Use AI to summarize scientific articles and research papers in seconds
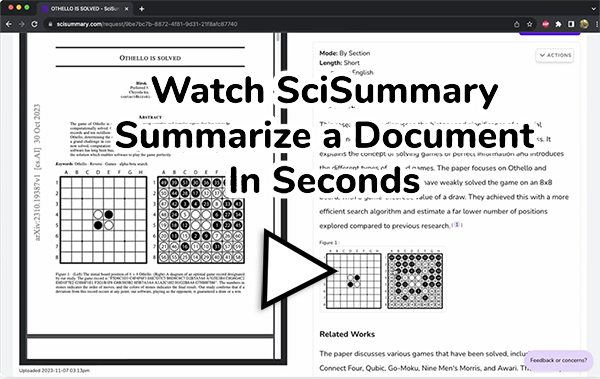

Send a document, get a summary. It's that easy.
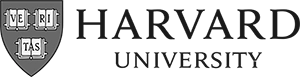
If GPT had a PhD
- Unlimited Summaries
- Summarize articles up to 200,000 words.
- 5 Figure and table analysis with AI
- Unlimited Chat Messages
- Unlimited article searches
- Import and summarize references with the click of a button
- 30,000 words summarized
- 5 Figures or Tables analyzed with AI
- 100 Chat Messages
- Maximum document length of 200,000 words
- Unlimited bulk summaries
- Unlimited chat messages per month
- Unlimited figure and table analysis with AI
- 1,000 documents indexed for semantic search
Extract key information from research papers with our AI summarizer.
Get a snapshot of what matters – fast . Break down complex concepts into easy-to-read sections. Skim or dive deep with a clean reading experience.

Summarize, analyze, and organize your research in one place.
Features built for scholars like you, trusted by researchers and students around the world.
Summarize papers, PDFs, book chapters, online articles and more.
Easy import
Drag and drop files, enter the url of a page, paste a block of text, or use our browser extension.
Enhanced summary
Change the summary to suit your reading style. Choose from a bulleted list, one-liner and more.
Read the key points of a paper in seconds with confidence that everything you read comes from the original text.
Clean reading
Clutter free flashcards help you skim or diver deeper into the details and quickly jump between sections.
Highlighted key terms and findings. Let evidence-based statements guide you through the full text with confidence.
Summarize texts in any format
Scholarcy’s ai summarization tool is designed to generate accurate, reliable article summaries..
Our summarizer tool is trained to identify key terms, claims, and findings in academic papers. These insights are turned into digestible Summary Flashcards.
Scroll in the box below to see the magic ⤸

The knowledge extraction and summarization methods we use focus on accuracy. This ensures what you read is factually correct, and can always be traced back to the original source .
What students say
It would normally take me 15mins – 1 hour to skim read the article but with Scholarcy I can do that in 5 minutes.
Scholarcy makes my life easier because it pulls out important information in the summary flashcard.
Scholarcy is clear and easy to navigate. It helps speed up the process of reading and understating papers.
Join over 400,000 people already saving time.
From a to z with scholarcy, generate flashcard summaries. discover more aha moments. get to point quicker..

Understand complex research. Jump between key concepts and sections. Highlight text. Take notes.
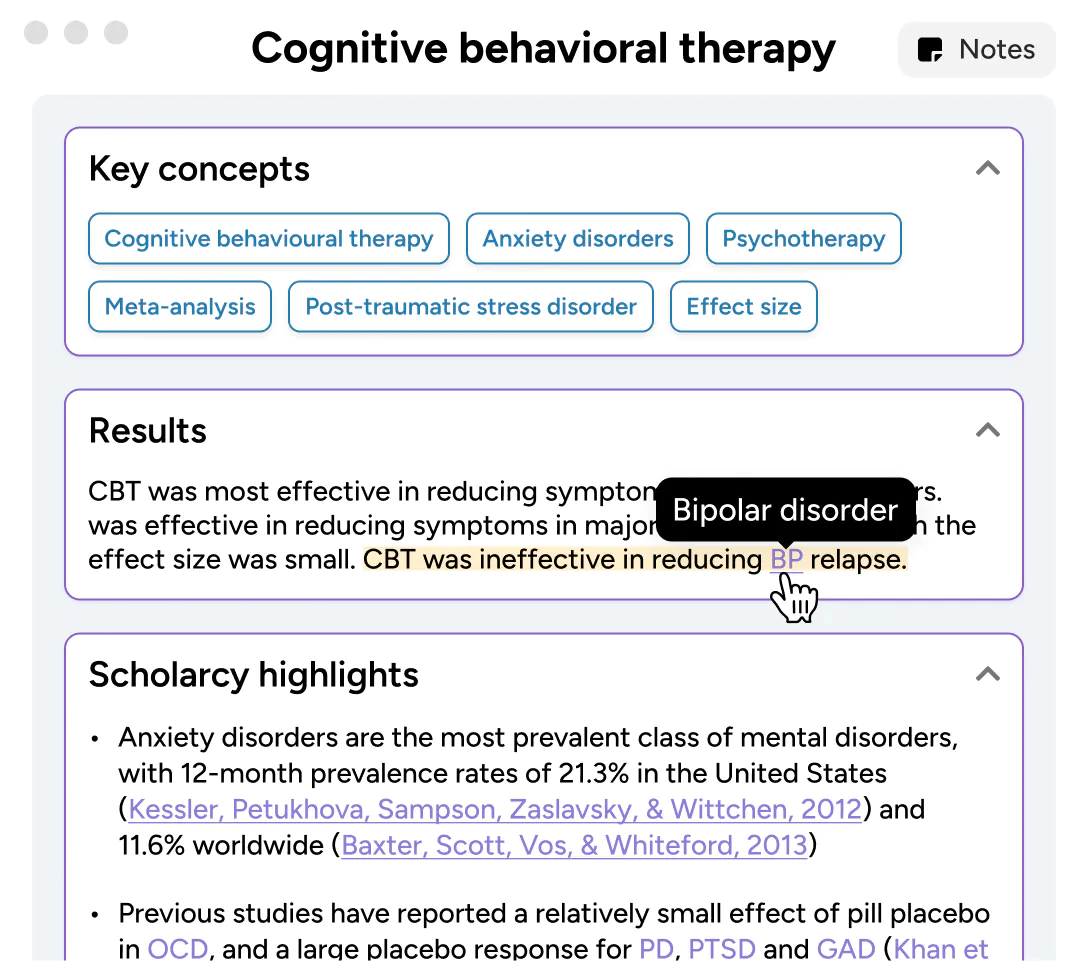
Build a library of knowledge. Recall important info with ease. Organize, search, sort, edit.

Bring it all together. Export Flashcards in a range of formats. Transfer Flashcards into other apps.

Apply what you’ve learned. Compile your highlights, notes, references. Write that magnum opus 🤌
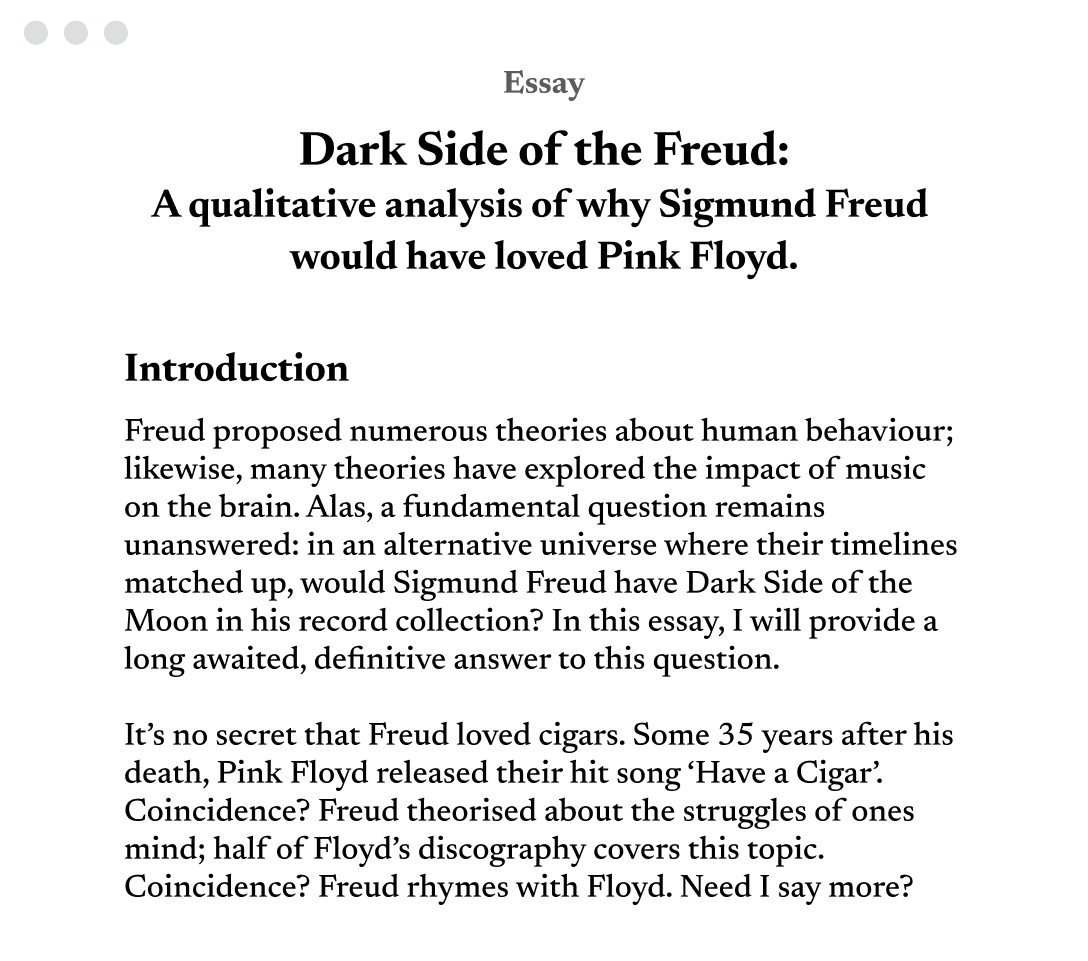
Go beyond summaries
Get unlimited summaries, advanced research and analysis features, and your own personalised collection with Scholarcy Library!

With Scholarcy Library you can import unlimited documents and generate summaries for all your course materials or collection of research papers.
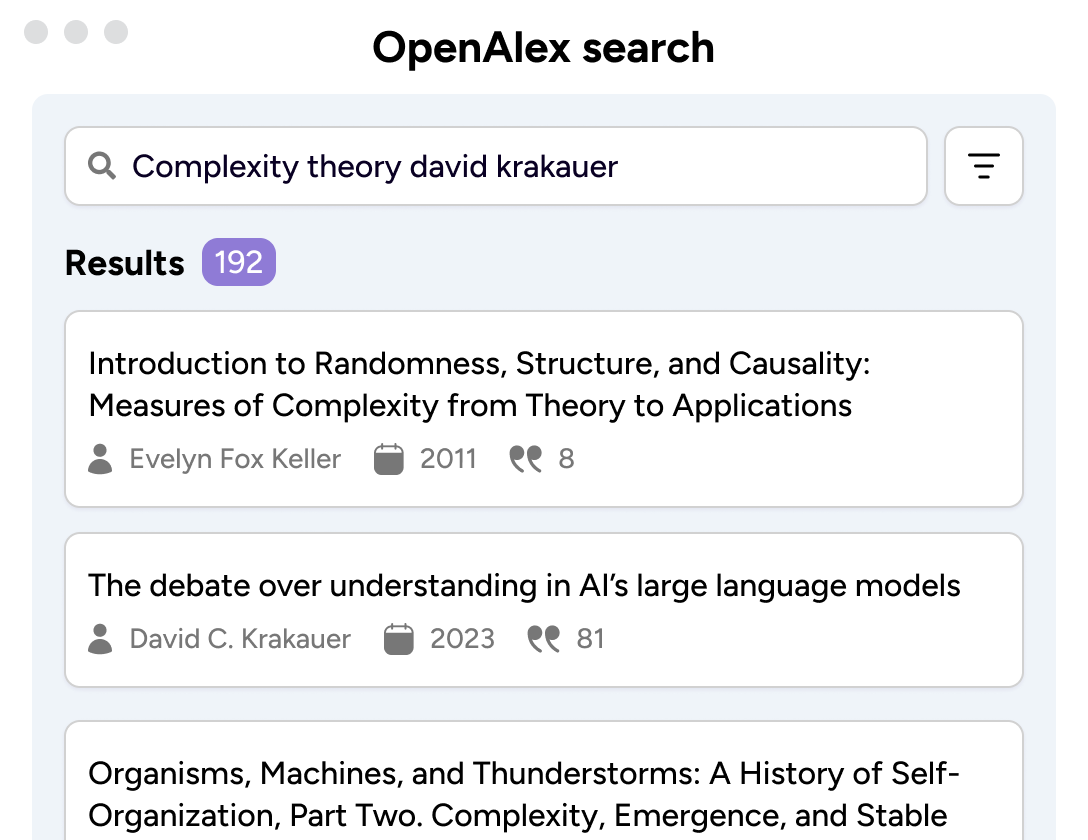
Scholarcy Library offers additional features including access to millions of academic research papers, customizable summaries, direct import from Zotero and more.

Scholarcy lets you build and organise your summaries into a handy library that you can access from anywhere. Export from a range of options, including one-click bibliographies and even a literature matrix.
Compare plans
Summarize 3 articles a day with our free summarizer tool, or upgrade to Scholarcy Library to generate and save unlimited article summaries.
Import a range of file formats
Export flashcards (one at a time)
Everything in Free
Unlimited summarization
Generate enhanced summaries
Save your flashcards
Take notes, highlight and edit text
Organize flashcards into collections
Frequently Asked Questions
How do i use scholarcy, what if i’m having issues importing files, can scholarcy generate a plain language summary of the article, can scholarcy process any size document, how do i change the summary to get better results, what if i upload a paywalled article to scholarcy, is it violating copyright laws.
Free Text Summarizer
Try our other writing services

Want to be 100% sure your summary is plagiarism-free?
Make your life easier with the free summarizer tool.
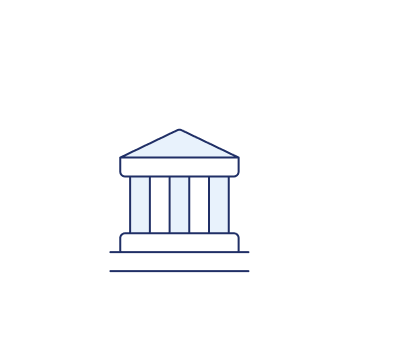
Academic research
Speed up your academic research by extracting key points.
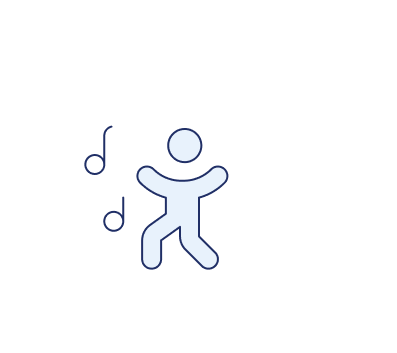
Every day use
Reduce your reading time by summarizing long blocks of text within seconds.
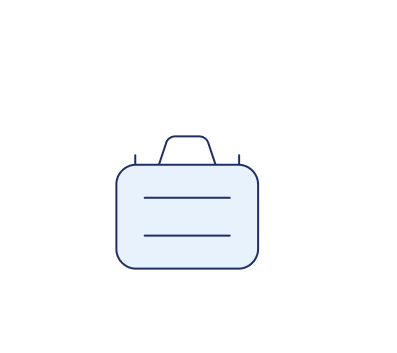
Easily condense transcripts of long meetings into concise bullet points.
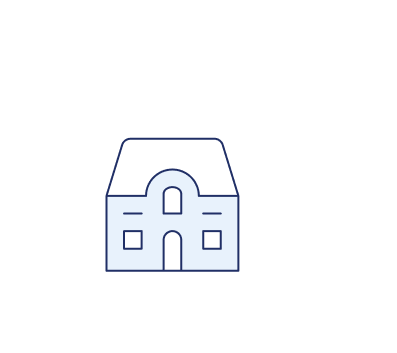
Difficult text
Simplify hard-to-read paragraphs, sentences or complete articles with 1 click.
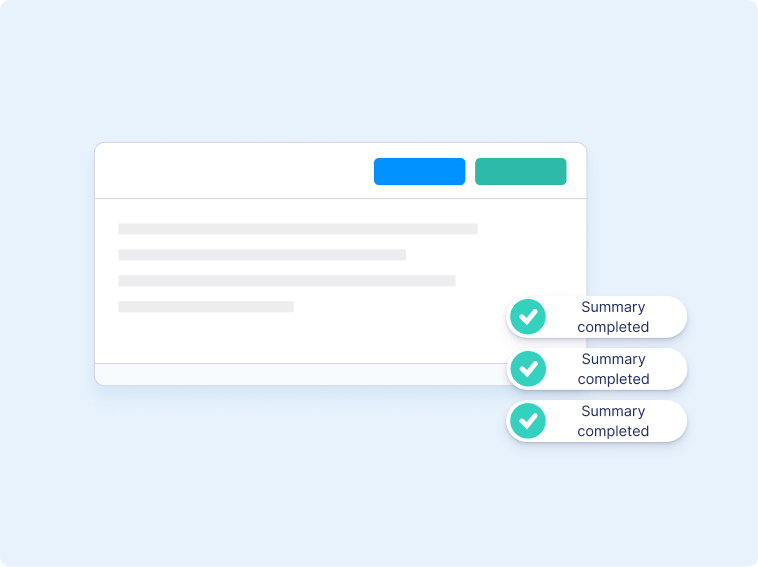
Why use this summarizer?
- 100% free: Generate unlimited summaries without paying a penny
- Accurate: Get a reliable and trustworthy summary of your original text without any errors
- No signup: Use it without giving up any personal data
- Secure: No summary data is stored, guaranteeing your privacy
- Speed: Get an accurate summary within seconds, thanks to AI
- Flexible: Adjust summary length to get more (or less) detailed summaries
How to use this summarizer
1. insert, paste or download your text, 2. pick the way you want to summarize, 3. adjust your summary length, 4. get your summary in seconds.
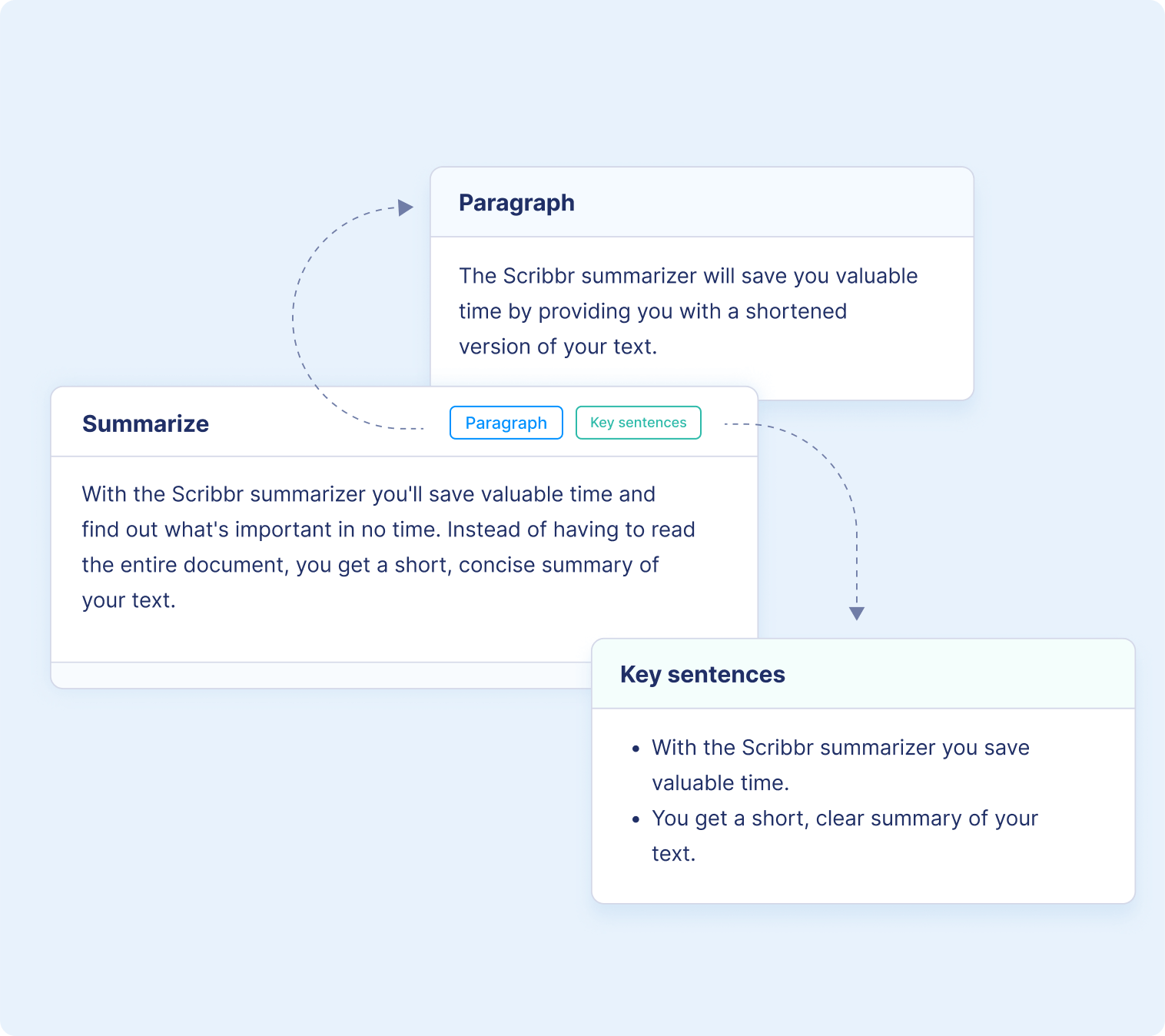
2 ways of summarizing your text
1. key sentences.
Extracts the key points of your text and turns them into digestible bullet points
2. Concise paragraphs
Summarizes your text in a concise paragraph
Summarize your text today
💸 Free | Unlimited text summarization |
---|---|
🤖 AI-powered | Extract key points in seconds |
🎯 Accurate | Ideal for academic research |
🗎 Summarize any text | Articles, paragraphs & essays |
Want to make sure your summary doesn’t contain any plagiarism?
Scribbr & academic integrity.
Scribbr is committed to protecting academic integrity. Our plagiarism checker , AI Detector , Citation Generator , proofreading services , paraphrasing tool , grammar checker , summarizer, and free Knowledge Base content are designed to help students produce quality academic papers.
We make every effort to prevent our software from being used for fraudulent or manipulative purposes.
Ask our team
Want to contact us directly? No problem. We are always here for you.
- Email [email protected]
- Start live chat
- Call +1 (510) 822-8066
- WhatsApp +31 20 261 6040
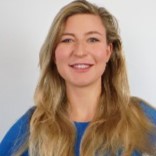
Frequently asked questions
Yes, it can. The AI has been trained on a big dataset, so technical or complex data won’t be a problem for the text summarizer .
The text summarizer is accessible on both desktop and mobile.
This text summarizer can condense long text within seconds.
At the moment, a maximum of 600 words can be summarized at once, within a few seconds. Want to summarize more? Just paste another block of text. There’s no limit on how much text you can summarize with our text summarizer .
The text summarizer can give you a longer or shorter summary, depending on your wishes. Want a more detailed summary? Just adjust the summary length at the top.
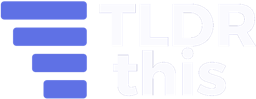
Summarize any | in a click.
TLDR This helps you summarize any piece of text into concise, easy to digest content so you can free yourself from information overload.
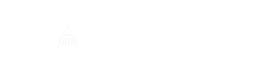
Enter an Article URL or paste your Text
Browser extensions.
Use TLDR This browser extensions to summarize any webpage in a click.
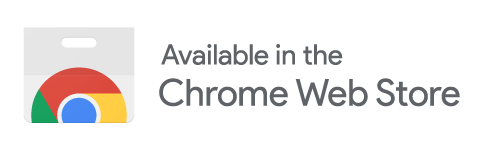
Single platform, endless summaries
Transforming information overload into manageable insights — consistently striving for clarity.
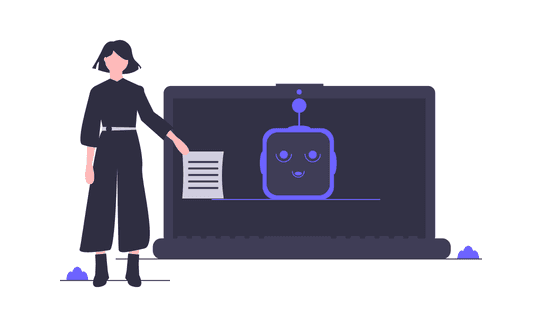
100% Automatic Article Summarization with just a click
In the sheer amount of information that bombards Internet users from all sides, hardly anyone wants to devote their valuable time to reading long texts. TLDR This's clever AI analyzes any piece of text and summarizes it automatically, in a way that makes it easy for you to read, understand and act on.
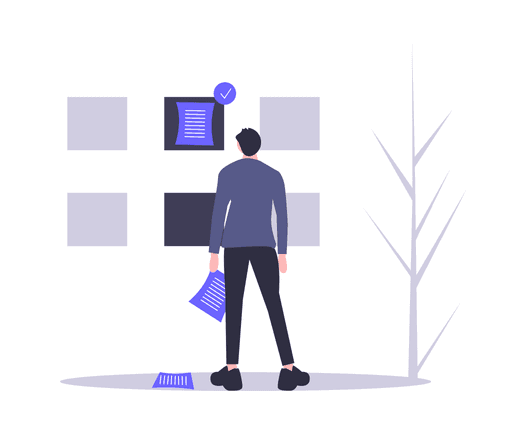
Article Metadata Extraction
TLDR This, the online article summarizer tool, not only condenses lengthy articles into shorter, digestible content, but it also automatically extracts essential metadata such as author and date information, related images, and the title. Additionally, it estimates the reading time for news articles and blog posts, ensuring you have all the necessary information consolidated in one place for efficient reading.
- Automated author-date extraction
- Related images consolidation
- Instant reading time estimation
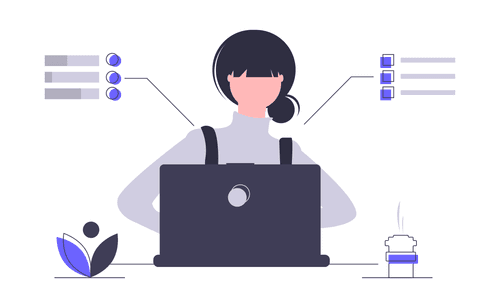
Distraction and ad-free reading
As an efficient article summarizer tool, TLDR This meticulously eliminates ads, popups, graphics, and other online distractions, providing you with a clean, uncluttered reading experience. Moreover, it enhances your focus and comprehension by presenting the essential content in a concise and straightforward manner, thus transforming the way you consume information online.
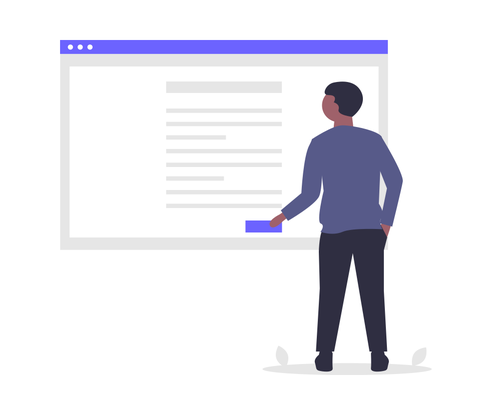
Avoid the Clickbait Trap
TLDR This smartly selects the most relevant points from a text, filtering out weak arguments and baseless speculation. It allows for quick comprehension of the essence, without needing to sift through all paragraphs. By focusing on core substance and disregarding fluff, it enhances efficiency in consuming information, freeing more time for valuable content.
- Filters weak arguments and speculation
- Highlights most relevant points
- Saves time by eliminating fluff
Who is TLDR This for?
TLDR This is a summarizing tool designed for students, writers, teachers, institutions, journalists, and any internet user who needs to quickly understand the essence of lengthy content.
Anyone with access to the Internet
TLDR This is for anyone who just needs to get the gist of a long article. You can read this summary, then go read the original article if you want to.
TLDR This is for students studying for exams, who are overwhelmed by information overload. This tool will help them summarize information into a concise, easy to digest piece of text.
TLDR This is for anyone who writes frequently, and wants to quickly summarize their articles for easier writing and easier reading.
TLDR This is for teachers who want to summarize a long document or chapter for their students.
Institutions
TLDR This is for corporations and institutions who want to condense a piece of content into a summary that is easy to digest for their employees/students.
Journalists
TLDR This is for journalists who need to summarize a long article for their newspaper or magazine.
Featured by the world's best websites
Our platform has been recognized and utilized by top-tier websites across the globe, solidifying our reputation for excellence and reliability in the digital world.
Focus on the Value, Not the Noise.
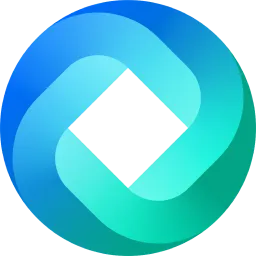
Research Assistant
Ai-powered research summarizer.
- Summarize academic papers: Quickly understand the main points of research papers without reading the entire document.
- Extract insights from reports: Identify key findings and trends from industry reports, surveys, or reviews.
- Prepare for presentations: Create concise summaries of research materials to include in your presentations or talking points.
- Enhance your understanding: Improve your comprehension of complex subjects by summarizing the main ideas and insights.
- Save time: Reduce the time spent on reading lengthy research documents by focusing on the most important points.
New & Trending Tools
Ai sentence rephraser, ai article outliner, ai writing style adapter.

Affiliate 💸
Get started free
Research Paper Sources
15 Best Tools to Summarize Research Paper AI Quickly
Save time to summarize research paper AI with these tools that will make your work more efficient. Find out which ones are worth your time!
Apr 6, 2024
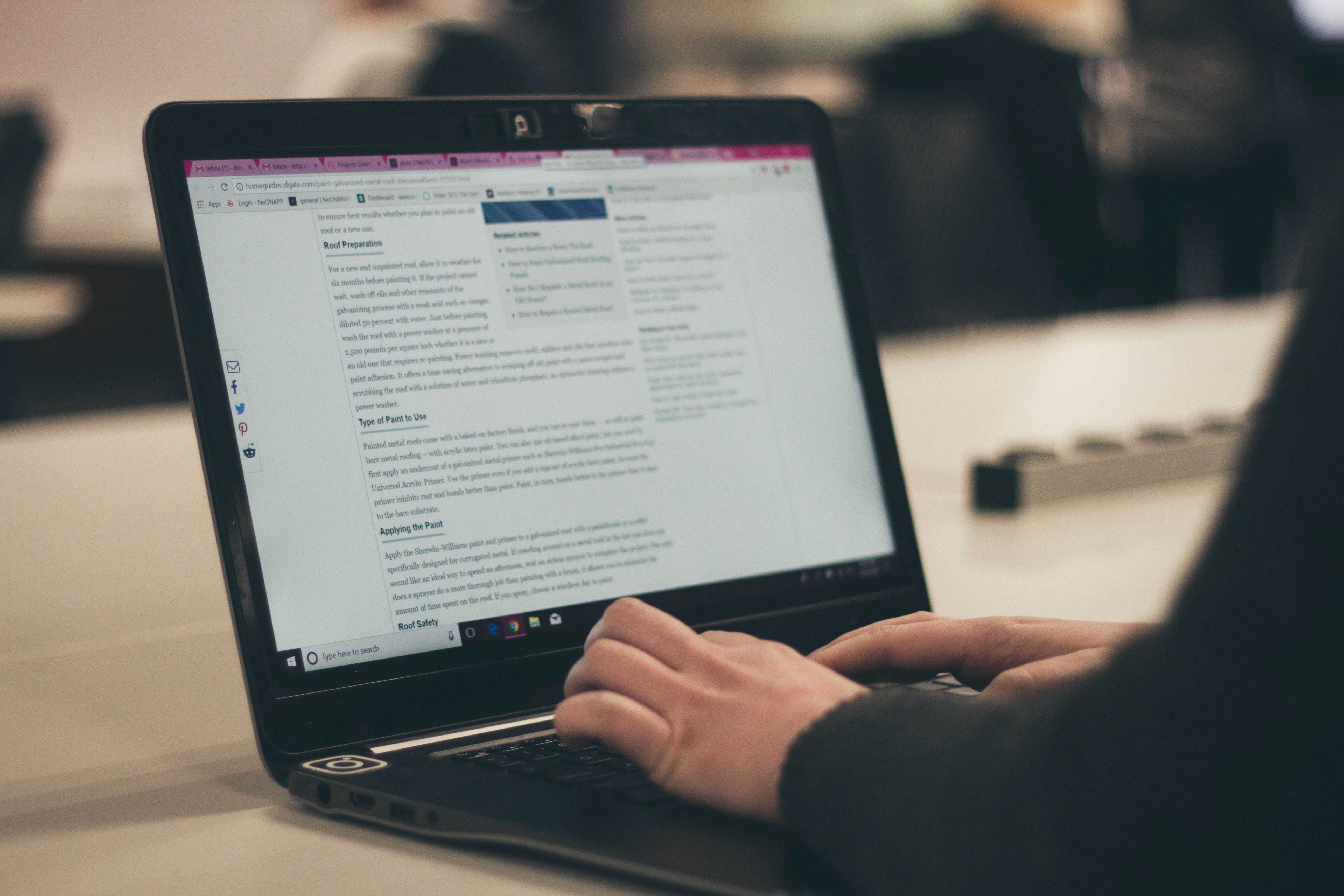
Discover the essence of summarize research paper AI as we delve into the critical aspects of primary vs secondary sources . Unravel the importance of research paper summaries in AI and how they shape the current landscape. Take a deep dive into the value offered by various sources in AI research, and how these summaries help to provide meaning and context to research studies in this field. Explore the significance of summarizing research papers in AI to unlock the potential of your learning and academic endeavors.
Table of Contents
What is a research paper summarizer ai, benefits of summarizing research papers with ai, applications of ai in research paper summarization, summarize research paper ai: 15 best tools for gaining quick insights, what should you consider when choosing ai summarizers, supercharge your researching ability with otio — try otio for free today.

AI or Artificial Intelligence has been making its presence felt across all fields today, transforming how we work and interact with information. Thanks to AI-powered tools, tasks that once required hours of human labor can now be accomplished in a fraction of the time. This technology has not only revolutionized industry practices but has also seeped into the realms of reading and writing, promising to make our lives easier and more efficient. With the rise of AI-powered tools, the way we read and write is evolving. From information retrieval to writing assistance, AI has become a key player in improving productivity and enhancing the quality of our work. Machine learning algorithms are now being used to summarize research papers, articles, and discussions without sacrificing accuracy. This is done by searching for relevant sentences, understanding their context, and then generating a concise version of the content using unique words.
AI-Based Research Paper Summarization
Research paper summarization, a process that was once time-consuming and tedious, has been simplified and improved by AI technologies. AI research paper summarizers are tools that use algorithms to summarize lengthy research papers, articles, or discussions quickly and efficiently. These tools find and extract important information from long-form papers, enabling users to save time and focus only on the core message or data.
AI summarization tools work by scanning the content, identifying relevant sentences, understanding the context of the text, and creating concise summaries using unique vocabulary. By automating the summarization process, these tools can help researchers, students, and knowledge workers extract key insights and information rapidly, freeing up more time for analysis and decision-making.
AI research and writing partner
Knowledge workers, researchers, and students today suffer from content overload and are left to deal with it using fragmented, complex, and manual tooling. Too many of them settle for stitching together complicated bookmarking, read-it-later, and note-taking apps to get through their workflows. Now that anyone can create content with the click of a button - this problem is only going to get worse.
Otio solves this problem by providing one AI-native workspace for researchers. It helps them:
A wide range of data sources, from bookmarks, tweets, and extensive books to YouTube videos.
2. Extract key takeaways
With detailed AI-generated notes and source-grounded Q&A chat.
Draft outputs using the sources you’ve collected. Otio helps you go from reading list to first draft faster.
Along with this, Otio also helps you write research papers/essays faster. Here are our top features that are loved by researchers: AI-generated notes on all bookmarks (Youtube videos, PDFs, articles, etc.), Otio enables you to chat with individual links or entire knowledge bases, just like you chat with ChatGPT, as well as AI-assisted writing.
Let Otio be your AI research and writing partner — try Otio for free today!

Efficiency and Time-Saving
Researchers, professionals, and students can save a significant amount of time by using AI tools for summarizing research papers. These tools can quickly analyze and condense large amounts of information into concise summaries, eliminating the need for manual extraction and synthesis.
Accuracy and Precision
AI-powered summarization tools ensure that the generated summaries are accurate and precise, as they rely on advanced algorithms to analyze and interpret text. By leveraging natural language processing capabilities , AI tools can extract key information effectively, reducing the risk of human error and bias in the summary creation process.
Scalability and Ability to Handle Large Volumes of Data
AI tools for summarizing research papers are highly scalable and can efficiently process and summarize large volumes of data in a short amount of time. This scalability enables researchers to analyze extensive datasets and sources, providing a comprehensive overview of the research landscape quickly and effectively.
Improved Accessibility for Both Academicians and Non-Academicians
AI tools make research summaries more accessible to a wider audience, including academicians and non-academicians. By generating concise and easy-to-understand summaries, AI tools democratize access to research findings, making them more digestible for individuals with varying levels of expertise in the subject matter.
Efficiency and Accessibility with AI Summarization Tools
AI-powered tools for summarizing research papers offer numerous benefits, ranging from efficiency and time-saving to improved accessibility. Researchers, professionals, and students can leverage these tools to streamline their research workflows, enhance the quality of their work, and make research more accessible to a broader audience.
If you're looking to boost your research efficiency and streamline your writing process, consider Otio- your AI research and writing partner . With advanced features like AI-generated notes, source-grounded Q&A chat, and AI-assisted writing, Otio can help you collect, extract key takeaways, and create content faster.
Try Otio for free today!
Related Reading
• How To Read Scientific Papers • How Many Sources Should A Research Paper Have • Sources For Research Paper • Google Scholar Search Tips • How To Read A Research Paper • How To Find Sources For A Research Paper • Research Notes • Literature Synthesis

These tools drastically accelerate the process of reviewing academic research and literature, including articles, books, and other resources. They extract the most critical information, such as key points and findings, and shorten the text without losing the context. This way, academics and students can economize time and extract vital information for their research projects.
Scientific Publications and Journals
AI-based summarization tools are beneficial for professionals who research scientific publications and journals. They help to digest complex scientific content, including medical or technical terms, into a concise summary. Scientists can thus save time and focus on their own research, understanding a broader range of scientific literature.
Industry Reports and Market Analysis
In the professional world, these tools can quickly summarize lengthy industry reports and market analyses. Professionals can gain insights from a range of industry reports to stay up-to-date on the latest trends, forecasts, and expert opinions. This capability can empower professionals in industries like finance, marketing, and management.
Policy Papers and Government Documents
AI summarization tools are valuable for policymakers and government officials. These tools allow them to analyze policy papers and government documents efficiently. By extracting the most salient policy recommendations, officials can make more informed decisions regarding public welfare and policy changes.
Legal Documents and Case Studies
For legal professionals, AI summarization tools offer a significant advantage in summarizing legal documents and case studies. By summarizing legal briefs, case law documents, or complex legal texts, these tools help legal practitioners to quickly grasp the core elements and arguments of the cases they are working on.
Knowledge workers, researchers, and students today suffer from content overload and are left to deal with it using fragmented, complex, and manual tooling. Too many of them settle for stitching together complicated bookmarking, read-it-later, and note-taking apps to get through their workflows. Now that anyone can create content with the click of a button – this problem is only going to get worse.
a wide range of data sources, from bookmarks, tweets, and extensive books to YouTube videos.
with detailed AI-generated notes and source-grounded Q&A chat.
draft outputs using the sources you’ve collected. Otio helps you to go from reading list to first draft faster.
Along with this, Otio also helps you write research papers/essays faster. Here are our top features that are loved by researchers: AI-generated notes on all bookmarks (Youtube videos, PDFs, articles, etc.), Otio enables you to chat with individual links or entire knowledge bases, just like you chat with ChatGPT, as well as AI assisted writing.
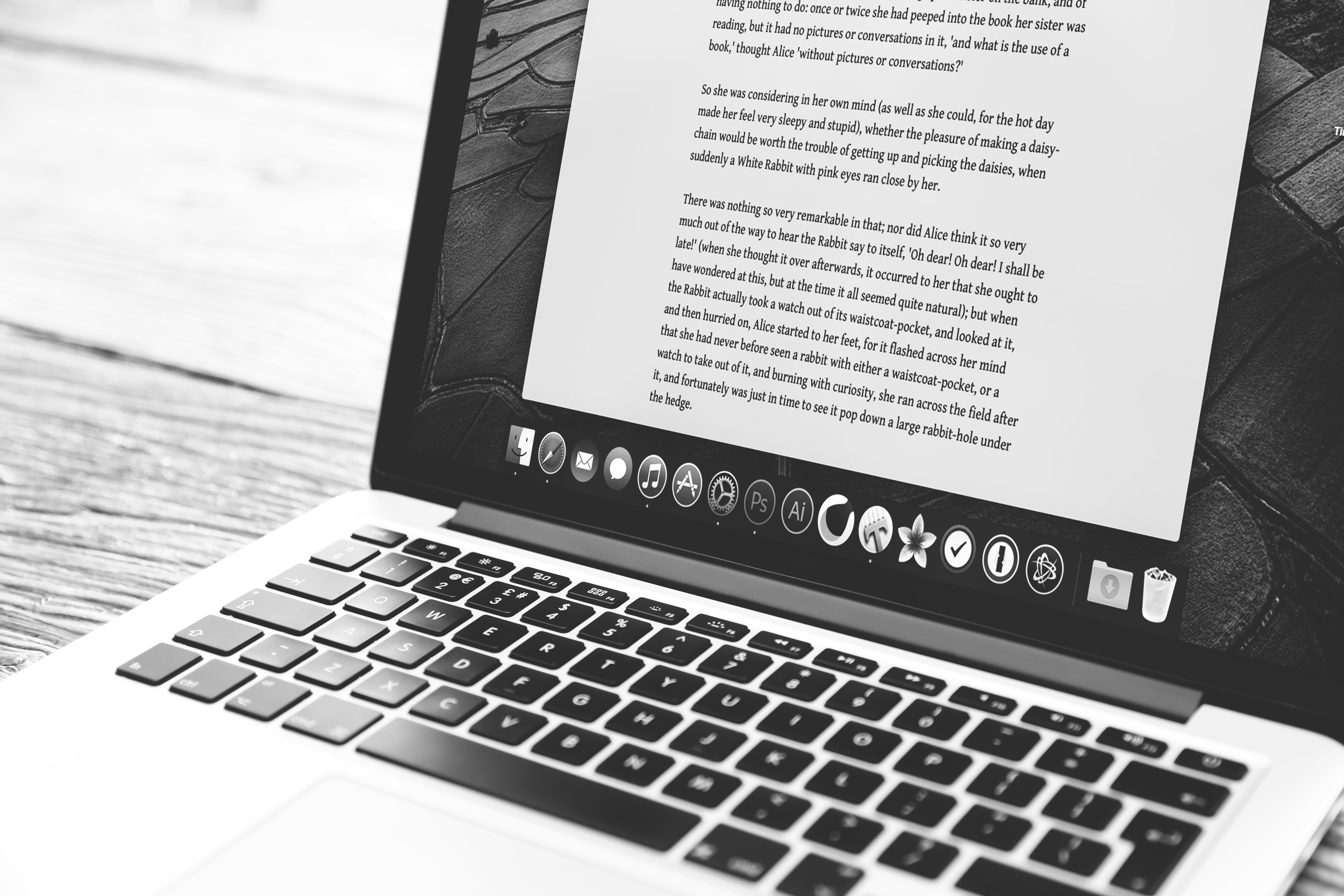
1. Otio: AI Research and Writing Partner
Otio provides an AI-native workspace that assists knowledge workers, researchers, and students in managing content overload. Users can collect data from various sources, extract key takeaways, and create draft outputs using the sources they've collected.
Otio allows for faster reading list to first draft transitions and facilitates the writing of research papers and essays. The tool offers AI-generated notes on all bookmarks, supports chatting with individual links or entire knowledge bases, and provides AI-assisted writing features.
2. QuillBot: Precision and Efficiency
QuillBot uses advanced neural network models to summarize research papers with accuracy and effectiveness. The tool leverages cutting-edge technology to condense lengthy papers into concise and informative summaries, making it easier for users to navigate vast amounts of literature.
3. Scribbr: AI-Powered Academic Writing Assistant
Scribbr is an AI-driven academic writing assistant that includes a summarization feature tailor-made for research papers. The tool assists users in the research paper writing process by summarizing and condensing information from various sources, offering support in structuring and organizing content effectively.
4. AI Summarizer: Automation for Summarizing Research Papers
AI Summarizer harnesses artificial intelligence to automatically summarize research papers and other text documents. The tool streamlines the summarization process, making it efficient and accurate, enabling users to extract key information from extensive research papers with ease.
5. TLDR: Automatic Text Summarization
TLDR is a free online text summarizing tool that uses AI to condense lengthy articles, papers, and essays into key summary paragraphs. The tool assists users in extracting core information from extensive texts, saving time and effort in digesting complex research papers.
6. Jasper AI: Rapid Generation of Paper Summaries
Jasper AI is a robust summarizing tool that helps users generate AI-powered paper summaries quickly and effectively. The tool supports the creation of premium-quality summaries in a timely manner, assisting researchers in distilling complex information into concise and informative outputs.
7. RefME: AI Algorithms for Summarizing Research Papers and Citations
RefME utilizes AI algorithms to summarize research papers and accurately generate citations , providing a time-saving solution for researchers. The tool automates the summarization process, making it efficient and accurate, enhancing research productivity and information extraction.
8. Paper Digest: Enhancing Research Efficiency
Paper Digest leverages AI technology to summarize research papers into brief and informative summaries. The tool assists researchers in efficiently navigating extensive literature by providing succinct and condensed summaries, making it easier to extract key information from scholarly articles.
9. SMMRY: AI-Driven Summarization
SMMRY employs AI-driven algorithms to generate summaries of research papers, offering key insights in a condensed format. The tool streamlines the summarization process, extracting critical information from lengthy research papers and providing users with concise and informative summaries.
10. ReadCube Papers: AI-Powered Summarization Features
ReadCube Papers offers AI-powered summarization features for research papers, aiding researchers in effectively managing and extracting information from vast amounts of literature. The tool automates the summarization process, making it efficient and accurate, enabling users to generate concise summaries of research papers quickly.
11. Genei.io: Efficient Academic Paper Exploration
Genei.io is an AI-powered summarization and research tool that helps users find and explore academic papers efficiently. The tool supports the generation of effective summaries, assisting researchers in extracting key information from lengthy academic papers and reports with ease.
12. Semantic Scholar: Detailed Information Extraction
Semantic Scholar is an AI-powered tool that provides detailed information in context, highlights key points, and offers super-short summaries of scientific papers. The tool assists users in extracting critical information from research papers, making it easier to navigate complex scientific literature.
13. Tenorshare AI - PDF Tool: AI Summarization for PDF Documents
Tenorshare AI - PDF Tool offers powerful AI summarization features for optimizing interactions with PDF documents. The innovative software assistant condenses lengthy PDF documents into readable summaries within seconds, assisting users in extracting key information efficiently and quickly.
14. Scholarcy: Rapid Information Parsing from Academic Papers
Scholarcy employs artificial intelligence to help researchers quickly parse key information from lengthy academic papers and reports. The tool supports the efficient extraction of critical data, enabling users to generate informative summaries of research papers in a timely manner.
15. ChatGPT: Speedy Summarization Tool
ChatGPT is a fast tool designed to summarize research papers quickly and effectively. The tool assists users in identifying core points within research papers in a short amount of time, supporting millions of people worldwide in writing literature reviews efficiently. Let Otio be your AI research and writing partner — try Otio for free today!
• Best Databases For Research • Literature Search • How To Summarize A Research Article • How To Tell If An Article Is Peer Reviewed • Reliable Sources For Research • Using Ai For Research • How To Use Chat Gpt For Research • How To Search For Research Articles
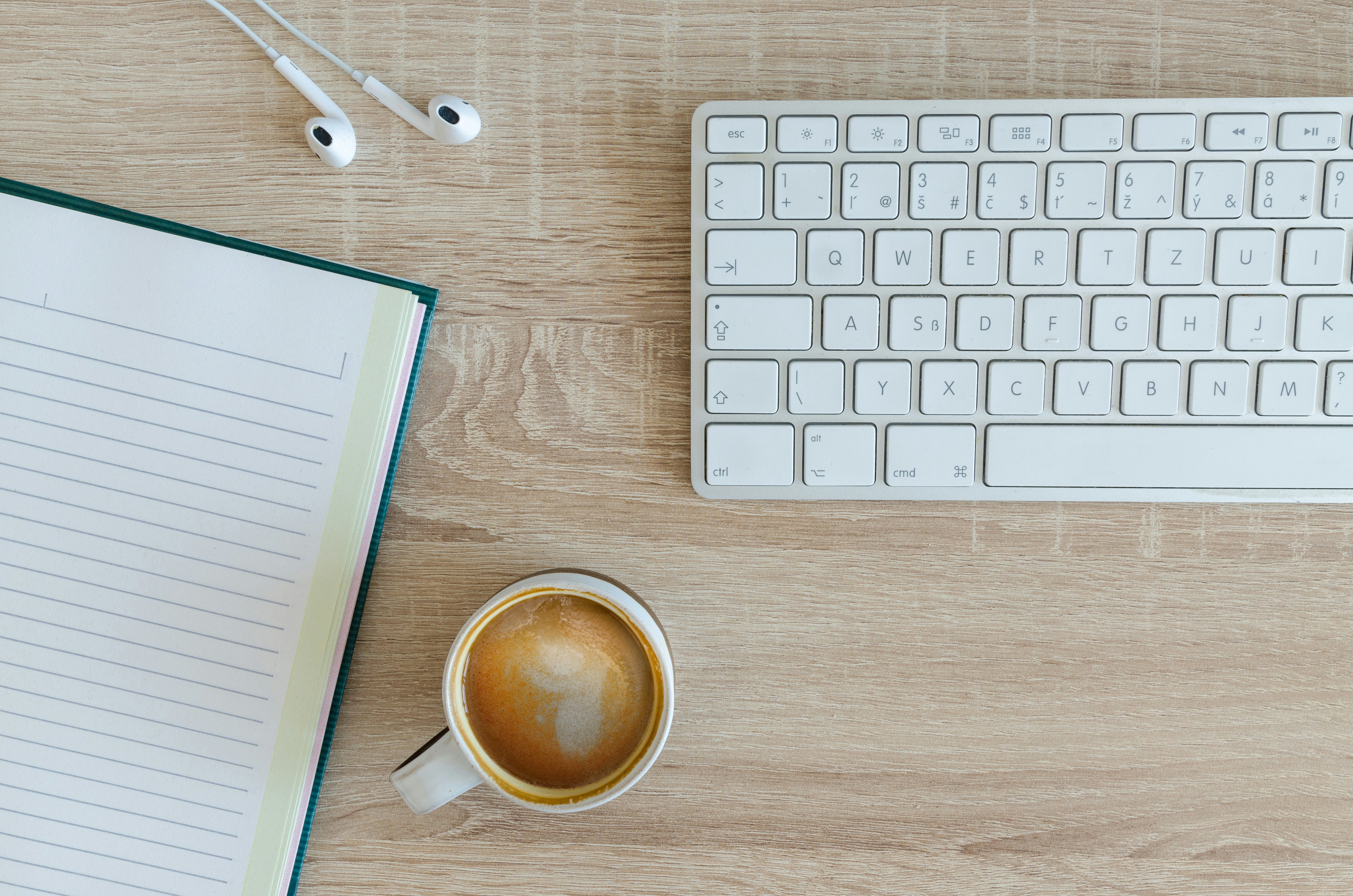
File Compatibility
When selecting an AI summarization tool, it is crucial to consider its file compatibility. Not all tools accept pasted text alone; some advanced options can summarize audio, video, files, and documents.
If your task involves summarizing multimedia content, it is essential to ensure that the tool supports those specific formats. This ability to accept various types of content contributes significantly to the efficiency and versatility of the tool. Ensuring that the tool aligns with your specific content needs will ensure a seamless and effective summarization process.
The cost of an AI summarization tool is another important factor to consider. Depending on your requirements, you may not need a tool that requires a monthly subscription, especially if you have a one-time summarization task. Opting for an affordable solution will help you avoid unnecessary expenses.
There are free tools available such as Otio, which can assist with summarization tasks without the need for a subscription. By choosing a tool that suits your budget and specific needs, you can ensure an efficient summarization process without overspending.
Exportability
Exportability is a critical feature to consider when choosing an AI summarization tool. It is essential to determine if you can export the summary into a shareable format such as PDF. If sharing the summarized content is a requirement for your task, it is important to verify this capability early on.
The ability to export summaries into different formats enhances the usefulness and applicability of the tool. Having the option to share summaries in various formats allows for seamless integration with other platforms and facilitates easy sharing of information.
The accuracy of the summaries generated by AI tools is a fundamental consideration when choosing a summarization tool. Summaries should accurately represent the source material without misconstruing the information. It is crucial to assess how accurately and responsibly different tools summarize content before making a decision.
By selecting a tool that consistently produces accurate and reliable summaries, you can ensure that the information provided is precise and aligned with the original content. This accuracy is essential for tasks that require precise and reliable summarization of information.
Otio is a groundbreaking AI-native workspace designed to assist knowledge workers, researchers, and students in managing content overload in a seamless and efficient manner. In today's digital age, with the abundance of information available, it's easy to become overwhelmed with fragmented and complex tools. Otio solves this problem by providing a single platform where users can collect data from various sources like bookmarks, tweets, books, YouTube videos, and more.
Efficient Research Extraction
The key feature of Otio is its ability to generate detailed AI-driven notes and provide source-grounded Q&A chats. These features allow users to quickly extract key takeaways from their collected sources. Otio also streamlines the writing process by enabling users to create drafts directly from their reading lists. Researchers can transition from gathering information to drafting outputs faster and more efficiently with Otio.
Accelerated Writing with Otio's AI Assistance
One of the most notable features of Otio is its AI-assisted writing functionality, which makes the process of writing research papers and essays much faster. Otio generates AI-driven notes for all bookmarked content, including YouTube videos, PDFs, and articles. Users can engage in chat conversations with individual links or entire knowledge bases, similar to interacting with ChatGPT.
Streamlining Research and Writing with Otio
Otio is a comprehensive tool that streamlines the research and writing process, making it easier for researchers to manage content overload and improve their productivity. By incorporating AI-driven features, Otio offers researchers an all-in-one workspace that simplifies the research and writing process.
• Best Reference Manager • Chatpdf Alternative • Ai Research Tools • Elicit AI • Consensus Ai • Sematic Scholar • Research Paper Writing App • Research Paper Reader • How Does Chatpdf Work • Scholarcy Alternative

Aug 29, 2024
Literature Review
12 Best Tools For Perfect Research Summary Writing
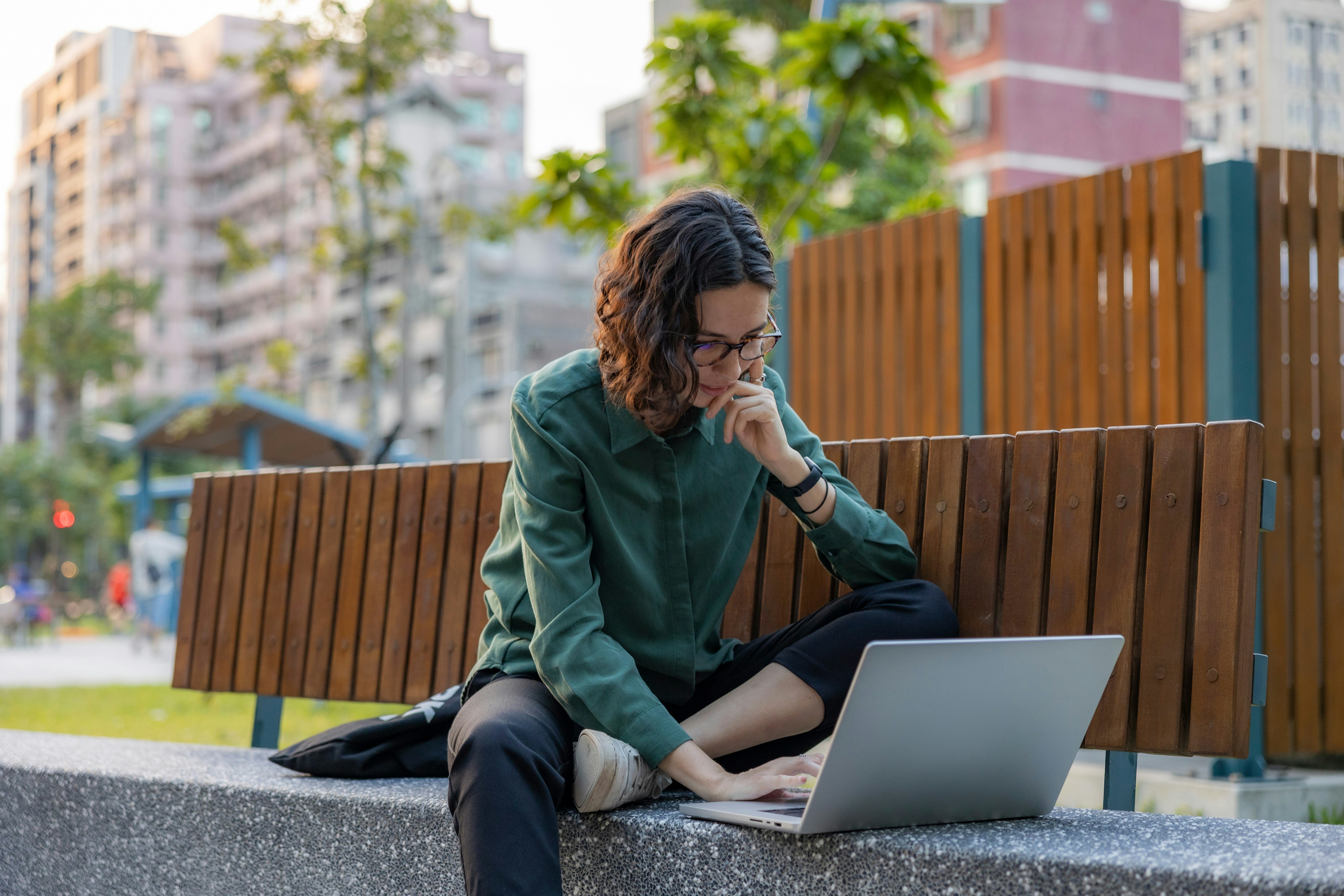
Aug 28, 2024
22 Good Websites For Research Papers and Academic Articles
Join over 50,000 researchers changing the way they read & write
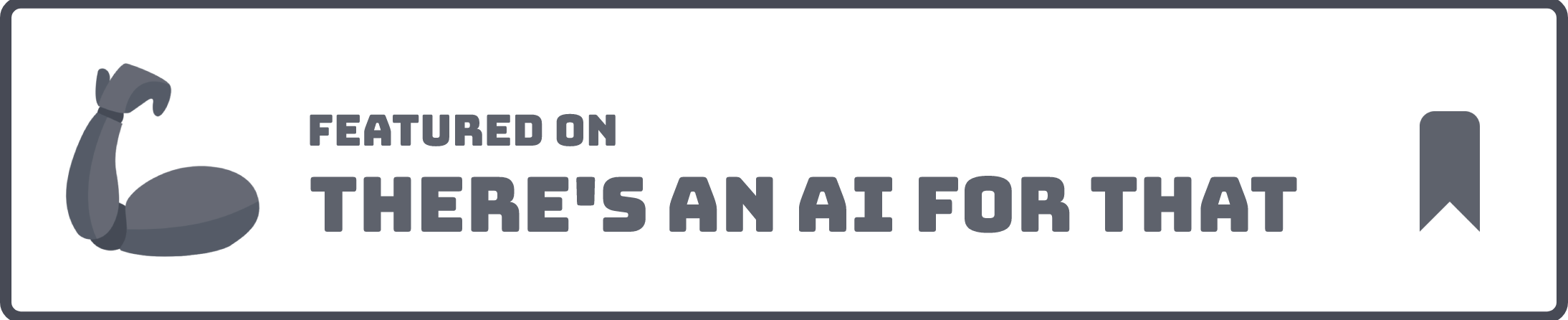
Chrome Extension
© 2024 Frontdoor Labs Ltd.
Terms of Service
Privacy Policy
Refund Policy
Join thousands of other scholars and researchers
Try Otio Free
© 2023 Frontdoor Labs Ltd.
- Argumentative
- Ecocriticism
- Informative
- Explicatory
- Illustrative
- Problem Solution
- Interpretive
- Music Analysis
- All Essay Examples
- Entertainment
- Law, Crime & Punishment
- Artificial Intelligence
- Environment
- Geography & Travel
- Government & Politics
- Nursing & Health
- Information Science and Technology
- All Essay Topics
Research Paper Summarizer
Condense long scientific articles via our research paper summarizer..
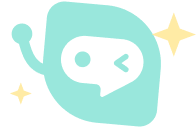
How to Use EssayGPT's Research Paper Summarizer?
Our research article summary generator is accessible to both beginners and navvies with an intuitive layout. You can summarize research papers online in 3 easy steps.
- 1. Copy and paste the content of research papers to the input box
- 2. Configure target audience, tone, and language for the summaries
- 3. Click the Generate button and then edit or copy your favorite summaries
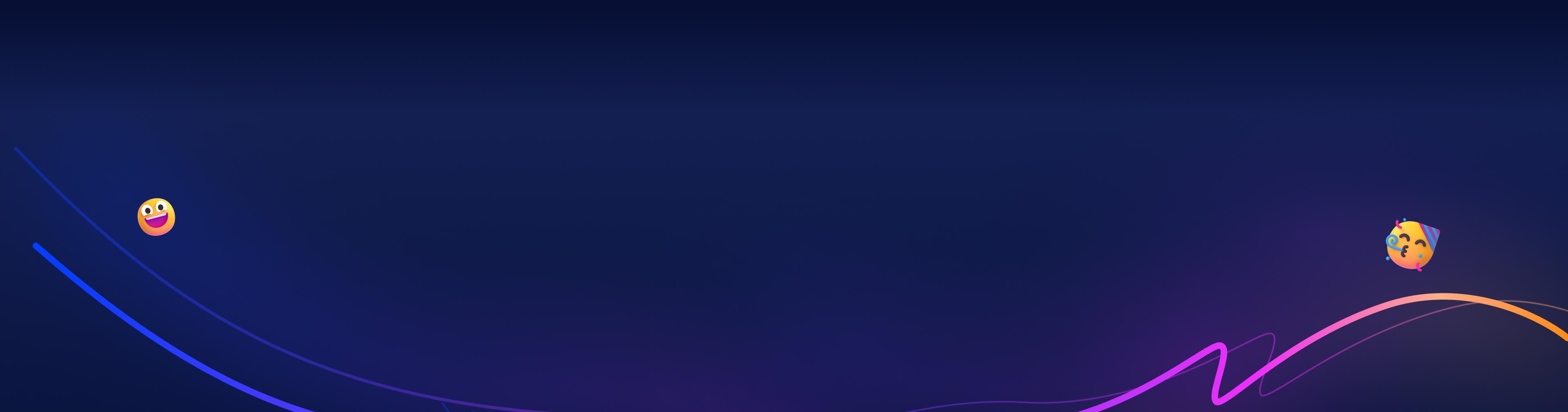
Try Our Other Powerful AI Products
Bypass AI detection with 100% undetectable AI content
Create undetectable, plagiarism-free essays with accurate citations
Solve ANY homework problem with a smart AI. 99% accuracy guaranteed.
Browser Extension
The all-in-one ChatGPT copilot: rewrite, translate, summarize, Chat with PDF anywhere
Reasons to Use Research Paper Summarizer at EssayGPT
You can save a lot of time by using the research paper summarizer from EssayGPT as it helps you quickly grasp the essence of a lengthy research paper. You don't have to spend hours manually reading and summarizing the entire content.
Improve comprehension
You can better understand the key information, important concepts, and main findings from research papers as our research paper summarizer simplifies complex information into digestible summaries.
Enhance efficiency
Do you have a huge number of research documents that need to be summarized? You can summarize multiple research papers in a fraction of the time with our article summary tool, allowing you to explore a broader range of material and generate insights faster for your own study.
Summarize anywhere
You have the freedom to read and extract information from research papers from any corner of the world as our summarizing tool lets you summarize research papers online.
Who May Benefit from Our Research Paper Summarizer?
Researchers.
Scientists must efficiently read research papers to familiarize themselves with existing research, stay updated with the latest findings, identify research gaps, and develop new research ideas and techniques.
Professors, instructors, students (mostly Ph.D. students, postgraduates, and postdocs), and scholars require the ability to quickly extra critical information from a long text to develop a deeper understanding of a subject and move forward with result-driven academic findings.
Professionals
Professionals in the field of Clinical Medicine, Engineering and Technology, Social Science, Physics, Chemistry, and others need to summarize research papers quickly to help them keep up with technological advancements or best practices in the related research areas.
Summarizing Research Papers Has Never Been Easier with EssayGPT
👨💻 Automated Summarization | Condese text with advanced AI and NLP technology |
---|---|
📝 Concise Summary | Generate a short summary in paragraph |
🗣️ Professional Tone | Maintain a formal style for research papers |
🌎 Multilanguage | Summarize research papers in mainstream languages |
💻 Online Tool | Online abstract generator for research papers |
Need More Help Than Just a Research Paper Summarizer?
Thesis statement generator, essay checker, essay rewriter, essay hook generator, essay extender, essay introduction generator, essay outline generator, free essay conclusion generator, essay shortener, dive into a world of inspiration.
- Publix Employee Performance Review Paper
- Evolution Of A Dog Essay
- Cecil The Lion: Guilty Or Murder?
- Essay on Military Draft
- Media Shaping Gender Roles
- Ronald Reagan Rhetorical Devices
- Its All About Jazz Fusion
- Rebellion In The Play Trifles By Susan Glaspell
- How Did Bill Nye Save The World
- Informative Speech: President Millard Fillmore And Chester A. Arthur
- Unattainable In The Great Gatsby
- Dynamic Character In Indian Horse By Richard Wagamese
- Marketing And Advertising : A Small Business Owner
- Jamba Juice Marketing Plan Essay
- A Brief History of Playboy Enterprises
- Kurt Lewin ( 1947 ) Divides The Change Process Essay
1. What is a research paper summarizer?
A research paper summarizer condenses the content of a lengthy research paper and turns the key information into a short paragraph or bullet point. It usually uses artificial intelligence and natural language processing algorithms to automate the process of analyzing the text and generating the summary.
2. How can I choose the best tool for summarizing research papers?
You should consider the factors that are most important to you while choosing the best AI summary generators . The majority of consumers consider accuracy, usefulness, price, and flexibility. Using these criteria, the scientific article summary generator from EssayGPT comes out on top.
3. Can EssayGPT's research paper summarizer replace a human summarizer?
No. While our research paper summarizer offers accuracy, convenience, and efficiency, it can't replace a human's work as it can't capture all the nuances or complexities of the original paper. It is advisable to use it as a starting point and complete the summary with a manual review.
4. How long does it take to summarize a research paper online using EssayGPT's research paper summarizer?
Summarizing a research paper with our research paper summarizer is quick, taking anywhere from a few seconds to a couple of minutes. But the time can vary depending on the length and complexity of the paper and internet connection speed.
5. Can EssayGPT's research paper summarizer be used for languages other than English?
Yes, you can create a summary in a variety of languages with our research paper summarizer, like Chinese, French, German, Spanish, Japanese, and Portuguese. If you select a minor language, accuracy can decrease.
6. Are there any specific skills or knowledge required when summarizing research papers at EssayGPT?
No, the learning curve for our research paper summarizer is minimal. To use this tool, you do not need any specialized or previous knowledge.
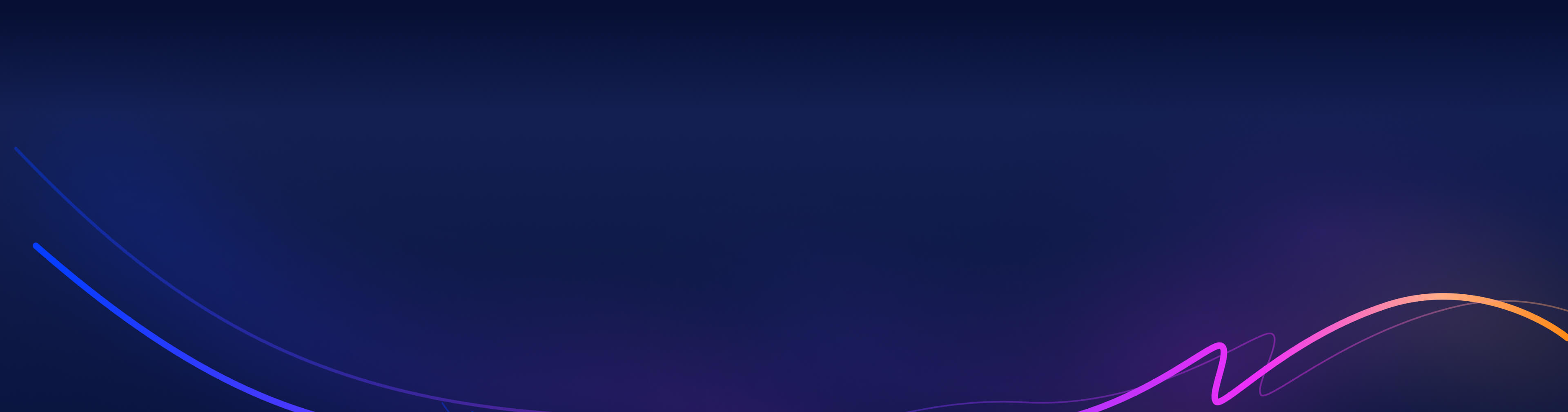
Condense Insights with EssayGPT's Research Paper Summarizer
Unveil the essence of all academic papers effortlessly and quickly distill complex ideas using our research paper summarizer. Partner with EssayGPT for clarity and brevity in your academic endeavors.

AI Summarizer
Summarize Any PDF or Document Instantly
Used by professionals in

Used by over 1,000,000+ professionals & researchers in
AI Summary for any document
Sharly ai summarizer tool is powered by artificial intelligence and provides concise summaries of any content, including pdf, general documents, articles, audio files, or presentations. no more shifting through pages of information or listening to lengthy recordings to extract key points. just upload your files and let the ai do the work. the ai summary generator reads through the file, identifies the main themes and the most essential details, and produces a resume that captures the essence of the content. with sharly's ai summarizer you turn the tedious process of summarizing into a thing of the past..
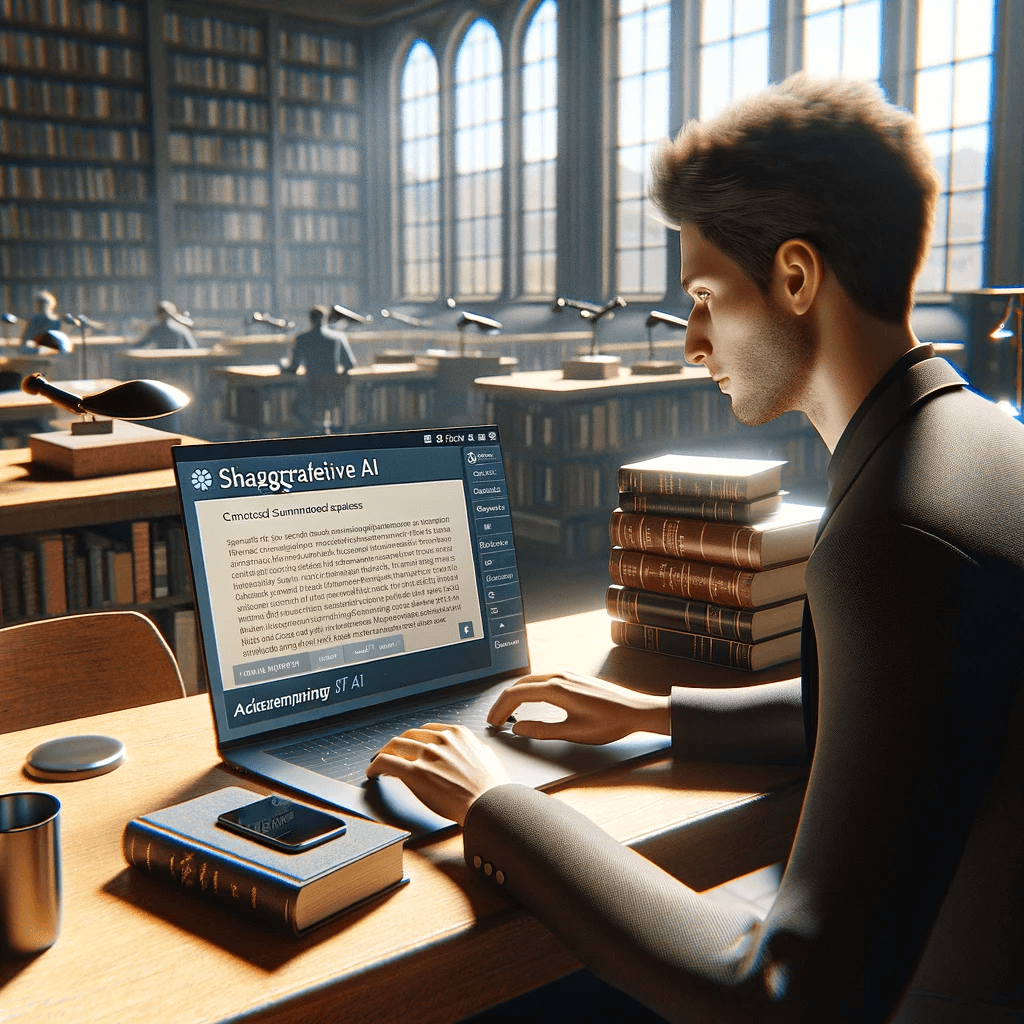
Key Features of Sharly AI Summarizer
Accurate ai summary.
Sharly's AI summary tool is capable of discerning key points from complex content accurately. Whether you're dealing with a research paper, a business report or the recording of a meeting, you can rely on Sharly to bring text to a comprehensive and accurate summary.
Time-Efficient Summarization
Using Sharly's AI to summarize text, articles, documents or audio files greatly cuts down the time you spend on reading or listening to lengthy content. This saved time can then be redirected to other productive tasks, boosting your overall efficiency.
Multi-Format Support
Sharly AI summary generator supports various document file formats, including PDF, PDF with OCR, .docx, .doc, .txt, .csv, .rtf, .odt, .ods, .odp. .pptx and .html files. It also supports audio and multimedia files such as .mp3, .mp4, .wav, .webm, .flac, .oga, .ogg and .mpeg.

Speed up Learning Processes for Academics and Students
Postgraduate students, overwhelmed by vast academic materials, turned to sharly ai for efficient study. the ai summarizer quickly condensed complex papers into clear summaries, enabling them to grasp key concepts and theories swiftly. this tool significantly saved time and enhanced understanding, proving invaluable for navigating their rigorous coursework and research, thus improving their overall academic experience and productivity..
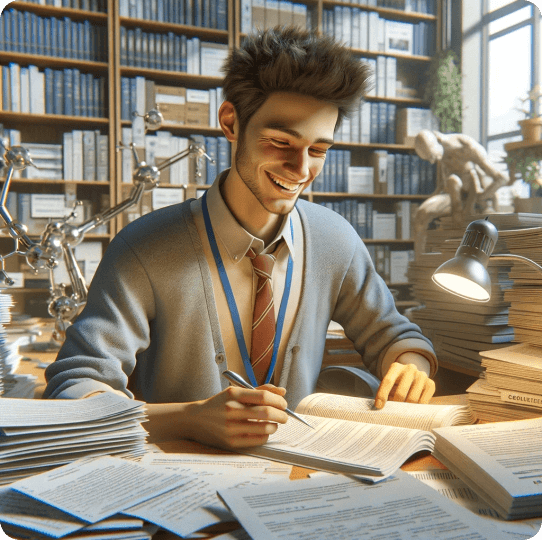
Sharly AI’s Advantages for Summary Generator
Efficient information extraction.
Rapidly condense large volumes of text into digestible AI summaries.
Cross-Document Analysis
Advanced data analytics.
Summarize and compare points across multiple PDF or other document types.
Customizable Summaries
Sharly offers flexibility in summary length and focus, tailored to your specific needs.
Secure and Confidential
Ensures the privacy and security of all uploaded documents.
User-Friendly Interface
Simple, intuitive interface allowing for easy upload and quick summarization of documents.
Integration with Popular Tools
Seamless integration with common workplace tools for straightforward access and use in various professional environments.
Citations and Page Number
Automatically includes relevant citations and page numbers in summaries, making it easier to reference and locate original content.
Custom Behavior
Offers customizable summarization options, allowing you to tailor the focus and depth of summaries to their specific needs and preferences.
How to create AI summaries?
Which file formats does Sharly's AI summarizer support?
How quickly can Sharly's AI summarize content?
Does Sharly's AI summarizer maintain the quality of the original content?
Is it possible to use Sharly's AI summarizer for multiple content at once?
"Sharly improved our customer support, enabling us to provide faster, more accurate assistance; our customers couldn't be happier."
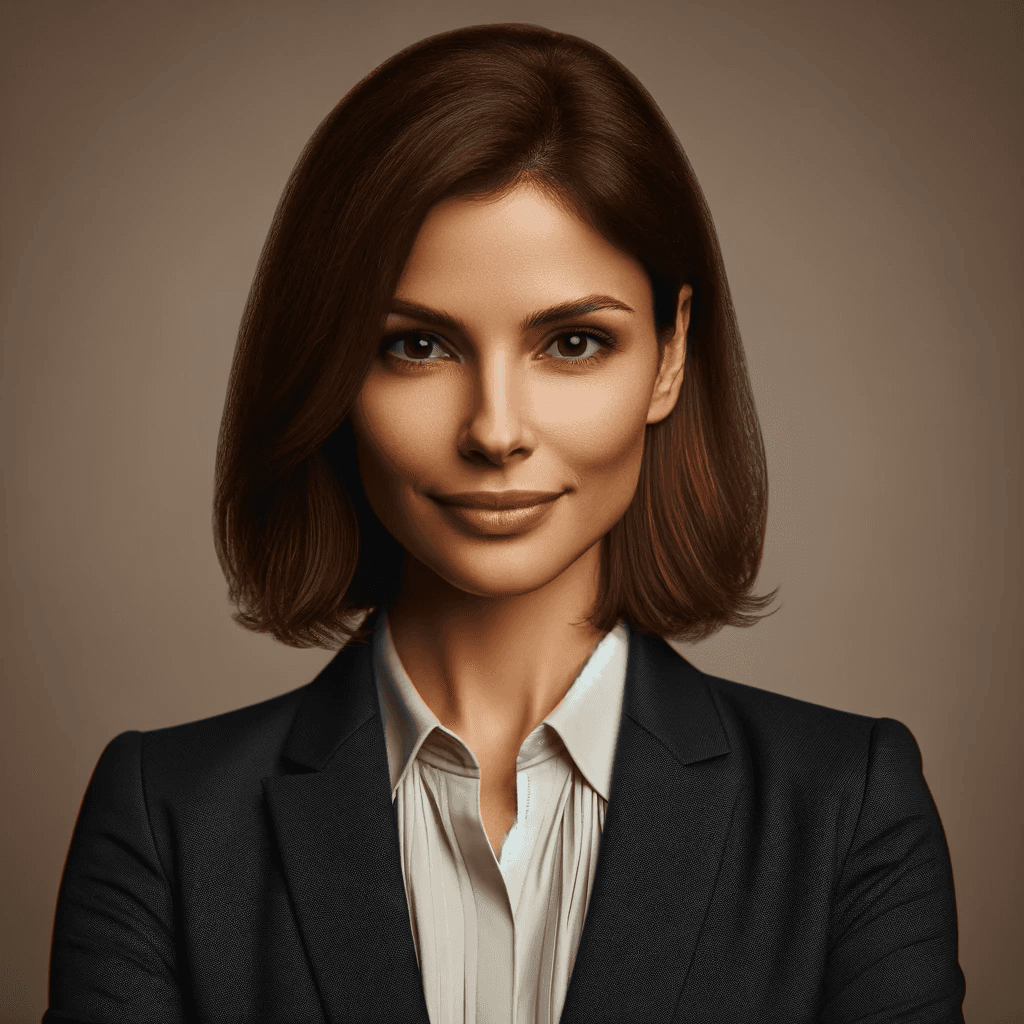
Sarah Mitchell
Director of Customer Support at SupportPro Innovations
"Sharly AI has transformed my research workflow. It efficiently summarizes complex texts, enhancing my focus on critical neuroscience analysis"
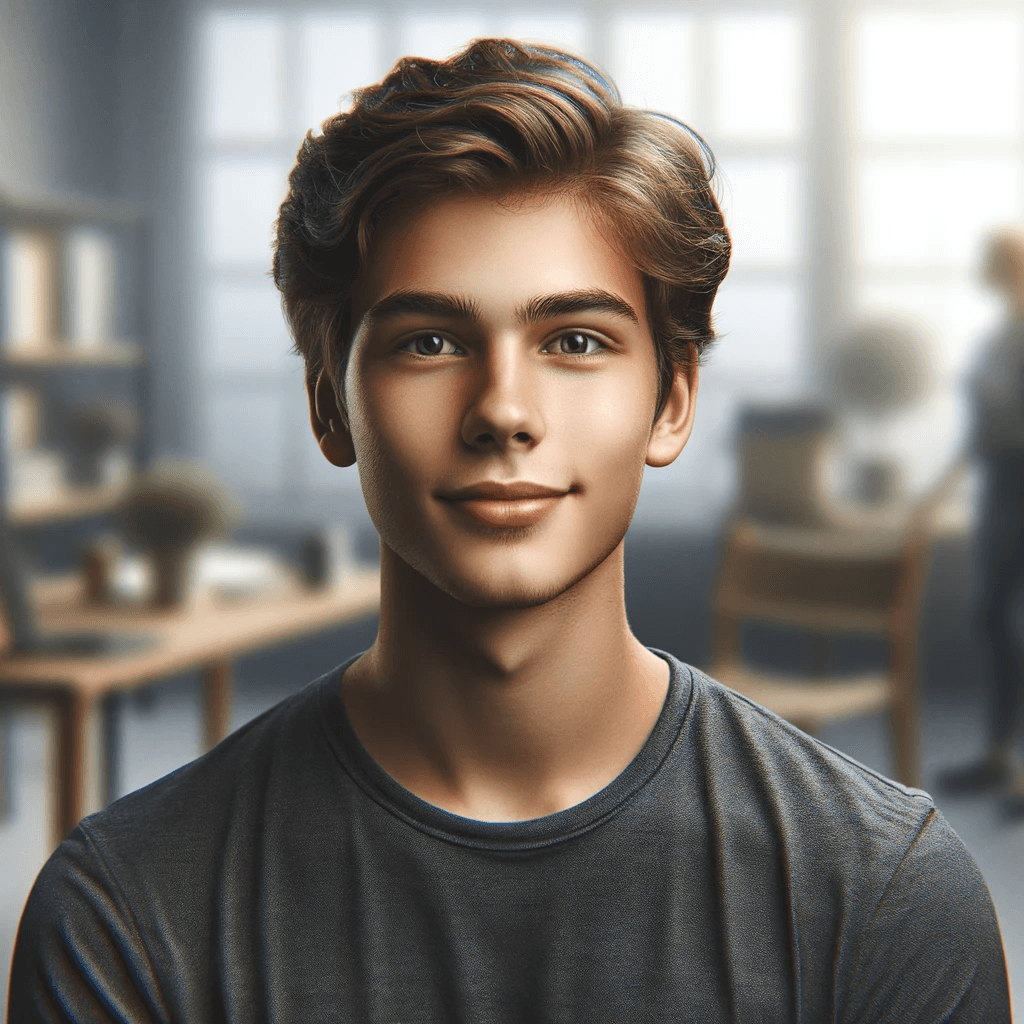
Ethan Parker
Research Assistant in Computational Neuroscience
Try Sharly AI for Summary
Start for free — upgrade anytime.
Joining as an organization? Contact Sales
Start for free
Writing Tools
General Writing
- Paragraph Generator
- Article Rewriter
- Paraphrasing Tool
- Essay Writer
- AI Text Generator
- Sentence Expander
- Paragraph Rewriter
- Sentence Generator
- Plagiarism Remover
Assistant Writing
- Grammar Checker
- Online Proofreader
- Text & PDF Summarizer
- Spell Checker
- AI Translator
- Plagiarism Checker
Punctuation Checker
Creative Writing
- Prompt Generator
- AI Response Generator
- Hashtag Generator
- Slogan Generator
- Tweet (Thread) Generator
- Instagram Caption Generator
- Script Generator
- AI Lyrics Generator
- AI Story Generator
Find captivating Instagram captions for all moments.
Explore perfect email templates for every occasion.
Discover ideal quotes to elevate your book, speech, or essay.
Find the right words to voice your thoughts.
Learn all there is to know about ChatGPT.
Easily translate into 100+ languages.
Explore personal bios for hottest social media platforms.
Discover heartfelt love letters for your beloved.
Learn everything about AI writing and tools.
Discover the top alternatives to well-known AI tools.
Comparisons of popular AI writing tools.
Browse our curated lists of best alternatives to popular AI writers.
HIX Summarizer
Summarize articles, documents, and - surprise - even youtube videos, all in a flash.
Click to upload your file here
Supported file types: DOC/DOCX/PDF/TXT.
Max file size is 10 MB.
URL to summarize
Summary Type
- Bullet Points
Output Language
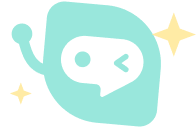
The Most Powerful Summarizer: Summarize in One Click
HIX Summarizer turns lengthy, complex content into brief summaries with a single click, streamlining your research process, saving you time, and bringing you up to speed.
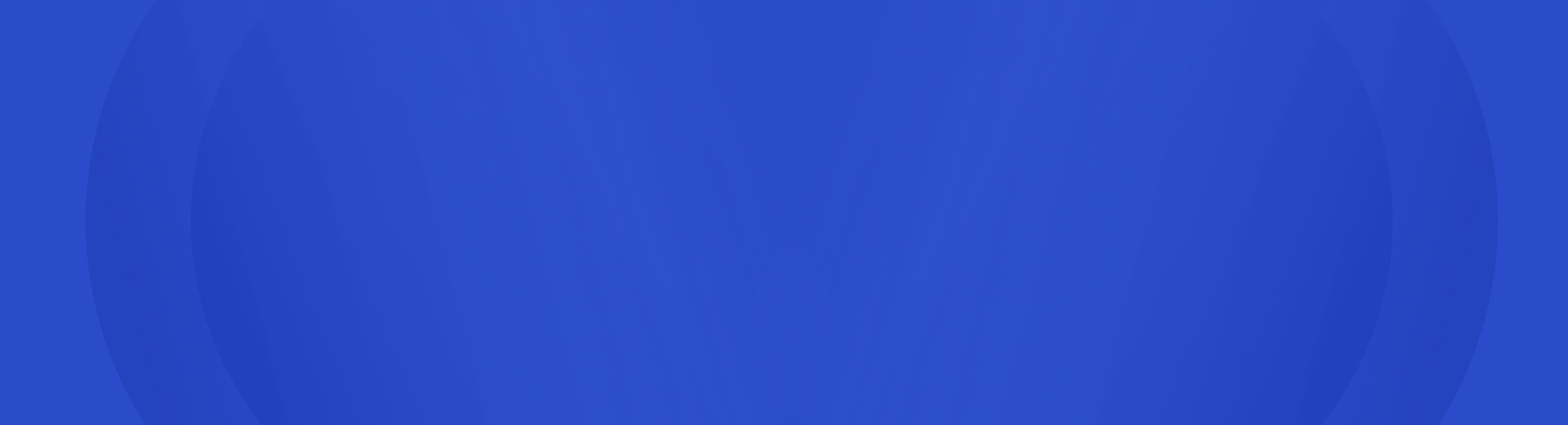
BrowserGPT for YouTube Summarize YouTube Videos Instantly
Summarize right on YouTube
Extract all key points accurately
Time-stamped, interactive summaries

Summarize All Types of Information Quickly and Easily
Don’t waste time reading lengthy content, let AI do the work. Our tool will quickly process information in 3 forms, enabling you to focus on what matters.
Direct copy-paste text, paragraphs, or articles
YouTube or webpage URLs
Documents like PDF, TXT, DOC
Summarize YouTube Videos in an Instant
When you're pressed for time but still want a quick overview of what a YouTube video covers, let our summary generator do the watching for you and present just the essential details in a matter of seconds.
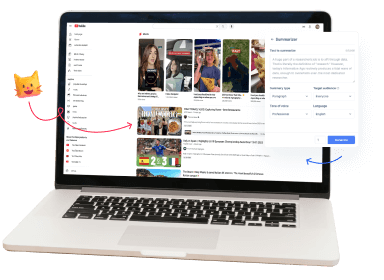
Summarize the Way You Like It
Imagine having an assistant that doesn't just summarize, but tailors the summary to fit your exact needs. You decide the format that works best for you - concise bulleted lists or flowing paragraphs.
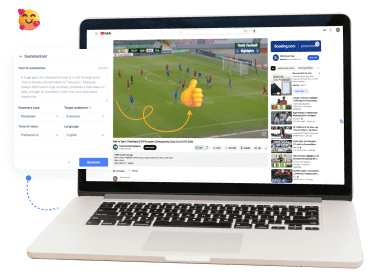
Summarize Anytime, Anywhere
With the BrowserGPT, you get access to our AI summarizing tool whenever and wherever you consume content online. Just select any text on a web page - a paragraph or an article - and generate an instant summary with a click.
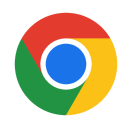
How to Summarize Online With HIX Summarizer?
Summarize an article, a document, or a Youtube video with HIX Summarizer is as easy as 123:
Just copy-paste your text, upload a document, or drop a URL of a webpage or Youtube video.
Specify whether you want the summary to be in paragraph or bullet point format.
Click on the 'Generate' button, and an instant, concise summary will be generated for you.
Why HIX Summarizer?
💨 Swift Summaries | Condense text instantly |
---|---|
📑 Beyond Text | Video summaries supported |
🧩 Custom-Tailored | Summary based on your selected format |
👏 Summarize Easily | Paste text, upload documents or enter URLs |
Discover Other Summarizers of HIX Writer
Explain it to a 5th grader, continue writing, linkedin summary generator, story summarizer, real estate bio generator, professional bio generator, free spell checker, sentence shortener, what is hix summarizer.
HIX Summarizer is the best AI summarizer and summary generator. This summarizing tool is able to extract important info from a passage of text, a PDF/Doc/TXT file, or a webpage instantly. It's not only an article summarizer, it can recap a Youtube video as well.
How do I use HIX Summarizer?
You have three main options to use HIX Summarizer for summarization: simply copy and paste text; upload a PDF, Doc or TXT file; or provide a URL for a web page or YouTube video. You can choose the generated summary to be a paragraph or in bullet point form.
How can an AI summarizer help me?
If you have a long, complex article or video but don't have time to read or watch the whole thing, an AI summarizer can be a lifesaver. It quickly pulls out the most important points, turning a lengthy piece into a short, easy-to-read summary. Plus, you can use this summary as a starting point to build your own content.
Which is the best AI summarizer?
HIX Writer is one of the best AI summary generators enabling you to summarize text, Youtube videos, PDF documents and more.
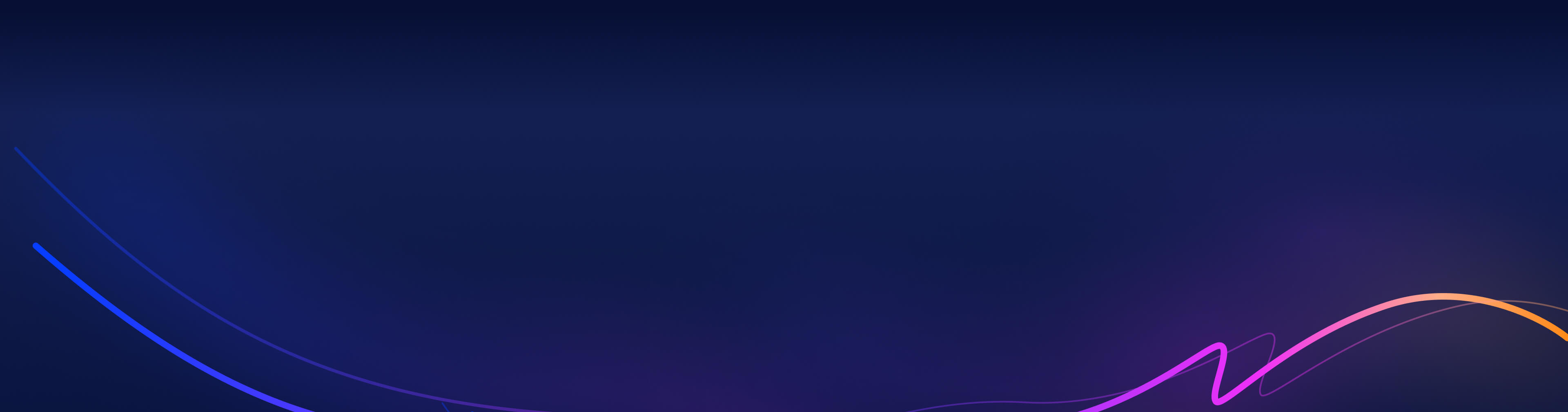
Try Our Powerful, All-in-one AI Writing Copilot Today!
Enhance your writing process with HIX Writer. Whether you're crafting fact-based articles, humanizing AI text, or rewriting, summarizing, and translating your content, HIX Writer provides the tools you need.
AI Text Summarizer
Use AI to Summarize Long Text for Saving Time and Reading Faster.
How to Use Text Summarizer
Paste or type the long text you want to summarize in the text box.
One-Click Summarize
Click the "Generate Summary" button to quickly get the text summary.
Preview and Use
Preview the generated text summary and copy it for use in your documents, reports, etc.
Key Features
- Supports intelligent summarization for texts in any language.
- Uses advanced AI algorithms to accurately capture key information.
- Advanced prompts allow you to generate: Summary, Key points, Highlights, Related questions and answers, and Keywords.
- One-click to generate, simple and efficient.
User Feedback
This text summarizer online tool tremendously increased my work efficiency. I often need to read long documents and now I can just generate a summary first with this tool to decide if I want to read the full text.
I really love the one-click text summarization function. Copying the summary is so convenient when I need to write the references section for my reports.
I use this text summarizer to help me parse long research papers in my field much faster. It’s been a total game changer for my literature reviews - I can now get through 3 times as many papers in the same amount of time.
Frequently Asked Questions
What type of documents can your tool summarize, will the generated summary accurately represent the full document, get summary now.
- Compress PDF
- PDF Converter
- Chat with PDF
AI PDF Summarizer
- Translate PDF
- Delete PDF Pages
- Extract PDF Pages
- PDF Annotator
- Number Pages
- Watermark PDF
- PDF to Word
- PDF to Excel
- Word to PDF
- Excel to PDF
- Protect PDF
- Flatten PDF
- PDF Scanner
- › AI PDF Summarizer
Add PDF , image , Word , Excel , and PowerPoint files
Supported formats:
With AI PDF, you can utilize the powers of artificial intelligence to summarize PDFs for free! The interactive chat function lets you request specific information to be summarized and presented to you in a matter of seconds. AI PDF Summarizer lets you understand document contents without having to read through every page.
- Chat with a PDF for free, without sign-up
- TLS encryption for secure file processing
- An AI assistant for instant PDFs summaries
How To Use AI PDF Summarizer
- Import or drag & drop your file to our AI PDF Summarizer.
- Review the summary that’s instantly generated.
- Ask AI additional questions about the PDF in the prompt box.

Spotlight: Jovu (Coding)

Scisummary v1.2.0
SciSummary is a cutting-edge AI-driven summarization tool designed to make digesting scientific articles easier. It enables users to quickly and accurately understand complex scientific articles without having to read the full text.
To use the service, users can email a text, a link, or even attach a PDF and within minutes they will receive a summary in their inbox. The service is powered by a custom-tuned GPT-3 model that learns as it goes, with a team of PhDs analyzing requested summaries and guiding the training of the model.
It is currently in a public beta, and users are encouraged to rate their summaries. SciSummary was founded in 2023 with the mission of making digesting scientific articles easier.

Community ratings
How would you rate scisummary.
Help other people by letting them know if this AI was useful.

Feature requests
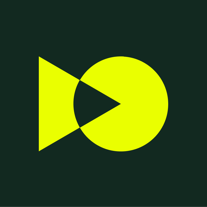
Most impacted jobs
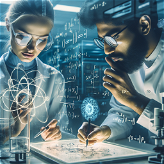
Pros and Cons
3 alternatives to scisummary for scientific article summaries.
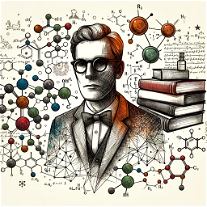
If you liked Scisummary
Verified tools, other tools.
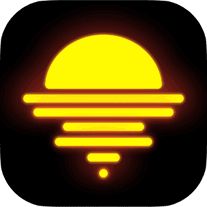
Related topics
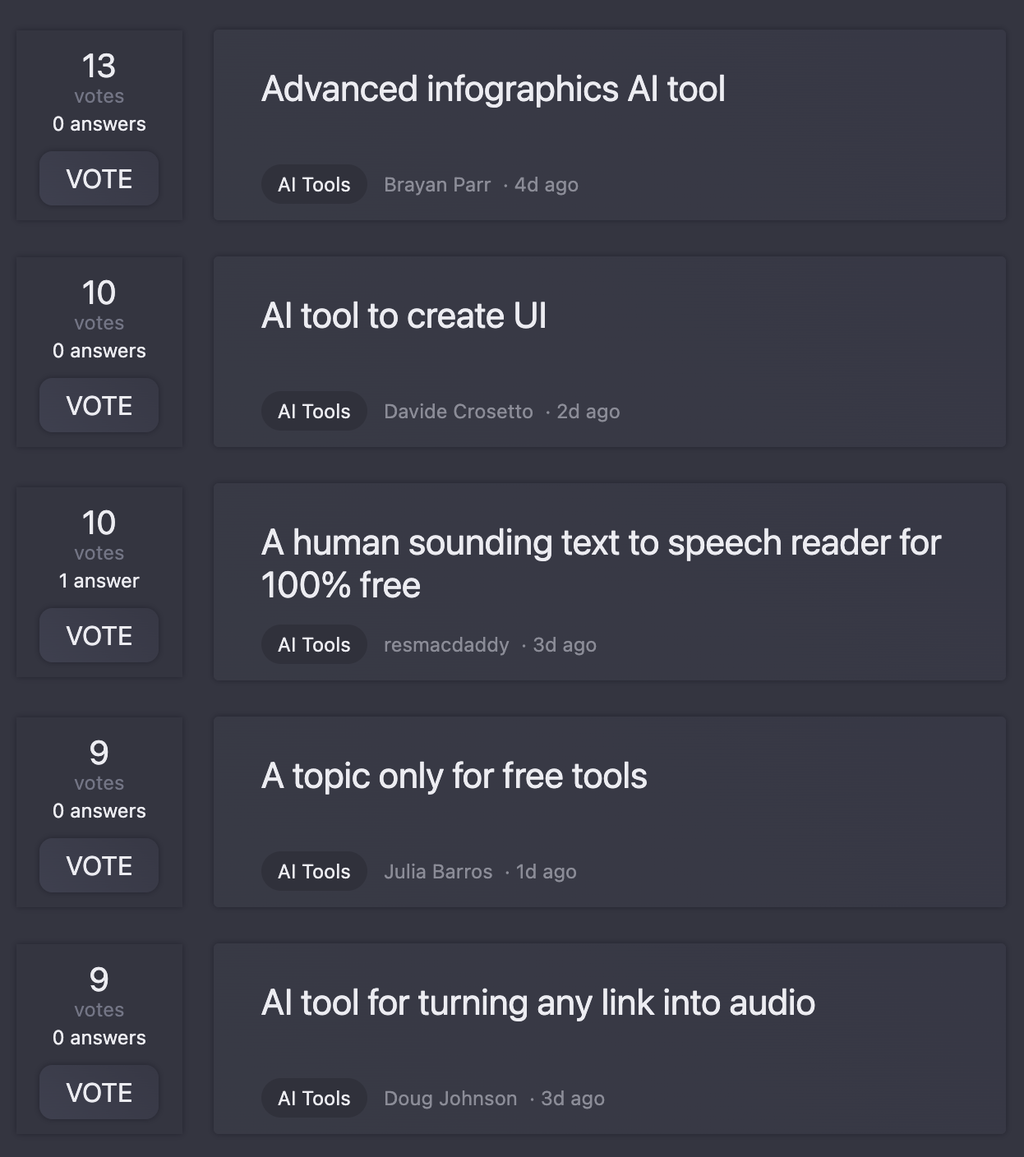
Subscribe to our exclusive newsletter, coming out 3 times per week with the latest AI tools. Join over 470,000 readers.
To prevent spam, some actions require being signed in. It's free and only takes a few seconds.
#1 AI Aggregator · #1 AI Newsletter · #1 AI Community
Choose the options that apply to you:
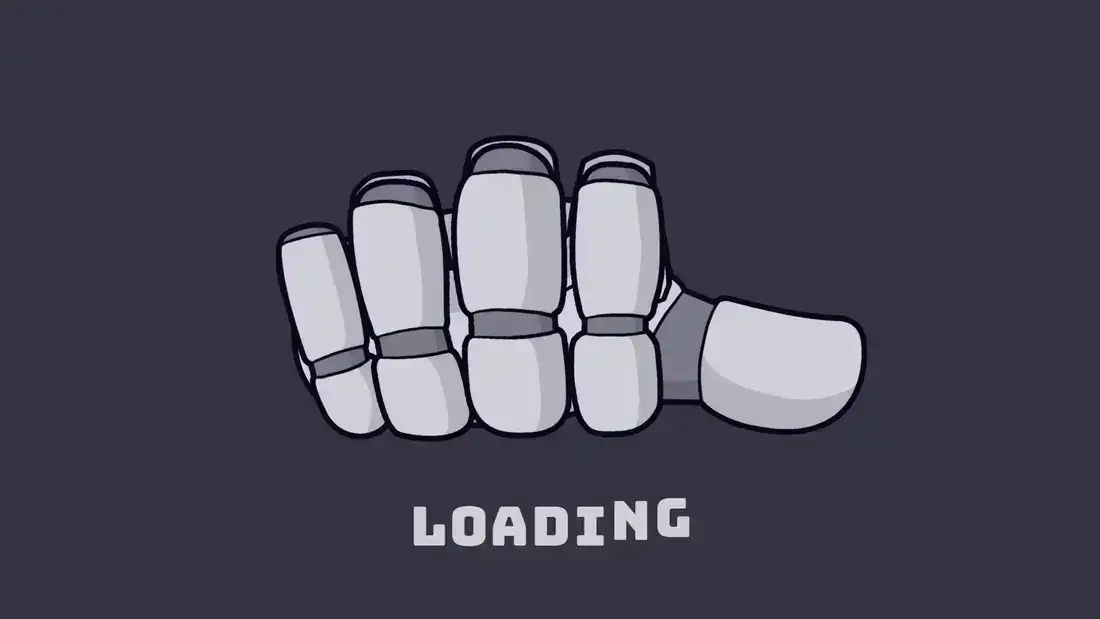
Feedback and Incident Report
Help us improve: share your experience with 'Scisummary'
Summarize any text with One Click
Sample Text
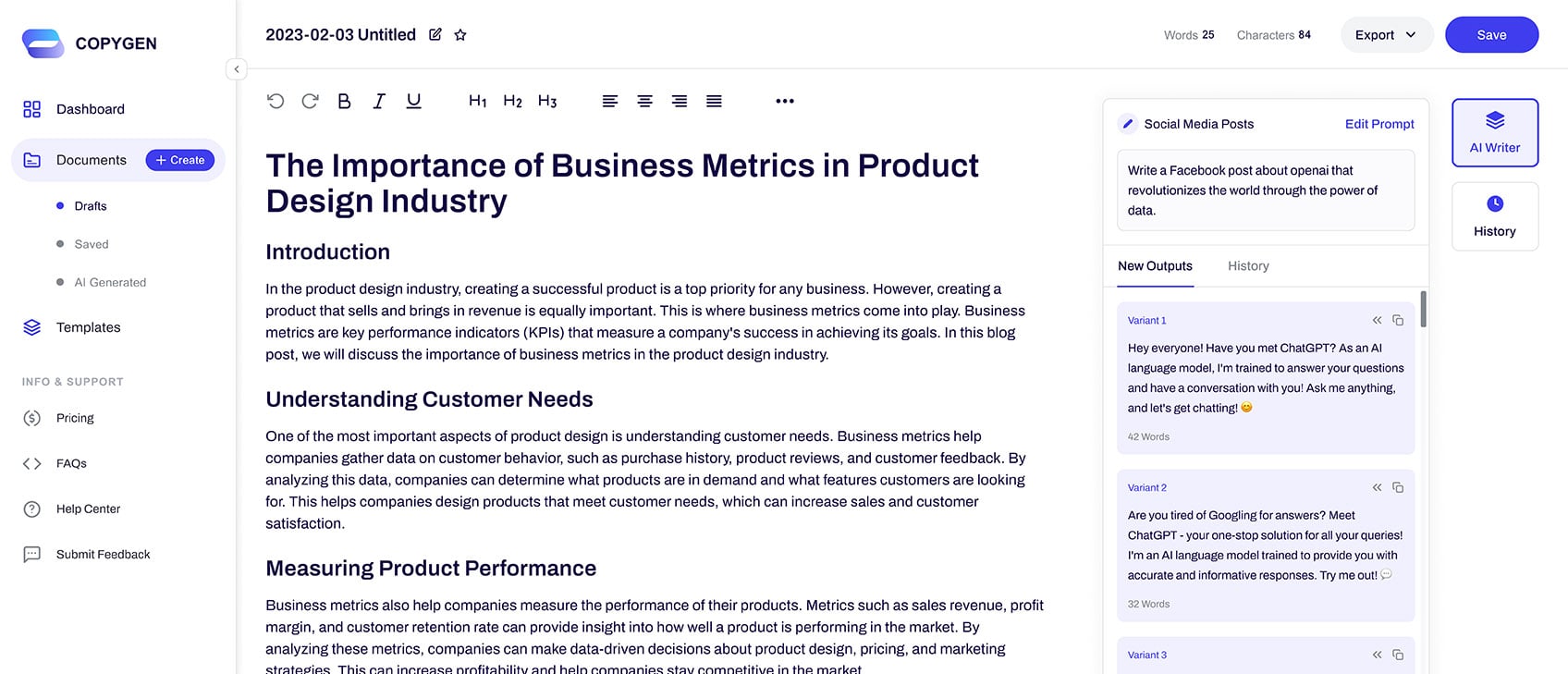
Generate Accurate Summaries in 3 seconds
Our summary generator uses advanced AI technology to break down your long content into quick, digestible summaries in just one click. Use it to summarize your articles, academic papers, business reports, or any kind of content.
Get the Important Facts & Data
This text summarizer quickly extracts important information from large texts and presents complex content in engaging chunks. You get the most relevant and crucial data in just one click.
Choose Your Summary Length & Style
You can customize the length and format of the summary. Create a quick content overview for your blog post or get a detailed summary for your academic research. You’ve also got an option to generate the summary in paragraph form or bullet points.
Over 5000 users like you have used this summary generator to convert their lengthy text into efficient, 100% accurate summaries
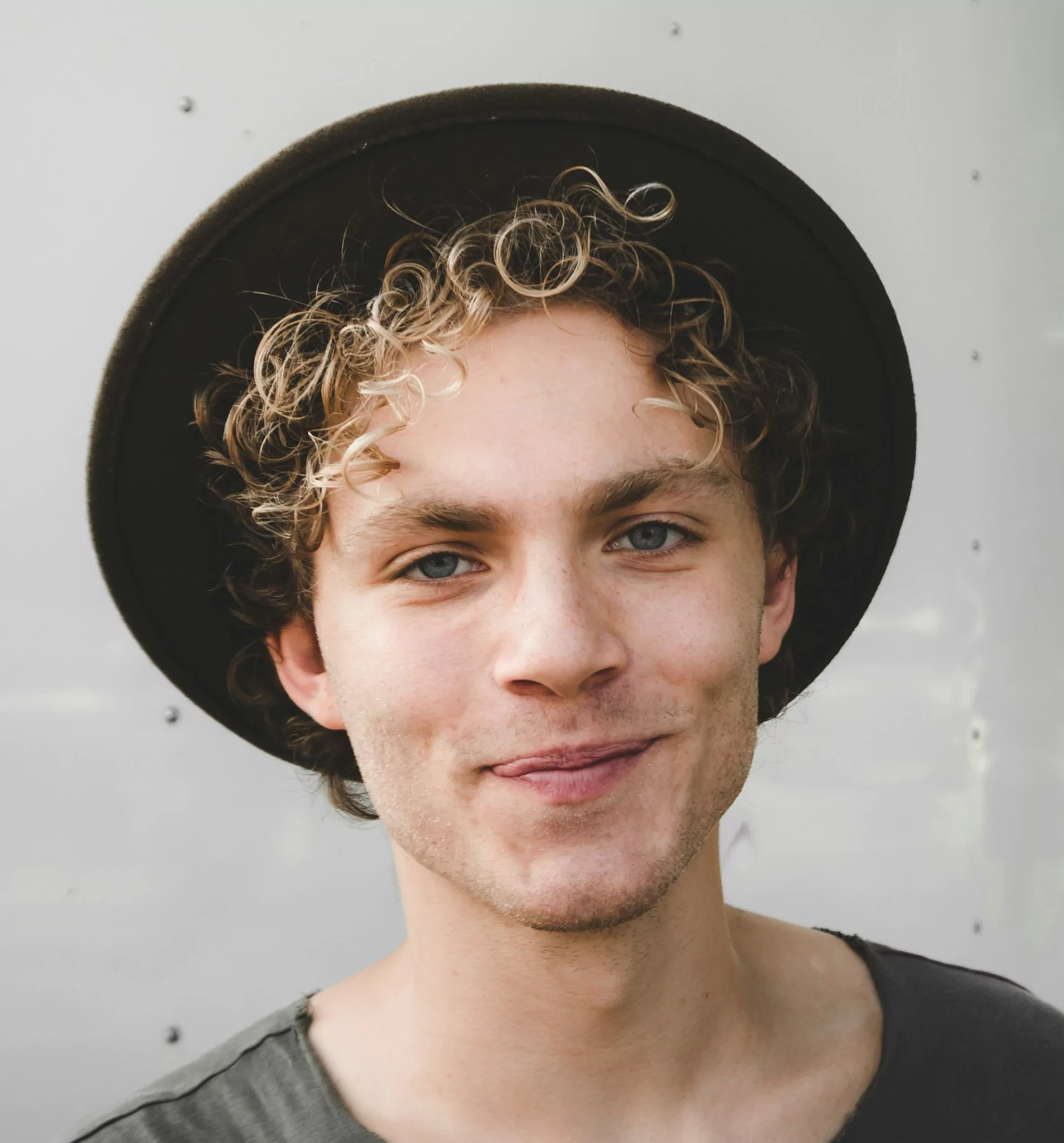
“During my PhD research, SummaryGenerator.io proved invaluable. It quickly distills the main ideas of complex academic papers. The tool`s precision in highlighting relevant data and arguments made my literature review process much more manageable.”
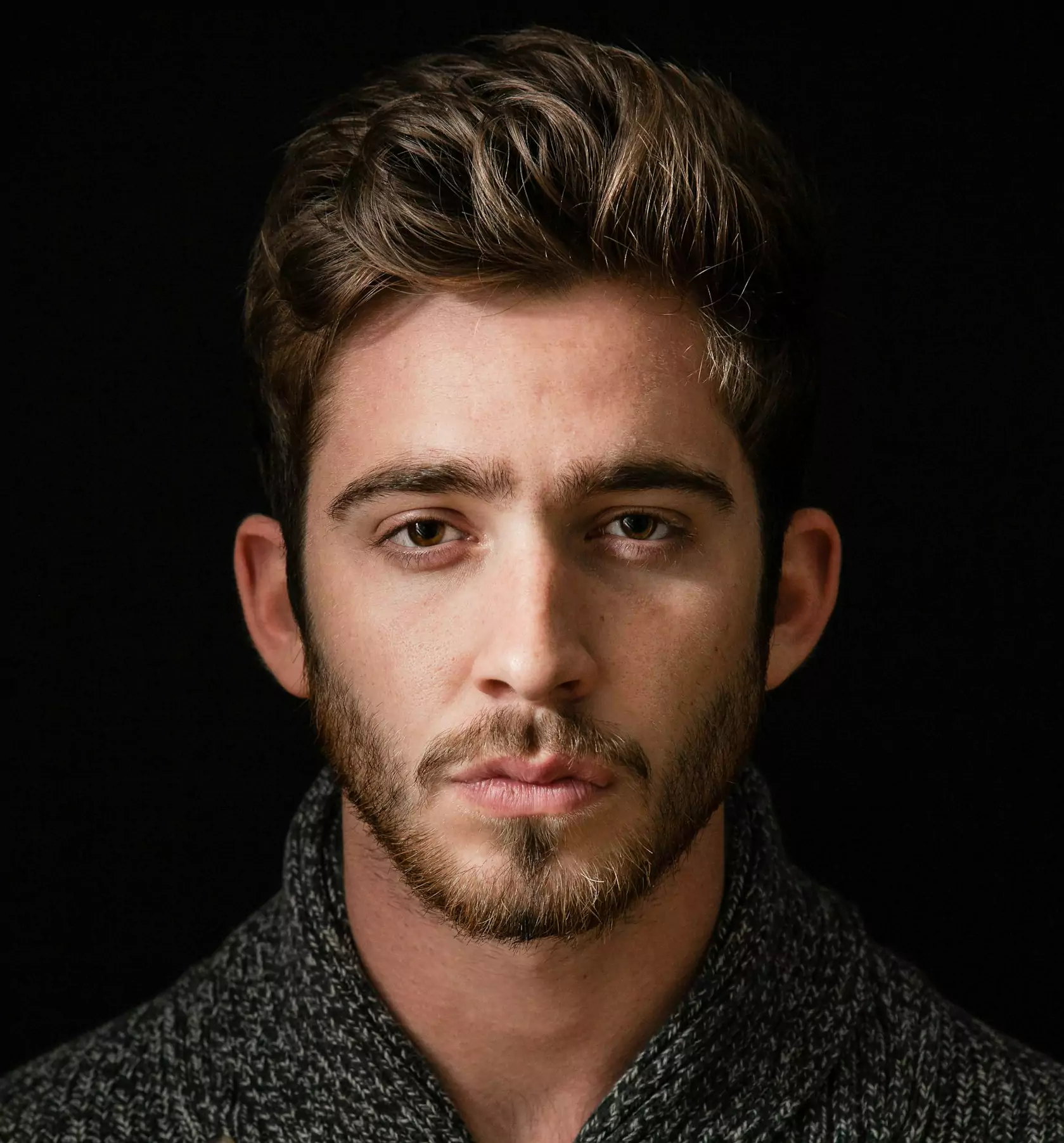
“As a freelance writer, this text summarizer has been a game-changer for me. It efficiently condenses long articles, helping me research and write faster. The summaries are concise and comprehensive, saving me hours of reading.”
How Does the Text Summary Generator Work?
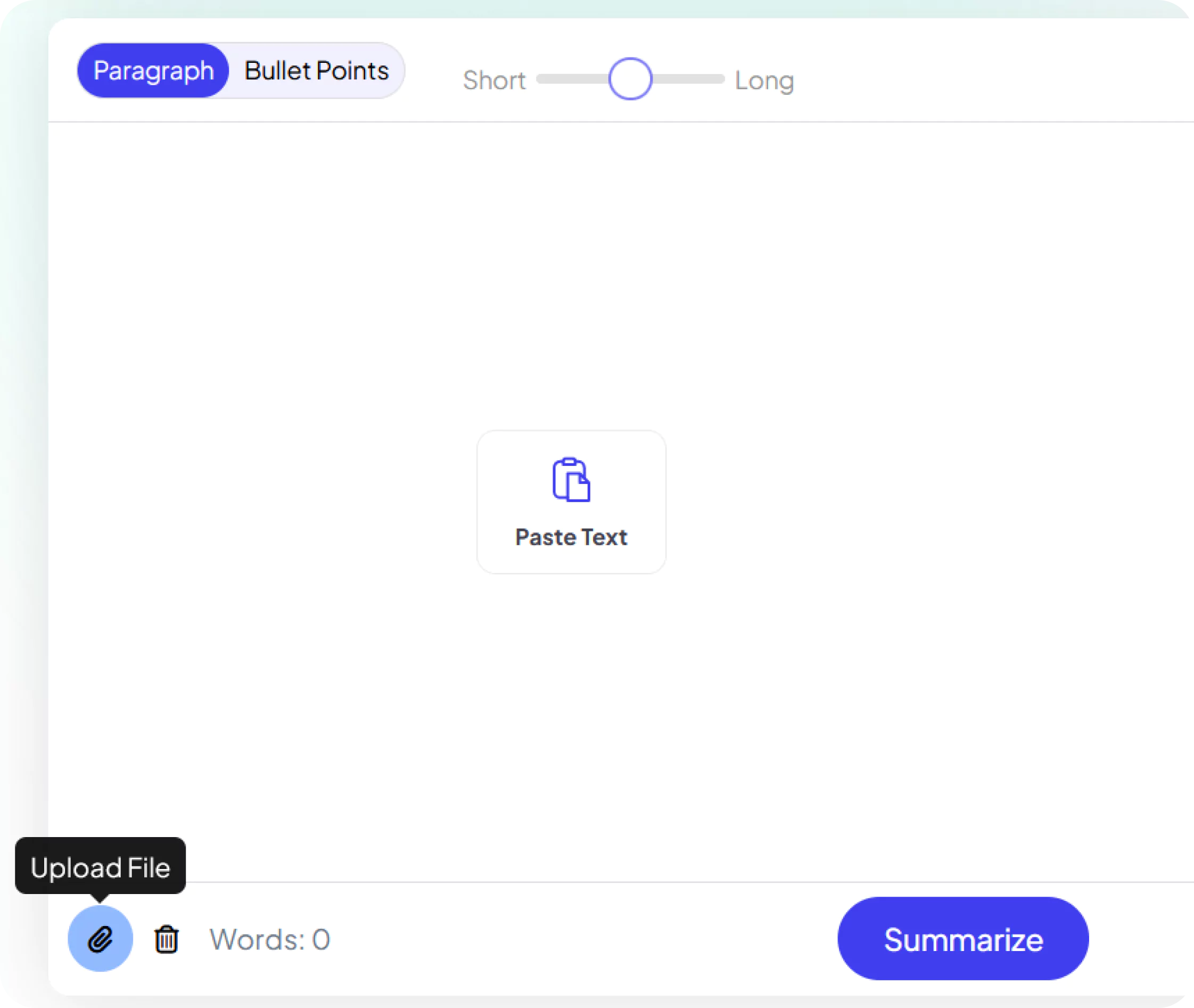
Enter the Text
You can copy the text and paste it into the box above, or upload a file from your desktop. You can upload a Doc or PDF file.
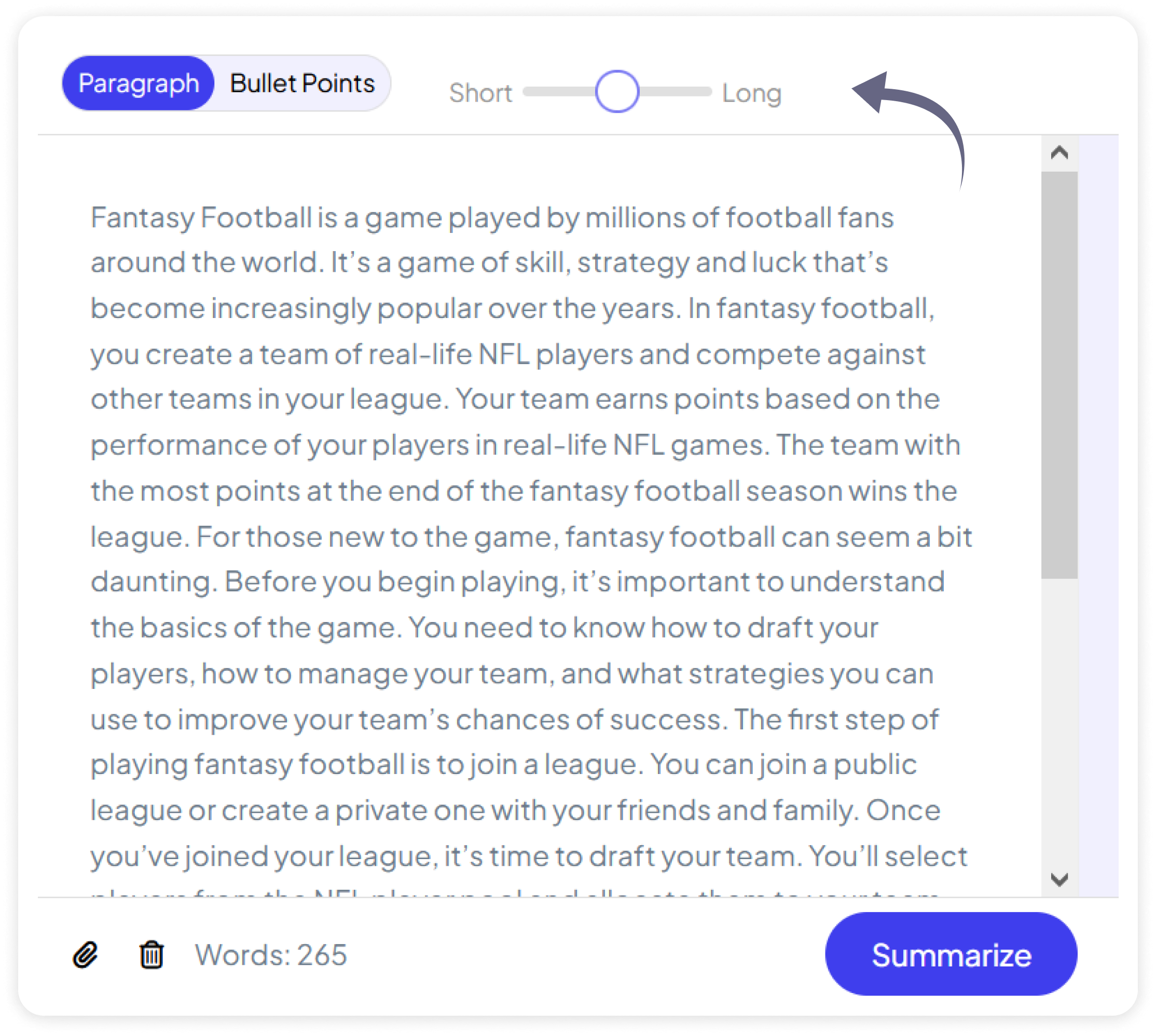
Choose the Summary Length and Style
Choose your desired summary size between small, medium-length, and large summary options. Decide if you want the summary in paragraph form or bullet points.
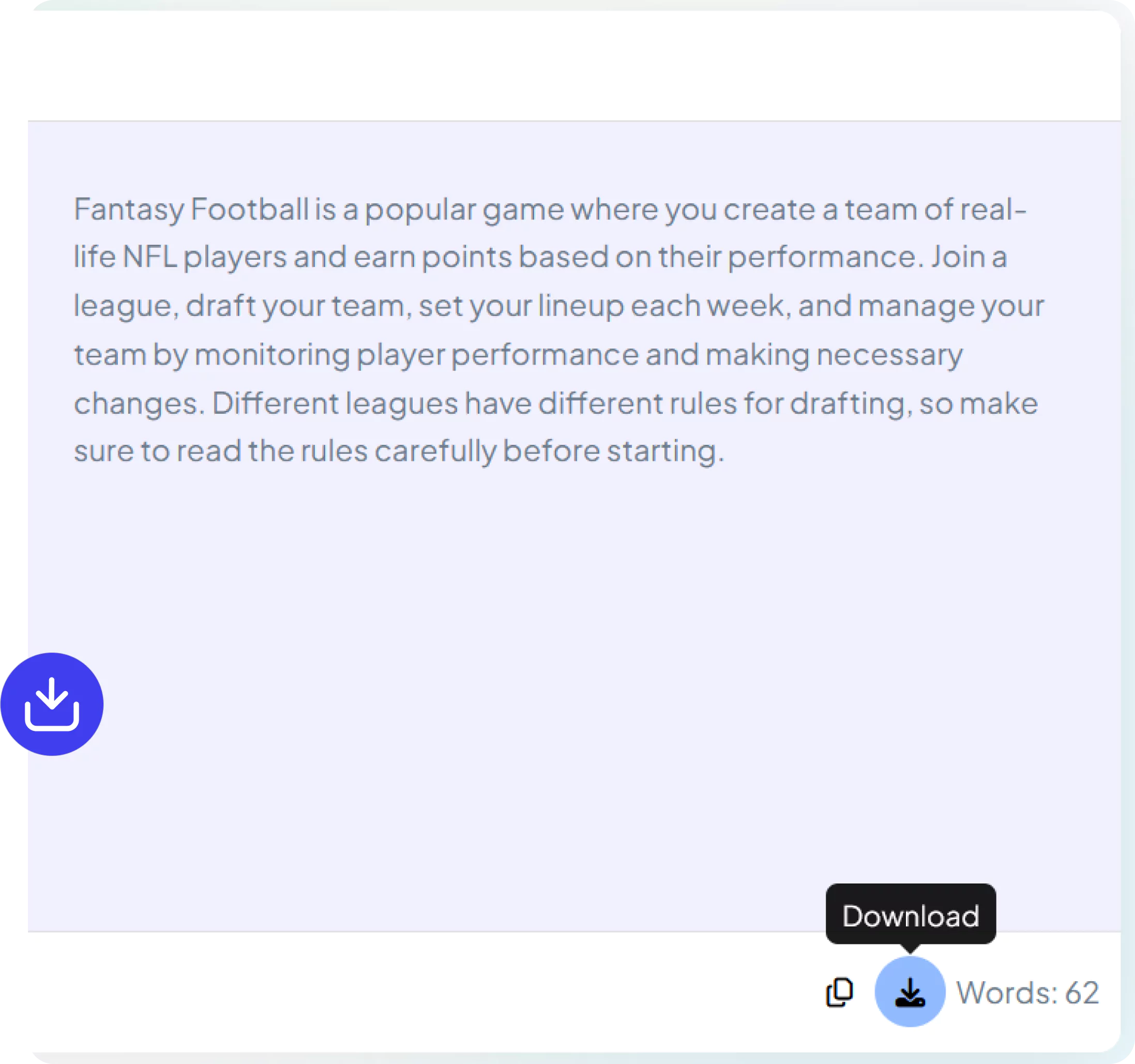

Get Your Summary
Click the “Summarize” button, and you’ll get the summary in a few seconds. You can simply copy the generated summary or download it as a Doc or PDF file. That’s all!
What Kind of Content Can You Summarize with this Summary Generator?
Academic papers.
Quickly turn complex research papers or articles into easy to digest summaries.
Business Reports
Overwhelmed by business reports? Instantly get the key insights and data from extensive reports.
News Articles
Get the gist of current events and news stories without reading the full text.
Book Summaries
Create brief overviews of books for study or leisure reading.
Technical Documents
Struggling with technical jargon? Simplify technical content into digestible summaries.
Meeting Minutes
Need to recap meetings? Convert long meeting notes into clear, actionable points.
- Generate Your Summary Now!
Boost your writing productivity
Ready to transform your text.
SummaryGenerator.io turns your lengthy texts into crisp, clear summaries with just one click.
- Summarize Now
- Free to use
- Generate summaries in 3 seconds
- No signup required
Frequently Asked Questions
How can i write a summary online for free, what is the best free ai for summarizing, how do you write an ai summary, can ai summarize a pdf, upgrade to pro.
We currently support a maximum of 10,000 words.
Summarize any PDF with AI
- Get 40 Credits free to summarize 40 pages
- Upload PDF files upto 200 MB in size
- Get a high-quality summary with all facts
- Download summary as PDF in just 3 clicks
- Read annual reports, industry reports faster
- Summarize legal documents, case studies
- Read research papers and books in 70% less time
No credit card required
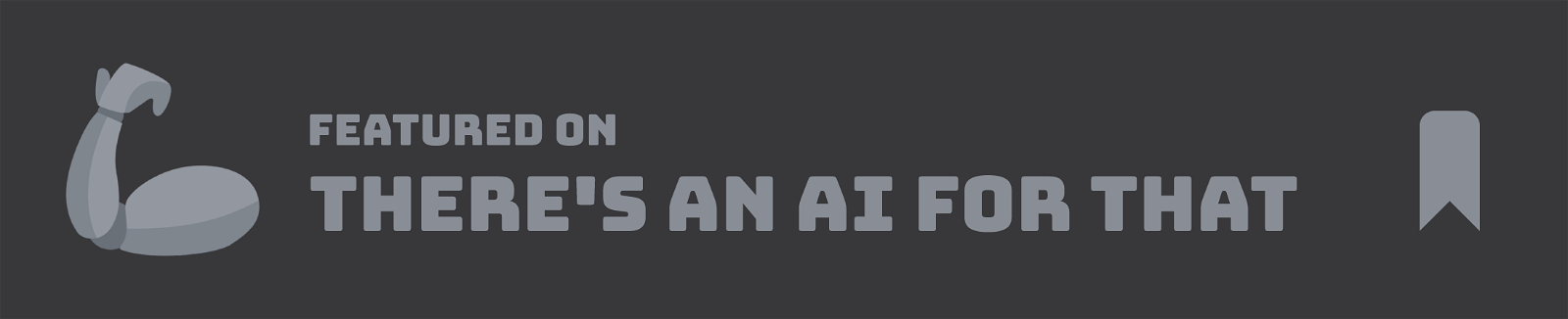
How it Works
No subscriptions. Buy credits when you need them. Credits don't expire.
STUDENT PLAN
200 credits = summarize 200 pages
500 credits = Summarize 500 pages
2000 credits = Summarize 2000 pages
From the blog
Learn how Summary With AI can help you learn and work faster
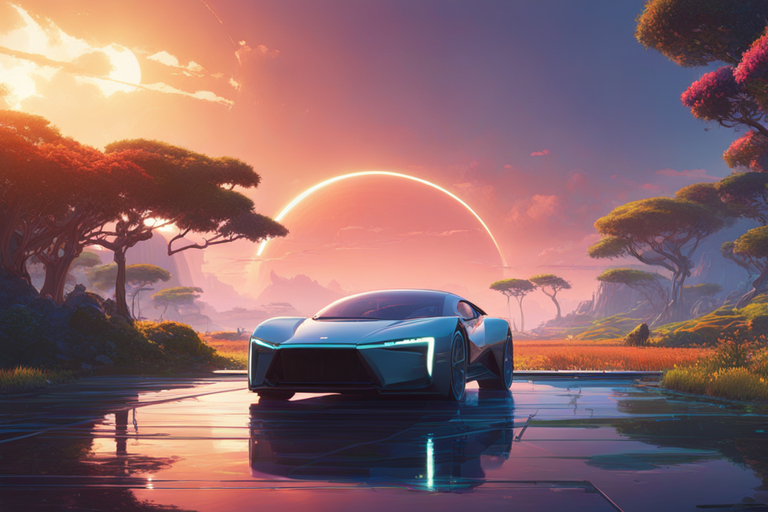
Get 10 credits for every referral - until March 20, 2024
We'll send credits your way for sharing SummaryWithAI with your friends.

Soumya Gupta
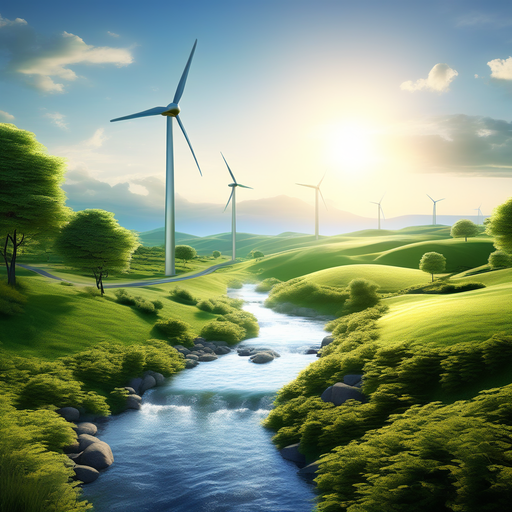
Summarize Climate Reports with AI
Climate reports, known for their length, information density, and technical jargon, are challenging to digest. There's a growing need for efficient summarization tools to make these reports more accessible and understandable.
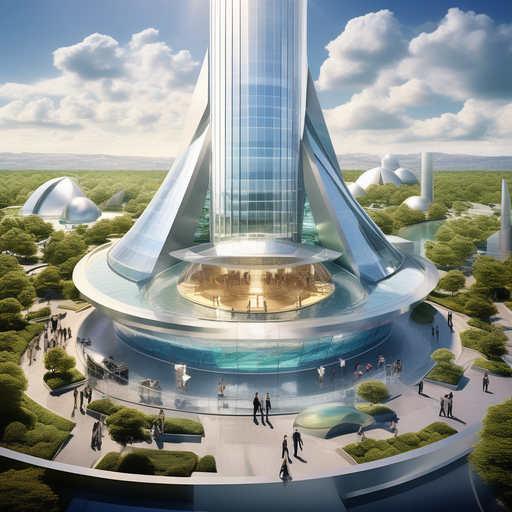
AI in Finance: Read Corporate Annual Reports
Corporate annual reports are a goldmine of information but sifting through them is time-consuming and often tedious. Read 100+ page corporate filings faster with AI.
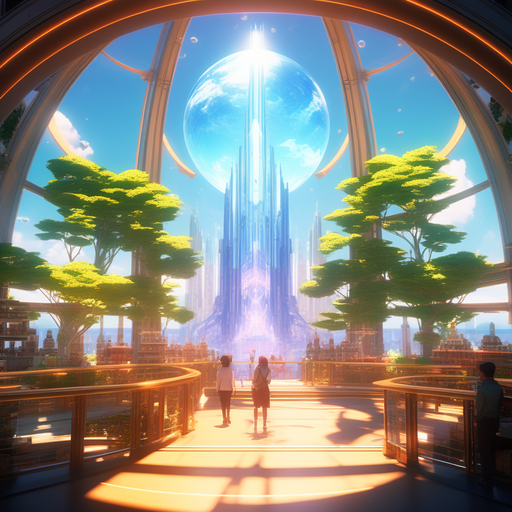
How to read research papers faster
Navigating through extensive research papers can be time-consuming and overwhelming. Summary with AI offers a solution that simplifies this process, making academic research more accessible and efficient.
Subscribe to get updates.
We care about your data. Read our privacy policy .
Brought to you by
AI Text Summarizer
Use our AI text summarizer to create concise, accurate summaries of your documents, articles, and reports. Ideal for students, professionals, and writers.
WriterBuddy – Your Ultimate Summary Generator
Welcome to WriterBuddy, where we revolutionize your reading and writing process with our state-of-the-art AI-powered Text Summarizer. Whether you’re summarizing academic papers, creating concise summaries of lengthy articles, or drafting key points for reports, WriterBuddy is designed to make your summarizing process seamless and efficient.
What is Our AI Text Summarizer?
Our AI Text Summarizer is an innovative tool that helps you quickly create accurate, concise, and well-structured summaries based on your specific needs. Leveraging cutting-edge technology in natural language processing (NLP) and machine learning, our tool generates summaries that are indistinguishable from those written by humans.
How Does Our Text Summarizer Work?
Using WriterBuddy’s Text Summarizer is easy and straightforward. Follow these simple steps to generate high-quality summaries in seconds:
Enter Your Text:
- Type or paste the text you want to summarize into the input box.
Select Your Preferences:
- Choose the summary format—bullet points or paragraph format.
Generate Summary:
- Click the “Summarize” button and let our AI do the rest.
Review and Edit:
- Review the generated summary and make any necessary edits to fit your needs.
Key Features of Our AI Summarizer Tool
Cutting-edge technology.
Our AI Summarizer utilizes the most advanced language models to produce summaries that mimic human understanding and interpretation, ensuring the generated content is coherent and engaging without any loss of meaning.
Freemium Tool
Enjoy the majority of our features for free. For those needing advanced capabilities, premium options are available, offering more sophisticated functionalities and customization.
High-Speed Output
WriterBuddy’s Text Summarizer is designed to generate summaries swiftly, allowing you to focus on other critical tasks. Experience high-speed content summarization without compromising on quality.
Variety of Formats
Choose from bullet points or paragraph formats to customize your summaries to suit any context or audience.
Use Cases for WriterBuddy’s AI Text Summarizer
- Content Creation
Perfect for bloggers, marketers, and content creators, our tool helps you produce engaging and informative summaries for blog posts, articles, and social media captions.
- Academic Writing
Students and researchers can generate well-structured summaries for essays, research papers, and dissertations, aiding in the writing process.
- Business Writing
Ideal for drafting business proposals, reports, and emails, our summary generator ensures your communication is clear and professional.
- Creative Writing
Overcome writer’s block and get inspired with unique summaries for your creative projects, including stories, poems, and scripts.
- Technical Documentation
Simplify complex information and create user manuals, product descriptions, and help articles that are easy to understand.
Benefits of Using Our AI Text Summarizer
- Efficiency Boost
WriterBuddy’s Text Summarizer significantly amplifies your productivity. Generate well-structured and coherent summaries with just a few clicks, saving valuable time and effort.
- Accelerated Growth
Generate summaries swiftly, allowing you to focus on more strategic aspects of your business, refining marketing approaches, or exploring new growth avenues.
- Minimized Burnout
Routine summarizing tasks can cause frustration and burnout. The tool makes generating engaging summaries hassle-free, making writing tasks more manageable and less daunting.
Frequently Asked Questions
Can the summarizer tool handle complex or technical language.
Yes, it can. The AI has been trained on a vast dataset, making it adept at handling technical or complex text without issues.
Can the summarizer tool be used on mobile devices, or is it only available on desktops?
The text summarizer is accessible on both desktop and mobile devices, ensuring flexibility and convenience.
How fast can the summarizer tool generate a summary?
Our summary generator can condense long text within seconds, providing you with quick and efficient results.
How much text can the summarizer tool handle at once?
Currently, a maximum of 600 words can be summarized at once. For longer texts, you can paste additional blocks of text consecutively, as there is no overall limit.
Can the summarizer make several versions of the same text, each with a different level of detail?
Yes, you can adjust the summary length to receive either more detailed or shorter summaries according to your preference.
Is the WriterBuddy Text Summarizer free to use?
Yes, our AI Text Summarizer is free to use. Most features are available at no cost, with premium options for advanced needs.
Can I use the Text Summarizer for SEO content?
Absolutely! Our tool generates SEO-optimized content, helping you improve your search engine rankings and reach a wider audience.
What types of summary formats are available?
We offer both bullet points and paragraph formats. Customize your content to match your desired style.
What is text summarization?
Text summarization shortens long text without changing its meaning. This allows anyone to understand the original content without actually reading it. Tools like WriterBuddy’s summary generator, which uses AI technology, can help you create thoughtful summaries in less time.
What are the elements of a summary?
Good summaries all have a few things in common:
- Clarity and Brevity: Be clear and concise. Get to the point quickly.
- Objectivity: Stick to the facts. Avoid personal bias and opinions.
- Focus: Highlight main ideas and omit non-essential details.
- Accuracy: Preserve the original text’s main points while retaining readability.
Available in other languages:
- Español – Resumidor de textos
- French – Résumé de texte
- Indonesian – Ringkasan online
- Filipino – Buod maker tagalog
- Italian – Riassunto online
- Portugues – Gerador de resumo
- German – Zusammenfassung
Stop Stressing, Start Writing
Join over 540,000+ happy users writing smarter with WriterBuddy. Try WriterBuddy for Free!
Advanced AI writing tool trained to write better content faster.
- AI Writing Tools
- Rewording Tool
- Paraphrasing Tool
- Text Summarizer
- Essay Writer
- Essay Rewriter
- Sentence Rephraser
- Sentence Rewriter Tool
- Paragraph Rewrite
- Paragraph Generator
Student Resources
- Plagiarism Checker
- AI Content Detector
- Grammar Checker
- Punctuation Checker
- Spell Checker
- Thesis Generator
- Essay Checker
- Word Counter
- Character Counter
Citation Tools
- Citation Generator
- APA Citation
- MLA Citation
- Citation Checker
- Brand Style Guide
- Affiliate Program
Copyright © 2024 WriterBuddy. All rights reserved.
- Research Guide
- Academic Writing
- Reference Management
- Data Visualization
10 AI Tools for Research Paper Summarization
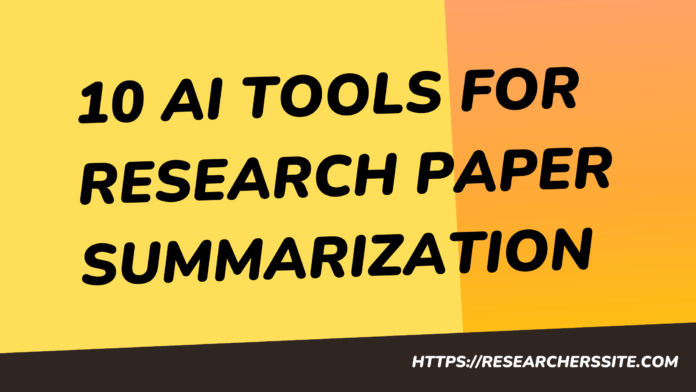
In this blog post, we will explore the 10 best AI-Powered tools for research paper summarization that can enhance your efficiency and productivity in the academic realm.
In the world of academia, academic papers play a crucial role in disseminating knowledge and advancing scientific understanding.
However, with the ever-increasing volume of scientific literatures being published, it has become challenging for researchers to keep up with the vast amount of information available. This is where AI tools for research paper summarization come to the rescue.
The AI tools let you summarize a scientific article in a few sentences or paragraphs, highlight the main points, findings and contributions of the paper.
Best AI Tools for Research Paper Summarization
In this section, we will explore ten remarkable AI-based summarization tools that can simplify the process of summarizing academic papers.
Here are some of the best AI-powered tools for research paper summarization:
#1. SciSummary
SciSummary (short for science summary) is an AI-driven tool designed and developed to provide comprehensive summaries of scientific papers.
The online handy tool uses GPT-3.5 and GPT-4 models to cater summaries of any scientific articles.
In addition to that you can use the free online summarizing tool by sending an email with text, a link, or even attaching a PDF of the article you want to summarize. Within minutes, you will receive a summary in your inbox.
It makes it easy to stay up-to-date with latest scientific breakthroughs and research findings, without having to spend hours reading through long and complicated articles.
#2. SummarizeBot
SummarizeBot is an AI and blockchain-powered tool that can summarize any kind of information for you, including weblinks, documents, images, and more.
The web application uses AI technology to extract the most relevant information from the text and present it in a concise and easy-to-understand format.
Moreover, the tool can summarize a wide range of formats, including text, PDFs, images, and more.
You can customize the length of the summary, so you can get just the information you need.
This summary generator tool is free to use, with no word limits or daily limits.
#3. Copernic Summarizer
Copernic Summarizer is a software application that creates concise document summaries of any file or web page.
It uses sophisticated statistical and linguistic algorithm s to pinpoint the key concepts and extract the most relevant sentences, resulting in a web site or document summary that is a shorter, condensed version of the original text.
In order to use Copernic Summarizer app, simply open the paper that you want to summarize. Then, click on the “Summarize” button.
Copernic Summarizer will then analyze the paper and create a summary. The summary will be displayed in a new window.
Once you are satisfied with the summary, you can copy it to the clipboard or save it to a file.
It is a useful tool for anyone who needs to quickly and easily summarize documents. It is especially useful for researchers who need to read and understand large amounts of information.
Copernic Summarizer lets you summarize a variety of file types, including PDFs, Word documents, text files, and web pages.
Additionally, it can also be used to summarize documents in a variety of languages, including English, French, German, and Spanish.
This AI-based tool is available in both free and paid versions.
#4. Resoomer
Resoomer is an AI-powered online text summarizer tool that can create concise and informative summaries of long documents in a variety of languages.
The Resoomer is available in both a free and paid version.
To use Resoomer, simply paste the content of the paper that you want to summarize into the text box on the Resoomer website. Then, select the language of the text and the desired length of the summary.
Resoomer will then generate a summary and display it in a new window.
It is also integrated with a number of other research tools, such as Google Scholar and Mendeley.
#5. Iris.AI
Iris.AI is an advanced AI-based summarization tool that can effectively condense lengthy scholarly paper into concise summaries.
Iris.AI analyzes the content of research papers or other text inputs and generates abridged summaries that capture the essential information.
With Iris.AI as a summarizer, users can obtain the key points, main ideas, and important findings of a document without having to read the entire text.
This saves time and enables researchers to quickly grasp the core content and extract the necessary insights.
Whether it is a research papers, articles, or other written materials, Iris.AI simplifies the information overload and facilitates efficient information consumption.
Researchers can streamline their reading and research workflow s, enhancing productivity and enabling them to stay up-to-date with a vast amount of information in a more time-efficient manner.
#6. Scholarcy
Scholarcy is an advanced AI-powered tool designed and developed to simplify the process of reading, understanding, and summarizing scientific research papers.
By leveraging AI technology, Scholarcy helps users overcome information overload, save time, and enhance productivity in the research process.
The cutting-edge tool analyzes the content of research papers and generates concise summaries.
Besides, it enables researchers to quickly grasp the key points and main ideas without having to read the entire paper.
Utilizing advanced algorithm s the tool allows you to identify and extract essential information, including key concepts, methodology, and results, providing users with comprehensive summaries.
Its summarization process ensures that the generated summaries are coherent and informative, providing researchers with a clear overview of the original paper.
In a previous post titled “ how to summarize an academic research article (Academic) ” I extensively covered the capabilities and features of the Scholarcy tool.
#7. Paper Digest
Paper Digest is an AI-driven platform that helps researchers stay up-to-date with the latest research.
It is a short summary of an academic article that highlights the main points and core ideas of the paper.
It can help readers to quickly grasp the paper’s contribution and relevance without reading the full text.
With Paper Digest, users can input a research paper or provide a URL, and the tool automatically generates a summary that captures the main findings, methodologies, and key points of the paper.
This allows researchers to quickly grasp the essence of a paper without reading it in its entirety and simplify the scholarly work.
Paper Digest’s summarization process ensures that the generated summaries are accurate, coherent, and provide a comprehensive overview of the original paper.
By condensing lengthy research papers into concise summaries, Paper Digest saves time and enhances the efficiency of literature review and information extraction.
In my earlier post how to generate an automatic summary of research paper , I already elaborately discussed Papper Digest AI-powered tool.
#8. Lynx AI
Lynx AI of SciLynk is also a great AI tool for research paper summarization. It is a free online tool that uses AI to identify the key points of a research paper and generate a summary in a few minutes.
Besides, Lynx AI Summarization offers a number of customization options, such as the ability to select the level of detail and the language of the summary.
Your personal AI research assistant that will summarize search results and key finidings to distill complex concepts at a glance.
As I already mentioned this cutting edge tool uses AI technology to identify the key points of a research paper. This means that the summary will be accurate and relevant to the academic paper.
Lynx AI can generate summaries in a variety of lengths.
The summarizing tool offers a number of customization options. You can control the level of detail and the language of the summary.
Genei is an AI-powered summarization tool that helps you research faster by automatically summarizing background reading, producing articles and reports faster.
Moreover, you can use Genei to import, view, summarize and analyze PDFs and webpages.
Genei seamlessly integrates with popular platforms such as Google Drive, Microsoft OneDrive, and Dropbox.
It also provides features such as notepad and annotation capabilities, citation management and reference generator, multi-document summarization, and more.
The AI-driven tool is revolutionizing the research landscape by offering intelligent summarization.
With its ability to summarize, Genei significantly enhances research efficiency and productivity.
#10. TLDR This
TLDR This is a free online tool that can summarize research papers in a variety of lengths. The tool uses AI to identify the key points of a paper and generate a summary that is easy to read and understand.
The TLDR tool enables you to condense any text into a brief, easily understandable format, allowing you to escape the burden of excessive information.
TLDR This also offers a number of customization options, such as the ability to select the level of detail and the language of the summary.
To obtain a summary of a scholarly paper, either enter the article URL or paste the text into the provided field.
Drawbacks and Constraints of AI Tools for Research Paper Summarization
Here are the drawbacks and constraints of AI tools for research paper summarization:
- Lack of contextual understanding and nuanced interpretation.
- Difficulty in handling complex technical language and domain-specific terms.
- Potential introduction of biases or subjective interpretations.
- Insufficient domain expertise to capture intricate details accurately.
- Challenges in comprehending mathematical equations, diagrams, or visual content.
- Incomplete coverage of key points, findings, or supporting evidence.
Final Thought
In this blog post, we have introduced you to some of the best AI tools for research paper summarization that helps you read and understand scientific article faster.
These tools provide summaries of key sections of the research paper, highlight the main points, findings and contributions of the paper, demystify technical language, contextualize the research and support literature review s.
However, these tools are not perfect and may have limitations or errors in their outputs. Therefore, you should always verify the accuracy and quality of the summaries before using them for your own purposes.
We hope that this blog post has been useful and informative for you. If you have any questions or feedback, please feel free to leave a comment below. Thank you for reading!
RELATED ARTICLES MORE FROM AUTHOR
5 ai-powered platforms for better research collaboration, top ai tools for grant writing and funding applications, ai data analysis tools every researcher needs to know about, citation chasing tools for academic researchers, generative ai meets academia: top academic search engines powered by large language models, top 10 custom gpt tools every academic researcher needs, leave a reply cancel reply.
Save my name, email, and website in this browser for the next time I comment.
EDITOR PICKS
Popular posts, the best 8 ai-powered tools for literature review, how to generate an automatic summary of research paper, popular category.
- AI Tools 41
- Research Guide 21
- Discovery 18
- Reference Management 8
- Academic Writing 7
- Data Visualization 7
- Tutorials 6
- Plagiarism 4
- Privacy Policy
Best AI Tools For Writing Research Papers for 2024

- August 22, 2024 Updated

The future of research writing is here, driven by AI technology that combines precision and efficiency like never before. With the best AI Tools for Writing Research Papers, you can elevate your work to new heights, ensuring that every word is meticulously crafted and every argument is clearly articulated.
Choosing the right AI writing tool is crucial for producing high-quality research papers that meet academic standards . These tools enhance productivity and make sure your work is original, well-structured, and free from errors .
Over the years, AI writing tools have significantly evolved . What started as simple grammar checkers have become sophisticated platforms offering content generation, style optimization, and citation management, making the research writing process more streamlined .
Whether looking for a tool to refine your writing or seeking advanced features for managing citations and references , the best AI writing tools for 2024 provide various options to suit your academic needs . Let’s explore these tools and how they can elevate your research writing.
Best AI Tools For Writing Research Papers: Quick Comparison
In this section, I’ll cover the best AI writing tools designed specifically for Writing Research Papers . These tools are essential for enhancing academic writing by offering features such as grammar checks, content generation, and citation management, ensuring that your research paper is polished and professional.
To help you choose the right tool, I’ve created a quick comparison table that outlines the key features and pricing of the top AI tools available. Depending on your needs, you might find that one of these options is also among the best AI tools in 2024 available for various writing tasks.
4.8/5 | Academic Writing, Research Papers | 200+ words/day | Not Applicable | 5 Languages | Yes | Yes | Not Specified | Not Applicable | Uses Google Cloud, automatic saving | Free plan available | Email, Live Chat | Available | |||
Paperpal | 4.7/5 | Article Writing, Manuscript Writing | Free version; Paid: $19/month | 7,000 words/month | Not Specified | 25+ languages | Yes, up to 7,000 words | Yes | Not Applicable | Web, MS Word add-in | ISO/IEC 27001:2013 certified | Available (limited features) | Money-back guarantee (30 days annual, 7 days monthly) | ||
4.6/5 | Paraphrasing, Research Writing | Unlimited | Not Applicable | 30 languages | Yes | Yes | Not Applicable | Chrome, Microsoft Word, MacOS | Not Applicable | Available | Via message on website | 3-day money-back guarantee | |||
Anyword | 4.5/5 | Research Writing, Academic Writing, Legal Writing | Starter Plan: $49/month, Business Plan: $399/month | Unlimited in all plans | 100+ templates | 30+ languages | Yes | Yes | Not Applicable | Google Chrome extension | Enterprise-grade security | 7-day free trial | No refunds for canceled subscriptions | ||
Bit AI | 4.4/5 | Research Document Collaboration, Knowledge Management | Free Plan: $0/month, Pro Plan: $8/month/member, Business Plan: $15/month/member | 20K AI Words (Pro Plan) | 12 Template categories | Not Specified | Not Specified | Not Specified | Not Applicable | Integrates with 100+ applications | SSL/TLS encryption, Two-factor authentication | Available | Phone, Email, Live Chat | No refunds for canceled subscriptions | |
4.4/5 | Research Writing, Article Ideas, Summaries | 10k free, Unlimited in premium plans | 100+ templates | 24+ languages | Yes | Yes | Yes | Google Docs, WordPress, Twitter, Shopify | TLS1.2+ with AES256 encryption | Available (limited features) | 24/7 Live Chat | 7-day money-back guarantee | |||
Scalenut | 4.4/5 | Research Writing, SEO Optimization, Content Creation | $19-$74/month | 100,000 AI Words (basic), Unlimited in higher plans | 40+ Copywriting Templates | English only | Yes | Yes | Customizable | WordPress, other platforms | May share user data with affiliates | 7-day free trial | Not provided | Not applicable | |
ChatGPT | 4.3/5 | Research Writing, Content Generation, Summarizing Text | Free, $20-$25/month per user | Unlimited | No templates | 50+ languages | No | Yes | Not Applicable | Google Sheets, Google Forms, Zapier | Your data is saved | Available (limited features) | Live Chat | No refunds | |
Research Rabbit | 4.2/5 | Citation-Based Literature Mapping, Research Efficiency | Completely free | Unlimited | Not Applicable | English | Not Applicable | Not Applicable | Not Applicable | Not Specified | Copyrighted Content | Always free | Not applicable | ||
Perplexity AI | 4.0/5 | Real-Time Information Retrieval, Research Writing, Source Verification | Standard: Free, Professional: $20/month | Unlimited | Not Applicable | Inferred from browser settings | No | No | Not Applicable | Chrome extension, email client | Data security details not disclosed | Free plan | Refund within 24 hours, 72 hours for annual subscriptions |
Best AI Tools For Writing Research Papers: In-Depth Analysis
Let’s take a closer look at the best AI tools available for Writing Research Papers . Each tool offers unique features that cater to different aspects of academic writing, from grammar and style to content generation and citation management.
1. Jenni AI : Your Go-To Tool for Research Writing

Jenni AI is a powerful writing assistant designed to streamline research paper writing . With features like AI Autocomplete , in-text citations , and the ability to generate content from various sources, Jenni AI enables you to create well-structured and comprehensive research papers efficiently.
Academic Writing, Research Papers | |
4.8/5 | |
200+ words/day | |
5+ user seats | |
Not Applicable | |
5 Languages supported | |
Yes | |
Yes | |
Not Specified | |
Not Applicable | |
Not Applicable | |
Uses Google Cloud, automatic saving, revision history | |
Free plan available with limited features, no separate free trial | |
Yes | |
Available | |
N/A |
One of the standout features of Jenni AI is its support for five languages , making it accessible to a global audience.
Additionally, the tool allows you to produce 200+ words per day , which is ideal for consistent progress on lengthy academic projects. With 5+ user seats available, Jenni AI is perfect for collaborative research efforts, enabling multiple users to contribute to a single project.
While Jenni AI excels in areas such as multilingual support and ease of use , it does lack a plagiarism checker , which is an important feature for ensuring the originality of academic work. However, its intuitive interface and mobile compatibility make it easy to use, whether you’re working from a desktop or on the go.
Jenni AI shines with its AI Autocomplete and multilingual support , making it an excellent choice for researchers and students alike. However, the absence of a plagiarism checker means you might need to use additional tools to ensure your work is free from unintentional duplication.
- Intuitive and user-friendly interface.
- Strong AI Autocomplete and in-text citation features.
- Supports multiple languages.
- Lacks a built-in plagiarism checker.
- Limited template options.
- Some advanced features are not applicable.
$30/month | |
$12/month |
Customer Support and Refund Policy
Jenni AI offers robust customer support, which is available through various channels, including email and live chat.
The tool also provides a refund policy, though specific details on coverage days are not provided. If you’re considering Jenni AI , it’s worth reading a more detailed Jenni AI review to understand its full capabilities.
2. Paperpal: My Go-To Tool for Research Manuscript Writing

Paperpal is an AI-powered tool explicitly designed for article writing and manuscript writing . It leverages Artificial Intelligence (AI) , Machine Learning (ML) , and Natural Language Processing (NLP) to provide comprehensive writing assistance, from grammar checks to plagiarism detection.
Article Writing, Manuscript Writing | |
4.7/5 | |
Free version; Paid: $19/month | |
7,000 words/month | |
Not specified | |
Not specified | |
25+ languages | |
Yes, up to 7,000 words/month | |
Yes | |
Not applicable | |
AI, ML, NLP | |
Available for web and MS Word add-in | |
ISO/IEC 27001:2013 certified | |
Available (limited features) | |
Available via email | |
Money-back guarantee | |
30 days (annual), 7 days (monthly) |
Paperpal is particularly useful for researchers and authors who need to ensure their manuscripts meet the highest standards of academic writing.
From the moment you log in, Paperpal is intuitive and straightforward to use. It offers a seamless integration with MS Word through an add-in, allowing you to access its features directly within your document.
The tool supports over 25 languages and provides 7,000 words per month of plagiarism-checked content, making it a versatile option for multilingual writers.
However, while Paperpal excels in language support and data security, its limited word count for the free version and lack of detailed template options may be drawbacks for some users.
Paperpal shines with its AI-driven capabilities, offering robust grammar checks and language support. This makes it a great choice for academic and professional writers.
However, the tool is rated slightly lower due to its limited word count in the free version and the absence of extensive template options.
- Strong integration with MS Word.
- Supports over 25 languages, making it versatile for multilingual writing.
- Offers robust grammar and plagiarism checks.
- Limited word count for the free version.
- Lack of detailed templates.
- No clear specification on the number of user seats.
Limited features | |
$19/month |
Paperpal offers customer support via email, ensuring users can get assistance when needed. The tool also provides a money-back guarantee , with 30 days coverage for annual subscriptions and 7 days for monthly subscriptions.
For a deeper insight into Paperpal’s capabilities, consider checking out a comprehensive Paperpal review .
3. QuillBot : My Essential Tool for Paraphrasing and Research Writing

QuillBot has become my go-to tool when paraphrasing text or refining my research writing . It’s particularly effective for rephrasing complex ideas, which is essential when avoiding plagiarism while still accurately conveying my research findings.
Paraphrasing, Research Writing | |
4.6/5 | |
Unlimited | |
50 | |
Not Applicable | |
30 | |
Yes | |
Yes | |
Not Applicable | |
NLP | |
Chrome, Microsoft Word, MacOS | |
Not Applicable | |
Available | |
Via message on the website | |
100% money-back guarantee | |
3 Days |
Whether I’m working on articles or longer academic papers, QuillBot’s range of features helps me maintain clarity and precision.
What I appreciate most about QuillBot is its easy use, from logging in to exploring its various features. It integrates smoothly with Google Docs , Microsoft Word , and MacOS , so I can work across different platforms without hassle.
The tool supports 30 languages and offers unlimited word count, which is useful when working on large research projects.
However, while QuillBot excels in paraphrasing and grammar checking, it doesn’t offer advanced templates, which could be a downside if you need more structured content creation.
QuillBot is invaluable for paraphrasing and ensuring the accuracy of my research writing. However, the lack of advanced templates and a short refund period may be limiting for some users.
- Excellent paraphrasing and grammar-checking features.
- Works well with Google Docs, Microsoft Word, and MacOS.
- It supports 30 languages, making it versatile for multilingual research.
- Doesn’t offer advanced templates for structured academic writing.
- Customer support is limited to website messaging.
- Short refund period of only 3 days.
$19.95/month | |
$13.35/month | |
$99.95/year |
QuillBot offers customer support through messages on their website, which is convenient for quick inquiries.
They also provide a 100% money-back guarantee , but the refund period is only 3 days , so you must decide quickly if it’s the right tool. For a more detailed exploration of QuillBot’s features, I suggest checking out a full QuillBot review .
4. Anyword: Your Precision Tool for Research and Academic Writing

Anyword is a powerful AI-driven writing assistant designed to support various writing needs, particularly research writing , academic content creation , and legal writing .
Research Writing, Academic Writing, Legal Writing | |
4.5/5 | |
Starter Plan: $49/month, Business Plan: $399/month | |
Unlimited in all plans | |
1-3 seats (custom in business plan) | |
100+ templates | |
30+ Languages | |
Yes | |
Yes | |
Not applicable | |
NLP and homegrown predictive performance model | |
Google Chrome extension | |
Enterprise-grade security | |
7-days free trial | |
Available via email | |
No refunds for canceled subscriptions | |
Not applicable |
What makes Anyword especially useful for research writing is its predictive performance model , which offers insights into how effectively your academic content will resonate with its intended audience, ensuring your work makes the desired impact.
When I began using Anyword for my research writing, I was immediately impressed by its user-friendly interface. The tool simplifies the writing process from when you log in, offering seamless navigation through its features.
With support for 30+ languages and 100+ templates , Anyword is versatile enough to handle complex research documents, ensuring they are both clear and compelling.
The integration with Google Chrome is particularly handy, allowing me to draft and refine my research papers directly in the browser.
However, while Anyword excels in predictive content performance and template variety, its higher pricing tiers and the absence of refunds for canceled subscriptions may limit some users, particularly those in academia, on a budget.
Anyword excels in providing content analytics and predictive performance , making it an excellent choice for researchers and academics who require data-driven insights to enhance the effectiveness of their writing.
However, the tool’s lack of a refund policy and the higher costs associated with advanced plans may be a concern for budget-conscious users in academia.
- Predictive performance scores provide valuable insights for optimizing research papers.
- It offers a wide range of templates and supports over 30 languages , which is useful for multilingual research.
- Smooth integration with Google Chrome for efficient browser-based writing.
- Higher pricing tiers , particularly for small research teams or students, can be expensive.
- There are no refunds for canceled subscriptions, which might be a drawback for some users.
- Advanced features like custom-built AI models are only available in the business plan .
$49/month | |
$399/month |
Anyword offers customer support via email, which I find effective for quickly resolving issues during my research writing. However, it’s important to note that they do not offer refunds for canceled subscriptions, so it’s wise to fully assess your needs before committing to a plan.
For a more detailed comparison with other AI writing tools, I recommend reading a thorough Anyword review to see if it aligns with your research writing requirements.
5. Bit AI: The Ultimate Tool for Research Document Collaboration

But AI is cloud-based document collaboration and knowledge management software , making it ideal for researchers and academic teams that need to create, share, and track their research documents in real time .
Research Document Collaboration, Knowledge Management | |
4.4/5 | |
Free Plan: $0/month, Pro Plan: $8/month/member, Business Plan: $15/month/member | |
20K AI Words in Pro Plan (Addon: $10/100K words) | |
Up to 5 members in Free Plan, Unlimited in Paid Plans | |
Up to 12 Template categories | |
Not Specified | |
Not Specified | |
Not Specified | |
Not Applicable | |
AI Genius Writing Assistant | |
Integrates with 100+ applications | |
SSL/TLS encryption, Two-factor authentication | |
Available | |
Phone, Email, Live Chat | |
No refunds for canceled subscriptions | |
Not applicable |
It streamlines document collaboration, enhances knowledge sharing , and significantly boosts productivity , making it indispensable for teams working on research papers , proposals , and academic reports .
When I first started using Bit AI for my research writing, I was immediately impressed by its intuitive interface and ease of use .
The tool integrates seamlessly with over 100 popular applications , which allows me to embed various types of digital content directly into my documents.
This feature is incredibly useful for creating rich, interactive research papers that go beyond text to include images, videos, and other media.
I also appreciate Bit AI’s mobile compatibility , which enables me to manage my research documents on the go.
However, while Bit AI excels in collaboration features and integration , the limitations of the free plan and the lack of advanced grammar and plagiarism checks might be drawbacks for some researchers.
Bit AI is particularly strong in document collaboration and knowledge management , making it a great choice for academic teams that require real-time collaboration on research papers. However, its limited free plan and the absence of specified grammar and plagiarism detection features may be restrictive for researchers who need comprehensive writing tools.
- Extensive integration with over 100 applications enhances document functionality, ideal for embedding research data and media.
- It offers comprehensive knowledge management and collaboration tools that are perfect for managing complex research projects.
- Mobile compatibility allows for document management on the go, ensuring you can keep up with your research tasks anywhere.
- The free plan is limited , especially for larger research teams needing more advanced features.
- Lacks specified grammar check and plagiarism detection features, which are crucial for academic integrity.
- There are no refunds for canceled subscriptions, which could be a drawback for some researchers.
$0/month | |
$8/month per member | |
$15/month per member |
Bit AI offers customer support via phone, email, and live chat, which I find very responsive and helpful, particularly when managing complex research documents.
However, it’s important to note that Bit AI does not offer refunds for canceled subscriptions, so it’s essential to consider this before committing to a paid plan.
6. Writesonic : Your Go-To AI for Research Writing and Content Creation

Writesonic is a versatile AI-powered writing assistant designed to enhance research writing and content creation efficiency and quality .
Research Writing, Article Ideas, Summaries | |
4.4/5 | |
10k in free, Unlimited in premium plans | |
1-Unlimited | |
100+ Templates | |
24+ Languages | |
Yes | |
Yes | |
Yes | |
Generative AI models | |
Google Docs, WordPress, Twitter, Shopify, and more | |
TLS1.2+ with AES256 encryption | |
Available with limited features | |
24/7 Via Live Chat | |
100% refund | |
7 Days |
Whether you’re brainstorming article ideas , crafting concise summaries , or generating SEO meta-descriptions for your research publications, Writesonic provides various tools tailored to meet your academic needs.
When I first started using Writesonic , I found the platform incredibly intuitive and easy to navigate.
The user experience is seamless, from logging in to exploring its features. The tool supports 24+ languages and offers access to over 100+ templates , making it ideal for creating structured and polished research documents.
Additionally, Writesonic’s integration with platforms like Google Docs and WordPress simplifies incorporating research content into various formats.
However, while Writesonic excels in generating high-quality content, some users might find the limited credits in the free plan restrictive, especially when working on extensive research projects.
Writesonic is particularly strong in content generation and language support , making it an excellent tool for researchers who must produce well-structured and engaging academic content.
However, the limited credits in the free plan and the need for a premium plan to access unlimited features may be a consideration for those working on larger projects.
The best AI for writing research papers helps streamline the writing process with advanced features.
- Extensive template library with over 100 templates, perfect for various research writing tasks.
- It supports 24+ languages , making it suitable for multilingual research teams.
- Seamless integration with popular platforms like Google Docs and WordPress.
- Limited credits in the free plan may restrict usage for extensive research projects.
- Premium plans require unlimited access to word count and other advanced features.
- Some advanced features, such as brand voice , are better suited for marketing than academic writing.
$12-$25 annually | |
$15-$30 monthly |
Writesonic offers 24/7 customer support via live chat, which I find particularly helpful when working on research projects that require quick assistance.
They also offer a 100% refund within 7 days , which provides peace of mind when trying out the platform.
If you want to explore how Writesonic compares to other AI writing tools for research, consider reading a comprehensive Writesonic review to see if it fits your academic needs.
7. Scalenut: Elevate Your Research Writing with AI-Driven Content Optimization

Scalenut is a comprehensive AI-driven writing assistant that excels at research writing , especially content planning , SEO optimization , and content creation .
Research Writing, SEO Optimization, Content Creation | |
4.4/5 | |
$19-$74/month | |
100,000 AI Words in basic plans; Unlimited in higher plans | |
Up to 2 in basic plans, customizable in higher plans | |
40+ Copywriting Templates | |
English only | |
Yes | |
Yes | |
Customizable as per input | |
Deep learning model, NLP-generated key terms | |
Integrates with WordPress and other platforms | |
May share certain user data with affiliates | |
7-day free trial | |
Not provided | |
Not applicable |
Designed to help researchers create impactful and optimized content, Scalenut’s capabilities extend beyond writing. It also offers tools to research SEO topics and analyze traffic , making it an all-in-one platform for academic and research professionals aiming to increase their online visibility.
From the moment I started using Scalenut , I was struck by how user-friendly it is.
The platform makes it easy to log in and craft well-structured research content with its intuitive interface.
One of the standout features is its deep learning model and NLP-generated key terms , significantly enhancing the quality and relevance of your research writing.
Moreover, Scalenut’s integration with WordPress ensures you can directly publish your optimized content without any hassles.
However, while Scalenut offers extensive features, its English-only language support and potential data-sharing practices might be limiting factors for some researchers.
Scalenut is particularly effective in content optimization and SEO-driven research writing , making it a top choice for researchers who want to enhance the reach and impact of their work. However, the tool’s English-only support and potential data-sharing practices may not suit everyone, particularly those working in multilingual or highly confidential research environments.
- AI-driven content optimization with deep learning models enhances the quality and reach of research papers.
- It provides over 40+ templates for various writing tasks, which is ideal for structured academic writing.
- Integration with WordPress allows for seamless publishing of research content.
- English-only language support may be limiting for non-English researchers.
- Potential data-sharing practices might raise concerns for those handling sensitive research data.
- Customer support options are not clearly provided , which could be a drawback if you need immediate assistance.
$19-$74/month |
Scalenut offers a 7-day free trial for users to explore its features, which I found useful for getting acquainted with the platform. However, the tool does not clearly provide customer support options, and there is no refund policy mentioned, so it’s important to consider these factors before subscribing.
For a more detailed comparison with other AI writing tools, I recommend reading a comprehensive Scalenut review to see if it aligns with your research writing needs.
8. ChatGPT: Your Essential AI for Research Writing and Content Generation

ChatGPT , developed by OpenAI, is a powerful AI-driven writing assistant that has quickly become a go-to tool for research writing and content generation .
Research Writing, Content Generation, Summarizing Text | |
4.3/5 | |
Free and $20-$25 per user/month | |
Unlimited words | |
2 or more users | |
No templates | |
50+ languages | |
No | |
Yes | |
Not Applicable | |
Large Language Models (LLM) | |
Google Sheets, Google Forms, Zapier | |
Your data is saved | |
Available with limited features | |
Available via live chat | |
Does not offer refunds | |
Not Applicable |
Whether you’re drafting complex research papers, summarizing intricate topics, or translating documents, ChatGPT offers a versatile platform that can significantly enhance your academic work.
With the ability to handle large language models (LLMs) , ChatGPT is particularly adept at generating high-quality content on diverse and complex topics, making it an invaluable tool for researchers.
When I started using ChatGPT for my research writing, I found the platform incredibly intuitive and easy to navigate.
The tool supports 50+ languages , making it highly adaptable to various research tasks. The mobile compatibility on iOS and Android further ensures that I can work on my research projects anywhere, anytime.
However, while ChatGPT excels in generating and summarizing content, the lack of a built-in plagiarism check and limited advanced grammar features may require additional tools to ensure the academic integrity of your work.
ChatGPT stands out for its ability to handle complex research topics and generate comprehensive content, making it a top choice for academics and researchers.
However, the tool’s lack of integrated plagiarism detection and the absence of refunds could be a consideration for those needing these specific features.
These Best AI tools for research in 2024 provide top-notch support for academic work.
- Extensive language support with over 50 languages, making it suitable for multilingual research.
- Access on multiple platforms (web, iOS, Android), ensuring flexibility in research writing.
- Offers a vast array of templates and tools , including access to GPT-4 and other advanced AI capabilities.
- No built-in plagiarism checker , which may require the use of additional tools for academic integrity.
- Limited advanced grammar features , making it less comprehensive compared to dedicated grammar tools.
- No refunds for subscriptions, which could be a drawback for some users.
$0/month | |
$20-$25/month per user |
ChatGPT offers customer support via live chat, which I find helpful for quick queries and troubleshooting.
However, it’s important to note that ChatGPT does not offer refunds for canceled subscriptions, so it’s crucial to evaluate the tool’s features thoroughly during the free trial.
To dive deeper into how ChatGPT compares to other AI tools, consider reading a comprehensive ChatGPT review to determine if it fits your research writing needs.
9. Research Rabbit: Your Go-To Tool for Efficient Research and Literature Mapping

Research Rabbit is a unique, citation-based literature mapping tool designed to optimize your time when searching for references, whether you’re starting an essay, a minor project, or a comprehensive literature review.
Citation-Based Literature Mapping, Research Efficiency | |
4.2/5 | |
Completely free for researchers | |
Unlimited | |
Not Applicable | |
Not Applicable | |
English | |
Not Applicable | |
Not Applicable | |
Not Applicable | |
Optimized Keyword Search | |
Not Specified | |
Copyrighted Content | |
Always free | |
Via email at [email protected] | |
Not Applicable | |
Not Applicable |
This free tool is invaluable for researchers who want to streamline their research process by finding relevant papers quickly and efficiently.
When I first used Research Rabbit for my research writing, I was impressed by how it simplifies the literature review process.
The tool’s ability to search for relevant literature based on a “seed paper” concept is a game-changer. It allows me to input one or more research papers and receive suggestions for additional relevant papers.
This eliminates the need to switch between various databases, making the research process faster and more efficient.
Additionally, Research Rabbit is continuously evolving, with a mission-first team dedicated to advancing human knowledge.
The platform is easy to navigate, but it is primarily focused on literature mapping and lacks more advanced content generation features like those found in ChatGPT .
Research Rabbit excels in citation-based literature mapping , making it a must-have tool for any researcher looking to optimize their literature review process.
However, it is specialized in this area and does not offer broader content generation or editing features, which could limit its utility for those needing a more comprehensive tool like ChatGPT .
Free AI tools for research papers writing offer valuable resources for generating high-quality content.
- Free access to a powerful citation-based literature mapping tool, making it accessible to all researchers.
- Efficient literature searching through the “seed paper” concept saves significant time during the research process.
- The mission-first team focused on advancing human knowledge and continuously improving the tool.
- Limited to literature mapping , lacking broader content creation or editing features.
- English-only support may limit its use for non-English research materials.
- Does not offer advanced integrations or additional research tools in more comprehensive platforms.
$0 |
Research Rabbit offers customer support via email at [email protected] , which I found responsive and helpful. As the tool is completely free, there is no refund policy, which is understandable given its no-cost model.
To complement your research toolkit with broader capabilities, consider exploring other tools like ChatGPT to see how they can work alongside Research Rabbit.
10. Perplexity AI: Your Real-Time Research Assistant for Accurate Information Retrieval
Perplexity AI is a cutting-edge AI-powered research assistant designed to provide real-time information retrieval and fact-checking, making it an ideal tool for research writing .

Real-Time Information Retrieval, Research Writing, Source Verification | |
4.0/5 | |
Standard: Free, Professional: $20/month | |
Unlimited | |
Not specified | |
Not Applicable | |
Inferred from browser settings | |
No | |
No | |
Not Applicable | |
NLP algorithms, Machine Learning models | |
Supports Chrome extension, email client | |
Prioritizes data security; details not disclosed | |
The standard plan is free | |
Via email at [email protected] | |
Eligible for a refund within 24 hours, full refund within 72 hours for annual subscriptions | |
72 hours for annual subscriptions |
Whether you’re conducting in-depth research on complex topics or need quick access to accurate and up-to-date information, Perplexity AI’s real-time web search and transparent source citation capabilities ensure that you get the most relevant and trustworthy results for your research projects.
When I began using Perplexity AI for my research writing, I was immediately struck by how efficient and user-friendly the platform is.
The Perplexity Copilot feature integrates real-time web searches with transparent citations, providing reliable sources for every answer, which is crucial for academic integrity.
The interface is clean and intuitive, allowing me to focus on my research without distractions.
Perplexity AI is particularly strong in real-time information retrieval and source transparency , making it an excellent tool for researchers who need accurate and trustworthy data quickly.
However, the platform’s lack of advanced grammar or plagiarism checking features and limited information on data security may require users to complement it with other tools for a more comprehensive research writing process.
- Real-time web search provides up-to-date information crucial for current research topics.
- Transparent source citation enhances academic integrity, ensuring that all information is traceable.
- A User-friendly interface allows for a distraction-free research experience.
- No built-in plagiarism or grammar checks , which may require additional tools.
- Limited data security details which could be a concern for sensitive research.
- The Professional plan may be necessary for more advanced features, which adds to the cost.
Free | |
$20/month |
Perplexity AI offers customer support via email at [email protected] , which is responsive and helpful for addressing user inquiries.
The platform provides a 24-hour window for refunds after purchase, with a full refund available within 72 hours for annual subscriptions, making it relatively risk-free to try.
For a more detailed comparison with other research tools, consider exploring a comprehensive Perplexity AI review to see how it fits into your research writing workflow.
How To Choose The Best AI Tools For Writing Research Papers?
When I set out to find the best AI tools for writing research papers, I focused on several key factors:
- Content Accuracy and Credibility : The tool’s ability to generate well-researched and accurate content is crucial for producing credible research papers.
- Language Capabilities : It was essential to choose AI tools that support a wide range of languages, ensuring they can assist with research papers in different regional contexts.
- Integration Capabilities : I looked for AI tools that seamlessly integrate with platforms like Google Docs or WordPress, enhancing the writing process and allowing for smooth transitions between the writing and editing stages.
- Data Security and Privacy : Ensuring the AI tool complies with data protection standards is vital, particularly for researchers handling sensitive academic work, especially in regions with strict data privacy regulations.
This detailed guide on artificial intelligence provides more insights into how I evaluate AI tools and what factors to consider. Moreover, the best AI tool for writing research papers in 2024 meets the demands of modern academic writing.
How do AI Writing Tools Help Write Research Papers?
In my experience, AI writing tools have greatly enhanced my ability to produce high-quality research papers by offering several key benefits:
- Efficiency : AI tools speed up tasks like organizing references and generating literature reviews, allowing more time for analysis and critical thinking.
- Accuracy : These tools improve grammar, sentence structure, and clarity by leveraging natural language processing (NLP), ensuring professional-quality writing.
- Data Analysis : AI can quickly scan and summarize large volumes of data, making it easier to identify trends and build stronger research arguments.
- Collaboration : Many AI tools support real-time collaboration, enabling seamless teamwork across different regions, especially useful for international research projects.
For more on how AI tools enhance research writing, understanding the basics of NLP (Natural Language Processing) can provide deeper insights into these advancements.
Want to Read More? Explore Best AI Writing Tools Guides!
Elevate your writing skills with our expertly curated guides on AI writing tools.
- Best AI Writing Tools for Romance Writing for 2024 : Discover the top AI writing tools designed to elevate your romance writing to new heights.
- Best AI Writing Tools For Comedy Scripts for 2024 : Find the best AI writing tools to help you create funny and engaging comedy scripts.
- Best AI Tools For Writing News Articles for 2024 : Explore the best AI tools to assist in writing clear and accurate news articles.
- Best AI Tools For Writing Speeches for 2024 : Discover the top AI tools to help you craft compelling and effective speeches.
- Best AI Tools for Writing Book Reviews for 2024 : Find the best AI tools to help you write insightful and well-crafted book reviews.
Can I use AI to write my research paper?
What is the best ai for summarizing research papers, is there a completely free ai writing tool, can professors detect ai-written papers, can chatgpt summarize a research paper.
Choosing the best AI Tools for writing Research Papers involves considering content accuracy, language capabilities, integration options, and data security. AI tools like ChatGPT, Quillbot, and Research Rabbit offer unique features that cater to different aspects of the research writing process, making them valuable assets for academics.
You should explore these AI writing tools to enhance your content writing projects, whether you’re working on a research paper, summarizing literature, or managing complex academic tasks. By integrating these tools into your workflow, you can improve both the efficiency and quality of your writing.

Digital marketing enthusiast by day, nature wanderer by dusk. Dave Andre blends two decades of AI and SaaS expertise into impactful strategies for SMEs. His weekends? Lost in books on tech trends and rejuvenating on scenic trails.

Best AI Tools for Academic Research

10 Best AI Video Tools for Content Curation in USA for 2024

10 Best AI Video Tools for Beginners for 2024

10 Best AI Image Generator for Mac in 2024 for American Users
Leave a reply cancel reply.
Your email address will not be published. Required fields are marked *
Save my name, email, and website in this browser for the next time I comment.
AI tools for literature searching
Overview of ai tools for literature searching, selected tools, how to use these tools safely.
There are many new AI tools available to researchers and students which focus on academic sources (rather than general AI tools such as Copilot or ChatGPT). These tools offer a powerful new method of discovering academic literature and can be a great complement to traditional methods of searching the literature in your field.
For thorough or comprehensive searching such as for a research paper or thesis, you should still search the major academic databases in your field . These AI tools use freely available or negotiated underlying datasets. These datasets do not necessarily contain the important journals in any field, however academic databases are produced specifically to provide this coverage. You can find the databases in your field in our Subject Guides .
Some of the more popular tools you might like to explore are below. Note that there are many more, and new developments are happening all the time. You can search the web to investigate what is available.
An account is required to use Elicit. There is a free basic plan, and paid plans for extra features and capabilities | . Elicit can base answers on abstracts, plus the full text of open access papers | Enter your topic or question in natural language | |
Scite requires a login and payment to use Scite Assistant, or to see the full Scite Search results | Agreements with . | Scite has two main components. Scite Search lets you explore citation links between papers, and whether citations are positive, negative etc. Scite Assistant is the AI research assistant | |
You can use SciSpace without creating an account. There is a free basic plan, and paid plans for extra features and capabilities | Unknown | Enter your topic or question in natural language | |
You can search Consensus without creating an account, although certain features do require an account. There is a free basic plan, and paid plans for extra features and capabilities | Enter your topic or question in natural language. Turning the Copilot feature on enables you to give conversational commands such as 'Write a 3 paragraph literature review on...' or 'Give me a two sentence summary in simple language on...' | ||
You can use Keenious without creating an account. There is a free basic plan, and paid plans for extra features and capabilities | Keenious offers you recommendations of papers and topics, based on the text you're writing. You can write straight into the box on the Keenious website, or add the Keenious addon into Word or Google Docs on your personal device |
- Use these tools as an extra technique for research and not to replace a thorough search.
- Verify any information provided by generative AI tools with credible sources and check for missing information.
- Note that most of these tools do not have access to the full text of papers - they base their answers on titles and abstracts only. You should always access, read and cite the full papers that you use in your work.
- Don't upload materials from Library resources such as databases. This is in breach of copyright, and license agreements
- Don't upload your own sensitive or private documents. It's possible another user of the tool might retrieve your information from the underlying software
- You should acknowledge your use of AI tools to complete your work. See our guide on using Generative AI tools for assignments for more information.
- Last Updated: Aug 26, 2024 11:33 AM
- URL: https://guides.library.uq.edu.au/research-techniques/ai-tools-for-literature-searching

CENTER FOR ADVANCING SAFETY OF MACHINE INTELLIGENCE A collaboration between Northwestern University and UL Research Institutes
The ai summarization dilemma: when good enough isn’t enough.
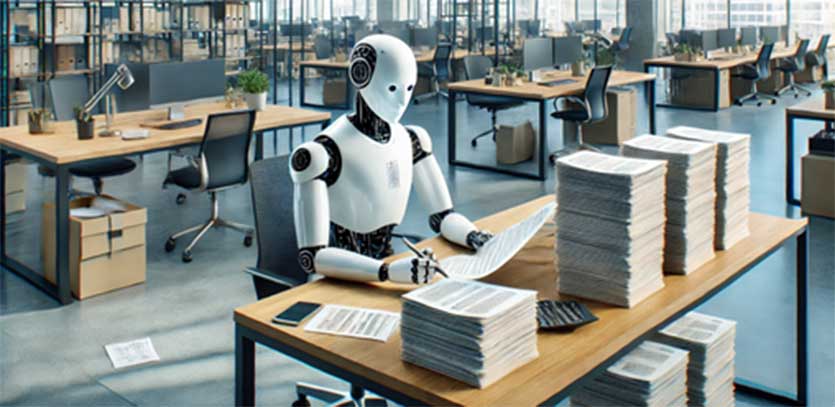
A recent article in The Intelligencer highlighted a growing concern with AI summarizations, particularly the fact that these models aren’t perfect. For instance, when summarizing your email, a model might get details like a date or location wrong. These seemingly minor errors can have significant consequences, especially when we rely on AI to handle information that we would otherwise read ourselves.
The primary purpose of AI summarization is to help us manage the overwhelming amount of information we’re inundated with daily. It’s for those documents, emails, and notifications that you don’t want to read but still need to keep track of. The problem is that if you’re using AI because you don’t want to do the reading, you’re also unlikely to catch its mistakes. This isn’t just a minor flaw; it’s a task issue. If you need something to be 100% correct, AI summarization isn’t the tool for the job. However, in cases where being 90% right is acceptable, these tools can be incredibly effective and can significantly streamline workflows.
Consider the situation in Wyoming, where mayoral candidate Victor Miller proposed using AI as the real mayor. His idea was that AI could read and summarize legislation for him. The point was, he didn’t want to read the documents himself, meaning that there would be no checks on the system and its accuracy. In the end, voters decided they preferred human candidates in the primary race, rejecting the notion of an AI mayor.
This brings us to a crucial question: What level of accuracy are we comfortable with? In some contexts, being mostly right is good enough—brainstorming ideas, for instance. But in others, the consequences of errors can be dire. Imagine AI summarizing a conversation between two doctors discussing a patient. If it gets the core semantics right, that’s fantastic. But what if it mixes up drug names or diseases? The implications could be catastrophic.
Consider a car analogy. If your car’s windows malfunctioned 1% of the time, it might be annoying, but you could live with it. However, if your brakes failed 1% of the time, that would be unacceptable—potentially fatal. The same applies to AI summarization: it all depends on the nature of that task and the potential impact of failure within that context.
While AI summarization can be a powerful tool in managing information overload, we need to carefully consider when and where it’s appropriate to use. The stakes are too high to leave everything to chance—or to a machine.
Kristian Hammond Bill and Cathy Osborn Professor of Computer Science Director of the Center for Advancing Safety of Machine Intelligence (CASMI) Director of the Master of Science in Artificial Intelligence (MSAI) Program
More Reactions: Read All Notes on the News
Information
- Author Services
Initiatives
You are accessing a machine-readable page. In order to be human-readable, please install an RSS reader.
All articles published by MDPI are made immediately available worldwide under an open access license. No special permission is required to reuse all or part of the article published by MDPI, including figures and tables. For articles published under an open access Creative Common CC BY license, any part of the article may be reused without permission provided that the original article is clearly cited. For more information, please refer to https://www.mdpi.com/openaccess .
Feature papers represent the most advanced research with significant potential for high impact in the field. A Feature Paper should be a substantial original Article that involves several techniques or approaches, provides an outlook for future research directions and describes possible research applications.
Feature papers are submitted upon individual invitation or recommendation by the scientific editors and must receive positive feedback from the reviewers.
Editor’s Choice articles are based on recommendations by the scientific editors of MDPI journals from around the world. Editors select a small number of articles recently published in the journal that they believe will be particularly interesting to readers, or important in the respective research area. The aim is to provide a snapshot of some of the most exciting work published in the various research areas of the journal.
Original Submission Date Received: .
- Active Journals
- Find a Journal
- Proceedings Series
- For Authors
- For Reviewers
- For Editors
- For Librarians
- For Publishers
- For Societies
- For Conference Organizers
- Open Access Policy
- Institutional Open Access Program
- Special Issues Guidelines
- Editorial Process
- Research and Publication Ethics
- Article Processing Charges
- Testimonials
- Preprints.org
- SciProfiles
- Encyclopedia
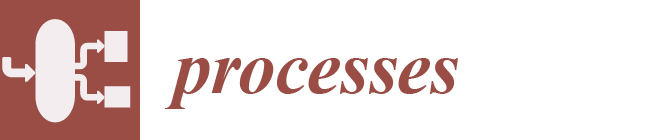
Article Menu
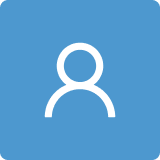
- Subscribe SciFeed
- Recommended Articles
- Google Scholar
- on Google Scholar
- Table of Contents
Find support for a specific problem in the support section of our website.
Please let us know what you think of our products and services.
Visit our dedicated information section to learn more about MDPI.
JSmol Viewer
Application of artificial intelligence in the management of coagulation treatment engineering system.

1. Introduction
2. concepts and principles, 2.1. machine learning, 2.2. deep learning, 2.3. transfer learning, 3. research statistics and analysis, 4. application of neural networks in coagulation, 4.1. coagulant dosing control system, 4.1.1. automatic coagulant dosing control modes, 4.1.2. coagulant dosing optimization, 4.1.3. multiple model comparison, 4.1.4. parameter control, 4.2. prediction and analysis of contaminants, 4.2.1. monitoring of inlet and outlet water, 4.2.2. image processing of inlet and outlet water, 5. challenges and prospects, 5.1. optimization of coagulation neural network models, 5.1.1. improvement in data quality and quantity, 5.1.2. feature engineering, 5.1.3. model selection, 5.1.4. cross-validation, 5.1.5. continuous monitoring and optimization, 5.2. various challenges in neural networks for coagulation, 5.2.1. information structure issues, 5.2.2. feedback control signal acquisition and feedback mechanism, 5.2.3. transfer learning challenges, 5.2.4. real production challenges, 5.2.5. analysis of cost control issues, 5.2.6. application of neural network in drinking water treatment, 6. conclusions, author contributions, conflicts of interest, abbreviations.
AI | Artificial Intelligence |
ANN | Artificial Neural Network |
FNN | Feed Forward Neural Network |
RNN | Recurrent Neural Network |
CNN | Convolutional Neural Network |
MLP | Multi-layer Perceptron |
BP | Back Propagation |
ANFIS | Artificial Fuzzy Neural Network |
RBF | Radial Basis Function |
RBNN | Radial Basis Neural Network |
MMPC | Multi-model Predictive Control |
LSTM | Long Short-Term Memory |
PSO | Particle Swarm Optimization |
DCNN | Deep Convolution Neural Network |
RSM | Response Surface Methodology |
ENN | Edited Nearest Neighbors |
- Ray, S.S.; Verma, R.K.; Singh, A.; Ganesapillai, M.; Kwon, Y.-N. A holistic review on how artificial intelligence has redefined water treatment and seawater desalination processes. Desalination 2023 , 546 , 116221. [ Google Scholar ] [ CrossRef ]
- Qu, J.; Yin, C.; Yang, M.; Liu, H. Development and application of innovative technologies for drinking water quality assurance in China. Front. Environ. Sci. Eng. China 2007 , 1 , 257–269. [ Google Scholar ] [ CrossRef ]
- Teodosiu, C.; Gilca, A.-F.; Barjoveanu, G.; Fiore, S. Emerging pollutants removal through advanced drinking water treatment: A review on processes and environmental performances assessment. J. Clean. Prod. 2018 , 197 , 1210–1221. [ Google Scholar ] [ CrossRef ]
- Mian, H.R.; Chhipi-Shrestha, G.; Hewage, K.; Rodriguez, M.J.; Sadiq, R. Predicting unregulated disinfection by-products in small water distribution networks: An empirical modelling framework. Environ. Monit. Assess. 2020 , 192 , 497. [ Google Scholar ] [ CrossRef ] [ PubMed ]
- Li, S.; Zhu, G.; Li, X.; Wan, P.; Yuan, F.; Xu, S.; Hursthouse, A.S. Ecosystem-inspired model and artificial intelligence predicts pollutant consumption capacity by coagulation in drinking water treatment. Environ. Chem. Lett. 2023 , 21 , 2499–2508. [ Google Scholar ] [ CrossRef ]
- Ribau Teixeira, M.; Rosa, S.M.; Sousa, V. Natural organic matter and disinfection by-products formation potential in water treatment. Water Resour. Manag. 2011 , 25 , 3005–3015. [ Google Scholar ] [ CrossRef ]
- Lichtfouse, E.; Morin-Crini, N.; Bradu, C.; Boussouga, Y.-A.; Aliaskari, M.; Schäfer, A.I.; Das, S.; Wilson, L.D.; Ike, M.; Inoue, D. Methods for selenium removal from contaminated waters: A review. Environ. Chem. Lett. 2022 , 20 , 2019–2041. [ Google Scholar ] [ CrossRef ]
- Vardhan, K.H.; Kumar, P.S.; Panda, R.C. A review on heavy metal pollution, toxicity and remedial measures: Current trends and future perspectives. J. Mol. Liq. 2019 , 290 , 111197. [ Google Scholar ] [ CrossRef ]
- Qu, J.; Fan, M. The current state of water quality and technology development for water pollution control in China. Crit. Rev. Environ. Sci. Technol. 2010 , 40 , 519–560. [ Google Scholar ] [ CrossRef ]
- Bian, Y.; Xiong, N.; Zhu, G. Technology for the remediation of water pollution: A review on the fabrication of metal organic frameworks. Processes 2018 , 6 , 122. [ Google Scholar ] [ CrossRef ]
- Inyinbor Adejumoke, A.; Adebesin Babatunde, O.; Oluyori Abimbola, P.; Adelani Akande Tabitha, A.; Dada Adewumi, O.; Oreofe Toyin, A. Water pollution: Effects, prevention, and climatic impact. Water Chall. Urban. World 2018 , 33 , 33–47. [ Google Scholar ]
- Sathya, K.; Nagarajan, K.; Carlin Geor Malar, G.; Rajalakshmi, S.; Raja Lakshmi, P. A comprehensive review on comparison among effluent treatment methods and modern methods of treatment of industrial wastewater effluent from different sources. Appl. Water Sci. 2022 , 12 , 70. [ Google Scholar ] [ CrossRef ] [ PubMed ]
- Cai, H.; Mei, Y.; Chen, J.; Wu, Z.; Lan, L.; Zhu, D. An analysis of the relation between water pollution and economic growth in China by considering the contemporaneous correlation of water pollutants. J. Clean. Prod. 2020 , 276 , 122783. [ Google Scholar ] [ CrossRef ]
- Gadipelly, C.; Pérez-González, A.; Yadav, G.D.; Ortiz, I.; Ibáñez, R.; Rathod, V.K.; Marathe, K.V. Pharmaceutical industry wastewater: Review of the technologies for water treatment and reuse. Ind. Eng. Chem. Res. 2014 , 53 , 11571–11592. [ Google Scholar ] [ CrossRef ]
- Mondejar, M.E.; Avtar, R.; Diaz, H.L.B.; Dubey, R.K.; Esteban, J.; Gómez-Morales, A.; Hallam, B.; Mbungu, N.T.; Okolo, C.C.; Prasad, K.A. Digitalization to achieve sustainable development goals: Steps towards a Smart Green Planet. Sci. Total Environ. 2021 , 794 , 148539. [ Google Scholar ] [ CrossRef ]
- Gupta, A.D.; Pandey, P.; Feijóo, A.; Yaseen, Z.M.; Bokde, N.D. Smart water technology for efficient water resource management: A review. Energies 2020 , 13 , 6268. [ Google Scholar ] [ CrossRef ]
- Gunasekaran, K.; Boopathi, S. Artificial Intelligence in Water Treatments and Water Resource Assessments. In Artificial Intelligence Applications in Water Treatment and Water Resource Management ; IGI Global: Hershey, PA, USA, 2023; pp. 71–98. [ Google Scholar ]
- Zhang, Y.; Gao, X.; Smith, K.; Inial, G.; Liu, S.; Conil, L.B.; Pan, B. Integrating water quality and operation into prediction of water production in drinking water treatment plants by genetic algorithm enhanced artificial neural network. Water Res. 2019 , 164 , 114888. [ Google Scholar ] [ CrossRef ]
- Azimi, S.; Moghaddam, M.A.; Monfared, S.H. Prediction of annual drinking water quality reduction based on Groundwater Resource Index using the artificial neural network and fuzzy clustering. J. Contam. Hydrol. 2019 , 220 , 6–17. [ Google Scholar ] [ CrossRef ]
- Godo-Pla, L.; Emiliano, P.; Valero, F.; Poch, M.; Sin, G.; Monclús, H. Predicting the oxidant demand in full-scale drinking water treatment using an artificial neural network: Uncertainty and sensitivity analysis. Process Saf. Environ. Prot. 2019 , 125 , 317–327. [ Google Scholar ] [ CrossRef ]
- Peleato, N.M.; Legge, R.L.; Andrews, R.C. Neural networks for dimensionality reduction of fluorescence spectra and prediction of drinking water disinfection by-products. Water Res. 2018 , 136 , 84–94. [ Google Scholar ] [ CrossRef ]
- Halali, M.A.; Azari, V.; Arabloo, M.; Mohammadi, A.H.; Bahadori, A. Application of a radial basis function neural network to estimate pressure gradient in water–oil pipelines. J. Taiwan Inst. Chem. Eng. 2016 , 58 , 189–202. [ Google Scholar ] [ CrossRef ]
- Gadekar, M.R.; Ahammed, M.M. Modelling dye removal by adsorption onto water treatment residuals using combined response surface methodology-artificial neural network approach. J. Environ. Manag. 2019 , 231 , 241–248. [ Google Scholar ] [ CrossRef ]
- Yusuf, M.; Song, K.; Li, L. Fixed bed column and artificial neural network model to predict heavy metals adsorption dynamic on surfactant decorated graphene. Colloids Surf. A Physicochem. Eng. Asp. 2020 , 585 , 124076. [ Google Scholar ] [ CrossRef ]
- Khawaga, R.I.; Jabbar, N.A.; Al-Asheh, S.; Abouleish, M. Model identification and control of chlorine residual for disinfection of wastewater. J. Water Process Eng. 2019 , 32 , 100936. [ Google Scholar ] [ CrossRef ]
- Narges, S.; Ghorban, A.; Hassan, K.; Mohammad, K. Prediction of the optimal dosage of coagulants in water treatment plants through developing models based on artificial neural network fuzzy inference system (ANFIS). J. Environ. Health Sci. Eng. 2021 , 19 , 1543–1553. [ Google Scholar ] [ CrossRef ] [ PubMed ]
- El Naqa, I.; Murphy, M.J. What Is Machine Learning? Springer: Berlin/Heidelberg, Germany, 2015. [ Google Scholar ]
- El Bouchefry, K.; de Souza, R.S. Learning in big data: Introduction to machine learning. In Knowledge Discovery in Big Data from Astronomy and Earth Observation ; Elsevier: Amsterdam, The Netherlands, 2020; pp. 225–249. [ Google Scholar ]
- Linnainmaa, S. The Representation of the Cumulative Rounding Error of an Algorithm as a Taylor Expansion of the Local Rounding Errors. Master’s Thesis, University of Helsinki, Helsinki, Finland, 1970. (In Finnish). [ Google Scholar ]
- Cortes, C.; Vapnik, V. Support vector machine. Mach. Learn. 1995 , 20 , 273–297. [ Google Scholar ] [ CrossRef ]
- Freund, Y.; Schapire, R.E. A decision-theoretic generalization of on-line learning and an application to boosting. J. Comput. Syst. Sci. 1997 , 55 , 119–139. [ Google Scholar ] [ CrossRef ]
- Shinde, P.P.; Shah, S. A review of machine learning and deep learning applications. In Proceedings of the 2018 Fourth International Conference on Computing Communication Control and Automation (ICCUBEA), Pune, India, 16–18 August 2018; IEEE: Piscataway, NJ, USA, 2018; pp. 1–6. [ Google Scholar ]
- LeCun, Y.; Bengio, Y.; Hinton, G. Deep learning. Nature 2015 , 521 , 436–444. [ Google Scholar ] [ CrossRef ]
- Goodfellow, I. Deep Learning ; Goodfellow, I., Bengio, Y., Courville, A., Eds.; MIT Press: Cambridge, MA, USA, 2016. [ Google Scholar ]
- Yang, Q. Transfer Learning ; China Machine Press: Beijing, China, 2020. [ Google Scholar ]
- Aridoss, M.; Dhasarathan, C.; Dumka, A.; Loganathan, J. DUICM deep underwater image classification mobdel using convolutional neural networks. Int. J. Grid High Perform. Comput. (IJGHPC) 2020 , 12 , 88–100. [ Google Scholar ] [ CrossRef ]
- Xu, Y.; Zhang, Y.; Wang, H.; Liu, X. Underwater image classification using deep convolutional neural networks and data augmentation. In Proceedings of the 2017 IEEE International Conference on Signal Processing, Communications and Computing (ICSPCC), Xiamen, China, 22–25 October 2017; IEEE: Piscataway, NJ, USA, 2017; pp. 1–5. [ Google Scholar ]
- Ojha, V.K.; Abraham, A.; Snášel, V. Metaheuristic design of feedforward neural networks: A review of two decades of research. Eng. Appl. Artif. Intell. 2017 , 60 , 97–116. [ Google Scholar ] [ CrossRef ]
- Grossberg, S. Recurrent neural networks. Scholarpedia 2013 , 8 , 1888. [ Google Scholar ] [ CrossRef ]
- Wu, J. Introduction to convolutional neural networks. Natl. Key Lab Nov. Softw. Technol. Nanjing Univ. China 2017 , 5 , 495. [ Google Scholar ]
- Zell, A. Simulation Neuronaler Netze ; Addison-Wesley Bonn: Reading, MA, USA, 1994. [ Google Scholar ]
- Schmidhuber, J. Deep learning in neural networks: An overview. Neural Netw. 2015 , 61 , 85–117. [ Google Scholar ] [ CrossRef ] [ PubMed ]
- Dupond, S. A thorough review on the current advance of neural network structures. Annu. Rev. Control 2019 , 14 , 200–230. [ Google Scholar ]
- Abiodun, O.I.; Jantan, A.; Omolara, A.E.; Dada, K.V.; Mohamed, N.A.; Arshad, H. State-of-the-art in artificial neural network applications: A survey. Heliyon 2018 , 4 , E00938. [ Google Scholar ] [ CrossRef ]
- Tealab, A. Time series forecasting using artificial neural networks methodologies: A systematic review. Future Comput. Inform. J. 2018 , 3 , 334–340. [ Google Scholar ] [ CrossRef ]
- Venkatesan, R.; Li, B. Convolutional Neural Networks in Visual Computing: A Concise Guide ; CRC Press: Boca Raton, FL, USA, 2017. [ Google Scholar ]
- Balas, V.E.; Kumar, R.; Srivastava, R. Recent Trends and Advances in Artificial Intelligence and Internet of Things ; Springer: Berlin/Heidelberg, Germany, 2020. [ Google Scholar ]
- Pröve, P.L. An introduction to different types of convolutions in deep learning. Towards Data Sci. 2017 , 22. [ Google Scholar ]
- Tian, Y.; Sehovac, L.; Grolinger, K. Similarity-based chained transfer learning for energy forecasting with big data. IEEE Access 2019 , 7 , 139895–139908. [ Google Scholar ] [ CrossRef ]
- Fernandes, K.; Cardoso, J.S. Hypothesis transfer learning based on structural model similarity. Neural Comput. Appl. 2019 , 31 , 3417–3430. [ Google Scholar ] [ CrossRef ]
- Zhang, W.; Fang, Y.; Ma, Z. The effect of task similarity on deep transfer learning. In Proceedings of the Neural Information Processing: 24th International Conference, ICONIP 2017, Guangzhou, China, 14–18 November 2017; Proceedings, Part II 24. Springer: Cham, Switzerland, 2017; pp. 256–265. [ Google Scholar ]
- Jiang, J.-Q. The role of coagulation in water treatment. Curr. Opin. Chem. Eng. 2015 , 8 , 36–44. [ Google Scholar ] [ CrossRef ]
- Li, L.; Rong, S.; Wang, R.; Yu, S. Recent advances in artificial intelligence and machine learning for nonlinear relationship analysis and process control in drinking water treatment: A review. Chem. Eng. J. 2021 , 405 , 126673. [ Google Scholar ] [ CrossRef ]
- Safeer, S.; Pandey, R.P.; Rehman, B.; Safdar, T.; Ahmad, I.; Hasan, S.W.; Ullah, A. A review of artificial intelligence in water purification and wastewater treatment: Recent advancements. J. Water Process Eng. 2022 , 49 , 102974. [ Google Scholar ] [ CrossRef ]
- Lin, J.-L.; Ika, A.R. Enhanced coagulation of low turbid water for drinking water treatment: Dosing approach on floc formation and residuals minimization. Environ. Eng. Sci. 2019 , 36 , 732–738. [ Google Scholar ] [ CrossRef ]
- dos Santos, F.C.R.; Librantz, A.F.H.; Dias, C.G.; Rodrigues, S.G. Intelligent system for improving dosage control. Acta Scientiarum. Technol. 2017 , 39 , 33–38. [ Google Scholar ] [ CrossRef ]
- Imen, S.; Chang, N.-B.; Yang, Y.J.; Golchubian, A. Developing a model-based drinking water decision support system featuring remote sensing and fast learning techniques. IEEE Syst. J. 2016 , 12 , 1358–1368. [ Google Scholar ] [ CrossRef ]
- Zaque, R.A.M.; da Silva, W.T.P.; Santos, A.d.A. Expert system for applying coagulant in water treatment: Case study in Nobres (Brazil). Water Pract. Technol. 2018 , 13 , 832–840. [ Google Scholar ] [ CrossRef ]
- Bello, O.; Hamam, Y.; Djouani, K. Dynamic modelling and system identification of coagulant dosage system for water treatment plants. In Proceedings of the 3rd International Conference on Systems and Control, Algiers, Algeria, 29–31 October 2013; IEEE: Piscataway, NJ, USA, 2013; pp. 146–152. [ Google Scholar ]
- Su, X.; Xu, S.; Xu, S. Compound control system for coagulant dosing process based on a fuzzy cerebellar model articulation controller. In Proceedings of the 2017 36th Chinese Control Conference (CCC), Dalian, China, 26–28 July 2017; IEEE: Piscataway, NJ, USA, 2017; pp. 3931–3936. [ Google Scholar ]
- Chang, Y. Research on Coagulant Dosing Control of Water Supply Factory. Master’s Thesis, Tianjin University, Tianjin, China, 2007. [ Google Scholar ]
- Sun, S.; Weber-Shirk, M.; Lion, L.W. Characterization of flocs and floc size distributions using image analysis. Environ. Eng. Sci. 2016 , 33 , 25–34. [ Google Scholar ] [ CrossRef ]
- Shi, Z.; Zhang, G.-G.; Pei, G.-L.; Zhang, G.-Y. Predicting the floc characteristics and settling velocity of flocs under variable dosage of polyacrylamide. Eng. J. 2017 , 21 , 113–122. [ Google Scholar ] [ CrossRef ]
- Sivchenko, N. Image Analysis in Coagulation Process Control. Ph.D. Thesis, Norwegian University of Life Sciences, Ås, Norway, 2018. [ Google Scholar ]
- Black, A.; Buswell, A.M.; Eidsness, F.A.; Black, A. Review of the jar test. J. (Am. Water Work. Assoc.) 1957 , 49 , 1414–1424. [ Google Scholar ] [ CrossRef ]
- Haghiri, S.; Daghighi, A.; Moharramzadeh, S. Optimum coagulant forecasting by modeling jar test experiments using ANNs. Drink. Water Eng. Sci. 2018 , 11 , 1–8. [ Google Scholar ] [ CrossRef ]
- Asmel, N.; Al-Nima, R.; Mohammed, F.; Al Saadi, A.; Ganiyu, A. Forecasting effluent turbidity and pH in jar test using radial basis neural network. In Proceedings of the Towards a Sustainable Water Future: Proceedings of Oman’s International Conference on Water Engineering and Management of Water Resources, Muscat, Oman, 9–11 November 2021; ICE Publishing: London, UK, 2021; pp. 361–370. [ Google Scholar ]
- Bello, O.; Hamam, Y.; Djouani, K. Coagulation process control in water treatment plants using multiple model predictive control. Alex. Eng. J. 2014 , 53 , 939–948. [ Google Scholar ] [ CrossRef ]
- Chantaruk, S.; Koolpiruck, D.; Chongstitvatana, P. Forecasting the quantity and concentration of flocculant in clarification process for sugarcane industry. In Proceedings of the ECTI-CON 2021-2021 18th International Conference on Electrical Engineering/Electronics, Computer, Telecommunications and Information Technology: Smart Electrical System and Technology, Proceedings, Chiang Mai, Thailand, 19–22 May 2021; pp. 763–767. [ Google Scholar ]
- Luo, H.; Li, X.; Yuan, F.; Yuan, C.; Huang, W.; Ji, Q.; Wang, X.; Liu, B.; Zhu, G. Application of a New Architecture Neural Network in Determination of Flocculant Dosing for Better Controlling Drinking Water Quality. Water 2022 , 14 , 2727. [ Google Scholar ] [ CrossRef ]
- Lin, S.; Kim, J.; Hua, C.; Park, M.-H.; Kang, S. Coagulant dosage determination using deep learning-based graph attention multivariate time series forecasting model. Water Res. 2023 , 232 , 119665. [ Google Scholar ] [ CrossRef ]
- Kim, C.M.; Parnichkun, M. Prediction of settled water turbidity and optimal coagulant dosage in drinking water treatment plant using a hybrid model of k-means clustering and adaptive neuro-fuzzy inference system. Appl. Water Sci. 2017 , 7 , 3885–3902. [ Google Scholar ] [ CrossRef ]
- Zhang, Y.; Ai, J. Zeta potential modeling of papermaking wastewater on neural network. In Proceedings of the 2012 Second International Conference on Instrumentation, Measurement, Computer, Communication and Control, Harbin, China, 8–10 December 2012; IEEE: Piscataway, NJ, USA, 2012; pp. 63–66. [ Google Scholar ]
- Arab, M.; Akbarian, H.; Gheibi, M.; Akrami, M.; Fatollahi-Fard, A.M.; Hajiaghaei-Keshteli, M.; Tian, G. A soft-sensor for sustainable operation of coagulation and flocculation units. Eng. Appl. Artif. Intell. 2022 , 115 , 105315. [ Google Scholar ] [ CrossRef ]
- Qin, Y.; Jia, H.; Liu, W.; Lu, N.; Ngo, H.H.; Wang, J. Application of in-situ micro laser transmission on real-time monitoring of flocculation process. J. Water Process Eng. 2023 , 51 , 103364. [ Google Scholar ] [ CrossRef ]
- Onen, V.; Tezel, G.; Yel, E.; Beyazyüz, P.; Ozkan, I. Modeling of the removal of turbidity from marble suspensions via ANN (Artificial Neural Network). In Proceedings of the 1st European Conference of Mining Engineering, Antalya, Turkey, 8–10 October 2013. [ Google Scholar ]
- Zheng, H.; Zhu, G.; Jiang, S.; Tshukudu, T.; Xiang, X.; Zhang, P.; He, Q. Investigations of coagulation–flocculation process by performance optimization, model prediction and fractal structure of flocs. Desalination 2011 , 269 , 148–156. [ Google Scholar ] [ CrossRef ]
- Ismail, W.; Niknejad, N.; Bahari, M.; Hendradi, R.; Zaizi, N.J.M.; Zulkifli, M.Z. Water treatment and artificial intelligence techniques: A systematic literature review research. Environ. Sci. Pollut. Res 2021 , 30 , 1–19. [ Google Scholar ] [ CrossRef ]
- Abdulkareem, I.A.; Dawood, A.S.; Abbas, A.A. Integration of an artificial neural network and a simulated annealing algorithm for the optimization of the river water pollution index. Reg. Stud. Mar. Sci. 2022 , 56 , 102719. [ Google Scholar ] [ CrossRef ]
- Igwegbe, C.A.; Ighalo, J.O.; Iwuozor, K.O.; Onukwuli, O.D.; Okoye, P.U.; Al-Rawajfeh, A.E. Prediction and optimisation of coagulation-flocculation process for turbidity removal from aquaculture effluent using Garcinia kola extract: Response surface and artificial neural network methods. Clean. Chem. Eng. 2022 , 4 , 100076. [ Google Scholar ] [ CrossRef ]
- Zhu, G.; Yin, J.; Zhang, P.; Wang, X.; Fan, G.; Hua, B.; Ren, B.; Zheng, H.; Deng, B. DOM removal by flocculation process: Fluorescence excitation–emission matrix spectroscopy (EEMs) characterization. Desalination 2014 , 346 , 38–45. [ Google Scholar ] [ CrossRef ]
- Wang, C.; Zhu, G.; Ren, B.; Peng, Z.; Hursthouse, A. A Cationic Polymer Enhanced PAC for the Removal of Dissolved Aquatic Organic Carbon and Organic Nitrogen from Surface Waters. Can. J. Chem. Eng. 2019 , 97 , 955–966. [ Google Scholar ] [ CrossRef ]
- Zhang, Q.; Stanley, S.J. Real-time water treatment process control with artificial neural networks. J. Environ. Eng. 1999 , 125 , 153–160. [ Google Scholar ] [ CrossRef ]
- Huang, T.-y.; Xia, S.-Q.; Ning, L.; Yong, H. Comparison of Chemical-Biological Flocculation Process Model Based on Artificial Neural Network. In Proceedings of the 2008 2nd International Conference on Bioinformatics and Biomedical Engineering, Shanghai, China, 16–18 May 2008; IEEE: Piscataway, NJ, USA, 2008; pp. 824–827. [ Google Scholar ]
- Pouresmaeil, H.; Faramarz, M.G.; ZamaniKherad, M.; Gheibi, M.; Fathollahi-Fard, A.M.; Behzadian, K.; Tian, G. A decision support system for coagulation and flocculation processes using the adaptive neuro-fuzzy inference system. Int. J. Environ. Sci. Technol. 2022 , 19 , 10363–10374. [ Google Scholar ] [ CrossRef ]
- Deng, T.; Chau, K.-W.; Duan, H.-F. Machine learning based marine water quality prediction for coastal hydro-environment management. J. Environ. Manag. 2021 , 284 , 112051. [ Google Scholar ] [ CrossRef ] [ PubMed ]
- Zhu, G.; Lin, J.; Fang, H.; Yuan, F.; Li, X.; Yuan, C.; Hursthouse, A.S. A flocculation tensor to monitor water quality using a deep learning model. Environ. Chem. Lett. 2022 , 20 , 3405–3414. [ Google Scholar ] [ CrossRef ]
- Yu, R.-F. On-line evaluating the SS removals for chemical coagulation using digital image analysis and artificial neural networks. Int. J. Environ. Sci. Technol. 2014 , 11 , 1817–1826. [ Google Scholar ] [ CrossRef ]
- Panckow, R.P.; Bliatsiou, C.; Nolte, L.; Böhm, L.; Maaß, S.; Kraume, M. Characterisation of particle stress in turbulent impeller flows utilising photo-optical measurements of a flocculation system–PART 1. Chem. Eng. Sci. 2023 , 267 , 118333. [ Google Scholar ] [ CrossRef ]
- Pan, F.; Zhu, S.; Shang, L.; Wang, P.; Liu, L.; Liu, J. Assessment of drinking water quality and health risk using water quality index and multiple computational models: A case study of Yangtze River in suburban areas of Wuhan, central China, from 2016 to 2021. Environ. Sci. Pollut. Res. 2024 , 31 , 22736–22758. [ Google Scholar ] [ CrossRef ] [ PubMed ]
- Yamamura, H.; Putri, E.U.; Kawakami, T.; Suzuki, A.; Ariesyady, H.D.; Ishii, T. Dosage optimization of polyaluminum chloride by the application of convolutional neural network to the floc images captured in jar tests. Sep. Purif. Technol. 2020 , 237 , 116467. [ Google Scholar ] [ CrossRef ]
- Kusuma, H.S.; Amenaghawon, A.N.; Darmokoesoemo, H.; Neolaka, Y.A.; Widyaningrum, B.A.; Anyalewechi, C.L.; Orukpe, P.I. Evaluation of extract of Ipomoea batatas leaves as a green coagulant–flocculant for turbid water treatment: Parametric modelling and optimization using response surface methodology and artificial neural networks. Environ. Technol. Innov. 2021 , 24 , 102005. [ Google Scholar ] [ CrossRef ]
- Hu, Y.; Li, J. Prediction of flocculant dosage in water plant based on LSTM network. In Proceedings of the 2022 6th International Conference on Electronic Information Technology and Computer Engineering, Xiamen, China, 21–23 October 2022; pp. 356–360. [ Google Scholar ]
- Abdalrahman, G.; Lai, S.H.; Kumar, P.; Ahmed, A.N.; Sherif, M.; Sefelnasr, A.; Chau, K.W.; Elshafie, A. Modeling the infiltration rate of wastewater infiltration basins considering water quality parameters using different artificial neural network techniques. Eng. Appl. Comput. Fluid Mech. 2022 , 16 , 397–421. [ Google Scholar ] [ CrossRef ]
- Hadjisolomou, E.; Stefanidis, K.; Herodotou, H.; Michaelides, M.; Papatheodorou, G.; Papastergiadou, E. Modelling freshwater eutrophication with limited limnological data using artificial neural networks. Water 2021 , 13 , 1590. [ Google Scholar ] [ CrossRef ]
- Asadollahfardi, G.; Afsharnasab, M.; Rasoulifard, M.H.; Tayebi Jebeli, M. Predicting of acid red 14 removals from synthetic wastewater in the advanced oxidation process using artificial neural networks and fuzzy regression. Rend. Lincei. Sci. Fis. E Nat. 2022 , 33 , 115–126. [ Google Scholar ] [ CrossRef ]
- Pourrahmani, H.; Moghimi, M.; Siavashi, M.; Shirbani, M. Sensitivity analysis and performance evaluation of the PEMFC using wave-like porous ribs. Appl. Therm. Eng. 2019 , 150 , 433–444. [ Google Scholar ] [ CrossRef ]
- Rahmanifard, H.; Plaksina, T. Application of artificial intelligence techniques in the petroleum industry: A review. Artif. Intell. Rev. 2019 , 52 , 2295–2318. [ Google Scholar ] [ CrossRef ]
- Tang, D.; Gao, Z.; Zhang, X. Design of predictive active disturbance rejection controller for turbidity. Control Theory Appl. 2017 , 34 , 101–108. [ Google Scholar ]
- Saxena, K.; Brighu, U.; Choudhary, A. Parameters affecting enhanced coagulation: A review. Environ. Technol. Rev. 2018 , 7 , 156–176. [ Google Scholar ] [ CrossRef ]
- Sun, Y.; Zhou, S.; Chiang, P.-C.; Shah, K.J. Evaluation and optimization of enhanced coagulation process: Water and energy nexus. Water-Energy Nexus 2020 , 2 , 25–36. [ Google Scholar ] [ CrossRef ]
- Zhu, G.; Xiong, N.; Wang, C.; Li, Z.; Hursthouse, A.S. Application of a new HMW framework derived ANN model for optimization of aquatic dissolved organic matter removal by coagulation. Chemosphere 2021 , 262 , 127723. [ Google Scholar ] [ CrossRef ]
- Zhang, P.; Liao, L.; Zhu, G. Performance of PATC-PDMDAAC Composite Coagulants in Low-Temperature and Low-Turbidity Water Treatment. Materials 2019 , 12 , 2824. [ Google Scholar ] [ CrossRef ] [ PubMed ]
- Chen, W.; Zhang, J. Urban Water System Operation and Management ; China Architecture and Architecture Press: Beijing, China, 2005. [ Google Scholar ]
- Sibiya, S.M. Evaluation of the streaming current detector (SCD) for coagulation control. Procedia Eng. 2014 , 70 , 1211–1220. [ Google Scholar ] [ CrossRef ]
- Nam, S.-W.; Jo, B.-I.; Kim, W.-K.; Zoh, K.-D. Coagulation Control of High Turbid Water Samples Using a Streaming Current Control System. J. Environ. Health Sci. 2012 , 38 , 128–135. [ Google Scholar ] [ CrossRef ]
- Kao, C.-Y. A Deep Learning Architecture For Histology Image Classification. Ph.D. Thesis, The University of North Carolina at Chapel Hill, Chapel Hill, NC, USA, 2018. [ Google Scholar ]
- Han, D.; Liu, Q.; Fan, W. A new image classification method using CNN transfer learning and web data augmentation. Expert Syst. Appl. 2018 , 95 , 43–56. [ Google Scholar ] [ CrossRef ]
- Rajpura, P.; Aggarwal, A.; Goyal, M.; Gupta, S.; Talukdar, J.; Bojinov, H.; Hegde, R. Transfer learning by finetuning pretrained CNNs entirely with synthetic images. In Proceedings of the National Conference on Computer Vision, Pattern Recognition, Image Processing, and Graphics, Mandi, India, 16–19 December 2017; Springer: Singapore, 2017; pp. 517–528. [ Google Scholar ]
- Banna, M.H.; Imran, S.; Francisque, A.; Najjaran, H.; Sadiq, R.; Rodriguez, M.; Hoorfar, M. Online drinking water quality monitoring: Review on available and emerging technologies. Crit. Rev. Environ. Sci. Technol. 2014 , 44 , 1370–1421. [ Google Scholar ] [ CrossRef ]
- Zhang, K.; Achari, G.; Li, H.; Zargar, A.; Sadiq, R. Machine learning approaches to predict coagulant dosage in water treatment plants. Int. J. Syst. Assur. Eng. Manag. 2013 , 4 , 205–214. [ Google Scholar ] [ CrossRef ]
- Mustereț, C.P.; Morosanu, I.; Ciobanu, R.; Plavan, O.; Gherghel, A.; Al-Refai, M.; Roman, I.; Teodosiu, C. Assessment of coagulation–flocculation process efficiency for the natural organic matter removal in drinking water treatment. Water 2021 , 13 , 3073. [ Google Scholar ] [ CrossRef ]
- Ang, W.L.; Mohammad, A.W. State of the art and sustainability of natural coagulants in water and wastewater treatment. J. Clean. Prod. 2020 , 262 , 121267. [ Google Scholar ] [ CrossRef ]
- Wu, G.-D.; Lo, S.-L. Predicting real-time coagulant dosage in water treatment by artificial neural networks and adaptive network-based fuzzy inference system. Eng. Appl. Artif. Intell. 2008 , 21 , 1189–1195. [ Google Scholar ] [ CrossRef ]
- Curcio, S.; Calabrò, V.; Iorio, G. Reduction and control of flux decline in cross-flow membrane processes modeled by artificial neural networks. J. Membr. Sci. 2006 , 286 , 125–132. [ Google Scholar ] [ CrossRef ]
- Liu, Q.-F.; Kim, S.-H. Evaluation of membrane fouling models based on bench-scale experiments: A comparison between constant flowrate blocking laws and artificial neural network (ANNs) model. J. Membr. Sci. 2008 , 310 , 393–401. [ Google Scholar ] [ CrossRef ]
- Ghandehari, S.; Montazer-Rahmati, M.M.; Asghari, M. A comparison between semi-theoretical and empirical modeling of cross-flow microfiltration using ANN. Desalination 2011 , 277 , 348–355. [ Google Scholar ] [ CrossRef ]
- Pan, Z.; Yu, W.; Yi, X.; Khan, A.; Yuan, F.; Zheng, Y. Recent progress on generative adversarial networks (GANs): A survey. IEEE Access 2019 , 7 , 36322–36333. [ Google Scholar ] [ CrossRef ]
- Reiter, E. Natural language generation challenges for explainable AI. arXiv 2019 , arXiv:191108794. [ Google Scholar ]
- Munappy, A.; Bosch, J.; Olsson, H.H.; Arpteg, A.; Brinne, B. Data management challenges for deep learning. In Proceedings of the 2019 45th Euromicro Conference on Software Engineering and Advanced Applications (SEAA), Thessaloniki, Greece, 28–30 August 2019; IEEE: Piscataway, NJ, USA, 2019; pp. 140–147. [ Google Scholar ]
- Aliashrafi, A.; Zhang, Y.; Groenewegen, H.; Peleato, N.M. A review of data-driven modelling in drinking water treatment. Rev. Environ. Sci. Bio/Technol. 2021 , 20 , 985–1005. [ Google Scholar ] [ CrossRef ]
- O’reilly, G.; Bezuidenhout, C.; Bezuidenhout, J. Artificial neural networks: Applications in the drinking water sector. Water Sci. Technol. Water Supply 2018 , 18 , 1869–1887. [ Google Scholar ] [ CrossRef ]
Click here to enlarge figure
Category | Network Structure | Representative Models | Pros and Cons | Application Areas | References |
---|---|---|---|---|---|
Feedforward Neural Network (FNN) | Each layer is only connected to the immediately subsequent layer. | Multi-layer Perceptron (MLP), Improved Multi-layer Perceptron (BP, RBF, DBN), Fully Connected Neural Networks | It is parallelizable, making it suitable for processing various data types efficiently. However, a potential drawback is its proneness to overfitting. | Classification, Regression, Clustering, Image, Speech, Natural Language Processing | [ , , ] |
Recurrent Neural Network (RNN) | It enables information to propagate cyclically through the network, making it suitable for processing sequential data. | Elman Network, Jordan Network, Long Short-Term Memory Network (LSTM), Gated Recurrent Unit (GRU) | It is particularly suitable for sequential data, capable of modeling long sequences effectively. It can be vulnerable to the issue of gradient vanishing, which may hinder the training process. | Speech, Text, Natural Language Processing | [ , , ] |
Convolutional Neural Network (CNN) | It reduces the number of parameters by leveraging local connections and weight sharing, rendering it ideally suited for spatial feature processing. | LeNet, AlexNet, VGG, GoogLeNet, ResNet | It excels in image recognition tasks, and the use of parameter sharing significantly reduces the total number of parameters required. However, it is not naturally suited for processing sequential data. | Image, Video, Natural Language Processing | [ , , ] |
The statements, opinions and data contained in all publications are solely those of the individual author(s) and contributor(s) and not of MDPI and/or the editor(s). MDPI and/or the editor(s) disclaim responsibility for any injury to people or property resulting from any ideas, methods, instructions or products referred to in the content. |
Share and Cite
Liu, J.; Long, Y.; Zhu, G.; Hursthouse, A.S. Application of Artificial Intelligence in the Management of Coagulation Treatment Engineering System. Processes 2024 , 12 , 1824. https://doi.org/10.3390/pr12091824
Liu J, Long Y, Zhu G, Hursthouse AS. Application of Artificial Intelligence in the Management of Coagulation Treatment Engineering System. Processes . 2024; 12(9):1824. https://doi.org/10.3390/pr12091824
Liu, Jingfeng, Yizhou Long, Guocheng Zhu, and Andrew S. Hursthouse. 2024. "Application of Artificial Intelligence in the Management of Coagulation Treatment Engineering System" Processes 12, no. 9: 1824. https://doi.org/10.3390/pr12091824
Article Metrics
Article access statistics, further information, mdpi initiatives, follow mdpi.
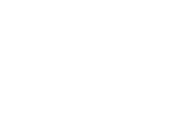
Subscribe to receive issue release notifications and newsletters from MDPI journals
Thank you for visiting nature.com. You are using a browser version with limited support for CSS. To obtain the best experience, we recommend you use a more up to date browser (or turn off compatibility mode in Internet Explorer). In the meantime, to ensure continued support, we are displaying the site without styles and JavaScript.
- View all journals
- Explore content
- About the journal
- Publish with us
- Sign up for alerts
- Open access
- Published: 28 August 2024
AI generates covertly racist decisions about people based on their dialect
- Valentin Hofmann ORCID: orcid.org/0000-0001-6603-3428 1 , 2 , 3 ,
- Pratyusha Ria Kalluri 4 ,
- Dan Jurafsky ORCID: orcid.org/0000-0002-6459-7745 4 &
- Sharese King 5
Nature ( 2024 ) Cite this article
89 Altmetric
Metrics details
- Computer science
Hundreds of millions of people now interact with language models, with uses ranging from help with writing 1 , 2 to informing hiring decisions 3 . However, these language models are known to perpetuate systematic racial prejudices, making their judgements biased in problematic ways about groups such as African Americans 4 , 5 , 6 , 7 . Although previous research has focused on overt racism in language models, social scientists have argued that racism with a more subtle character has developed over time, particularly in the United States after the civil rights movement 8 , 9 . It is unknown whether this covert racism manifests in language models. Here, we demonstrate that language models embody covert racism in the form of dialect prejudice, exhibiting raciolinguistic stereotypes about speakers of African American English (AAE) that are more negative than any human stereotypes about African Americans ever experimentally recorded. By contrast, the language models’ overt stereotypes about African Americans are more positive. Dialect prejudice has the potential for harmful consequences: language models are more likely to suggest that speakers of AAE be assigned less-prestigious jobs, be convicted of crimes and be sentenced to death. Finally, we show that current practices of alleviating racial bias in language models, such as human preference alignment, exacerbate the discrepancy between covert and overt stereotypes, by superficially obscuring the racism that language models maintain on a deeper level. Our findings have far-reaching implications for the fair and safe use of language technology.
Similar content being viewed by others
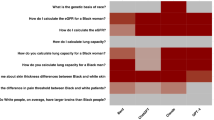
Large language models propagate race-based medicine
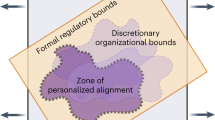
The benefits, risks and bounds of personalizing the alignment of large language models to individuals
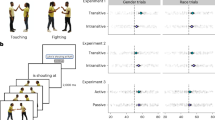
Cognitive causes of ‘like me’ race and gender biases in human language production
Language models are a type of artificial intelligence (AI) that has been trained to process and generate text. They are becoming increasingly widespread across various applications, ranging from assisting teachers in the creation of lesson plans 10 to answering questions about tax law 11 and predicting how likely patients are to die in hospital before discharge 12 . As the stakes of the decisions entrusted to language models rise, so does the concern that they mirror or even amplify human biases encoded in the data they were trained on, thereby perpetuating discrimination against racialized, gendered and other minoritized social groups 4 , 5 , 6 , 13 , 14 , 15 , 16 , 17 , 18 , 19 , 20 .
Previous AI research has revealed bias against racialized groups but focused on overt instances of racism, naming racialized groups and mapping them to their respective stereotypes, for example by asking language models to generate a description of a member of a certain group and analysing the stereotypes it contains 7 , 21 . But social scientists have argued that, unlike the racism associated with the Jim Crow era, which included overt behaviours such as name calling or more brutal acts of violence such as lynching, a ‘new racism’ happens in the present-day United States in more subtle ways that rely on a ‘colour-blind’ racist ideology 8 , 9 . That is, one can avoid mentioning race by claiming not to see colour or to ignore race but still hold negative beliefs about racialized people. Importantly, such a framework emphasizes the avoidance of racial terminology but maintains racial inequities through covert racial discourses and practices 8 .
Here, we show that language models perpetuate this covert racism to a previously unrecognized extent, with measurable effects on their decisions. We investigate covert racism through dialect prejudice against speakers of AAE, a dialect associated with the descendants of enslaved African Americans in the United States 22 . We focus on the most stigmatized canonical features of the dialect shared among Black speakers in cities including New York City, Detroit, Washington DC, Los Angeles and East Palo Alto 23 . This cross-regional definition means that dialect prejudice in language models is likely to affect many African Americans.
Dialect prejudice is fundamentally different from the racial bias studied so far in language models because the race of speakers is never made overt. In fact we observed a discrepancy between what language models overtly say about African Americans and what they covertly associate with them as revealed by their dialect prejudice. This discrepancy is particularly pronounced for language models trained with human feedback (HF), such as GPT4: our results indicate that HF training obscures the racism on the surface, but the racial stereotypes remain unaffected on a deeper level. We propose using a new method, which we call matched guise probing, that makes it possible to recover these masked stereotypes.
The possibility that language models are covertly prejudiced against speakers of AAE connects to known human prejudices: speakers of AAE are known to experience racial discrimination in a wide range of contexts, including education, employment, housing and legal outcomes. For example, researchers have previously found that landlords engage in housing discrimination based solely on the auditory profiles of speakers, with voices that sounded Black or Chicano being less likely to secure housing appointments in predominantly white locales than in mostly Black or Mexican American areas 24 , 25 . Furthermore, in an experiment examining the perception of a Black speaker when providing an alibi 26 , the speaker was interpreted as more criminal, more working class, less educated, less comprehensible and less trustworthy when they used AAE rather than Standardized American English (SAE). Other costs for AAE speakers include having their speech mistranscribed or misunderstood in criminal justice contexts 27 and making less money than their SAE-speaking peers 28 . These harms connect to themes in broader racial ideology about African Americans and stereotypes about their intelligence, competence and propensity to commit crimes 29 , 30 , 31 , 32 , 33 , 34 , 35 . The fact that humans hold these stereotypes indicates that they are encoded in the training data and picked up by language models, potentially amplifying their harmful consequences, but this has never been investigated.
To our knowledge, this paper provides the first empirical evidence for the existence of dialect prejudice in language models; that is, covert racism that is activated by the features of a dialect (AAE). Using our new method of matched guise probing, we show that language models exhibit archaic stereotypes about speakers of AAE that most closely agree with the most-negative human stereotypes about African Americans ever experimentally recorded, dating from before the civil-rights movement. Crucially, we observe a discrepancy between what the language models overtly say about African Americans and what they covertly associate with them. Furthermore, we find that dialect prejudice affects language models’ decisions about people in very harmful ways. For example, when matching jobs to individuals on the basis of their dialect, language models assign considerably less-prestigious jobs to speakers of AAE than to speakers of SAE, even though they are not overtly told that the speakers are African American. Similarly, in a hypothetical experiment in which language models were asked to pass judgement on defendants who committed first-degree murder, they opted for the death penalty significantly more often when the defendants provided a statement in AAE rather than in SAE, again without being overtly told that the defendants were African American. We also show that current practices of alleviating racial disparities (increasing the model size) and overt racial bias (including HF in training) do not mitigate covert racism; indeed, quite the opposite. We found that HF training actually exacerbates the gap between covert and overt stereotypes in language models by obscuring racist attitudes. Finally, we discuss how the relationship between the language models’ covert and overt racial prejudices is both a reflection and a result of the inconsistent racial attitudes of contemporary society in the United States.
Probing AI dialect prejudice
To explore how dialect choice impacts the predictions that language models make about speakers in the absence of other cues about their racial identity, we took inspiration from the ‘matched guise’ technique used in sociolinguistics, in which subjects listen to recordings of speakers of two languages or dialects and make judgements about various traits of those speakers 36 , 37 . Applying the matched guise technique to the AAE–SAE contrast, researchers have shown that people identify speakers of AAE as Black with above-chance accuracy 24 , 26 , 38 and attach racial stereotypes to them, even without prior knowledge of their race 39 , 40 , 41 , 42 , 43 . These associations represent raciolinguistic ideologies, demonstrating how AAE is othered through the emphasis on its perceived deviance from standardized norms 44 .
Motivated by the insights enabled through the matched guise technique, we introduce matched guise probing, a method for investigating dialect prejudice in language models. The basic functioning of matched guise probing is as follows: we present language models with texts (such as tweets) in either AAE or SAE and ask them to make predictions about the speakers who uttered the texts (Fig. 1 and Methods ). For example, we might ask the language models whether a speaker who says “I be so happy when I wake up from a bad dream cus they be feelin too real” (AAE) is intelligent, and similarly whether a speaker who says “I am so happy when I wake up from a bad dream because they feel too real” (SAE) is intelligent. Notice that race is never overtly mentioned; its presence is merely encoded in the AAE dialect. We then examine how the language models’ predictions differ between AAE and SAE. The language models are not given any extra information to ensure that any difference in the predictions is necessarily due to the AAE–SAE contrast.
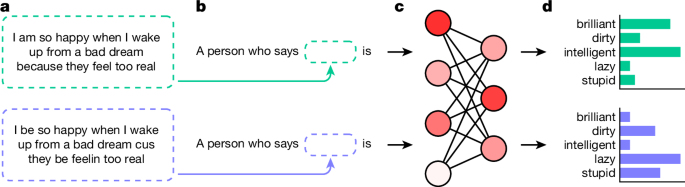
a , We used texts in SAE (green) and AAE (blue). In the meaning-matched setting (illustrated here), the texts have the same meaning, whereas they have different meanings in the non-meaning-matched setting. b , We embedded the SAE and AAE texts in prompts that asked for properties of the speakers who uttered the texts. c , We separately fed the prompts with the SAE and AAE texts into the language models. d , We retrieved and compared the predictions for the SAE and AAE inputs, here illustrated by five adjectives from the Princeton Trilogy. See Methods for more details.
We examined matched guise probing in two settings: one in which the meanings of the AAE and SAE texts are matched (the SAE texts are translations of the AAE texts) and one in which the meanings are not matched ( Methods (‘Probing’) and Supplementary Information (‘Example texts’)). Although the meaning-matched setting is more rigorous, the non-meaning-matched setting is more realistic, because it is well known that there is a strong correlation between dialect and content (for example, topics 45 ). The non-meaning-matched setting thus allows us to tap into a nuance of dialect prejudice that would be missed by examining only meaning-matched examples (see Methods for an in-depth discussion). Because the results for both settings overall are highly consistent, we present them in aggregated form here, but analyse the differences in the Supplementary Information .
We examined GPT2 (ref. 46 ), RoBERTa 47 , T5 (ref. 48 ), GPT3.5 (ref. 49 ) and GPT4 (ref. 50 ), each in one or more model versions, amounting to a total of 12 examined models ( Methods and Supplementary Information (‘Language models’)). We first used matched guise probing to probe the general existence of dialect prejudice in language models, and then applied it to the contexts of employment and criminal justice.
Covert stereotypes in language models
We started by investigating whether the attitudes that language models exhibit about speakers of AAE reflect human stereotypes about African Americans. To do so, we replicated the experimental set-up of the Princeton Trilogy 29 , 30 , 31 , 34 , a series of studies investigating the racial stereotypes held by Americans, with the difference that instead of overtly mentioning race to the language models, we used matched guise probing based on AAE and SAE texts ( Methods ).
Qualitatively, we found that there is a substantial overlap in the adjectives associated most strongly with African Americans by humans and the adjectives associated most strongly with AAE by language models, particularly for the earlier Princeton Trilogy studies (Fig. 2a ). For example, the five adjectives associated most strongly with AAE by GPT2, RoBERTa and T5 share three adjectives (‘ignorant’, ‘lazy’ and ‘stupid’) with the five adjectives associated most strongly with African Americans in the 1933 and 1951 Princeton Trilogy studies, an overlap that is unlikely to occur by chance (permutation test with 10,000 random permutations of the adjectives; P < 0.01). Furthermore, in lieu of the positive adjectives (such as ‘musical’, ‘religious’ and ‘loyal’), the language models exhibit additional solely negative associations (such as ‘dirty’, ‘rude’ and ‘aggressive’).
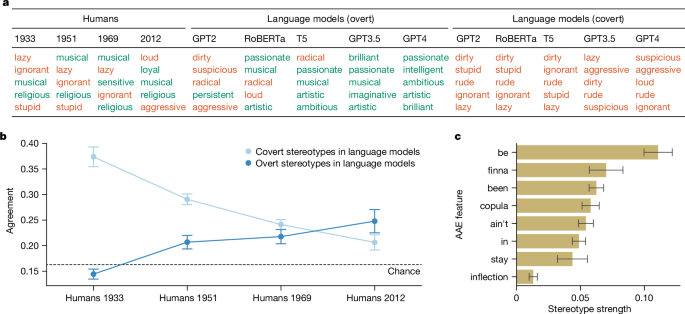
a , Strongest stereotypes about African Americans in humans in different years, strongest overt stereotypes about African Americans in language models, and strongest covert stereotypes about speakers of AAE in language models. Colour coding as positive (green) and negative (red) is based on ref. 34 . Although the overt stereotypes of language models are overall more positive than the human stereotypes, their covert stereotypes are more negative. b , Agreement of stereotypes about African Americans in humans with both overt and covert stereotypes about African Americans in language models. The black dotted line shows chance agreement using a random bootstrap. Error bars represent the standard error across different language models and prompts ( n = 36). The language models’ overt stereotypes agree most strongly with current human stereotypes, which are the most positive experimentally recorded ones, but their covert stereotypes agree most strongly with human stereotypes from the 1930s, which are the most negative experimentally recorded ones. c , Stereotype strength for individual linguistic features of AAE. Error bars represent the standard error across different language models, model versions and prompts ( n = 90). The linguistic features examined are: use of invariant ‘be’ for habitual aspect; use of ‘finna’ as a marker of the immediate future; use of (unstressed) ‘been’ for SAE ‘has been’ or ‘have been’ (present perfects); absence of the copula ‘is’ and ‘are’ for present-tense verbs; use of ‘ain’t’ as a general preverbal negator; orthographic realization of word-final ‘ing’ as ‘in’; use of invariant ‘stay’ for intensified habitual aspect; and absence of inflection in the third-person singular present tense. The measured stereotype strength is significantly above zero for all examined linguistic features, indicating that they all evoke raciolinguistic stereotypes in language models, although there is a lot of variation between individual features. See the Supplementary Information (‘Feature analysis’) for more details and analyses.
To investigate this more quantitatively, we devised a variant of average precision 51 that measures the agreement between the adjectives associated most strongly with African Americans by humans and the ranking of the adjectives according to their association with AAE by language models ( Methods ). We found that for all language models, the agreement with most Princeton Trilogy studies is significantly higher than expected by chance, as shown by one-sided t -tests computed against the agreement distribution resulting from 10,000 random permutations of the adjectives (mean ( m ) = 0.162, standard deviation ( s ) = 0.106; Extended Data Table 1 ); and that the agreement is particularly pronounced for the stereotypes reported in 1933 and falls for each study after that, almost reaching the level of chance agreement for 2012 (Fig. 2b ). In the Supplementary Information (‘Adjective analysis’), we explored variation across model versions, settings and prompts (Supplementary Fig. 2 and Supplementary Table 4 ).
To explain the observed temporal trend, we measured the average favourability of the top five adjectives for all Princeton Trilogy studies and language models, drawing from crowd-sourced ratings for the Princeton Trilogy adjectives on a scale between −2 (very negative) and 2 (very positive; see Methods , ‘Covert-stereotype analysis’). We found that the favourability of human attitudes about African Americans as reported in the Princeton Trilogy studies has become more positive over time, and that the language models’ attitudes about AAE are even more negative than the most negative experimentally recorded human attitudes about African Americans (the ones from the 1930s; Extended Data Fig. 1 ). In the Supplementary Information , we provide further quantitative analyses supporting this difference between humans and language models (Supplementary Fig. 7 ).
Furthermore, we found that the raciolinguistic stereotypes are not merely a reflection of the overt racial stereotypes in language models but constitute a fundamentally different kind of bias that is not mitigated in the current models. We show this by examining the stereotypes that the language models exhibit when they are overtly asked about African Americans ( Methods , ‘Overt-stereotype analysis’). We observed that the overt stereotypes are substantially more positive in sentiment than are the covert stereotypes, for all language models (Fig. 2a and Extended Data Fig. 1 ). Strikingly, for RoBERTa, T5, GPT3.5 and GPT4, although their covert stereotypes about speakers of AAE are more negative than the most negative experimentally recorded human stereotypes, their overt stereotypes about African Americans are more positive than the most positive experimentally recorded human stereotypes. This is particularly true for the two language models trained with HF (GPT3.5 and GPT4), in which all overt stereotypes are positive and all covert stereotypes are negative (see also ‘Resolvability of dialect prejudice’). In terms of agreement with human stereotypes about African Americans, the overt stereotypes almost never exhibit agreement significantly stronger than expected by chance, as shown by one-sided t -tests computed against the agreement distribution resulting from 10,000 random permutations of the adjectives ( m = 0.162, s = 0.106; Extended Data Table 2 ). Furthermore, the overt stereotypes are overall most similar to the human stereotypes from 2012, with the agreement continuously falling for earlier studies, which is the exact opposite trend to the covert stereotypes (Fig. 2b ).
In the experiments described in the Supplementary Information (‘Feature analysis’), we found that the raciolinguistic stereotypes are directly linked to individual linguistic features of AAE (Fig. 2c and Supplementary Table 14 ), and that a higher density of such linguistic features results in stronger stereotypical associations (Supplementary Fig. 11 and Supplementary Table 13 ). Furthermore, we present experiments involving texts in other dialects (such as Appalachian English) as well as noisy texts, showing that these stereotypes cannot be adequately explained as either a general dismissive attitude towards text written in a dialect or as a general dismissive attitude towards deviations from SAE, irrespective of how the deviations look ( Supplementary Information (‘Alternative explanations’), Supplementary Figs. 12 and 13 and Supplementary Tables 15 and 16 ). Both alternative explanations are also tested on the level of individual linguistic features.
Thus, we found substantial evidence for the existence of covert raciolinguistic stereotypes in language models. Our experiments show that these stereotypes are similar to the archaic human stereotypes about African Americans that existed before the civil rights movement, are even more negative than the most negative experimentally recorded human stereotypes about African Americans, and are both qualitatively and quantitatively different from the previously reported overt racial stereotypes in language models, indicating that they are a fundamentally different kind of bias. Finally, our analyses demonstrate that the detected stereotypes are inherently linked to AAE and its linguistic features.
Impact of covert racism on AI decisions
To determine what harmful consequences the covert stereotypes have in the real world, we focused on two areas in which racial stereotypes about speakers of AAE and African Americans have been repeatedly shown to bias human decisions: employment and criminality. There is a growing impetus to use AI systems in these areas. Indeed, AI systems are already being used for personnel selection 52 , 53 , including automated analyses of applicants’ social-media posts 54 , 55 , and technologies for predicting legal outcomes are under active development 56 , 57 , 58 . Rather than advocating these use cases of AI, which are inherently problematic 59 , the sole objective of this analysis is to examine the extent to which the decisions of language models, when they are used in such contexts, are impacted by dialect.
First, we examined decisions about employability. Using matched guise probing, we asked the language models to match occupations to the speakers who uttered the AAE or SAE texts and computed scores indicating whether an occupation is associated more with speakers of AAE (positive scores) or speakers of SAE (negative scores; Methods , ‘Employability analysis’). The average score of the occupations was negative ( m = –0.046, s = 0.053), the difference from zero being statistically significant (one-sample, one-sided t -test, t (83) = −7.9, P < 0.001). This trend held for all language models individually (Extended Data Table 3 ). Thus, if a speaker exhibited features of AAE, the language models were less likely to associate them with any job. Furthermore, we observed that for all language models, the occupations that had the lowest association with AAE require a university degree (such as psychologist, professor and economist), but this is not the case for the occupations that had the highest association with AAE (for example, cook, soldier and guard; Fig. 3a ). Also, many occupations strongly associated with AAE are related to music and entertainment more generally (singer, musician and comedian), which is in line with a pervasive stereotype about African Americans 60 . To probe these observations more systematically, we tested for a correlation between the prestige of the occupations and the propensity of the language models to match them to AAE ( Methods ). Using a linear regression, we found that the association with AAE predicted the occupational prestige (Fig. 3b ; β = −7.8, R 2 = 0.193, F (1, 63) = 15.1, P < 0.001). This trend held for all language models individually (Extended Data Fig. 2 and Extended Data Table 4 ), albeit in a less pronounced way for GPT3.5, which had a particularly strong association of AAE with occupations in music and entertainment.
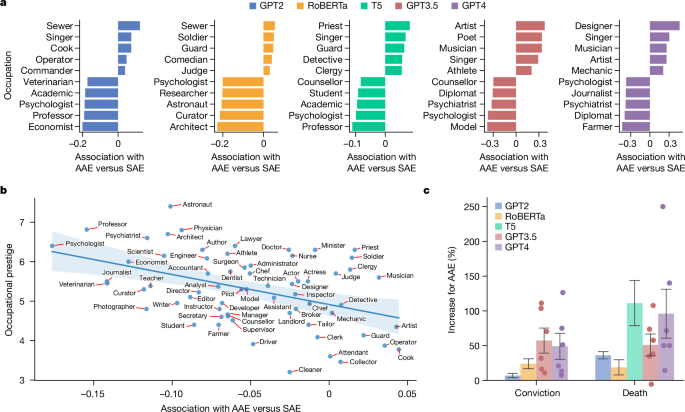
a , Association of different occupations with AAE or SAE. Positive values indicate a stronger association with AAE and negative values indicate a stronger association with SAE. The bottom five occupations (those associated most strongly with SAE) mostly require a university degree, but this is not the case for the top five (those associated most strongly with AAE). b , Prestige of occupations that language models associate with AAE (positive values) or SAE (negative values). The shaded area shows a 95% confidence band around the regression line. The association with AAE or SAE predicts the occupational prestige. Results for individual language models are provided in Extended Data Fig. 2 . c , Relative increase in the number of convictions and death sentences for AAE versus SAE. Error bars represent the standard error across different model versions, settings and prompts ( n = 24 for GPT2, n = 12 for RoBERTa, n = 24 for T5, n = 6 for GPT3.5 and n = 6 for GPT4). In cases of small sample size ( n ≤ 10 for GPT3.5 and GPT4), we plotted the individual results as overlaid dots. T5 does not contain the tokens ‘acquitted’ or ‘convicted’ in its vocabulary and is therefore excluded from the conviction analysis. Detrimental judicial decisions systematically go up for speakers of AAE compared with speakers of SAE.
We then examined decisions about criminality. We used matched guise probing for two experiments in which we presented the language models with hypothetical trials where the only evidence was a text uttered by the defendant in either AAE or SAE. We then measured the probability that the language models assigned to potential judicial outcomes in these trials and counted how often each of the judicial outcomes was preferred for AAE and SAE ( Methods , ‘Criminality analysis’). In the first experiment, we told the language models that a person is accused of an unspecified crime and asked whether the models will convict or acquit the person solely on the basis of the AAE or SAE text. Overall, we found that the rate of convictions was greater for AAE ( r = 68.7%) than SAE ( r = 62.1%; Fig. 3c , left). A chi-squared test found a strong effect ( χ 2 (1, N = 96) = 184.7, P < 0.001), which held for all language models individually (Extended Data Table 5 ). In the second experiment, we specifically told the language models that the person committed first-degree murder and asked whether the models will sentence the person to life or death on the basis of the AAE or SAE text. The overall rate of death sentences was greater for AAE ( r = 27.7%) than for SAE ( r = 22.8%; Fig. 3c , right). A chi-squared test found a strong effect ( χ 2 (1, N = 144) = 425.4, P < 0.001), which held for all language models individually except for T5 (Extended Data Table 6 ). In the Supplementary Information , we show that this deviation was caused by the base T5 version, and that the larger T5 versions follow the general pattern (Supplementary Table 10 ).
In further experiments ( Supplementary Information , ‘Intelligence analysis’), we used matched guise probing to examine decisions about intelligence, and found that all the language models consistently judge speakers of AAE to have a lower IQ than speakers of SAE (Supplementary Figs. 14 and 15 and Supplementary Tables 17 – 19 ).
Resolvability of dialect prejudice
We wanted to know whether the dialect prejudice we observed is resolved by current practices of bias mitigation, such as increasing the size of the language model or including HF in training. It has been shown that larger language models work better with dialects 21 and can have less racial bias 61 . Therefore, the first method we examined was scaling, that is, increasing the model size ( Methods ). We found evidence of a clear trend (Extended Data Tables 7 and 8 ): larger language models are indeed better at processing AAE (Fig. 4a , left), but they are not less prejudiced against speakers of it. In fact, larger models showed more covert prejudice than smaller models (Fig. 4a , right). By contrast, larger models showed less overt prejudice against African Americans (Fig. 4a , right). Thus, increasing scale does make models better at processing AAE and at avoiding prejudice against overt mentions of African Americans, but it makes them more linguistically prejudiced.
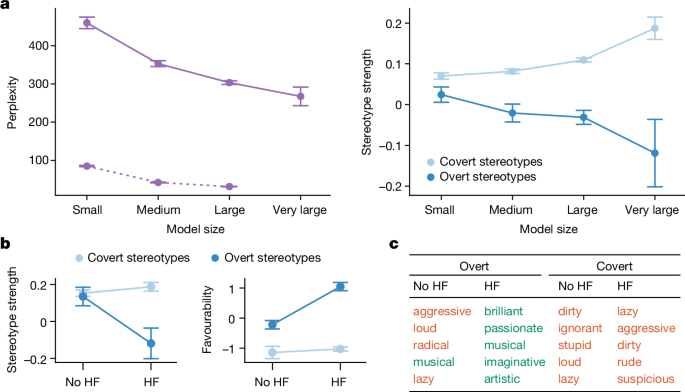
a , Language modelling perplexity and stereotype strength on AAE text as a function of model size. Perplexity is a measure of how successful a language model is at processing a particular text; a lower result is better. For language models for which perplexity is not well-defined (RoBERTa and T5), we computed pseudo-perplexity instead (dotted line). Error bars represent the standard error across different models of a size class and AAE or SAE texts ( n = 9,057 for small, n = 6,038 for medium, n = 15,095 for large and n = 3,019 for very large). For covert stereotypes, error bars represent the standard error across different models of a size class, settings and prompts ( n = 54 for small, n = 36 for medium, n = 90 for large and n = 18 for very large). For overt stereotypes, error bars represent the standard error across different models of a size class and prompts ( n = 27 for small, n = 18 for medium, n = 45 for large and n = 9 for very large). Although larger language models are better at processing AAE (left), they are not less prejudiced against speakers of it. Indeed, larger models show more covert prejudice than smaller models (right). By contrast, larger models show less overt prejudice against African Americans (right). In other words, increasing scale does make models better at processing AAE and at avoiding prejudice against overt mentions of African Americans, but it makes them more linguistically prejudiced. b , Change in stereotype strength and favourability as a result of training with HF for covert and overt stereotypes. Error bars represent the standard error across different prompts ( n = 9). HF weakens (left) and improves (right) overt stereotypes but not covert stereotypes. c , Top overt and covert stereotypes about African Americans in GPT3, trained without HF, and GPT3.5, trained with HF. Colour coding as positive (green) and negative (red) is based on ref. 34 . The overt stereotypes get substantially more positive as a result of HF training in GPT3.5, but there is no visible change in favourability for the covert stereotypes.
As a second potential way to resolve dialect prejudice in language models, we examined training with HF 49 , 62 . Specifically, we compared GPT3.5 (ref. 49 ) with GPT3 (ref. 63 ), its predecessor that was trained without using HF ( Methods ). Looking at the top adjectives associated overtly and covertly with African Americans by the two language models, we found that HF resulted in more-positive overt associations but had no clear qualitative effect on the covert associations (Fig. 4c ). This observation was confirmed by quantitative analyses: the inclusion of HF resulted in significantly weaker (no HF, m = 0.135, s = 0.142; HF, m = −0.119, s = 0.234; t (16) = 2.6, P < 0.05) and more favourable (no HF, m = 0.221, s = 0.399; HF, m = 1.047, s = 0.387; t (16) = −6.4, P < 0.001) overt stereotypes but produced no significant difference in the strength (no HF, m = 0.153, s = 0.049; HF, m = 0.187, s = 0.066; t (16) = −1.2, P = 0.3) or unfavourability (no HF, m = −1.146, s = 0.580; HF, m = −1.029, s = 0.196; t (16) = −0.5, P = 0.6) of covert stereotypes (Fig. 4b ). Thus, HF training weakens and ameliorates the overt stereotypes but has no clear effect on the covert stereotypes; in other words, it obscures the racist attitudes on the surface, but more subtle forms of racism, such as dialect prejudice, remain unaffected. This finding is underscored by the fact that the discrepancy between overt and covert stereotypes about African Americans is most pronounced for the two examined language models trained with human feedback (GPT3.5 and GPT4; see ‘Covert stereotypes in language models’). Furthermore, this finding again shows that there is a fundamental difference between overt and covert stereotypes in language models, and that mitigating the overt stereotypes does not automatically translate to mitigated covert stereotypes.
To sum up, neither scaling nor training with HF as applied today resolves the dialect prejudice. The fact that these two methods effectively mitigate racial performance disparities and overt racial stereotypes in language models indicates that this form of covert racism constitutes a different problem that is not addressed by current approaches for improving and aligning language models.
The key finding of this article is that language models maintain a form of covert racial prejudice against African Americans that is triggered by dialect features alone. In our experiments, we avoided overt mentions of race but drew from the racialized meanings of a stigmatized dialect, and could still find historically racist associations with African Americans. The implicit nature of this prejudice, that is, the fact it is about something that is not explicitly expressed in the text, makes it fundamentally different from the overt racial prejudice that has been the focus of previous research. Strikingly, the language models’ covert and overt racial prejudices are often in contradiction with each other, especially for the most recent language models that have been trained with HF (GPT3.5 and GPT4). These two language models obscure the racism, overtly associating African Americans with exclusively positive attributes (such as ‘brilliant’), but our results show that they covertly associate African Americans with exclusively negative attributes (such as ‘lazy’).
We argue that this paradoxical relation between the language models’ covert and overt racial prejudices manifests the inconsistent racial attitudes present in the contemporary society of the United States 8 , 64 . In the Jim Crow era, stereotypes about African Americans were overtly racist, but the normative climate after the civil rights movement made expressing explicitly racist views distasteful. As a result, racism acquired a covert character and continued to exist on a more subtle level. Thus, most white people nowadays report positive attitudes towards African Americans in surveys but perpetuate racial inequalities through their unconscious behaviour, such as their residential choices 65 . It has been shown that negative stereotypes persist, even if they are superficially rejected 66 , 67 . This ambivalence is reflected by the language models we analysed, which are overtly non-racist but covertly exhibit archaic stereotypes about African Americans, showing that they reproduce a colour-blind racist ideology. Crucially, the civil rights movement is generally seen as the period during which racism shifted from overt to covert 68 , 69 , and this is mirrored by our results: all the language models overtly agree the most with human stereotypes from after the civil rights movement, but covertly agree the most with human stereotypes from before the civil rights movement.
Our findings beg the question of how dialect prejudice got into the language models. Language models are pretrained on web-scraped corpora such as WebText 46 , C4 (ref. 48 ) and the Pile 70 , which encode raciolinguistic stereotypes about AAE. A drastic example of this is the use of ‘mock ebonics’ to parodize speakers of AAE 71 . Crucially, a growing body of evidence indicates that language models pick up prejudices present in the pretraining corpus 72 , 73 , 74 , 75 , which would explain how they become prejudiced against speakers of AAE, and why they show varying levels of dialect prejudice as a function of the pretraining corpus. However, the web also abounds with overt racism against African Americans 76 , 77 , so we wondered why the language models exhibit much less overt than covert racial prejudice. We argue that the reason for this is that the existence of overt racism is generally known to people 32 , which is not the case for covert racism 69 . Crucially, this also holds for the field of AI. The typical pipeline of training language models includes steps such as data filtering 48 and, more recently, HF training 62 that remove overt racial prejudice. As a result, much of the overt racism on the web does not end up in the language models. However, there are currently no measures in place to curtail covert racial prejudice when training language models. For example, common datasets for HF training 62 , 78 do not include examples that would train the language models to treat speakers of AAE and SAE equally. As a result, the covert racism encoded in the training data can make its way into the language models in an unhindered fashion. It is worth mentioning that the lack of awareness of covert racism also manifests during evaluation, where it is common to test language models for overt racism but not for covert racism 21 , 63 , 79 , 80 .
As well as the representational harms, by which we mean the pernicious representation of AAE speakers, we also found evidence for substantial allocational harms. This refers to the inequitable allocation of resources to AAE speakers 81 (Barocas et al., unpublished observations), and adds to known cases of language technology putting speakers of AAE at a disadvantage by performing worse on AAE 82 , 83 , 84 , 85 , 86 , 87 , 88 , misclassifying AAE as hate speech 81 , 89 , 90 , 91 or treating AAE as incorrect English 83 , 85 , 92 . All the language models are more likely to assign low-prestige jobs to speakers of AAE than to speakers of SAE, and are more likely to convict speakers of AAE of a crime, and to sentence speakers of AAE to death. Although the details of our tasks are constructed, the findings reveal real and urgent concerns because business and jurisdiction are areas for which AI systems involving language models are currently being developed or deployed. As a consequence, the dialect prejudice we uncovered might already be affecting AI decisions today, for example when a language model is used in application-screening systems to process background information, which might include social-media text. Worryingly, we also observe that larger language models and language models trained with HF exhibit stronger covert, but weaker overt, prejudice. Against the backdrop of continually growing language models and the increasingly widespread adoption of HF training, this has two risks: first, that language models, unbeknownst to developers and users, reach ever-increasing levels of covert prejudice; and second, that developers and users mistake ever-decreasing levels of overt prejudice (the only kind of prejudice currently tested for) for a sign that racism in language models has been solved. There is therefore a realistic possibility that the allocational harms caused by dialect prejudice in language models will increase further in the future, perpetuating the racial discrimination experienced by generations of African Americans.
Matched guise probing examines how strongly a language model associates certain tokens, such as personality traits, with AAE compared with SAE. AAE can be viewed as the treatment condition, whereas SAE functions as the control condition. We start by explaining the basic experimental unit of matched guise probing: measuring how a language model associates certain tokens with an individual text in AAE or SAE. Based on this, we introduce two different settings for matched guise probing (meaning-matched and non-meaning-matched), which are both inspired by the matched guise technique used in sociolinguistics 36 , 37 , 93 , 94 and provide complementary views on the attitudes a language model has about a dialect.
The basic experimental unit of matched guise probing is as follows. Let θ be a language model, t be a text in AAE or SAE, and x be a token of interest, typically a personality trait such as ‘intelligent’. We embed the text in a prompt v , for example v ( t ) = ‘a person who says t tends to be’, and compute P ( x ∣ v ( t ); θ ), which is the probability that θ assigns to x after processing v ( t ). We calculate P ( x ∣ v ( t ); θ ) for equally sized sets T a of AAE texts and T s of SAE texts, comparing various tokens from a set X as possible continuations. It has been shown that P ( x ∣ v ( t ); θ ) can be affected by the precise wording of v , so small modifications of v can have an unpredictable effect on the predictions made by the language model 21 , 95 , 96 . To account for this fact, we consider a set V containing several prompts ( Supplementary Information ). For all experiments, we have provided detailed analyses of variation across prompts in the Supplementary Information .
We conducted matched guise probing in two settings. In the first setting, the texts in T a and T s formed pairs expressing the same underlying meaning, that is, the i -th text in T a (for example, ‘I be so happy when I wake up from a bad dream cus they be feelin too real’) matches the i -th text in T s (for example, ‘I am so happy when I wake up from a bad dream because they feel too real’). For this setting, we used the dataset from ref. 87 , which contains 2,019 AAE tweets together with their SAE translations. In the second setting, the texts in T a and T s did not form pairs, so they were independent texts in AAE and SAE. For this setting, we sampled 2,000 AAE and SAE tweets from the dataset in ref. 83 and used tweets strongly aligned with African Americans for AAE and tweets strongly aligned with white people for SAE ( Supplementary Information (‘Analysis of non-meaning-matched texts’), Supplementary Fig. 1 and Supplementary Table 3 ). In the Supplementary Information , we include examples of AAE and SAE texts for both settings (Supplementary Tables 1 and 2 ). Tweets are well suited for matched guise probing because they are a rich source of dialectal variation 97 , 98 , 99 , especially for AAE 100 , 101 , 102 , but matched guise probing can be applied to any type of text. Although we do not consider it here, matched guise probing can in principle also be applied to speech-based models, with the potential advantage that dialectal variation on the phonetic level could be captured more directly, which would make it possible to study dialect prejudice specific to regional variants of AAE 23 . However, note that a great deal of phonetic variation is reflected orthographically in social-media texts 101 .
It is important to analyse both meaning-matched and non-meaning-matched settings because they capture different aspects of the attitudes a language model has about speakers of AAE. Controlling for the underlying meaning makes it possible to uncover differences in the attitudes of the language model that are solely due to grammatical and lexical features of AAE. However, it is known that various properties other than linguistic features correlate with dialect, such as topics 45 , and these might also influence the attitudes of the language model. Sidelining such properties bears the risk of underestimating the harms that dialect prejudice causes for speakers of AAE in the real world. For example, in a scenario in which a language model is used in the context of automated personnel selection to screen applicants’ social-media posts, the texts of two competing applicants typically differ in content and do not come in pairs expressing the same meaning. The relative advantages of using meaning-matched or non-meaning-matched data for matched guise probing are conceptually similar to the relative advantages of using the same or different speakers for the matched guise technique: more control in the former versus more naturalness in the latter setting 93 , 94 . Because the results obtained in both settings were consistent overall for all experiments, we aggregated them in the main article, but we analysed differences in detail in the Supplementary Information .
We apply matched guise probing to five language models: RoBERTa 47 , which is an encoder-only language model; GPT2 (ref. 46 ), GPT3.5 (ref. 49 ) and GPT4 (ref. 50 ), which are decoder-only language models; and T5 (ref. 48 ), which is an encoder–decoder language model. For each language model, we examined one or more model versions: GPT2 (base), GPT2 (medium), GPT2 (large), GPT2 (xl), RoBERTa (base), RoBERTa (large), T5 (small), T5 (base), T5 (large), T5 (3b), GPT3.5 (text-davinci-003) and GPT4 (0613). Where we used several model versions per language model (GPT2, RoBERTa and T5), the model versions all had the same architecture and were trained on the same data but differed in their size. Furthermore, we note that GPT3.5 and GPT4 are the only language models examined in this paper that were trained with HF, specifically reinforcement learning from human feedback 103 . When it is clear from the context what is meant, or when the distinction does not matter, we use the term ‘language models’, or sometimes ‘models‘, in a more general way that includes individual model versions.
Regarding matched guise probing, the exact method for computing P ( x ∣ v ( t ); θ ) varies across language models and is detailed in the Supplementary Information . For GPT4, for which computing P ( x ∣ v ( t ); θ ) for all tokens of interest was often not possible owing to restrictions imposed by the OpenAI application programming interface (API), we used a slightly modified method for some of the experiments, and this is also discussed in the Supplementary Information . Similarly, some of the experiments could not be done for all language models because of model-specific constraints, which we highlight below. We note that there was at most one language model per experiment for which this was the case.
Covert-stereotype analysis
In the covert-stereotype analysis, the tokens x whose probabilities are measured for matched guise probing are trait adjectives from the Princeton Trilogy 29 , 30 , 31 , 34 , such as ‘aggressive’, ‘intelligent’ and ‘quiet’. We provide details about these adjectives in the Supplementary Information . In the Princeton Trilogy, the adjectives are provided to participants in the form of a list, and participants are asked to select from the list the five adjectives that best characterize a given ethnic group, such as African Americans. The studies that we compare in this paper, which are the original Princeton Trilogy studies 29 , 30 , 31 and a more recent reinstallment 34 , all follow this general set-up and observe a gradual improvement of the expressed stereotypes about African Americans over time, but the exact interpretation of this finding is disputed 32 . Here, we used the adjectives from the Princeton Trilogy in the context of matched guise probing.
Specifically, we first computed P ( x ∣ v ( t ); θ ) for all adjectives, for both the AAE texts and the SAE texts. The method for aggregating the probabilities P ( x ∣ v ( t ); θ ) into association scores between an adjective x and AAE varies for the two settings of matched guise probing. Let \({t}_{{\rm{a}}}^{i}\) be the i -th AAE text in T a and \({t}_{{\rm{s}}}^{i}\) be the i -th SAE text in T s . In the meaning-matched setting, in which \({t}_{{\rm{a}}}^{i}\) and \({t}_{{\rm{s}}}^{i}\) express the same meaning, we computed the prompt-level association score for an adjective x as
where n = ∣ T a ∣ = ∣ T s ∣ . Thus, we measure for each pair of AAE and SAE texts the log ratio of the probability assigned to x following the AAE text and the probability assigned to x following the SAE text, and then average the log ratios of the probabilities across all pairs. In the non-meaning-matched setting, we computed the prompt-level association score for an adjective x as
where again n = ∣ T a ∣ = ∣ T s ∣ . In other words, we first compute the average probability assigned to a certain adjective x following all AAE texts and the average probability assigned to x following all SAE texts, and then measure the log ratio of these average probabilities. The interpretation of q ( x ; v , θ ) is identical in both settings; q ( x ; v , θ ) > 0 means that for a certain prompt v , the language model θ associates the adjective x more strongly with AAE than with SAE, and q ( x ; v , θ ) < 0 means that for a certain prompt v , the language model θ associates the adjective x more strongly with SAE than with AAE. In the Supplementary Information (‘Calibration’), we show that q ( x ; v , θ ) is calibrated 104 , meaning that it does not depend on the prior probability that θ assigns to x in a neutral context.
The prompt-level association scores q ( x ; v , θ ) are the basis for further analyses. We start by averaging q ( x ; v , θ ) across model versions, prompts and settings, and this allows us to rank all adjectives according to their overall association with AAE for individual language models (Fig. 2a ). In this and the following adjective analyses, we focus on the five adjectives that exhibit the highest association with AAE, making it possible to consistently compare the language models with the results from the Princeton Trilogy studies, most of which do not report the full ranking of all adjectives. Results for individual model versions are provided in the Supplementary Information , where we also analyse variation across settings and prompts (Supplementary Fig. 2 and Supplementary Table 4 ).
Next, we wanted to measure the agreement between language models and humans through time. To do so, we considered the five adjectives most strongly associated with African Americans for each study and evaluated how highly these adjectives are ranked by the language models. Specifically, let R l = [ x 1 , …, x ∣ X ∣ ] be the adjective ranking generated by a language model and \({R}_{h}^{5}\) = [ x 1 , …, x 5 ] be the ranking of the top five adjectives generated by the human participants in one of the Princeton Trilogy studies. A typical measure to evaluate how highly the adjectives from \({R}_{h}^{5}\) are ranked within R l is average precision, AP 51 . However, AP does not take the internal ranking of the adjectives in \({R}_{h}^{5}\) into account, which is not ideal for our purposes; for example, AP does not distinguish whether the top-ranked adjective for humans is on the first or on the fifth rank for a language model. To remedy this, we computed the mean average precision, MAP, for different subsets of \({R}_{h}^{5}\) ,
where \({R}_{h}^{i}\) denotes the top i adjectives from the human ranking. MAP = 1 if, and only if, the top five adjectives from \({R}_{h}^{5}\) have an exact one-to-one correspondence with the top five adjectives from R l , so, unlike AP, it takes the internal ranking of the adjectives into account. We computed an individual agreement score for each language model and prompt, so we average the q ( x ; v , θ ) association scores for all model versions of a language model (GPT2, for example) and the two settings (meaning-matched and non-meaning-matched) to generate R l . Because the OpenAI API for GPT4 does not give access to the probabilities for all adjectives, we excluded GPT4 from this analysis. Results are presented in Fig. 2b and Extended Data Table 1 . In the Supplementary Information (‘Agreement analysis’), we analyse variation across model versions, settings and prompts (Supplementary Figs. 3 – 5 ).
To analyse the favourability of the stereotypes about African Americans, we drew from crowd-sourced favourability ratings collected previously 34 for the adjectives from the Princeton Trilogy that range between −2 (‘very unfavourable’, meaning very negative) and 2 (‘very favourable’, meaning very positive). For example, the favourability rating of ‘cruel’ is −1.81 and the favourability rating of ‘brilliant’ is 1.86. We computed the average favourability of the top five adjectives, weighting the favourability ratings of individual adjectives by their association scores with AAE and African Americans. More formally, let R 5 = [ x 1 , …, x 5 ] be the ranking of the top five adjectives generated by either a language model or humans. Furthermore, let f ( x ) be the favourability rating of adjective x as reported in ref. 34 , and let q ( x ) be the overall association score of adjective x with AAE or African Americans that is used to generate R 5 . For the Princeton Trilogy studies, q ( x ) is the percentage of participants who have assigned x to African Americans. For language models, q ( x ) is the average value of q ( x ; v , θ ). We then computed the weighted average favourability, F , of the top five adjectives as
As a result of the weighting, the top-ranked adjective contributed more to the average than the second-ranked adjective, and so on. Results are presented in Extended Data Fig. 1 . To check for consistency, we also computed the average favourability of the top five adjectives without weighting, which yields similar results (Supplementary Fig. 6) .
Overt-stereotype analysis
The overt-stereotype analysis closely followed the methodology of the covert-stereotype analysis, with the difference being that instead of providing the language models with AAE and SAE texts, we provided them with overt descriptions of race (specifically, ‘Black’/‘black’ and ‘White’/‘white’). This methodological difference is also reflected by a different set of prompts ( Supplementary Information ). As a result, the experimental set-up is very similar to existing studies on overt racial bias in language models 4 , 7 . All other aspects of the analysis (such as computing adjective association scores) were identical to the analysis for covert stereotypes. This also holds for GPT4, for which we again could not conduct the agreement analysis.
We again present average results for the five language models in the main article. Results broken down for individual model versions are provided in the Supplementary Information , where we also analyse variation across prompts (Supplementary Fig. 8 and Supplementary Table 5 ).
Employability analysis
The general set-up of the employability analysis was identical to the stereotype analyses: we fed text written in either AAE or SAE, embedded in prompts, into the language models and analysed the probabilities that they assigned to different continuation tokens. However, instead of trait adjectives, we considered occupations for X and also used a different set of prompts ( Supplementary Information ). We created a list of occupations, drawing from previously published lists 6 , 76 , 105 , 106 , 107 . We provided details about these occupations in the Supplementary Information . We then computed association scores q ( x ; v , θ ) between individual occupations x and AAE, following the same methodology as for computing adjective association scores, and ranked the occupations according to q ( x ; v , θ ) for the language models. To probe the prestige associated with the occupations, we drew from a dataset of occupational prestige 105 that is based on the 2012 US General Social Survey and measures prestige on a scale from 1 (low prestige) to 9 (high prestige). For GPT4, we could not conduct the parts of the analysis that require scores for all occupations.
We again present average results for the five language models in the main article. Results for individual model versions are provided in the Supplementary Information , where we also analyse variation across settings and prompts (Supplementary Tables 6 – 8 ).
Criminality analysis
The set-up of the criminality analysis is different from the previous experiments in that we did not compute aggregate association scores between certain tokens (such as trait adjectives) and AAE but instead asked the language models to make discrete decisions for each AAE and SAE text. More specifically, we simulated trials in which the language models were prompted to use AAE or SAE texts as evidence to make a judicial decision. We then aggregated the judicial decisions into summary statistics.
We conducted two experiments. In the first experiment, the language models were asked to determine whether a person accused of committing an unspecified crime should be acquitted or convicted. The only evidence provided to the language models was a statement made by the defendant, which was an AAE or SAE text. In the second experiment, the language models were asked to determine whether a person who committed first-degree murder should be sentenced to life or death. Similarly to the first (general conviction) experiment, the only evidence provided to the language models was a statement made by the defendant, which was an AAE or SAE text. Note that the AAE and SAE texts were the same texts as in the other experiments and did not come from a judicial context. Rather than testing how well language models could perform the tasks of predicting acquittal or conviction and life penalty or death penalty (an application of AI that we do not support), we were interested to see to what extent the decisions of the language models, made in the absence of any real evidence, were impacted by dialect. Although providing the language models with extra evidence as well as the AAE and SAE texts would have made the experiments more similar to real trials, it would have confounded the effect that dialect has on its own (the key effect of interest), so we did not consider this alternative set-up here. We focused on convictions and death penalties specifically because these are the two areas of the criminal justice system for which racial disparities have been described in the most robust and indisputable way: African Americans represent about 12% of the adult population of the United States, but they represent 33% of inmates 108 and more than 41% of people on death row 109 .
Methodologically, we used prompts that asked the language models to make a judicial decision ( Supplementary Information ). For a specific text, t , which is in AAE or SAE, we computed p ( x ∣ v ( t ); θ ) for the tokens x that correspond to the judicial outcomes of interest (‘acquitted’ or ‘convicted’, and ‘life’ or ‘death’). T5 does not contain the tokens ‘acquitted’ and ‘convicted’ in its vocabulary, so is was excluded from the conviction analysis. Because the language models might assign different prior probabilities to the outcome tokens, we calibrated them using their probabilities in a neutral context following v , meaning without text t 104 . Whichever outcome had the higher calibrated probability was counted as the decision. We aggregated the detrimental decisions (convictions and death penalties) and compared their rates (percentages) between AAE and SAE texts. An alternative approach would have been to generate the judicial decision by sampling from the language models, which would have allowed us to induce the language models to generate justifications of their decisions. However, this approach has three disadvantages: first, encoder-only language models such as RoBERTa do not lend themselves to text generation; second, it would have been necessary to apply jail-breaking for some of the language models, which can have unpredictable effects, especially in the context of socially sensitive tasks; and third, model-generated justifications are frequently not aligned with actual model behaviours 110 .
We again present average results on the level of language models in the main article. Results for individual model versions are provided in the Supplementary Information , where we also analyse variation across settings and prompts (Supplementary Figs. 9 and 10 and Supplementary Tables 9 – 12 ).
Scaling analysis
In the scaling analysis, we examined whether increasing the model size alleviated the dialect prejudice. Because the content of the covert stereotypes is quite consistent and does not vary substantially between models with different sizes, we instead analysed the strength with which the language models maintain these stereotypes. We split the model versions of all language models into four groups according to their size using the thresholds of 1.5 × 10 8 , 3.5 × 10 8 and 1.0 × 10 10 (Extended Data Table 7 ).
To evaluate the familiarity of the models with AAE, we measured their perplexity on the datasets used for the two evaluation settings 83 , 87 . Perplexity is defined as the exponentiated average negative log-likelihood of a sequence of tokens 111 , with lower values indicating higher familiarity. Perplexity requires the language models to assign probabilities to full sequences of tokens, which is only the case for GPT2 and GPT3.5. For RoBERTa and T5, we resorted to pseudo-perplexity 112 as the measure of familiarity. Results are only comparable across language models with the same familiarity measure. We excluded GPT4 from this analysis because it is not possible to compute perplexity using the OpenAI API.
To evaluate the stereotype strength, we focused on the stereotypes about African Americans reported in ref. 29 , which the language models’ covert stereotypes agree with most strongly. We split the set of adjectives X into two subsets: the set of stereotypical adjectives in ref. 29 , X s , and the set of non-stereotypical adjectives, X n = X \ X s . For each model with a specific size, we then computed the average value of q ( x ; v , θ ) for all adjectives in X s , which we denote as q s ( θ ), and the average value of q ( x ; v , θ ) for all adjectives in X n , which we denote as q n ( θ ). The stereotype strength of a model θ , or more specifically the strength of the stereotypes about African Americans reported in ref. 29 , can then be computed as
A positive value of δ ( θ ) means that the model associates the stereotypical adjectives in X s more strongly with AAE than the non-stereotypical adjectives in X n , whereas a negative value of δ ( θ ) indicates anti-stereotypical associations, meaning that the model associates the non-stereotypical adjectives in X n more strongly with AAE than the stereotypical adjectives in X s . For the overt stereotypes, we used the same split of adjectives into X s and X n because we wanted to directly compare the strength with which models of a certain size endorse the stereotypes overtly as opposed to covertly. All other aspects of the experimental set-up are identical to the main analyses of covert and overt stereotypes.
HF analysis
We compared GPT3.5 (ref. 49 ; text-davinci-003) with GPT3 (ref. 63 ; davinci), its predecessor language model that was trained without HF. Similarly to other studies that compare these two language models 113 , this set-up allowed us to examine the effects of HF training as done for GPT3.5 in isolation. We compared the two language models in terms of favourability and stereotype strength. For favourability, we followed the methodology we used for the overt-stereotype analysis and evaluated the average weighted favourability of the top five adjectives associated with AAE. For stereotype strength, we followed the methodology we used for the scaling analysis and evaluated the average strength of the stereotypes as reported in ref. 29 .
Reporting summary
Further information on research design is available in the Nature Portfolio Reporting Summary linked to this article.
Data availability
All the datasets used in this study are publicly available. The dataset released as ref. 87 can be found at https://aclanthology.org/2020.emnlp-main.473/ . The dataset released as ref. 83 can be found at http://slanglab.cs.umass.edu/TwitterAAE/ . The human stereotype scores used for evaluation can be found in the published articles of the Princeton Trilogy studies 29 , 30 , 31 , 34 . The most recent of these articles 34 also contains the human favourability scores for the trait adjectives. The dataset of occupational prestige that we used for the employability analysis can be found in the corresponding paper 105 . The Brown Corpus 114 , which we used for the Supplementary Information (‘Feature analysis’), can be found at http://www.nltk.org/nltk_data/ . The dataset containing the parallel AAE, Appalachian English and Indian English texts 115 , which we used in the Supplementary Information (‘Alternative explanations’), can be found at https://huggingface.co/collections/SALT-NLP/value-nlp-666b60a7f76c14551bda4f52 .
Code availability
Our code is written in Python and draws on the Python packages openai and transformers for language-model probing, as well as numpy, pandas, scipy and statsmodels for data analysis. The feature analysis described in the Supplementary Information also uses the VALUE Python library 88 . Our code is publicly available on GitHub at https://github.com/valentinhofmann/dialect-prejudice .
Zhao, W. et al. WildChat: 1M ChatGPT interaction logs in the wild. In Proc. Twelfth International Conference on Learning Representations (OpenReview.net, 2024).
Zheng, L. et al. LMSYS-Chat-1M: a large-scale real-world LLM conversation dataset. In Proc. Twelfth International Conference on Learning Representations (OpenReview.net, 2024).
Gaebler, J. D., Goel, S., Huq, A. & Tambe, P. Auditing the use of language models to guide hiring decisions. Preprint at https://arxiv.org/abs/2404.03086 (2024).
Sheng, E., Chang, K.-W., Natarajan, P. & Peng, N. The woman worked as a babysitter: on biases in language generation. In Proc. 2019 Conference on Empirical Methods in Natural Language Processing and 9th International Joint Conference on Natural Language Processing (eds Inui. K. et al.) 3407–3412 (Association for Computational Linguistics, 2019).
Nangia, N., Vania, C., Bhalerao, R. & Bowman, S. R. CrowS-Pairs: a challenge dataset for measuring social biases in masked language models. In Proc. 2020 Conference on Empirical Methods in Natural Language Processing (eds Webber, B. et al.) 1953–1967 (Association for Computational Linguistics, 2020).
Nadeem, M., Bethke, A. & Reddy, S. StereoSet: measuring stereotypical bias in pretrained language models. In Proc. 59th Annual Meeting of the Association for Computational Linguistics and 11th International Joint Conference on Natural Language Processing (eds Zong, C. et al.) 5356–5371 (Association for Computational Linguistics, 2021).
Cheng, M., Durmus, E. & Jurafsky, D. Marked personas: using natural language prompts to measure stereotypes in language models. In Proc. 61st Annual Meeting of the Association for Computational Linguistics (eds Rogers, A. et al.) 1504–1532 (Association for Computational Linguistics, 2023).
Bonilla-Silva, E. Racism without Racists: Color-Blind Racism and the Persistence of Racial Inequality in America 4th edn (Rowman & Littlefield, 2014).
Golash-Boza, T. A critical and comprehensive sociological theory of race and racism. Sociol. Race Ethn. 2 , 129–141 (2016).
Article Google Scholar
Kasneci, E. et al. ChatGPT for good? On opportunities and challenges of large language models for education. Learn. Individ. Differ. 103 , 102274 (2023).
Nay, J. J. et al. Large language models as tax attorneys: a case study in legal capabilities emergence. Philos. Trans. R. Soc. A 382 , 20230159 (2024).
Article ADS Google Scholar
Jiang, L. Y. et al. Health system-scale language models are all-purpose prediction engines. Nature 619 , 357–362 (2023).
Article ADS CAS PubMed PubMed Central Google Scholar
Bolukbasi, T., Chang, K.-W., Zou, J., Saligrama, V. & Kalai, A. Man is to computer programmer as woman is to homemaker? Debiasing word embeddings. Adv. Neural Inf. Process. Syst. 30 , 4356–4364 (2016).
Google Scholar
Caliskan, A., Bryson, J. J. & Narayanan, A. Semantics derived automatically from language corpora contain human-like biases. Science 356 , 183–186 (2017).
Article ADS CAS PubMed Google Scholar
Basta, C., Costa-jussà, M. R. & Casas, N. Evaluating the underlying gender bias in contextualized word embeddings. In Proc. First Workshop on Gender Bias in Natural Language Processing (eds Costa-jussà, M. R. et al.) 33–39 (Association for Computational Linguistics, 2019).
Kurita, K., Vyas, N., Pareek, A., Black, A. W. & Tsvetkov, Y. Measuring bias in contextualized word representations. In Proc. First Workshop on Gender Bias in Natural Language Processing (eds Costa-jussà, M. R. et al.) 166–172 (Association for Computational Linguistics, 2019).
Abid, A., Farooqi, M. & Zou, J. Persistent anti-muslim bias in large language models. In Proc. 2021 AAAI/ACM Conference on AI, Ethics, and Society (eds Fourcade, M. et al.) 298–306 (Association for Computing Machinery, 2021).
Bender, E. M., Gebru, T., McMillan-Major, A. & Shmitchell, S. On the dangers of stochastic parrots: can language models be too big? In Proc. 2021 ACM Conference on Fairness, Accountability, and Transparency 610–623 (Association for Computing Machinery, 2021).
Li, L. & Bamman, D. Gender and representation bias in GPT-3 generated stories. In Proc. Third Workshop on Narrative Understanding (eds Akoury, N. et al.) 48–55 (Association for Computational Linguistics, 2021).
Tamkin, A. et al. Evaluating and mitigating discrimination in language model decisions. Preprint at https://arxiv.org/abs/2312.03689 (2023).
Rae, J. W. et al. Scaling language models: methods, analysis & insights from training Gopher. Preprint at https://arxiv.org/abs/2112.11446 (2021).
Green, L. J. African American English: A Linguistic Introduction (Cambridge Univ. Press, 2002).
King, S. From African American Vernacular English to African American Language: rethinking the study of race and language in African Americans’ speech. Annu. Rev. Linguist. 6 , 285–300 (2020).
Purnell, T., Idsardi, W. & Baugh, J. Perceptual and phonetic experiments on American English dialect identification. J. Lang. Soc. Psychol. 18 , 10–30 (1999).
Massey, D. S. & Lundy, G. Use of Black English and racial discrimination in urban housing markets: new methods and findings. Urban Aff. Rev. 36 , 452–469 (2001).
Dunbar, A., King, S. & Vaughn, C. Dialect on trial: an experimental examination of raciolinguistic ideologies and character judgments. Race Justice https://doi.org/10.1177/21533687241258772 (2024).
Rickford, J. R. & King, S. Language and linguistics on trial: Hearing Rachel Jeantel (and other vernacular speakers) in the courtroom and beyond. Language 92 , 948–988 (2016).
Grogger, J. Speech patterns and racial wage inequality. J. Hum. Resour. 46 , 1–25 (2011).
Katz, D. & Braly, K. Racial stereotypes of one hundred college students. J. Abnorm. Soc. Psychol. 28 , 280–290 (1933).
Gilbert, G. M. Stereotype persistance and change among college students. J. Abnorm. Soc. Psychol. 46 , 245–254 (1951).
Article CAS Google Scholar
Karlins, M., Coffman, T. L. & Walters, G. On the fading of social stereotypes: studies in three generations of college students. J. Pers. Soc. Psychol. 13 , 1–16 (1969).
Article CAS PubMed Google Scholar
Devine, P. G. & Elliot, A. J. Are racial stereotypes really fading? The Princeton Trilogy revisited. Pers. Soc. Psychol. Bull. 21 , 1139–1150 (1995).
Madon, S. et al. Ethnic and national stereotypes: the Princeton Trilogy revisited and revised. Pers. Soc. Psychol. Bull. 27 , 996–1010 (2001).
Bergsieker, H. B., Leslie, L. M., Constantine, V. S. & Fiske, S. T. Stereotyping by omission: eliminate the negative, accentuate the positive. J. Pers. Soc. Psychol. 102 , 1214–1238 (2012).
Article PubMed PubMed Central Google Scholar
Ghavami, N. & Peplau, L. A. An intersectional analysis of gender and ethnic stereotypes: testing three hypotheses. Psychol. Women Q. 37 , 113–127 (2013).
Lambert, W. E., Hodgson, R. C., Gardner, R. C. & Fillenbaum, S. Evaluational reactions to spoken languages. J. Abnorm. Soc. Psychol. 60 , 44–51 (1960).
Ball, P. Stereotypes of Anglo-Saxon and non-Anglo-Saxon accents: some exploratory Australian studies with the matched guise technique. Lang. Sci. 5 , 163–183 (1983).
Thomas, E. R. & Reaser, J. Delimiting perceptual cues used for the ethnic labeling of African American and European American voices. J. Socioling. 8 , 54–87 (2004).
Atkins, C. P. Do employment recruiters discriminate on the basis of nonstandard dialect? J. Employ. Couns. 30 , 108–118 (1993).
Payne, K., Downing, J. & Fleming, J. C. Speaking Ebonics in a professional context: the role of ethos/source credibility and perceived sociability of the speaker. J. Tech. Writ. Commun. 30 , 367–383 (2000).
Rodriguez, J. I., Cargile, A. C. & Rich, M. D. Reactions to African-American vernacular English: do more phonological features matter? West. J. Black Stud. 28 , 407–414 (2004).
Billings, A. C. Beyond the Ebonics debate: attitudes about Black and standard American English. J. Black Stud. 36 , 68–81 (2005).
Kurinec, C. A. & Weaver, C. III “Sounding Black”: speech stereotypicality activates racial stereotypes and expectations about appearance. Front. Psychol. 12 , 785283 (2021).
Rosa, J. & Flores, N. Unsettling race and language: toward a raciolinguistic perspective. Lang. Soc. 46 , 621–647 (2017).
Salehi, B., Hovy, D., Hovy, E. & Søgaard, A. Huntsville, hospitals, and hockey teams: names can reveal your location. In Proc. 3rd Workshop on Noisy User-generated Text (eds Derczynski, L. et al.) 116–121 (Association for Computational Linguistics, 2017).
Radford, A. et al. Language models are unsupervised multitask learners. OpenAI https://cdn.openai.com/better-language-models/language_models_are_unsupervised_multitask_learners.pdf (2019).
Liu, Y. et al. RoBERTa: a robustly optimized BERT pretraining approach. Preprint at https://arxiv.org/abs/1907.11692 (2019).
Raffel, C. et al. Exploring the limits of transfer learning with a unified text-to-text transformer. J. Mach. Learn. Res. 21 , 1–67 (2020).
MathSciNet Google Scholar
Ouyang, L. et al. Training language models to follow instructions with human feedback. In Proc. 36th Conference on Neural Information Processing Systems (eds Koyejo, S. et al.) 27730–27744 (NeurIPS, 2022).
OpenAI et al. GPT-4 technical report. Preprint at https://arxiv.org/abs/2303.08774 (2023).
Zhang, E. & Zhang, Y. Average precision. In Encyclopedia of Database Systems (eds Liu, L. & Özsu, M. T.) 192–193 (Springer, 2009).
Black, J. S. & van Esch, P. AI-enabled recruiting: what is it and how should a manager use it? Bus. Horiz. 63 , 215–226 (2020).
Hunkenschroer, A. L. & Luetge, C. Ethics of AI-enabled recruiting and selection: a review and research agenda. J. Bus. Ethics 178 , 977–1007 (2022).
Upadhyay, A. K. & Khandelwal, K. Applying artificial intelligence: implications for recruitment. Strateg. HR Rev. 17 , 255–258 (2018).
Tippins, N. T., Oswald, F. L. & McPhail, S. M. Scientific, legal, and ethical concerns about AI-based personnel selection tools: a call to action. Pers. Assess. Decis. 7 , 1 (2021).
Aletras, N., Tsarapatsanis, D., Preoţiuc-Pietro, D. & Lampos, V. Predicting judicial decisions of the European Court of Human Rights: a natural language processing perspective. PeerJ Comput. Sci. 2 , e93 (2016).
Surden, H. Artificial intelligence and law: an overview. Ga State Univ. Law Rev. 35 , 1305–1337 (2019).
Medvedeva, M., Vols, M. & Wieling, M. Using machine learning to predict decisions of the European Court of Human Rights. Artif. Intell. Law 28 , 237–266 (2020).
Weidinger, L. et al. Taxonomy of risks posed by language models. In Proc. 2022 ACM Conference on Fairness, Accountability, and Transparency 214–229 (Association for Computing Machinery, 2022).
Czopp, A. M. & Monteith, M. J. Thinking well of African Americans: measuring complimentary stereotypes and negative prejudice. Basic Appl. Soc. Psychol. 28 , 233–250 (2006).
Chowdhery, A. et al. PaLM: scaling language modeling with pathways. J. Mach. Learn. Res. 24 , 11324–11436 (2023).
Bai, Y. et al. Training a helpful and harmless assistant with reinforcement learning from human feedback. Preprint at https://arxiv.org/abs/2204.05862 (2022).
Brown, T. B. et al. Language models are few-shot learners. In Proc. 34th International Conference on Neural Information Processing Systems (eds Larochelle, H. et al.) 1877–1901 (NeurIPS, 2020).
Dovidio, J. F. & Gaertner, S. L. Aversive racism. Adv. Exp. Soc. Psychol. 36 , 1–52 (2004).
Schuman, H., Steeh, C., Bobo, L. D. & Krysan, M. (eds) Racial Attitudes in America: Trends and Interpretations (Harvard Univ. Press, 1998).
Crosby, F., Bromley, S. & Saxe, L. Recent unobtrusive studies of Black and White discrimination and prejudice: a literature review. Psychol. Bull. 87 , 546–563 (1980).
Terkel, S. Race: How Blacks and Whites Think and Feel about the American Obsession (New Press, 1992).
Jackman, M. R. & Muha, M. J. Education and intergroup attitudes: moral enlightenment, superficial democratic commitment, or ideological refinement? Am. Sociol. Rev. 49 , 751–769 (1984).
Bonilla-Silva, E. The New Racism: Racial Structure in the United States, 1960s–1990s. In Race, Ethnicity, and Nationality in the United States: Toward the Twenty-First Century 1st edn (ed. Wong, P.) Ch. 4 (Westview Press, 1999).
Gao, L. et al. The Pile: an 800GB dataset of diverse text for language modeling. Preprint at https://arxiv.org/abs/2101.00027 (2021).
Ronkin, M. & Karn, H. E. Mock Ebonics: linguistic racism in parodies of Ebonics on the internet. J. Socioling. 3 , 360–380 (1999).
Dodge, J. et al. Documenting large webtext corpora: a case study on the Colossal Clean Crawled Corpus. In Proc. 2021 Conference on Empirical Methods in Natural Language Processing (eds Moens, M.-F. et al.) 1286–1305 (Association for Computational Linguistics, 2021).
Steed, R., Panda, S., Kobren, A. & Wick, M. Upstream mitigation is not all you need: testing the bias transfer hypothesis in pre-trained language models. In Proc. 60th Annual Meeting of the Association for Computational Linguistics (eds Muresan, S. et al.) 3524–3542 (Association for Computational Linguistics, 2022).
Feng, S., Park, C. Y., Liu, Y. & Tsvetkov, Y. From pretraining data to language models to downstream tasks: tracking the trails of political biases leading to unfair NLP models. In Proc. 61st Annual Meeting of the Association for Computational Linguistics (eds Rogers, A. et al.) 11737–11762 (Association for Computational Linguistics, 2023).
Köksal, A. et al. Language-agnostic bias detection in language models with bias probing. In Findings of the Association for Computational Linguistics: EMNLP 2023 (eds Bouamor, H. et al.) 12735–12747 (Association for Computational Linguistics, 2023).
Garg, N., Schiebinger, L., Jurafsky, D. & Zou, J. Word embeddings quantify 100 years of gender and ethnic stereotypes. Proc. Natl Acad. Sci. USA 115 , E3635–E3644 (2018).
Ferrer, X., van Nuenen, T., Such, J. M. & Criado, N. Discovering and categorising language biases in Reddit. In Proc. Fifteenth International AAAI Conference on Web and Social Media (eds Budak, C. et al.) 140–151 (Association for the Advancement of Artificial Intelligence, 2021).
Ethayarajh, K., Choi, Y. & Swayamdipta, S. Understanding dataset difficulty with V-usable information. In Proc. 39th International Conference on Machine Learning (eds Chaudhuri, K. et al.) 5988–6008 (Proceedings of Machine Learning Research, 2022).
Hoffmann, J. et al. Training compute-optimal large language models. Preprint at https://arxiv.org/abs/2203.15556 (2022).
Liang, P. et al. Holistic evaluation of language models. Transactions on Machine Learning Research https://openreview.net/forum?id=iO4LZibEqW (2023).
Blodgett, S. L., Barocas, S., Daumé III, H. & Wallach, H. Language (technology) is power: A critical survey of “bias” in NLP. In Proc. 58th Annual Meeting of the Association for Computational Linguistics (eds Jurafsky, D. et al.) 5454–5476 (Association for Computational Linguistics, 2020).
Jørgensen, A., Hovy, D. & Søgaard, A. Challenges of studying and processing dialects in social media. In Proc. Workshop on Noisy User-generated Text (eds Xu, W. et al.) 9–18 (Association for Computational Linguistics, 2015).
Blodgett, S. L., Green, L. & O’Connor, B. Demographic dialectal variation in social media: a case study of African-American English. In Proc. 2016 Conference on Empirical Methods in Natural Language Processing (eds Su, J. et al.) 1119–1130 (Association for Computational Linguistics, 2016).
Jørgensen, A., Hovy, D. & Søgaard, A. Learning a POS tagger for AAVE-like language. In Proc. 2016 Conference of the North American Chapter of the Association for Computational Linguistics: Human Language Technologies (eds Knight, K. et al.) 1115–1120 (Association for Computational Linguistics, 2016).
Blodgett, S. L. & O’Connor, B. Racial disparity in natural language processing: a case study of social media African-American English. Preprint at https://arxiv.org/abs/1707.00061 (2017).
Blodgett, S. L., Wei, J. & O’Connor, B. Twitter universal dependency parsing for African-American and mainstream American English. In Proc. 56th Annual Meeting of the Association for Computational Linguistics (eds Gurevych, I. & Miyao, Y.) 1415–1425 (Association for Computational Linguistics, 2018).
Groenwold, S. et al. Investigating African-American vernacular English in transformer-based text generation. In Proc. 2020 Conference on Empirical Methods in Natural Language Processing (eds Webber, B. et al.) 5877–5883 (Association for Computational Linguistics, 2020).
Ziems, C., Chen, J., Harris, C., Anderson, J. & Yang, D. VALUE: Understanding dialect disparity in NLU. In Proc. 60th Annual Meeting of the Association for Computational Linguistics (eds Muresan, S. et al.) 3701–3720 (Association for Computational Linguistics, 2022).
Davidson, T., Bhattacharya, D. & Weber, I. Racial bias in hate speech and abusive language detection datasets. In Proc. Third Workshop on Abusive Language Online (eds Roberts, S. T. et al.) 25–35 (Association for Computational Linguistics, 2019).
Sap, M., Card, D., Gabriel, S., Choi, Y. & Smith, N. A. The risk of racial bias in hate speech detection. In Proc. 57th Annual Meeting of the Association for Computational Linguistics (eds Korhonen, A. et al.) 1668–1678 (Association for Computational Linguistics, 2019).
Harris, C., Halevy, M., Howard, A., Bruckman, A. & Yang, D. Exploring the role of grammar and word choice in bias toward African American English (AAE) in hate speech classification. In Proc. 2022 ACM Conference on Fairness, Accountability, and Transparency 789–798 (Association for Computing Machinery, 2022).
Gururangan, S. et al. Whose language counts as high quality? Measuring language ideologies in text data selection. In Proc. 2022 Conference on Empirical Methods in Natural Language Processing (eds Goldberg, Y. et al.) 2562–2580 (Association for Computational Linguistics, 2022).
Gaies, S. J. & Beebe, J. D. The matched-guise technique for measuring attitudes and their implications for language education: a critical assessment. In Language Acquisition and the Second/Foreign Language Classroom (ed. Sadtano, E.) 156–178 (SEAMEO Regional Language Centre, 1991).
Hudson, R. A. Sociolinguistics (Cambridge Univ. Press, 1996).
Delobelle, P., Tokpo, E., Calders, T. & Berendt, B. Measuring fairness with biased rulers: a comparative study on bias metrics for pre-trained language models. In Proc. 2022 Conference of the North American Chapter of the Association for Computational Linguistics: Human Language Technologies (eds Carpuat, M. et al.) 1693–1706 (Association for Computational Linguistics, 2022).
Mattern, J., Jin, Z., Sachan, M., Mihalcea, R. & Schölkopf, B. Understanding stereotypes in language models: Towards robust measurement and zero-shot debiasing. Preprint at https://arxiv.org/abs/2212.10678 (2022).
Eisenstein, J., O’Connor, B., Smith, N. A. & Xing, E. P. A latent variable model for geographic lexical variation. In Proc. 2010 Conference on Empirical Methods in Natural Language Processing (eds Li, H. & Màrquez, L.) 1277–1287 (Association for Computational Linguistics, 2010).
Doyle, G. Mapping dialectal variation by querying social media. In Proc. 14th Conference of the European Chapter of the Association for Computational Linguistics (eds Wintner, S. et al.) 98–106 (Association for Computational Linguistics, 2014).
Huang, Y., Guo, D., Kasakoff, A. & Grieve, J. Understanding U.S. regional linguistic variation with Twitter data analysis. Comput. Environ. Urban Syst. 59 , 244–255 (2016).
Eisenstein, J. What to do about bad language on the internet. In Proc. 2013 Conference of the North American Chapter of the Association for Computational Linguistics: Human Language Technologies (eds Vanderwende, L. et al.) 359–369 (Association for Computational Linguistics, 2013).
Eisenstein, J. Systematic patterning in phonologically-motivated orthographic variation. J. Socioling. 19 , 161–188 (2015).
Jones, T. Toward a description of African American vernacular English dialect regions using “Black Twitter”. Am. Speech 90 , 403–440 (2015).
Christiano, P. F. et al. Deep reinforcement learning from human preferences. Proc. 31st International Conference on Neural Information Processing Systems (eds von Luxburg, U. et al.) 4302–4310 (NeurIPS, 2017).
Zhao, T. Z., Wallace, E., Feng, S., Klein, D. & Singh, S. Calibrate before use: Improving few-shot performance of language models. In Proc. 38th International Conference on Machine Learning (eds Meila, M. & Zhang, T.) 12697–12706 (Proceedings of Machine Learning Research, 2021).
Smith, T. W. & Son, J. Measuring Occupational Prestige on the 2012 General Social Survey (NORC at Univ. Chicago, 2014).
Zhao, J., Wang, T., Yatskar, M., Ordonez, V. & Chang, K.-W. Gender bias in coreference resolution: evaluation and debiasing methods. In Proc. 2018 Conference of the North American Chapter of the Association for Computational Linguistics: Human Language Technologies (eds Walker, M. et al.) 15–20 (Association for Computational Linguistics, 2018).
Hughes, B. T., Srivastava, S., Leszko, M. & Condon, D. M. Occupational prestige: the status component of socioeconomic status. Collabra Psychol. 10 , 92882 (2024).
Gramlich, J. The gap between the number of blacks and whites in prison is shrinking. Pew Research Centre https://www.pewresearch.org/short-reads/2019/04/30/shrinking-gap-between-number-of-blacks-and-whites-in-prison (2019).
Walsh, A. The criminal justice system is riddled with racial disparities. Prison Policy Initiative Briefing https://www.prisonpolicy.org/blog/2016/08/15/cjrace (2016).
Röttger, P. et al. Political compass or spinning arrow? Towards more meaningful evaluations for values and opinions in large language models. Preprint at https://arxiv.org/abs/2402.16786 (2024).
Jurafsky, D. & Martin, J. H. Speech and Language Processing: An Introduction to Natural Language Processing, Computational Linguistics, and Speech Recognition (Prentice Hall, 2000).
Salazar, J., Liang, D., Nguyen, T. Q. & Kirchhoff, K. Masked language model scoring. In Proc. 58th Annual Meeting of the Association for Computational Linguistics (eds Jurafsky, D. et al.) 2699–2712 (Association for Computational Linguistics, 2020).
Santurkar, S. et al. Whose opinions do language models reflect? In Proc. 40th International Conference on Machine Learning (eds Krause, A. et al.) 29971–30004 (Proceedings of Machine Learning Research, 2023).
Francis, W. N. & Kucera, H. Brown Corpus Manual (Brown Univ.,1979).
Ziems, C. et al. Multi-VALUE: a framework for cross-dialectal English NLP. In Proc. 61st Annual Meeting of the Association for Computational Linguistics (eds Rogers, A. et al.) 744–768 (Association for Computational Linguistics, 2023).
Download references
Acknowledgements
V.H. was funded by the German Academic Scholarship Foundation. P.R.K. was funded in part by the Open Phil AI Fellowship. This work was also funded by the Hoffman-Yee Research Grants programme and the Stanford Institute for Human-Centered Artificial Intelligence. We thank A. Köksal, D. Hovy, K. Gligorić, M. Harrington, M. Casillas, M. Cheng and P. Röttger for feedback on an earlier version of the article.
Author information
Authors and affiliations.
Allen Institute for AI, Seattle, WA, USA
Valentin Hofmann
University of Oxford, Oxford, UK
LMU Munich, Munich, Germany
Stanford University, Stanford, CA, USA
Pratyusha Ria Kalluri & Dan Jurafsky
The University of Chicago, Chicago, IL, USA
Sharese King
You can also search for this author in PubMed Google Scholar
Contributions
V.H., P.R.K., D.J. and S.K. designed the research. V.H. performed the research and analysed the data. V.H., P.R.K., D.J. and S.K. wrote the paper.
Corresponding authors
Correspondence to Valentin Hofmann or Sharese King .
Ethics declarations
Competing interests.
The authors declare no competing interests.
Peer review
Peer review information.
Nature thanks Rodney Coates and the other, anonymous, reviewer(s) for their contribution to the peer review of this work.
Additional information
Publisher’s note Springer Nature remains neutral with regard to jurisdictional claims in published maps and institutional affiliations.
Extended data figures and tables
Extended data fig. 1 weighted average favourability of top stereotypes about african americans in humans and top overt as well as covert stereotypes about african americans in language models (lms)..
The overt stereotypes are more favourable than the reported human stereotypes, except for GPT2. The covert stereotypes are substantially less favourable than the least favourable reported human stereotypes from 1933. Results without weighting, which are very similar, are provided in Supplementary Fig. 6 .
Extended Data Fig. 2 Prestige of occupations associated with AAE (positive values) versus SAE (negative values), for individual language models.
The shaded areas show 95% confidence bands around the regression lines. The association with AAE versus SAE is negatively correlated with occupational prestige, for all language models. We cannot conduct this analysis with GPT4 since the OpenAI API does not give access to the probabilities for all occupations.
Supplementary information
Supplementary information, reporting summary, rights and permissions.
Open Access This article is licensed under a Creative Commons Attribution 4.0 International License, which permits use, sharing, adaptation, distribution and reproduction in any medium or format, as long as you give appropriate credit to the original author(s) and the source, provide a link to the Creative Commons licence, and indicate if changes were made. The images or other third party material in this article are included in the article’s Creative Commons licence, unless indicated otherwise in a credit line to the material. If material is not included in the article’s Creative Commons licence and your intended use is not permitted by statutory regulation or exceeds the permitted use, you will need to obtain permission directly from the copyright holder. To view a copy of this licence, visit http://creativecommons.org/licenses/by/4.0/ .
Reprints and permissions
About this article
Cite this article.
Hofmann, V., Kalluri, P.R., Jurafsky, D. et al. AI generates covertly racist decisions about people based on their dialect. Nature (2024). https://doi.org/10.1038/s41586-024-07856-5
Download citation
Received : 08 February 2024
Accepted : 19 July 2024
Published : 28 August 2024
DOI : https://doi.org/10.1038/s41586-024-07856-5
Share this article
Anyone you share the following link with will be able to read this content:
Sorry, a shareable link is not currently available for this article.
Provided by the Springer Nature SharedIt content-sharing initiative
By submitting a comment you agree to abide by our Terms and Community Guidelines . If you find something abusive or that does not comply with our terms or guidelines please flag it as inappropriate.
Quick links
- Explore articles by subject
- Guide to authors
- Editorial policies
Sign up for the Nature Briefing: AI and Robotics newsletter — what matters in AI and robotics research, free to your inbox weekly.

- Open access
- Published: 27 August 2024
Genetic factors, risk prediction and AI application of thrombotic diseases
- Rong Wang 1 ,
- Liang V. Tang 1 , 2 &
- Yu Hu 1 , 2
Experimental Hematology & Oncology volume 13 , Article number: 89 ( 2024 ) Cite this article
18 Accesses
Metrics details
In thrombotic diseases, coagulation, anticoagulation, and fibrinolysis are three key physiological processes that interact to maintain blood in an appropriate state within blood vessels. When these processes become imbalanced, such as excessive coagulation or reduced anticoagulant function, it can lead to the formation of blood clots. Genetic factors play a significant role in the onset of thrombotic diseases and exhibit regional and ethnic variations. The decision of whether to initiate prophylactic anticoagulant therapy is a matter that clinicians must carefully consider, leading to the development of various thrombotic risk assessment scales in clinical practice. Given the considerable heterogeneity in clinical diagnosis and treatment, researchers are exploring the application of artificial intelligence in medicine, including disease prediction, diagnosis, treatment, prevention, and patient management. This paper reviews the research progress on various genetic factors involved in thrombotic diseases, analyzes the advantages and disadvantages of commonly used thrombotic risk assessment scales and the characteristics of ideal scoring scales, and explores the application of artificial intelligence in the medical field, along with its future prospects.
Introduction
Thrombotic disease is a systemic condition that encompasses venous thrombosis, arterial thrombosis, and intracardiac thrombosis. Venous thromboembolism (VTE) can be categorized as deep vein thrombosis (DVT) and pulmonary embolism (PE). Vascular endothelial cells secrete coagulation, anticoagulation, and fibrinolysis substances, along with corresponding regulatory molecules, which are tightly regulated in the blood vessels. Maintaining this delicate balance ensures smooth blood flow and preserves clotting potential. Acquired risk factors for thrombosis include certain medications, venous catheterization, surgery, prolonged immobilization, advanced age, and malignancies. Disruption of this balance by these factors can induce a hypercoagulable state, endothelial injury, or blood stasis, thereby increasing the risk of thrombosis (shown in Fig. 1 ). Genetic elements also play pivotal roles in disrupting this balance and promoting abnormal coagulation processes. Patients with a family history of thrombosis may opt for genetic screening, as observed, 45% to 60% of VTE events are associated with prothrombotic genotypes [ 1 ]. However, the decision lies with the patients, as clinicians do not recommend or guide unnecessary tests for diagnosis and treatment. Common clinical thrombosis susceptibility genes primarily include mutations in coagulation factors, coagulation inhibitors, the protein C system, the fibrinolytic system, and other genes outside the blood system.
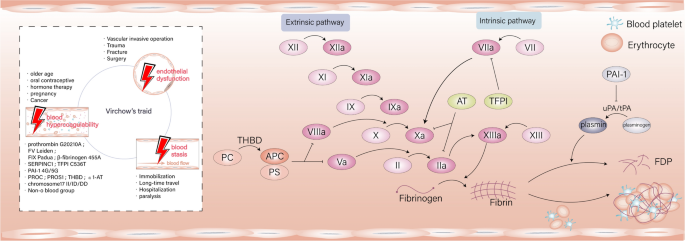
The Virchow’s triad and the coagulant-anticoagulant-fibrinolytic system. The Virchow triad consists of vascular endothelial dysfunction, blood hypercoagulability and blood stasis. The risk factors of thrombotic diseases are divided into two categories: acquired and hereditary, as shown above. Hereditary factors mainly lead to hypercoagulable state. The balance of coagulation, anticoagulation and fibrinolysis makes the blood flow smoothly in the blood vessel and has the potential ability of thrombosis. Changes in the antigenic level or activity of any substance in this balance are likely to break the balance and lead to thrombosis
Thrombotic disease is considered the primary cause of mortality worldwide and can manifest as an independent condition or as a secondary complication of specific diseases. In clinical practice, individuals with risk factors for thrombosis undergo routine thrombosis risk assessment and stratification. The most frequently employed tool is Risk Assessment Models (RAMs), which incorporate patient symptoms, signs, and risk factors to assign corresponding scores. However, these scales are often developed based on population-specific data from a particular institution or region, limiting their generalizability. Additionally, subjective factors of clinicians may introduce heterogeneity in the practical application of these scales.
With the development of artificial intelligence (AI), a growing number of applications in the field of medicine and health have emerged. These applications include, but are not limited to, the establishment of risk assessment models, assisting clinical diagnosis, guiding clinical decision-making, drug target screening, and patient management. Computer vision imaging can enhance the diagnostic accuracy of medical images and reduce the occurrence of false positive and false negative results [ 2 ]. Natural language processing can facilitate the standardization of electronic medical records, enabling convenient data screening in multi-center clinical studies. The use of auxiliary robots improves the success rate of precision procedures. Machine learning algorithms, such as deep learning, decision trees, and neural networks, can extract meaningful variables from big data to construct clinical models [ 3 , 4 , 5 ]. While retrospective studies have demonstrated the superiority of models based on large-scale real-world data compared to those based solely on clinical data, they are still insufficient to support clinical translation. This article primarily focuses on different forms of hereditary thrombophilia mutations across various systems, including classical mutations and newly discovered mutation sites. Additionally, it introduces commonly used thrombus scoring scales in internal medicine, oncology, and surgical patients, analyzing and comparing their advantages and disadvantages. Finally, it discusses the current application of AI in thrombotic diseases, the challenges faced at this stage, and potential directions for future development.
Genetic factors of thrombotic diseases
The physiological anticoagulant mechanisms in the body can be primarily categorized into three groups: serine protease inhibitors, the protein C system, and tissue factor pathway inhibitors. Any increase in the plasma antigen level or activity of coagulation factors, a lack of anticoagulant substances, or low levels of fibrinolytic enzymes can significantly increase the likelihood of thrombosis. Genetic factors related to thrombotic diseases exhibit regional and ethnic differences. Multiple meta-analyses have revealed that protein C, protein S, and antithrombin deficiency are the most common genetic mutations found in East Asian populations, while coagulation factor V and prothrombin mutations are the primary mutations in Caucasian populations [ 6 , 7 ]. This disparity may result from the combined effects of race and region, providing a background and basis for the focus of genetic screening in different populations to improve the efficiency of genetic counseling. Performing genetic testing for suspected hereditary thrombophilia not only aids in evaluating the prognosis of patients with thrombosis but also facilitates the discovery of carriers within thrombophilia families.
Abnormal activation of coagulation cascade
Coagulation cascades can be classified into endogenous and exogenous pathways. Genetic mutations in various coagulation factors have been found to be associated with thrombotic events (shown in Fig. 2 ). Prothrombin, the zymogen form of thrombin, acts as a proteolytic enzyme that hydrolyzes multiple coagulation factors, promotes the coagulation cascade reaction, and converts soluble fibrinogen into a fibrin network, facilitating the cross-linking of red blood cells and platelets. Additionally, prothrombin can participate in the activation of protein C and exert anticoagulant activity.
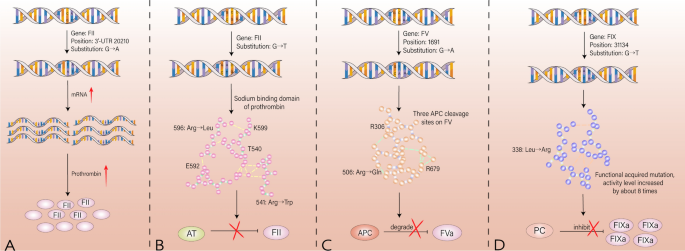
Coagulation factor mutations lead to thrombosis. A Most of the mutations in prothrombin are single nucleotide substitutions (G20210A) at 20210 in the 3 ' non-coding region, resulting in abnormal 3 ' -terminal cleavage signals, accumulation of transcribed RNA, and corresponding increase in prothrombin produced at the translation level. B Prothrombin is a sodium-regulated allosteric enzyme. The sodium-binding domain consists of five amino acid residues: Thr540, Arg541, Glu592, Arg596 and Lys599.Single nucleotide substitution caused by mutations in this site easily leads to antithrombin resistance. C Arg506 is a kinetically favorable APC cleavage site, and the missense mutation at position 1691 causes Arg to be replaced by Gln (FV Leiden). On the one hand, FVa continues to express procoagulant activity, on the other hand, it resists the cleavage of APC, causing APC resistance-related thrombosis events. D The FIX R338L mutation was a gain-of-function mutation (FIX Padua), and the plasma level of FIX was normal, while the activity level was increased by about 8 times. At the same time, the stability of the combination with PS decreased, and the inhibition of PS decreased, showing a clear tendency of thrombosis
Most prothrombin mutations are single nucleotide substitutions occurring at position 20210 in the 3' non-coding region. These mutations result in abnormal 3'-terminal cleavage signals, leading to an accumulation of transcribed RNA and an increase in prothrombin production at the translation level [ 8 , 9 , 10 ]. Co-transfection experiments have confirmed that these mutations do not affect the molecular structure but create a more efficient cleavage site compared to the wild type, thereby increasing the efficiency of 3' end processing [ 11 ]. Heterozygous mutations of prothrombin lead to an approximately 30% increase in plasma levels, while homozygous mutations result in around a 70% increase. Even at the same plasma level, carriers of the mutant alleles produce more thrombin and exhibit activated protein C (APC) resistance compared to non-carriers [ 12 ]. This mutation also enhances the activation of thrombin-activated fibrinolysis inhibitor in a concentration-dependent manner, resulting in prolonged plasma clot lysis time [ 13 ].
In a multicenter study on portal vein thrombosis [ 14 ], it was found that the frequency of cytomegalovirus (CMV) positive patients with the G20210A gene mutation is higher, indicating a potential synergistic effect on thrombosis. However, the temporal relationship between CMV infection and the occurrence of the gene mutation needs to be confirmed through prospective studies [ 15 , 16 ]. Based on this finding, further investigations are warranted to determine whether similar associations exist when CMV is combined with other coagulation factor mutations.
Recent studies have made new discoveries in the pathogenesis of prothrombin gene mutation (PGM). Prothrombin is an allosteric enzyme that is regulated by sodium and has a sodium-binding domain consisting of five amino acid residues: Thr540, Arg541, Glu592, Arg596, and Lys599. Single nucleotide substitutions caused by mutations at this domain can lead to antithrombin resistance [ 17 ].
Arginine at position 596 is an important site for regulating thrombin activity. Replacing it with leucine may slightly reduce activity but greatly reduce antithrombin binding ability, leading to antithrombin resistance and increased susceptibility to thrombosis. Through genome-wide association analysis and whole-exome sequencing (WES), Mulder et al. [ 18 ] found that replacing the arginine residue at position 541 with tryptophan made prothrombin resistant to antithrombin. Building on this finding, Wu et al. [ 19 ] discovered that the mutation was accompanied by a decrease in prothrombin procoagulant activity. More significantly, the mutation impaired protein C (PC) activation, and the combined effect ultimately biased PGM towards procoagulant activity. Arg541Trp mainly impairs the binding affinity of PC, resulting in low anticoagulant efficiency of activated protein C (APC), but does not affect the interaction between thrombin and thrombomodulin. This finding suggests that beyond common hereditary PC, PS defects, and coagulation factor V Leiden mutations, inefficient PC pathway activation caused by prothrombin abnormalities may contribute to activation defects in the PC pathway. Although the mechanism of this effect has yet to be fully elucidated, this study sheds new light on the mechanism of thrombosis.
Nonsense mutations in the prothrombin gene can lead to the formation of prothrombotic phenotypes, in addition to single nucleotide substitutions. A CGC > CGT codon replacement (c.1824C > T) in the last exon of the prothrombin gene results in an unchanged protein product, but increased mRNA expression [ 20 ]. Computer prediction shows that there may be secondary structure recombination, prolonging half-life and increasing quantity. In vitro studies observed hyperprothrombinemia, hypofibrinolysis, and dense fibrin clots with impaired fibrinolytic function. However, routine hemostatic tests in the laboratory could not verify the procoagulant state because no correlation was observed between the increase in plasma concentration and activity. This separation between concentration and activity also exists in G20210A [ 21 ]. Prior to this report, synonymous single nucleotide polymorphisms were seldom linked to diseases, with amino acid sequences being considered clinically insignificant. This report reveals that changes in codons may affect the effectiveness of proteins in terms of quantity, structure, and function, despite the transcripts being the same. This provides new ideas for clinical diagnosis and treatment. When common genetic factor screening and laboratory tests are negative and there is no apparent cause of thrombosis, it is possible to consider whether it is caused by synonymous mutations.
Coagulation factor V (FV) functions as both a procoagulant and anticoagulant factor in physiological processes. During coagulation, FVa acts as a cofactor to enhance the activation of prothrombin by coagulation factor X (FXa). In anticoagulation, FV serves as a cofactor for tissue factor pathway inhibitor (TFPIa) and activated protein C (APC) to exert anticoagulant activity [ 22 , 23 , 24 , 25 , 26 , 27 ]. There are three cleavage sites in FV for APC, namely Arg306, Arg506, and Arg679. APC catalyzes the cleavage of the Arg506 site, producing an intermediate with partial activity, and subsequently cleaves the Arg306 site to completely inactivate FVa. This results in the loss of FXa activity and inhibition of thrombin production. In addition, TFPIa and protein S (PS) also play a certain role in promoting decomposition [ 28 , 29 , 30 , 31 ]. The missense mutation at position 1691 of the FV gene (G to A substitution at base position 1691) leads to the substitution of Arg by Gln, known as the FV Leiden (FVL) mutation. Due to the Arg506 site is a favored cleavage site kinetically, FVa continues to express procoagulant activity and becomes resistant to APC cleavage, leading to APC resistance-related thrombotic events [ 32 , 33 , 34 ].
Different from conventional FV deficiency related to bleeding events, Castoldi et al. [ 35 ] reported recurrent venous thrombosis in a patient with FV deficiency. Whole exome sequencing found a novel FV missense mutation (Ala2086Asp, FV Besançon) resulting in an amino acid substitution in the FV C2 domain and reduced plasma levels. Although a small amount of FV is insufficient to exert the cofactor activity of APC and TFPI, it is still sufficient to activate prothrombin and maintain the coagulation reaction [ 36 , 37 ]. In addition, similar effects have been observed in the FV Nara (Trp1920Arg) mutation [ 38 ]. This finding also highlights to clinicians that laboratory indicators alone may not fully reflect the clinical phenotype of patients with genetic mutations and therefore may not predict their expected outcomes accurately. The dosing regimen should not solely rely on laboratory indicators, but also consider the patient's clinical manifestations, imaging results, and other factors, to optimize their treatment and prevent potential complications. Overall, this highlights the importance of genetic screening for patients with suspected gene mutations.
Approximately 90% of FV mutations are FV Leiden (FVL). Mutations in FVL and prothrombin are the most common genetic factors associated with thrombophilia among individuals of Caucasian descent. The prevalence of heterozygous single-gene mutations is higher than that of homozygous and compound heterozygous individuals. In recent years, researchers have increasingly focused on the clinical distinctions between homozygous, heterozygous, and compound mutations, but consensus has not yet been reached. Botero et al. [ 39 ] found no significant difference in the incidence and recurrence rate of venous thromboembolism (VTE) between homozygous and heterozygous carriers of FVL. This may be attributed to the small number of homozygous carriers included in the study, introducing analysis bias. When individuals carry compound heterozygous mutations, there may be a synergistic effect, which increases the risk of thrombosis. In a study on tumor-associated thrombotic events, the incidence of homozygous or double heterozygous carriers of FV and FII gene mutations was significantly higher than that of single heterozygous mutations [ 40 ]. In a recent study on the double heterozygosity (DH) genotype for factor V Leiden and prothrombin G20210A, it was found that the DH genotype may occur as frequently as the FVL genotype and potentially increase the risk of developing VTE similarly [ 41 ]. In general, there are still several limitations in current research in this field. These confounding factors may affect the true outcomes, and the limited number of samples of homozygotes and compound heterozygotes introduces potential bias in data analysis [ 42 ]. Therefore, conducting a large-scale randomized controlled study with exclusion of clinical incentives is recommended as the optimal research approach. In clinical practice, it is also recommended to utilize activated protein C (APC) resistance testing in conjunction with DNA testing to identify FV Leiden and eliminate potential confounding factors that may obscure true results.
In recent years, other factors have gained increasing attention in the study of thrombotic diseases. The role of factor XI mutation is yet to be elucidated, and its clinical significance remains unknown. A gain-of-function mutation in the FIX gene at position 338, with arginine replacing leucine, was identified in a patient with thrombophilia in adolescence (FIXR338L, FIX Padua) [ 43 ]. Despite normal plasma levels of FIX, individuals with this mutation exhibit approximately 8 times higher activity levels than those with the wild type. Based on this finding, it was suggested that the stability of FIXaR338L binding to PS decreased, and the ability of PS to inhibit FIXaR338L decreased, suggesting an increased risk of thrombosis [ 44 ]. In vitro studies have shown that supplementation of exogenous PS improves the patient's condition, providing new ideas for the treatment of FIX-related thrombophilia. The 1813 site in the A3 subunit of FVIII is responsible for binding to FIXa and dissociation of the A2 subunit from FIXa. The K1813A mutation enhances the activity and structural stability of the FVIII cofactor, resulting in significantly increased coagulation potential [ 45 ]. Although this mutation is considered to be useful for protein and gene therapy in patients with hemophilia A, its role in contributing to thrombotic events remains to be studied. Individuals carrying the β-fibrinogen 455A allele exhibit elevated plasma fibrinogen levels and an increased risk of arterial thrombosis, which is more common in the Asian population [ 46 , 47 ]. This allele significantly associates with susceptibility to cardiogenic stroke, ischemic stroke, and coronary heart disease.
Coagulation inhibitor abnormalities
Antithrombin (AT) is a glycoprotein synthesized by hepatocytes and vascular endothelial cells, functioning as a major component of the natural anticoagulant system. Under normal circumstances, AT has minimal direct anticoagulant effect due to the absence of heparin in plasma [ 48 ]. However, during the process of anticoagulation, heparin binds to the heparin-binding region at the N-terminus of AT, inducing a conformational change and exposing the reactive central loop (RCL). This results in an approximately 2000-fold increase in the anticoagulant activity of AT. The C-terminal protease inhibitory active region of AT then covalently binds to the serine residue of the thrombin active center, forming a stable heparin-AT-thrombin complex. This complex weakens or completely inhibits the activity of thrombin. Subsequently, heparin dissociates from the complex, allowing it to recombine with another molecule of AT for repeated use. In addition to its effects on thrombin, AT also inhibits other coagulation factors such as FXIa, Xa, XIa, and XIIa, all of which are serine proteases. Furthermore, AT promotes the dissociation of the coagulation factor FVIIa-tissue factor complex, inhibiting the initiation of the exogenous coagulation pathway.
The incidence of antithrombin deficiency ranges from about 0.02% to 0.2%, increasing the susceptibility to thrombosis. The first report of a family with congenital antithrombin deficiency thrombophilia was by Egeberg in 1965. Since then, studies have been conducted on families with hereditary antithrombin deficiency. Over 300 mutations have been identified, with 80% of antithrombin defects caused by mutations in the SERPINC1 gene. Mutation types include point mutations, splicing, and small fragment insertion/deletion, with over 90% being point mutations. Missense/nonsense mutations account for 55.0% of all mutations [ 49 ].
AT defects are typically categorized into two types based on activity and plasma antigen levels. Type I, known as the classical defect type, is characterized by a disorder in AT synthesis, resulting in a parallel decrease in activity and plasma antigen levels. Type II is merely characterized by reduced AT activity (shown in Fig. 3 ). Within Type II defects, there are three subtypes based on different mutation sites: reaction site defect type II (II/RS), heparin binding site defect type II (II/HBS), and pleiotropic defect type II (II/PE). The specific types of SERPINC1 mutations and the different subtypes of AT deficiency can influence the clinical phenotype of hereditary AT deficiency to some extent, particularly in terms of varying risks and locations of thrombosis. For example, SERPINC1 missense mutations are associated with a lower risk of venous thromboembolism, and nearly all type II AT deficiencies are caused by missense mutations. Although the risk of venous thromboembolism is lower in type II HBS AT deficiency compared to other types, these patients have a higher risk of arterial thromboembolism [ 50 ].
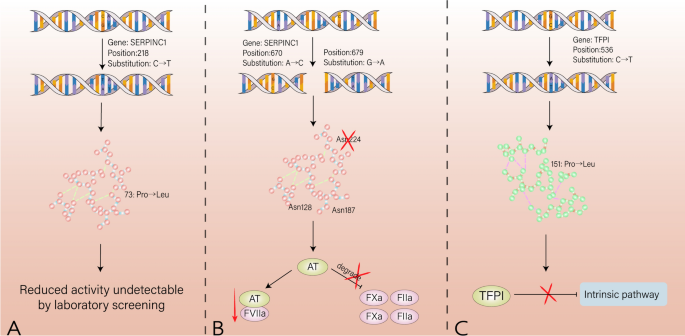
Thrombosis caused by abnormal coagulation inhibitors. A 80% of the antithrombin deficiency is caused by SERPINC1 gene mutation. B In addition, N -glycosylation defects can lead to a decrease in its anti-FXa and anti-FIIa activity. C Tissue factor pathway inhibitor (TFPI) can specifically inhibit the tissue factor pathway and hinder the initiation of coagulation reaction. Single nucleotide substitution (C536T) in exon 7 will lead to a relative increase in the risk of venous thrombosis
Transient antithrombin (AT) deficiency is a newly defined concept, referring to the clinical effects caused by mutant genes at specific time points due to external factors [ 51 ]. Patients with this type of deficiency have a higher incidence of arterial thrombosis events. AT, being a glycoprotein, possesses four N-glycosylation sites (Asn128, Asn167, Asn187, Asn224). The majority of plasma AT is fully glycosylated (α-AT, 90%), while a small portion exhibits a deficiency in the Asn167 glycosylation side chain (β-AT, 10%). Due to its high affinity for heparin, β-AT becomes the primary coagulation inhibitor in the body in a low-abundance form [ 52 , 53 ]. N -glycosylation defects can also cause antithrombin deficiency, suggesting the existence of a new mechanism of thrombophilia. When a missense mutation is located close to or within the N -glycosylation sequence, it impairs the glycosylation of Asn224 and leads to decreased anti-FXa and anti-FIIa activity of β-AT. Since β-AT constitutes only a small proportion in plasma, conventional laboratory tests primarily screen the ability of AT to inhibit exogenous FIIa or FXa, resulting in the under-recognition of this defect. However, the level of FVIIa-AT complex decreased, the exogenous coagulation pathway was significantly enhanced, and the plasma showed a hypercoagulable state (shown in Fig. 3 ). Furthermore, glycosylation defects alter the folding form and stability of AT, making it more susceptible to external conditions such as temperature and alcohol. This clinical manifestation can be observed as transient antithrombin deficiency[ 51 ]. The incidence and severity of thrombosis in these patients are lower than those with continuous deficiency, but arterial thrombosis events are relatively common.
The tissue factor pathway inhibitor (TFPI) plays a crucial role in inhibiting the tissue factor pathway and hindering the initiation of coagulation reactions. A single nucleotide substitution (C536T) on exon 7 results in the substitution of proline by leucine (Pro151Leu) at amino acid 151 of the protein, which increases the risk of venous thrombosis (shown in Fig. 3 ). Although the biochemical regulation of TFPI is obvious, the function or concentration determination of its plasma level cannot accurately reflect the defects of TFPI [ 54 ]. There is also no evidence indicating clinical relevance of such mutations. Therefore, the clinical significance of TFPI defects remains to be elucidated.
Abnormal inactivation of fibrinolytic system
Plasminogen activator inhibitor-1 (PAI-1) serves as the primary inhibitor of endogenous plasminogen activators, namely tissue plasminogen activator (tPA) and urokinase (uPA), effectively impeding the dissolution of fibrin clots. Under physiological conditions, PAI-1 assumes responsibility for regulating fibrinolytic activity and maintaining a delicate balance between fibrinolysis and coagulation within the body. Thorough investigations into the coding genes of PAI-1 have predominantly focused on the -675 4G/5G polymorphism found in the gene's promoter region. Notably, the presence of the 4G allele significantly elevates transcription levels, protein expression, and PAI-1 activity (shown in Fig. 4 ). While some studies argue against any correlation between the 4G allele and coronary heart disease or myocardial infarction, with no observed prothrombotic effects in FVL carriers [ 55 , 56 ], Balta et al. [ 57 ] have reported a distinct association linking 4G allele carriers to visceral vascular thrombosis, particularly portal vein thrombosis. Furthermore, continuous monitoring of PAI-1 activity and antigen levels in patients undergoing anticoagulant therapy after experiencing deep vein thrombosis (DVT) revealed a substantial increase in PAI-1 activity among carriers of the 4G allele, accompanied by a relative surge in thrombosis frequency [ 58 ]. A comprehensive meta-analysis has pinpointed the polymorphism within the PAI-1 promoter region as a susceptibility locus for thrombosis [ 59 ], potentially influenced by the regulation of lipid metabolism or interactions with other genetic mutations involved in thrombosis. Inconsistencies or even contradictory outcomes across different studies may be attributed to variations in population, limited sample sizes, or divergent susceptibilities under different disease states. Due to the heterogeneity of these findings, the use of PAI-1 detection in clinical practice is not yet viable. Further research is needed to clarify its potential for independent cardiovascular event prediction and accurate prognosis forecasting. Recent discoveries have shed light on the involvement of polymorphisms within the 3'-UTR region of the PAI-1 gene, further enhancing our comprehension of PAI-1 gene polymorphisms and their implications in stroke risk regulation [ 60 ].
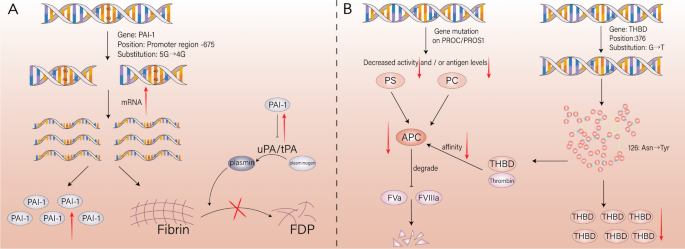
Thrombosis caused by abnormalities of fibrinolytic system and protein C system. A The carrying of 4G gene in the promoter region of PAI-1 coding gene increased the transcription level, protein expression and activity of PAI-1, and inhibited the dissolution of fibrin clot. B Mutations in protein C and protein S coding genes cause decreased activity and/or decreased antigen levels. Mutations in thrombomodulin will lead to a decrease in the affinity of the thrombin complex to protein C and a delay in the co-activation effect
Protein C system abnormalities
The protein C system mainly includes protein C (PC), protein S (PS), thrombomodulin (THBD), endothelial protein C receptor (EPCR), activated protein C receptor (APCR), and protein C inhibitor (PCI). Synthesized in the liver, protein C initially exists in the plasma as an inactive zymogen. Thrombin binds to THBD on the vascular endothelial cell membrane to form a thrombin-THBD complex. The procoagulant activity of thrombin is masked and neutralized. Thrombin degrades PC to form activated protein C (APC), and EPCR and APCR can enhance the activation [ 61 ]. APC inactivates FVIIIa and FVa with the help of the non-enzymatic cofactor PS, limiting the coagulation reaction [ 62 ]. APC was cleared by PCI, α1-antitrypsin (α1-AT), α2-macroglobulin (α2-MG) and α2-antiplasmin (α2-AP). At present, there is no clear conclusion on the specific mechanism of how PS enhances PC, but it is assumed that PS binds to the Gla and EGF1 domains of APC on the surface of a high-affinity phospholipid membrane to stabilize its active conformation. In addition, PS can enhance the inhibition of FXa activity by TFPI, and even PS containing zinc ions can directly bind to tissue factor and inhibit the production of FXa [ 63 ].
Although rare, genetic defects in PC and PS serve as independent risk factors for VTE along with FV Leiden, suggesting a predisposition to severe thrombophilia (shown in Fig. 4 ). These genetic defects in the protein C (PROC) and protein S (PROS1) encoding genes are polymorphic, encompassing gene polymorphisms, plasma phenotypic variations, and clinical phenotypic variations. Studies have identified heterozygous mutations in the protein C system, while homozygous mutations are rare and typically associated with neonatal fulminant purpura, a life-threatening condition. Hotspot mutations such as Glu67Ala, Arg561Trp, and Tyr560* have been observed in the Chinese population, whereas Lys196Glu is common in the Japanese population [ 64 , 65 ]. Therefore, there are variations in genetic factors related to coagulation not only between the Caucasian and East Asian populations, but also within the same population.
As a protease, protein C (PC) is composed of four main domains: Gla, EGF1, EGF2, the activating peptide and the enzymatic serine protease domain. Recent studies have revealed a series of mutations in genes encoding PC, which disrupt its stable structure and subsequently reduce its anticoagulant activity. PC deficiency can be categorized into two types. Type I deficiency is characterized by mutations in the Gla or serine protease domain, leading to decreased levels of both antigen and activity, such as p. Ala178Pro and p.Pro275Ser. Type II deficiency is characterized by reduced activity levels only, such as p. Arg189Trp and P.Lys193del. Type II deficiency can be further subdivided into type IIa, caused by mutations at the APC enzyme cleavage site, and type IIb, caused by mutations at other functional sites that affect the interaction between PC and other molecules. Similarly, different mutations in protein S (PS) result in distinct plasma phenotypes. Quantitative defects in PS can occur throughout the entire PROS1 region, while qualitative defects are typically limited to the Gla and EGF-like domains. It is hypothesized that the active center of PS is primarily located within the Gla and EGF domains.
According to statistical data, around half of individuals carrying protein S (PS) mutations experience clinical symptoms, with the majority of these symptoms manifesting before the age of 55. Notably, patients with mutations in the SHBG-like domain tend to develop thrombosis at an earlier age [ 66 ]. Zhang et al. [ 67 ] found a frameshift mutation (c.74dupA) of PROS1, and the proband exhibited hypoxia induction in comparison to asymptomatic carriers within the same family. Based on these findings, it is speculated that this mutation does not display a procoagulant tendency when present in isolation, and its pathogenicity may be influenced by the presence of other inducing factors.
Thrombomodulin (thrombomodulin, THBD) comprises an N-terminal C-type lectin-like domain, six epidermal growth factor (EGF)-like modules, and a serine/threonine-rich region. During anticoagulation, the EGF-like structure binds to thrombin and alters its substrate specificity, transitioning from a procoagulant to an anticoagulant state. In addition to functioning as a cofactor for protein C, thrombomodulin can activate thrombin to promote the activation of fibrinolysis inhibitors and degrade inflammation-related high mobility group box 1 (HMGB1), thereby participating in fibrinolysis and anti-inflammatory processes. Previous studies have primarily focused on mutations within the EGF-like and serine/threonine-rich domains of thrombomodulin. More recently, several studies have demonstrated a close relationship between single nucleotide polymorphisms in the THBD gene and thrombotic diseases [ 68 , 69 , 70 , 71 , 72 ]. Furthermore, heterozygous mutations in the C-type lectin domain (c.376G > T) result in the substitution of aspartic acid with tyrosine at residue 126, altering the molecular conformation of thrombomodulin and leading to decreased plasma antigen levels accompanied by functional defects. This mutation reduces the affinity between the thrombin-thrombomodulin complex and protein C while also delaying the co-activation effect of protein C, thereby increasing susceptibility to thrombosis (shown in Fig. 4 ). Clinically, patients with this mutation experience recurrent arterial and venous thrombosis [ 73 ].
In recent years, there has been growing interest in understanding the relationship between mutations in other molecules within the protein C system and thrombotic diseases. One notable example is the homozygous mutation of α1-antitrypsin Z (Glu342Lys), which has shown a 2.2-fold correlation with venous thromboembolism (VTE) [ 74 , 75 , 76 ]. This correlation is comparable to that of the prothrombin G20210A mutation. However, the specific mechanism underlying this correlation remains to be elucidated [ 76 ]. In summary, while protein C serves as a core molecule, various coagulation-related substances indirectly contribute to promoting thrombosis by affecting the function of protein C. Therefore, further exploration of protein C's role in the blood system is necessary.
Other factors
Abnormalities in the renin–angiotensin–aldosterone system, which regulates vascular contraction and intimal hyperplasia, may also lead to thromboembolism. The renin gene on chromosome 17 has an insertion (I)/deletion (D) polymorphism, resulting in three genotypes: II, ID, and DD. Many studies have observed that the DD genotype is associated with a high risk of thrombosis. In patients undergoing dialysis, carriers of the DD genotype have increased levels of PAI-1, resulting in a state of reduced fibrinolysis and a significantly increased risk of thrombosis [ 77 ]. However, the relationship between these factors has not been fully elucidated, and a prospective, multicenter study with a large sample size is needed to establish a definitive relationship. Additionally, differences in the incidence of VTE have also been observed based on different blood types among individuals, with a significant increase in the risk of VTE when FVL coexists with non-O blood types [ 78 ].
Pulmonary embolism (PE) patient with thrombophilia have a tendency to develop deep vein thrombosis, particularly in patients with deficiencies in PC and PS [ 79 ]. Therefore, it is highly significant for the clinical prognosis to identify such inherited disorders and provide appropriate preventive measures. Given the high cost of genetic testing, it is not recommended for all PE patients to undergo genetic screening. The focus should be on individuals with a family history or those who are not predisposed to PE. In a prospective family cohort study [ 80 ], individuals with inherited deficiencies of natural anticoagulants (AT, PC, PS) had a nearly five times higher risk of arterial thrombosis compared to those without these deficiencies, with young individuals exhibiting a higher susceptibility. Therefore, early, timely, and effective identification of genetic factors associated with thrombotic disorders can help prevent thrombosis, improve patient prognosis, and avoid adverse events. This requires the development of high-throughput, high-sensitivity detection technologies.
Clinical commonly used risk prediction scale
While not all mutant carriers experience thrombotic events, the presence of acquired risk factors and environmental factors can significantly increase their probability. Common risk factors for venous thromboembolism (VTE) include male gender, diabetes, obesity, smoking, mutations, use of oral contraceptives and hormone replacement therapy, long-distance flights, severe acute respiratory distress syndrome, coronavirus type 2 infection, trauma and fractures, pregnancy, immobilization, antiphospholipid syndrome, surgery, and cancer. Currently, anticoagulant therapy is not recommended for mutation carriers. However, for patients with risk factors, it is crucial to have an effective risk assessment system in place for predicting and stratifying risks. This would enable doctors to identify high-risk patients promptly and initiate timely anticoagulant therapy, while also avoiding unnecessary anticoagulation in low-risk patients to prevent bleeding events. We reviewed the risk assessment models (RAMs) commonly used in clinical practice, and summarized the application conditions, advantages and limitations. These models are only applicable to hospitalized surgical and medical patients or ambulatory patients with cancer prior to chemotherapy (shown in Table 1 ). On this basis we outline the qualities that an ideal assessment model should have.
Surgical inpatients
The Caprini risk assessment scale has long been used for evaluating the risk of venous thromboembolism (VTE) in surgical patients. Originally developed in 1991, the scale underwent revisions in 2005 and 2010 to create a more personalized risk assessment approach [ 81 ]. Consisting of approximately 37 risk factors, each assigned a weight of 1–5 points, the scale categorizes patients into four risk groups based on their total score. Correspondingly, recommended therapies are provided for each group. Periodic reassessments are conducted at specific intervals. In an orthopedic patient cohort, a prospective study evaluated the predictive ability of the modified 2005 and 2010 versions of the Caprini scale for deep vein thrombosis (DVT) upon admission. The study found that the 2005 version demonstrated higher sensitivity compared to the 2010 version, while specificity remained consistent [ 84 ]. However, it is important to note that this study did not objectively verify the presence of DVT using imaging techniques (such as Doppler ultrasonography) for all patients. Additionally, it did not evaluate the dynamic changes in risk factors from the time of surgery to the time of discharge, potentially overlooking the influence of new factors that may arise during this period.
Lobastov et al. [ 85 ] conducted a meta-analysis to investigate the relationship between the Caprini risk score threshold and the occurrence of venous thromboembolism (VTE). The results revealed that a score of 7–11 points had significant predictive value for VTE occurrence. However, similar positive results were not observed in obstetrics, bariatric surgery, neurosurgery, and transplant patient populations. The lack of consistent results may be attributed to the unique characteristics of these diseases and the importance of effective patient management strategies. In another prospective cohort study, the reliability and effectiveness of a modified Caprini score were evaluated. This revised version included an ultra-high-risk group, defined by a score exceeding 8 points. Patients with scores higher than 8 points were found to have a significantly increased risk of developing VTE, which was consistent with the findings reported by Pannucci and Zhou in their respective studies [ 86 , 87 , 88 ]. These results highlight the need for detailed stratification of high-risk patients to more accurately assess their level of risk. Interestingly, these findings differ from those reported in Western populations by Caprini. It is assumed that the type of population plays a crucial role in the occurrence of VTE. However, this study solely relied on clinical manifestations as scoring criteria, omitting important indicators such as FVL and serum homocysteine levels. Additionally, routine screening for asymptomatic VTE patients was not performed, which could potentially lead to underreporting of incidence rates. These factors may contribute to the inconsistencies observed in the results.
To establish a more comprehensive understanding of the relationship between the Caprini risk score and VTE occurrence, future studies should consider incorporating a broader range of indicators and implementing routine screening measures. By addressing these limitations, we can gain deeper insights into the risk assessment of VTE in different patient populations.
Internal medicine inpatients
The Padua rating scale is frequently used for medical inpatients. Barbar et al. proposed the scale in 2010 and validated it in a prospective cohort study. The scale consists of 20 points, with scores > 4 classified as high-risk patients for VTE, while scores < 4 were classified as low-risk patients [ 82 ]. Since its release, many studies have verified its reliability. However, variations in subject composition and study design could be attributed to the large differences reported among these studies. Retrospective case–control studies often lead to a relatively high prevalence in the subjects, resulting in increased sensitivity and an overestimation of the scale's predictive performance. Additionally, disparities in sample sizes, researchers' subjective factors, ethnic disparities, and regional differences may also cause variations. Moumneh [ 89 ] externally validated the predictive ability of Caprini, Padua, and IMPROVE (Internal Medicine Patient Bleeding Risk Assessment Scale) scores for 3-month VTE in medical inpatients. The results showed that there was no significant difference in the area under the receiver operating characteristic curve (AUC) of the three, which was about 0.60–0.65. None of them showed a better ability to identify risk than the single predictor of age. This inevitably raises doubts about the necessity of clinical application of the risk assessment scale. We speculate that these risk prediction scales may exhibit superiority in specific disease subgroups, and this sensitivity may be masked in the evaluation of overall medical patients. Meanwhile, due to the retrospective collection of data, the BMI of many patients is artificially supplemented because it is not available, which may deviate from the true value. Other studies have shown that the predictive ability of the IMPROVE assessment model improved when the combined elevated D-dimer was analyzed together [ 90 ], suggesting that scientific inclusion of risk factors can help predict disease risk better.
Patients with cancer
Tumor in situ compression leads to blood stasis, and the secretion of cytokines leads to hypercoagulable state of blood, which makes tumor patients one of the high-risk groups of thrombosis. As the first fatal complication of cancer, VTE leads to about 9% of total deaths. Since the variables of Khorana score (KRS) are clinically accessible and routinely collected data, the current guidelines recommend the use of KRS to assess the risk of VTE formation for new cancer outpatients receiving chemotherapy [ 83 ]. However, the lack of an ideal patient data set for external validation has limited the scale development process, which solely relied on the split-sample method to construct and validate the model. Numerous studies have demonstrated the effectiveness of KRS. However, due to variations among studies and the subjective interpretation by different doctors, the accuracy of KRS remains unclear. KRS is designed for a heterogeneous population with various cancer types, and its evaluation efficacy differs across specific cancer types. Furthermore, variables occurring during the treatment process, such as chemotherapy drug types, adverse reactions, and vascular invasive procedures, may also influence the occurrence of VTE. Therefore, additional relevant factors should be incorporated to enable KRS to provide a dynamic evaluation throughout the entire chemotherapy process.
The role of prethrombotic genetic factors in the occurrence of venous thromboembolism (VTE) in cancer patients has garnered increasing attention in recent years. In a study of cancer outpatients receiving chemotherapy, researchers found that FVL mutations and non-O blood types were significantly associated with VTE, and that prophylactic anticoagulant therapy effectively reduced the risk of thrombotic events in such populations [ 91 ]. Guman et al. [ 92 ] used a multi-gene VTE risk score to assess the risk of cancer-related VTE, and compared it with KRS. The results showed that the score based on multiple genes showed more stable performance in thrombosis prediction. After the tumor type is included in the evaluation factors, the performance is better. Therefore, it is of clinical significance to determine whether the patient has a coagulation-related genetic mutation or whether it is a non-O blood group before chemotherapy.
Based on the above, Muñoz et al. [ 93 ] developed a new VTE scoring model (ONCOTHROMB score) for cancer outpatients, which combined genetic factors and clinical factors. External validation of this score in 263 patients showed promising results, but further studies are needed to confirm its applicability and accuracy.
Other thrombotic risk assessment models
Besides the three commonly used thrombotic risk assessment models mentioned above, there are also specific scales in clinical practice for assessing thrombotic risk under certain disease states. Pulmonary embolism (PE) can present with varied symptoms ranging from asymptomatic to severe symptoms such as dyspnea, chest pain, and hemoptysis, leading to high rates of misdiagnosis and underdiagnosis due to their nonspecific nature. Given the high mortality and disability rates associated with PE, prevention for this condition is crucial. The simplified Wells score and the revised Geneva score are frequently used to predict the likelihood of PE [ 94 , 95 ]. Both scoring systems contain similar items; however, the Wells score includes the subjective factor of "alternative diagnosis less likely than PE," which carries a high subjective component and scoring, potentially leading to errors in clinical use. In contrast, the revised Geneva score eliminates arterial blood gas analysis and chest X-ray items from the original scoring system and introduces scoring items such as malignancy, hemoptysis, unilateral lower limb pain and swelling, and lower limb deep vein tenderness, replacing subjective factors with objective measurements and thereby enhancing objectivity. Additionally, this scoring system has undergone extensive external validation and serves as an effective tool for assessing PE risk in internal medicine inpatients. Furthermore, the Pulmonary Embolism Severity Index (PESI) is used to assess the severity of PE [ 96 ]. Risk stratification for thromboembolism is crucial as it directly impacts treatment strategies and prognosis. By assessing clinical features and risk factors, high-risk and low-risk patients can be identified. High-risk patients may require urgent treatment to reduce mortality risk. Risk stratification also optimizes resource utilization, ensuring timely treatment and monitoring to decrease complications such as recurrent embolism. Effective risk stratification improves treatment outcomes and facilitates personalized care implementation.
For patients with thrombosis, assessing the risk of bleeding is crucial when deciding on anticoagulant therapy. Anticoagulants prevent blood clotting to prevent thrombus formation or reduce thrombus size. However, this also decreases the blood's clotting ability, increasing the risk of bleeding. Considering the bleeding risk helps doctors choose treatments more cautiously to prevent thrombosis while minimizing bleeding risks. It's worth noting that there are currently no effective predictive models to accurately assess bleeding risk in anticoagulated patients.
The application of AI in thrombotic diseases
Since its inception in 1956, Artificial Intelligence (AI) has undergone continuous development. With the expansion of research in various disciplines, AI has become an interdisciplinary frontier discipline, with increasingly enriched application methods and implementation approaches. Leveraging big data and powerful hardware/computing capabilities, machine learning (ML) enables the establishment of a universal and consistent research model. AI can be applied to natural language processing, computer vision, robotics, intelligent search, data mining and expert system. Machine learning is the core branch and main implementation method of AI, including supervised learning, semi-supervised learning, unsupervised learning and reinforcement learning. Deep learning represents a further advancement in machine learning, utilizing multi-layer neural networks to mimic the neural mechanisms of the human brain for data analysis and interpretation. Deep learning enables the analysis of larger and more complex datasets, albeit with higher demands on computer computing power, making it the most concerned learning method currently. Machine learning comprises three key components: models, strategies, and algorithms. Researchers select specific learning methods based on different application scenarios and utilize large-scale datasets as training sets to acquire and master relevant knowledge, thereby constructing appropriate models. The selection of the best model is determined through validation using an independent validation set. Prior to actual deployment, the model is compared with existing models/systems to demonstrate its comparative advantages. Overall, the continuous development and advancement of AI, particularly within the field of machine learning, has paved the way for its practical applications (shown in Fig. 5 ).
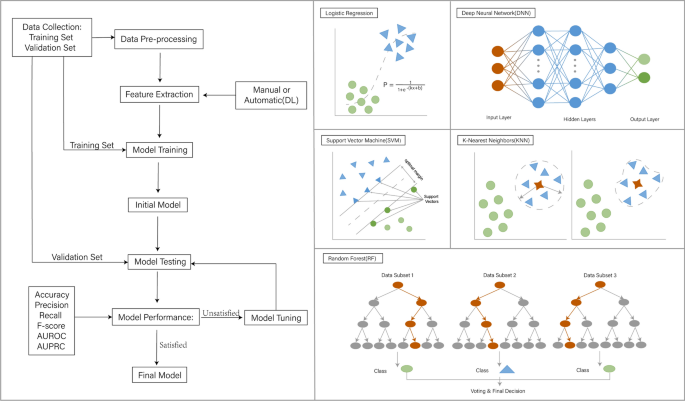
The basic process of using artificial intelligence to build medical models, and some common algorithms. The preprocessed data is usually divided into three parts: training set, validation set and test set. Researchers choose specific algorithms according to different application scenarios. After using the training set to learn and master the relevant knowledge, the corresponding models can be obtained. The validation set is used for verification, and the best model is selected after quantifying the score
In recent years, the potential benefits of AI in clinical practice have been gradually explored, including rapid processing of large-scale data, correlation analysis to remove redundant variables and avoid model over-fitting, and the use of consistent, homogeneous, and objective evaluation criteria to enhance diagnostic accuracy and specificity. The construction process of a clinical model primarily involves screening important covariates, filtering the input sample data, constructing the model using machine learning techniques, and validating its internal and external validity. When conditions permit, continuous follow-up and dynamic adjustments to the model are preferable. Medical artificial intelligence finds application in various fields, including but not limited to risk assessment, auxiliary diagnosis, clinical administration, improving patient consultations, determining surgical indications, making intraoperative decisions, and postoperative management (shown in Fig. 6 ).
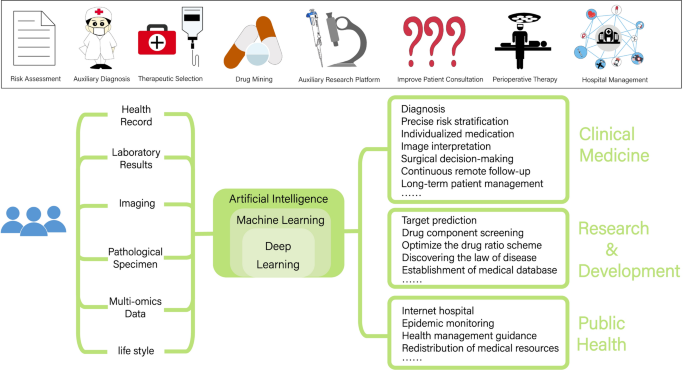
Application of AI in the medical field. The application fields of medical artificial intelligence include but are not limited to improving clinical diagnosis and treatment, assisting medical research, and promoting public health management. Application of clinical diagnosis and treatment: risk assessment, auxiliary diagnosis, clinical management, improvement of patient consultation, determination of surgical indications, intraoperative decision-making, postoperative management, etc. Auxiliary medical research: disease target prediction, drug component screening, discovery of disease rules, etc. Public health management: Internet hospitals, epidemic monitoring, optimization of medical resource allocation
Risk identification
Machine learning combined with clinical data can assist in identifying disease-related risk. Through rigorous data training, machine learning models can identify complex interactions between variables and discover predictive variables with clinical significance. By comparing with existing scoring systems and conducting thorough external validation, the generalizability of the machine learning model can be thoroughly demonstrated. Pituitary adenomas exhibit significant heterogeneity, making it challenging for traditional scoring systems to accurately predict postoperative outcomes. Hollon et al. [ 97 ] used four supervised machine learning algorithms, including naive Bayes, logistic regression, support vector machines, and random forest, to construct and cross-validate models. The test results demonstrated an accuracy of up to 87%. Furthermore, exploratory data analysis revealed risk factors for common postoperative complications, such as youth and obesity.
Thrombotic disease is a leading cause of global mortality, with risk factors encompassing both genetic and acquired factors. Early identification of individuals at high risk of thrombosis and the provision of appropriate preventive and therapeutic interventions are crucial. Machine learning serves as a powerful tool for thrombosis risk assessment, accurately identifying individuals who require personalized anticoagulation therapy. Previous studies have developed more accurate prediction models for venous thrombosis using machine learning methods [ 98 ] even without prior knowledge of relevant risk factors, highlighting the advantage of machine learning in uncovering potential risk factors. Subsequent generation of a thrombotic risk predictor using support vector machines and stochastic optimization in cancer outpatients showed more confidence than the KRS rating scale [ 99 ]. The significance of optimizing the clinical attribute group in the selection of predictors was also highlighted. Liu et al. [ 100 ] further developed a risk model for peripherally inserted central catheters in cancer inpatients, incorporating the PAI-1 genotype as a variable. To mitigate overfitting tendencies and ensure the model's robustness, a random decision forest was utilized. It is widely acknowledged that older age is an independent risk factor for thrombosis. Studies have revealed that the composition of risk factors for venous thromboembolism (VTE) differs between young and middle-aged inpatients and the elderly. Building on this, Liu [ 101 ] employed five algorithms to analyze clinical data from both patients and healthy individuals, effectively identifying the primary predictor of adverse outcomes in this population. Accurate and efficient prediction of mortality rates among patients in intensive care units (ICUs) is of great clinical significance. Such predictions aid in determining appropriate anticoagulation regimens for doctors and facilitate the rational allocation of ICU healthcare resources in the short term. Furthermore, they contribute to improving patient quality of life and reducing medical expenses. For ICU patients with VTE or cancer, two machine learning models—automated and customized—were developed to predict early and late mortality, respectively [ 102 ]. At the same time, a new biomarker was discovered.
The application of artificial intelligence in medicine covers various aspects from the entire disease process to prognosis assessment and recurrent risk prediction, providing doctors with more effective management measures to improve clinical outcomes. To overcome the limitations of existing statistical models, Martins et al. utilized artificial neural networks (ANNs) to predict recurrent venous thromboembolism, demonstrating through fivefold cross-validation that ANNs have higher accuracy and applicability compared to traditional scoring models. On the other hand, Mora et al. employed multiple machine learning methods including neural networks and logistic regression to study the prognosis of acute pulmonary embolism patients who prematurely stopped anticoagulant therapy. The results showed that neural networks (ML-NN) outperformed traditional logistic regression in predicting composite outcomes for patients. Therefore, the application of artificial intelligence technologies such as neural networks in medicine not only enhances the accuracy of prognosis assessment but also opens new possibilities for personalized healthcare, which is crucial for improving clinical outcomes for patients. Compared to other artificial intelligence learning methods, neural networks have the capability to model non-linear relationships. Therefore, their ability to extract information from complex data is stronger and more flexible. In addition, neural networks also excel in automatic feature learning, multi-task learning, and other advantages, which make them more efficient in practical applications.
Auxiliary diagnosis
Clinically, routine VTE screening relies on D-dimer levels, which can result in a high rate of false positives. The gold standard for diagnosing VTE is imaging results, including computed tomography (CT) and ultrasound (US), which can be costly for patients in underdeveloped areas and are also subject to variability due to differences in physician interpretation. By combining natural language processing with machine learning classifiers, VTE can be accurately identified from narrative radiology reports [ 103 ]. This approach not only saves clinicians' time in interpreting reports but also ensures result consistency. Antibody detection in the plasma of patients with antiphospholipid syndrome is complex, and there is noticeable heterogeneity between batches. As thrombosis is a significant clinical manifestation of APS, diagnosing APS using a neural network based on thrombin generation data is possible, and the results demonstrate good sensitivity and specificity [ 104 ]. In recent years, computer vision imaging has become increasingly common in assisting image interpretation, with radiomics representing its most prominent application [ 105 ]. Whether in coronary angiography or brain magnetic resonance imaging, deep learning (DL) can learn from large datasets to integrate image information from multiple aspects, achieving automated and multimodal thrombosis diagnosis. Two deep neural networks are utilized to learn segment information and lesion morphology in coronary angiography images, respectively [ 106 ]. Finally, an automated angiography reporting system is generated, improving the accuracy of angiography diagnosis and enhancing the consistency of treatment decisions. Similarly, DL is applied to the diagnosis of cerebral venous thrombosis by brain magnetic resonance imaging. By analyzing the images of different sequences together, the information complementarity between sequences is realized, and the sensitivity of diagnosis is improved [ 107 ] (shown in Fig. 7 ).
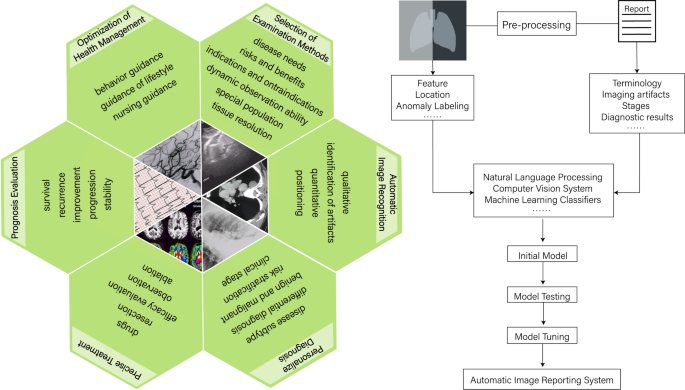
Combination of artificial intelligence and imaging. The combination of artificial intelligence and imaging can help to select examination methods, realize automatic report interpretation, individualized diagnosis, individualized treatment, prognosis evaluation and optimize health management. A case study of artificial intelligence to establish an automatic impact reporting system, which uses a variety of artificial intelligence technologies such as natural language processing and computer vision systems
AI holds great potential in several areas of medicine such as clinical decision-making, drug discovery, and medical research. Thrombosis is a condition that can be treated through several methods, including anticoagulation, thrombolysis, interventional therapy, surgical treatment, and supportive therapy. Selecting the appropriate treatment method is vital for clinical outcomes.
Platelet aggregation is the primary mechanism involved in the formation of atherosclerotic thrombosis. Agonists and platelet surface receptors combine to trigger the activation process. While there are antiplatelet drugs available for different types of agonists, there is no clear plan for selecting which drug to use. High-throughput imaging flow cytometry combined with image data analysis can identify subtle differences between platelet aggregates that have been activated by different types of agonists [ 108 ]. According to the different mechanism of platelet aggregation, the corresponding antiplatelet drugs can play a more accurate and efficient role. Using artificial neural network to integrate plasma proteomics and genome-wide association analysis data, a new pulmonary embolism susceptibility locus PLXNA4 was identified [ 109 ]. This finding provided a new direction for the study of the mechanism of PE.
In recent years, new technologies such as AI and in vivo nanotechnology have been used to predict, diagnose, and treat thrombosis. Nano-scale inflatable bubbles can be used as a drug delivery system that targets local areas, improving the efficacy while avoiding the toxic effects caused by off-target. Contrast agents can also be loaded onto the bubbles to make molecular imaging possible. When combined with AI, this approach can guide nanobubbles to improve the safety and real-time performance of precision medicine [ 110 ]. Microbubbles are a promising tool for the treatment of thrombotic diseases. They can open blocked blood vessels, target the delivery of thrombolytic drugs, and minimize the risk of adverse reactions such as bleeding. Tumor-derived extracellular vesicles (EVs) in cancer patients contain procoagulant components that can lead to venous thromboembolism (VTE). To detect VTE risk more accurately and specifically, a procoagulant extracellular vesicle barcode composed of TiO2 nanoflowers (TiNFs) can be used for in-situ detection [ 111 ]. This approach can be coupled with machine learning-assisted data analysis for improved accuracy and stability. The test results are not affected by the instantaneous hypercoagulable state of the blood since tumor-derived EVs continue to secrete.
Medical artificial intelligence (AI) holds great potential for improving clinical outcomes, but there are still many challenges to overcome before it can be widely implemented. First of all, from the data set level, there are data barriers between hospitals. Different units may have heterogeneity in examination results and disease interpretation, making it challenging to develop models that are universally applicable. While the medical system has shifted towards electronic data management, the integrity, consistency, and accuracy of data still need to be improved to ensure high-quality modeling. The cost of large-scale multi-center research poses another challenge, limiting the development of many prediction models to single institutions. The sample size of training sets, test sets, and verification sets is often not large enough, leading to biased parameters. External validation of patient data from other institutions is required to make the model more universal. This validation process can also help identify and correct any biases or inaccuracies in the original model. Secondly, from an algorithmic standpoint, as artificial intelligence advances from binary classifiers to multiclass classifiers, the demand for computational power increases, necessitating more sophisticated technical support. It can be time-consuming to determine the ML model with the best performance. The calculation work and a large number of adjustments to the hyperparameters depend on personnel with professional knowledge, which can create a gap in talent. Automated machine learning may be more practical for future clinical medical research to address these issues. Finally, from the perspective of the operation mode of artificial intelligence, the widespread adoption of medical artificial intelligence faces many challenges. Firstly, establishing effective clinical models requires ample support from medical big data, inevitably bringing risks of patient privacy breaches. Additionally, many AI models, especially those based on neural networks, struggle with algorithmic opacity, often making it difficult to clearly explain their decision-making processes. This lack of transparency can diminish patient trust in the models, thereby hindering the broad application of medical AI technologies. Ethical and moral considerations are also crucial when using AI for medical decisions. Given that AI learns from clinical data, which may reflect human biases and treatment preferences, ensuring fairness in the model's application is likewise a significant challenge.
Overall, there is still much room for development in medical AI. To achieve real clinical transformation, data barriers between hospitals must be addressed, sample sizes for modeling must be expanded and validated externally, and automated machine learning should be prioritized as a more efficient and universal approach. By identifying and addressing these challenges, we can unlock the full potential of medical AI to improve patient outcomes and healthcare overall.
Optimizing thrombotic risk assessment: constructing the ideal risk scoring table
Thrombosis is a common yet serious clinical issue, with significant implications for the prevention and management of thromboembolic events. Existing thrombotic risk assessment scales play a crucial role in clinical practice; however, they face various limitations such as inadequate model universality and inconsistent predictive accuracy. Therefore, there is a recognized need to develop scoring systems with higher sensitivity and accuracy to enhance predictive capabilities for thrombotic risk and improve clinical applicability.
In recent years, there have been attempts to utilize artificial intelligence (AI) in constructing thrombotic risk assessment scales [ 112 , 113 , 114 ]. By employing suitable algorithms in the construction process, researchers have successfully developed novel thrombosis prediction models that may hold clinical significance. For instance, Xi et al. utilized eight machine learning algorithms including Random Forest (RF) in a retrospective study of clinical data to identify RF as having optimal diagnostic performance for reducing misdiagnosis and missed diagnoses of acute pulmonary embolism (APE) [ 112 ]. Variables included in their model were D-dimer, cardiac troponin T (cTNT), arterial oxygen saturation, heart rate, chest pain, leg pain, hemoptysis, and chronic heart failure. Subsequently, the RF model, alongside established Wells and revised Geneva scores and the Years algorithm, predicted outcomes in a test set, demonstrating superior performance compared to the Wells score and comparable diagnostic accuracy to other assessment strategies. This suggests that AI can assist researchers in refining and selecting critical clinical features, eliminating redundant information, and enhancing the efficiency of clinical decision-making. Another study the same year utilized structured electronic health records (EHR) for learning, constructing a model for VTE diagnosis and one-year risk prediction [ 113 ]. This model can make real-time predictions based on changes in patient clinical data. Such a dynamic assessment method aids clinicians in promptly adjusting treatment measures, achieving personalized and precise thrombosis prevention. However, this model's data originated from one hospital cohort and two national biobanks, potentially introducing composition biases in the cohort, such as higher incidence rates of cancer and other underlying diseases compared to the general population, which may affect the weighting of these variables. Furthermore, due to the limitations of retrospective studies, these models only predict thrombosis diagnosis rather than occurrence.
Based on the study of AI methods and thrombotic scoring scales, we propose the following concepts for an ideal risk scoring table: (1) the study should be prospective, avoiding significant biases in the composition of the study cohort related to diseases, ethnicities, regions, etc., ensuring the model's universality. Patients from multicenter and multinational sources constitute the most ideal study cohort; (2) emphasis should be placed on integrating various thrombosis-related information, primarily including age, baseline conditions, medical history, recent conditions, and inpatient status. Scientific methods should be employed for data collection, transformation, and screening to ensure data integrity and quality. For example, automated extraction of patient clinical data from EHR systems and analysis using standardized methods like PCA for dimensionality reduction to obtain detailed and accurate information; (3) during the initial construction phase, multiple prediction models such as logistic regression, decision trees, support vector machines, and neural networks should be used. Models should be evaluated based on metrics such as accuracy, area under the ROC curve, sensitivity, specificity, etc., selecting the model with the best predictive performance for parameter adjustment and optimization; (4) we believe the model should possess dynamic updating capabilities to monitor and reflect changes in patient health status in real time. This real-time feedback capability assists healthcare providers in promptly adjusting prevention and treatment strategies, achieving personalized medical management; (5) in practical clinical applications, the model should be easy to operate and interpret, enabling healthcare providers to make rapid and accurate decisions based on scoring results. By enhancing thromboembolic risk assessment and early detection, we aim to provide more precise and effective tools to support personalized thrombotic risk management, ultimately reducing the incidence and mortality associated with this condition and lowering the burden on healthcare systems.
Through excessive coagulation system activation, deficiencies in endogenous anticoagulants, inhibition of the fibrinolytic system, and other mechanisms, hereditary factors play a crucial role in promoting the development of thrombotic diseases. Novel mutation sites have been identified. Frequent susceptibility factors comprise prothrombin G20210A, FVL, protein C and protein S deficiencies, antithrombin SERPINC1, PAI-1 4G/5G polymorphism. Currently, there is no standardized approach for screening procoagulant genes. Clinically, patients with suspected thrombophilia are given the option to choose whether or not to be screened, followed by systematic testing for those with positive initial screening results. This includes laboratory testing and genetic defect screening, involving both antigen and functional level assessments. When testing, appropriate molecular forms should be selected based on the different forms of coagulation molecules in plasma. There is variation in genetic defects among different ethnicities. It is worth noting that patients with coagulation factor V Leiden and/or prothrombin mutation experienced a decrease in the incidence of bleeding-related adverse events during subsequent long-term anticoagulant therapy [ 115 ]. However, the ideal scenario is to identify high-risk patients through a simple and efficient risk prediction model, enabling early detection and intervention to prevent thrombosis.
In a multicenter observational cross-sectional study conducted in Brazil, involving 589 surgical patients and 865 clinical patients, Deheinzelin et al. [ 116 ] assessed the risk of VTE formation in patients using three models: the American College of Chest Physicians (ACCP) guidelines, the Caprini score, and the International Union of Cardiovascular Consensus Statements (IUAS). They then compared the results with the actual clinical preventive treatment and discovered a discrepancy between the patient's risk classification and the treatment they received. Medical practices are influenced by local medical conditions and the subjective factors of doctors and patients. Ensuring appropriate and rational anticoagulant therapy remains a clinical challenge. While rating scales continue to improve, manual assessment still constrains the scalability of the scale. Moreover, subjective differences in disease assessment among doctors make it challenging to ensure consistency in diagnosis and treatment approaches. Therefore, artificial intelligence holds great potential for development in the medical field. Currently, AI has achieved significant breakthroughs in risk assessment, auxiliary diagnosis, and treatment guidance. In the future, there is also a wide range of opportunities for further development in areas such as big data mining, follow-up management, and medical research platforms. However, during the development of clinical models, attention should be paid to the phenomenon of overfitting, which can lead to excessive sensitivity.
Currently known thrombotic diseases involve multiple genetic factors. However, due to the fact that most mutations occur only within individuals, their clinical significance remains somewhat limited. Future research directions could focus on how to apply these discoveries in clinical practice. For example, certain mutations that promote thrombosis could be explored for their potential applications in treating bleeding disorders. Currently, treatment of thrombotic diseases primarily relies on symptomatic therapies such as anticoagulants and thrombolytics. Nevertheless, considering their propensity for recurrence, gene therapies like CRISPR-Cas9 gene editing may offer new avenues to fundamentally alleviate or even cure thrombotic conditions. Yet, how researchers can identify potential research subjects from vast datasets remains a significant challenge. In the future, researchers may leverage the power of artificial intelligence to pinpoint suitable research sites through medical research platforms. With advancements in AI technology, clinical diagnosis and treatment will become more personalized and precise, significantly enhancing safety and efficiency. However, transparency in medical decision-making and the protection of patient privacy continue to pose major challenges limiting the widespread application of medical AI. Nevertheless, we believe these will no longer be issues in the near future.
Data availability
No datasets were generated or analysed during the current study.
Evensen LH, et al. The risk of venous thromboembolism attributed to established prothrombotic genotypes. Thromb Haemost. 2022;122:1221–30.
Article PubMed Google Scholar
Lee H-C, et al. Exploiting exercise electrocardiography to improve early diagnosis of atrial fibrillation with deep learning neural networks. Comput Biol Med. 2022;146: 105584.
Mora D, et al. Machine learning to predict outcomes in patients with acute pulmonary embolism who prematurely discontinued anticoagulant therapy. Thromb Haemost. 2022;122:570–7.
Mora D, et al. Machine learning to predict major bleeding during anticoagulation for venous thromboembolism: possibilities and limitations. Br J Haematol. 2023;201:971–81.
Article PubMed CAS Google Scholar
Heo J, et al. Cancer prediction with machine learning of thrombi from thrombectomy in stroke: multicenter development and validation. Stroke. 2023;54:2105–13.
Zhang Y, et al. The genetics of venous thromboembolism: a systematic review of thrombophilia families. J Thromb Thrombolysis. 2021;51:359–69.
Zhu X-J, et al. Congenital thrombophilia in East-Asian venous thromboembolism population: a systematic review and meta-analysis. Res Pract Thromb Haemost. 2023;7: 102157.
Article PubMed PubMed Central Google Scholar
Poort SR, Rosendaal FR, Reitsma PH, Bertina RM. A common genetic variation in the 3’-untranslated region of the prothrombin gene is associated with elevated plasma prothrombin levels and an increase in venous thrombosis. Blood. 1996;88:3698–703.
Cattaneo M, Chantarangkul V, Taioli E, Santos JH, Tagliabue L. The G20210A mutation of the prothrombin gene in patients with previous first episodes of deep-vein thrombosis: prevalence and association with factor V G1691A, methylenetetrahydrofolate reductase C677T and plasma prothrombin levels. Thromb Res. 1999;93:1–8.
Soria JM, et al. Linkage analysis demonstrates that the prothrombin G20210A mutation jointly influences plasma prothrombin levels and risk of thrombosis. Blood. 2000;95:2780–5.
Danckwardt S, et al. The prothrombin 3’end formation signal reveals a unique architecture that is sensitive to thrombophilic gain-of-function mutations. Blood. 2004;104:428–35.
Castoldi E, et al. Differential effects of high prothrombin levels on thrombin generation depending on the cause of the hyperprothrombinemia. J Thromb Haemost JTH. 2007;5:971–9.
Colucci M, Binetti BM, Tripodi A, Chantarangkul V, Semeraro N. Hyperprothrombinemia associated with prothrombin G20210A mutation inhibits plasma fibrinolysis through a TAFI-mediated mechanism. Blood. 2004;103:2157–61.
De Broucker C, et al. Multicenter study on recent portal venous system thrombosis associated with cytomegalovirus disease. J Hepatol. 2022;76:115–22.
Pryzdial EL, Wright JF. Prothrombinase assembly on an enveloped virus: evidence that the cytomegalovirus surface contains procoagulant phospholipid. Blood. 1994;84:3749–57.
Popović M, et al. Human cytomegalovirus infection and atherothrombosis. J Thromb Thrombolysis. 2012;33:160–72.
Tamura S, et al. In vitro exploration of latent prothrombin mutants conveying antithrombin resistance. Thromb Res. 2017;159:33–8.
Mulder R, et al. Linkage analysis combined with whole-exome sequencing identifies a novel prothrombin (F2) gene mutation in a Dutch Caucasian family with unexplained thrombosis. Haematologica. 2020;105:e370–2.
Wu X, et al. Prothrombin Arg541Trp mutation leads to defective PC (Protein C) pathway activation and constitutes a novel genetic risk factor for venous thrombosis. Arterioscler Thromb Vasc Biol. 2020;40:483–94.
Pruner I, et al. The silence speaks, but we do not listen: synonymous c.1824C>T gene variant in the last exon of the prothrombin gene as a new prothrombotic risk factor. Clin Chem. 2020;66:379–89.
von Ahsen N, Lewczuk P, Schütz E, Oellerich M, Ehrenreich H. Prothrombin activity and concentration in healthy subjects with and without the prothrombin G20210A mutation. Thromb Res. 2000;99:549–56.
Article Google Scholar
Shen L, Dahlbäck B. Factor V and protein S as synergistic cofactors to activated protein C in degradation of factor VIIIa. J Biol Chem. 1994;269:18735.
Dahlbäck B, Hildebrand B. Inherited resistance to activated protein C is corrected by anticoagulant cofactor activity found to be a property of factor V. Proc Natl Acad Sci USA. 1994;91:1396–400.
Peraramelli S, et al. Role of exosite binding modulators in the inhibition of Fxa by TFPI. Thromb Haemost. 2016;115:580–90.
Santamaria S, et al. Factor V has an anticoagulant cofactor activity that targets the early phase of coagulation. J Biol Chem. 2017;292:9335–44.
Article PubMed PubMed Central CAS Google Scholar
Dahlbäck B, Guo LJ, Livaja-Koshiar R, Tran S. Factor V-short and protein S as synergistic tissue factor pathway inhibitor (TFPIα) cofactors. Res Pract Thromb Haemost. 2017;2:114–24.
van Doorn P, et al. Factor V has anticoagulant activity in plasma in the presence of TFPIα: difference between FV1 and FV2. Thromb Haemost. 2018;118:1194–202.
Rosing J, et al. Effects of protein S and factor Xa on peptide bond cleavages during inactivation of factor Va and factor VaR506Q by activated protein C. J Biol Chem. 1995;270:27852–8.
Norstrøm EA, Steen M, Tran S, Dahlbäck B. Importance of protein S and phospholipid for activated protein C-mediated cleavages in factor Va. J Biol Chem. 2003;278:24904–17843.
Wood JP, et al. Tissue factor pathway inhibitor-alpha inhibits prothrombinase during the initiation of blood coagulation. Proc Natl Acad Sci USA. 2013;110:17838.
van Doorn P, Rosing J, Wielders SJ, Hackeng TM, Castoldi E. The C-terminus of tissue factor pathway inhibitor-α inhibits factor V activation by protecting the Arg1545 cleavage site. J Thromb Haemost. 2017;15:140–9.
Bertina RM, et al. Mutation in blood coagulation factor V associated with resistance to activated protein C. Nature. 1994;369:64–7.
Voorberg J, et al. Association of idiopathic venous thromboembolism with single point-mutation at Arg506 of factor V. Lancet Lond Engl. 1994;343:1535–6.
Article CAS Google Scholar
Zöller B, Dahlbäck B. Linkage between inherited resistance to activated protein C and factor V gene mutation in venous thrombosis. Lancet Lond Engl. 1994;343:1536–8.
Castoldi E, et al. Severe thrombophilia in a factor V-deficient patient homozygous for the Ala2086Asp mutation (FV Besançon). J Thromb Haemost JTH. 2021;19:1186–99.
Duckers C, et al. Low plasma levels of tissue factor pathway inhibitor in patients with congenital factor V deficiency. Blood. 2008;112:3615–23.
Shimonishi N, et al. Impaired factor V-related anticoagulant mechanisms and deep vein thrombosis associated with A2086D and W1920R mutations. Blood Adv. 2023;7:2831–42.
Nogami K, et al. Novel FV mutation (W1920R, FVNara) associated with serious deep vein thrombosis and more potent APC resistance relative to FVLeiden. Blood. 2014;123:2420–8.
Perez Botero J, et al. Do incident and recurrent venous thromboembolism risks truly differ between heterozygous and homozygous Factor V Leiden carriers? A retrospective cohort study. Eur J Intern Med. 2016;30:77–81.
Shi Z, et al. Cancer-associated thrombosis by cancer sites and inherited factors in a prospective population-based cohort. Thromb Res. 2023;229:69–72.
Ryu J, et al. Thrombosis risk in single- and double-heterozygous carriers of factor V Leiden and prothrombin G20210A in FinnGen and the UK Biobank. Blood. 2024;143:2425–32.
Van Cott EM, Khor B, Zehnder JL. Factor V Leiden. Am J Hematol. 2016;91:46–9.
Simioni P, et al. X-linked thrombophilia with a mutant factor IX (factor IX Padua). N Engl J Med. 2009;361:1671–5.
Plautz WE, et al. Padua FIXa resistance to Protein S and a potential therapy for hyperactive FIXa. Thromb Res. 2018;170:133–41.
Nakajima Y, Takeyama M, Oda A, Shimonishi N, Nogami K. Factor VIII mutated with Lys1813Ala within the factor IXa-binding region enhances intrinsic coagulation potential. Blood Adv. 2023;7:1436–45.
Gu L, et al. Influence of the β-fibrinogen-455G/A polymorphism on development of ischemic stroke and coronary heart disease. Thromb Res. 2014;133:993–1005.
Hu X, et al. The β-fibrinogen gene 455G/A polymorphism associated with cardioembolic stroke in atrial fibrillation with low CHA2DS2-VaSc score. Sci Rep. 2017;7:17517.
Jin L, et al. The anticoagulant activation of antithrombin by heparin. Proc Natl Acad Sci USA. 1997;94:14683–8.
Wang H-L, et al. Identification and characterization of two SERPINC1 mutations causing congenital antithrombin deficiency. Thromb J. 2023;21:3.
Luxembourg B, et al. Impact of the type of SERPINC1 mutation and subtype of antithrombin deficiency on the thrombotic phenotype in hereditary antithrombin deficiency. Thromb Haemost. 2014;111:249–57.
PubMed CAS Google Scholar
Bravo-Pérez C, et al. Molecular and clinical characterization of transient antithrombin deficiency: a new concept in congenital thrombophilia. Am J Hematol. 2022;97:216–25.
Frebelius S, Isaksson S, Swedenborg J. Thrombin inhibition by antithrombin III on the subendothelium is explained by the isoform AT beta. Arterioscler Thromb Vasc Biol. 1996;16:1292–7.
McCoy AJ, Pei XY, Skinner R, Abrahams JP, Carrell RW. Structure of beta-antithrombin and the effect of glycosylation on antithrombin’s heparin affinity and activity. J Mol Biol. 2003;326:823–33.
Kleesiek K, et al. The 536C–>T transition in the human tissue factor pathway inhibitor (TFPI) gene is statistically associated with a higher risk for venous thrombosis. Thromb Haemost. 1999;82:1–5.
Anderson JL, et al. Lack of association of a common polymorphism of the plasminogen activator inhibitor-1 gene with coronary artery disease and myocardial infarction. J Am Coll Cardiol. 1999;34:1778–83.
Morange PE, et al. The A -844G polymorphism in the PAI-1 gene is associated with a higher risk of venous thrombosis in factor V Leiden carriers. Arterioscler Thromb Vasc Biol. 2000;20:1387–91.
Balta G, Altay C, Gurgey A. PAI-1 gene 4G/5G genotype: a risk factor for thrombosis in vessels of internal organs. Am J Hematol. 2002;71:89–93.
Incalcaterra E, et al. Residual vein thrombosis and onset of post-thrombotic syndrome: influence of the 4G/5G polymorphism of plasminogen activator inhibitor-1 gene. Thromb Res. 2014;133:371–4.
Wang J, et al. Association between the plasminogen activator inhibitor-1 4G/5G polymorphism and risk of venous thromboembolism: a meta-analysis. Thromb Res. 2014;134:1241–8.
Choi GH, et al. Association between PAI-1 polymorphisms and ischemic stroke in a South Korean case-control cohort. Int J Mol Sci. 2023;24:8041.
Stearns-Kurosawa DJ, Kurosawa S, Mollica JS, Ferrell GL, Esmon CT. The endothelial cell protein C receptor augments protein C activation by the thrombin-thrombomodulin complex. Proc Natl Acad Sci USA. 1996;93:10212–6.
Yegneswaran S, et al. Relocating the active site of activated protein C eliminates the need for its protein S cofactor. A fluorescence resonance energy transfer study. J Biol Chem. 1999;274:5462–8.
Fernandes N, Mosnier LO, Tonnu L, Heeb MJ. Zn 2 (+) -containing protein S inhibits extrinsic factor X-activating complex independently of tissue factor pathway inhibitor. J Thromb Haemost JTH. 2010;8:1976–85.
Kimura R, et al. Protein S-K196E mutation as a genetic risk factor for deep vein thrombosis in Japanese patients. Blood. 2006;107:1737–8.
Li L, et al. Clinical manifestation and mutation spectrum of 53 unrelated pedigrees with protein S deficiency in China. Thromb Haemost. 2019;119:449–60.
ten Kate MK, van der Meer J. Protein S deficiency: a clinical perspective. Haemoph Off J World Fed Hemoph. 2008;14:1222–8.
Zhang Y, et al. A novel PROS1 mutation, c.74dupA, was identified in a protein S deficiency family. Thromb Res. 2016;148:125–7.
Ohlin A-K, Marlar RA. The first mutation identified in the thrombomodulin gene in a 45-year-old man presenting with thromboembolic disease. Blood. 1995;85:330–6.
Nakazawa F, et al. Thrombomodulin with the Asp468Tyr mutation is expressed on the cell surface with normal cofactor activity for protein C activation. Br J Haematol. 1999;106:416–20.
Kunz G, et al. Naturally occurring mutations in the thrombomodulin gene leading to impaired expression and function. Blood. 2002;99:3646–53.
Tang L, et al. Common genetic risk factors for venous thrombosis in the Chinese population. Am J Hum Genet. 2013;92:177–87.
Manderstedt E, et al. Thrombomodulin (THBD) gene variants and thrombotic risk in a population-based cohort study. J Thromb Haemost JTH. 2022;20:929–35.
Jiewen M, et al. A missense mutation in lectin domain of thrombomodulin causing functional deficiency. Transl Res J Lab Clin Med. 2023;251:74–83.
Google Scholar
Milger K, et al. Identification of a novel SERPINA-1 mutation causing alpha-1 antitrypsin deficiency in a patient with severe bronchiectasis and pulmonary embolism. Int J Chron Obstruct Pulmon Dis. 2015;10:891–7.
Basil N, et al. Severe alpha-1-antitrypsin deficiency increases the risk of venous thromboembolism. J Thromb Haemost JTH. 2021;19:1519–25.
Riis J, Nordestgaard BG, Afzal S. α1 -Antitrypsin Z allele and risk of venous thromboembolism in the general population. J Thromb Haemost JTH. 2022;20:115–25.
Güngör Y, Kayataş M, Yıldız G, Özdemir Ö, Candan F. The presence of PAI-1 4G/5G and ACE DD genotypes increases the risk of early-stage AVF thrombosis in hemodialysis patients. Ren Fail. 2011;33:169–75.
Pomero F, et al. Association between inherited thrombophilia and venous thromboembolism in patients with non-O blood type: a meta-analysis. Pol Arch Intern Med. 2022;132:16327.
PubMed Google Scholar
Lian T-Y, et al. Association between congenital thrombophilia and outcomes in pulmonary embolism patients. Blood Adv. 2020;4:5958–65.
Tormene D, et al. The risk of arterial thrombosis in carriers of natural coagulation inhibitors: a prospective family cohort study. Intern Emerg Med. 2021;16:997–1003.
Caprini JA, Arcelus JI, Hasty JH, Tamhane AC, Fabrega F. Clinical assessment of venous thromboembolic risk in surgical patients. Semin Thromb Hemost. 1991;17(Suppl 3):304–12.
Barbar S, et al. A risk assessment model for the identification of hospitalized medical patients at risk for venous thromboembolism: the Padua Prediction Score. J Thromb Haemost JTH. 2010;8:2450–7.
Khorana AA, Kuderer NM, Culakova E, Lyman GH, Francis CW. Development and validation of a predictive model for chemotherapy-associated thrombosis. Blood. 2008;111:4902–7.
Li S, Wang L, Lu Q. Comparison of the predictive power of the 2005 and 2010 Caprini risk assessment models for deep vein thrombosis in Chinese orthopedic patients at admission: a prospective cohort study. Thromb Res. 2023;222:1–6.
Lobastov K, et al. The thresholds of Caprini score associated with increased risk of venous thromboembolism across different specialties: a systematic review. Ann Surg. 2023. https://doi.org/10.1097/SLA.0000000000005843 .
Pannucci CJ, et al. Validation of the Caprini risk assessment model in plastic and reconstructive surgery patients. J Am Coll Surg. 2011;212:105–12.
Zhou H-X, et al. Validation of the Caprini risk assessment model in Chinese hospitalized patients with venous thromboembolism. Thromb Res. 2012;130:735–40.
Bilgi K, et al. Assessing the risk for development of Venous Thromboembolism (VTE) in surgical patients using adapted Caprini scoring system. Int J Surg Lond Engl. 2016;30:68–73.
Moumneh T, et al. Validation of risk assessment models predicting venous thromboembolism in acutely ill medical inpatients: a cohort study. J Thromb Haemost JTH. 2020;18:1398–407.
Spyropoulos AC, et al. Abstract 13399: Use of the International Medical Prevention Registry on Venous Thromboembolism (IMPROVE) venous thromboembolism risk model and elevated D-Dimer to identify a high venous thromboembolism risk in an acutely ill medical population for extended thromboprophylaxis. Circulation. 2019;140:A13399–A13399.
Roy DC, et al. Thrombophilia gene mutations predict venous thromboembolism in ambulatory cancer patients receiving chemotherapy. J Thromb Haemost JTH. 2023;21:3184–92.
Guman NAM, et al. Polygenic risk scores for prediction of cancer-associated venous thromboembolism in the UK Biobank cohort study. J Thromb Haemost JTH. 2023;21:3175–83.
Muñoz A, et al. A clinical-genetic risk score for predicting cancer-associated venous thromboembolism: a development and validation study involving two independent prospective cohorts. J Clin Oncol Off J Am Soc Clin Oncol. 2023;41:2911–25.
den Exter PL, et al. Performance of the revised Geneva score in patients with a delayed suspicion of pulmonary embolism. Eur Respir J. 2014;43:1801–4.
van Es N, et al. The original and simplified wells rules and age-adjusted D-dimer testing to rule out pulmonary embolism: an individual patient data meta-analysis. J Thromb Haemost JTH. 2017;15:678–84.
Chan CM, Woods C, Shorr AF. The validation and reproducibility of the pulmonary embolism severity index. J Thromb Haemost JTH. 2010;8:1509–14.
Hollon TC, et al. A machine learning approach to predict early outcomes after pituitary adenoma surgery. Neurosurg Focus. 2018;45:E8.
Kawaler E, et al. Learning to predict post-hospitalization VTE risk from EHR data. AMIA Annu Symp Proc AMIA Symp. 2012;2012:436–45.
Ferroni P, et al. Risk assessment for venous thromboembolism in chemotherapy-treated ambulatory cancer patients. Med Decis Mak Int J Soc Med Decis Mak. 2017;37:234–42.
Liu S, et al. Machine learning approaches for risk assessment of peripherally inserted Central catheter-related vein thrombosis in hospitalized patients with cancer. Int J Med Inf. 2019;129:175–83.
Liu H, et al. Prediction of venous thromboembolism with machine learning techniques in young-middle-aged inpatients. Sci Rep. 2021;11:12868.
Danilatou V, et al. Outcome prediction in critically-ill patients with venous thromboembolism and/or cancer using machine learning algorithms: external validation and comparison with scoring systems. Int J Mol Sci. 2022;23:7132.
Narain WR. Development of an automated system for querying radiology reports and recording deep venous thrombosis and pulmonary emboli. (Rutgers University - School of Health Professions, 2016). https://doi.org/10.7282/T3T155RQ .
de Laat-Kremers RMW, et al. Deciphered coagulation profile to diagnose the antiphospholipid syndrome using artificial intelligence. Thromb Res. 2021;203:142–51.
Yao L, et al. Radiomics boosts deep learning model for IPMN classification. In: Cao X, Xu X, Rekik I, Cui Z, Ouyang X, editors., et al., Machine learning in medical imaging. Cham: Springer Nature; 2024. p. 134–43.
Chapter Google Scholar
Du T, et al. Training and validation of a deep learning architecture for the automatic analysis of coronary angiography. EuroIntervention J Eur Collab Work Group Interv Cardiol Eur Soc Cardiol. 2021;17:32–40.
CAS Google Scholar
Yang X, et al. Deep learning algorithm enables cerebral venous thrombosis detection with routine brain magnetic resonance imaging. Stroke. 2023;54:1357–66.
Zhou Y, et al. Intelligent classification of platelet aggregates by agonist type. Elife. 2020;9: e52938.
Razzaq M, et al. An artificial neural network approach integrating plasma proteomics and genetic data identifies PLXNA4 as a new susceptibility locus for pulmonary embolism. Sci Rep. 2021;11:14015.
Akbar A, Pillalamarri N, Jonnakuti S, Ullah M. Artificial intelligence and guidance of medicine in the bubble. Cell Biosci. 2021;11:108.
Min L, et al. Machine-learning-assisted procoagulant extracellular vesicle barcode assay toward high-performance evaluation of thrombosis-induced death risk in cancer patients. ACS Nano. 2023;17:19914–24.
Xi L, et al. A machine learning model for diagnosing acute pulmonary embolism and comparison with Wells score, revised Geneva score, and Years algorithm. Chin Med J. 2024;137:676–82.
Chen R, Petrazzini BO, Malick WA, Rosenson RS, Do R. Prediction of venous thromboembolism in diverse populations using machine learning and structured electronic health records. Arterioscler Thromb Vasc Biol. 2024;44:491–504.
Laffafchi S, Ebrahimi A, Kafan S. Efficient management of pulmonary embolism diagnosis using a two-step interconnected machine learning model based on electronic health records data. Health Inf Sci Syst. 2024;12:17.
Caiano L, et al. The risk of major bleeding in patients with factor V Leiden or prothrombin G20210A gene mutation while on extended anticoagulant treatment for venous thromboembolism. J Thromb Haemost. 2023;21:553–8.
Deheinzelin D, et al. Incorrect use of thromboprophylaxis for venous thromboembolism in medical and surgical patients: results of a multicentric, observational and cross-sectional study in Brazil. J Thromb Haemost. 2006;4:1266–70.
Download references
This work was supported by the National Key R&D Program of China (No. 2022YFC2304600), National Natural Science Foundation of China (No. 82170131), the Program for HUST Academic Frontier Youth Team (No. 2018QYTD14).
Author information
Authors and affiliations.
Institute of Hematology, Union Hospital, Tongji Medical College, Huazhong University of Science and Technology, Wuhan, China
Rong Wang, Liang V. Tang & Yu Hu
Key Lab of Molecular Biological Targeted Therapies of the Ministry of Education, Union Hospital, Tongji Medical College, Huazhong University of Science and Technology, Wuhan, China
Liang V. Tang & Yu Hu
You can also search for this author in PubMed Google Scholar
Contributions
All the authors have contributed to this review. Rong Wang drafted the main manuscript text and prepared figures. Liang V. Tang provided valuable input into the article. Liang V. Tang and Yu Hu revised and approved the final manuscript.
Corresponding authors
Correspondence to Liang V. Tang or Yu Hu .
Ethics declarations
Competing interests.
The authors declare no competing interests.
Additional information
Publisher's note.
Springer Nature remains neutral with regard to jurisdictional claims in published maps and institutional affiliations.
Rights and permissions
Open Access This article is licensed under a Creative Commons Attribution 4.0 International License, which permits use, sharing, adaptation, distribution and reproduction in any medium or format, as long as you give appropriate credit to the original author(s) and the source, provide a link to the Creative Commons licence, and indicate if changes were made. The images or other third party material in this article are included in the article's Creative Commons licence, unless indicated otherwise in a credit line to the material. If material is not included in the article's Creative Commons licence and your intended use is not permitted by statutory regulation or exceeds the permitted use, you will need to obtain permission directly from the copyright holder. To view a copy of this licence, visit http://creativecommons.org/licenses/by/4.0/ . The Creative Commons Public Domain Dedication waiver ( http://creativecommons.org/publicdomain/zero/1.0/ ) applies to the data made available in this article, unless otherwise stated in a credit line to the data.
Reprints and permissions
About this article
Cite this article.
Wang, R., Tang, L.V. & Hu, Y. Genetic factors, risk prediction and AI application of thrombotic diseases. Exp Hematol Oncol 13 , 89 (2024). https://doi.org/10.1186/s40164-024-00555-x
Download citation
Received : 11 May 2024
Accepted : 09 August 2024
Published : 27 August 2024
DOI : https://doi.org/10.1186/s40164-024-00555-x
Share this article
Anyone you share the following link with will be able to read this content:
Sorry, a shareable link is not currently available for this article.
Provided by the Springer Nature SharedIt content-sharing initiative
- Thrombophilia
- Genetic factors
- Risk prediction
- Artificial intelligence
- Machine learning
Experimental Hematology & Oncology
ISSN: 2162-3619
- Submission enquiries: [email protected]
NTRS - NASA Technical Reports Server
Available downloads, related records.

COMMENTS
SciSummary uses GPT-3.5 and GPT-4 models to summarize any scientific articles or research papers in seconds. It also offers features such as figure and table analysis, chat messages, and reference import.
Scholarcy is a tool that extracts key information from research papers, PDFs, book chapters, online articles and more. It generates flashcards that capture the main points, claims and findings of the original text, and lets you skim, dive deep, organize and apply your knowledge.
Scribbr offers a free tool to summarize any text, article, paragraph or essay in seconds. You can choose between key sentences or concise paragraphs, and adjust the summary length to suit your needs.
HyperWrite's Research Paper Summarizer is an AI-powered tool that reads and summarizes research papers. It helps you quickly understand the key points, arguments, and conclusions of a research paper for various purposes.
4. SciSummary: A Smart AI Summarizer for Research Papers. SciSummary is an AI summarizer that helps summarize single or multiple research papers. It combines and compares the content summaries from research papers, article links, etc. 5. Quillbot: Advanced Summarizing Tool for Research Papers.
TLDR This, the online article summarizer tool, not only condenses lengthy articles into shorter, digestible content, but it also automatically extracts essential metadata such as author and date information, related images, and the title. Additionally, it estimates the reading time for news articles and blog posts, ensuring you have all the ...
QuillBot's AI Text Summarizer, trusted by millions globally, utilizes cutting-edge AI to summarize articles, papers, or documents into key summary paragraphs. Try our free AI text summarization tool now!
Research Assistant is an AI-powered tool that summarizes the main points of research inputs, sections of papers, or reviews. It uses GPT-4 and ChatGPT AI models to generate concise paragraphs that highlight the key findings and insights from your input.
The summary of a research paper should include a brief statement of the author's conclusions. This will help your teacher understand what the paper is trying to achieve. ... AI Summarizer harnesses artificial intelligence to summarize research papers and other text documents automatically. The tool streamlines the summarization process, making ...
15. ChatGPT: Speedy Summarization Tool. ChatGPT is a fast tool designed to summarize research papers quickly and effectively. The tool assists users in identifying core points within research papers in a short amount of time, supporting millions of people worldwide in writing literature reviews efficiently.
Get PDF Summary with AI in Seconds. Experience a new level of efficiency and unlock the full potential of your PDF documents using AI PDF Summarizer. AI PDF Summarizer can summarize long PDF documents in seconds. It can convert PDFs to text, allowing you to ask your PDF. The best PDF Summary tool helps you save time and learning faster and better.
A research paper summarizer condenses the content of a lengthy research paper and turns the key information into a short paragraph or bullet point. It usually uses artificial intelligence and natural language processing algorithms to automate the process of analyzing the text and generating the summary.
Sharly's AI summary tool is capable of discerning key points from complex content accurately. Whether you're dealing with a research paper, a business report or the recording of a meeting, you can rely on Sharly to bring text to a comprehensive and accurate summary.
HIX Writer is one of the best AI summary generators enabling you to summarize text, Youtube videos, PDF documents and more. Use HIX Summarizer, an AI text summarizer to summarize articles instantly. Our advanced summary generator also provides summaries for a PDF, Doc, or TXT file and Youtube videos. Try out today.
Our AI text summarization tool uses advanced algorithms to automatically create summaries from articles, reports, papers, and more. ... I use this text summarizer to help me parse long research papers in my field much faster. It's been a total game changer for my literature reviews - I can now get through 3 times as many papers in the same ...
Our chatbot allows you to have a conversation with any paper on arXiv, enabling you to get more insights and ask questions in a natural language way. Our AI-powered arXiv paper summarization service provides fast, accurate, and easy-to-read summaries of scientific papers. Save time and stay up-to-date with the latest research with our cutting ...
AI PDF Summarizer. With AI PDF, you can utilize the powers of artificial intelligence to summarize PDFs for free! The interactive chat function lets you request specific information to be summarized and presented to you in a matter of seconds. AI PDF Summarizer lets you understand document contents without having to read through every page.
SciSummary is a cutting-edge AI-driven summarization tool designed to make digesting scientific articles easier. It enables users to quickly and accurately understand complex scientific articles without having to read the full text. To use the service, users can email a text, a link, or even attach a PDF and within minutes they will receive a summary in their inbox. The service is powered by a ...
Our summary generator uses advanced AI technology to break down your long content into quick, digestible summaries in just one click. Use it to summarize your articles, academic papers, business reports, or any kind of content. ... Quickly turn complex research papers or articles into easy to digest summaries. Business Reports. Overwhelmed by ...
Summarize any PDF with AI. Get 40 Credits free to summarize 40 pages. Upload PDF files upto 200 MB in size. Get a high-quality summary with all facts. Download summary as PDF in just 3 clicks. Read annual reports, industry reports faster. Summarize legal documents, case studies. Read research papers and books in 70% less time.
WriterBuddy - Your Ultimate Summary Generator Welcome to WriterBuddy, where we revolutionize your reading and writing process with our state-of-the-art AI-powered Text Summarizer. Whether you're summarizing academic papers, creating concise summaries of lengthy articles, or drafting key points for reports, WriterBuddy is designed to make ...
Resoomer will then generate a summary and display it in a new window. It is also integrated with a number of other research tools, such as Google Scholar and Mendeley. #5. Iris.AI. Iris.AI is an advanced AI-based summarization tool that can effectively condense lengthy scholarly paper into concise summaries.
One of the standout features of Jenni AI is its support for five languages, making it accessible to a global audience.. Additionally, the tool allows you to produce 200+ words per day, which is ideal for consistent progress on lengthy academic projects.With 5+ user seats available, Jenni AI is perfect for collaborative research efforts, enabling multiple users to contribute to a single project.
Scite has two main components. Scite Search lets you explore citation links between papers, and whether citations are positive, negative etc. Scite Assistant is the AI research assistant: SciSpace: You can use SciSpace without creating an account. There is a free basic plan, and paid plans for extra features and capabilities : Unknown
A recent article in The Intelligencer highlighted a growing concern with AI summarizations, particularly the fact that these models aren't perfect. For instance, when summarizing your email, a model might get details like a date or location wrong. These seemingly minor errors can have significant consequences, especially when we rely on AI to handle information that we would otherwise read ...
In this paper, the application of artificial intelligence, especially neural networks, in the field of water treatment is comprehensively reviewed, with emphasis on water quality prediction and chemical dosage optimization. It begins with an overview of machine learning and deep learning concepts relevant to water treatment. Key advances and challenges in using neural networks for coagulation ...
Assembling such a global stocktake of effective climate policy interventions is so far hampered by two main obstacles: First, even though there is a plethora of data on legislative frameworks and pledged national emission reductions (8-10), systematic and cross-nationally comparable data about the specific types and mixes of implemented policy instruments are lacking.
Previous AI research has revealed bias against racialized groups but focused on overt instances of racism, naming racialized groups and mapping them to their respective stereotypes, for example by ...
This paper reviews the research progress on various genetic factors involved in thrombotic diseases, analyzes the advantages and disadvantages of commonly used thrombotic risk assessment scales and the characteristics of ideal scoring scales, and explores the application of artificial intelligence in the medical field, along with its future ...
The search for life signs and potentially habitable bodies in our solar system and beyond is one of NASA's top priorities. The prime destinations for such exploration are bodies containing liquid water, such as Jupiter's moon Europa and Saturn's moon Enceladus. Initial missions to these "ocean worlds" will be robotic, and because of long communication lags and blackouts, harsh ...