a data coding system. Based on your initial ideas, establish a set of codes that you can apply to categorise your data.
- Assign codes to the data. For example, in qualitative survey analysis, this may mean going through each participant’s responses and tagging them with codes in a spreadsheet. As you go through your data, you can create new codes to add to your system if necessary.
- Identify recurring themes. Link codes together into cohesive, overarching themes.
There are several specific approaches to analysing qualitative data. Although these methods share similar processes, they emphasise different concepts.
Approach | When to use | Example |
---|---|---|
To describe and categorise common words, phrases, and ideas in qualitative data. | A market researcher could perform content analysis to find out what kind of language is used in descriptions of therapeutic apps. | |
To identify and interpret patterns and themes in qualitative data. | A psychologist could apply thematic analysis to travel blogs to explore how tourism shapes self-identity. | |
To examine the content, structure, and design of texts. | A media researcher could use textual analysis to understand how news coverage of celebrities has changed in the past decade. | |
To study communication and how language is used to achieve effects in specific contexts. | A political scientist could use discourse analysis to study how politicians generate trust in election campaigns. |
Qualitative research often tries to preserve the voice and perspective of participants and can be adjusted as new research questions arise. Qualitative research is good for:
- Flexibility
The data collection and analysis process can be adapted as new ideas or patterns emerge. They are not rigidly decided beforehand.
- Natural settings
Data collection occurs in real-world contexts or in naturalistic ways.
- Meaningful insights
Detailed descriptions of people’s experiences, feelings and perceptions can be used in designing, testing or improving systems or products.
- Generation of new ideas
Open-ended responses mean that researchers can uncover novel problems or opportunities that they wouldn’t have thought of otherwise.
Researchers must consider practical and theoretical limitations in analysing and interpreting their data. Qualitative research suffers from:
- Unreliability
The real-world setting often makes qualitative research unreliable because of uncontrolled factors that affect the data.
- Subjectivity
Due to the researcher’s primary role in analysing and interpreting data, qualitative research cannot be replicated . The researcher decides what is important and what is irrelevant in data analysis, so interpretations of the same data can vary greatly.
- Limited generalisability
Small samples are often used to gather detailed data about specific contexts. Despite rigorous analysis procedures, it is difficult to draw generalisable conclusions because the data may be biased and unrepresentative of the wider population .
- Labour-intensive
Although software can be used to manage and record large amounts of text, data analysis often has to be checked or performed manually.
Quantitative research deals with numbers and statistics, while qualitative research deals with words and meanings.
Quantitative methods allow you to test a hypothesis by systematically collecting and analysing data, while qualitative methods allow you to explore ideas and experiences in depth.
There are five common approaches to qualitative research :
- Grounded theory involves collecting data in order to develop new theories.
- Ethnography involves immersing yourself in a group or organisation to understand its culture.
- Narrative research involves interpreting stories to understand how people make sense of their experiences and perceptions.
- Phenomenological research involves investigating phenomena through people’s lived experiences.
- Action research links theory and practice in several cycles to drive innovative changes.
Data collection is the systematic process by which observations or measurements are gathered in research. It is used in many different contexts by academics, governments, businesses, and other organisations.
There are various approaches to qualitative data analysis , but they all share five steps in common:
- Prepare and organise your data.
- Review and explore your data.
- Develop a data coding system.
- Assign codes to the data.
- Identify recurring themes.
The specifics of each step depend on the focus of the analysis. Some common approaches include textual analysis , thematic analysis , and discourse analysis .
Cite this Scribbr article
If you want to cite this source, you can copy and paste the citation or click the ‘Cite this Scribbr article’ button to automatically add the citation to our free Reference Generator.
Bhandari, P. (2023, January 30). What Is Qualitative Research? | Methods & Examples. Scribbr. Retrieved 2 September 2024, from https://www.scribbr.co.uk/research-methods/introduction-to-qualitative-research/
Is this article helpful?
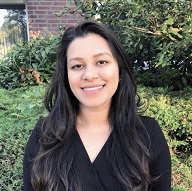
Pritha Bhandari
- Tools and Resources
- Customer Services
- Business Education
- Business Law
- Business Policy and Strategy
- Entrepreneurship
- Human Resource Management
- Information Systems
- International Business
- Negotiations and Bargaining
- Operations Management
- Organization Theory
- Organizational Behavior
- Problem Solving and Creativity
- Research Methods
- Social Issues
- Technology and Innovation Management
- Share This Facebook LinkedIn Twitter
Article contents
Qualitative research: foundations, approaches, and practices.
- Thomas Greckhamer Thomas Greckhamer Rucks Department of Management, Louisiana State University
- and Sebnem Cilesiz Sebnem Cilesiz Department of Educational Foundations and Leadership, University of Louisiana at Lafayette
- https://doi.org/10.1093/acrefore/9780190224851.013.214
- Published online: 23 March 2022
Qualitative research is an umbrella term that is typically used in contrast to quantitative research and captures research approaches that predominantly rely on collecting and analyzing qualitative data (i.e., data in the form of words, still or moving images, and artifacts). Qualitative research encompasses a wide range of research approaches with different philosophical and theoretical foundations and empirical procedures. Different assumptions about reality and knowledge underlying these diverse approaches guide researchers with respect to epistemological and methodological questions and inform their choices regarding research questions, data collection, data analysis, and the writing of research accounts. While at present a few dominant approaches are commonly used by researchers, a rich repertoire of qualitative approaches is available to management researchers that has the potential to facilitate deeper and broader insights into management phenomena.
- qualitative research
- research paradigms
- methodology
- research design
- qualitative approaches
- data collection
- data analysis
You do not currently have access to this article
Please login to access the full content.
Access to the full content requires a subscription
Printed from Oxford Research Encyclopedias, Business and Management. Under the terms of the licence agreement, an individual user may print out a single article for personal use (for details see Privacy Policy and Legal Notice).
date: 03 September 2024
- Cookie Policy
- Privacy Policy
- Legal Notice
- Accessibility
- [81.177.182.136]
- 81.177.182.136
Character limit 500 /500
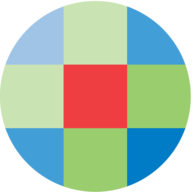
- Get new issue alerts Get alerts
- Submit a Manuscript
Secondary Logo
Journal logo.
Colleague's E-mail is Invalid
Your message has been successfully sent to your colleague.
Save my selection
Introduction to qualitative research methods – Part I
Bhangu, Shagufta; Provost, Fabien; Caduff, Carlo
Department of Global Health and Social Medicine, King's College London, London, United Kingdom
Address for correspondence: Prof. Carlo Caduf, Department of Global Health and Social Medicine, King's College London, Strand, London WC2R 2LS, United Kingdom. E-mail: [email protected]
Received November 28, 2022
Accepted November 29, 2022
Qualitative research methods are widely used in the social sciences and the humanities, but they can also complement quantitative approaches used in clinical research. In this article, we discuss the key features and contributions of qualitative research methods.
INTRODUCTION
Qualitative research methods refer to techniques of investigation that rely on nonstatistical and nonnumerical methods of data collection, analysis, and evidence production. Qualitative research techniques provide a lens for learning about nonquantifiable phenomena such as people's experiences, languages, histories, and cultures. In this article, we describe the strengths and role of qualitative research methods and how these can be employed in clinical research.
Although frequently employed in the social sciences and humanities, qualitative research methods can complement clinical research. These techniques can contribute to a better understanding of the social, cultural, political, and economic dimensions of health and illness. Social scientists and scholars in the humanities rely on a wide range of methods, including interviews, surveys, participant observation, focus groups, oral history, and archival research to examine both structural conditions and lived experience [ Figure 1 ]. Such research can not only provide robust and reliable data but can also humanize and add richness to our understanding of the ways in which people in different parts of the world perceive and experience illness and how they interact with medical institutions, systems, and therapeutics.

Qualitative research methods should not be seen as tools that can be applied independently of theory. It is important for these tools to be based on more than just method. In their research, social scientists and scholars in the humanities emphasize social theory. Departing from a reductionist psychological model of individual behavior that often blames people for their illness, social theory focuses on relations – disease happens not simply in people but between people. This type of theoretically informed and empirically grounded research thus examines not just patients but interactions between a wide range of actors (e.g., patients, family members, friends, neighbors, local politicians, medical practitioners at all levels, and from many systems of medicine, researchers, policymakers) to give voice to the lived experiences, motivations, and constraints of all those who are touched by disease.
PHILOSOPHICAL FOUNDATIONS OF QUALITATIVE RESEARCH METHODS
In identifying the factors that contribute to the occurrence and persistence of a phenomenon, it is paramount that we begin by asking the question: what do we know about this reality? How have we come to know this reality? These two processes, which we can refer to as the “what” question and the “how” question, are the two that all scientists (natural and social) grapple with in their research. We refer to these as the ontological and epistemological questions a research study must address. Together, they help us create a suitable methodology for any research study[ 1 ] [ Figure 2 ]. Therefore, as with quantitative methods, there must be a justifiable and logical method for understanding the world even for qualitative methods. By engaging with these two dimensions, the ontological and the epistemological, we open a path for learning that moves away from commonsensical understandings of the world, and the perpetuation of stereotypes and toward robust scientific knowledge production.

Every discipline has a distinct research philosophy and way of viewing the world and conducting research. Philosophers and historians of science have extensively studied how these divisions and specializations have emerged over centuries.[ 1 2 3 ] The most important distinction between quantitative and qualitative research techniques lies in the nature of the data they study and analyze. While the former focus on statistical, numerical, and quantitative aspects of phenomena and employ the same in data collection and analysis, qualitative techniques focus on humanistic, descriptive, and qualitative aspects of phenomena.[ 4 ]
For the findings of any research study to be reliable, they must employ the appropriate research techniques that are uniquely tailored to the phenomena under investigation. To do so, researchers must choose techniques based on their specific research questions and understand the strengths and limitations of the different tools available to them. Since clinical work lies at the intersection of both natural and social phenomena, it means that it must study both: biological and physiological phenomena (natural, quantitative, and objective phenomena) and behavioral and cultural phenomena (social, qualitative, and subjective phenomena). Therefore, clinical researchers can gain from both sets of techniques in their efforts to produce medical knowledge and bring forth scientifically informed change.
KEY FEATURES AND CONTRIBUTIONS OF QUALITATIVE RESEARCH METHODS
In this section, we discuss the key features and contributions of qualitative research methods [ Figure 3 ]. We describe the specific strengths and limitations of these techniques and discuss how they can be deployed in scientific investigations.

One of the most important contributions of qualitative research methods is that they provide rigorous, theoretically sound, and rational techniques for the analysis of subjective, nebulous, and difficult-to-pin-down phenomena. We are aware, for example, of the role that social factors play in health care but find it hard to qualify and quantify these in our research studies. Often, we find researchers basing their arguments on “common sense,” developing research studies based on assumptions about the people that are studied. Such commonsensical assumptions are perhaps among the greatest impediments to knowledge production. For example, in trying to understand stigma, surveys often make assumptions about its reasons and frequently associate it with vague and general common sense notions of “fear” and “lack of information.” While these may be at work, to make such assumptions based on commonsensical understandings, and without conducting research inhibit us from exploring the multiple social factors that are at work under the guise of stigma.
In unpacking commonsensical understandings and researching experiences, relationships, and other phenomena, qualitative researchers are assisted by their methodological commitment to open-ended research. By open-ended research, we mean that these techniques take on an unbiased and exploratory approach in which learnings from the field and from research participants, are recorded and analyzed to learn about the world.[ 5 ] This orientation is made possible by qualitative research techniques that are particularly effective in learning about specific social, cultural, economic, and political milieus.
Second, qualitative research methods equip us in studying complex phenomena. Qualitative research methods provide scientific tools for exploring and identifying the numerous contributing factors to an occurrence. Rather than establishing one or the other factor as more important, qualitative methods are open-ended, inductive (ground-up), and empirical. They allow us to understand the object of our analysis from multiple vantage points and in its dispersion and caution against predetermined notions of the object of inquiry. They encourage researchers instead to discover a reality that is not yet given, fixed, and predetermined by the methods that are used and the hypotheses that underlie the study.
Once the multiple factors at work in a phenomenon have been identified, we can employ quantitative techniques and embark on processes of measurement, establish patterns and regularities, and analyze the causal and correlated factors at work through statistical techniques. For example, a doctor may observe that there is a high patient drop-out in treatment. Before carrying out a study which relies on quantitative techniques, qualitative research methods such as conversation analysis, interviews, surveys, or even focus group discussions may prove more effective in learning about all the factors that are contributing to patient default. After identifying the multiple, intersecting factors, quantitative techniques can be deployed to measure each of these factors through techniques such as correlational or regression analyses. Here, the use of quantitative techniques without identifying the diverse factors influencing patient decisions would be premature. Qualitative techniques thus have a key role to play in investigations of complex realities and in conducting rich exploratory studies while embracing rigorous and philosophically grounded methodologies.
Third, apart from subjective, nebulous, and complex phenomena, qualitative research techniques are also effective in making sense of irrational, illogical, and emotional phenomena. These play an important role in understanding logics at work among patients, their families, and societies. Qualitative research techniques are aided by their ability to shift focus away from the individual as a unit of analysis to the larger social, cultural, political, economic, and structural forces at work in health. As health-care practitioners and researchers focused on biological, physiological, disease and therapeutic processes, sociocultural, political, and economic conditions are often peripheral or ignored in day-to-day clinical work. However, it is within these latter processes that both health-care practices and patient lives are entrenched. Qualitative researchers are particularly adept at identifying the structural conditions such as the social, cultural, political, local, and economic conditions which contribute to health care and experiences of disease and illness.
For example, the decision to delay treatment by a patient may be understood as an irrational choice impacting his/her chances of survival, but the same may be a result of the patient treating their child's education as a financial priority over his/her own health. While this appears as an “emotional” choice, qualitative researchers try to understand the social and cultural factors that structure, inform, and justify such choices. Rather than assuming that it is an irrational choice, qualitative researchers try to understand the norms and logical grounds on which the patient is making this decision. By foregrounding such logics, stories, fears, and desires, qualitative research expands our analytic precision in learning about complex social worlds, recognizing reasons for medical successes and failures, and interrogating our assumptions about human behavior. These in turn can prove useful in arriving at conclusive, actionable findings which can inform institutional and public health policies and have a very important role to play in any change and transformation we may wish to bring to the societies in which we work.
Financial support and sponsorship
Conflicts of interest.
There are no conflicts of interest.
Qualitative research; social sciences; sociology
- + Favorites
- View in Gallery
Readers Of this Article Also Read
Study designs: part 2 – descriptive studies, designing and validating a research questionnaire - part 1, study designs: part 1 – an overview and classification, the changing perspective of clinical trial designs, advice to authors for avoiding flaws in preparation of original research....
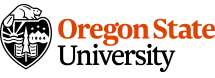
Chapter 1. Introduction
“Science is in danger, and for that reason it is becoming dangerous” -Pierre Bourdieu, Science of Science and Reflexivity
Why an Open Access Textbook on Qualitative Research Methods?
I have been teaching qualitative research methods to both undergraduates and graduate students for many years. Although there are some excellent textbooks out there, they are often costly, and none of them, to my mind, properly introduces qualitative research methods to the beginning student (whether undergraduate or graduate student). In contrast, this open-access textbook is designed as a (free) true introduction to the subject, with helpful, practical pointers on how to conduct research and how to access more advanced instruction.
Textbooks are typically arranged in one of two ways: (1) by technique (each chapter covers one method used in qualitative research); or (2) by process (chapters advance from research design through publication). But both of these approaches are necessary for the beginner student. This textbook will have sections dedicated to the process as well as the techniques of qualitative research. This is a true “comprehensive” book for the beginning student. In addition to covering techniques of data collection and data analysis, it provides a road map of how to get started and how to keep going and where to go for advanced instruction. It covers aspects of research design and research communication as well as methods employed. Along the way, it includes examples from many different disciplines in the social sciences.
The primary goal has been to create a useful, accessible, engaging textbook for use across many disciplines. And, let’s face it. Textbooks can be boring. I hope readers find this to be a little different. I have tried to write in a practical and forthright manner, with many lively examples and references to good and intellectually creative qualitative research. Woven throughout the text are short textual asides (in colored textboxes) by professional (academic) qualitative researchers in various disciplines. These short accounts by practitioners should help inspire students. So, let’s begin!
What is Research?
When we use the word research , what exactly do we mean by that? This is one of those words that everyone thinks they understand, but it is worth beginning this textbook with a short explanation. We use the term to refer to “empirical research,” which is actually a historically specific approach to understanding the world around us. Think about how you know things about the world. [1] You might know your mother loves you because she’s told you she does. Or because that is what “mothers” do by tradition. Or you might know because you’ve looked for evidence that she does, like taking care of you when you are sick or reading to you in bed or working two jobs so you can have the things you need to do OK in life. Maybe it seems churlish to look for evidence; you just take it “on faith” that you are loved.
Only one of the above comes close to what we mean by research. Empirical research is research (investigation) based on evidence. Conclusions can then be drawn from observable data. This observable data can also be “tested” or checked. If the data cannot be tested, that is a good indication that we are not doing research. Note that we can never “prove” conclusively, through observable data, that our mothers love us. We might have some “disconfirming evidence” (that time she didn’t show up to your graduation, for example) that could push you to question an original hypothesis , but no amount of “confirming evidence” will ever allow us to say with 100% certainty, “my mother loves me.” Faith and tradition and authority work differently. Our knowledge can be 100% certain using each of those alternative methods of knowledge, but our certainty in those cases will not be based on facts or evidence.
For many periods of history, those in power have been nervous about “science” because it uses evidence and facts as the primary source of understanding the world, and facts can be at odds with what power or authority or tradition want you to believe. That is why I say that scientific empirical research is a historically specific approach to understand the world. You are in college or university now partly to learn how to engage in this historically specific approach.
In the sixteenth and seventeenth centuries in Europe, there was a newfound respect for empirical research, some of which was seriously challenging to the established church. Using observations and testing them, scientists found that the earth was not at the center of the universe, for example, but rather that it was but one planet of many which circled the sun. [2] For the next two centuries, the science of astronomy, physics, biology, and chemistry emerged and became disciplines taught in universities. All used the scientific method of observation and testing to advance knowledge. Knowledge about people , however, and social institutions, however, was still left to faith, tradition, and authority. Historians and philosophers and poets wrote about the human condition, but none of them used research to do so. [3]
It was not until the nineteenth century that “social science” really emerged, using the scientific method (empirical observation) to understand people and social institutions. New fields of sociology, economics, political science, and anthropology emerged. The first sociologists, people like Auguste Comte and Karl Marx, sought specifically to apply the scientific method of research to understand society, Engels famously claiming that Marx had done for the social world what Darwin did for the natural world, tracings its laws of development. Today we tend to take for granted the naturalness of science here, but it is actually a pretty recent and radical development.
To return to the question, “does your mother love you?” Well, this is actually not really how a researcher would frame the question, as it is too specific to your case. It doesn’t tell us much about the world at large, even if it does tell us something about you and your relationship with your mother. A social science researcher might ask, “do mothers love their children?” Or maybe they would be more interested in how this loving relationship might change over time (e.g., “do mothers love their children more now than they did in the 18th century when so many children died before reaching adulthood?”) or perhaps they might be interested in measuring quality of love across cultures or time periods, or even establishing “what love looks like” using the mother/child relationship as a site of exploration. All of these make good research questions because we can use observable data to answer them.
What is Qualitative Research?
“All we know is how to learn. How to study, how to listen, how to talk, how to tell. If we don’t tell the world, we don’t know the world. We’re lost in it, we die.” -Ursula LeGuin, The Telling
At its simplest, qualitative research is research about the social world that does not use numbers in its analyses. All those who fear statistics can breathe a sigh of relief – there are no mathematical formulae or regression models in this book! But this definition is less about what qualitative research can be and more about what it is not. To be honest, any simple statement will fail to capture the power and depth of qualitative research. One way of contrasting qualitative research to quantitative research is to note that the focus of qualitative research is less about explaining and predicting relationships between variables and more about understanding the social world. To use our mother love example, the question about “what love looks like” is a good question for the qualitative researcher while all questions measuring love or comparing incidences of love (both of which require measurement) are good questions for quantitative researchers. Patton writes,
Qualitative data describe. They take us, as readers, into the time and place of the observation so that we know what it was like to have been there. They capture and communicate someone else’s experience of the world in his or her own words. Qualitative data tell a story. ( Patton 2002:47 )
Qualitative researchers are asking different questions about the world than their quantitative colleagues. Even when researchers are employed in “mixed methods” research ( both quantitative and qualitative), they are using different methods to address different questions of the study. I do a lot of research about first-generation and working-college college students. Where a quantitative researcher might ask, how many first-generation college students graduate from college within four years? Or does first-generation college status predict high student debt loads? A qualitative researcher might ask, how does the college experience differ for first-generation college students? What is it like to carry a lot of debt, and how does this impact the ability to complete college on time? Both sets of questions are important, but they can only be answered using specific tools tailored to those questions. For the former, you need large numbers to make adequate comparisons. For the latter, you need to talk to people, find out what they are thinking and feeling, and try to inhabit their shoes for a little while so you can make sense of their experiences and beliefs.
Examples of Qualitative Research
You have probably seen examples of qualitative research before, but you might not have paid particular attention to how they were produced or realized that the accounts you were reading were the result of hours, months, even years of research “in the field.” A good qualitative researcher will present the product of their hours of work in such a way that it seems natural, even obvious, to the reader. Because we are trying to convey what it is like answers, qualitative research is often presented as stories – stories about how people live their lives, go to work, raise their children, interact with one another. In some ways, this can seem like reading particularly insightful novels. But, unlike novels, there are very specific rules and guidelines that qualitative researchers follow to ensure that the “story” they are telling is accurate , a truthful rendition of what life is like for the people being studied. Most of this textbook will be spent conveying those rules and guidelines. Let’s take a look, first, however, at three examples of what the end product looks like. I have chosen these three examples to showcase very different approaches to qualitative research, and I will return to these five examples throughout the book. They were all published as whole books (not chapters or articles), and they are worth the long read, if you have the time. I will also provide some information on how these books came to be and the length of time it takes to get them into book version. It is important you know about this process, and the rest of this textbook will help explain why it takes so long to conduct good qualitative research!
Example 1 : The End Game (ethnography + interviews)
Corey Abramson is a sociologist who teaches at the University of Arizona. In 2015 he published The End Game: How Inequality Shapes our Final Years ( 2015 ). This book was based on the research he did for his dissertation at the University of California-Berkeley in 2012. Actually, the dissertation was completed in 2012 but the work that was produced that took several years. The dissertation was entitled, “This is How We Live, This is How We Die: Social Stratification, Aging, and Health in Urban America” ( 2012 ). You can see how the book version, which was written for a more general audience, has a more engaging sound to it, but that the dissertation version, which is what academic faculty read and evaluate, has a more descriptive title. You can read the title and know that this is a study about aging and health and that the focus is going to be inequality and that the context (place) is going to be “urban America.” It’s a study about “how” people do something – in this case, how they deal with aging and death. This is the very first sentence of the dissertation, “From our first breath in the hospital to the day we die, we live in a society characterized by unequal opportunities for maintaining health and taking care of ourselves when ill. These disparities reflect persistent racial, socio-economic, and gender-based inequalities and contribute to their persistence over time” ( 1 ). What follows is a truthful account of how that is so.
Cory Abramson spent three years conducting his research in four different urban neighborhoods. We call the type of research he conducted “comparative ethnographic” because he designed his study to compare groups of seniors as they went about their everyday business. It’s comparative because he is comparing different groups (based on race, class, gender) and ethnographic because he is studying the culture/way of life of a group. [4] He had an educated guess, rooted in what previous research had shown and what social theory would suggest, that people’s experiences of aging differ by race, class, and gender. So, he set up a research design that would allow him to observe differences. He chose two primarily middle-class (one was racially diverse and the other was predominantly White) and two primarily poor neighborhoods (one was racially diverse and the other was predominantly African American). He hung out in senior centers and other places seniors congregated, watched them as they took the bus to get prescriptions filled, sat in doctor’s offices with them, and listened to their conversations with each other. He also conducted more formal conversations, what we call in-depth interviews, with sixty seniors from each of the four neighborhoods. As with a lot of fieldwork , as he got closer to the people involved, he both expanded and deepened his reach –
By the end of the project, I expanded my pool of general observations to include various settings frequented by seniors: apartment building common rooms, doctors’ offices, emergency rooms, pharmacies, senior centers, bars, parks, corner stores, shopping centers, pool halls, hair salons, coffee shops, and discount stores. Over the course of the three years of fieldwork, I observed hundreds of elders, and developed close relationships with a number of them. ( 2012:10 )
When Abramson rewrote the dissertation for a general audience and published his book in 2015, it got a lot of attention. It is a beautifully written book and it provided insight into a common human experience that we surprisingly know very little about. It won the Outstanding Publication Award by the American Sociological Association Section on Aging and the Life Course and was featured in the New York Times . The book was about aging, and specifically how inequality shapes the aging process, but it was also about much more than that. It helped show how inequality affects people’s everyday lives. For example, by observing the difficulties the poor had in setting up appointments and getting to them using public transportation and then being made to wait to see a doctor, sometimes in standing-room-only situations, when they are unwell, and then being treated dismissively by hospital staff, Abramson allowed readers to feel the material reality of being poor in the US. Comparing these examples with seniors with adequate supplemental insurance who have the resources to hire car services or have others assist them in arranging care when they need it, jolts the reader to understand and appreciate the difference money makes in the lives and circumstances of us all, and in a way that is different than simply reading a statistic (“80% of the poor do not keep regular doctor’s appointments”) does. Qualitative research can reach into spaces and places that often go unexamined and then reports back to the rest of us what it is like in those spaces and places.
Example 2: Racing for Innocence (Interviews + Content Analysis + Fictional Stories)
Jennifer Pierce is a Professor of American Studies at the University of Minnesota. Trained as a sociologist, she has written a number of books about gender, race, and power. Her very first book, Gender Trials: Emotional Lives in Contemporary Law Firms, published in 1995, is a brilliant look at gender dynamics within two law firms. Pierce was a participant observer, working as a paralegal, and she observed how female lawyers and female paralegals struggled to obtain parity with their male colleagues.
Fifteen years later, she reexamined the context of the law firm to include an examination of racial dynamics, particularly how elite white men working in these spaces created and maintained a culture that made it difficult for both female attorneys and attorneys of color to thrive. Her book, Racing for Innocence: Whiteness, Gender, and the Backlash Against Affirmative Action , published in 2012, is an interesting and creative blending of interviews with attorneys, content analyses of popular films during this period, and fictional accounts of racial discrimination and sexual harassment. The law firm she chose to study had come under an affirmative action order and was in the process of implementing equitable policies and programs. She wanted to understand how recipients of white privilege (the elite white male attorneys) come to deny the role they play in reproducing inequality. Through interviews with attorneys who were present both before and during the affirmative action order, she creates a historical record of the “bad behavior” that necessitated new policies and procedures, but also, and more importantly , probed the participants ’ understanding of this behavior. It should come as no surprise that most (but not all) of the white male attorneys saw little need for change, and that almost everyone else had accounts that were different if not sometimes downright harrowing.
I’ve used Pierce’s book in my qualitative research methods courses as an example of an interesting blend of techniques and presentation styles. My students often have a very difficult time with the fictional accounts she includes. But they serve an important communicative purpose here. They are her attempts at presenting “both sides” to an objective reality – something happens (Pierce writes this something so it is very clear what it is), and the two participants to the thing that happened have very different understandings of what this means. By including these stories, Pierce presents one of her key findings – people remember things differently and these different memories tend to support their own ideological positions. I wonder what Pierce would have written had she studied the murder of George Floyd or the storming of the US Capitol on January 6 or any number of other historic events whose observers and participants record very different happenings.
This is not to say that qualitative researchers write fictional accounts. In fact, the use of fiction in our work remains controversial. When used, it must be clearly identified as a presentation device, as Pierce did. I include Racing for Innocence here as an example of the multiple uses of methods and techniques and the way that these work together to produce better understandings by us, the readers, of what Pierce studied. We readers come away with a better grasp of how and why advantaged people understate their own involvement in situations and structures that advantage them. This is normal human behavior , in other words. This case may have been about elite white men in law firms, but the general insights here can be transposed to other settings. Indeed, Pierce argues that more research needs to be done about the role elites play in the reproduction of inequality in the workplace in general.
Example 3: Amplified Advantage (Mixed Methods: Survey Interviews + Focus Groups + Archives)
The final example comes from my own work with college students, particularly the ways in which class background affects the experience of college and outcomes for graduates. I include it here as an example of mixed methods, and for the use of supplementary archival research. I’ve done a lot of research over the years on first-generation, low-income, and working-class college students. I am curious (and skeptical) about the possibility of social mobility today, particularly with the rising cost of college and growing inequality in general. As one of the few people in my family to go to college, I didn’t grow up with a lot of examples of what college was like or how to make the most of it. And when I entered graduate school, I realized with dismay that there were very few people like me there. I worried about becoming too different from my family and friends back home. And I wasn’t at all sure that I would ever be able to pay back the huge load of debt I was taking on. And so I wrote my dissertation and first two books about working-class college students. These books focused on experiences in college and the difficulties of navigating between family and school ( Hurst 2010a, 2012 ). But even after all that research, I kept coming back to wondering if working-class students who made it through college had an equal chance at finding good jobs and happy lives,
What happens to students after college? Do working-class students fare as well as their peers? I knew from my own experience that barriers continued through graduate school and beyond, and that my debtload was higher than that of my peers, constraining some of the choices I made when I graduated. To answer these questions, I designed a study of students attending small liberal arts colleges, the type of college that tried to equalize the experience of students by requiring all students to live on campus and offering small classes with lots of interaction with faculty. These private colleges tend to have more money and resources so they can provide financial aid to low-income students. They also attract some very wealthy students. Because they enroll students across the class spectrum, I would be able to draw comparisons. I ended up spending about four years collecting data, both a survey of more than 2000 students (which formed the basis for quantitative analyses) and qualitative data collection (interviews, focus groups, archival research, and participant observation). This is what we call a “mixed methods” approach because we use both quantitative and qualitative data. The survey gave me a large enough number of students that I could make comparisons of the how many kind, and to be able to say with some authority that there were in fact significant differences in experience and outcome by class (e.g., wealthier students earned more money and had little debt; working-class students often found jobs that were not in their chosen careers and were very affected by debt, upper-middle-class students were more likely to go to graduate school). But the survey analyses could not explain why these differences existed. For that, I needed to talk to people and ask them about their motivations and aspirations. I needed to understand their perceptions of the world, and it is very hard to do this through a survey.
By interviewing students and recent graduates, I was able to discern particular patterns and pathways through college and beyond. Specifically, I identified three versions of gameplay. Upper-middle-class students, whose parents were themselves professionals (academics, lawyers, managers of non-profits), saw college as the first stage of their education and took classes and declared majors that would prepare them for graduate school. They also spent a lot of time building their resumes, taking advantage of opportunities to help professors with their research, or study abroad. This helped them gain admission to highly-ranked graduate schools and interesting jobs in the public sector. In contrast, upper-class students, whose parents were wealthy and more likely to be engaged in business (as CEOs or other high-level directors), prioritized building social capital. They did this by joining fraternities and sororities and playing club sports. This helped them when they graduated as they called on friends and parents of friends to find them well-paying jobs. Finally, low-income, first-generation, and working-class students were often adrift. They took the classes that were recommended to them but without the knowledge of how to connect them to life beyond college. They spent time working and studying rather than partying or building their resumes. All three sets of students thought they were “doing college” the right way, the way that one was supposed to do college. But these three versions of gameplay led to distinct outcomes that advantaged some students over others. I titled my work “Amplified Advantage” to highlight this process.
These three examples, Cory Abramson’s The End Game , Jennifer Peirce’s Racing for Innocence, and my own Amplified Advantage, demonstrate the range of approaches and tools available to the qualitative researcher. They also help explain why qualitative research is so important. Numbers can tell us some things about the world, but they cannot get at the hearts and minds, motivations and beliefs of the people who make up the social worlds we inhabit. For that, we need tools that allow us to listen and make sense of what people tell us and show us. That is what good qualitative research offers us.
How Is This Book Organized?
This textbook is organized as a comprehensive introduction to the use of qualitative research methods. The first half covers general topics (e.g., approaches to qualitative research, ethics) and research design (necessary steps for building a successful qualitative research study). The second half reviews various data collection and data analysis techniques. Of course, building a successful qualitative research study requires some knowledge of data collection and data analysis so the chapters in the first half and the chapters in the second half should be read in conversation with each other. That said, each chapter can be read on its own for assistance with a particular narrow topic. In addition to the chapters, a helpful glossary can be found in the back of the book. Rummage around in the text as needed.
Chapter Descriptions
Chapter 2 provides an overview of the Research Design Process. How does one begin a study? What is an appropriate research question? How is the study to be done – with what methods ? Involving what people and sites? Although qualitative research studies can and often do change and develop over the course of data collection, it is important to have a good idea of what the aims and goals of your study are at the outset and a good plan of how to achieve those aims and goals. Chapter 2 provides a road map of the process.
Chapter 3 describes and explains various ways of knowing the (social) world. What is it possible for us to know about how other people think or why they behave the way they do? What does it mean to say something is a “fact” or that it is “well-known” and understood? Qualitative researchers are particularly interested in these questions because of the types of research questions we are interested in answering (the how questions rather than the how many questions of quantitative research). Qualitative researchers have adopted various epistemological approaches. Chapter 3 will explore these approaches, highlighting interpretivist approaches that acknowledge the subjective aspect of reality – in other words, reality and knowledge are not objective but rather influenced by (interpreted through) people.
Chapter 4 focuses on the practical matter of developing a research question and finding the right approach to data collection. In any given study (think of Cory Abramson’s study of aging, for example), there may be years of collected data, thousands of observations , hundreds of pages of notes to read and review and make sense of. If all you had was a general interest area (“aging”), it would be very difficult, nearly impossible, to make sense of all of that data. The research question provides a helpful lens to refine and clarify (and simplify) everything you find and collect. For that reason, it is important to pull out that lens (articulate the research question) before you get started. In the case of the aging study, Cory Abramson was interested in how inequalities affected understandings and responses to aging. It is for this reason he designed a study that would allow him to compare different groups of seniors (some middle-class, some poor). Inevitably, he saw much more in the three years in the field than what made it into his book (or dissertation), but he was able to narrow down the complexity of the social world to provide us with this rich account linked to the original research question. Developing a good research question is thus crucial to effective design and a successful outcome. Chapter 4 will provide pointers on how to do this. Chapter 4 also provides an overview of general approaches taken to doing qualitative research and various “traditions of inquiry.”
Chapter 5 explores sampling . After you have developed a research question and have a general idea of how you will collect data (Observations? Interviews?), how do you go about actually finding people and sites to study? Although there is no “correct number” of people to interview , the sample should follow the research question and research design. Unlike quantitative research, qualitative research involves nonprobability sampling. Chapter 5 explains why this is so and what qualities instead make a good sample for qualitative research.
Chapter 6 addresses the importance of reflexivity in qualitative research. Related to epistemological issues of how we know anything about the social world, qualitative researchers understand that we the researchers can never be truly neutral or outside the study we are conducting. As observers, we see things that make sense to us and may entirely miss what is either too obvious to note or too different to comprehend. As interviewers, as much as we would like to ask questions neutrally and remain in the background, interviews are a form of conversation, and the persons we interview are responding to us . Therefore, it is important to reflect upon our social positions and the knowledges and expectations we bring to our work and to work through any blind spots that we may have. Chapter 6 provides some examples of reflexivity in practice and exercises for thinking through one’s own biases.
Chapter 7 is a very important chapter and should not be overlooked. As a practical matter, it should also be read closely with chapters 6 and 8. Because qualitative researchers deal with people and the social world, it is imperative they develop and adhere to a strong ethical code for conducting research in a way that does not harm. There are legal requirements and guidelines for doing so (see chapter 8), but these requirements should not be considered synonymous with the ethical code required of us. Each researcher must constantly interrogate every aspect of their research, from research question to design to sample through analysis and presentation, to ensure that a minimum of harm (ideally, zero harm) is caused. Because each research project is unique, the standards of care for each study are unique. Part of being a professional researcher is carrying this code in one’s heart, being constantly attentive to what is required under particular circumstances. Chapter 7 provides various research scenarios and asks readers to weigh in on the suitability and appropriateness of the research. If done in a class setting, it will become obvious fairly quickly that there are often no absolutely correct answers, as different people find different aspects of the scenarios of greatest importance. Minimizing the harm in one area may require possible harm in another. Being attentive to all the ethical aspects of one’s research and making the best judgments one can, clearly and consciously, is an integral part of being a good researcher.
Chapter 8 , best to be read in conjunction with chapter 7, explains the role and importance of Institutional Review Boards (IRBs) . Under federal guidelines, an IRB is an appropriately constituted group that has been formally designated to review and monitor research involving human subjects . Every institution that receives funding from the federal government has an IRB. IRBs have the authority to approve, require modifications to (to secure approval), or disapprove research. This group review serves an important role in the protection of the rights and welfare of human research subjects. Chapter 8 reviews the history of IRBs and the work they do but also argues that IRBs’ review of qualitative research is often both over-inclusive and under-inclusive. Some aspects of qualitative research are not well understood by IRBs, given that they were developed to prevent abuses in biomedical research. Thus, it is important not to rely on IRBs to identify all the potential ethical issues that emerge in our research (see chapter 7).
Chapter 9 provides help for getting started on formulating a research question based on gaps in the pre-existing literature. Research is conducted as part of a community, even if particular studies are done by single individuals (or small teams). What any of us finds and reports back becomes part of a much larger body of knowledge. Thus, it is important that we look at the larger body of knowledge before we actually start our bit to see how we can best contribute. When I first began interviewing working-class college students, there was only one other similar study I could find, and it hadn’t been published (it was a dissertation of students from poor backgrounds). But there had been a lot published by professors who had grown up working class and made it through college despite the odds. These accounts by “working-class academics” became an important inspiration for my study and helped me frame the questions I asked the students I interviewed. Chapter 9 will provide some pointers on how to search for relevant literature and how to use this to refine your research question.
Chapter 10 serves as a bridge between the two parts of the textbook, by introducing techniques of data collection. Qualitative research is often characterized by the form of data collection – for example, an ethnographic study is one that employs primarily observational data collection for the purpose of documenting and presenting a particular culture or ethnos. Techniques can be effectively combined, depending on the research question and the aims and goals of the study. Chapter 10 provides a general overview of all the various techniques and how they can be combined.
The second part of the textbook moves into the doing part of qualitative research once the research question has been articulated and the study designed. Chapters 11 through 17 cover various data collection techniques and approaches. Chapters 18 and 19 provide a very simple overview of basic data analysis. Chapter 20 covers communication of the data to various audiences, and in various formats.
Chapter 11 begins our overview of data collection techniques with a focus on interviewing , the true heart of qualitative research. This technique can serve as the primary and exclusive form of data collection, or it can be used to supplement other forms (observation, archival). An interview is distinct from a survey, where questions are asked in a specific order and often with a range of predetermined responses available. Interviews can be conversational and unstructured or, more conventionally, semistructured , where a general set of interview questions “guides” the conversation. Chapter 11 covers the basics of interviews: how to create interview guides, how many people to interview, where to conduct the interview, what to watch out for (how to prepare against things going wrong), and how to get the most out of your interviews.
Chapter 12 covers an important variant of interviewing, the focus group. Focus groups are semistructured interviews with a group of people moderated by a facilitator (the researcher or researcher’s assistant). Focus groups explicitly use group interaction to assist in the data collection. They are best used to collect data on a specific topic that is non-personal and shared among the group. For example, asking a group of college students about a common experience such as taking classes by remote delivery during the pandemic year of 2020. Chapter 12 covers the basics of focus groups: when to use them, how to create interview guides for them, and how to run them effectively.
Chapter 13 moves away from interviewing to the second major form of data collection unique to qualitative researchers – observation . Qualitative research that employs observation can best be understood as falling on a continuum of “fly on the wall” observation (e.g., observing how strangers interact in a doctor’s waiting room) to “participant” observation, where the researcher is also an active participant of the activity being observed. For example, an activist in the Black Lives Matter movement might want to study the movement, using her inside position to gain access to observe key meetings and interactions. Chapter 13 covers the basics of participant observation studies: advantages and disadvantages, gaining access, ethical concerns related to insider/outsider status and entanglement, and recording techniques.
Chapter 14 takes a closer look at “deep ethnography” – immersion in the field of a particularly long duration for the purpose of gaining a deeper understanding and appreciation of a particular culture or social world. Clifford Geertz called this “deep hanging out.” Whereas participant observation is often combined with semistructured interview techniques, deep ethnography’s commitment to “living the life” or experiencing the situation as it really is demands more conversational and natural interactions with people. These interactions and conversations may take place over months or even years. As can be expected, there are some costs to this technique, as well as some very large rewards when done competently. Chapter 14 provides some examples of deep ethnographies that will inspire some beginning researchers and intimidate others.
Chapter 15 moves in the opposite direction of deep ethnography, a technique that is the least positivist of all those discussed here, to mixed methods , a set of techniques that is arguably the most positivist . A mixed methods approach combines both qualitative data collection and quantitative data collection, commonly by combining a survey that is analyzed statistically (e.g., cross-tabs or regression analyses of large number probability samples) with semi-structured interviews. Although it is somewhat unconventional to discuss mixed methods in textbooks on qualitative research, I think it is important to recognize this often-employed approach here. There are several advantages and some disadvantages to taking this route. Chapter 16 will describe those advantages and disadvantages and provide some particular guidance on how to design a mixed methods study for maximum effectiveness.
Chapter 16 covers data collection that does not involve live human subjects at all – archival and historical research (chapter 17 will also cover data that does not involve interacting with human subjects). Sometimes people are unavailable to us, either because they do not wish to be interviewed or observed (as is the case with many “elites”) or because they are too far away, in both place and time. Fortunately, humans leave many traces and we can often answer questions we have by examining those traces. Special collections and archives can be goldmines for social science research. This chapter will explain how to access these places, for what purposes, and how to begin to make sense of what you find.
Chapter 17 covers another data collection area that does not involve face-to-face interaction with humans: content analysis . Although content analysis may be understood more properly as a data analysis technique, the term is often used for the entire approach, which will be the case here. Content analysis involves interpreting meaning from a body of text. This body of text might be something found in historical records (see chapter 16) or something collected by the researcher, as in the case of comment posts on a popular blog post. I once used the stories told by student loan debtors on the website studentloanjustice.org as the content I analyzed. Content analysis is particularly useful when attempting to define and understand prevalent stories or communication about a topic of interest. In other words, when we are less interested in what particular people (our defined sample) are doing or believing and more interested in what general narratives exist about a particular topic or issue. This chapter will explore different approaches to content analysis and provide helpful tips on how to collect data, how to turn that data into codes for analysis, and how to go about presenting what is found through analysis.
Where chapter 17 has pushed us towards data analysis, chapters 18 and 19 are all about what to do with the data collected, whether that data be in the form of interview transcripts or fieldnotes from observations. Chapter 18 introduces the basics of coding , the iterative process of assigning meaning to the data in order to both simplify and identify patterns. What is a code and how does it work? What are the different ways of coding data, and when should you use them? What is a codebook, and why do you need one? What does the process of data analysis look like?
Chapter 19 goes further into detail on codes and how to use them, particularly the later stages of coding in which our codes are refined, simplified, combined, and organized. These later rounds of coding are essential to getting the most out of the data we’ve collected. As students are often overwhelmed with the amount of data (a corpus of interview transcripts typically runs into the hundreds of pages; fieldnotes can easily top that), this chapter will also address time management and provide suggestions for dealing with chaos and reminders that feeling overwhelmed at the analysis stage is part of the process. By the end of the chapter, you should understand how “findings” are actually found.
The book concludes with a chapter dedicated to the effective presentation of data results. Chapter 20 covers the many ways that researchers communicate their studies to various audiences (academic, personal, political), what elements must be included in these various publications, and the hallmarks of excellent qualitative research that various audiences will be expecting. Because qualitative researchers are motivated by understanding and conveying meaning , effective communication is not only an essential skill but a fundamental facet of the entire research project. Ethnographers must be able to convey a certain sense of verisimilitude , the appearance of true reality. Those employing interviews must faithfully depict the key meanings of the people they interviewed in a way that rings true to those people, even if the end result surprises them. And all researchers must strive for clarity in their publications so that various audiences can understand what was found and why it is important.
The book concludes with a short chapter ( chapter 21 ) discussing the value of qualitative research. At the very end of this book, you will find a glossary of terms. I recommend you make frequent use of the glossary and add to each entry as you find examples. Although the entries are meant to be simple and clear, you may also want to paraphrase the definition—make it “make sense” to you, in other words. In addition to the standard reference list (all works cited here), you will find various recommendations for further reading at the end of many chapters. Some of these recommendations will be examples of excellent qualitative research, indicated with an asterisk (*) at the end of the entry. As they say, a picture is worth a thousand words. A good example of qualitative research can teach you more about conducting research than any textbook can (this one included). I highly recommend you select one to three examples from these lists and read them along with the textbook.
A final note on the choice of examples – you will note that many of the examples used in the text come from research on college students. This is for two reasons. First, as most of my research falls in this area, I am most familiar with this literature and have contacts with those who do research here and can call upon them to share their stories with you. Second, and more importantly, my hope is that this textbook reaches a wide audience of beginning researchers who study widely and deeply across the range of what can be known about the social world (from marine resources management to public policy to nursing to political science to sexuality studies and beyond). It is sometimes difficult to find examples that speak to all those research interests, however. A focus on college students is something that all readers can understand and, hopefully, appreciate, as we are all now or have been at some point a college student.
Recommended Reading: Other Qualitative Research Textbooks
I’ve included a brief list of some of my favorite qualitative research textbooks and guidebooks if you need more than what you will find in this introductory text. For each, I’ve also indicated if these are for “beginning” or “advanced” (graduate-level) readers. Many of these books have several editions that do not significantly vary; the edition recommended is merely the edition I have used in teaching and to whose page numbers any specific references made in the text agree.
Barbour, Rosaline. 2014. Introducing Qualitative Research: A Student’s Guide. Thousand Oaks, CA: SAGE. A good introduction to qualitative research, with abundant examples (often from the discipline of health care) and clear definitions. Includes quick summaries at the ends of each chapter. However, some US students might find the British context distracting and can be a bit advanced in some places. Beginning .
Bloomberg, Linda Dale, and Marie F. Volpe. 2012. Completing Your Qualitative Dissertation . 2nd ed. Thousand Oaks, CA: SAGE. Specifically designed to guide graduate students through the research process. Advanced .
Creswell, John W., and Cheryl Poth. 2018 Qualitative Inquiry and Research Design: Choosing among Five Traditions . 4th ed. Thousand Oaks, CA: SAGE. This is a classic and one of the go-to books I used myself as a graduate student. One of the best things about this text is its clear presentation of five distinct traditions in qualitative research. Despite the title, this reasonably sized book is about more than research design, including both data analysis and how to write about qualitative research. Advanced .
Lareau, Annette. 2021. Listening to People: A Practical Guide to Interviewing, Participant Observation, Data Analysis, and Writing It All Up . Chicago: University of Chicago Press. A readable and personal account of conducting qualitative research by an eminent sociologist, with a heavy emphasis on the kinds of participant-observation research conducted by the author. Despite its reader-friendliness, this is really a book targeted to graduate students learning the craft. Advanced .
Lune, Howard, and Bruce L. Berg. 2018. 9th edition. Qualitative Research Methods for the Social Sciences. Pearson . Although a good introduction to qualitative methods, the authors favor symbolic interactionist and dramaturgical approaches, which limits the appeal primarily to sociologists. Beginning .
Marshall, Catherine, and Gretchen B. Rossman. 2016. 6th edition. Designing Qualitative Research. Thousand Oaks, CA: SAGE. Very readable and accessible guide to research design by two educational scholars. Although the presentation is sometimes fairly dry, personal vignettes and illustrations enliven the text. Beginning .
Maxwell, Joseph A. 2013. Qualitative Research Design: An Interactive Approach . 3rd ed. Thousand Oaks, CA: SAGE. A short and accessible introduction to qualitative research design, particularly helpful for graduate students contemplating theses and dissertations. This has been a standard textbook in my graduate-level courses for years. Advanced .
Patton, Michael Quinn. 2002. Qualitative Research and Evaluation Methods . Thousand Oaks, CA: SAGE. This is a comprehensive text that served as my “go-to” reference when I was a graduate student. It is particularly helpful for those involved in program evaluation and other forms of evaluation studies and uses examples from a wide range of disciplines. Advanced .
Rubin, Ashley T. 2021. Rocking Qualitative Social Science: An Irreverent Guide to Rigorous Research. Stanford : Stanford University Press. A delightful and personal read. Rubin uses rock climbing as an extended metaphor for learning how to conduct qualitative research. A bit slanted toward ethnographic and archival methods of data collection, with frequent examples from her own studies in criminology. Beginning .
Weis, Lois, and Michelle Fine. 2000. Speed Bumps: A Student-Friendly Guide to Qualitative Research . New York: Teachers College Press. Readable and accessibly written in a quasi-conversational style. Particularly strong in its discussion of ethical issues throughout the qualitative research process. Not comprehensive, however, and very much tied to ethnographic research. Although designed for graduate students, this is a recommended read for students of all levels. Beginning .
Patton’s Ten Suggestions for Doing Qualitative Research
The following ten suggestions were made by Michael Quinn Patton in his massive textbooks Qualitative Research and Evaluations Methods . This book is highly recommended for those of you who want more than an introduction to qualitative methods. It is the book I relied on heavily when I was a graduate student, although it is much easier to “dip into” when necessary than to read through as a whole. Patton is asked for “just one bit of advice” for a graduate student considering using qualitative research methods for their dissertation. Here are his top ten responses, in short form, heavily paraphrased, and with additional comments and emphases from me:
- Make sure that a qualitative approach fits the research question. The following are the kinds of questions that call out for qualitative methods or where qualitative methods are particularly appropriate: questions about people’s experiences or how they make sense of those experiences; studying a person in their natural environment; researching a phenomenon so unknown that it would be impossible to study it with standardized instruments or other forms of quantitative data collection.
- Study qualitative research by going to the original sources for the design and analysis appropriate to the particular approach you want to take (e.g., read Glaser and Straus if you are using grounded theory )
- Find a dissertation adviser who understands or at least who will support your use of qualitative research methods. You are asking for trouble if your entire committee is populated by quantitative researchers, even if they are all very knowledgeable about the subject or focus of your study (maybe even more so if they are!)
- Really work on design. Doing qualitative research effectively takes a lot of planning. Even if things are more flexible than in quantitative research, a good design is absolutely essential when starting out.
- Practice data collection techniques, particularly interviewing and observing. There is definitely a set of learned skills here! Do not expect your first interview to be perfect. You will continue to grow as a researcher the more interviews you conduct, and you will probably come to understand yourself a bit more in the process, too. This is not easy, despite what others who don’t work with qualitative methods may assume (and tell you!)
- Have a plan for analysis before you begin data collection. This is often a requirement in IRB protocols , although you can get away with writing something fairly simple. And even if you are taking an approach, such as grounded theory, that pushes you to remain fairly open-minded during the data collection process, you still want to know what you will be doing with all the data collected – creating a codebook? Writing analytical memos? Comparing cases? Having a plan in hand will also help prevent you from collecting too much extraneous data.
- Be prepared to confront controversies both within the qualitative research community and between qualitative research and quantitative research. Don’t be naïve about this – qualitative research, particularly some approaches, will be derided by many more “positivist” researchers and audiences. For example, is an “n” of 1 really sufficient? Yes! But not everyone will agree.
- Do not make the mistake of using qualitative research methods because someone told you it was easier, or because you are intimidated by the math required of statistical analyses. Qualitative research is difficult in its own way (and many would claim much more time-consuming than quantitative research). Do it because you are convinced it is right for your goals, aims, and research questions.
- Find a good support network. This could be a research mentor, or it could be a group of friends or colleagues who are also using qualitative research, or it could be just someone who will listen to you work through all of the issues you will confront out in the field and during the writing process. Even though qualitative research often involves human subjects, it can be pretty lonely. A lot of times you will feel like you are working without a net. You have to create one for yourself. Take care of yourself.
- And, finally, in the words of Patton, “Prepare to be changed. Looking deeply at other people’s lives will force you to look deeply at yourself.”
- We will actually spend an entire chapter ( chapter 3 ) looking at this question in much more detail! ↵
- Note that this might have been news to Europeans at the time, but many other societies around the world had also come to this conclusion through observation. There is often a tendency to equate “the scientific revolution” with the European world in which it took place, but this is somewhat misleading. ↵
- Historians are a special case here. Historians have scrupulously and rigorously investigated the social world, but not for the purpose of understanding general laws about how things work, which is the point of scientific empirical research. History is often referred to as an idiographic field of study, meaning that it studies things that happened or are happening in themselves and not for general observations or conclusions. ↵
- Don’t worry, we’ll spend more time later in this book unpacking the meaning of ethnography and other terms that are important here. Note the available glossary ↵
An approach to research that is “multimethod in focus, involving an interpretative, naturalistic approach to its subject matter. This means that qualitative researchers study things in their natural settings, attempting to make sense of, or interpret, phenomena in terms of the meanings people bring to them. Qualitative research involves the studied use and collection of a variety of empirical materials – case study, personal experience, introspective, life story, interview, observational, historical, interactional, and visual texts – that describe routine and problematic moments and meanings in individuals’ lives." ( Denzin and Lincoln 2005:2 ). Contrast with quantitative research .
In contrast to methodology, methods are more simply the practices and tools used to collect and analyze data. Examples of common methods in qualitative research are interviews , observations , and documentary analysis . One’s methodology should connect to one’s choice of methods, of course, but they are distinguishable terms. See also methodology .
A proposed explanation for an observation, phenomenon, or scientific problem that can be tested by further investigation. The positing of a hypothesis is often the first step in quantitative research but not in qualitative research. Even when qualitative researchers offer possible explanations in advance of conducting research, they will tend to not use the word “hypothesis” as it conjures up the kind of positivist research they are not conducting.
The foundational question to be addressed by the research study. This will form the anchor of the research design, collection, and analysis. Note that in qualitative research, the research question may, and probably will, alter or develop during the course of the research.
An approach to research that collects and analyzes numerical data for the purpose of finding patterns and averages, making predictions, testing causal relationships, and generalizing results to wider populations. Contrast with qualitative research .
Data collection that takes place in real-world settings, referred to as “the field;” a key component of much Grounded Theory and ethnographic research. Patton ( 2002 ) calls fieldwork “the central activity of qualitative inquiry” where “‘going into the field’ means having direct and personal contact with people under study in their own environments – getting close to people and situations being studied to personally understand the realities of minutiae of daily life” (48).
The people who are the subjects of a qualitative study. In interview-based studies, they may be the respondents to the interviewer; for purposes of IRBs, they are often referred to as the human subjects of the research.
The branch of philosophy concerned with knowledge. For researchers, it is important to recognize and adopt one of the many distinguishing epistemological perspectives as part of our understanding of what questions research can address or fully answer. See, e.g., constructivism , subjectivism, and objectivism .
An approach that refutes the possibility of neutrality in social science research. All research is “guided by a set of beliefs and feelings about the world and how it should be understood and studied” (Denzin and Lincoln 2005: 13). In contrast to positivism , interpretivism recognizes the social constructedness of reality, and researchers adopting this approach focus on capturing interpretations and understandings people have about the world rather than “the world” as it is (which is a chimera).
The cluster of data-collection tools and techniques that involve observing interactions between people, the behaviors, and practices of individuals (sometimes in contrast to what they say about how they act and behave), and cultures in context. Observational methods are the key tools employed by ethnographers and Grounded Theory .
Research based on data collected and analyzed by the research (in contrast to secondary “library” research).
The process of selecting people or other units of analysis to represent a larger population. In quantitative research, this representation is taken quite literally, as statistically representative. In qualitative research, in contrast, sample selection is often made based on potential to generate insight about a particular topic or phenomenon.
A method of data collection in which the researcher asks the participant questions; the answers to these questions are often recorded and transcribed verbatim. There are many different kinds of interviews - see also semistructured interview , structured interview , and unstructured interview .
The specific group of individuals that you will collect data from. Contrast population.
The practice of being conscious of and reflective upon one’s own social location and presence when conducting research. Because qualitative research often requires interaction with live humans, failing to take into account how one’s presence and prior expectations and social location affect the data collected and how analyzed may limit the reliability of the findings. This remains true even when dealing with historical archives and other content. Who we are matters when asking questions about how people experience the world because we, too, are a part of that world.
The science and practice of right conduct; in research, it is also the delineation of moral obligations towards research participants, communities to which we belong, and communities in which we conduct our research.
An administrative body established to protect the rights and welfare of human research subjects recruited to participate in research activities conducted under the auspices of the institution with which it is affiliated. The IRB is charged with the responsibility of reviewing all research involving human participants. The IRB is concerned with protecting the welfare, rights, and privacy of human subjects. The IRB has the authority to approve, disapprove, monitor, and require modifications in all research activities that fall within its jurisdiction as specified by both the federal regulations and institutional policy.
Research, according to US federal guidelines, that involves “a living individual about whom an investigator (whether professional or student) conducting research: (1) Obtains information or biospecimens through intervention or interaction with the individual, and uses, studies, or analyzes the information or biospecimens; or (2) Obtains, uses, studies, analyzes, or generates identifiable private information or identifiable biospecimens.”
One of the primary methodological traditions of inquiry in qualitative research, ethnography is the study of a group or group culture, largely through observational fieldwork supplemented by interviews. It is a form of fieldwork that may include participant-observation data collection. See chapter 14 for a discussion of deep ethnography.
A form of interview that follows a standard guide of questions asked, although the order of the questions may change to match the particular needs of each individual interview subject, and probing “follow-up” questions are often added during the course of the interview. The semi-structured interview is the primary form of interviewing used by qualitative researchers in the social sciences. It is sometimes referred to as an “in-depth” interview. See also interview and interview guide .
A method of observational data collection taking place in a natural setting; a form of fieldwork . The term encompasses a continuum of relative participation by the researcher (from full participant to “fly-on-the-wall” observer). This is also sometimes referred to as ethnography , although the latter is characterized by a greater focus on the culture under observation.
A research design that employs both quantitative and qualitative methods, as in the case of a survey supplemented by interviews.
An epistemological perspective that posits the existence of reality through sensory experience similar to empiricism but goes further in denying any non-sensory basis of thought or consciousness. In the social sciences, the term has roots in the proto-sociologist August Comte, who believed he could discern “laws” of society similar to the laws of natural science (e.g., gravity). The term has come to mean the kinds of measurable and verifiable science conducted by quantitative researchers and is thus used pejoratively by some qualitative researchers interested in interpretation, consciousness, and human understanding. Calling someone a “positivist” is often intended as an insult. See also empiricism and objectivism.
A place or collection containing records, documents, or other materials of historical interest; most universities have an archive of material related to the university’s history, as well as other “special collections” that may be of interest to members of the community.
A method of both data collection and data analysis in which a given content (textual, visual, graphic) is examined systematically and rigorously to identify meanings, themes, patterns and assumptions. Qualitative content analysis (QCA) is concerned with gathering and interpreting an existing body of material.
A word or short phrase that symbolically assigns a summative, salient, essence-capturing, and/or evocative attribute for a portion of language-based or visual data (Saldaña 2021:5).
Usually a verbatim written record of an interview or focus group discussion.
The primary form of data for fieldwork , participant observation , and ethnography . These notes, taken by the researcher either during the course of fieldwork or at day’s end, should include as many details as possible on what was observed and what was said. They should include clear identifiers of date, time, setting, and names (or identifying characteristics) of participants.
The process of labeling and organizing qualitative data to identify different themes and the relationships between them; a way of simplifying data to allow better management and retrieval of key themes and illustrative passages. See coding frame and codebook.
A methodological tradition of inquiry and approach to analyzing qualitative data in which theories emerge from a rigorous and systematic process of induction. This approach was pioneered by the sociologists Glaser and Strauss (1967). The elements of theory generated from comparative analysis of data are, first, conceptual categories and their properties and, second, hypotheses or generalized relations among the categories and their properties – “The constant comparing of many groups draws the [researcher’s] attention to their many similarities and differences. Considering these leads [the researcher] to generate abstract categories and their properties, which, since they emerge from the data, will clearly be important to a theory explaining the kind of behavior under observation.” (36).
A detailed description of any proposed research that involves human subjects for review by IRB. The protocol serves as the recipe for the conduct of the research activity. It includes the scientific rationale to justify the conduct of the study, the information necessary to conduct the study, the plan for managing and analyzing the data, and a discussion of the research ethical issues relevant to the research. Protocols for qualitative research often include interview guides, all documents related to recruitment, informed consent forms, very clear guidelines on the safekeeping of materials collected, and plans for de-identifying transcripts or other data that include personal identifying information.
Introduction to Qualitative Research Methods Copyright © 2023 by Allison Hurst is licensed under a Creative Commons Attribution-ShareAlike 4.0 International License , except where otherwise noted.
Educational resources and simple solutions for your research journey
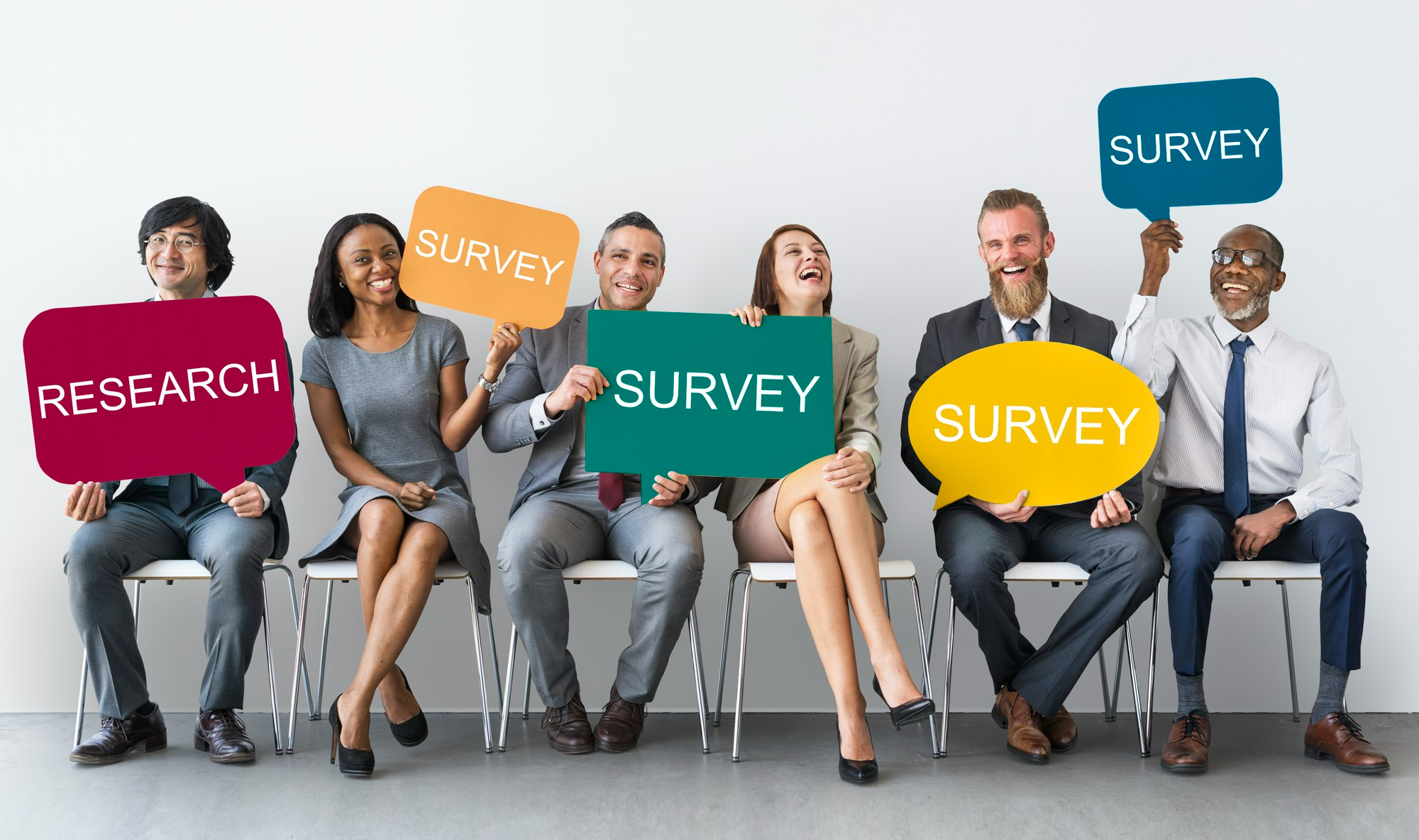
What is Qualitative Research? Methods, Types, Approaches and Examples
Qualitative research is a type of method that researchers use depending on their study requirements. Research can be conducted using several methods, but before starting the process, researchers should understand the different methods available to decide the best one for their study type. The type of research method needed depends on a few important criteria, such as the research question, study type, time, costs, data availability, and availability of respondents. The two main types of methods are qualitative research and quantitative research. Sometimes, researchers may find it difficult to decide which type of method is most suitable for their study. Keeping in mind a simple rule of thumb could help you make the correct decision. Quantitative research should be used to validate or test a theory or hypothesis and qualitative research should be used to understand a subject or event or identify reasons for observed patterns.
Qualitative research methods are based on principles of social sciences from several disciplines like psychology, sociology, and anthropology. In this method, researchers try to understand the feelings and motivation of their respondents, which would have prompted them to select or give a particular response to a question. Here are two qualitative research examples :
- Two brands (A & B) of the same medicine are available at a pharmacy. However, Brand A is more popular and has higher sales. In qualitative research , the interviewers would ideally visit a few stores in different areas and ask customers their reason for selecting either brand. Respondents may have different reasons that motivate them to select one brand over the other, such as brand loyalty, cost, feedback from friends, doctor’s suggestion, etc. Once the reasons are known, companies could then address challenges in that specific area to increase their product’s sales.
- A company organizes a focus group meeting with a random sample of its product’s consumers to understand their opinion on a new product being launched.

Table of Contents
What is qualitative research? 1
Qualitative research is the process of collecting, analyzing, and interpreting non-numerical data. The findings of qualitative research are expressed in words and help in understanding individuals’ subjective perceptions about an event, condition, or subject. This type of research is exploratory and is used to generate hypotheses or theories from data. Qualitative data are usually in the form of text, videos, photographs, and audio recordings. There are multiple qualitative research types , which will be discussed later.
Qualitative research methods 2
Researchers can choose from several qualitative research methods depending on the study type, research question, the researcher’s role, data to be collected, etc.
The following table lists the common qualitative research approaches with their purpose and examples, although there may be an overlap between some.
Narrative | Explore the experiences of individuals and tell a story to give insight into human lives and behaviors. Narratives can be obtained from journals, letters, conversations, autobiographies, interviews, etc. | A researcher collecting information to create a biography using old documents, interviews, etc. |
Phenomenology | Explain life experiences or phenomena, focusing on people’s subjective experiences and interpretations of the world. | Researchers exploring the experiences of family members of an individual undergoing a major surgery. |
Grounded theory | Investigate process, actions, and interactions, and based on this grounded or empirical data a theory is developed. Unlike experimental research, this method doesn’t require a hypothesis theory to begin with. | A company with a high attrition rate and no prior data may use this method to understand the reasons for which employees leave. |
Ethnography | Describe an ethnic, cultural, or social group by observation in their naturally occurring environment. | A researcher studying medical personnel in the immediate care division of a hospital to understand the culture and staff behaviors during high capacity. |
Case study | In-depth analysis of complex issues in real-life settings, mostly used in business, law, and policymaking. Learnings from case studies can be implemented in other similar contexts. | A case study about how a particular company turned around its product sales and the marketing strategies they used could help implement similar methods in other companies. |
Types of qualitative research 3,4
The data collection methods in qualitative research are designed to assess and understand the perceptions, motivations, and feelings of the respondents about the subject being studied. The different qualitative research types include the following:
- In-depth or one-on-one interviews : This is one of the most common qualitative research methods and helps the interviewers understand a respondent’s subjective opinion and experience pertaining to a specific topic or event. These interviews are usually conversational and encourage the respondents to express their opinions freely. Semi-structured interviews, which have open-ended questions (where the respondents can answer more than just “yes” or “no”), are commonly used. Such interviews can be either face-to-face or telephonic, and the duration can vary depending on the subject or the interviewer. Asking the right questions is essential in this method so that the interview can be led in the suitable direction. Face-to-face interviews also help interviewers observe the respondents’ body language, which could help in confirming whether the responses match.
- Document study/Literature review/Record keeping : Researchers’ review of already existing written materials such as archives, annual reports, research articles, guidelines, policy documents, etc.
- Focus groups : Usually include a small sample of about 6-10 people and a moderator, to understand the participants’ opinion on a given topic. Focus groups ensure constructive discussions to understand the why, what, and, how about the topic. These group meetings need not always be in-person. In recent times, online meetings are also encouraged, and online surveys could also be administered with the option to “write” subjective answers as well. However, this method is expensive and is mostly used for new products and ideas.
- Qualitative observation : In this method, researchers collect data using their five senses—sight, smell, touch, taste, and hearing. This method doesn’t include any measurements but only the subjective observation. For example, “The dessert served at the bakery was creamy with sweet buttercream frosting”; this observation is based on the taste perception.
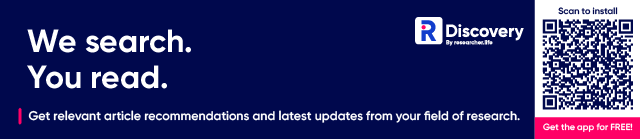
Qualitative research : Data collection and analysis
- Qualitative data collection is the process by which observations or measurements are gathered in research.
- The data collected are usually non-numeric and subjective and could be recorded in various methods, for instance, in case of one-to-one interviews, the responses may be recorded using handwritten notes, and audio and video recordings, depending on the interviewer and the setting or duration.
- Once the data are collected, they should be transcribed into meaningful or useful interpretations. An experienced researcher could take about 8-10 hours to transcribe an interview’s recordings. All such notes and recordings should be maintained properly for later reference.
- Some interviewers make use of “field notes.” These are not exactly the respondents’ answers but rather some observations the interviewer may have made while asking questions and may include non-verbal cues or any information about the setting or the environment. These notes are usually informal and help verify respondents’ answers.
2. Qualitative data analysis
- This process involves analyzing all the data obtained from the qualitative research methods in the form of text (notes), audio-video recordings, and pictures.
- Text analysis is a common form of qualitative data analysis in which researchers examine the social lives of the participants and analyze their words, actions, etc. in specific contexts. Social media platforms are now playing an important role in this method with researchers analyzing all information shared online.
There are usually five steps in the qualitative data analysis process: 5
- Prepare and organize the data
- Transcribe interviews
- Collect and document field notes and other material
- Review and explore the data
- Examine the data for patterns or important observations
- Develop a data coding system
- Create codes to categorize and connect the data
- Assign these codes to the data or responses
- Review the codes
- Identify recurring themes, opinions, patterns, etc.
- Present the findings
- Use the best possible method to present your observations
The following table 6 lists some common qualitative data analysis methods used by companies to make important decisions, with examples and when to use each. The methods may be similar and can overlap.
Content analysis | To identify patterns in text, by grouping content into words, concepts, and themes; that is, determine presence of certain words or themes in some text | Researchers examining the language used in a journal article to search for bias |
Narrative analysis | To understand people’s perspectives on specific issues. Focuses on people’s stories and the language used to tell these stories | A researcher conducting one or several in-depth interviews with an individual over a long period |
Discourse analysis | To understand political, cultural, and power dynamics in specific contexts; that is, how people express themselves in different social contexts | A researcher studying a politician’s speeches across multiple contexts, such as audience, region, political history, etc. |
Thematic analysis | To interpret the meaning behind the words used by people. This is done by identifying repetitive patterns or themes by reading through a dataset | Researcher analyzing raw data to explore the impact of high-stakes examinations on students and parents |
Characteristics of qualitative research methods 4
- Unstructured raw data : Qualitative research methods use unstructured, non-numerical data , which are analyzed to generate subjective conclusions about specific subjects, usually presented descriptively, instead of using statistical data.
- Site-specific data collection : In qualitative research methods , data are collected at specific areas where the respondents or researchers are either facing a challenge or have a need to explore. The process is conducted in a real-world setting and participants do not need to leave their original geographical setting to be able to participate.
- Researchers’ importance : Researchers play an instrumental role because, in qualitative research , communication with respondents is an essential part of data collection and analysis. In addition, researchers need to rely on their own observation and listening skills during an interaction and use and interpret that data appropriately.
- Multiple methods : Researchers collect data through various methods, as listed earlier, instead of relying on a single source. Although there may be some overlap between the qualitative research methods , each method has its own significance.
- Solving complex issues : These methods help in breaking down complex problems into more useful and interpretable inferences, which can be easily understood by everyone.
- Unbiased responses : Qualitative research methods rely on open communication where the participants are allowed to freely express their views. In such cases, the participants trust the interviewer, resulting in unbiased and truthful responses.
- Flexible : The qualitative research method can be changed at any stage of the research. The data analysis is not confined to being done at the end of the research but can be done in tandem with data collection. Consequently, based on preliminary analysis and new ideas, researchers have the liberty to change the method to suit their objective.
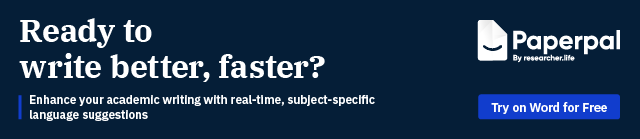
When to use qualitative research 4
The following points will give you an idea about when to use qualitative research .
- When the objective of a research study is to understand behaviors and patterns of respondents, then qualitative research is the most suitable method because it gives a clear insight into the reasons for the occurrence of an event.
- A few use cases for qualitative research methods include:
- New product development or idea generation
- Strengthening a product’s marketing strategy
- Conducting a SWOT analysis of product or services portfolios to help take important strategic decisions
- Understanding purchasing behavior of consumers
- Understanding reactions of target market to ad campaigns
- Understanding market demographics and conducting competitor analysis
- Understanding the effectiveness of a new treatment method in a particular section of society
A qualitative research method case study to understand when to use qualitative research 7
Context : A high school in the US underwent a turnaround or conservatorship process and consequently experienced a below average teacher retention rate. Researchers conducted qualitative research to understand teachers’ experiences and perceptions of how the turnaround may have influenced the teachers’ morale and how this, in turn, would have affected teachers’ retention.
Method : Purposive sampling was used to select eight teachers who were employed with the school before the conservatorship process and who were subsequently retained. One-on-one semi-structured interviews were conducted with these teachers. The questions addressed teachers’ perspectives of morale and their views on the conservatorship process.
Results : The study generated six factors that may have been influencing teachers’ perspectives: powerlessness, excessive visitations, loss of confidence, ineffective instructional practices, stress and burnout, and ineffective professional development opportunities. Based on these factors, four recommendations were made to increase teacher retention by boosting their morale.
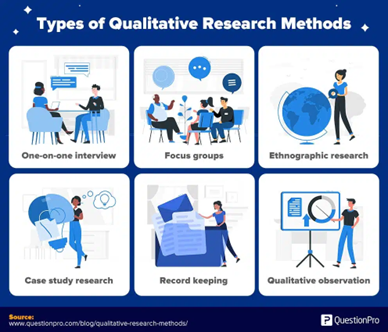
Advantages of qualitative research 1
- Reflects real-world settings , and therefore allows for ambiguities in data, as well as the flexibility to change the method based on new developments.
- Helps in understanding the feelings or beliefs of the respondents rather than relying only on quantitative data.
- Uses a descriptive and narrative style of presentation, which may be easier to understand for people from all backgrounds.
- Some topics involving sensitive or controversial content could be difficult to quantify and so qualitative research helps in analyzing such content.
- The availability of multiple data sources and research methods helps give a holistic picture.
- There’s more involvement of participants, which gives them an assurance that their opinion matters, possibly leading to unbiased responses.
Disadvantages of qualitative research 1
- Large-scale data sets cannot be included because of time and cost constraints.
- Ensuring validity and reliability may be a challenge because of the subjective nature of the data, so drawing definite conclusions could be difficult.
- Replication by other researchers may be difficult for the same contexts or situations.
- Generalization to a wider context or to other populations or settings is not possible.
- Data collection and analysis may be time consuming.
- Researcher’s interpretation may alter the results causing an unintended bias.
Differences between qualitative research and quantitative research 1
Purpose and design | Explore ideas, formulate hypotheses; more subjective | Test theories and hypotheses, discover causal relationships; measurable and more structured |
Data collection method | Semi-structured interviews/surveys with open-ended questions, document study/literature reviews, focus groups, case study research, ethnography | Experiments, controlled observations, questionnaires and surveys with a rating scale or closed-ended questions. The methods can be experimental, quasi-experimental, descriptive, or correlational. |
Data analysis | Content analysis (determine presence of certain words/concepts in texts), grounded theory (hypothesis creation by data collection and analysis), thematic analysis (identify important themes/patterns in data and use these to address an issue) | Statistical analysis using applications such as Excel, SPSS, R |
Sample size | Small | Large |
Example | A company organizing focus groups or one-to-one interviews to understand customers’ (subjective) opinions about a specific product, based on which the company can modify their marketing strategy | Customer satisfaction surveys sent out by companies. Customers are asked to rate their experience on a rating scale of 1 to 5 |
Frequently asked questions on qualitative research
Q: how do i know if qualitative research is appropriate for my study .
A: Here’s a simple checklist you could use:
- Not much is known about the subject being studied.
- There is a need to understand or simplify a complex problem or situation.
- Participants’ experiences/beliefs/feelings are required for analysis.
- There’s no existing hypothesis to begin with, rather a theory would need to be created after analysis.
- You need to gather in-depth understanding of an event or subject, which may not need to be supported by numeric data.
Q: How do I ensure the reliability and validity of my qualitative research findings?
A: To ensure the validity of your qualitative research findings you should explicitly state your objective and describe clearly why you have interpreted the data in a particular way. Another method could be to connect your data in different ways or from different perspectives to see if you reach a similar, unbiased conclusion.
To ensure reliability, always create an audit trail of your qualitative research by describing your steps and reasons for every interpretation, so that if required, another researcher could trace your steps to corroborate your (or their own) findings. In addition, always look for patterns or consistencies in the data collected through different methods.
Q: Are there any sampling strategies or techniques for qualitative research ?
A: Yes, the following are few common sampling strategies used in qualitative research :
1. Convenience sampling
Selects participants who are most easily accessible to researchers due to geographical proximity, availability at a particular time, etc.
2. Purposive sampling
Participants are grouped according to predefined criteria based on a specific research question. Sample sizes are often determined based on theoretical saturation (when new data no longer provide additional insights).
3. Snowball sampling
Already selected participants use their social networks to refer the researcher to other potential participants.
4. Quota sampling
While designing the study, the researchers decide how many people with which characteristics to include as participants. The characteristics help in choosing people most likely to provide insights into the subject.

Q: What ethical standards need to be followed with qualitative research ?
A: The following ethical standards should be considered in qualitative research:
- Anonymity : The participants should never be identified in the study and researchers should ensure that no identifying information is mentioned even indirectly.
- Confidentiality : To protect participants’ confidentiality, ensure that all related documents, transcripts, notes are stored safely.
- Informed consent : Researchers should clearly communicate the objective of the study and how the participants’ responses will be used prior to engaging with the participants.
Q: How do I address bias in my qualitative research ?
A: You could use the following points to ensure an unbiased approach to your qualitative research :
- Check your interpretations of the findings with others’ interpretations to identify consistencies.
- If possible, you could ask your participants if your interpretations convey their beliefs to a significant extent.
- Data triangulation is a way of using multiple data sources to see if all methods consistently support your interpretations.
- Contemplate other possible explanations for your findings or interpretations and try ruling them out if possible.
- Conduct a peer review of your findings to identify any gaps that may not have been visible to you.
- Frame context-appropriate questions to ensure there is no researcher or participant bias.
We hope this article has given you answers to the question “ what is qualitative research ” and given you an in-depth understanding of the various aspects of qualitative research , including the definition, types, and approaches, when to use this method, and advantages and disadvantages, so that the next time you undertake a study you would know which type of research design to adopt.
References:
- McLeod, S. A. Qualitative vs. quantitative research. Simply Psychology [Accessed January 17, 2023]. www.simplypsychology.org/qualitative-quantitative.html
- Omniconvert website [Accessed January 18, 2023]. https://www.omniconvert.com/blog/qualitative-research-definition-methodology-limitation-examples/
- Busetto L., Wick W., Gumbinger C. How to use and assess qualitative research methods. Neurological Research and Practice [Accessed January 19, 2023] https://neurolrespract.biomedcentral.com/articles/10.1186/s42466-020-00059
- QuestionPro website. Qualitative research methods: Types & examples [Accessed January 16, 2023]. https://www.questionpro.com/blog/qualitative-research-methods/
- Campuslabs website. How to analyze qualitative data [Accessed January 18, 2023]. https://baselinesupport.campuslabs.com/hc/en-us/articles/204305675-How-to-analyze-qualitative-data
- Thematic website. Qualitative data analysis: Step-by-guide [Accessed January 20, 2023]. https://getthematic.com/insights/qualitative-data-analysis/
- Lane L. J., Jones D., Penny G. R. Qualitative case study of teachers’ morale in a turnaround school. Research in Higher Education Journal . https://files.eric.ed.gov/fulltext/EJ1233111.pdf
- Meetingsnet website. 7 FAQs about qualitative research and CME [Accessed January 21, 2023]. https://www.meetingsnet.com/cme-design/7-faqs-about-qualitative-research-and-cme
- Qualitative research methods: A data collector’s field guide. Khoury College of Computer Sciences. Northeastern University. https://course.ccs.neu.edu/is4800sp12/resources/qualmethods.pdf
Editage All Access is a subscription-based platform that unifies the best AI tools and services designed to speed up, simplify, and streamline every step of a researcher’s journey. The Editage All Access Pack is a one-of-a-kind subscription that unlocks full access to an AI writing assistant, literature recommender, journal finder, scientific illustration tool, and exclusive discounts on professional publication services from Editage.
Based on 22+ years of experience in academia, Editage All Access empowers researchers to put their best research forward and move closer to success. Explore our top AI Tools pack, AI Tools + Publication Services pack, or Build Your Own Plan. Find everything a researcher needs to succeed, all in one place – Get All Access now starting at just $14 a month !
Related Posts

Back to School – Lock-in All Access Pack for a Year at the Best Price

Journal Turnaround Time: Researcher.Life and Scholarly Intelligence Join Hands to Empower Researchers with Publication Time Insights

An official website of the United States government
The .gov means it’s official. Federal government websites often end in .gov or .mil. Before sharing sensitive information, make sure you’re on a federal government site.
The site is secure. The https:// ensures that you are connecting to the official website and that any information you provide is encrypted and transmitted securely.
- Publications
- Account settings
Preview improvements coming to the PMC website in October 2024. Learn More or Try it out now .
- Advanced Search
- Journal List
- Neurol Res Pract

How to use and assess qualitative research methods
Loraine busetto.
1 Department of Neurology, Heidelberg University Hospital, Im Neuenheimer Feld 400, 69120 Heidelberg, Germany

Wolfgang Wick
2 Clinical Cooperation Unit Neuro-Oncology, German Cancer Research Center, Heidelberg, Germany
Christoph Gumbinger
Associated data.
Not applicable.
This paper aims to provide an overview of the use and assessment of qualitative research methods in the health sciences. Qualitative research can be defined as the study of the nature of phenomena and is especially appropriate for answering questions of why something is (not) observed, assessing complex multi-component interventions, and focussing on intervention improvement. The most common methods of data collection are document study, (non-) participant observations, semi-structured interviews and focus groups. For data analysis, field-notes and audio-recordings are transcribed into protocols and transcripts, and coded using qualitative data management software. Criteria such as checklists, reflexivity, sampling strategies, piloting, co-coding, member-checking and stakeholder involvement can be used to enhance and assess the quality of the research conducted. Using qualitative in addition to quantitative designs will equip us with better tools to address a greater range of research problems, and to fill in blind spots in current neurological research and practice.
The aim of this paper is to provide an overview of qualitative research methods, including hands-on information on how they can be used, reported and assessed. This article is intended for beginning qualitative researchers in the health sciences as well as experienced quantitative researchers who wish to broaden their understanding of qualitative research.
What is qualitative research?
Qualitative research is defined as “the study of the nature of phenomena”, including “their quality, different manifestations, the context in which they appear or the perspectives from which they can be perceived” , but excluding “their range, frequency and place in an objectively determined chain of cause and effect” [ 1 ]. This formal definition can be complemented with a more pragmatic rule of thumb: qualitative research generally includes data in form of words rather than numbers [ 2 ].
Why conduct qualitative research?
Because some research questions cannot be answered using (only) quantitative methods. For example, one Australian study addressed the issue of why patients from Aboriginal communities often present late or not at all to specialist services offered by tertiary care hospitals. Using qualitative interviews with patients and staff, it found one of the most significant access barriers to be transportation problems, including some towns and communities simply not having a bus service to the hospital [ 3 ]. A quantitative study could have measured the number of patients over time or even looked at possible explanatory factors – but only those previously known or suspected to be of relevance. To discover reasons for observed patterns, especially the invisible or surprising ones, qualitative designs are needed.
While qualitative research is common in other fields, it is still relatively underrepresented in health services research. The latter field is more traditionally rooted in the evidence-based-medicine paradigm, as seen in " research that involves testing the effectiveness of various strategies to achieve changes in clinical practice, preferably applying randomised controlled trial study designs (...) " [ 4 ]. This focus on quantitative research and specifically randomised controlled trials (RCT) is visible in the idea of a hierarchy of research evidence which assumes that some research designs are objectively better than others, and that choosing a "lesser" design is only acceptable when the better ones are not practically or ethically feasible [ 5 , 6 ]. Others, however, argue that an objective hierarchy does not exist, and that, instead, the research design and methods should be chosen to fit the specific research question at hand – "questions before methods" [ 2 , 7 – 9 ]. This means that even when an RCT is possible, some research problems require a different design that is better suited to addressing them. Arguing in JAMA, Berwick uses the example of rapid response teams in hospitals, which he describes as " a complex, multicomponent intervention – essentially a process of social change" susceptible to a range of different context factors including leadership or organisation history. According to him, "[in] such complex terrain, the RCT is an impoverished way to learn. Critics who use it as a truth standard in this context are incorrect" [ 8 ] . Instead of limiting oneself to RCTs, Berwick recommends embracing a wider range of methods , including qualitative ones, which for "these specific applications, (...) are not compromises in learning how to improve; they are superior" [ 8 ].
Research problems that can be approached particularly well using qualitative methods include assessing complex multi-component interventions or systems (of change), addressing questions beyond “what works”, towards “what works for whom when, how and why”, and focussing on intervention improvement rather than accreditation [ 7 , 9 – 12 ]. Using qualitative methods can also help shed light on the “softer” side of medical treatment. For example, while quantitative trials can measure the costs and benefits of neuro-oncological treatment in terms of survival rates or adverse effects, qualitative research can help provide a better understanding of patient or caregiver stress, visibility of illness or out-of-pocket expenses.
How to conduct qualitative research?
Given that qualitative research is characterised by flexibility, openness and responsivity to context, the steps of data collection and analysis are not as separate and consecutive as they tend to be in quantitative research [ 13 , 14 ]. As Fossey puts it : “sampling, data collection, analysis and interpretation are related to each other in a cyclical (iterative) manner, rather than following one after another in a stepwise approach” [ 15 ]. The researcher can make educated decisions with regard to the choice of method, how they are implemented, and to which and how many units they are applied [ 13 ]. As shown in Fig. 1 , this can involve several back-and-forth steps between data collection and analysis where new insights and experiences can lead to adaption and expansion of the original plan. Some insights may also necessitate a revision of the research question and/or the research design as a whole. The process ends when saturation is achieved, i.e. when no relevant new information can be found (see also below: sampling and saturation). For reasons of transparency, it is essential for all decisions as well as the underlying reasoning to be well-documented.

Iterative research process
While it is not always explicitly addressed, qualitative methods reflect a different underlying research paradigm than quantitative research (e.g. constructivism or interpretivism as opposed to positivism). The choice of methods can be based on the respective underlying substantive theory or theoretical framework used by the researcher [ 2 ].
Data collection
The methods of qualitative data collection most commonly used in health research are document study, observations, semi-structured interviews and focus groups [ 1 , 14 , 16 , 17 ].
Document study
Document study (also called document analysis) refers to the review by the researcher of written materials [ 14 ]. These can include personal and non-personal documents such as archives, annual reports, guidelines, policy documents, diaries or letters.
Observations
Observations are particularly useful to gain insights into a certain setting and actual behaviour – as opposed to reported behaviour or opinions [ 13 ]. Qualitative observations can be either participant or non-participant in nature. In participant observations, the observer is part of the observed setting, for example a nurse working in an intensive care unit [ 18 ]. In non-participant observations, the observer is “on the outside looking in”, i.e. present in but not part of the situation, trying not to influence the setting by their presence. Observations can be planned (e.g. for 3 h during the day or night shift) or ad hoc (e.g. as soon as a stroke patient arrives at the emergency room). During the observation, the observer takes notes on everything or certain pre-determined parts of what is happening around them, for example focusing on physician-patient interactions or communication between different professional groups. Written notes can be taken during or after the observations, depending on feasibility (which is usually lower during participant observations) and acceptability (e.g. when the observer is perceived to be judging the observed). Afterwards, these field notes are transcribed into observation protocols. If more than one observer was involved, field notes are taken independently, but notes can be consolidated into one protocol after discussions. Advantages of conducting observations include minimising the distance between the researcher and the researched, the potential discovery of topics that the researcher did not realise were relevant and gaining deeper insights into the real-world dimensions of the research problem at hand [ 18 ].
Semi-structured interviews
Hijmans & Kuyper describe qualitative interviews as “an exchange with an informal character, a conversation with a goal” [ 19 ]. Interviews are used to gain insights into a person’s subjective experiences, opinions and motivations – as opposed to facts or behaviours [ 13 ]. Interviews can be distinguished by the degree to which they are structured (i.e. a questionnaire), open (e.g. free conversation or autobiographical interviews) or semi-structured [ 2 , 13 ]. Semi-structured interviews are characterized by open-ended questions and the use of an interview guide (or topic guide/list) in which the broad areas of interest, sometimes including sub-questions, are defined [ 19 ]. The pre-defined topics in the interview guide can be derived from the literature, previous research or a preliminary method of data collection, e.g. document study or observations. The topic list is usually adapted and improved at the start of the data collection process as the interviewer learns more about the field [ 20 ]. Across interviews the focus on the different (blocks of) questions may differ and some questions may be skipped altogether (e.g. if the interviewee is not able or willing to answer the questions or for concerns about the total length of the interview) [ 20 ]. Qualitative interviews are usually not conducted in written format as it impedes on the interactive component of the method [ 20 ]. In comparison to written surveys, qualitative interviews have the advantage of being interactive and allowing for unexpected topics to emerge and to be taken up by the researcher. This can also help overcome a provider or researcher-centred bias often found in written surveys, which by nature, can only measure what is already known or expected to be of relevance to the researcher. Interviews can be audio- or video-taped; but sometimes it is only feasible or acceptable for the interviewer to take written notes [ 14 , 16 , 20 ].
Focus groups
Focus groups are group interviews to explore participants’ expertise and experiences, including explorations of how and why people behave in certain ways [ 1 ]. Focus groups usually consist of 6–8 people and are led by an experienced moderator following a topic guide or “script” [ 21 ]. They can involve an observer who takes note of the non-verbal aspects of the situation, possibly using an observation guide [ 21 ]. Depending on researchers’ and participants’ preferences, the discussions can be audio- or video-taped and transcribed afterwards [ 21 ]. Focus groups are useful for bringing together homogeneous (to a lesser extent heterogeneous) groups of participants with relevant expertise and experience on a given topic on which they can share detailed information [ 21 ]. Focus groups are a relatively easy, fast and inexpensive method to gain access to information on interactions in a given group, i.e. “the sharing and comparing” among participants [ 21 ]. Disadvantages include less control over the process and a lesser extent to which each individual may participate. Moreover, focus group moderators need experience, as do those tasked with the analysis of the resulting data. Focus groups can be less appropriate for discussing sensitive topics that participants might be reluctant to disclose in a group setting [ 13 ]. Moreover, attention must be paid to the emergence of “groupthink” as well as possible power dynamics within the group, e.g. when patients are awed or intimidated by health professionals.
Choosing the “right” method
As explained above, the school of thought underlying qualitative research assumes no objective hierarchy of evidence and methods. This means that each choice of single or combined methods has to be based on the research question that needs to be answered and a critical assessment with regard to whether or to what extent the chosen method can accomplish this – i.e. the “fit” between question and method [ 14 ]. It is necessary for these decisions to be documented when they are being made, and to be critically discussed when reporting methods and results.
Let us assume that our research aim is to examine the (clinical) processes around acute endovascular treatment (EVT), from the patient’s arrival at the emergency room to recanalization, with the aim to identify possible causes for delay and/or other causes for sub-optimal treatment outcome. As a first step, we could conduct a document study of the relevant standard operating procedures (SOPs) for this phase of care – are they up-to-date and in line with current guidelines? Do they contain any mistakes, irregularities or uncertainties that could cause delays or other problems? Regardless of the answers to these questions, the results have to be interpreted based on what they are: a written outline of what care processes in this hospital should look like. If we want to know what they actually look like in practice, we can conduct observations of the processes described in the SOPs. These results can (and should) be analysed in themselves, but also in comparison to the results of the document analysis, especially as regards relevant discrepancies. Do the SOPs outline specific tests for which no equipment can be observed or tasks to be performed by specialized nurses who are not present during the observation? It might also be possible that the written SOP is outdated, but the actual care provided is in line with current best practice. In order to find out why these discrepancies exist, it can be useful to conduct interviews. Are the physicians simply not aware of the SOPs (because their existence is limited to the hospital’s intranet) or do they actively disagree with them or does the infrastructure make it impossible to provide the care as described? Another rationale for adding interviews is that some situations (or all of their possible variations for different patient groups or the day, night or weekend shift) cannot practically or ethically be observed. In this case, it is possible to ask those involved to report on their actions – being aware that this is not the same as the actual observation. A senior physician’s or hospital manager’s description of certain situations might differ from a nurse’s or junior physician’s one, maybe because they intentionally misrepresent facts or maybe because different aspects of the process are visible or important to them. In some cases, it can also be relevant to consider to whom the interviewee is disclosing this information – someone they trust, someone they are otherwise not connected to, or someone they suspect or are aware of being in a potentially “dangerous” power relationship to them. Lastly, a focus group could be conducted with representatives of the relevant professional groups to explore how and why exactly they provide care around EVT. The discussion might reveal discrepancies (between SOPs and actual care or between different physicians) and motivations to the researchers as well as to the focus group members that they might not have been aware of themselves. For the focus group to deliver relevant information, attention has to be paid to its composition and conduct, for example, to make sure that all participants feel safe to disclose sensitive or potentially problematic information or that the discussion is not dominated by (senior) physicians only. The resulting combination of data collection methods is shown in Fig. 2 .

Possible combination of data collection methods
Attributions for icons: “Book” by Serhii Smirnov, “Interview” by Adrien Coquet, FR, “Magnifying Glass” by anggun, ID, “Business communication” by Vectors Market; all from the Noun Project
The combination of multiple data source as described for this example can be referred to as “triangulation”, in which multiple measurements are carried out from different angles to achieve a more comprehensive understanding of the phenomenon under study [ 22 , 23 ].
Data analysis
To analyse the data collected through observations, interviews and focus groups these need to be transcribed into protocols and transcripts (see Fig. 3 ). Interviews and focus groups can be transcribed verbatim , with or without annotations for behaviour (e.g. laughing, crying, pausing) and with or without phonetic transcription of dialects and filler words, depending on what is expected or known to be relevant for the analysis. In the next step, the protocols and transcripts are coded , that is, marked (or tagged, labelled) with one or more short descriptors of the content of a sentence or paragraph [ 2 , 15 , 23 ]. Jansen describes coding as “connecting the raw data with “theoretical” terms” [ 20 ]. In a more practical sense, coding makes raw data sortable. This makes it possible to extract and examine all segments describing, say, a tele-neurology consultation from multiple data sources (e.g. SOPs, emergency room observations, staff and patient interview). In a process of synthesis and abstraction, the codes are then grouped, summarised and/or categorised [ 15 , 20 ]. The end product of the coding or analysis process is a descriptive theory of the behavioural pattern under investigation [ 20 ]. The coding process is performed using qualitative data management software, the most common ones being InVivo, MaxQDA and Atlas.ti. It should be noted that these are data management tools which support the analysis performed by the researcher(s) [ 14 ].

From data collection to data analysis
Attributions for icons: see Fig. Fig.2, 2 , also “Speech to text” by Trevor Dsouza, “Field Notes” by Mike O’Brien, US, “Voice Record” by ProSymbols, US, “Inspection” by Made, AU, and “Cloud” by Graphic Tigers; all from the Noun Project
How to report qualitative research?
Protocols of qualitative research can be published separately and in advance of the study results. However, the aim is not the same as in RCT protocols, i.e. to pre-define and set in stone the research questions and primary or secondary endpoints. Rather, it is a way to describe the research methods in detail, which might not be possible in the results paper given journals’ word limits. Qualitative research papers are usually longer than their quantitative counterparts to allow for deep understanding and so-called “thick description”. In the methods section, the focus is on transparency of the methods used, including why, how and by whom they were implemented in the specific study setting, so as to enable a discussion of whether and how this may have influenced data collection, analysis and interpretation. The results section usually starts with a paragraph outlining the main findings, followed by more detailed descriptions of, for example, the commonalities, discrepancies or exceptions per category [ 20 ]. Here it is important to support main findings by relevant quotations, which may add information, context, emphasis or real-life examples [ 20 , 23 ]. It is subject to debate in the field whether it is relevant to state the exact number or percentage of respondents supporting a certain statement (e.g. “Five interviewees expressed negative feelings towards XYZ”) [ 21 ].
How to combine qualitative with quantitative research?
Qualitative methods can be combined with other methods in multi- or mixed methods designs, which “[employ] two or more different methods [ …] within the same study or research program rather than confining the research to one single method” [ 24 ]. Reasons for combining methods can be diverse, including triangulation for corroboration of findings, complementarity for illustration and clarification of results, expansion to extend the breadth and range of the study, explanation of (unexpected) results generated with one method with the help of another, or offsetting the weakness of one method with the strength of another [ 1 , 17 , 24 – 26 ]. The resulting designs can be classified according to when, why and how the different quantitative and/or qualitative data strands are combined. The three most common types of mixed method designs are the convergent parallel design , the explanatory sequential design and the exploratory sequential design. The designs with examples are shown in Fig. 4 .

Three common mixed methods designs
In the convergent parallel design, a qualitative study is conducted in parallel to and independently of a quantitative study, and the results of both studies are compared and combined at the stage of interpretation of results. Using the above example of EVT provision, this could entail setting up a quantitative EVT registry to measure process times and patient outcomes in parallel to conducting the qualitative research outlined above, and then comparing results. Amongst other things, this would make it possible to assess whether interview respondents’ subjective impressions of patients receiving good care match modified Rankin Scores at follow-up, or whether observed delays in care provision are exceptions or the rule when compared to door-to-needle times as documented in the registry. In the explanatory sequential design, a quantitative study is carried out first, followed by a qualitative study to help explain the results from the quantitative study. This would be an appropriate design if the registry alone had revealed relevant delays in door-to-needle times and the qualitative study would be used to understand where and why these occurred, and how they could be improved. In the exploratory design, the qualitative study is carried out first and its results help informing and building the quantitative study in the next step [ 26 ]. If the qualitative study around EVT provision had shown a high level of dissatisfaction among the staff members involved, a quantitative questionnaire investigating staff satisfaction could be set up in the next step, informed by the qualitative study on which topics dissatisfaction had been expressed. Amongst other things, the questionnaire design would make it possible to widen the reach of the research to more respondents from different (types of) hospitals, regions, countries or settings, and to conduct sub-group analyses for different professional groups.
How to assess qualitative research?
A variety of assessment criteria and lists have been developed for qualitative research, ranging in their focus and comprehensiveness [ 14 , 17 , 27 ]. However, none of these has been elevated to the “gold standard” in the field. In the following, we therefore focus on a set of commonly used assessment criteria that, from a practical standpoint, a researcher can look for when assessing a qualitative research report or paper.
Assessors should check the authors’ use of and adherence to the relevant reporting checklists (e.g. Standards for Reporting Qualitative Research (SRQR)) to make sure all items that are relevant for this type of research are addressed [ 23 , 28 ]. Discussions of quantitative measures in addition to or instead of these qualitative measures can be a sign of lower quality of the research (paper). Providing and adhering to a checklist for qualitative research contributes to an important quality criterion for qualitative research, namely transparency [ 15 , 17 , 23 ].
Reflexivity
While methodological transparency and complete reporting is relevant for all types of research, some additional criteria must be taken into account for qualitative research. This includes what is called reflexivity, i.e. sensitivity to the relationship between the researcher and the researched, including how contact was established and maintained, or the background and experience of the researcher(s) involved in data collection and analysis. Depending on the research question and population to be researched this can be limited to professional experience, but it may also include gender, age or ethnicity [ 17 , 27 ]. These details are relevant because in qualitative research, as opposed to quantitative research, the researcher as a person cannot be isolated from the research process [ 23 ]. It may influence the conversation when an interviewed patient speaks to an interviewer who is a physician, or when an interviewee is asked to discuss a gynaecological procedure with a male interviewer, and therefore the reader must be made aware of these details [ 19 ].
Sampling and saturation
The aim of qualitative sampling is for all variants of the objects of observation that are deemed relevant for the study to be present in the sample “ to see the issue and its meanings from as many angles as possible” [ 1 , 16 , 19 , 20 , 27 ] , and to ensure “information-richness [ 15 ]. An iterative sampling approach is advised, in which data collection (e.g. five interviews) is followed by data analysis, followed by more data collection to find variants that are lacking in the current sample. This process continues until no new (relevant) information can be found and further sampling becomes redundant – which is called saturation [ 1 , 15 ] . In other words: qualitative data collection finds its end point not a priori , but when the research team determines that saturation has been reached [ 29 , 30 ].
This is also the reason why most qualitative studies use deliberate instead of random sampling strategies. This is generally referred to as “ purposive sampling” , in which researchers pre-define which types of participants or cases they need to include so as to cover all variations that are expected to be of relevance, based on the literature, previous experience or theory (i.e. theoretical sampling) [ 14 , 20 ]. Other types of purposive sampling include (but are not limited to) maximum variation sampling, critical case sampling or extreme or deviant case sampling [ 2 ]. In the above EVT example, a purposive sample could include all relevant professional groups and/or all relevant stakeholders (patients, relatives) and/or all relevant times of observation (day, night and weekend shift).
Assessors of qualitative research should check whether the considerations underlying the sampling strategy were sound and whether or how researchers tried to adapt and improve their strategies in stepwise or cyclical approaches between data collection and analysis to achieve saturation [ 14 ].
Good qualitative research is iterative in nature, i.e. it goes back and forth between data collection and analysis, revising and improving the approach where necessary. One example of this are pilot interviews, where different aspects of the interview (especially the interview guide, but also, for example, the site of the interview or whether the interview can be audio-recorded) are tested with a small number of respondents, evaluated and revised [ 19 ]. In doing so, the interviewer learns which wording or types of questions work best, or which is the best length of an interview with patients who have trouble concentrating for an extended time. Of course, the same reasoning applies to observations or focus groups which can also be piloted.
Ideally, coding should be performed by at least two researchers, especially at the beginning of the coding process when a common approach must be defined, including the establishment of a useful coding list (or tree), and when a common meaning of individual codes must be established [ 23 ]. An initial sub-set or all transcripts can be coded independently by the coders and then compared and consolidated after regular discussions in the research team. This is to make sure that codes are applied consistently to the research data.
Member checking
Member checking, also called respondent validation , refers to the practice of checking back with study respondents to see if the research is in line with their views [ 14 , 27 ]. This can happen after data collection or analysis or when first results are available [ 23 ]. For example, interviewees can be provided with (summaries of) their transcripts and asked whether they believe this to be a complete representation of their views or whether they would like to clarify or elaborate on their responses [ 17 ]. Respondents’ feedback on these issues then becomes part of the data collection and analysis [ 27 ].
Stakeholder involvement
In those niches where qualitative approaches have been able to evolve and grow, a new trend has seen the inclusion of patients and their representatives not only as study participants (i.e. “members”, see above) but as consultants to and active participants in the broader research process [ 31 – 33 ]. The underlying assumption is that patients and other stakeholders hold unique perspectives and experiences that add value beyond their own single story, making the research more relevant and beneficial to researchers, study participants and (future) patients alike [ 34 , 35 ]. Using the example of patients on or nearing dialysis, a recent scoping review found that 80% of clinical research did not address the top 10 research priorities identified by patients and caregivers [ 32 , 36 ]. In this sense, the involvement of the relevant stakeholders, especially patients and relatives, is increasingly being seen as a quality indicator in and of itself.
How not to assess qualitative research
The above overview does not include certain items that are routine in assessments of quantitative research. What follows is a non-exhaustive, non-representative, experience-based list of the quantitative criteria often applied to the assessment of qualitative research, as well as an explanation of the limited usefulness of these endeavours.
Protocol adherence
Given the openness and flexibility of qualitative research, it should not be assessed by how well it adheres to pre-determined and fixed strategies – in other words: its rigidity. Instead, the assessor should look for signs of adaptation and refinement based on lessons learned from earlier steps in the research process.
Sample size
For the reasons explained above, qualitative research does not require specific sample sizes, nor does it require that the sample size be determined a priori [ 1 , 14 , 27 , 37 – 39 ]. Sample size can only be a useful quality indicator when related to the research purpose, the chosen methodology and the composition of the sample, i.e. who was included and why.
Randomisation
While some authors argue that randomisation can be used in qualitative research, this is not commonly the case, as neither its feasibility nor its necessity or usefulness has been convincingly established for qualitative research [ 13 , 27 ]. Relevant disadvantages include the negative impact of a too large sample size as well as the possibility (or probability) of selecting “ quiet, uncooperative or inarticulate individuals ” [ 17 ]. Qualitative studies do not use control groups, either.
Interrater reliability, variability and other “objectivity checks”
The concept of “interrater reliability” is sometimes used in qualitative research to assess to which extent the coding approach overlaps between the two co-coders. However, it is not clear what this measure tells us about the quality of the analysis [ 23 ]. This means that these scores can be included in qualitative research reports, preferably with some additional information on what the score means for the analysis, but it is not a requirement. Relatedly, it is not relevant for the quality or “objectivity” of qualitative research to separate those who recruited the study participants and collected and analysed the data. Experiences even show that it might be better to have the same person or team perform all of these tasks [ 20 ]. First, when researchers introduce themselves during recruitment this can enhance trust when the interview takes place days or weeks later with the same researcher. Second, when the audio-recording is transcribed for analysis, the researcher conducting the interviews will usually remember the interviewee and the specific interview situation during data analysis. This might be helpful in providing additional context information for interpretation of data, e.g. on whether something might have been meant as a joke [ 18 ].
Not being quantitative research
Being qualitative research instead of quantitative research should not be used as an assessment criterion if it is used irrespectively of the research problem at hand. Similarly, qualitative research should not be required to be combined with quantitative research per se – unless mixed methods research is judged as inherently better than single-method research. In this case, the same criterion should be applied for quantitative studies without a qualitative component.
The main take-away points of this paper are summarised in Table Table1. 1 . We aimed to show that, if conducted well, qualitative research can answer specific research questions that cannot to be adequately answered using (only) quantitative designs. Seeing qualitative and quantitative methods as equal will help us become more aware and critical of the “fit” between the research problem and our chosen methods: I can conduct an RCT to determine the reasons for transportation delays of acute stroke patients – but should I? It also provides us with a greater range of tools to tackle a greater range of research problems more appropriately and successfully, filling in the blind spots on one half of the methodological spectrum to better address the whole complexity of neurological research and practice.
Take-away-points
• Assessing complex multi-component interventions or systems (of change) • What works for whom when, how and why? • Focussing on intervention improvement | • Document study • Observations (participant or non-participant) • Interviews (especially semi-structured) • Focus groups | • Transcription of audio-recordings and field notes into transcripts and protocols • Coding of protocols • Using qualitative data management software |
• Combinations of quantitative and/or qualitative methods, e.g.: • : quali and quanti in parallel • : quanti followed by quali • : quali followed by quanti | • Checklists • Reflexivity • Sampling strategies • Piloting • Co-coding • Member checking • Stakeholder involvement | • Protocol adherence • Sample size • Randomization • Interrater reliability, variability and other “objectivity checks” • Not being quantitative research |
Acknowledgements
Abbreviations.
EVT | Endovascular treatment |
RCT | Randomised Controlled Trial |
SOP | Standard Operating Procedure |
SRQR | Standards for Reporting Qualitative Research |
Authors’ contributions
LB drafted the manuscript; WW and CG revised the manuscript; all authors approved the final versions.
no external funding.
Availability of data and materials
Ethics approval and consent to participate, consent for publication, competing interests.
The authors declare no competing interests.
Publisher’s Note
Springer Nature remains neutral with regard to jurisdictional claims in published maps and institutional affiliations.

Home > Blog >
Data analysis in qualitative research, theertha raj, august 30, 2024.
While numbers tell us "what" and "how much," qualitative data reveals the crucial "why" and "how." But let's face it - turning mountains of text, images, and observations into meaningful insights can be daunting.
This guide dives deep into the art and science of how to analyze qualitative data. We'll explore cutting-edge techniques, free qualitative data analysis software, and strategies to make your analysis more rigorous and insightful. Expect practical, actionable advice on qualitative data analysis methods, whether you're a seasoned researcher looking to refine your skills or a team leader aiming to extract more value from your qualitative data.
What is qualitative data?
Qualitative data is non-numerical information that describes qualities or characteristics. It includes text, images, audio, and video.
This data type captures complex human experiences, behaviors, and opinions that numbers alone can't express.
A qualitative data example can include interview transcripts, open-ended survey responses, field notes from observations, social media posts and customer reviews
Importance of qualitative data
Qualitative data is vital for several reasons:
- It provides a deep, nuanced understanding of complex phenomena.
- It captures the 'why' behind behaviors and opinions.
- It allows for unexpected discoveries and new research directions.
- It puts people's experiences and perspectives at the forefront.
- It enhances quantitative findings with depth and detail.
What is data analysis in qualitative research?
Data analysis in qualitative research is the process of examining and interpreting non-numerical data to uncover patterns, themes, and insights. It aims to make sense of rich, detailed information gathered through methods like interviews, focus groups, or observations.
This analysis moves beyond simple description. It seeks to understand the underlying meanings, contexts, and relationships within the data. The goal is to create a coherent narrative that answers research questions and generates new knowledge.
How is qualitative data analysis different from quantitative data analysis?
Qualitative and quantitative data analyses differ in several key ways:
- Data type: Qualitative analysis uses non-numerical data (text, images), while quantitative analysis uses numerical data.
- Approach: Qualitative analysis is inductive and exploratory. Quantitative analysis is deductive and confirmatory.
- Sample size: Qualitative studies often use smaller samples. Quantitative studies typically need larger samples for statistical validity.
- Depth vs. breadth: Qualitative analysis provides in-depth insights about a few cases. Quantitative analysis offers broader insights across many cases.
- Subjectivity: Qualitative analysis involves more subjective interpretation. Quantitative analysis aims for objective, statistical measures.
What are the 3 main components of qualitative data analysis?
The three main components of qualitative data analysis are:
- Data reduction: Simplifying and focusing the raw data through coding and categorization.
- Data display: Organizing the reduced data into visual formats like matrices, charts, or networks.
- Conclusion drawing/verification: Interpreting the displayed data and verifying the conclusions.
These components aren't linear steps. Instead, they form an iterative process where researchers move back and forth between them throughout the analysis.
How do you write a qualitative analysis?
Step 1: organize your data.
Start with bringing all your qualitative research data in one place. A repository can be of immense help here. Transcribe interviews , compile field notes, and gather all relevant materials.
Immerse yourself in the data. Read through everything multiple times.
Step 2: Code & identify themes
Identify and label key concepts, themes, or patterns. Group related codes into broader themes or categories. Try to connect themes to tell a coherent story that answers your research questions.
Pick out direct quotes from your data to illustrate key points.
Step 3: Interpret and reflect
Explain what your results mean in the context of your research and existing literature.
Als discuss, identify and try to eliminate potential biases or limitations in your analysis.
Summarize main insights and their implications.
What are the 5 qualitative data analysis methods?
Thematic Analysis Identifying, analyzing, and reporting patterns (themes) within data.
Content Analysis Systematically categorizing and counting the occurrence of specific elements in text.
Grounded Theory Developing theory from data through iterative coding and analysis.
Discourse Analysis Examining language use and meaning in social contexts.
Narrative Analysis Interpreting stories and personal accounts to understand experiences and meanings.
Each method suits different research goals and data types. Researchers often combine methods for comprehensive analysis.
What are the 4 data collection methods in qualitative research?
When it comes to collecting qualitative data, researchers primarily rely on four methods.
- Interviews : One-on-one conversations to gather in-depth information.
- Focus Groups : Group discussions to explore collective opinions and experiences.
- Observations : Watching and recording behaviors in natural settings.
- Document Analysis : Examining existing texts, images, or artifacts.
Researchers often use multiple methods to gain a comprehensive understanding of their topic.
How is qualitative data analysis measured?
Unlike quantitative data, qualitative data analysis isn't measured in traditional numerical terms. Instead, its quality is evaluated based on several criteria.
Trustworthiness is key, encompassing the credibility, transferability, dependability, and confirmability of the findings. The rigor of the analysis - the thoroughness and care taken in data collection and analysis - is another crucial factor.
Transparency in documenting the analysis process and decision-making is essential, as is reflexivity - acknowledging and examining the researcher's own biases and influences.
Employing techniques like member checking and triangulation all contribute to the strength of qualitative analysis.
Benefits of qualitative data analysis
The benefits of qualitative data analysis are numerous. It uncovers rich, nuanced understanding of complex phenomena and allows for unexpected discoveries and new research directions.
By capturing the 'why' behind behaviors and opinions, qualitative data analysis methods provide crucial context.
Qualitative analysis can also lead to new theoretical frameworks or hypotheses and enhances quantitative findings with depth and detail. It's particularly adept at capturing cultural nuances that might be missed in quantitative studies.
Challenges of Qualitative Data Analysis
Researchers face several challenges when conducting qualitative data analysis.
Managing and making sense of large volumes of rich, complex data can lead to data overload. Maintaining consistent coding across large datasets or between multiple coders can be difficult.
There's a delicate balance to strike between providing enough context and maintaining focus on analysis. Recognizing and mitigating researcher biases in data interpretation is an ongoing challenge.
The learning curve for qualitative data analysis software can be steep and time-consuming. Ethical considerations, particularly around protecting participant anonymity while presenting rich, detailed data, require careful navigation. Integrating different types of data from various sources can be complex. Time management is crucial, as researchers must balance the depth of analysis with project timelines and resources. Finally, communicating complex qualitative insights in clear, compelling ways can be challenging.
Best Software to Analyze Qualitative Data
G2 rating: 4.6/5
Pricing: Starts at $30 monthly.
Looppanel is an AI-powered research assistant and repository platform that can make it 5x faster to get to insights, by automating all the manual, tedious parts of your job.
Here’s how Looppanel’s features can help with qualitative data analysis:
- Automatic Transcription: Quickly turn speech into accurate text; it works across 8 languages and even heavy accents, with over 90% accuracy.
- AI Note-Taking: The research assistant can join you on calls and take notes, as well as automatically sort your notes based on your interview questions.
- Automatic Tagging: Easily tag and organize your data with free AI tools.
- Insight Generation: Create shareable insights that fit right into your other tools.
- Repository Search: Run Google-like searches within your projects and calls to find a data snippet/quote in seconds
- Smart Summary: Ask the AI a question on your research, and it will give you an answer, using extracts from your data as citations.
Looppanel’s focus on automating research tasks makes it perfect for researchers who want to save time and work smarter.
G2 rating: 4.7/5
Pricing: Free version available, with the Plus version costing $20 monthly.
ChatGPT, developed by OpenAI, offers a range of capabilities for qualitative data analysis including:
- Document analysis : It can easily extract and analyze text from various file formats.
- Summarization : GPT can condense lengthy documents into concise summaries.
- Advanced Data Analysis (ADA) : For paid users, Chat-GPT offers quantitative analysis of data documents.
- Sentiment analysis: Although not Chat-GPT’s specialty, it can still perform basic sentiment analysis on text data.
ChatGPT's versatility makes it valuable for researchers who need quick insights from diverse text sources.
How to use ChatGPT for qualitative data analysis
ChatGPT can be a handy sidekick in your qualitative analysis, if you do the following:
- Use it to summarize long documents or transcripts
- Ask it to identify key themes in your data
- Use it for basic sentiment analysis
- Have it generate potential codes based on your research questions
- Use it to brainstorm interpretations of your findings
G2 rating: 4.7/5 Pricing: Custom
Atlas.ti is a powerful platform built for detailed qualitative and mixed-methods research, offering a lot of capabilities for running both quantitative and qualitative research.
It’s key data analysis features include:
- Multi-format Support: Analyze text, PDFs, images, audio, video, and geo data all within one platform.
- AI-Powered Coding: Uses AI to suggest codes and summarize documents.
- Collaboration Tools: Ideal for teams working on complex research projects.
- Data Visualization: Create network views and other visualizations to showcase relationships in your data.
G2 rating: 4.1/5 Pricing: Custom
NVivo is another powerful platform for qualitative and mixed-methods research. It’s analysis features include:
- Data Import and Organization: Easily manage different data types, including text, audio, and video.
- AI-Powered Coding: Speeds up the coding process with machine learning.
- Visualization Tools: Create charts, graphs, and diagrams to represent your findings.
- Collaboration Features: Suitable for team-based research projects.
NVivo combines AI capabilities with traditional qualitative analysis tools, making it versatile for various research needs.
Can Excel do qualitative data analysis?
Excel can be a handy tool for qualitative data analysis, especially if you're just starting out or working on a smaller project. While it's not specialized qualitative data analysis software, you can use it to organize your data, maybe putting different themes in different columns. It's good for basic coding, where you label bits of text with keywords. You can use its filter feature to focus on specific themes. Excel can also create simple charts to visualize your findings. But for bigger or more complex projects, you might want to look into software designed specifically for qualitative data analysis. These tools often have more advanced features that can save you time and help you dig deeper into your data.
How do you show qualitative analysis?
Showing qualitative data analysis is about telling the story of your data. In qualitative data analysis methods, we use quotes from interviews or documents to back up our points. Create charts or mind maps to show how different ideas connect, which is a common practice in data analysis in qualitative research. Group your findings into themes that make sense. Then, write it all up in a way that flows, explaining what you found and why it matters.
What is the best way to analyze qualitative data?
There's no one-size-fits-all approach to how to analyze qualitative data, but there are some tried-and-true steps.
Start by getting your data in order. Then, read through it a few times to get familiar with it. As you go, start marking important bits with codes - this is a fundamental qualitative data analysis method. Group similar codes into bigger themes. Look for patterns in these themes - how do they connect?
Finally, think about what it all means in the bigger picture of your research. Remember, it's okay to go back and forth between these steps as you dig deeper into your data. Qualitative data analysis software can be a big help in this process, especially for managing large amounts of data.
In qualitative methods of test analysis, what do test developers do to generate data?
Test developers in qualitative research might sit down with people for in-depth chats or run group discussions, which are key qualitative data analysis methods. They often use surveys with open-ended questions that let people express themselves freely. Sometimes, they'll observe people in their natural environment, taking notes on what they see. They might also dig into existing documents or artifacts that relate to their topic. The goal is to gather rich, detailed information that helps them understand the full picture, which is crucial in data analysis in qualitative research.
Which is not a purpose of reflexivity during qualitative data analysis?
Reflexivity in qualitative data analysis isn't about proving you're completely objective. That's not the goal. Instead, it's about being honest about who you are as a researcher. It's recognizing that your own experiences and views might influence how you see the data. By being upfront about this, you actually make your research more trustworthy. It's also a way to dig deeper into your data, seeing things you might have missed at first glance. This self-awareness is a crucial part of qualitative data analysis methods.
What is a qualitative data analysis example?
A simple example is analyzing customer feedback for a new product. You might collect feedback, read through responses, create codes like "ease of use" or "design," and group similar codes into themes. You'd then identify patterns and support findings with specific quotes. This process helps transform raw feedback into actionable insights.
How to analyze qualitative data from a survey?
First, gather all your responses in one place. Read through them to get a feel for what people are saying. Then, start labeling responses with codes - short descriptions of what each bit is about. This coding process is a fundamental qualitative data analysis method. Group similar codes into bigger themes. Look for patterns in these themes. Are certain ideas coming up a lot? Do different groups of people have different views? Use actual quotes from your survey to back up what you're seeing. Think about how your findings relate to your original research questions.
Which one is better, NVivo or Atlas.ti?
NVivo is known for being user-friendly and great for team projects. Atlas.ti shines when it comes to visual mapping of concepts and handling geographic data. Both can handle a variety of data types and have powerful tools for qualitative data analysis. The best way to decide is to try out both if you can.
While these are powerful tools, the core of qualitative data analysis still relies on your analytical skills and understanding of qualitative data analysis methods.
Do I need to use NVivo for qualitative data analysis?
You don't necessarily need NVivo for qualitative data analysis, but it can definitely make your life easier, especially for bigger projects. Think of it like using a power tool versus a hand tool - you can get the job done either way, but the power tool might save you time and effort. For smaller projects or if you're just starting out, you might be fine with simpler tools or even free qualitative data analysis software. But if you're dealing with lots of data, or if you need to collaborate with a team, or if you want to do more complex analysis, then specialized qualitative data analysis software like NVivo can be a big help. It's all about finding the right tool for your specific research needs and the qualitative data analysis methods you're using.
Here’s a guide that can help you decide.
How to use NVivo for qualitative data analysis
First, you import all your data - interviews, documents, videos, whatever you've got. Then you start creating "nodes," which are like folders for different themes or ideas in your data. As you read through your material, you highlight bits that relate to these themes and file them under the right nodes. NVivo lets you easily search through all this organized data, find connections between different themes, and even create visual maps of how everything relates.
How much does NVivo cost?
NVivo's pricing isn't one-size-fits-all. They offer different plans for individuals, teams, and large organizations, but they don't publish their prices openly. Contact the team here for a custom quote.
What are the four steps of qualitative data analysis?
While qualitative data analysis is often iterative, it generally follows these four main steps:
1. Data Collection: Gathering raw data through interviews, observations, or documents.
2. Data Preparation: Organizing and transcribing the collected data.
3. Data Coding: Identifying and labeling important concepts or themes in the data.
4. Interpretation: Drawing meaning from the coded data and developing insights.
Follow us on
Get the best resources for ux research, in your inbox, related articles.
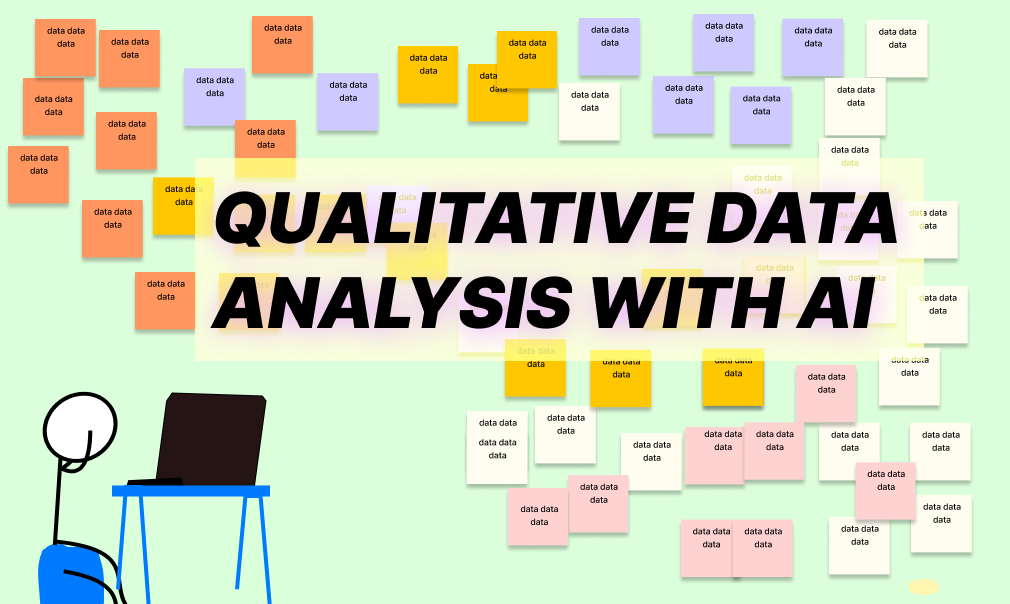
Resources & Guides
February 15, 2024
How to use AI for Qualitative Data Analysis

August 15, 2024
Transcription in Qualitative Research: A Comprehensive Guide for UX Researchers
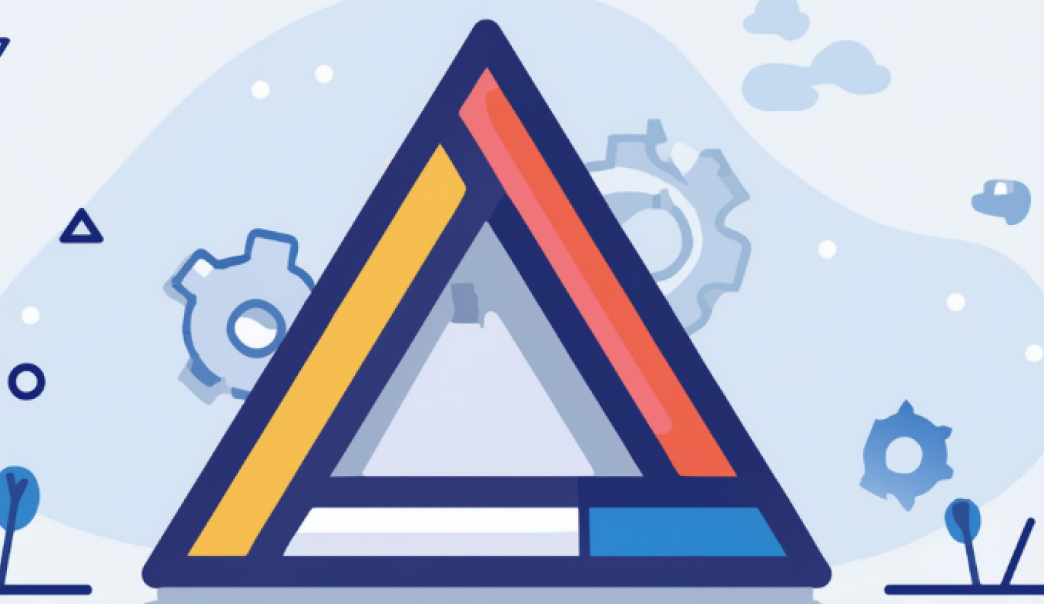
May 22, 2024
Triangulation in Qualitative Research: A Comprehensive Guide [2024]
Looppanel automatically records your calls, transcribes them, and centralizes all your research data in one place
Thank you for visiting nature.com. You are using a browser version with limited support for CSS. To obtain the best experience, we recommend you use a more up to date browser (or turn off compatibility mode in Internet Explorer). In the meantime, to ensure continued support, we are displaying the site without styles and JavaScript.
- View all journals
- Explore content
- About the journal
- Publish with us
- Sign up for alerts
- Open access
- Published: 31 August 2024
Knowledge mapping and evolution of research on older adults’ technology acceptance: a bibliometric study from 2013 to 2023
- Xianru Shang ORCID: orcid.org/0009-0000-8906-3216 1 ,
- Zijian Liu 1 ,
- Chen Gong 1 ,
- Zhigang Hu 1 ,
- Yuexuan Wu 1 &
- Chengliang Wang ORCID: orcid.org/0000-0003-2208-3508 2
Humanities and Social Sciences Communications volume 11 , Article number: 1115 ( 2024 ) Cite this article
Metrics details
- Science, technology and society
The rapid expansion of information technology and the intensification of population aging are two prominent features of contemporary societal development. Investigating older adults’ acceptance and use of technology is key to facilitating their integration into an information-driven society. Given this context, the technology acceptance of older adults has emerged as a prioritized research topic, attracting widespread attention in the academic community. However, existing research remains fragmented and lacks a systematic framework. To address this gap, we employed bibliometric methods, utilizing the Web of Science Core Collection to conduct a comprehensive review of literature on older adults’ technology acceptance from 2013 to 2023. Utilizing VOSviewer and CiteSpace for data assessment and visualization, we created knowledge mappings of research on older adults’ technology acceptance. Our study employed multidimensional methods such as co-occurrence analysis, clustering, and burst analysis to: (1) reveal research dynamics, key journals, and domains in this field; (2) identify leading countries, their collaborative networks, and core research institutions and authors; (3) recognize the foundational knowledge system centered on theoretical model deepening, emerging technology applications, and research methods and evaluation, uncovering seminal literature and observing a shift from early theoretical and influential factor analyses to empirical studies focusing on individual factors and emerging technologies; (4) moreover, current research hotspots are primarily in the areas of factors influencing technology adoption, human-robot interaction experiences, mobile health management, and aging-in-place technology, highlighting the evolutionary context and quality distribution of research themes. Finally, we recommend that future research should deeply explore improvements in theoretical models, long-term usage, and user experience evaluation. Overall, this study presents a clear framework of existing research in the field of older adults’ technology acceptance, providing an important reference for future theoretical exploration and innovative applications.
Similar content being viewed by others
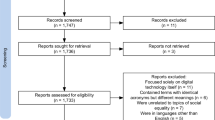
Research progress and intellectual structure of design for digital equity (DDE): A bibliometric analysis based on citespace
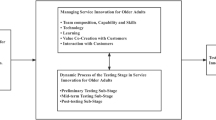
Exploring the role of interaction in older-adult service innovation: insights from the testing stage
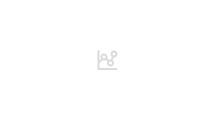
Smart device interest, perceived usefulness, and preferences in rural Alabama seniors
Introduction.
In contemporary society, the rapid development of information technology has been intricately intertwined with the intensifying trend of population aging. According to the latest United Nations forecast, by 2050, the global population aged 65 and above is expected to reach 1.6 billion, representing about 16% of the total global population (UN 2023 ). Given the significant challenges of global aging, there is increasing evidence that emerging technologies have significant potential to maintain health and independence for older adults in their home and healthcare environments (Barnard et al. 2013 ; Soar 2010 ; Vancea and Solé-Casals 2016 ). This includes, but is not limited to, enhancing residential safety with smart home technologies (Touqeer et al. 2021 ; Wang et al. 2022 ), improving living independence through wearable technologies (Perez et al. 2023 ), and increasing medical accessibility via telehealth services (Kruse et al. 2020 ). Technological innovations are redefining the lifestyles of older adults, encouraging a shift from passive to active participation (González et al. 2012 ; Mostaghel 2016 ). Nevertheless, the effective application and dissemination of technology still depends on user acceptance and usage intentions (Naseri et al. 2023 ; Wang et al. 2023a ; Xia et al. 2024 ; Yu et al. 2023 ). Particularly, older adults face numerous challenges in accepting and using new technologies. These challenges include not only physical and cognitive limitations but also a lack of technological experience, along with the influences of social and economic factors (Valk et al. 2018 ; Wilson et al. 2021 ).
User acceptance of technology is a significant focus within information systems (IS) research (Dai et al. 2024 ), with several models developed to explain and predict user behavior towards technology usage, including the Technology Acceptance Model (TAM) (Davis 1989 ), TAM2, TAM3, and the Unified Theory of Acceptance and Use of Technology (UTAUT) (Venkatesh et al. 2003 ). Older adults, as a group with unique needs, exhibit different behavioral patterns during technology acceptance than other user groups, and these uniquenesses include changes in cognitive abilities, as well as motivations, attitudes, and perceptions of the use of new technologies (Chen and Chan 2011 ). The continual expansion of technology introduces considerable challenges for older adults, rendering the understanding of their technology acceptance a research priority. Thus, conducting in-depth research into older adults’ acceptance of technology is critically important for enhancing their integration into the information society and improving their quality of life through technological advancements.
Reviewing relevant literature to identify research gaps helps further solidify the theoretical foundation of the research topic. However, many existing literature reviews primarily focus on the factors influencing older adults’ acceptance or intentions to use technology. For instance, Ma et al. ( 2021 ) conducted a comprehensive analysis of the determinants of older adults’ behavioral intentions to use technology; Liu et al. ( 2022 ) categorized key variables in studies of older adults’ technology acceptance, noting a shift in focus towards social and emotional factors; Yap et al. ( 2022 ) identified seven categories of antecedents affecting older adults’ use of technology from an analysis of 26 articles, including technological, psychological, social, personal, cost, behavioral, and environmental factors; Schroeder et al. ( 2023 ) extracted 119 influencing factors from 59 articles and further categorized these into six themes covering demographics, health status, and emotional awareness. Additionally, some studies focus on the application of specific technologies, such as Ferguson et al. ( 2021 ), who explored barriers and facilitators to older adults using wearable devices for heart monitoring, and He et al. ( 2022 ) and Baer et al. ( 2022 ), who each conducted in-depth investigations into the acceptance of social assistive robots and mobile nutrition and fitness apps, respectively. In summary, current literature reviews on older adults’ technology acceptance exhibit certain limitations. Due to the interdisciplinary nature and complex knowledge structure of this field, traditional literature reviews often rely on qualitative analysis, based on literature analysis and periodic summaries, which lack sufficient objectivity and comprehensiveness. Additionally, systematic research is relatively limited, lacking a macroscopic description of the research trajectory from a holistic perspective. Over the past decade, research on older adults’ technology acceptance has experienced rapid growth, with a significant increase in literature, necessitating the adoption of new methods to review and examine the developmental trends in this field (Chen 2006 ; Van Eck and Waltman 2010 ). Bibliometric analysis, as an effective quantitative research method, analyzes published literature through visualization, offering a viable approach to extracting patterns and insights from a large volume of papers, and has been widely applied in numerous scientific research fields (Achuthan et al. 2023 ; Liu and Duffy 2023 ). Therefore, this study will employ bibliometric methods to systematically analyze research articles related to older adults’ technology acceptance published in the Web of Science Core Collection from 2013 to 2023, aiming to understand the core issues and evolutionary trends in the field, and to provide valuable references for future related research. Specifically, this study aims to explore and answer the following questions:
RQ1: What are the research dynamics in the field of older adults’ technology acceptance over the past decade? What are the main academic journals and fields that publish studies related to older adults’ technology acceptance?
RQ2: How is the productivity in older adults’ technology acceptance research distributed among countries, institutions, and authors?
RQ3: What are the knowledge base and seminal literature in older adults’ technology acceptance research? How has the research theme progressed?
RQ4: What are the current hot topics and their evolutionary trajectories in older adults’ technology acceptance research? How is the quality of research distributed?
Methodology and materials
Research method.
In recent years, bibliometrics has become one of the crucial methods for analyzing literature reviews and is widely used in disciplinary and industrial intelligence analysis (Jing et al. 2023 ; Lin and Yu 2024a ; Wang et al. 2024a ; Xu et al. 2021 ). Bibliometric software facilitates the visualization analysis of extensive literature data, intuitively displaying the network relationships and evolutionary processes between knowledge units, and revealing the underlying knowledge structure and potential information (Chen et al. 2024 ; López-Robles et al. 2018 ; Wang et al. 2024c ). This method provides new insights into the current status and trends of specific research areas, along with quantitative evidence, thereby enhancing the objectivity and scientific validity of the research conclusions (Chen et al. 2023 ; Geng et al. 2024 ). VOSviewer and CiteSpace are two widely used bibliometric software tools in academia (Pan et al. 2018 ), recognized for their robust functionalities based on the JAVA platform. Although each has its unique features, combining these two software tools effectively constructs mapping relationships between literature knowledge units and clearly displays the macrostructure of the knowledge domains. Particularly, VOSviewer, with its excellent graphical representation capabilities, serves as an ideal tool for handling large datasets and precisely identifying the focal points and hotspots of research topics. Therefore, this study utilizes VOSviewer (version 1.6.19) and CiteSpace (version 6.1.R6), combined with in-depth literature analysis, to comprehensively examine and interpret the research theme of older adults’ technology acceptance through an integrated application of quantitative and qualitative methods.
Data source
Web of Science is a comprehensively recognized database in academia, featuring literature that has undergone rigorous peer review and editorial scrutiny (Lin and Yu 2024b ; Mongeon and Paul-Hus 2016 ; Pranckutė 2021 ). This study utilizes the Web of Science Core Collection as its data source, specifically including three major citation indices: Science Citation Index Expanded (SCIE), Social Sciences Citation Index (SSCI), and Arts & Humanities Citation Index (A&HCI). These indices encompass high-quality research literature in the fields of science, social sciences, and arts and humanities, ensuring the comprehensiveness and reliability of the data. We combined “older adults” with “technology acceptance” through thematic search, with the specific search strategy being: TS = (elder OR elderly OR aging OR ageing OR senile OR senior OR old people OR “older adult*”) AND TS = (“technology acceptance” OR “user acceptance” OR “consumer acceptance”). The time span of literature search is from 2013 to 2023, with the types limited to “Article” and “Review” and the language to “English”. Additionally, the search was completed by October 27, 2023, to avoid data discrepancies caused by database updates. The initial search yielded 764 journal articles. Given that searches often retrieve articles that are superficially relevant but actually non-compliant, manual screening post-search was essential to ensure the relevance of the literature (Chen et al. 2024 ). Through manual screening, articles significantly deviating from the research theme were eliminated and rigorously reviewed. Ultimately, this study obtained 500 valid sample articles from the Web of Science Core Collection. The complete PRISMA screening process is illustrated in Fig. 1 .
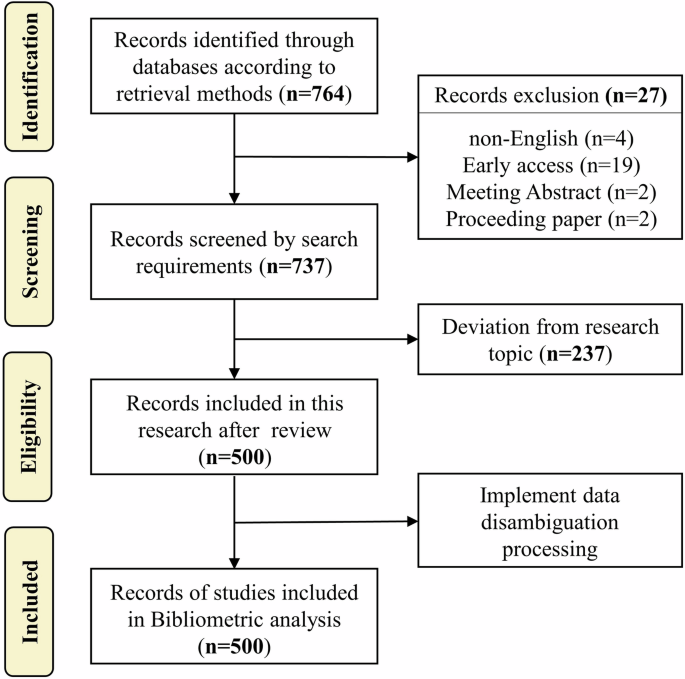
Presentation of the data culling process in detail.
Data standardization
Raw data exported from databases often contain multiple expressions of the same terminology (Nguyen and Hallinger 2020 ). To ensure the accuracy and consistency of data, it is necessary to standardize the raw data (Strotmann and Zhao 2012 ). This study follows the data standardization process proposed by Taskin and Al ( 2019 ), mainly executing the following operations:
(1) Standardization of author and institution names is conducted to address different name expressions for the same author. For instance, “Chan, Alan Hoi Shou” and “Chan, Alan H. S.” are considered the same author, and distinct authors with the same name are differentiated by adding identifiers. Diverse forms of institutional names are unified to address variations caused by name changes or abbreviations, such as standardizing “FRANKFURT UNIV APPL SCI” and “Frankfurt University of Applied Sciences,” as well as “Chinese University of Hong Kong” and “University of Hong Kong” to consistent names.
(2) Different expressions of journal names are unified. For example, “International Journal of Human-Computer Interaction” and “Int J Hum Comput Interact” are standardized to a single name. This ensures consistency in journal names and prevents misclassification of literature due to differing journal names. Additionally, it involves checking if the journals have undergone name changes in the past decade to prevent any impact on the analysis due to such changes.
(3) Keywords data are cleansed by removing words that do not directly pertain to specific research content (e.g., people, review), merging synonyms (e.g., “UX” and “User Experience,” “aging-in-place” and “aging in place”), and standardizing plural forms of keywords (e.g., “assistive technologies” and “assistive technology,” “social robots” and “social robot”). This reduces redundant information in knowledge mapping.
Bibliometric results and analysis
Distribution power (rq1), literature descriptive statistical analysis.
Table 1 presents a detailed descriptive statistical overview of the literature in the field of older adults’ technology acceptance. After deduplication using the CiteSpace software, this study confirmed a valid sample size of 500 articles. Authored by 1839 researchers, the documents encompass 792 research institutions across 54 countries and are published in 217 different academic journals. As of the search cutoff date, these articles have accumulated 13,829 citations, with an annual average of 1156 citations, and an average of 27.66 citations per article. The h-index, a composite metric of quantity and quality of scientific output (Kamrani et al. 2021 ), reached 60 in this study.
Trends in publications and disciplinary distribution
The number of publications and citations are significant indicators of the research field’s development, reflecting its continuity, attention, and impact (Ale Ebrahim et al. 2014 ). The ranking of annual publications and citations in the field of older adults’ technology acceptance studies is presented chronologically in Fig. 2A . The figure shows a clear upward trend in the amount of literature in this field. Between 2013 and 2017, the number of publications increased slowly and decreased in 2018. However, in 2019, the number of publications increased rapidly to 52 and reached a peak of 108 in 2022, which is 6.75 times higher than in 2013. In 2022, the frequency of document citations reached its highest point with 3466 citations, reflecting the widespread recognition and citation of research in this field. Moreover, the curve of the annual number of publications fits a quadratic function, with a goodness-of-fit R 2 of 0.9661, indicating that the number of future publications is expected to increase even more rapidly.
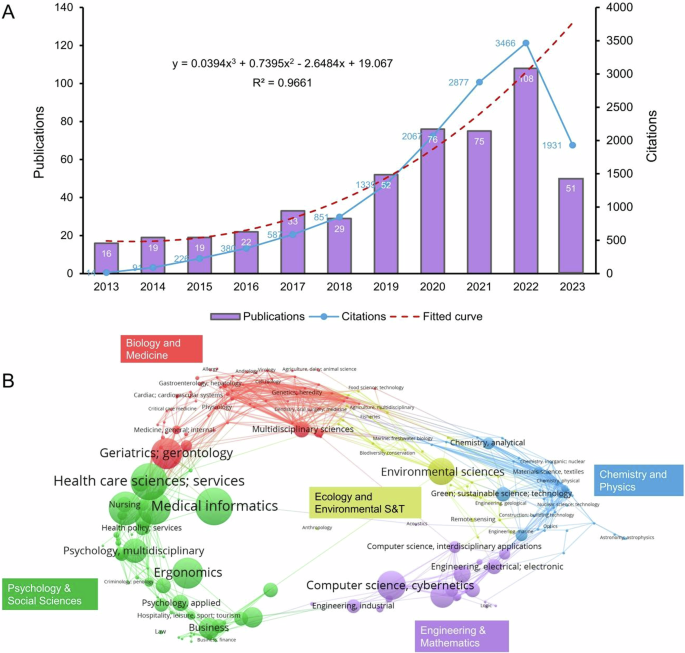
A Trends in trends in annual publications and citations (2013–2023). B Overlay analysis of the distribution of discipline fields.
Figure 2B shows that research on older adults’ technology acceptance involves the integration of multidisciplinary knowledge. According to Web of Science Categories, these 500 articles are distributed across 85 different disciplines. We have tabulated the top ten disciplines by publication volume (Table 2 ), which include Medical Informatics (75 articles, 15.00%), Health Care Sciences & Services (71 articles, 14.20%), Gerontology (61 articles, 12.20%), Public Environmental & Occupational Health (57 articles, 11.40%), and Geriatrics & Gerontology (52 articles, 10.40%), among others. The high output in these disciplines reflects the concentrated global academic interest in this comprehensive research topic. Additionally, interdisciplinary research approaches provide diverse perspectives and a solid theoretical foundation for studies on older adults’ technology acceptance, also paving the way for new research directions.
Knowledge flow analysis
A dual-map overlay is a CiteSpace map superimposed on top of a base map, which shows the interrelationships between journals in different domains, representing the publication and citation activities in each domain (Chen and Leydesdorff 2014 ). The overlay map reveals the link between the citing domain (on the left side) and the cited domain (on the right side), reflecting the knowledge flow of the discipline at the journal level (Leydesdorff and Rafols 2012 ). We utilize the in-built Z-score algorithm of the software to cluster the graph, as shown in Fig. 3 .
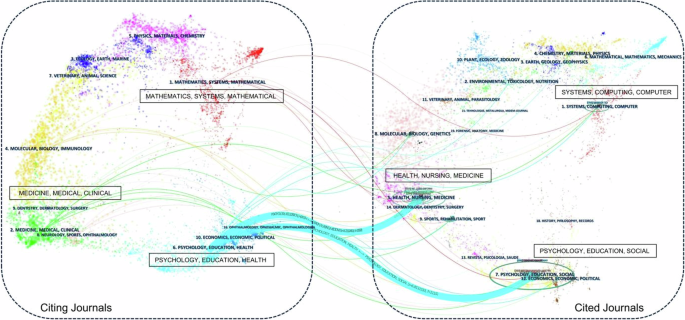
The left side shows the citing journal, and the right side shows the cited journal.
Figure 3 shows the distribution of citing journals clusters for older adults’ technology acceptance on the left side, while the right side refers to the main cited journals clusters. Two knowledge flow citation trajectories were obtained; they are presented by the color of the cited regions, and the thickness of these trajectories is proportional to the Z-score scaled frequency of citations (Chen et al. 2014 ). Within the cited regions, the most popular fields with the most records covered are “HEALTH, NURSING, MEDICINE” and “PSYCHOLOGY, EDUCATION, SOCIAL”, and the elliptical aspect ratio of these two fields stands out. Fields have prominent elliptical aspect ratios, highlighting their significant influence on older adults’ technology acceptance research. Additionally, the major citation trajectories originate in these two areas and progress to the frontier research area of “PSYCHOLOGY, EDUCATION, HEALTH”. It is worth noting that the citation trajectory from “PSYCHOLOGY, EDUCATION, SOCIAL” has a significant Z-value (z = 6.81), emphasizing the significance and impact of this development path. In the future, “MATHEMATICS, SYSTEMS, MATHEMATICAL”, “MOLECULAR, BIOLOGY, IMMUNOLOGY”, and “NEUROLOGY, SPORTS, OPHTHALMOLOGY” may become emerging fields. The fields of “MEDICINE, MEDICAL, CLINICAL” may be emerging areas of cutting-edge research.
Main research journals analysis
Table 3 provides statistics for the top ten journals by publication volume in the field of older adults’ technology acceptance. Together, these journals have published 137 articles, accounting for 27.40% of the total publications, indicating that there is no highly concentrated core group of journals in this field, with publications being relatively dispersed. Notably, Computers in Human Behavior , Journal of Medical Internet Research , and International Journal of Human-Computer Interaction each lead with 15 publications. In terms of citation metrics, International Journal of Medical Informatics and Computers in Human Behavior stand out significantly, with the former accumulating a total of 1,904 citations, averaging 211.56 citations per article, and the latter totaling 1,449 citations, with an average of 96.60 citations per article. These figures emphasize the academic authority and widespread impact of these journals within the research field.
Research power (RQ2)
Countries and collaborations analysis.
The analysis revealed the global research pattern for country distribution and collaboration (Chen et al. 2019 ). Figure 4A shows the network of national collaborations on older adults’ technology acceptance research. The size of the bubbles represents the amount of publications in each country, while the thickness of the connecting lines expresses the closeness of the collaboration among countries. Generally, this research subject has received extensive international attention, with China and the USA publishing far more than any other countries. China has established notable research collaborations with the USA, UK and Malaysia in this field, while other countries have collaborations, but the closeness is relatively low and scattered. Figure 4B shows the annual publication volume dynamics of the top ten countries in terms of total publications. Since 2017, China has consistently increased its annual publications, while the USA has remained relatively stable. In 2019, the volume of publications in each country increased significantly, this was largely due to the global outbreak of the COVID-19 pandemic, which has led to increased reliance on information technology among the elderly for medical consultations, online socialization, and health management (Sinha et al. 2021 ). This phenomenon has led to research advances in technology acceptance among older adults in various countries. Table 4 shows that the top ten countries account for 93.20% of the total cumulative number of publications, with each country having published more than 20 papers. Among these ten countries, all of them except China are developed countries, indicating that the research field of older adults’ technology acceptance has received general attention from developed countries. Currently, China and the USA were the leading countries in terms of publications with 111 and 104 respectively, accounting for 22.20% and 20.80%. The UK, Germany, Italy, and the Netherlands also made significant contributions. The USA and China ranked first and second in terms of the number of citations, while the Netherlands had the highest average citations, indicating the high impact and quality of its research. The UK has shown outstanding performance in international cooperation, while the USA highlights its significant academic influence in this field with the highest h-index value.
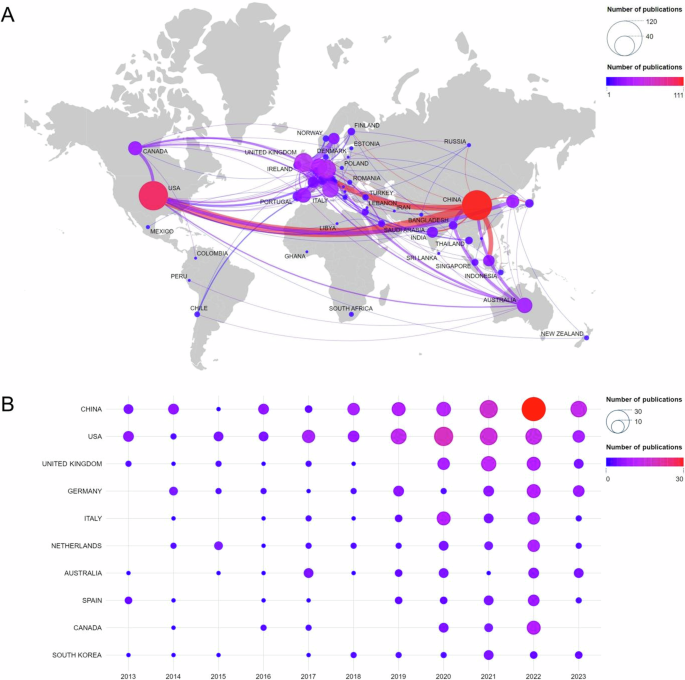
A National collaboration network. B Annual volume of publications in the top 10 countries.
Institutions and authors analysis
Analyzing the number of publications and citations can reveal an institution’s or author’s research strength and influence in a particular research area (Kwiek 2021 ). Tables 5 and 6 show the statistics of the institutions and authors whose publication counts are in the top ten, respectively. As shown in Table 5 , higher education institutions hold the main position in this research field. Among the top ten institutions, City University of Hong Kong and The University of Hong Kong from China lead with 14 and 9 publications, respectively. City University of Hong Kong has the highest h-index, highlighting its significant influence in the field. It is worth noting that Tilburg University in the Netherlands is not among the top five in terms of publications, but the high average citation count (130.14) of its literature demonstrates the high quality of its research.
After analyzing the authors’ output using Price’s Law (Redner 1998 ), the highest number of publications among the authors counted ( n = 10) defines a publication threshold of 3 for core authors in this research area. As a result of quantitative screening, a total of 63 core authors were identified. Table 6 shows that Chen from Zhejiang University, China, Ziefle from RWTH Aachen University, Germany, and Rogers from Macquarie University, Australia, were the top three authors in terms of the number of publications, with 10, 9, and 8 articles, respectively. In terms of average citation rate, Peek and Wouters, both scholars from the Netherlands, have significantly higher rates than other scholars, with 183.2 and 152.67 respectively. This suggests that their research is of high quality and widely recognized. Additionally, Chen and Rogers have high h-indices in this field.
Knowledge base and theme progress (RQ3)
Research knowledge base.
Co-citation relationships occur when two documents are cited together (Zhang and Zhu 2022 ). Co-citation mapping uses references as nodes to represent the knowledge base of a subject area (Min et al. 2021). Figure 5A illustrates co-occurrence mapping in older adults’ technology acceptance research, where larger nodes signify higher co-citation frequencies. Co-citation cluster analysis can be used to explore knowledge structure and research boundaries (Hota et al. 2020 ; Shiau et al. 2023 ). The co-citation clustering mapping of older adults’ technology acceptance research literature (Fig. 5B ) shows that the Q value of the clustering result is 0.8129 (>0.3), and the average value of the weight S is 0.9391 (>0.7), indicating that the clusters are uniformly distributed with a significant and credible structure. This further proves that the boundaries of the research field are clear and there is significant differentiation in the field. The figure features 18 cluster labels, each associated with thematic color blocks corresponding to different time slices. Highlighted emerging research themes include #2 Smart Home Technology, #7 Social Live, and #10 Customer Service. Furthermore, the clustering labels extracted are primarily classified into three categories: theoretical model deepening, emerging technology applications, research methods and evaluation, as detailed in Table 7 .
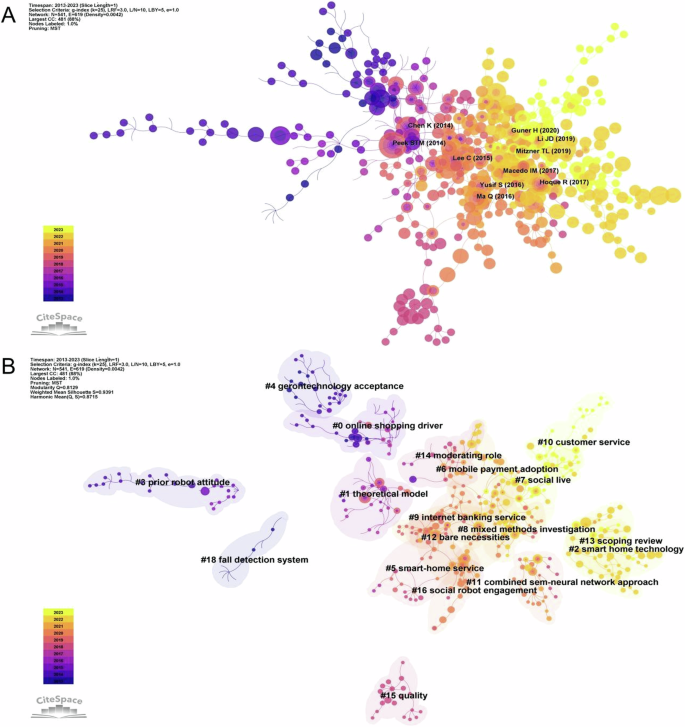
A Co-citation analysis of references. B Clustering network analysis of references.
Seminal literature analysis
The top ten nodes in terms of co-citation frequency were selected for further analysis. Table 8 displays the corresponding node information. Studies were categorized into four main groups based on content analysis. (1) Research focusing on specific technology usage by older adults includes studies by Peek et al. ( 2014 ), Ma et al. ( 2016 ), Hoque and Sorwar ( 2017 ), and Li et al. ( 2019 ), who investigated the factors influencing the use of e-technology, smartphones, mHealth, and smart wearables, respectively. (2) Concerning the development of theoretical models of technology acceptance, Chen and Chan ( 2014 ) introduced the Senior Technology Acceptance Model (STAM), and Macedo ( 2017 ) analyzed the predictive power of UTAUT2 in explaining older adults’ intentional behaviors and information technology usage. (3) In exploring older adults’ information technology adoption and behavior, Lee and Coughlin ( 2015 ) emphasized that the adoption of technology by older adults is a multifactorial process that includes performance, price, value, usability, affordability, accessibility, technical support, social support, emotion, independence, experience, and confidence. Yusif et al. ( 2016 ) conducted a literature review examining the key barriers affecting older adults’ adoption of assistive technology, including factors such as privacy, trust, functionality/added value, cost, and stigma. (4) From the perspective of research into older adults’ technology acceptance, Mitzner et al. ( 2019 ) assessed the long-term usage of computer systems designed for the elderly, whereas Guner and Acarturk ( 2020 ) compared information technology usage and acceptance between older and younger adults. The breadth and prevalence of this literature make it a vital reference for researchers in the field, also providing new perspectives and inspiration for future research directions.
Research thematic progress
Burst citation is a node of literature that guides the sudden change in dosage, which usually represents a prominent development or major change in a particular field, with innovative and forward-looking qualities. By analyzing the emergent literature, it is often easy to understand the dynamics of the subject area, mapping the emerging thematic change (Chen et al. 2022 ). Figure 6 shows the burst citation mapping in the field of older adults’ technology acceptance research, with burst citations represented by red nodes (Fig. 6A ). For the ten papers with the highest burst intensity (Fig. 6B ), this study will conduct further analysis in conjunction with literature review.
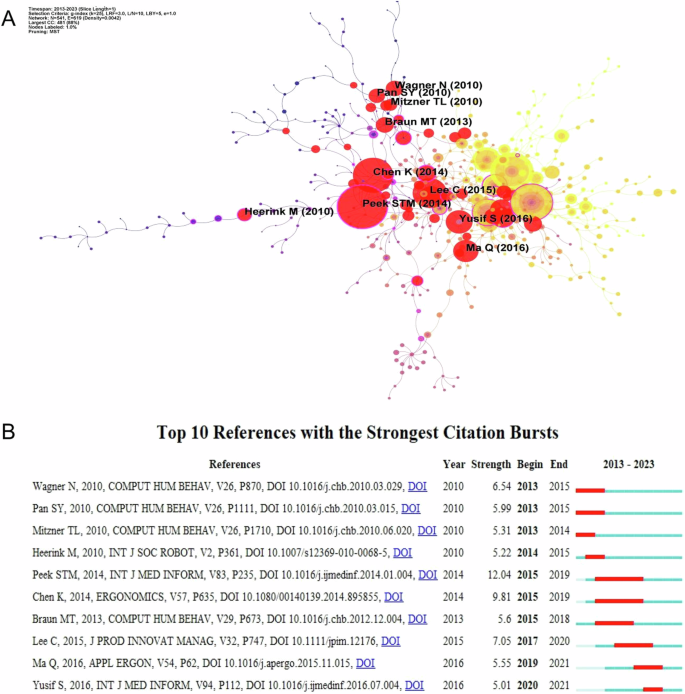
A Burst detection of co-citation. B The top 10 references with the strongest citation bursts.
As shown in Fig. 6 , Mitzner et al. ( 2010 ) broke the stereotype that older adults are fearful of technology, found that they actually have positive attitudes toward technology, and emphasized the centrality of ease of use and usefulness in the process of technology acceptance. This finding provides an important foundation for subsequent research. During the same period, Wagner et al. ( 2010 ) conducted theory-deepening and applied research on technology acceptance among older adults. The research focused on older adults’ interactions with computers from the perspective of Social Cognitive Theory (SCT). This expanded the understanding of technology acceptance, particularly regarding the relationship between behavior, environment, and other SCT elements. In addition, Pan and Jordan-Marsh ( 2010 ) extended the TAM to examine the interactions among predictors of perceived usefulness, perceived ease of use, subjective norm, and convenience conditions when older adults use the Internet, taking into account the moderating roles of gender and age. Heerink et al. ( 2010 ) adapted and extended the UTAUT, constructed a technology acceptance model specifically designed for older users’ acceptance of assistive social agents, and validated it using controlled experiments and longitudinal data, explaining intention to use by combining functional assessment and social interaction variables.
Then the research theme shifted to an in-depth analysis of the factors influencing technology acceptance among older adults. Two papers with high burst strengths emerged during this period: Peek et al. ( 2014 ) (Strength = 12.04), Chen and Chan ( 2014 ) (Strength = 9.81). Through a systematic literature review and empirical study, Peek STM and Chen K, among others, identified multidimensional factors that influence older adults’ technology acceptance. Peek et al. ( 2014 ) analyzed literature on the acceptance of in-home care technology among older adults and identified six factors that influence their acceptance: concerns about technology, expected benefits, technology needs, technology alternatives, social influences, and older adult characteristics, with a focus on differences between pre- and post-implementation factors. Chen and Chan ( 2014 ) constructed the STAM by administering a questionnaire to 1012 older adults and adding eight important factors, including technology anxiety, self-efficacy, cognitive ability, and physical function, based on the TAM. This enriches the theoretical foundation of the field. In addition, Braun ( 2013 ) highlighted the role of perceived usefulness, trust in social networks, and frequency of Internet use in older adults’ use of social networks, while ease of use and social pressure were not significant influences. These findings contribute to the study of older adults’ technology acceptance within specific technology application domains.
Recent research has focused on empirical studies of personal factors and emerging technologies. Ma et al. ( 2016 ) identified key personal factors affecting smartphone acceptance among older adults through structured questionnaires and face-to-face interviews with 120 participants. The study found that cost, self-satisfaction, and convenience were important factors influencing perceived usefulness and ease of use. This study offers empirical evidence to comprehend the main factors that drive smartphone acceptance among Chinese older adults. Additionally, Yusif et al. ( 2016 ) presented an overview of the obstacles that hinder older adults’ acceptance of assistive technologies, focusing on privacy, trust, and functionality.
In summary, research on older adults’ technology acceptance has shifted from early theoretical deepening and analysis of influencing factors to empirical studies in the areas of personal factors and emerging technologies, which have greatly enriched the theoretical basis of older adults’ technology acceptance and provided practical guidance for the design of emerging technology products.
Research hotspots, evolutionary trends, and quality distribution (RQ4)
Core keywords analysis.
Keywords concise the main idea and core of the literature, and are a refined summary of the research content (Huang et al. 2021 ). In CiteSpace, nodes with a centrality value greater than 0.1 are considered to be critical nodes. Analyzing keywords with high frequency and centrality helps to visualize the hot topics in the research field (Park et al. 2018 ). The merged keywords were imported into CiteSpace, and the top 10 keywords were counted and sorted by frequency and centrality respectively, as shown in Table 9 . The results show that the keyword “TAM” has the highest frequency (92), followed by “UTAUT” (24), which reflects that the in-depth study of the existing technology acceptance model and its theoretical expansion occupy a central position in research related to older adults’ technology acceptance. Furthermore, the terms ‘assistive technology’ and ‘virtual reality’ are both high-frequency and high-centrality terms (frequency = 17, centrality = 0.10), indicating that the research on assistive technology and virtual reality for older adults is the focus of current academic attention.
Research hotspots analysis
Using VOSviewer for keyword co-occurrence analysis organizes keywords into groups or clusters based on their intrinsic connections and frequencies, clearly highlighting the research field’s hot topics. The connectivity among keywords reveals correlations between different topics. To ensure accuracy, the analysis only considered the authors’ keywords. Subsequently, the keywords were filtered by setting the keyword frequency to 5 to obtain the keyword clustering map of the research on older adults’ technology acceptance research keyword clustering mapping (Fig. 7 ), combined with the keyword co-occurrence clustering network (Fig. 7A ) and the corresponding density situation (Fig. 7B ) to make a detailed analysis of the following four groups of clustered themes.
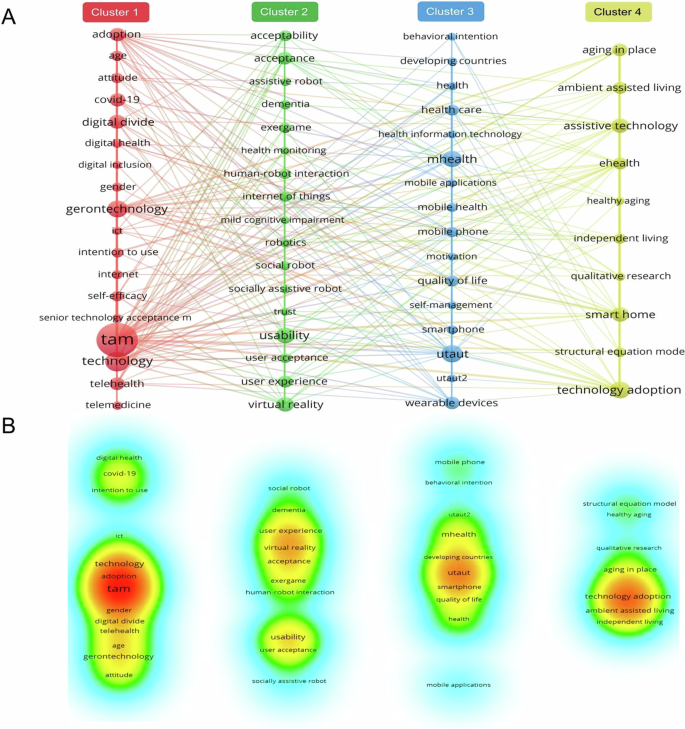
A Co-occurrence clustering network. B Keyword density.
Cluster #1—Research on the factors influencing technology adoption among older adults is a prominent topic, covering age, gender, self-efficacy, attitude, and and intention to use (Berkowsky et al. 2017 ; Wang et al. 2017 ). It also examined older adults’ attitudes towards and acceptance of digital health technologies (Ahmad and Mozelius, 2022 ). Moreover, the COVID-19 pandemic, significantly impacting older adults’ technology attitudes and usage, has underscored the study’s importance and urgency. Therefore, it is crucial to conduct in-depth studies on how older adults accept, adopt, and effectively use new technologies, to address their needs and help them overcome the digital divide within digital inclusion. This will improve their quality of life and healthcare experiences.
Cluster #2—Research focuses on how older adults interact with assistive technologies, especially assistive robots and health monitoring devices, emphasizing trust, usability, and user experience as crucial factors (Halim et al. 2022 ). Moreover, health monitoring technologies effectively track and manage health issues common in older adults, like dementia and mild cognitive impairment (Lussier et al. 2018 ; Piau et al. 2019 ). Interactive exercise games and virtual reality have been deployed to encourage more physical and cognitive engagement among older adults (Campo-Prieto et al. 2021 ). Personalized and innovative technology significantly enhances older adults’ participation, improving their health and well-being.
Cluster #3—Optimizing health management for older adults using mobile technology. With the development of mobile health (mHealth) and health information technology, mobile applications, smartphones, and smart wearable devices have become effective tools to help older users better manage chronic conditions, conduct real-time health monitoring, and even receive telehealth services (Dupuis and Tsotsos 2018 ; Olmedo-Aguirre et al. 2022 ; Kim et al. 2014 ). Additionally, these technologies can mitigate the problem of healthcare resource inequality, especially in developing countries. Older adults’ acceptance and use of these technologies are significantly influenced by their behavioral intentions, motivational factors, and self-management skills. These internal motivational factors, along with external factors, jointly affect older adults’ performance in health management and quality of life.
Cluster #4—Research on technology-assisted home care for older adults is gaining popularity. Environmentally assisted living enhances older adults’ independence and comfort at home, offering essential support and security. This has a crucial impact on promoting healthy aging (Friesen et al. 2016 ; Wahlroos et al. 2023 ). The smart home is a core application in this field, providing a range of solutions that facilitate independent living for the elderly in a highly integrated and user-friendly manner. This fulfills different dimensions of living and health needs (Majumder et al. 2017 ). Moreover, eHealth offers accurate and personalized health management and healthcare services for older adults (Delmastro et al. 2018 ), ensuring their needs are met at home. Research in this field often employs qualitative methods and structural equation modeling to fully understand older adults’ needs and experiences at home and analyze factors influencing technology adoption.
Evolutionary trends analysis
To gain a deeper understanding of the evolutionary trends in research hotspots within the field of older adults’ technology acceptance, we conducted a statistical analysis of the average appearance times of keywords, using CiteSpace to generate the time-zone evolution mapping (Fig. 8 ) and burst keywords. The time-zone mapping visually displays the evolution of keywords over time, intuitively reflecting the frequency and initial appearance of keywords in research, commonly used to identify trends in research topics (Jing et al. 2024a ; Kumar et al. 2021 ). Table 10 lists the top 15 keywords by burst strength, with the red sections indicating high-frequency citations and their burst strength in specific years. These burst keywords reveal the focus and trends of research themes over different periods (Kleinberg 2002 ). Combining insights from the time-zone mapping and burst keywords provides more objective and accurate research insights (Wang et al. 2023b ).
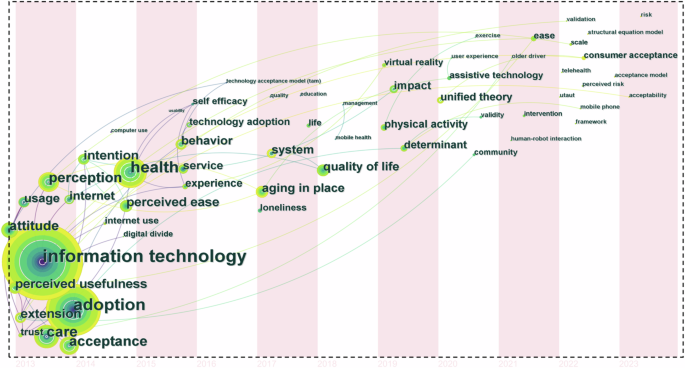
Reflecting the frequency and time of first appearance of keywords in the study.
An integrated analysis of Fig. 8 and Table 10 shows that early research on older adults’ technology acceptance primarily focused on factors such as perceived usefulness, ease of use, and attitudes towards information technology, including their use of computers and the internet (Pan and Jordan-Marsh 2010 ), as well as differences in technology use between older adults and other age groups (Guner and Acarturk 2020 ). Subsequently, the research focus expanded to improving the quality of life for older adults, exploring how technology can optimize health management and enhance the possibility of independent living, emphasizing the significant role of technology in improving the quality of life for the elderly. With ongoing technological advancements, recent research has shifted towards areas such as “virtual reality,” “telehealth,” and “human-robot interaction,” with a focus on the user experience of older adults (Halim et al. 2022 ). The appearance of keywords such as “physical activity” and “exercise” highlights the value of technology in promoting physical activity and health among older adults. This phase of research tends to make cutting-edge technology genuinely serve the practical needs of older adults, achieving its widespread application in daily life. Additionally, research has focused on expanding and quantifying theoretical models of older adults’ technology acceptance, involving keywords such as “perceived risk”, “validation” and “UTAUT”.
In summary, from 2013 to 2023, the field of older adults’ technology acceptance has evolved from initial explorations of influencing factors, to comprehensive enhancements in quality of life and health management, and further to the application and deepening of theoretical models and cutting-edge technologies. This research not only reflects the diversity and complexity of the field but also demonstrates a comprehensive and in-depth understanding of older adults’ interactions with technology across various life scenarios and needs.
Research quality distribution
To reveal the distribution of research quality in the field of older adults’ technology acceptance, a strategic diagram analysis is employed to calculate and illustrate the internal development and interrelationships among various research themes (Xie et al. 2020 ). The strategic diagram uses Centrality as the X-axis and Density as the Y-axis to divide into four quadrants, where the X-axis represents the strength of the connection between thematic clusters and other themes, with higher values indicating a central position in the research field; the Y-axis indicates the level of development within the thematic clusters, with higher values denoting a more mature and widely recognized field (Li and Zhou 2020 ).
Through cluster analysis and manual verification, this study categorized 61 core keywords (Frequency ≥5) into 11 thematic clusters. Subsequently, based on the keywords covered by each thematic cluster, the research themes and their directions for each cluster were summarized (Table 11 ), and the centrality and density coordinates for each cluster were precisely calculated (Table 12 ). Finally, a strategic diagram of the older adults’ technology acceptance research field was constructed (Fig. 9 ). Based on the distribution of thematic clusters across the quadrants in the strategic diagram, the structure and developmental trends of the field were interpreted.
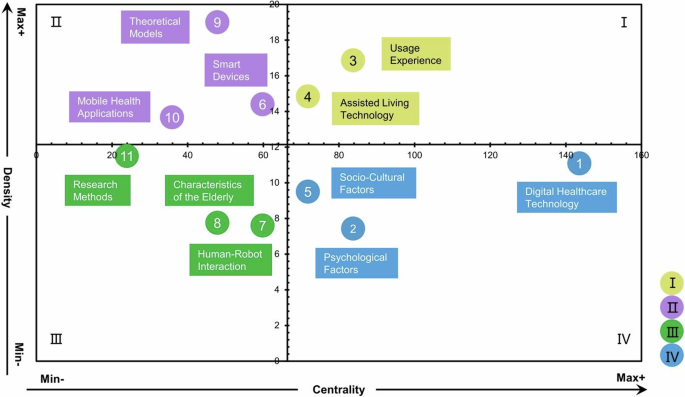
Classification and visualization of theme clusters based on density and centrality.
As illustrated in Fig. 9 , (1) the theme clusters of #3 Usage Experience and #4 Assisted Living Technology are in the first quadrant, characterized by high centrality and density. Their internal cohesion and close links with other themes indicate their mature development, systematic research content or directions have been formed, and they have a significant influence on other themes. These themes play a central role in the field of older adults’ technology acceptance and have promising prospects. (2) The theme clusters of #6 Smart Devices, #9 Theoretical Models, and #10 Mobile Health Applications are in the second quadrant, with higher density but lower centrality. These themes have strong internal connections but weaker external links, indicating that these three themes have received widespread attention from researchers and have been the subject of related research, but more as self-contained systems and exhibit independence. Therefore, future research should further explore in-depth cooperation and cross-application with other themes. (3) The theme clusters of #7 Human-Robot Interaction, #8 Characteristics of the Elderly, and #11 Research Methods are in the third quadrant, with lower centrality and density. These themes are loosely connected internally and have weak links with others, indicating their developmental immaturity. Compared to other topics, they belong to the lower attention edge and niche themes, and there is a need for further investigation. (4) The theme clusters of #1 Digital Healthcare Technology, #2 Psychological Factors, and #5 Socio-Cultural Factors are located in the fourth quadrant, with high centrality but low density. Although closely associated with other research themes, the internal cohesion within these clusters is relatively weak. This suggests that while these themes are closely linked to other research areas, their own development remains underdeveloped, indicating a core immaturity. Nevertheless, these themes are crucial within the research domain of elderly technology acceptance and possess significant potential for future exploration.
Discussion on distribution power (RQ1)
Over the past decade, academic interest and influence in the area of older adults’ technology acceptance have significantly increased. This trend is evidenced by a quantitative analysis of publication and citation volumes, particularly noticeable in 2019 and 2022, where there was a substantial rise in both metrics. The rise is closely linked to the widespread adoption of emerging technologies such as smart homes, wearable devices, and telemedicine among older adults. While these technologies have enhanced their quality of life, they also pose numerous challenges, sparking extensive research into their acceptance, usage behaviors, and influencing factors among the older adults (Pirzada et al. 2022 ; Garcia Reyes et al. 2023 ). Furthermore, the COVID-19 pandemic led to a surge in technology demand among older adults, especially in areas like medical consultation, online socialization, and health management, further highlighting the importance and challenges of technology. Health risks and social isolation have compelled older adults to rely on technology for daily activities, accelerating its adoption and application within this demographic. This phenomenon has made technology acceptance a critical issue, driving societal and academic focus on the study of technology acceptance among older adults.
The flow of knowledge at the level of high-output disciplines and journals, along with the primary publishing outlets, indicates the highly interdisciplinary nature of research into older adults’ technology acceptance. This reflects the complexity and breadth of issues related to older adults’ technology acceptance, necessitating the integration of multidisciplinary knowledge and approaches. Currently, research is primarily focused on medical health and human-computer interaction, demonstrating academic interest in improving health and quality of life for older adults and addressing the urgent needs related to their interactions with technology. In the field of medical health, research aims to provide advanced and innovative healthcare technologies and services to meet the challenges of an aging population while improving the quality of life for older adults (Abdi et al. 2020 ; Wilson et al. 2021 ). In the field of human-computer interaction, research is focused on developing smarter and more user-friendly interaction models to meet the needs of older adults in the digital age, enabling them to actively participate in social activities and enjoy a higher quality of life (Sayago, 2019 ). These studies are crucial for addressing the challenges faced by aging societies, providing increased support and opportunities for the health, welfare, and social participation of older adults.
Discussion on research power (RQ2)
This study analyzes leading countries and collaboration networks, core institutions and authors, revealing the global research landscape and distribution of research strength in the field of older adults’ technology acceptance, and presents quantitative data on global research trends. From the analysis of country distribution and collaborations, China and the USA hold dominant positions in this field, with developed countries like the UK, Germany, Italy, and the Netherlands also excelling in international cooperation and research influence. The significant investment in technological research and the focus on the technological needs of older adults by many developed countries reflect their rapidly aging societies, policy support, and resource allocation.
China is the only developing country that has become a major contributor in this field, indicating its growing research capabilities and high priority given to aging societies and technological innovation. Additionally, China has close collaborations with countries such as USA, the UK, and Malaysia, driven not only by technological research needs but also by shared challenges and complementarities in aging issues among these nations. For instance, the UK has extensive experience in social welfare and aging research, providing valuable theoretical guidance and practical experience. International collaborations, aimed at addressing the challenges of aging, integrate the strengths of various countries, advancing in-depth and widespread development in the research of technology acceptance among older adults.
At the institutional and author level, City University of Hong Kong leads in publication volume, with research teams led by Chan and Chen demonstrating significant academic activity and contributions. Their research primarily focuses on older adults’ acceptance and usage behaviors of various technologies, including smartphones, smart wearables, and social robots (Chen et al. 2015 ; Li et al. 2019 ; Ma et al. 2016 ). These studies, targeting specific needs and product characteristics of older adults, have developed new models of technology acceptance based on existing frameworks, enhancing the integration of these technologies into their daily lives and laying a foundation for further advancements in the field. Although Tilburg University has a smaller publication output, it holds significant influence in the field of older adults’ technology acceptance. Particularly, the high citation rate of Peek’s studies highlights their excellence in research. Peek extensively explored older adults’ acceptance and usage of home care technologies, revealing the complexity and dynamics of their technology use behaviors. His research spans from identifying systemic influencing factors (Peek et al. 2014 ; Peek et al. 2016 ), emphasizing familial impacts (Luijkx et al. 2015 ), to constructing comprehensive models (Peek et al. 2017 ), and examining the dynamics of long-term usage (Peek et al. 2019 ), fully reflecting the evolving technology landscape and the changing needs of older adults. Additionally, the ongoing contributions of researchers like Ziefle, Rogers, and Wouters in the field of older adults’ technology acceptance demonstrate their research influence and leadership. These researchers have significantly enriched the knowledge base in this area with their diverse perspectives. For instance, Ziefle has uncovered the complex attitudes of older adults towards technology usage, especially the trade-offs between privacy and security, and how different types of activities affect their privacy needs (Maidhof et al. 2023 ; Mujirishvili et al. 2023 ; Schomakers and Ziefle 2023 ; Wilkowska et al. 2022 ), reflecting a deep exploration and ongoing innovation in the field of older adults’ technology acceptance.
Discussion on knowledge base and thematic progress (RQ3)
Through co-citation analysis and systematic review of seminal literature, this study reveals the knowledge foundation and thematic progress in the field of older adults’ technology acceptance. Co-citation networks and cluster analyses illustrate the structural themes of the research, delineating the differentiation and boundaries within this field. Additionally, burst detection analysis offers a valuable perspective for understanding the thematic evolution in the field of technology acceptance among older adults. The development and innovation of theoretical models are foundational to this research. Researchers enhance the explanatory power of constructed models by deepening and expanding existing technology acceptance theories to address theoretical limitations. For instance, Heerink et al. ( 2010 ) modified and expanded the UTAUT model by integrating functional assessment and social interaction variables to create the almere model. This model significantly enhances the ability to explain the intentions of older users in utilizing assistive social agents and improves the explanation of actual usage behaviors. Additionally, Chen and Chan ( 2014 ) extended the TAM to include age-related health and capability features of older adults, creating the STAM, which substantially improves predictions of older adults’ technology usage behaviors. Personal attributes, health and capability features, and facilitating conditions have a direct impact on technology acceptance. These factors more effectively predict older adults’ technology usage behaviors than traditional attitudinal factors.
With the advancement of technology and the application of emerging technologies, new research topics have emerged, increasingly focusing on older adults’ acceptance and use of these technologies. Prior to this, the study by Mitzner et al. ( 2010 ) challenged the stereotype of older adults’ conservative attitudes towards technology, highlighting the central roles of usability and usefulness in the technology acceptance process. This discovery laid an important foundation for subsequent research. Research fields such as “smart home technology,” “social life,” and “customer service” are emerging, indicating a shift in focus towards the practical and social applications of technology in older adults’ lives. Research not only focuses on the technology itself but also on how these technologies integrate into older adults’ daily lives and how they can improve the quality of life through technology. For instance, studies such as those by Ma et al. ( 2016 ), Hoque and Sorwar ( 2017 ), and Li et al. ( 2019 ) have explored factors influencing older adults’ use of smartphones, mHealth, and smart wearable devices.
Furthermore, the diversification of research methodologies and innovation in evaluation techniques, such as the use of mixed methods, structural equation modeling (SEM), and neural network (NN) approaches, have enhanced the rigor and reliability of the findings, enabling more precise identification of the factors and mechanisms influencing technology acceptance. Talukder et al. ( 2020 ) employed an effective multimethodological strategy by integrating SEM and NN to leverage the complementary strengths of both approaches, thus overcoming their individual limitations and more accurately analyzing and predicting older adults’ acceptance of wearable health technologies (WHT). SEM is utilized to assess the determinants’ impact on the adoption of WHT, while neural network models validate SEM outcomes and predict the significance of key determinants. This combined approach not only boosts the models’ reliability and explanatory power but also provides a nuanced understanding of the motivations and barriers behind older adults’ acceptance of WHT, offering deep research insights.
Overall, co-citation analysis of the literature in the field of older adults’ technology acceptance has uncovered deeper theoretical modeling and empirical studies on emerging technologies, while emphasizing the importance of research methodological and evaluation innovations in understanding complex social science issues. These findings are crucial for guiding the design and marketing strategies of future technology products, especially in the rapidly growing market of older adults.
Discussion on research hotspots and evolutionary trends (RQ4)
By analyzing core keywords, we can gain deep insights into the hot topics, evolutionary trends, and quality distribution of research in the field of older adults’ technology acceptance. The frequent occurrence of the keywords “TAM” and “UTAUT” indicates that the applicability and theoretical extension of existing technology acceptance models among older adults remain a focal point in academia. This phenomenon underscores the enduring influence of the studies by Davis ( 1989 ) and Venkatesh et al. ( 2003 ), whose models provide a robust theoretical framework for explaining and predicting older adults’ acceptance and usage of emerging technologies. With the widespread application of artificial intelligence (AI) and big data technologies, these theoretical models have incorporated new variables such as perceived risk, trust, and privacy issues (Amin et al. 2024 ; Chen et al. 2024 ; Jing et al. 2024b ; Seibert et al. 2021 ; Wang et al. 2024b ), advancing the theoretical depth and empirical research in this field.
Keyword co-occurrence cluster analysis has revealed multiple research hotspots in the field, including factors influencing technology adoption, interactive experiences between older adults and assistive technologies, the application of mobile health technology in health management, and technology-assisted home care. These studies primarily focus on enhancing the quality of life and health management of older adults through emerging technologies, particularly in the areas of ambient assisted living, smart health monitoring, and intelligent medical care. In these domains, the role of AI technology is increasingly significant (Qian et al. 2021 ; Ho 2020 ). With the evolution of next-generation information technologies, AI is increasingly integrated into elder care systems, offering intelligent, efficient, and personalized service solutions by analyzing the lifestyles and health conditions of older adults. This integration aims to enhance older adults’ quality of life in aspects such as health monitoring and alerts, rehabilitation assistance, daily health management, and emotional support (Lee et al. 2023 ). A survey indicates that 83% of older adults prefer AI-driven solutions when selecting smart products, demonstrating the increasing acceptance of AI in elder care (Zhao and Li 2024 ). Integrating AI into elder care presents both opportunities and challenges, particularly in terms of user acceptance, trust, and long-term usage effects, which warrant further exploration (Mhlanga 2023 ). These studies will help better understand the profound impact of AI technology on the lifestyles of older adults and provide critical references for optimizing AI-driven elder care services.
The Time-zone evolution mapping and burst keyword analysis further reveal the evolutionary trends of research hotspots. Early studies focused on basic technology acceptance models and user perceptions, later expanding to include quality of life and health management. In recent years, research has increasingly focused on cutting-edge technologies such as virtual reality, telehealth, and human-robot interaction, with a concurrent emphasis on the user experience of older adults. This evolutionary process demonstrates a deepening shift from theoretical models to practical applications, underscoring the significant role of technology in enhancing the quality of life for older adults. Furthermore, the strategic coordinate mapping analysis clearly demonstrates the development and mutual influence of different research themes. High centrality and density in the themes of Usage Experience and Assisted Living Technology indicate their mature research status and significant impact on other themes. The themes of Smart Devices, Theoretical Models, and Mobile Health Applications demonstrate self-contained research trends. The themes of Human-Robot Interaction, Characteristics of the Elderly, and Research Methods are not yet mature, but they hold potential for development. Themes of Digital Healthcare Technology, Psychological Factors, and Socio-Cultural Factors are closely related to other themes, displaying core immaturity but significant potential.
In summary, the research hotspots in the field of older adults’ technology acceptance are diverse and dynamic, demonstrating the academic community’s profound understanding of how older adults interact with technology across various life contexts and needs. Under the influence of AI and big data, research should continue to focus on the application of emerging technologies among older adults, exploring in depth how they adapt to and effectively use these technologies. This not only enhances the quality of life and healthcare experiences for older adults but also drives ongoing innovation and development in this field.
Research agenda
Based on the above research findings, to further understand and promote technology acceptance and usage among older adults, we recommend future studies focus on refining theoretical models, exploring long-term usage, and assessing user experience in the following detailed aspects:
Refinement and validation of specific technology acceptance models for older adults: Future research should focus on developing and validating technology acceptance models based on individual characteristics, particularly considering variations in technology acceptance among older adults across different educational levels and cultural backgrounds. This includes factors such as age, gender, educational background, and cultural differences. Additionally, research should examine how well specific technologies, such as wearable devices and mobile health applications, meet the needs of older adults. Building on existing theoretical models, this research should integrate insights from multiple disciplines such as psychology, sociology, design, and engineering through interdisciplinary collaboration to create more accurate and comprehensive models, which should then be validated in relevant contexts.
Deepening the exploration of the relationship between long-term technology use and quality of life among older adults: The acceptance and use of technology by users is a complex and dynamic process (Seuwou et al. 2016 ). Existing research predominantly focuses on older adults’ initial acceptance or short-term use of new technologies; however, the impact of long-term use on their quality of life and health is more significant. Future research should focus on the evolution of older adults’ experiences and needs during long-term technology usage, and the enduring effects of technology on their social interactions, mental health, and life satisfaction. Through longitudinal studies and qualitative analysis, this research reveals the specific needs and challenges of older adults in long-term technology use, providing a basis for developing technologies and strategies that better meet their requirements. This understanding aids in comprehensively assessing the impact of technology on older adults’ quality of life and guiding the optimization and improvement of technological products.
Evaluating the Importance of User Experience in Research on Older Adults’ Technology Acceptance: Understanding the mechanisms of information technology acceptance and use is central to human-computer interaction research. Although technology acceptance models and user experience models differ in objectives, they share many potential intersections. Technology acceptance research focuses on structured prediction and assessment, while user experience research concentrates on interpreting design impacts and new frameworks. Integrating user experience to assess older adults’ acceptance of technology products and systems is crucial (Codfrey et al. 2022 ; Wang et al. 2019 ), particularly for older users, where specific product designs should emphasize practicality and usability (Fisk et al. 2020 ). Researchers need to explore innovative age-appropriate design methods to enhance older adults’ usage experience. This includes studying older users’ actual usage preferences and behaviors, optimizing user interfaces, and interaction designs. Integrating feedback from older adults to tailor products to their needs can further promote their acceptance and continued use of technology products.
Conclusions
This study conducted a systematic review of the literature on older adults’ technology acceptance over the past decade through bibliometric analysis, focusing on the distribution power, research power, knowledge base and theme progress, research hotspots, evolutionary trends, and quality distribution. Using a combination of quantitative and qualitative methods, this study has reached the following conclusions:
Technology acceptance among older adults has become a hot topic in the international academic community, involving the integration of knowledge across multiple disciplines, including Medical Informatics, Health Care Sciences Services, and Ergonomics. In terms of journals, “PSYCHOLOGY, EDUCATION, HEALTH” represents a leading field, with key publications including Computers in Human Behavior , Journal of Medical Internet Research , and International Journal of Human-Computer Interaction . These journals possess significant academic authority and extensive influence in the field.
Research on technology acceptance among older adults is particularly active in developed countries, with China and USA publishing significantly more than other nations. The Netherlands leads in high average citation rates, indicating the depth and impact of its research. Meanwhile, the UK stands out in terms of international collaboration. At the institutional level, City University of Hong Kong and The University of Hong Kong in China are in leading positions. Tilburg University in the Netherlands demonstrates exceptional research quality through its high average citation count. At the author level, Chen from China has the highest number of publications, while Peek from the Netherlands has the highest average citation count.
Co-citation analysis of references indicates that the knowledge base in this field is divided into three main categories: theoretical model deepening, emerging technology applications, and research methods and evaluation. Seminal literature focuses on four areas: specific technology use by older adults, expansion of theoretical models of technology acceptance, information technology adoption behavior, and research perspectives. Research themes have evolved from initial theoretical deepening and analysis of influencing factors to empirical studies on individual factors and emerging technologies.
Keyword analysis indicates that TAM and UTAUT are the most frequently occurring terms, while “assistive technology” and “virtual reality” are focal points with high frequency and centrality. Keyword clustering analysis reveals that research hotspots are concentrated on the influencing factors of technology adoption, human-robot interaction experiences, mobile health management, and technology for aging in place. Time-zone evolution mapping and burst keyword analysis have revealed the research evolution from preliminary exploration of influencing factors, to enhancements in quality of life and health management, and onto advanced technology applications and deepening of theoretical models. Furthermore, analysis of research quality distribution indicates that Usage Experience and Assisted Living Technology have become core topics, while Smart Devices, Theoretical Models, and Mobile Health Applications point towards future research directions.
Through this study, we have systematically reviewed the dynamics, core issues, and evolutionary trends in the field of older adults’ technology acceptance, constructing a comprehensive Knowledge Mapping of the domain and presenting a clear framework of existing research. This not only lays the foundation for subsequent theoretical discussions and innovative applications in the field but also provides an important reference for relevant scholars.
Limitations
To our knowledge, this is the first bibliometric analysis concerning technology acceptance among older adults, and we adhered strictly to bibliometric standards throughout our research. However, this study relies on the Web of Science Core Collection, and while its authority and breadth are widely recognized, this choice may have missed relevant literature published in other significant databases such as PubMed, Scopus, and Google Scholar, potentially overlooking some critical academic contributions. Moreover, given that our analysis was confined to literature in English, it may not reflect studies published in other languages, somewhat limiting the global representativeness of our data sample.
It is noteworthy that with the rapid development of AI technology, its increasingly widespread application in elderly care services is significantly transforming traditional care models. AI is profoundly altering the lifestyles of the elderly, from health monitoring and smart diagnostics to intelligent home systems and personalized care, significantly enhancing their quality of life and health care standards. The potential for AI technology within the elderly population is immense, and research in this area is rapidly expanding. However, due to the restrictive nature of the search terms used in this study, it did not fully cover research in this critical area, particularly in addressing key issues such as trust, privacy, and ethics.
Consequently, future research should not only expand data sources, incorporating multilingual and multidatabase literature, but also particularly focus on exploring older adults’ acceptance of AI technology and its applications, in order to construct a more comprehensive academic landscape of older adults’ technology acceptance, thereby enriching and extending the knowledge system and academic trends in this field.
Data availability
The datasets analyzed during the current study are available in the Dataverse repository: https://doi.org/10.7910/DVN/6K0GJH .
Abdi S, de Witte L, Hawley M (2020) Emerging technologies with potential care and support applications for older people: review of gray literature. JMIR Aging 3(2):e17286. https://doi.org/10.2196/17286
Article PubMed PubMed Central Google Scholar
Achuthan K, Nair VK, Kowalski R, Ramanathan S, Raman R (2023) Cyberbullying research—Alignment to sustainable development and impact of COVID-19: Bibliometrics and science mapping analysis. Comput Human Behav 140:107566. https://doi.org/10.1016/j.chb.2022.107566
Article Google Scholar
Ahmad A, Mozelius P (2022) Human-Computer Interaction for Older Adults: a Literature Review on Technology Acceptance of eHealth Systems. J Eng Res Sci 1(4):119–126. https://doi.org/10.55708/js0104014
Ale Ebrahim N, Salehi H, Embi MA, Habibi F, Gholizadeh H, Motahar SM (2014) Visibility and citation impact. Int Educ Stud 7(4):120–125. https://doi.org/10.5539/ies.v7n4p120
Amin MS, Johnson VL, Prybutok V, Koh CE (2024) An investigation into factors affecting the willingness to disclose personal health information when using AI-enabled caregiver robots. Ind Manag Data Syst 124(4):1677–1699. https://doi.org/10.1108/IMDS-09-2023-0608
Baer NR, Vietzke J, Schenk L (2022) Middle-aged and older adults’ acceptance of mobile nutrition and fitness apps: a systematic mixed studies review. PLoS One 17(12):e0278879. https://doi.org/10.1371/journal.pone.0278879
Barnard Y, Bradley MD, Hodgson F, Lloyd AD (2013) Learning to use new technologies by older adults: Perceived difficulties, experimentation behaviour and usability. Comput Human Behav 29(4):1715–1724. https://doi.org/10.1016/j.chb.2013.02.006
Berkowsky RW, Sharit J, Czaja SJ (2017) Factors predicting decisions about technology adoption among older adults. Innov Aging 3(1):igy002. https://doi.org/10.1093/geroni/igy002
Braun MT (2013) Obstacles to social networking website use among older adults. Comput Human Behav 29(3):673–680. https://doi.org/10.1016/j.chb.2012.12.004
Article MathSciNet Google Scholar
Campo-Prieto P, Rodríguez-Fuentes G, Cancela-Carral JM (2021) Immersive virtual reality exergame promotes the practice of physical activity in older people: An opportunity during COVID-19. Multimodal Technol Interact 5(9):52. https://doi.org/10.3390/mti5090052
Chen C (2006) CiteSpace II: Detecting and visualizing emerging trends and transient patterns in scientific literature. J Am Soc Inf Sci Technol 57(3):359–377. https://doi.org/10.1002/asi.20317
Chen C, Dubin R, Kim MC (2014) Emerging trends and new developments in regenerative medicine: a scientometric update (2000–2014). Expert Opin Biol Ther 14(9):1295–1317. https://doi.org/10.1517/14712598.2014.920813
Article PubMed Google Scholar
Chen C, Leydesdorff L (2014) Patterns of connections and movements in dual‐map overlays: A new method of publication portfolio analysis. J Assoc Inf Sci Technol 65(2):334–351. https://doi.org/10.1002/asi.22968
Chen J, Wang C, Tang Y (2022) Knowledge mapping of volunteer motivation: A bibliometric analysis and cross-cultural comparative study. Front Psychol 13:883150. https://doi.org/10.3389/fpsyg.2022.883150
Chen JY, Liu YD, Dai J, Wang CL (2023) Development and status of moral education research: Visual analysis based on knowledge graph. Front Psychol 13:1079955. https://doi.org/10.3389/fpsyg.2022.1079955
Chen K, Chan AH (2011) A review of technology acceptance by older adults. Gerontechnology 10(1):1–12. https://doi.org/10.4017/gt.2011.10.01.006.00
Chen K, Chan AH (2014) Gerontechnology acceptance by elderly Hong Kong Chinese: a senior technology acceptance model (STAM). Ergonomics 57(5):635–652. https://doi.org/10.1080/00140139.2014.895855
Chen K, Zhang Y, Fu X (2019) International research collaboration: An emerging domain of innovation studies? Res Policy 48(1):149–168. https://doi.org/10.1016/j.respol.2018.08.005
Chen X, Hu Z, Wang C (2024) Empowering education development through AIGC: A systematic literature review. Educ Inf Technol 1–53. https://doi.org/10.1007/s10639-024-12549-7
Chen Y, Chen CM, Liu ZY, Hu ZG, Wang XW (2015) The methodology function of CiteSpace mapping knowledge domains. Stud Sci Sci 33(2):242–253. https://doi.org/10.16192/j.cnki.1003-2053.2015.02.009
Codfrey GS, Baharum A, Zain NHM, Omar M, Deris FD (2022) User Experience in Product Design and Development: Perspectives and Strategies. Math Stat Eng Appl 71(2):257–262. https://doi.org/10.17762/msea.v71i2.83
Dai J, Zhang X, Wang CL (2024) A meta-analysis of learners’ continuance intention toward online education platforms. Educ Inf Technol 1–36. https://doi.org/10.1007/s10639-024-12654-7
Davis FD (1989) Perceived usefulness, perceived ease of use, and user acceptance of information technology. MIS Q 13(3):319–340. https://doi.org/10.2307/249008
Delmastro F, Dolciotti C, Palumbo F, Magrini M, Di Martino F, La Rosa D, Barcaro U (2018) Long-term care: how to improve the quality of life with mobile and e-health services. In 2018 14th International Conference on Wireless and Mobile Computing, Networking and Communications (WiMob), pp. 12–19. IEEE. https://doi.org/10.1109/WiMOB.2018.8589157
Dupuis K, Tsotsos LE (2018) Technology for remote health monitoring in an older population: a role for mobile devices. Multimodal Technol Interact 2(3):43. https://doi.org/10.3390/mti2030043
Ferguson C, Hickman LD, Turkmani S, Breen P, Gargiulo G, Inglis SC (2021) Wearables only work on patients that wear them”: Barriers and facilitators to the adoption of wearable cardiac monitoring technologies. Cardiovasc Digit Health J 2(2):137–147. https://doi.org/10.1016/j.cvdhj.2021.02.001
Fisk AD, Czaja SJ, Rogers WA, Charness N, Sharit J (2020) Designing for older adults: Principles and creative human factors approaches. CRC Press. https://doi.org/10.1201/9781420080681
Friesen S, Brémault-Phillips S, Rudrum L, Rogers LG (2016) Environmental design that supports healthy aging: Evaluating a new supportive living facility. J Hous Elderly 30(1):18–34. https://doi.org/10.1080/02763893.2015.1129380
Garcia Reyes EP, Kelly R, Buchanan G, Waycott J (2023) Understanding Older Adults’ Experiences With Technologies for Health Self-management: Interview Study. JMIR Aging 6:e43197. https://doi.org/10.2196/43197
Geng Z, Wang J, Liu J, Miao J (2024) Bibliometric analysis of the development, current status, and trends in adult degenerative scoliosis research: A systematic review from 1998 to 2023. J Pain Res 17:153–169. https://doi.org/10.2147/JPR.S437575
González A, Ramírez MP, Viadel V (2012) Attitudes of the elderly toward information and communications technologies. Educ Gerontol 38(9):585–594. https://doi.org/10.1080/03601277.2011.595314
Guner H, Acarturk C (2020) The use and acceptance of ICT by senior citizens: a comparison of technology acceptance model (TAM) for elderly and young adults. Univ Access Inf Soc 19(2):311–330. https://doi.org/10.1007/s10209-018-0642-4
Halim I, Saptari A, Perumal PA, Abdullah Z, Abdullah S, Muhammad MN (2022) A Review on Usability and User Experience of Assistive Social Robots for Older Persons. Int J Integr Eng 14(6):102–124. https://penerbit.uthm.edu.my/ojs/index.php/ijie/article/view/8566
He Y, He Q, Liu Q (2022) Technology acceptance in socially assistive robots: Scoping review of models, measurement, and influencing factors. J Healthc Eng 2022(1):6334732. https://doi.org/10.1155/2022/6334732
Heerink M, Kröse B, Evers V, Wielinga B (2010) Assessing acceptance of assistive social agent technology by older adults: the almere model. Int J Soc Robot 2:361–375. https://doi.org/10.1007/s12369-010-0068-5
Ho A (2020) Are we ready for artificial intelligence health monitoring in elder care? BMC Geriatr 20(1):358. https://doi.org/10.1186/s12877-020-01764-9
Hoque R, Sorwar G (2017) Understanding factors influencing the adoption of mHealth by the elderly: An extension of the UTAUT model. Int J Med Inform 101:75–84. https://doi.org/10.1016/j.ijmedinf.2017.02.002
Hota PK, Subramanian B, Narayanamurthy G (2020) Mapping the intellectual structure of social entrepreneurship research: A citation/co-citation analysis. J Bus Ethics 166(1):89–114. https://doi.org/10.1007/s10551-019-04129-4
Huang R, Yan P, Yang X (2021) Knowledge map visualization of technology hotspots and development trends in China’s textile manufacturing industry. IET Collab Intell Manuf 3(3):243–251. https://doi.org/10.1049/cim2.12024
Article ADS Google Scholar
Jing Y, Wang C, Chen Y, Wang H, Yu T, Shadiev R (2023) Bibliometric mapping techniques in educational technology research: A systematic literature review. Educ Inf Technol 1–29. https://doi.org/10.1007/s10639-023-12178-6
Jing YH, Wang CL, Chen ZY, Shen SS, Shadiev R (2024a) A Bibliometric Analysis of Studies on Technology-Supported Learning Environments: Hotopics and Frontier Evolution. J Comput Assist Learn 1–16. https://doi.org/10.1111/jcal.12934
Jing YH, Wang HM, Chen XJ, Wang CL (2024b) What factors will affect the effectiveness of using ChatGPT to solve programming problems? A quasi-experimental study. Humanit Soc Sci Commun 11:319. https://doi.org/10.1057/s41599-024-02751-w
Kamrani P, Dorsch I, Stock WG (2021) Do researchers know what the h-index is? And how do they estimate its importance? Scientometrics 126(7):5489–5508. https://doi.org/10.1007/s11192-021-03968-1
Kim HS, Lee KH, Kim H, Kim JH (2014) Using mobile phones in healthcare management for the elderly. Maturitas 79(4):381–388. https://doi.org/10.1016/j.maturitas.2014.08.013
Article MathSciNet PubMed Google Scholar
Kleinberg J (2002) Bursty and hierarchical structure in streams. In Proceedings of the eighth ACM SIGKDD international conference on Knowledge discovery and data mining, pp. 91–101. https://doi.org/10.1145/775047.775061
Kruse C, Fohn J, Wilson N, Patlan EN, Zipp S, Mileski M (2020) Utilization barriers and medical outcomes commensurate with the use of telehealth among older adults: systematic review. JMIR Med Inform 8(8):e20359. https://doi.org/10.2196/20359
Kumar S, Lim WM, Pandey N, Christopher Westland J (2021) 20 years of electronic commerce research. Electron Commer Res 21:1–40. https://doi.org/10.1007/s10660-021-09464-1
Kwiek M (2021) What large-scale publication and citation data tell us about international research collaboration in Europe: Changing national patterns in global contexts. Stud High Educ 46(12):2629–2649. https://doi.org/10.1080/03075079.2020.1749254
Lee C, Coughlin JF (2015) PERSPECTIVE: Older adults’ adoption of technology: an integrated approach to identifying determinants and barriers. J Prod Innov Manag 32(5):747–759. https://doi.org/10.1111/jpim.12176
Lee CH, Wang C, Fan X, Li F, Chen CH (2023) Artificial intelligence-enabled digital transformation in elderly healthcare field: scoping review. Adv Eng Inform 55:101874. https://doi.org/10.1016/j.aei.2023.101874
Leydesdorff L, Rafols I (2012) Interactive overlays: A new method for generating global journal maps from Web-of-Science data. J Informetr 6(2):318–332. https://doi.org/10.1016/j.joi.2011.11.003
Li J, Ma Q, Chan AH, Man S (2019) Health monitoring through wearable technologies for older adults: Smart wearables acceptance model. Appl Ergon 75:162–169. https://doi.org/10.1016/j.apergo.2018.10.006
Article ADS PubMed Google Scholar
Li X, Zhou D (2020) Product design requirement information visualization approach for intelligent manufacturing services. China Mech Eng 31(07):871, http://www.cmemo.org.cn/EN/Y2020/V31/I07/871
Google Scholar
Lin Y, Yu Z (2024a) An integrated bibliometric analysis and systematic review modelling students’ technostress in higher education. Behav Inf Technol 1–25. https://doi.org/10.1080/0144929X.2024.2332458
Lin Y, Yu Z (2024b) A bibliometric analysis of artificial intelligence chatbots in educational contexts. Interact Technol Smart Educ 21(2):189–213. https://doi.org/10.1108/ITSE-12-2022-0165
Liu L, Duffy VG (2023) Exploring the future development of Artificial Intelligence (AI) applications in chatbots: a bibliometric analysis. Int J Soc Robot 15(5):703–716. https://doi.org/10.1007/s12369-022-00956-0
Liu R, Li X, Chu J (2022) Evolution of applied variables in the research on technology acceptance of the elderly. In: International Conference on Human-Computer Interaction, Cham: Springer International Publishing, pp 500–520. https://doi.org/10.1007/978-3-031-05581-23_5
Luijkx K, Peek S, Wouters E (2015) “Grandma, you should do it—It’s cool” Older Adults and the Role of Family Members in Their Acceptance of Technology. Int J Environ Res Public Health 12(12):15470–15485. https://doi.org/10.3390/ijerph121214999
Lussier M, Lavoie M, Giroux S, Consel C, Guay M, Macoir J, Bier N (2018) Early detection of mild cognitive impairment with in-home monitoring sensor technologies using functional measures: a systematic review. IEEE J Biomed Health Inform 23(2):838–847. https://doi.org/10.1109/JBHI.2018.2834317
López-Robles JR, Otegi-Olaso JR, Porto Gomez I, Gamboa-Rosales NK, Gamboa-Rosales H, Robles-Berumen H (2018) Bibliometric network analysis to identify the intellectual structure and evolution of the big data research field. In: International Conference on Intelligent Data Engineering and Automated Learning, Cham: Springer International Publishing, pp 113–120. https://doi.org/10.1007/978-3-030-03496-2_13
Ma Q, Chan AH, Chen K (2016) Personal and other factors affecting acceptance of smartphone technology by older Chinese adults. Appl Ergon 54:62–71. https://doi.org/10.1016/j.apergo.2015.11.015
Ma Q, Chan AHS, Teh PL (2021) Insights into Older Adults’ Technology Acceptance through Meta-Analysis. Int J Hum-Comput Interact 37(11):1049–1062. https://doi.org/10.1080/10447318.2020.1865005
Macedo IM (2017) Predicting the acceptance and use of information and communication technology by older adults: An empirical examination of the revised UTAUT2. Comput Human Behav 75:935–948. https://doi.org/10.1016/j.chb.2017.06.013
Maidhof C, Offermann J, Ziefle M (2023) Eyes on privacy: acceptance of video-based AAL impacted by activities being filmed. Front Public Health 11:1186944. https://doi.org/10.3389/fpubh.2023.1186944
Majumder S, Aghayi E, Noferesti M, Memarzadeh-Tehran H, Mondal T, Pang Z, Deen MJ (2017) Smart homes for elderly healthcare—Recent advances and research challenges. Sensors 17(11):2496. https://doi.org/10.3390/s17112496
Article ADS PubMed PubMed Central Google Scholar
Mhlanga D (2023) Artificial Intelligence in elderly care: Navigating ethical and responsible AI adoption for seniors. Available at SSRN 4675564. 4675564 min) Identifying citation patterns of scientific breakthroughs: A perspective of dynamic citation process. Inf Process Manag 58(1):102428. https://doi.org/10.1016/j.ipm.2020.102428
Mitzner TL, Boron JB, Fausset CB, Adams AE, Charness N, Czaja SJ, Sharit J (2010) Older adults talk technology: Technology usage and attitudes. Comput Human Behav 26(6):1710–1721. https://doi.org/10.1016/j.chb.2010.06.020
Mitzner TL, Savla J, Boot WR, Sharit J, Charness N, Czaja SJ, Rogers WA (2019) Technology adoption by older adults: Findings from the PRISM trial. Gerontologist 59(1):34–44. https://doi.org/10.1093/geront/gny113
Mongeon P, Paul-Hus A (2016) The journal coverage of Web of Science and Scopus: a comparative analysis. Scientometrics 106:213–228. https://doi.org/10.1007/s11192-015-1765-5
Mostaghel R (2016) Innovation and technology for the elderly: Systematic literature review. J Bus Res 69(11):4896–4900. https://doi.org/10.1016/j.jbusres.2016.04.049
Mujirishvili T, Maidhof C, Florez-Revuelta F, Ziefle M, Richart-Martinez M, Cabrero-García J (2023) Acceptance and privacy perceptions toward video-based active and assisted living technologies: Scoping review. J Med Internet Res 25:e45297. https://doi.org/10.2196/45297
Naseri RNN, Azis SN, Abas N (2023) A Review of Technology Acceptance and Adoption Models in Consumer Study. FIRM J Manage Stud 8(2):188–199. https://doi.org/10.33021/firm.v8i2.4536
Nguyen UP, Hallinger P (2020) Assessing the distinctive contributions of Simulation & Gaming to the literature, 1970–2019: A bibliometric review. Simul Gaming 51(6):744–769. https://doi.org/10.1177/1046878120941569
Olmedo-Aguirre JO, Reyes-Campos J, Alor-Hernández G, Machorro-Cano I, Rodríguez-Mazahua L, Sánchez-Cervantes JL (2022) Remote healthcare for elderly people using wearables: A review. Biosensors 12(2):73. https://doi.org/10.3390/bios12020073
Pan S, Jordan-Marsh M (2010) Internet use intention and adoption among Chinese older adults: From the expanded technology acceptance model perspective. Comput Human Behav 26(5):1111–1119. https://doi.org/10.1016/j.chb.2010.03.015
Pan X, Yan E, Cui M, Hua W (2018) Examining the usage, citation, and diffusion patterns of bibliometric map software: A comparative study of three tools. J Informetr 12(2):481–493. https://doi.org/10.1016/j.joi.2018.03.005
Park JS, Kim NR, Han EJ (2018) Analysis of trends in science and technology using keyword network analysis. J Korea Ind Inf Syst Res 23(2):63–73. https://doi.org/10.9723/jksiis.2018.23.2.063
Peek ST, Luijkx KG, Rijnaard MD, Nieboer ME, Van Der Voort CS, Aarts S, Wouters EJ (2016) Older adults’ reasons for using technology while aging in place. Gerontology 62(2):226–237. https://doi.org/10.1159/000430949
Peek ST, Luijkx KG, Vrijhoef HJ, Nieboer ME, Aarts S, van der Voort CS, Wouters EJ (2017) Origins and consequences of technology acquirement by independent-living seniors: Towards an integrative model. BMC Geriatr 17:1–18. https://doi.org/10.1186/s12877-017-0582-5
Peek ST, Wouters EJ, Van Hoof J, Luijkx KG, Boeije HR, Vrijhoef HJ (2014) Factors influencing acceptance of technology for aging in place: a systematic review. Int J Med Inform 83(4):235–248. https://doi.org/10.1016/j.ijmedinf.2014.01.004
Peek STM, Luijkx KG, Vrijhoef HJM, Nieboer ME, Aarts S, Van Der Voort CS, Wouters EJM (2019) Understanding changes and stability in the long-term use of technologies by seniors who are aging in place: a dynamical framework. BMC Geriatr 19:1–13. https://doi.org/10.1186/s12877-019-1241-9
Perez AJ, Siddiqui F, Zeadally S, Lane D (2023) A review of IoT systems to enable independence for the elderly and disabled individuals. Internet Things 21:100653. https://doi.org/10.1016/j.iot.2022.100653
Piau A, Wild K, Mattek N, Kaye J (2019) Current state of digital biomarker technologies for real-life, home-based monitoring of cognitive function for mild cognitive impairment to mild Alzheimer disease and implications for clinical care: systematic review. J Med Internet Res 21(8):e12785. https://doi.org/10.2196/12785
Pirzada P, Wilde A, Doherty GH, Harris-Birtill D (2022) Ethics and acceptance of smart homes for older adults. Inform Health Soc Care 47(1):10–37. https://doi.org/10.1080/17538157.2021.1923500
Pranckutė R (2021) Web of Science (WoS) and Scopus: The titans of bibliographic information in today’s academic world. Publications 9(1):12. https://doi.org/10.3390/publications9010012
Qian K, Zhang Z, Yamamoto Y, Schuller BW (2021) Artificial intelligence internet of things for the elderly: From assisted living to health-care monitoring. IEEE Signal Process Mag 38(4):78–88. https://doi.org/10.1109/MSP.2021.3057298
Redner S (1998) How popular is your paper? An empirical study of the citation distribution. Eur Phys J B-Condens Matter Complex Syst 4(2):131–134. https://doi.org/10.1007/s100510050359
Sayago S (ed.) (2019) Perspectives on human-computer interaction research with older people. Switzerland: Springer International Publishing. https://doi.org/10.1007/978-3-030-06076-3
Schomakers EM, Ziefle M (2023) Privacy vs. security: trade-offs in the acceptance of smart technologies for aging-in-place. Int J Hum Comput Interact 39(5):1043–1058. https://doi.org/10.1080/10447318.2022.2078463
Schroeder T, Dodds L, Georgiou A, Gewald H, Siette J (2023) Older adults and new technology: Mapping review of the factors associated with older adults’ intention to adopt digital technologies. JMIR Aging 6(1):e44564. https://doi.org/10.2196/44564
Seibert K, Domhoff D, Bruch D, Schulte-Althoff M, Fürstenau D, Biessmann F, Wolf-Ostermann K (2021) Application scenarios for artificial intelligence in nursing care: rapid review. J Med Internet Res 23(11):e26522. https://doi.org/10.2196/26522
Seuwou P, Banissi E, Ubakanma G (2016) User acceptance of information technology: A critical review of technology acceptance models and the decision to invest in Information Security. In: Global Security, Safety and Sustainability-The Security Challenges of the Connected World: 11th International Conference, ICGS3 2017, London, UK, January 18-20, 2017, Proceedings 11:230-251. Springer International Publishing. https://doi.org/10.1007/978-3-319-51064-4_19
Shiau WL, Wang X, Zheng F (2023) What are the trend and core knowledge of information security? A citation and co-citation analysis. Inf Manag 60(3):103774. https://doi.org/10.1016/j.im.2023.103774
Sinha S, Verma A, Tiwari P (2021) Technology: Saving and enriching life during COVID-19. Front Psychol 12:647681. https://doi.org/10.3389/fpsyg.2021.647681
Soar J (2010) The potential of information and communication technologies to support ageing and independent living. Ann Telecommun 65:479–483. https://doi.org/10.1007/s12243-010-0167-1
Strotmann A, Zhao D (2012) Author name disambiguation: What difference does it make in author‐based citation analysis? J Am Soc Inf Sci Technol 63(9):1820–1833. https://doi.org/10.1002/asi.22695
Talukder MS, Sorwar G, Bao Y, Ahmed JU, Palash MAS (2020) Predicting antecedents of wearable healthcare technology acceptance by elderly: A combined SEM-Neural Network approach. Technol Forecast Soc Change 150:119793. https://doi.org/10.1016/j.techfore.2019.119793
Taskin Z, Al U (2019) Natural language processing applications in library and information science. Online Inf Rev 43(4):676–690. https://doi.org/10.1108/oir-07-2018-0217
Touqeer H, Zaman S, Amin R, Hussain M, Al-Turjman F, Bilal M (2021) Smart home security: challenges, issues and solutions at different IoT layers. J Supercomput 77(12):14053–14089. https://doi.org/10.1007/s11227-021-03825-1
United Nations Department of Economic and Social Affairs (2023) World population ageing 2023: Highlights. https://www.un.org/zh/193220
Valk CAL, Lu Y, Randriambelonoro M, Jessen J (2018) Designing for technology acceptance of wearable and mobile technologies for senior citizen users. In: 21st DMI: Academic Design Management Conference (ADMC 2018), Design Management Institute, pp 1361–1373. https://www.dmi.org/page/ADMC2018
Van Eck N, Waltman L (2010) Software survey: VOSviewer, a computer program for bibliometric mapping. Scientometrics 84(2):523–538. https://doi.org/10.1007/s11192-009-0146-3
Vancea M, Solé-Casals J (2016) Population aging in the European Information Societies: towards a comprehensive research agenda in eHealth innovations for elderly. Aging Dis 7(4):526. https://doi.org/10.14336/AD.2015.1214
Venkatesh V, Morris MG, Davis GB, Davis FD (2003) User acceptance of information technology: Toward a unified view. MIS Q 27(3):425–478. https://doi.org/10.2307/30036540
Wagner N, Hassanein K, Head M (2010) Computer use by older adults: A multi-disciplinary review. Comput Human Behav 26(5):870–882. https://doi.org/10.1016/j.chb.2010.03.029
Wahlroos N, Narsakka N, Stolt M, Suhonen R (2023) Physical environment maintaining independence and self-management of older people in long-term care settings—An integrative literature review. J Aging Environ 37(3):295–313. https://doi.org/10.1080/26892618.2022.2092927
Wang CL, Chen XJ, Yu T, Liu YD, Jing YH (2024a) Education reform and change driven by digital technology: a bibliometric study from a global perspective. Humanit Soc Sci Commun 11(1):1–17. https://doi.org/10.1057/s41599-024-02717-y
Wang CL, Dai J, Zhu KK, Yu T, Gu XQ (2023a) Understanding the Continuance Intention of College Students Toward New E-learning Spaces Based on an Integrated Model of the TAM and TTF. Int J Hum-comput Int 1–14. https://doi.org/10.1080/10447318.2023.2291609
Wang CL, Wang HM, Li YY, Dai J, Gu XQ, Yu T (2024b) Factors Influencing University Students’ Behavioral Intention to Use Generative Artificial Intelligence: Integrating the Theory of Planned Behavior and AI Literacy. Int J Hum-comput Int 1–23. https://doi.org/10.1080/10447318.2024.2383033
Wang J, Zhao W, Zhang Z, Liu X, Xie T, Wang L, Zhang Y (2024c) A journey of challenges and victories: a bibliometric worldview of nanomedicine since the 21st century. Adv Mater 36(15):2308915. https://doi.org/10.1002/adma.202308915
Wang J, Chen Y, Huo S, Mai L, Jia F (2023b) Research hotspots and trends of social robot interaction design: A bibliometric analysis. Sensors 23(23):9369. https://doi.org/10.3390/s23239369
Wang KH, Chen G, Chen HG (2017) A model of technology adoption by older adults. Soc Behav Personal 45(4):563–572. https://doi.org/10.2224/sbp.5778
Wang S, Bolling K, Mao W, Reichstadt J, Jeste D, Kim HC, Nebeker C (2019) Technology to Support Aging in Place: Older Adults’ Perspectives. Healthcare 7(2):60. https://doi.org/10.3390/healthcare7020060
Wang Z, Liu D, Sun Y, Pang X, Sun P, Lin F, Ren K (2022) A survey on IoT-enabled home automation systems: Attacks and defenses. IEEE Commun Surv Tutor 24(4):2292–2328. https://doi.org/10.1109/COMST.2022.3201557
Wilkowska W, Offermann J, Spinsante S, Poli A, Ziefle M (2022) Analyzing technology acceptance and perception of privacy in ambient assisted living for using sensor-based technologies. PloS One 17(7):e0269642. https://doi.org/10.1371/journal.pone.0269642
Wilson J, Heinsch M, Betts D, Booth D, Kay-Lambkin F (2021) Barriers and facilitators to the use of e-health by older adults: a scoping review. BMC Public Health 21:1–12. https://doi.org/10.1186/s12889-021-11623-w
Xia YQ, Deng YL, Tao XY, Zhang SN, Wang CL (2024) Digital art exhibitions and psychological well-being in Chinese Generation Z: An analysis based on the S-O-R framework. Humanit Soc Sci Commun 11:266. https://doi.org/10.1057/s41599-024-02718-x
Xie H, Zhang Y, Duan K (2020) Evolutionary overview of urban expansion based on bibliometric analysis in Web of Science from 1990 to 2019. Habitat Int 95:102100. https://doi.org/10.1016/j.habitatint.2019.10210
Xu Z, Ge Z, Wang X, Skare M (2021) Bibliometric analysis of technology adoption literature published from 1997 to 2020. Technol Forecast Soc Change 170:120896. https://doi.org/10.1016/j.techfore.2021.120896
Yap YY, Tan SH, Choon SW (2022) Elderly’s intention to use technologies: a systematic literature review. Heliyon 8(1). https://doi.org/10.1016/j.heliyon.2022.e08765
Yu T, Dai J, Wang CL (2023) Adoption of blended learning: Chinese university students’ perspectives. Humanit Soc Sci Commun 10:390. https://doi.org/10.1057/s41599-023-01904-7
Yusif S, Soar J, Hafeez-Baig A (2016) Older people, assistive technologies, and the barriers to adoption: A systematic review. Int J Med Inform 94:112–116. https://doi.org/10.1016/j.ijmedinf.2016.07.004
Zhang J, Zhu L (2022) Citation recommendation using semantic representation of cited papers’ relations and content. Expert Syst Appl 187:115826. https://doi.org/10.1016/j.eswa.2021.115826
Zhao Y, Li J (2024) Opportunities and challenges of integrating artificial intelligence in China’s elderly care services. Sci Rep 14(1):9254. https://doi.org/10.1038/s41598-024-60067-w
Article ADS MathSciNet PubMed PubMed Central Google Scholar
Download references
Acknowledgements
This research was supported by the Social Science Foundation of Shaanxi Province in China (Grant No. 2023J014).
Author information
Authors and affiliations.
School of Art and Design, Shaanxi University of Science and Technology, Xi’an, China
Xianru Shang, Zijian Liu, Chen Gong, Zhigang Hu & Yuexuan Wu
Department of Education Information Technology, Faculty of Education, East China Normal University, Shanghai, China
Chengliang Wang
You can also search for this author in PubMed Google Scholar
Contributions
Conceptualization, XS, YW, CW; methodology, XS, ZL, CG, CW; software, XS, CG, YW; writing-original draft preparation, XS, CW; writing-review and editing, XS, CG, ZH, CW; supervision, ZL, ZH, CW; project administration, ZL, ZH, CW; funding acquisition, XS, CG. All authors read and approved the final manuscript. All authors have read and approved the re-submission of the manuscript.
Corresponding author
Correspondence to Chengliang Wang .
Ethics declarations
Competing interests.
The authors declare no competing interests.
Ethical approval
Ethical approval was not required as the study did not involve human participants.
Informed consent
Informed consent was not required as the study did not involve human participants.
Additional information
Publisher’s note Springer Nature remains neutral with regard to jurisdictional claims in published maps and institutional affiliations.
Rights and permissions
Open Access This article is licensed under a Creative Commons Attribution-NonCommercial-NoDerivatives 4.0 International License, which permits any non-commercial use, sharing, distribution and reproduction in any medium or format, as long as you give appropriate credit to the original author(s) and the source, provide a link to the Creative Commons licence, and indicate if you modified the licensed material. You do not have permission under this licence to share adapted material derived from this article or parts of it. The images or other third party material in this article are included in the article’s Creative Commons licence, unless indicated otherwise in a credit line to the material. If material is not included in the article’s Creative Commons licence and your intended use is not permitted by statutory regulation or exceeds the permitted use, you will need to obtain permission directly from the copyright holder. To view a copy of this licence, visit http://creativecommons.org/licenses/by-nc-nd/4.0/ .
Reprints and permissions
About this article
Cite this article.
Shang, X., Liu, Z., Gong, C. et al. Knowledge mapping and evolution of research on older adults’ technology acceptance: a bibliometric study from 2013 to 2023. Humanit Soc Sci Commun 11 , 1115 (2024). https://doi.org/10.1057/s41599-024-03658-2
Download citation
Received : 20 June 2024
Accepted : 21 August 2024
Published : 31 August 2024
DOI : https://doi.org/10.1057/s41599-024-03658-2
Share this article
Anyone you share the following link with will be able to read this content:
Sorry, a shareable link is not currently available for this article.
Provided by the Springer Nature SharedIt content-sharing initiative
Quick links
- Explore articles by subject
- Guide to authors
- Editorial policies

- Publisher Home

A Critical Review of Medemer from Ideological Perspectives
Main article content.
Blended Ideology, Ethiopia, Ideologies, Medemer
Medemer, both as a book and as an idea, is trapped between two extremes: admiration and rejection. Writers from both ends offer their thoughts, criticisms, and reviews of Medemer. The aim of this article is to analyze Medemer from an ideological perspective. Raising some political and policy questions about Medemer’s application is also the aim of this article. This article used a qualitative research approach and comparative and critical research methods. Secondary sources of data were used to compare Medemer with various political ideologies. Ideologically, Medemer meets the three criteria (critics of the present order, vision of the future society, and theory of change). Medemer is a “blended ideology” that combines concepts, principles, and ideas from liberalism, socialism, conservatism, and fascism. Furthermore, Medemer ideology attempted to combine concepts from opposing political ideologies and proposed it as a solution to Ethiopia’s complex sociopolitical and economic problems. Despite the government’s claim that Medemer is Ethiopia’s redeemer, several political questions remain unanswered given the country’s current situation. Therefore, the country’s complicated sociopolitical and economic problems under the regime of the Prosperity Party challenged the applicability of Medemer. Is Medemer thus the sole (or perhaps the only) instrument, policy framework, ideology, and mechanism for resolving Ethiopia’s multiple, dynamic, and complex socio-political problems? These remain a point of contention among academics and politicians.
Article Metrics Graph
Article sidebar.
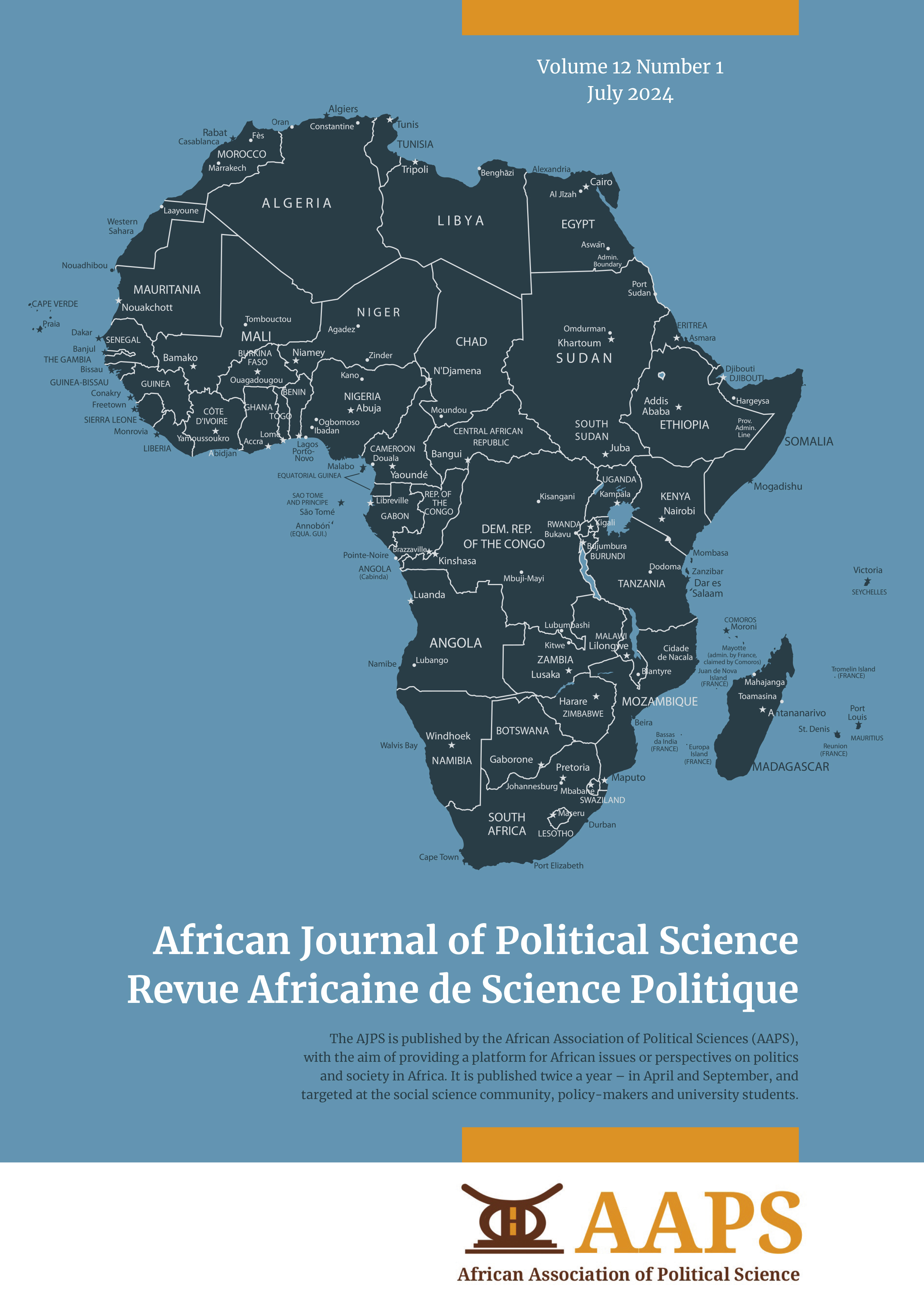
Article Details

This work is licensed under a Creative Commons Attribution-NonCommercial 4.0 International License .
Licensing information
Creative Commons CC Attribution-NonCommercial 4.0 International Public License
© Author(s)
How to Cite
- Endnote/Zotero/Mendeley (RIS)
Similar Articles
- Seife T.K., The Debate Between Conventional Ideology and Ethnic Politics in Africa , African Journal of Political Science: Vol. 10 No. 1 (2022)
- Shimelash Dagnew, Tebarek Lika Megento, Effects of Ethiopia’s landlocked status on ties with its neighbours , African Journal of Political Science: Vol. 12 No. 1 (2024)
- Merera Gudina, The State, Competing Ethnic Nationalisms and Democratisation in Ethiopia , African Journal of Political Science: Vol. 9 No. 1 (2004)
- Edmond J. Keller, Ethnic Federalism, Fiscal reform, Development and Democracy in Ethiopia , African Journal of Political Science: Vol. 7 No. 1 (2002)
- Nana Kwame Nkrumah, Daniel Dramani Kipo-Sunyehzi, Africa-China Relations and the Issue of South-South Cooperation , African Journal of Political Science: Vol. 12 No. 1 (2024)
You may also start an advanced similarity search for this article.
Modal Header
Lecturers’ perceptions of the influence of AI on a blended learning approach in a South African higher education institution
- Open access
- Published: 02 September 2024
- Volume 3 , article number 135 , ( 2024 )
Cite this article
You have full access to this open access article
- Debbie A. Sanders 1 , 2 &
- Shirley S. Mukhari 1 , 3
In this study, the researchers explore lecturers’ perspectives on the impact artificial intelligence (AI) has on blended learning within the context of South African higher education. AI is transforming traditional teaching and learning by enabling academic institutions to offer computerised, effective, and objective educational processes. The research was conducted to address the growing need to understand lecturers’ viewpoints on how AI can enhance educational practices and overcome existing challenges in blended learning environments. To investigate this phenomenon, the researchers applied the Substitution, Augmentation, Modification, and Redefinition (SAMR) model as theoretical framework for the study. Their qualitative research undertaking employed a singular case study design focusing on 15 lecturers from the College of Education at a selected academic institution, to arrive at an in-depth understanding of lecturers’ experiences and perceptions of how AI is integrated in blended learning. The researchers examined both the benefits and challenges associated with a blended teaching and learning mode, in the context of AI integration. The data collection process involved semi-structured focus group interviews that allowed for in-depth discussions to be conducted. This was complemented by detailed document analysis to analyse the course materials and teaching methods used by the lecturers. Homogeneous, purposeful sampling was applied to select participating lecturers who shared specific characteristics relevant to the study. Data analysis involved coding through the induction method, which helped to reveal relevant codes that were subsequently categorised. The study also included a comprehensive literature review of recent research findings, which were correlated with the collected data. The findings underscored the critical need for supportive measures, such as management backing, enhanced training opportunities, professional development initiatives, reliable technological infrastructure, improved internet connectivity, and additional time allocation, for the successful implementation of blended learning which integrates AI. This study contributes valuable insights into, and discussions on, the implications of adopting AI in a hybrid learning environment.
Explore related subjects
- Artificial Intelligence
Avoid common mistakes on your manuscript.
1 Introduction
Over the past few years there have been notable advances in supporting lecturers to enhance their teaching methods, and in improving students’ learning experiences through the adoption of blended learning. Defined as a combination of face-to-face (F2F) and online learning, blended learning offers more flexible learning experiences that are also deemed to be more effective. Also known as "brick-and-click" instruction, hybrid learning, dual-mode instruction, blended pedagogies, or HyFlex, targeted, multimodal or flipped learning [ 5 , 38 ], this approach is becoming increasingly popular. The approach, which combines traditional classroom F2F learning with online components, facilitates the application of asynchronous teaching and learning in educational settings [ 16 ]. In recent years, educational institutions have widely embraced blended learning as the preferred teaching method, expressing appreciation for its flexibility, timeliness, and uninterrupted learning opportunities. As hybrid learning gains popularity, so it has become increasingly important to find new ways of improving the effectiveness thereof [ 17 ]. Recent developments in artificial intelligence (AI) are one way of enhancing the efficacy of blended learning approaches. With the integration of AI into academic environments, individualised learning experiences can be provided, administrative tasks can be automated, and such systems can be adapted to student needs [ 20 , 44 ]. For these reasons, the researchers sought to understand lecturers’ views on the relationship between AI and blended learning, as those perspectives are crucial for developing effective teaching and learning practices in higher education contexts.
AI involves the study and development of computer programs that display ‘intelligent’ behaviour, mindful of the fact that machine intelligence is distinct from the natural intelligence that is inherent in humans and animals. Other definitions of AI examine efforts to enable computers to possess intelligence [ 19 ]. Ultimately, AI extends much further than just robotics, however, to include the human capacity to program computers and other technology-enabled devices, so that they comprehend the principles of intelligent thought and behaviour. As a key invention of the Fourth Industrial Revolution (4IR), AI is considered one of the most influential technologies of our time [ 19 ]. For the purposes of this research, AI will be taken to refer to the development of computer systems capable of performing tasks that typically require human intelligence, such as learning, problem solving, and decision making. From the point of view of lecturers, such integration would require them to adapt their teaching and learning approaches, to make them more efficient and effective in addressing diverse student needs [ 25 ].
It is against this background that the researchers felt the need to investigate what impact AI has on blended learning, which includes lecturers having to revisit the way in which they usually lecture (the educator teaching, and students listening and regurgitating what they have been taught), to scenarios where AI is infused into a hybrid learning approach. It is crucial to emphasise that, in the context of this paper, blended learning is deemed to comprise more than the mere incorporation of technology into an academic programme. The adoption of the term, in this instance, aligns with what Lee [ 24 ] describes as a hybrid teaching approach, integrating traditional F2F lecturing with the latest, updated technologies. This mode aims to enhance student success and promote the relevance of the course content. Interaction among students, and between lecturers and the student cohort, is accomplished through various internet-enabled learning technologies, including platforms such as online discussion forums [ 3 ]. These technologies play a crucial role in promoting communication between educational stakeholders. Consequently, the smooth integration of conventional classroom instruction with e-learning offers valuable support for students’ asynchronous and collaborative learning [ 15 ]. In addition, the use of AI supplements these interactions by providing personalised feedback, allowing for two-way discussions, and for learning resources to be adapted to individual students’ needs. This combination of traditional and e-learning environments through the adoption of AI technologies makes for a more engaging and effective educational experience. It improves educational access, and promotes inclusive and equitable education, resulting in a sustainable, efficient, and accessible system of blended learning [ 3 ].
Although blended learning is not a novel concept, its use has remained largely unchanged. Its numerous challenges require further and more in-depth research into its efficacy [ 5 , 38 ]. Various aspects, including the specific technological tools and learning approaches used, and the overall quality of the teaching and learning on offer, need examination [ 5 ]. While blended learning has long been used as an approach to enhance students’ learning experiences, much of the research has focused on countries in the Global North, such as Belgium, the United Kingdom (UK), and Italy [ 6 ]. Limited research has been conducted in the South African context in this regard [ 43 ]. Notably, a search on Google Scholar revealed that only minimal related research has been published in the past 5 years (only eight research resources), with none of them originating from South Africa. Despite the increased uptake of hybrid learning in academia, AI is often perceived as a separate technological tool with limited influence on teaching and learning approaches. To enhance the effectiveness of blended learning in higher education contexts, it is essential to identify and understand lecturers’ views on the incorporation of AI into their teaching and learning, taking into account the SAMR model [ 34 ].
The significance of the study thus lies in elucidating lecturers’ viewpoints on the impact which AI and blended learning have on teaching and learning. The researchers also set out to assist higher education institutions (HEIs) in creating, adapting or changing conditions so that they are more relevant and meaningful, and ultimately enable lecturers to ensure that students are more successful in achieving specific learning outcomes. Clearly, AI is a tool that must be embraced in this modern, ever-evolving technological world.
The main research question designed to guide the study, was:
How do lecturers perceive the influence of AI on blended learning in the context of a South African higher education institution?
Four sub-questions were also formulated in this regard:
How do lecturers in South African higher education institutions perceive and integrate AI technologies into blended learning lessons within the SAMR framework?
What challenges do lecturers identify when incorporating AI into blended learning lessons, considering the SAMR levels of substitution, augmentation, modification, and redefinition?
How does AI influence student engagement, interaction, and learning outcomes in blended learning environments?
What support mechanisms do lecturers require to ensure the successful incorporation of AI into blended learning lessons?
Following the above introductory discussion on lecturers’ perspectives on blended learning and AI integration, the sections which follow focus on a comprehensive literature review on the topic, the theoretical framework chosen for this research, an exploration of the selected research methodology, the findings, and recommendations for the successful implementation of blended learning infused with AI. Lastly, concluding remarks summarise the key findings, and outline implications for future research and educational practice.
After exploring the background and rationale for this study, it is crucial that this study examines the existing body of research related to blended learning and Artificial Intelligence integration in higher education which is the focus of the next section.
2 Literature review
2.1 blended learning as an approach to teaching and learning.
In recent years, the educational domain has experienced significant transformations, driven by the continued evolution of information technology. One notable outcome is the emergence of blended learning, a pedagogical approach that integrates diverse methods of delivering information, such as web-based software courses, coupled with the management of practical knowledge [ 33 ]. According to Damanik [ 12 ], Choi and Park [ 10 ], and Qiu et al. [ 35 ], blended learning can be implemented both on- and offline. Bozkurt [ 8 ] expands on this, emphasising that blended learning encompasses F2F interactions and online engagement through specific mediums. The positive impact of the blended learning model on students’ learning outcomes, through fostering heightened engagement, is echoed by Santosa et al. [ 39 ]. This model, as observed by Nugraha et al. [ 31 ], also enhances students’ problem-solving abilities and understanding of the module content. This ensures adaptability and flexibility that caters to individual students’ needs, preferences, and schedules [ 43 ]. While initially designed for specific modules and their content, this approach prioritises student-centred satisfaction [ 43 ], thereby supporting HEIs in pursuing their goals and ultimately achieving the successful attainment of the learning outcomes set [ 38 ]. At its core, the concept of blended learning is built on the understanding that learning is not a singular, isolated event, but rather an ongoing, continuous process [ 33 ]. This transformational shift aligns with the modification level of the SAMR model, as it goes beyond merely substituting traditional teaching methods with technology, instead modifying the entire learning experience. Admittedly, the development of efficient blended learning systems can be demanding, particularly in respect of their endurance and flexibility to adapt to modern technological developments [ 3 ].
The integration of Artificial Intelligence (AI) in blended learning environments has been the subject of increasing debate in recent years. A review of the literature reveals that while there are some global studies completed which have explored various aspects of AI in education, research originating from South Africa is notably sparse. Alshahrani [ 3 ], Ferry et al. [ 13 ], and Rahman et al. [ 37 ] have all examined the impact of AI on student engagement and learning outcomes in blended learning, highlighting the potential benefits of AI-driven feedback and personalised learning experiences. However, research from a South African context is underrepresented, which may limit the generalisability of these findings to local settings. This gap highlights the need for more region-specific studies, particularly in HEIs.
The year 2017 marked a significant milestone, with extraordinary and unique developments in our understanding of the possibilities of the merging of technology and AI. As a rapidly advancing field, AI has the potential to influence the future of information technology and, for this reason, training in that regard is imperative [ 33 ]. The study of AI is fascinating and intriguing, representing the future of information technology. AI has the potential to enhance people’s lives by ensuring that tasks are accomplished more rapidly and more accurately. Petrova [ 33 ] suggests that soon AI will be integrated into all platforms and technologies, across different spheres. This development represents a shift toward the redefinition level of Puentedura’s [ 34 ] SAMR model. It transcends the traditional roles of both lecturers and students and introduces new possibilities for teaching and learning through the use of technology. While there is still substantial work ahead, AI empowers lecturers to achieve more—and with greater efficiency—than ever before. In the past, AI was a technology that instilled fear in many. The notion that computers could think and learn like humans raised concerns about our ability to comprehend and constrain machines. However, as we move away from the pursuit of human-like AI, we can now view its progress as a tool serving to develop and enhance every industry [ 33 ].
AI stands out as a potential answer to improve the efficiency and durability of blended learning systems [ 3 , 23 ]. Through the use of AI techniques such as machine learning (ML), natural language processing (NLP), and chatbots, opportunities are created which allow for the automation of diverse features of the learning journey, including content delivery, assessment, and feedback [ 3 , 22 ]. Furthermore, AI allows for the customisation of the learning experience for individual students, ensuring increased engagement and enhancing learning outcomes [ 3 ]. The fact that AI makes it possible for lecturers to adapt and automate their teaching, represents a change in traditional teaching and learning methods, aligning with the substitution as well as modification levels of the SAMR model, as technology can be used as a direct substitute for conventional teaching and learning methods, while also accommodating or revealing new capabilities. It offers a vast range of new possibilities to help ensure the successful achievement of a module’s learning outcomes—something that was not possible with conventional approaches.
It became clear to the researchers that while relevant, limited studies on this theme exist in South Africa, Mhlanga [ 27 ] and Mokoena [ 28 ] explored the challenges and opportunities of implementing AI in South African HEIs. These studies highlight the need for more specific approaches that consider the unique socio-economic and technological constraints, such as limited access to high-speed internet and the variability in digital literacy among both students and staff. These insights are crucial for understanding how AI can be effectively integrated into blended learning environments in South Africa, ensuring that such integration is successful, equitable and sustainable.
Moreover, there is a critical need for research that addresses the localised implementation of AI-driven blended learning solutions, particularly in rural and under-resourced areas where access to technology is inconsistent [ 27 ]. Such studies would provide valuable insights into how AI can be utilised not only to enhance learning outcomes but also to bridge the educational disparities that persist across different regions of the country. While limited, some relevant studies do exist. Mhlanga [ 27 ] and Mokoena [ 28 ] explored the challenges and opportunities of implementing AI in South African HEIs. These studies highlight the need for more specific approaches that consider the unique socio-economic and technological constraints, such as limited access to high-speed internet and the variability in digital literacy among both students and staff. These insights are crucial for understanding how AI can be effectively integrated into blended learning environments in South Africa, ensuring that such integration is successful, equitable and sustainable.
Integrating AI into blended learning systems offers the potential to establish an education system that is not only efficient, but also sustainable. The use of AI in education, particularly in blended learning, revolves around delivering personalised learning experiences, and optimising course delivery [ 3 , 2 , 24 ]. Through the adoption of AI technology in hybrid learning systems, it is easier for lecturers to analyse student performance data for a personalised learning experience which aligns with individual strengths, weaknesses, and interests [ 3 , 25 ]. The implementation of AI in blended learning streamlines tailored assistance for students. Alshahrani [ 3 ] concurs that AI allows for responsive interaction. This corresponds to the augmentation (A) level of the SAMR [ 34 ] model, where technology is used to improve the learning experience, exceeding what was achievable with traditional methods. Personalised support can easily be based on individual student needs. The personalised approach assists students in navigating complex concepts, thus helping to ensure the achievement of learning outcomes, and ultimate success.
AI is also conducive to enhancing teaching and learning methods, increasing efficiency through automated administrative tasks, and refining content delivery. Introducing AI tools to ensure a sustainable and efficient blended learning system allows lecturers to lessen the strain on the environment, by reducing paper usage and minimising the carbon dioxide emissions associated with physical (F2F) lectures or meetings. This not only improves educational effectiveness and accessibility, but also empowers students to acquire the essential knowledge and skills for building a sustainable future [ 3 , 36 ].
Viktorivna et al. [ 40 ] point out that AI serves to enhance student engagement, and the effectiveness of their learning. AI also facilitates a more straightforward explanation of subject matter [ 32 ], thereby encouraging students to develop and enhance skills required in the twenty-first century [ 11 , 42 ]. AI is a valuable educational resource for blended learning, as it grants access to an ever-expanding range of learning materials. Furthermore, AI helps in the creation of lessons, quizzes, and rubrics which allow lecturers to reorganise the curriculum and content of a module. AI-generated resources can even be customised to align with students’ instructional preferences, thereby fostering a flexible and inclusive learning experience [ 3 ].
Various studies have shown that the infusion of AI in a blended learning module enriches the learning process for students, helping them attain specific learning outcomes [ 13 , 14 , 37 , 39 ]. The collaborative and conversational capabilities of AI enhance the overall learning experience, resulting in an enjoyment of the course and heightening active participation among the student cohort. Concerted engagement delivers improved learning outcomes, and a more profound understanding of the subject matter [ 3 ]. This aligns with the Augmentation (A) level of the SAMR model, as technology (AI in this instance) goes beyond merely enhancing traditional methods, to develop a more interactive and engaging learning environment, thus fostering increased student participation and leading to a better grasp of the subject matter.
The AI-based blended learning model boosts students’ digital literacy levels as well as their 21st-century thinking skills [ 37 ]. This innovative approach helps to improve their critical thinking skills, for use in the learning process [ 18 ]. Ultimately, models can be created using a variety of AI-based technologies, thereby saving lecturers time and enhancing students’ learning opportunities [ 37 ].
In higher education, large class sizes make it difficult for lecturers to offer individualised teaching and can impede swift and direct student support. AI negates this challenge by rendering personalised support. As such, AI delivers real-time answers and support, easing the workload on lecturers and enriching the learning experience. The rise in popularity of AI has initiated extensive discourse and research regarding its potential influence in the education sector, particularly in higher education where limited lecturer–student ratios present unique challenges [ 3 ]. Thus, it is clear that AI serves as a valuable asset in blended learning.
AI also enables the delivery of customised support, feedback, and motivation to students. Investigating these aspects will further our understanding of AI’s integration in blended learning, unveiling fresh insights to guide the design, implementation, and ethical use of related technologies in educational environments that adopt a hybrid learning approach [ 3 ]. Since this is a relatively new technological development and thus a relatively novel approach to teaching and learning, further research is needed, especially at HEIs, to analyse the exact impact on students’ performance. As Rahman et al. [ 37 ] concur, this approach needs further development. This research paper extends the current knowledge base in the field of blended learning, particularly in higher education, by providing insights into the integration of AI for enhanced student literacy, thereby filling a significant gap in the existing literature. Closing this gap will not only expand our understanding of emerging educational practices, but also provide valuable insights for educators, institutions, and policymakers aiming to optimise the student learning experience.
The follow section reviews the theoretical framework that guided this research.
3 Theoretical framework
For this research, the Substitution, Augmentation, Modification, and Redefinition (SAMR) theoretical model was selected, to establish a solid foundation for investigating intricate aspects of AI’s influence on blended learning in HEIs. The model was chosen for its applicability to an understanding of the transformative impact of AI on blended learning, within the South African higher education milieu.
As per Puentedura’s [ 34 ] SAMR model, digital technologies can either enhance or transform educational practice. Enhancement involves substitution without functional change, or augmentation with functional improvement. Transformation, by contrast, requires significant task redesign or redefinition, leading to the creation of new tasks that were previously inconceivable. The model, which explores the creative application of technology to enhance the learning experience, serves as a useful guide for lecturers facing pedagogical changes as a result of using new learning technologies in their courses [ 30 ].
The SAMR model comprises four hierarchical levels. Firstly, the substitution level in which technology is used as a direct substitute for a traditional tool, with no functional change. At this level, the lecturer is tasked with substituting an older technology to perform the same activities as previously. While this may set the stage for future development, it is unlikely to have a significant impact on student outcomes at this stage [ 30 ]. The second level, augmentation, prompts lecturers to consider whether or not the available technology improves their teaching and learning. Instead of merely observing how students performed a given task before, lecturers must now focus on specific features of the technology, to accomplish the task more effectively, informatively, and swiftly. This approach aims to enhance students’ performance in completing assigned tasks [ 30 ]. Thus, technology acts as a direct substitute, with some functional improvement. The third level is the modification level in which technology allows for significant task redesign and, during modification, the lecturers’ objectives are to successfully achieve lesson outcomes with technological assistance. Teaching methods are thus adapted to ensure the incorporation of technology. While the syllabus remains unchanged, teaching approaches are modified to enable students to attain new goals that were previously deemed challenging [ 30 ]. The final level of redefinition empowers lecturers to replace older teaching techniques with newer, more effective teaching ideas. This is achieved through the use of technology, which allows for the creation of tasks once deemed inconceivable [ 7 ]. These teaching methods mainly seek to capture and retain students’ attention [ 30 ].
The SAMR framework enhances the value of the accumulated data, by offering a decision-making model for assessing the design of research interventions. The lowest levels—substitution and augmentation—encourage participants to actively engage, thus overcoming challenges related to technology, pedagogy, and their consequences. At the higher levels—modification and redefinition—the design of research questions becomes crucial for considering potential challenges in participants’ understanding of increasingly complex topics. This approach aims to purposefully overcome obstacles associated with the evolving nature of the scheduled tasks [ 4 ].
The application of the SAMR model in the context of this research involved a comprehensive examination of how AI influences blended learning practices. At the Substitution level, the study explored how AI replaces or replicates traditional teaching methods, offering insights into its role in directly substituting conventional approaches. Moving to the Augmentation level, the research assessed how AI enhances or improves existing educational practices, particularly in terms of providing additional features or functionalities that support teaching and learning. The Modification level focused on analysing how AI introduces significant changes in the execution of educational tasks, transforming traditional methods into more dynamic and effective practices. Finally, at the Redefinition level, the study evaluated how AI facilitates entirely new and transformative educational practices that were previously unattainable, showcasing its potential to revolutionise blended learning environments in ways that were not possible before.
Using this framework ensured that the research could follow a systematic approach to assessing the influence AI exerts on blended learning. It allowed the research to progress from simple enhancements to transformative changes. By offering a structured method for assessing the extent of AI integration in various facets of teaching and learning, the researchers gained valuable insights into the evolving landscape of educational technologies.
It is against this background that the chosen methodology is discussed next.
4 Methodology
4.1 research approach.
This study applied a qualitative methodology to investigate lecturers’ views on the influence AI has on blended learning. A qualitative approach involves a thorough exploration and grasp of phenomena, using non-numerical data and highlighting context, meanings, and subjective experiences [ 21 ]. The researchers deemed this method best suited for its exploratory nature of extracting relevant information. Focus group interviews were conducted, as Islam and Aldaihani [ 21 ] suggest, to allow for the coordination of discussions among a small group of participants, to mine and gather their views on a specific topic or phenomenon. For this research the focus was on the modules, lessons, and assessments of the participating lecturers. In addition, the researchers employed document analysis, which enabled them to explore the actual course content, lesson plans, and discussion forums.
In this way, the researchers arrived at an in-depth understanding of the specific phenomenon under investigation, as proposed by Morgan [ 29 ]. This approach enabled the researchers to scrutinise the lecturers’ experiences and opinions, focusing on their knowledge of, and encounters with, AI and blended learning.
The researchers applied a singular case study research design. This involved focusing on a single participant or unit of analysis, for an in-depth exploration of the intricacies and dynamics of a specific case [ 1 ]. This approach made it possible to conduct a thorough examination of the perceptions of lecturers employed in the College of Education at the HEI in question. The choice of design was prompted by ongoing developments in both AI and blended learning, which enabled the researchers to gain insights from lecturers actively engaged in related emerging educational practices.
4.2 Population and sample
Identifying a population for a particular research study enables the researchers to gather pertinent information from a smaller representative sample. This ensures that each distinct element of the collected information with similar characteristics is given the opportunity to be part of the sample. The researchers opted to employ a homogeneous purposeful sampling technique, intentionally selecting a group of participants who shared specific characteristics or traits deemed relevant to the research objectives. Participants were thus chosen based on shared traits, including gender, age, years of experience, the college in which they lectured, and their use of AI and blended learning, in order to align with the study’s purpose and objectives (Table 1 ).
Here, the group of participants selected were part of the same college at the specific HEI. The criteria for selection encompassed their approachability, availability to actively participate in the study, responsiveness to the interview questions, and willingness to share the content of their modules, lessons, and assessments. For this study, 15 lecturers agreed to participate: two males and 13 females, ranging in age between 32 and 63. Importantly, age has an impact on a user’s acceptance and embrace of AI in teaching and learning. Older lecturers often express discomfort with new technology adoption, and tend to be resistant to change. They are usually more comfortable with traditional ways of teaching and are fearful of using cutting-edge technological innovations. The participants’ readiness to openly share their course content, lessons and assessments, assisted the researchers in effectively analysing the collected data through the chosen document analysis data-collection technique. Consequently, the participants contributed valuable information that enhanced the depth of the study. Their active involvement in university affairs (especially the teaching and learning programmes) provided information that was highly relevant to this research .
4.3 Data collection
For this study, data were acquired by conducting interviews with the participating lecturers, enhanced by document analysis (see appendices A and B). The application of these data-collection techniques enabled the researchers to gather pertinent insights into the lecturers’ practical encounters with AI and blended learning in their teaching and learning. The use of open-ended, semi-structured interviews, along with document analysis, facilitated the analysis of the data, thus ensuring a thorough and precise in-depth study of the subject matter. The thematic approach adopted in this research aimed to pinpoint repeated topics identified in the data gathered. This enabled the researchers to concentrate on emerging themes specific to the realm of AI and blended learning, rather than providing mere synopses of the data [ 9 ].
4.4 Data analysis
To gain valuable insights from the participants’ answers to the interview questions, and information derived from the document analysis, a thorough study and interpretation of the collected data was imperative—an analytical process which is crucial for answering the research questions effectively. The researchers actively engaged in interpreting, consolidating, and synthesising the lecturer participants’ statements, to assign meaning to the data. This involved transcribing, comparing, and scrutinising the interview responses, along with the content of the modules, lessons, and assessments. The participant responses were coded manually, using letters of the alphabet, to ensure anonymity. Each response was tagged with a corresponding letter, making it possible to trace every piece of data back to the specific participant who supplied it. Each statement was carefully linked to specific codes and themes, especially given the fact that AI does not replace F2F lecturing, but rather augments teaching and learning. The coding process involved categorising data into the SAMR [ 34 ] levels, to reach conclusions about how lecturers perceive AI's influence on different aspects of blended learning.
The thematic approach was used to identify patterns and themes in the data, which were then related back to Puentedura’s [ 34 ] SAMR model. This allowed for a comprehensive review of how AI is being used at different levels of integration in a specific hybrid learning environment. An inductive approach, specifically axial coding, was followed to analyse the data collected. This involved a systematic comparison of the gathered data to identify codes, categories, and subcategories. A natural analysis of the data, without preconceived notions, was achieved by using an inductive approach, which enabled an unbiased analysis of the lecturers’ actual experiences. Through this comparative analysis, the researchers aligned the collected data with information derived from the literature review. The adoption of these methodologies facilitated the analysis of findings, reinforcing the credibility and reliability of the data. The theoretical justifications for this approach included grounding the findings within the SAMR framework, to enable the data-analysis process to align with the study objectives and research questions throughout.
4.5 Trustworthiness in data collection and analysis
Ensuring the credibility and trustworthiness of research findings is the prerogative of every qualitative researcher. In this study, the researchers developed a lasting, reliable, and open relationship with the participants. This approach guaranteed the latter’s willingness to actively participate in the study, and to share their personal experiences of the impact which AI has on blended learning. Moreover, the lecturers were encouraged to review and offer feedback on the researchers’ summary of the interview responses, further confirming the accurate representation of all data, and strengthening the trustworthiness of the research.
The coding process for this study was primarily conducted by Researcher A who began the initial coding of the qualitative data, identifying preliminary themes and patterns. To enhance the reliability of the analysis, researcher B participated in the second phase, where both researchers reviewed and validated the initial codes and themes. This collaborative approach involved both open coding and axial coding and ensured a thorough and unbiased interpretation of the data. A critical reader provided feedback and suggestions, which helped refine the coding framework and resolve any discrepancies. This process promoted credibility by introducing different perspectives, which prevented individual prejudice and improved the accuracy of the data interpretation. Transparency was achieved by clearly documenting each researcher’s role and contributions, making the process open to scrutiny and validation by other future researchers. The method ensured the reliability and comprehensiveness of the data analysis and actual results.
The researchers adhered strictly to qualitative research principles, ensuring transparency in their data-collection methods and meticulousness in their data-analysis techniques. Participants were continually asked to check the researchers’ notes, interpretation of the interviews, and transcriptions (member checking). Detailed descriptions of the participants’ experiences were provided to enable the transferability of the findings. This precise approach guaranteed the reliability and validity of the findings. By integrating the findings from the interviews, document analysis, and literature review, the validity and trustworthiness of the conclusions were further enhanced. Through this methodological approach, the researchers ensured the trustworthiness of the research findings and were able to make informed recommendations based on the results reported on here.
4.6 Ethical issues
The chair of the department in which the research was undertaken, obtained comprehensive ethical clearance covering the entire department from the Research Ethics Review Committee of the College of Education of the particular HEI. This clearance authorises all researchers in the department to conduct research within the institution, under ethics clearance number 90060059MC.
In ensuring that the highest ethical standards were maintained, the researchers pledged to use codes to protect the identity and privacy of the participating lecturers. The lecturers were also required to give the researchers permission to record the interviews, and to analyse their module content, lessons, and assignments. They were explicitly informed that their participation was voluntary, and that they were free to withdraw from the study at any stage without fear of penalty.
4.7 Research findings
Here, the researchers summarise the outcomes of the research based on insights derived from the responses provided during the interviews with the participating lecturers, and the document analysis. The findings are organised to address the main research question and sub-questions.
4.8 Lecturers’ perceptions of incorporating AI technologies in their blended learning and teaching approach
Of the 15 participants interviewed, 12 reported using AI to ensure that student queries were answered, and that they could find additional information as required, thus personalising the entire academic journey. In the words of Lecturer H:
I use AI in my modules to ensure that students can easily obtain answers to their questions. It is an amazing tool which helps suggest supplementary resources based on students' progress. This ensures a learning experience which is better, as it is adapted to my students’ progress.
Lecturer C corroborated this:
These systems can answer questions, provide information, and simulate conversation, creating an amazing and enjoyable interactive environment.
The same 12 lecturers deemed AI very useful for facilitating discussions between lecturers and students, and students amongst themselves. This was achieved because AI streamlined communication, enhanced interaction, and provided valuable support. Lecturer F said:
AI has significantly improved communication channels; it allows me to develop interactive and engaging discussions between students and between students and myself, and even encourages students to discuss the course content amongst themselves.
Lecturer H concurred:
The use of AI chatbots has created a space for students to collaborate effectively. This offers immediate assistance and helps develop a sense of collaboration in our blended learning environment.
All the interviewees maintained that the use of AI to generate relevant and customised learning materials and assessments was a very useful feature that could easily be adopted in blended learning modules. In this regard, Lecturer C said:
I use AI to create customised learning materials, quizzes and even games that align with the specific learning outcomes of my modules.
Lecturer G stated:
I find that the fact that AI can create adaptive assessments that adjust difficulty levels based on the individual performance of my students, is very useful.
Five participants highlighted the value of AI for translation. This was considered extremely useful, particularly in the South African context with 11 official languages. Lecturer M explained:
The ability of AI to facilitate translation greatly benefits our students from diverse backgrounds. It is so easy for any of us [lecturers and students] to quickly translate a word or even a whole paragraph, which makes the understanding of the module so much easier.
Lecturer H added:
I find that it helps students who are more comfortable in their home language to participate in the course content. This ensures that learning materials are accessible to everyone, regardless of their language preference.
The researchers’ document analysis showed that lecturers who mentioned the benefits of AI for creating customised learning materials and adaptive assessments had indeed merged these elements into their module sites. This correlated with the findings obtained from the interviews, where 12 of the 15 participating lecturers highlighted the positive impact AI had on facilitating communication, enhancing interaction, and offering support in hybrid learning environments. In addition, the analysis revealed instances where AI tools were used to support F2F classes by providing real-time feedback and interactive activities, thus enriching the blended learning experience. Using technology to individualise learning experiences and adapt teaching strategies in real-time helps students adapt to such approaches, thereby supporting traditional teaching methods and enriches learning environments.
Lecturer C had integrated AI-generated, scenario-based case studies into the course material. The document analysis revealed a scenario related to cultural integration through language teaching and learning. Students were presented with a case study involving a classroom with learners from diverse linguistic and cultural backgrounds. They were tasked with designing a language lesson that not only focused on language acquisition, but also promoted cultural understanding and integration. AI was used to evaluate the students’ answers to the case study. Based on individual performance, the system provided feedback to each individual student, and suggested additional resources or challenges to focus on specific areas of improvement in designing the language lesson.
The document analysis (as outlined in the second criterion, which aimed to “examine evidence of how assessments reflect the unique contributions of AI to student learning outcomes”) also ascertained the presence of adaptive assessments that were able to adjust complexity levels based on individual student performance. Lecturer G, who felt that AI was beneficial for creating such assessments, had incorporated quizzes with dynamic difficulty levels into the module site. Students were able to complete personalised assessments, with questions based on their previous performance.
The researchers noted the integration of AI-based translation services. Lecturer M, who highlighted the value of AI for translation, had implemented an AI-driven language translation tool on the module site. The researchers noted that some students had translated sections of the course content into their preferred language, promoting inclusivity and ensuring that the specific learning materials were clear to everyone, regardless of their language preference.
4.9 Lecturers’ perspectives on the challenges of incorporating AI into blended learning
Four of the lecturers interviewed, described the adaptation of new methods of teaching and learning, when using AI in their blended learning modules, as a challenge. In response to interview question 5 (What challenges have you encountered when incorporating AI into blended learning, and how did you overcome them?), Lecturer H commented:
Incorporating AI into my modules requires a delicate balance. I found that at times AI tends to minimise the importance of traditional teaching and learning methods, and not actually enhance them.
Lecturer B said:
Finding the right blend is crucial, so students benefit from the best of both worlds. AI must enrich my module and definitely not disrupt it … [We have to find] a balance between the technology and the personalised touch.
Lecturer C indicated:
… it can be a challenge to decide exactly where AI should be incorporated into the actual content of the course. Determining this often requires me to rethink my learning outcomes and approaches to teaching the content of my modules.
Twelve participants expressed the view that resistance to change was a major impediment to the successful adaptation of AI in blended learning modules. This aligns with responses to Interview Question 9 (In your experience, what support or resources do lecturers currently require when implementing AI in blended learning?) where Lecturer G noted:
Change is always met with resistance, especially when it comes to technology, particularly amongst us older lecturers. Some may see AI as a threat to the traditional way of teaching.
Lecturer H stated:
There's a comfort in the familiar, and AI represents a significant shift. Overcoming resistance requires effective communication. It also requires practically exploring the uses and benefits of AI.
All the participants mentioned that, although AI definitely saved time, problems were experienced with finding additional time to investigate new technologies and adapt their modules accordingly. In the words of Lecturer A:
While AI streamlines certain processes, the challenge lies in actually finding dedicated time for exploring its full potential to ensure that AI helps both me and my students successfully achieve the outcomes of the specific module.
Lecturer G mentioned:
Despite the efficiency AI brings, we must confront the reality of time constraints. It is essential to find a balance between adopting new technologies and meeting existing teaching demands.
Eight participants mentioned that it was becoming increasingly challenging to cope with the problem of the “digital divide”, which pertains to the technological proficiency of the students. Lecturer E noted:
There's a noticeable difference in access to technology among our students, and it's becoming increasingly challenging for us lecturers to bridge this gap as a result of the fast pace of new technological developments.
Lecturer F concurred, adding:
The issue of unequal access is growing. We need effective strategies to ensure all students are given equal learning experiences, regardless of their experience using computers for actual learning.
Several lecturers discussed ethical and privacy-related challenges with regard to the integration of AI in their blended learning module. As Lecturer F indicated:
I find that a huge challenge is that of ethical considerations, especially with regard to the privacy of student data. Finding the correct balance between using AI and protecting our students' privacy is an ongoing challenge. Additionally, there's a need for clear guidelines from management on how AI should be used ethically in our teaching, to avoid unintended consequences.
Lecturer G opined:
The challenge lies in providing the benefits of using AI to achieve the outcomes of our modules without compromising the privacy rights of our students. Open discussions on ethical guidelines and continuous awareness among lecturers and management as well as lecturers and students [are] essential to overcoming these challenges successfully.
4.10 Lecturers’ perspectives on AI's impact on student engagement
All 15 participating lecturers noted that using AI in their blended learning modules was beneficial, but not all believed they were using AI to its full potential, admitting there was room for improvement. Lecturer F stated:
While AI has enhanced certain aspects of my lecturing and interaction with my students, I really feel that there's much further potential for the use of AI in my modules, especially with regard to the advanced AI functionalities and typing in the correct prompts.
Lecturer O opined:
Integrating AI into blended learning helps me improve the actual teaching of the content of my modules. This allows me to individualise the learning experiences of each of my students, to ensure that their needs and preferences are met.
Lecturer A agreed:
Using AI in my blended learning course helps me adapt to my students' needs. This makes the teaching and learning much more flexible and meaningful, as it allows me to develop an individualised teaching approach to each student's strengths and weaknesses.
Nine of the participants highlighted the significance of AI’s prompt feedback to the inputs provided and queries posted on the AI system. In response to interview question 6 ("Have you received any feedback from students regarding their experiences with AI-infused blended learning?"), Lecturer B mentioned,
The quick feedback of AI has really changed the learning experience. Students receive real-time feedback [on] their progress, allowing them to make [the] necessary changes immediately.
Lecturer K echoed this:
I see AI as a game changer. Its ability to offer instant, personalised feedback has been a real […] eye-opener. It helps students understand their strengths and weaknesses without delay. This helps ensure a more integrated and authentic learning environment. It helps in identifying gaps in understanding and adapting teaching strategies.
Lecturer N concurred, adding:
From where I stand, AI's ability to analyse student data can provide valuable insights for personalised teaching and learning, and allows for instantaneous feedback. As a result, students' entire learning process is enhanced, resulting in an improved ability to achieve their learning goals.
Lecturer B, who viewed the instant feedback of AI as beneficial for enhancing teaching and learning, had used AI to create scenario-based feedback activities. The document analysis identified instances where students were presented with virtual scenarios representing diverse language teaching situations, such as classroom settings, one-on-one tutoring sessions, and language immersion programmes. AI was able to instantaneously analyse students' responses and actions in each scenario, providing immediate, real-time personalised feedback on their answers. This integration of AI thus enhanced both asynchronous learning and synchronous F2F interactions, by offering immediate feedback during live sessions.
The interview responses of seven of the participants revealed that AI is able to easily automate administrative tasks, through machine learning algorithms and natural language processing. This analytical capability allows instructional approaches to be adapted to individual student needs, ensuring that they successfully attain the learning outcomes of the module. Lecturer C said:
AI tools can streamline administrative tasks, allowing me to devote more time to my students and support them, especially where they are encountering challenges.
Lecturer F added:
I've used AI to analyse student performance data, which helps me adapt the content of my modules and teaching methods to make them more interactive. This can easily be based on my individual students’ needs.
The document analysis, which aimed to examine evidence of how assessments reflect the unique contributions of AI to student learning outcomes (the fourth criterion on the document analysis) also showed that modules where AI was integrated into feedback mechanisms saw improved student engagement. Studying the module site of Lecturer F, the researchers discovered that s/he used AI to automatically grade assignments (multiple-choice and written) and give immediate feedback. The reports generated were instantaneous and showed specific trends which helped the lecturer adapt the teaching and learning of this particular module.
It is indeed important to note how AI supports F2F teaching in class. As a result of this approach, learning during live lectures is made more dynamic and responsive to student needs. This point was highlighted by Lecturer M, who said:
The use of AI tools allows for instantaneous feedback to my students’ questions during lectures. It can give them various suggestions for additional materials and let them engage in interactive activities during face-to-face classes that will allow them to engage more deeply with the material.
4.11 Lecturers’ perspectives on the support they require to successfully implement AI in blended learning
All the participating lecturers confirmed the importance of comprehensive training and professional development. The need for comprehensive training and institutional support emerged as a critical theme. Interview Question 8 ("What kind of training or professional development opportunities do you believe are necessary for lecturers to effectively integrate AI into their blended teaching methods?") prompted responses highlighting the importance of ongoing professional development. In the words of Lecturer G:
Access to ongoing professional development courses focused on AI is essential for us lecturers to keep up to date with the latest developments in this field.
Lecturer M noted:
Professional development should include […] theoretical knowledge of AI as well as, specifically for us, its practical application in blended learning contexts.
Four participants stated that technological support was imperative if AI was to be instituted successfully. Lecturer O suggested:
Dedicated support teams must be specifically set up to assist with any technical challenges we may come across during the implementation of AI into our teaching and learning. This includes prompt responses to technical glitches and troubleshooting, to ensure that everything works properly for both me and my students.
We need assistance with initial setup and implementation, and with ongoing technical issues that may arise. This could be problematic as our IT help desk is already so overburdened. More IT staff definitely need to be employed.
Having institutional support for incorporating AI into the curriculum, is crucial. This involves not only providing resources, but also creating a culture that values and encourages the integration of AI technologies into teaching practices. This was echoed by all the lecturers interviewed. In the words of Lecturer A:
Having institutional support for incorporating AI into the curriculum is crucial. This involves providing resources as well as creating an institution that values and encourages the integration of AI into our teaching and learning.
Lecturer O echoed this:
Institutional commitment is key to the successful integration of AI. This should also include dedicated policies, so that we lecturers know exactly the correct process of AI.
Additionally, setting aside dedicated time for lecturers to adopt AI technologies was deemed imperative, as mentioned by ten of the participants. Lecturer H opined:
Allocating specific time for training and hands-on experience with AI tools is crucial. We need the opportunity to explore and familiarise ourselves with this new, exciting technology. This will definitely help us.
Lecturer E noted:
Having dedicated time for learning and experimentation is essential. This would give us more confidence in the actual implementation. But our schedules are already so busy that I have to wonder if this is at all possible.
Next, we examine the findings of the research.
5 Discussion of research findings
Using the research findings as a starting point for drawing meaningful conclusions and contributing to scholarly discourse on the subject, this section provides a summary of the findings that correlate with the literature review. From the utterances of many of the participants it became clear that there is a positive attitude towards AI, its significance for blended learning, and the benefits for tertiary students, as long as HEIs make certain adaptations. This aligns with the Redefinition and Modification aspects of the SAMR [ 34 ] model used for this study.
The research questions sought to explore how AI influences student engagement, interaction, and learning outcomes in blended learning environments. Lecturer N’s opinion, that AI boosts the learning process as a whole, resulting in an improved ability to successfully complete the course , is consistent with the findings of Alshahrani [ 3 ], Ferry et al. [ 13 ], Fradila et al. [ 14 ], Rahman et al. [ 37 ] and Santosa et al. [ 39 ], who found that infusing AI into a blended learning module enriches the learning process for students, helping them to achieve the specified learning outcomes. The collaborative and conversational capabilities of AI enhance the overall learning experience, leading to an enjoyment of the course, and active participation by students. These findings support the SAMR [ 34 ] model’s Redefinition level, where AI transforms the learning experience. Accordingly, the researchers of this study recognised that while AI does enhance learning experiences, its integration must be carefully managed to avoid over-reliance on technology at the expense of fundamental pedagogical principles.
The research findings corroborate the potential benefits AI holds for blended learning, as identified by the interviewees. Lecturer H's use of AI for immediate student support aligns with the views of Alsaleem and Alghalith [ 2 ], Alshahrani [ 3 ] and Lee [ 24 ], who emphasise AI’s capacity for personalising learning experiences. Moreover, Lecturer B's opinion on the importance of using AI for the prompt integration of AI-driven feedback, is consistent with the findings of Alshahrani [ 3 ] and Khosravi and Heidari [ 22 ], which emphasise AI’s functionality of supplying instantaneous feedback to enhance the learning experience. This aligns with the Augmentation level of the SAMR [ 34 ] model, where AI enhances existing teaching and learning practices. This made it clear to the researchers that while AI-driven feedback can significantly improve learning efficiency, it also raises concerns about data privacy and the need for transparent feedback mechanisms.
The views of Weber et al. [ 41 ]—that resistance to change may be an obstacle to the effective implementation of AI—are consistent with the opinions of 12 of the study participants. Specifically, Lecturer G noted that transformation is often met with resistance, especially when it comes to technology, and AI may be perceived as a risk to the conventional mode of teaching. Addressing this resistance requires policy interventions and professional development programs to ease the transition and encourage AI adoption. This indicated to the researchers that creating a culture of continuous improvement and gradually embracing this new approach may prevent resistance to adopting AI by lecturers and their higher education institutions.
The perspectives of all the participants, as regards the significance of tailored training and professional development which are customised to their specific needs, align with the findings of Luckin et al. [ 26 ]. According to that study, training should be more specific, and be contextually relevant to the unique demands and settings of the educational environment. This approach encourages active engagement and participation. Lecturer M specifically noted that any related training should focus mainly on its application to blended learning, to be successful. This highlights the importance of ongoing professional development to keep pace with technological advances. Clearly, HEIs need to adapt their policies to integrate AI tools that support personalised and interactive learning experiences. This suggested to the researchers that for AI technologies to be successful in higher education, professional development programmes must be made easily accessible for lecturers.
Finally, as featured in Alshahrani’s [ 3 ] study, the ethical use of AI in educational environments that adopt a blended learning approach, must be considered. Two participants (F and G) expressed the same sentiment, stating that open discussions on ethical guidelines and continuous dialogue among lecturers, management, and students are essential for navigating these issues. This suggests that policy should include ethical guidelines for AI use in education, ensuring that such integration supports not only academic integrity, but also responsible teaching and learning practices. In view of these findings, the researchers concluded that there was a distinct need for the creation of specific ethical frameworks that would assist all stakeholders to address the emerging ethical concerns associated with AI use in higher education institutions.
5.1 Limitations of the research
It is important to note the limitations of this study, which affect the generalisability of the findings. First, the study was restricted to a single South African higher HEI and one specific college, which may limit the applicability of the results to other contexts or institutions. Additionally, the full impact of AI on the blended learning approach may only become apparent in the future, as the students from this cohort progress in their careers and enter their respective professions. Furthermore, AI is a rapidly evolving field, and its continual advancements could mean that the study’s findings might become outdated relatively quickly. Finally, the successful implementation of AI in blended learning modules may be hindered by the lack of requisite technological resources and infrastructure in some educational institutions, potentially affecting the feasibility and effectiveness of AI integration.
6 Conclusion and recommendations
This paper discussed the impact of AI on a blended approach to teaching and learning in a particular HEI. It was based on the perceptions of 15 participating lecturers who lecture in the same college, albeit in different departments. The insights were based on the lecturers’ familiarity, experiences of, and involvement with, AI, and its impact on their teaching and learning. This positioned them to discuss the perceived advantages, disadvantages and supportive measures needed for such an approach to be successful. The use of focus group interviews and document analysis enabled the researchers to correlate what was actually taking place in this field of research, with the literature review undertaken.
Puentedura’s [ 34 ] SAMR model was chosen as theoretical framework to guide this undertaking, since it enabled the researchers to investigate how AI could bring about transformative changes in blended learning within the domain of higher education. The results highlight the significance of using AI in hybrid learning contexts, which has great potential for transforming traditional teaching methods. The study highlighted the implications of adopting AI to enhance the effectiveness of blended learning which offers personalised feedback, interactive discussions, and adaptive resources to cater to individual student needs. The findings draw attention to the crucial role of supportive measures such as management backing, improved training and professional development opportunities, reliable technological infrastructure, and improved internet connectivity, in ensuring the successful use of AI for blended learning modules. The findings thus enhance the knowledge base of this emerging field of study, by clarifying the perspectives of the lecturer participants at a particular HEI. Moreover, the findings can support future research on this topic, and may be used by other educational institutions—even those catering for different age groups.
6.1 Recommendations for further research
Recommendations for further research include several key areas to enhance the understanding and implementation of AI in blended learning environments. First, investigating AI and blended learning across various HEIs, both within South Africa and internationally, would provide a more comprehensive understanding of lecturers' perceptions of AI's impact. Additionally, research should focus on the effect of AI on students’ achievement of learning outcomes, their engagement with modules, and their overall enjoyment of learning within hybrid environments. Examining specific support measures, particularly relevant training, could further assist lecturers in effectively integrating AI into their modules. Longitudinal studies are also recommended to track changes in lecturers’ perceptions as they adapt to and integrate AI over time. A thorough exploration of the challenges HEIs face during the implementation process should be considered to address potential barriers. Furthermore, research into the ethical implications of AI in education, including the development of necessary guidelines, is essential. Finally, future studies should aim to validate and expand upon these findings using quantitative methods, as this study was purely qualitative.
Data availability
The data that support the findings of this study are not openly available due to the privacy and confidentiality agreements with the participants. However, the data will be made available by the corresponding author upon reasonable request, subject to review and approval by the research ethics committee of the involved institution. Requests for data access can be made by contacting the corresponding author at [email protected].
Allan G. Qualitative research. In: Allan G, Skinner C, editors. Handbook for research students in the social sciences. Routledge; 2020. p. 177–89. https://doi.org/10.4324/9781003070993-18 .
Chapter Google Scholar
Alsaleem B, Alghalith A. Blended learning using artificial intelligence. IEEE Access. 2019;7:60275–85. https://doi.org/10.7897/j.ijdns.2023.7.750 .
Article Google Scholar
Alshahrani A. The impact of ChatGPT on blended learning: current trends and future research directions. Int J Data Netw Sci. 2023;7(4):2029–40. https://doi.org/10.5267/j.ijdns.2023.6.010 .
Arantes J. The SAMR model as a framework for scaffolding online chat: a theoretical discussion of the SAMR model as a research method during these “interesting” times. Qual Res J. 2022;22(3):294–306. https://doi.org/10.1108/qrj-08-2021-0088 .
Ashraf MA, Zhang MYY, Denden M, Tlili A, Liu J, Huang R, Burgos D. A systematic review of systematic reviews on blended learning: trends, gaps and future directions. Psychol Res Behav Manag. 2021;14(1):1525–41. https://doi.org/10.2147/prbm.s331741 .
Birgili B, Seggie FN, Oğuz E. The trends and outcomes of flipped learning research between 2012 and 2018: a descriptive content analysis. J Comput Educ. 2021;8:1–30. https://doi.org/10.1007/s40692-021-00183-y .
Blundell CN, Mukherjee M, Nykvist S. A scoping review of the application of the SAMR model in research. Comput Educ Open. 2022;3: 100093. https://doi.org/10.1016/j.caeo.2022.100093 .
Bozkurt A. A retro perspective on blended/hybrid learning: systematic review, mapping and visualization of the scholarly landscape. J Interact Media Educ. 2022;2022(1):1–15. https://doi.org/10.5334/jime.751 .
Braun V, Clarke V. Conceptual and design thinking for thematic analysis. Qual Psychol. 2022. https://doi.org/10.1037/qup0000196 .
Choi Y, Park N. The improvement of attitudes toward convergence of preservice teachers: Blended learning versus online learning in Science teaching method courses. J Curric Teach. 2022;11(5):87–94. https://doi.org/10.5430/jct.v11n5p87 .
Cope B, Kalantzis M, Searsmith D. Artificial intelligence for education: knowledge and its assessment in AI-enabled learning ecologies. Educ Philos Theory. 2021;53(12):1229–45. https://doi.org/10.1080/00131857.2020.1728732 .
Damanik EL. Blended learning: an innovative approach on social sciences at indonesian higher education. Educ Quart Rev. 2020;3(1):52–65. https://doi.org/10.31014/aior.1993.03.01.117 .
Ferry D, Santosa T, Kamil D. Pengetahuan mahasiswa institut agama Islam negeri Kerinci tentang teori asal usul manusia [Knowledge of students at the State Islamic Institute of Kerinci about the theory of human origins]. BIOEDUCA J Biol Educ. 2020;1(1):12–7. https://doi.org/10.21580/bioeduca.v1i1.4945 .
Fradila E, Razak A, Santosa TA, Arsih F, Chatri M. Development of e-module-based problem-based learning (PBL) applications using Sigil—the course ecology and environmental education students master of biology. Int J Progr Sci Technol. 2021;27(2):673–82. https://doi.org/10.52155/ijpsat.v27 .
Geng S, Law KMY, Niu B. Investigating self-directed learning and technology readiness in blending learning environment. J Educ Technol High Educ. 2019;16(1):1–22. https://doi.org/10.1186/s41239-019-0147-0 .
Gunes S. What are the perceptions of the students about asynchronous distance learning and blended learning? World J Educ Technol Curr Issues. 2019;11(4):230–7. https://doi.org/10.18844/wjet.v11i4.4274 .
Hamadneh NN, Atawneh S, Khan WA, Almejalli KA, Alhomoud A. Using artificial intelligence to predict students’ academic performance in blended learning. Sustainability. 2022;14(18):11642. https://doi.org/10.3390/su141811642 .
Harahap F, Nasution NEA, Manurung B. The effect of blended learning on students’ learning achievement and science process skills in plant tissue culture course. Int J Instr. 2019;12(1):521–38. https://doi.org/10.29333/iji.2019.12134a .
Hassani H, Silva ES, Unger S, TajMazinani M, Mac Feely S. Artificial intelligence (AI) or intelligence augmentation (IA): what is the future? AI. 2020;1(2):8.
Hinojo Lucena FJ, Lopez Belmonte J, Fuentes Cabrera A, Trujillo Torres JM, Pozo Sanchez S. Academic effects of the use of flipped learning in physical education. Int J Environ Res Public Health. 2020;17(1):276. https://doi.org/10.3390/ijerph17010276 .
Islam MA, Aldaihani FMF. Justification for adopting qualitative research method, research approaches, sampling strategy, sample size, interview method, saturation, and data analysis. J Int Bus Manag. 2022;5(1):1–11. https://doi.org/10.37227/jibm-2021-09-1494 .
Khosravi F, Heidari M. The role of artificial intelligence in designing a sustainable blended learning system. In: Procedures of the 2019 international conference on education and E-learning. Atlantis Press. 2019; p. 44–48.
Kizilcec R, Williams J, Bailenson J. Virtual classrooms: how online college courses affect student success. Comput Educ. 2015;88:14–24.
Google Scholar
Lee J. A study on application of artificial intelligence in the field of education: Focusing on blended learning. J Dig Converg. 2020;18(7):429–36.
Liu S, Liu S, Chen H. From blended learning to intelligent blended learning: research trends and future directions. Educ Inf Technol. 2021;26(6):6749–71.
Luckin R, Cukurova M, Kent C, du Boulay B. Empowering educators to be AI-ready. Comput Educ Artif Intell. 2022;3: 100076. https://doi.org/10.1016/j.caeai.2022.100076 .
Mhlanga D. Open AI in education, the responsible and ethical use of ChatGPT towards lifelong learning. In: FinTech and artificial intelligence for sustainable development: the role of smart technologies in achieving development goals. Cham: Springer Nature Switzerland; 2023. p. 387–409.
Mokoena KK. A holistic ubuntu artificial intelligence ethics approach in South Africa. Verbum et Ecclesia. 2024;45(1):1–8. https://doi.org/10.4102/ve.v45i1.3100 .
Morgan H. Conducting a qualitative document analysis. Qual Rep. 2022;27(1):64–77. https://doi.org/10.46743/2160-3715/2022.5044 .
Nair RS, Chuan TC. Integrating technology that uses modified SAMR model as a pedagogical framework in evaluating learning performance of undergraduates. Educ Rev USA. 2021;5(10):373–84. https://doi.org/10.26855/er.2021.10.001 .
Nugraha DGAP, Astawa IWP, Ardana IM. Pengaruh model pembelajaran blended learning terhadap pemahaman konsep dan kelancaran prosedur matematis [The influence of the blended learning model on conceptual understanding and the fluency of mathematical procedures]. Jurnal Riset Pendidikan Matematika. 2019;6(1):75–86. https://doi.org/10.21831/jrpm.v6i.20074 .
Pakpahan R. Analisa pengaruh implementasi artificial intelligence dalam kehidupan manusia [Analysis of the impact of the implementation of artificial intelligence in human life]. JISICOM J Inf Syst Inf Comput. 2021;5(2):506–13. https://doi.org/10.52362/jisicom.v5i2.616 .
Petrova M. Blended learning applied to the artificial intelligence training. In: EDULEARN19 proceedings. 2019. IATED. p. 9207–9213. https://doi.org/10.21125/edulearn.2019.2285
Puentedura RR. SAMR and TPCK: A hands-on approach to classroom practice. Hipassus; 2014. http://www.hippasus.com/rrpweblog/archives/2012/09/03/BuildingUponSAMR.pdf
Qiu C, Shukor SS, Singh CKS, Wang G, Zhong X, Tian Y. A systematic review on the effectiveness of blended learning on learners’ EFL vocabulary performance. Pegem J Educ Instr. 2022;12(4):204–19. https://doi.org/10.47750/pegegog.12.04.21 .
Racine M, Moore R. Artificial intelligence and education through a sustainable development lens: implications for applying data science and technology to transform learning. J Educ Sustain Dev. 2020;14(1):5–20.
Rahman A, Santosa TA, Ilwandri I, Suharyat Y, Aprilisia S, Suhaimi S. The effectiveness of AI-based blended learning on student scientific literacy: meta-analysis. LITERACY Int Sci J Soc Educ Hum. 2023;2(1):141–50. https://doi.org/10.56910/literacy.v2i1.542 .
Sanders DA, Mukhari SS. The perceptions of lecturers about blended learning at a particular higher institution in South Africa. Educ Inf Technol. 2023;29(1):11517–32. https://doi.org/10.1007/s10639-023-12302-6 .
Santosa TA, Razak A, Anhar A, Sumarmin R. The effectiveness of the blended learning model on student learning outcomes in Zoology subjects in the Covid-19 era. Pendidikan Biologi. 2021;7(1):77–83. https://doi.org/10.22437/bio.v7i01.11708 .
Viktorivna L, Oleksandrovych A, Oleksandrivna I, Oleksandrivna N. Artificial intelligence in language learning: What are we afraid of? Arab World Engl J. 2022;8:262–73. https://doi.org/10.24093/awej/call8.18 .
Weber E, Büttgen M, Bartsch S. How to take employees on the digital transformation journey: an experimental study on complementary leadership behaviors in managing organizational change. J Bus Res. 2022;143:225–38. https://doi.org/10.1016/j.jbusres.2022.01.036 .
Westman S, Kauttonen J, Klemetti A, Korhonen N, Manninen M, Mononen A, Paananen H. Artificial intelligence for career guidance—current requirements and prospects for the future. IAFOR J Educ. 2021;9(4):43–62.
Wittmann GE, Olivier J. Blended learning as an approach to foster self-directed learning in teacher professional development programmes. Independent J Teach Learn. 2021;16(2):71–84.
Zawacki-Richter O, Marín VI, Bond M, Gouverneur F. Systematic review of research on artificial intelligence applications in higher education–where are the educators? Int J Educ Technol High Educ. 2019;16(1):1–27. https://doi.org/10.1186/s41239-019-0171-0 .
Download references
Acknowledgements
The authors acknowledge the cooperation of the lecturers who participated in the data-collection process, and the HEI under study, for allowing the research to be conducted.
Author information
Authors and affiliations.
Department of Language Education, Arts and Culture, University of South Africa, Pretoria, South Africa
Debbie A. Sanders & Shirley S. Mukhari
Johannesburg, 2192, South Africa
Debbie A. Sanders
Pretoria, 0122, South Africa
Shirley S. Mukhari
You can also search for this author in PubMed Google Scholar
Contributions
Authors both reviewed the manuscript and checked the references. Author A was responsible for the introduction and literature reviewer. Both authors were responsible for the data collection. Author B analysed the data and drafted the results section and then both authors together discussed the results and decided on the conclusion.
Corresponding author
Correspondence to Debbie A. Sanders .
Ethics declarations
Consent for publication.
Informed consent was obtained from all participants in this study.
Competing interests
The authors declare no competing interests.
Additional information
Publisher's note.
Springer Nature remains neutral with regard to jurisdictional claims in published maps and institutional affiliations.
1.1 Appendix A: Interview question
The following is the set of open-ended interview questions the researchers used by the researchers to assess the lecturer’s view of the impact of AI on blended learning:
Can you describe your experience incorporating AI technologies into your blended learning lessons?
What specific AI technologies or tools have you used in your blended learning approach?
Can you share examples of instances where AI enhanced the effectiveness of your blended learning lessons?
In your opinion, what are the key advantages of integrating AI into blended learning?
What challenges have you encountered when incorporating AI into blended learning, and how did you overcome them?
Have you received any feedback from students regarding their experiences with AI-infused blended learning?
Have you noticed any differences in student performance or understanding between traditional and AI-infused blended learning?
What kind of training or professional development opportunities do you believe are necessary for lecturers to effectively integrate AI into their blended teaching methods?
In your experience, what support or resources do lecturers currently require when implementing AI in blended learning?
1.2 Appendix B: document analysis guide
The researchers used the following guidelines when analysing the module contents, lessons and assessments:
Assess whether the content and learning objectives of the module feature the integration of AI technologies ─ look for objectives that explicitly mention the use of AI to enhance specific skills or competencies.
Identify specific occurrences where AI enhances interactivity within lessons.
Look for evidence that assessments capture the unique contributions of AI to student learning outcomes.
Search for features that assist in the immediacy and effectiveness of feedback mechanisms through AI.
Rights and permissions
Open Access This article is licensed under a Creative Commons Attribution-NonCommercial-NoDerivatives 4.0 International License, which permits any non-commercial use, sharing, distribution and reproduction in any medium or format, as long as you give appropriate credit to the original author(s) and the source, provide a link to the Creative Commons licence, and indicate if you modified the licensed material. You do not have permission under this licence to share adapted material derived from this article or parts of it. The images or other third party material in this article are included in the article’s Creative Commons licence, unless indicated otherwise in a credit line to the material. If material is not included in the article’s Creative Commons licence and your intended use is not permitted by statutory regulation or exceeds the permitted use, you will need to obtain permission directly from the copyright holder. To view a copy of this licence, visit http://creativecommons.org/licenses/by-nc-nd/4.0/ .
Reprints and permissions
About this article
Sanders, D.A., Mukhari, S.S. Lecturers’ perceptions of the influence of AI on a blended learning approach in a South African higher education institution. Discov Educ 3 , 135 (2024). https://doi.org/10.1007/s44217-024-00235-2
Download citation
Received : 08 May 2024
Accepted : 21 August 2024
Published : 02 September 2024
DOI : https://doi.org/10.1007/s44217-024-00235-2
Share this article
Anyone you share the following link with will be able to read this content:
Sorry, a shareable link is not currently available for this article.
Provided by the Springer Nature SharedIt content-sharing initiative
- Artificial Intelligence (AI)
- Blended learning
- Face-to-face learning
- Online learning
- Substitution, Augmentation, Modification, and Redefinition (SAMR) theoretical model
Advertisement
- Find a journal
- Publish with us
- Track your research

IMAGES
VIDEO
COMMENTS
Qualitative research is a method of inquiry used in various disciplines, including social sciences, education, and health, to explore and understand human behavior, experiences, and social phenomena. It focuses on collecting non-numerical data, such as words, images, or objects, to gain in-depth insights into people's thoughts, feelings, motivations, and perspectives.
Qualitative research is used to understand how people experience the world. While there are many approaches to qualitative research, they tend to be flexible and focus on retaining rich meaning when interpreting data. Common approaches include grounded theory, ethnography, action research, phenomenological research, and narrative research.
Abstract. This guide explains the focus, rigor, and relevance of qualitative research, highlighting its role in dissecting complex social phenomena and providing in-depth, human-centered insights. The guide also examines the rationale for employing qualitative methods, underscoring their critical importance. An exploration of the methodology ...
Qualitative Research. Qualitative research is a type of research methodology that focuses on exploring and understanding people's beliefs, attitudes, behaviors, and experiences through the collection and analysis of non-numerical data. It seeks to answer research questions through the examination of subjective data, such as interviews, focus ...
Philosophical underpinnings to qualitative research. Qualitative research uses an inductive approach and stems from interpretivism or constructivism and assumes that realities are multiple, socially constructed, and holistic. 10 According to this philosophical viewpoint, humans build reality through their interactions with the world around them. 10 As a result, qualitative research aims to ...
Qualitative research methods are widely used in the social sciences and the humanities, but they can also complement quantitative approaches used in clinical research. In this article, we discuss the key features and contributions of qualitative research methods. Keywords: Qualitative research, social sciences, sociology.
Characteristics of Qualitative Research. Qualitative research is distinguished from the quantitative or mixed . methods approaches by a grounding in the social constructionist world - view described previously. Scholars identify a set of characteristics that reflect the qualitative approach, as listed below (Creswell & Poth, 2018;
Qualitative research is the naturalistic study of social meanings and processes, using interviews, observations, and the analysis of texts and images. In contrast to quantitative researchers, whose statistical methods enable broad generalizations about populations (for example, comparisons of the percentages of U.S. demographic groups who vote in particular ways), qualitative researchers use ...
While many books and articles guide various qualitative research methods and analyses, there is currently no concise resource that explains and differentiates among the most common qualitative approaches. We believe novice qualitative researchers, students planning the design of a qualitative study or taking an introductory qualitative research course, and faculty teaching such courses can ...
Qualitative research methods. Each of the research approaches involve using one or more data collection methods.These are some of the most common qualitative methods: Observations: recording what you have seen, heard, or encountered in detailed field notes. Interviews: personally asking people questions in one-on-one conversations. Focus groups: asking questions and generating discussion among ...
Qualitative research is an umbrella term that is typically used in contrast to quantitative research and captures research approaches that predominantly rely on collecting and analyzing qualitative data (i.e., data in the form of words, still or moving images, and artifacts).
Qualitative research Footnote 1 —research that primarily or exclusively uses non-numerical data—is one of the most commonly used types of research and methodology in the social sciences. Unfortunately, qualitative research is commonly misunderstood. It is often considered "easy to do" (thus anyone can do it with no training), an "anything goes approach" (lacks rigor, validity and ...
Qualitative research is defined as an exploratory method that aims to understand complex phenomena, often within their natural settings, by examining subjective experiences, beliefs, attitudes, and behaviors. Unlike quantitative research, which focuses on numerical measurements and statistical analysis, qualitative research employs a range of ...
Key features of qualitative research methods. One of the most important contributions of qualitative research methods is that they provide rigorous, theoretically sound, and rational techniques for the analysis of subjective, nebulous, and difficult-to-pin-down phenomena. We are aware, for example, of the role that social factors play in health ...
An approach to research that is "multimethod in focus, involving an interpretative, naturalistic approach to its subject matter. This means that qualitative researchers study things in their natural settings, attempting to make sense of, or interpret, phenomena in terms of the meanings people bring to them.
Qualitative research is a type of research that explores and provides deeper insights into real-world problems.[1] Instead of collecting numerical data points or intervening or introducing treatments just like in quantitative research, qualitative research helps generate hypothenar to further investigate and understand quantitative data. Qualitative research gathers participants' experiences ...
qualitative methods to address questions about people's ways of organizing, relating to, and interacting with the world. Despite the interdisciplinary recognition of the value of "qualitative research" (or perhaps because of it), qualitative research is not a unified field of theory and practice. On the contrary, a plethora of viewpoints ...
Theory and Methodology. Good research follows from a reasonable starting point, a theoretical concept or perspective. Quantitative research uses a positivist perspective in which evidence is objectively and systematically obtained to prove a causal model or hypothesis; what works is the focus. 3 Alternatively, qualitative approaches focus on how and why something works, to build understanding ...
A fourth issue is that the "implicit use of methods in qualitative research makes the field far less standardized than the quantitative paradigm" ... But even in this instance we would make a weak case arguing that these are strictly the defining features of qualitative research. Some researchers seem to focus on the approach or the methods ...
In this Rip Out, we describe 3 different qualitative research approaches commonly used in medical education: grounded theory, ethnography, and phenomenology. Each acts as a pivotal frame that shapes the research question (s), the method (s) of data collection, and how data are analyzed. 4, 5. Go to:
Fundamental Criteria: General Research Quality. Various researchers have put forward criteria for evaluating qualitative research, which have been summarized in Table 3.Also, the criteria outlined in Table 4 effectively deliver the various approaches to evaluate and assess the quality of qualitative work. The entries in Table 4 are based on Tracy's "Eight big‐tent criteria for excellent ...
Types of qualitative research 3,4. The data collection methods in qualitative research are designed to assess and understand the perceptions, motivations, and feelings of the respondents about the subject being studied. The different qualitative research types include the following: . In-depth or one-on-one interviews: This is one of the most common qualitative research methods and helps the ...
The final section of the paper advocates a "pragmatic approach" as a new guiding paradigm in social science research methods, both as a basis for supporting work that combines qualitative and ...
A fourth issue is that the "implicit use of methods in qualitative research makes the field far less standardized than the quantitative paradigm" ... But even in this instance we would make a weak case arguing that these are strictly the defining features of qualitative research. Some researchers seem to focus on the approach or the methods ...
Abstract. This paper aims to provide an overview of the use and assessment of qualitative research methods in the health sciences. Qualitative research can be defined as the study of the nature of phenomena and is especially appropriate for answering questions of why something is (not) observed, assessing complex multi-component interventions ...
Qualitative research methods are used to address research questions that require description and understanding of social phenomena and their contexts. So, it is the nature of the required information that will lead to a choice of a qualitative approach. ... Four specific features of qualitative research - understanding research participants ...
Atlas.ti is a powerful platform built for detailed qualitative and mixed-methods research, offering a lot of capabilities for running both quantitative and qualitative research. ... NVivo is another powerful platform for qualitative and mixed-methods research. It's analysis features include: Data Import and Organization: Easily manage ...
Our study employed multidimensional methods such as co-occurrence analysis, clustering, and burst analysis to: (1) reveal research dynamics, key journals, and domains in this field; (2) identify ...
This article used a qualitative research approach and comparative and critical research methods. Secondary sources of data were used to compare Medemer with various political ideologies. ... From an ideological point of view, Medemer satisfies the three features of ideology (critics of the present order, vision of the future society, and theory ...
4.1 Research approach. This study applied a qualitative methodology to investigate lecturers' views on the influence AI has on blended learning. A qualitative approach involves a thorough exploration and grasp of phenomena, using non-numerical data and highlighting context, meanings, and subjective experiences . The researchers deemed this ...