
An official website of the United States government
The .gov means it’s official. Federal government websites often end in .gov or .mil. Before sharing sensitive information, make sure you’re on a federal government site.
The site is secure. The https:// ensures that you are connecting to the official website and that any information you provide is encrypted and transmitted securely.
- Publications
- Account settings
Preview improvements coming to the PMC website in October 2024. Learn More or Try it out now .
- Advanced Search
- Journal List
- Belitung Nurs J
- v.8(4); 2022
- PMC10401366


Online ‘chatting’ interviews: An acceptable method for qualitative data collection
Joko gunawan.
1 Faculty of Nursing, Chulalongkorn University, Bangkok, Thailand
2 Belitung Raya Foundation, Manggar, East Belitung, Bangka Belitung, Indonesia
Colleen Marzilli
3 The University of Texas at Tyler, School of Nursing, 3900 University Blvd., Tyler, TX 75799, USA
Yupin Aungsuroch
Associated data.
Not applicable.
Qualitative research methods allow researchers to understand the experiences of patients, nurses, and other healthcare professionals. Qualitative research also provides scientists with information about how decisions are made and the aspects of existing interventions. However, to get to obtain this important information, qualitative research requires holistic, rich, and nuanced data that can be analyzed to determine themes, categories, or emerging patterns. Generally, offline or in-person interviews, focus group discussions, and observations are three core approaches to data collection. However, geographical barriers, logistic challenges, and emergency conditions, such as the COVID-19 pandemic have necessitated the utilization of online interviews, including chatting as an alternative way of collecting data. This editorial aims to discuss the possibility of online chat interviews as an acceptable design in qualitative data collection.
Data collection is the process of gathering information on variables of interest using accurate, authentic, systematic, and appropriate techniques to answer research questions, hypotheses, and desired outcomes. Rigorous data collection is essential to maintaining research integrity and scientific validity of study results (Barrett & Twycross, 2018 ).
Data collection methods are divided into two methods, namely secondary and primary data collection methods. Secondary data is from secondary sources, or sources not compiled directly by the researchers. The data may include published and unpublished works based on research that relies on primary sources (Rabianski, 2003 ). The secondary data collection method does not take long, and the resources of effort and cost are less. Secondary data is now growing as a preferred source of data for researchers due to the movement of open data science and the emergence of Open Access Initiatives (OAI). Along with open data and OAI, the accompanying policies that promote open access are an opportunity for researchers to gain access to data that may have been difficult to obtain in the past.
In contrast, primary data is real-time data, or first-hand obtained directly by researchers. This usually requires significant time, effort, and cost (Rabianski, 2003 ). Primary data collection methods are generally divided into quantitative and qualitative data. The quantitative data is based on mathematical calculations in various formats including inferential and descriptive statistics. The data is usually returned using a questionnaire with closed questions, which are then analyzed using the methods of correlation, regression, prediction, mean, mode, median, and other statistical methods. The other source of primary data is qualitative data, and with this type of data, mathematical calculations are not involved. Data analysis is obtained through words, sounds, feelings, emotions, body language, colors, and other elements that cannot be counted. Qualitative data collection is usually collected using interviews, focus group discussions, and observations which are the core approaches to this type of data collection (Barrett & Twycross, 2018 ). There are many reasons why a researcher may need quantitative or qualitative data, and this depends on the nature of the research, the concept and phenomena of interest, and the study objectives and hypothesis. Therefore, we do not need to argue if quantitative or qualitative data, secondary or primary data collection is best.
This editorial specifically discusses collecting qualitative data using the online “chatting” method. It should be noted that texting and chatting are often used interchangeably. However, there is a slight difference between the two terms. As nouns, “text” consists of various characters, glyphs, symbols, and sentences, but “chat” is an uncountable informal conversation (Wikidiff, n.d. ). As verbs, “text” is sending a text message using either a short message service (SMS) or a multimedia messaging service (MMS) between two or more users via a cellular network or internet connection using mobile devices, laptops, and other compatible computers (Wikidiff, n.d. ). While “chat” is engagement in an informal conversation, or to talk lightly and casually, discuss in an easy and familiar manner, or exchange messages (Free Dictionary, 2022 ). Texting is part of the chatting itself (Wikidiff, n.d. ). In other words, online chatting is defined as an informal conversation over the Internet that offers real-time transmission from the sender to the recipient. Chat messages are usually short so that the recipient responds quickly and is involved in the conversation (Wikipedia, 2022 ). In addition, chatting and instant messaging (IM) are similar, especially when using WhatsApp, Line, Messenger, or other apps. For the sake of consistency, chatting is used in this editorial.
Conversely, it should also be noted that the literature on online chatting as a qualitative data collection method is scarce and creates many contradictions because it is rarely used. Therefore, its validity and reliability are also often questioned. However, validity and reliability are not compromised when using chats for data collection, but the rationale for this method should be reasonable and justifiable. The following are reasons that can be used as references or strengths for the chatting method.
First, during the COVID-19 pandemic, the authors learned that it is tough to collect quantitative and qualitative research data, especially in social science, behavioral science, nursing science, or other disciplines related to humans. Face-to-face interviews are not possible because of the COVID-19 restrictions. This first point reflects that researchers cannot force the use of the typical data collection methods, such as face-to-face, focus groups, and direct observation. Instead, online chat interviews, or chatting, may be used as an alternative way of collecting data. It is not impossible that researchers may face situations like this pandemic again in the future, and that researchers have already prepared another way for data collection through chatting.
Second, in addition to the pandemic or emergency conditions, this chatting method is applicable for multi-settings research design. For example, it is common today to find studies conducted in various regions or comparisons in multiple countries, although they are constrained by geographical conditions. With the technology that supports internet-based chatting, researchers do not need to visit the research setting, which saves time and money (Stieger & Göritz, 2006 ). This provides a convenient option for researchers that eliminates barriers that create difficulties when collecting data from multiple sites across the globe.
Third, there is an argument about the use of telephone or online interviews instead of chatting. To answer this, the authors must first differentiate between telephone and online interviews. Telephone and online interviews are slightly different. Telephone/phone interviews are often conducted without being online, where researchers directly call, or voice-call research respondents through the contact number of the telephone device and mobile phone, or smartphone. Online interviews include (i) telephone/phone online interviews using voice-call features, (ii) video interviews using Zoom, Facetime, Skype, video conferencing, or other video apps, (iii) chat interviews using chat or messenger apps, and (iv) email texting. This online interview may be done formally and informally. However, email texting may not reflect a real-time conversation and take more time (Dowling, 2012 ). It is important to consider why researchers should use chatting instead of video or telephone interviews, and this is related to who and where, or the interview setting.
Who . Suppose researchers collect data on today’s young people, or Gen Z or the internet generation. In that case, the research participants may prefer to use online interviews, especially chatting, such as using Facebook messenger, WhatsApp, Instagram, Line, WeChat, KakaoTalk, and other apps. This is to reduce the formality of the interview itself, which sometimes makes respondents afraid, reluctant, or uncomfortable to answer questions in a formal manner. Researchers will rationally choose a method that makes participants feel comfortable and free to express their ideas and perspectives without limits.
The next element to consider is, why would the researchers prefer chatting over video interviews? Based on the authors’ experience in data collection, some respondents felt embarrassed to show their faces in front of the camera, were unconfident, and made the interview environment uncomfortable (Gunawan et al., 2022 ). Chatting was selected as a data collection technique to promote ease amongst participants. Researchers must also consider the needs and the conditions of the participants. If the participants have physical deficiencies, such as deafness, then it is not possible to conduct telephone or video interviews. Likewise, if the respondent is blind, chatting is not applicable.
Where . This is related to what applications are used, which is in accordance with the location of the target participants. For example, if the research participants are based in Indonesia, using WhatsApp is preferable (Gunawan et al., 2022 ). As of June 23, 2022, 148 million people in Indonesia use WhatsApp (Rizaty, 2022 ). WhatsApp has features for phone calls, video calls, chats, and voice delivery. Two studies (Gunawan et al., 2022 ; Gunawan et al., 2021 ) used WhatsApp in data collection, and the respondents were happy to answer questions using chat and voice recordings. However, in China, using WeChat is preferable for data retrieval (Weil et al., 2020 ). Both WhatsApp and WeChat have multiple features that enable options for various data collections in the form of words, chats, sounds, voices, videos, and even attached documents.
Fourth, repeated interviews are also an important factor to consider for chatting. It is not impossible that interviews need to be repeated after the initial analysis of the data. However, this often presents difficulties because it takes time to reschedule face-to-face or online phone/video interviews. Therefore, chatting is a practical and convenient solution to this problem, either to explore more data or to clarify the statements from the respondents. Based on Gunawan et al. ( 2022 ) related to research on COVID-19 vaccination, if there are two different statements from two research participants, a clarification is needed. For example, in a statement of “it is mandatory to bring a vaccine certificate to make a driver’s license,” one participant said yes, and the other said no. A confirmation is necessary between the two. As a result, the statement was clarified, “For those who want to make a driver’s license, people who have been vaccinated would be prioritized over people who have not; but, that does not mean they are not served, only the process is slowed down” (Gunawan et al., 2022 ). Chatting is an opportunity to clarify with less challenges.
Fifth, the practicality and validity of the chatting data collection method are noted. Chatting is more practical than telephone/video interviews. For example, when researchers conduct telephone/video interviews, audio or video are recorded, followed by verbatim transcription before data analysis, and this process is lengthy. Bryman ( 2012 ) said that transcribing a one-hour interview takes five to six hours and is costly. While in the online chatting method, all conversations, chats, voices, and attached documents are recorded automatically in the mobile app. Researchers can access the stored archive and re-read the content. Chatting facilitates efficient use of time wherein the researchers do not transcribe verbatim or use additional staff resources to transcribe the interviews. Validity of the data is essential for the researcher, and both chatting and telephone/video interviews require a significant amount of coding amongst the various data sources, but the contents and substances between both are not different (Saarijärvi & Bratt, 2021 ). The interviewers’ skills are needed to ask questions and receive answers according to the study purposes.
Additionally, although chatting can be considered an acceptable method for qualitative data collection, it has a weakness. For example, if the research topic or subject under study requires an in-depth interpretation technique where voice’ intonation, rhythm, and volume (emotional tone), as well as body language, are necessary, then chatting is limited and may be inappropriate. However, regardless of the advantages and disadvantages of the chatting method, researchers must control the quality of the data, and this should be addressed for each individual measurement, personal observation, and the entire data set according to the aims of the study.
There are two summary points in this editorial. First, the use of the online chatting method is acceptable if the conditions for video/online interviews are not possible or desirable, either due to limited conditions in the research settings or the study subjects. Second, using chatting as an additional data collection method is suitable if it makes sense and can be accounted. The data collected from different sources in a single study may provide trustworthy findings. However, researchers cannot impose one method for data collection. Freedom and flexibility are needed to gain more understanding of the phenomenon in order to obtain holistic, rich, and nuanced data.
Acknowledgment
Declaration of conflicting interest.
The author declares that they have no conflict of interest in this study.
Second Century Fund (C2F), Chulalongkorn University, Bangkok, Thailand.
Authors’ Contributions
All authors contributed equally in this article.
Authors’ Biographies
Joko Gunawan, S.Kep.Ners, PhD is Managing Editor of Belitung Nursing Journal. He is also Postdoctoral Researcher at the Faculty of Nursing, Chulalongkorn University, Bangkok, Thailand.
Colleen Marzilli, PhD, DNP, MBA, RN-BC, CCM, PHNA-BC, CNE, NEA-BC, FNAP is Associate Professor at the University of Texas at Tyler, USA. She is also on the Editorial Advisory Board of Belitung Nursing Journal.
Yupin Aungsuroch, PhD, RN is Associate Professor at the Faculty of Nursing, Chulalongkorn University, Bangkok, Thailand. She is also an Editor-in-Chief of Belitung Nursing Journal.
Data Availability
Ethical consideration.
- Barrett, D., & Twycross, A. (2018). Data collection in qualitative research . Evidence Based Nursing , 21 ( 3 ), 63-64. 10.1136/eb-2018-102939 [ PubMed ] [ CrossRef ] [ Google Scholar ]
- Bryman, A. (2012). Social research methods (4th ed.). Oxford: Oxford university press. [ Google Scholar ]
- Dowling, S. (2012). Online asynchronous and face-to-face interviewing: Comparing methods for exploring women’s experiences of breastfeeding long term . Cases in Online Interview Research , 277-296. 10.4135/9781506335155.n11 [ CrossRef ] [ Google Scholar ]
- Free Dictionary . (2022). Chat . https://www.thefreedictionary.com/chatting
- Gunawan, J., Aungsuroch, Y., Fisher, M. L., Marzilli, C., & Sukarna, A. (2022). Identifying and understanding challenges to inform new approaches to improve vaccination rates: A qualitative study in Indonesia . Journal of Nursing Scholarship . 10.1111/jnu.12793 [ CrossRef ] [ Google Scholar ]
- Gunawan, J., Aungsuroch, Y., Marzilli, C., Fisher, M. L., & Sukarna, A. (2021). A phenomenological study of the lived experience of nurses in the battle of COVID-19 . Nursing Outlook , 69 ( 4 ), 652-659. 10.1016/j.outlook.2021.01.020 [ PMC free article ] [ PubMed ] [ CrossRef ] [ Google Scholar ]
- Rabianski, J. S. (2003). Primary and secondary data: Concepts, concerns, errors, and issues . The Appraisal Journal , 71 ( 1 ), 43-55. [ Google Scholar ]
- Rizaty, M. A. (2022). WhatsApp global users touch 2.2 billion figures until first quarter 2022 [in Bahasa] . https://databoks.katadata.co.id/datapublish/2022/06/23/pengguna-whatsapp-global-sentuh-angka-22-miliar-hingga-kuartal-i-2022
- Saarijärvi, M., & Bratt, E.-L. (2021). When face-to-face interviews are not possible: Tips and tricks for video, telephone, online chat, and email interviews in qualitative research . European Journal of Cardiovascular Nursing , 20 ( 4 ), 392-396. 10.1093/eurjcn/zvab038 [ PMC free article ] [ PubMed ] [ CrossRef ] [ Google Scholar ]
- Stieger, S., & Göritz, A. (2006). Using Instant Messaging for Internet-based interviews . Cyberpsychology & Behavior , 9 , 552-559. 10.1089/cpb.2006.9.552 [ PubMed ] [ CrossRef ] [ Google Scholar ]
- Weil, J., Karlin, N., & Lyu, Z. (2020). Mobile messenger apps as data-collection method among older adults: WeChat in a health-related survey in the People’s Republic of China . SAGE Publication. 10.4135/9781529707755 [ CrossRef ] [ Google Scholar ]
- Wikidiff . (n.d.). Texting vs chatting - What's the difference? https://wikidiff.com/chatting/texting
- Wikipedia . (2022). Online chat . https://en.wikipedia.org/wiki/Online_chat
- Search Menu
Sign in through your institution
- Browse content in Arts and Humanities
- Browse content in Archaeology
- Anglo-Saxon and Medieval Archaeology
- Archaeological Methodology and Techniques
- Archaeology by Region
- Archaeology of Religion
- Archaeology of Trade and Exchange
- Biblical Archaeology
- Contemporary and Public Archaeology
- Environmental Archaeology
- Historical Archaeology
- History and Theory of Archaeology
- Industrial Archaeology
- Landscape Archaeology
- Mortuary Archaeology
- Prehistoric Archaeology
- Underwater Archaeology
- Zooarchaeology
- Browse content in Architecture
- Architectural Structure and Design
- History of Architecture
- Residential and Domestic Buildings
- Theory of Architecture
- Browse content in Art
- Art Subjects and Themes
- History of Art
- Industrial and Commercial Art
- Theory of Art
- Biographical Studies
- Byzantine Studies
- Browse content in Classical Studies
- Classical History
- Classical Philosophy
- Classical Mythology
- Classical Numismatics
- Classical Literature
- Classical Reception
- Classical Art and Architecture
- Classical Oratory and Rhetoric
- Greek and Roman Papyrology
- Greek and Roman Epigraphy
- Greek and Roman Law
- Greek and Roman Archaeology
- Late Antiquity
- Religion in the Ancient World
- Social History
- Digital Humanities
- Browse content in History
- Colonialism and Imperialism
- Diplomatic History
- Environmental History
- Genealogy, Heraldry, Names, and Honours
- Genocide and Ethnic Cleansing
- Historical Geography
- History by Period
- History of Emotions
- History of Agriculture
- History of Education
- History of Gender and Sexuality
- Industrial History
- Intellectual History
- International History
- Labour History
- Legal and Constitutional History
- Local and Family History
- Maritime History
- Military History
- National Liberation and Post-Colonialism
- Oral History
- Political History
- Public History
- Regional and National History
- Revolutions and Rebellions
- Slavery and Abolition of Slavery
- Social and Cultural History
- Theory, Methods, and Historiography
- Urban History
- World History
- Browse content in Language Teaching and Learning
- Language Learning (Specific Skills)
- Language Teaching Theory and Methods
- Browse content in Linguistics
- Applied Linguistics
- Cognitive Linguistics
- Computational Linguistics
- Forensic Linguistics
- Grammar, Syntax and Morphology
- Historical and Diachronic Linguistics
- History of English
- Language Evolution
- Language Reference
- Language Acquisition
- Language Variation
- Language Families
- Lexicography
- Linguistic Anthropology
- Linguistic Theories
- Linguistic Typology
- Phonetics and Phonology
- Psycholinguistics
- Sociolinguistics
- Translation and Interpretation
- Writing Systems
- Browse content in Literature
- Bibliography
- Children's Literature Studies
- Literary Studies (Romanticism)
- Literary Studies (American)
- Literary Studies (Asian)
- Literary Studies (European)
- Literary Studies (Eco-criticism)
- Literary Studies (Modernism)
- Literary Studies - World
- Literary Studies (1500 to 1800)
- Literary Studies (19th Century)
- Literary Studies (20th Century onwards)
- Literary Studies (African American Literature)
- Literary Studies (British and Irish)
- Literary Studies (Early and Medieval)
- Literary Studies (Fiction, Novelists, and Prose Writers)
- Literary Studies (Gender Studies)
- Literary Studies (Graphic Novels)
- Literary Studies (History of the Book)
- Literary Studies (Plays and Playwrights)
- Literary Studies (Poetry and Poets)
- Literary Studies (Postcolonial Literature)
- Literary Studies (Queer Studies)
- Literary Studies (Science Fiction)
- Literary Studies (Travel Literature)
- Literary Studies (War Literature)
- Literary Studies (Women's Writing)
- Literary Theory and Cultural Studies
- Mythology and Folklore
- Shakespeare Studies and Criticism
- Browse content in Media Studies
- Browse content in Music
- Applied Music
- Dance and Music
- Ethics in Music
- Ethnomusicology
- Gender and Sexuality in Music
- Medicine and Music
- Music Cultures
- Music and Media
- Music and Religion
- Music and Culture
- Music Education and Pedagogy
- Music Theory and Analysis
- Musical Scores, Lyrics, and Libretti
- Musical Structures, Styles, and Techniques
- Musicology and Music History
- Performance Practice and Studies
- Race and Ethnicity in Music
- Sound Studies
- Browse content in Performing Arts
- Browse content in Philosophy
- Aesthetics and Philosophy of Art
- Epistemology
- Feminist Philosophy
- History of Western Philosophy
- Metaphysics
- Moral Philosophy
- Non-Western Philosophy
- Philosophy of Language
- Philosophy of Mind
- Philosophy of Perception
- Philosophy of Science
- Philosophy of Action
- Philosophy of Law
- Philosophy of Religion
- Philosophy of Mathematics and Logic
- Practical Ethics
- Social and Political Philosophy
- Browse content in Religion
- Biblical Studies
- Christianity
- East Asian Religions
- History of Religion
- Judaism and Jewish Studies
- Qumran Studies
- Religion and Education
- Religion and Health
- Religion and Politics
- Religion and Science
- Religion and Law
- Religion and Art, Literature, and Music
- Religious Studies
- Browse content in Society and Culture
- Cookery, Food, and Drink
- Cultural Studies
- Customs and Traditions
- Ethical Issues and Debates
- Hobbies, Games, Arts and Crafts
- Natural world, Country Life, and Pets
- Popular Beliefs and Controversial Knowledge
- Sports and Outdoor Recreation
- Technology and Society
- Travel and Holiday
- Visual Culture
- Browse content in Law
- Arbitration
- Browse content in Company and Commercial Law
- Commercial Law
- Company Law
- Browse content in Comparative Law
- Systems of Law
- Competition Law
- Browse content in Constitutional and Administrative Law
- Government Powers
- Judicial Review
- Local Government Law
- Military and Defence Law
- Parliamentary and Legislative Practice
- Construction Law
- Contract Law
- Browse content in Criminal Law
- Criminal Procedure
- Criminal Evidence Law
- Sentencing and Punishment
- Employment and Labour Law
- Environment and Energy Law
- Browse content in Financial Law
- Banking Law
- Insolvency Law
- History of Law
- Human Rights and Immigration
- Intellectual Property Law
- Browse content in International Law
- Private International Law and Conflict of Laws
- Public International Law
- IT and Communications Law
- Jurisprudence and Philosophy of Law
- Law and Politics
- Law and Society
- Browse content in Legal System and Practice
- Courts and Procedure
- Legal Skills and Practice
- Legal System - Costs and Funding
- Primary Sources of Law
- Regulation of Legal Profession
- Medical and Healthcare Law
- Browse content in Policing
- Criminal Investigation and Detection
- Police and Security Services
- Police Procedure and Law
- Police Regional Planning
- Browse content in Property Law
- Personal Property Law
- Restitution
- Study and Revision
- Terrorism and National Security Law
- Browse content in Trusts Law
- Wills and Probate or Succession
- Browse content in Medicine and Health
- Browse content in Allied Health Professions
- Arts Therapies
- Clinical Science
- Dietetics and Nutrition
- Occupational Therapy
- Operating Department Practice
- Physiotherapy
- Radiography
- Speech and Language Therapy
- Browse content in Anaesthetics
- General Anaesthesia
- Clinical Neuroscience
- Browse content in Clinical Medicine
- Acute Medicine
- Cardiovascular Medicine
- Clinical Genetics
- Clinical Pharmacology and Therapeutics
- Dermatology
- Endocrinology and Diabetes
- Gastroenterology
- Genito-urinary Medicine
- Geriatric Medicine
- Infectious Diseases
- Medical Toxicology
- Medical Oncology
- Pain Medicine
- Palliative Medicine
- Rehabilitation Medicine
- Respiratory Medicine and Pulmonology
- Rheumatology
- Sleep Medicine
- Sports and Exercise Medicine
- Community Medical Services
- Critical Care
- Emergency Medicine
- Forensic Medicine
- Haematology
- History of Medicine
- Browse content in Medical Skills
- Clinical Skills
- Communication Skills
- Nursing Skills
- Surgical Skills
- Browse content in Medical Dentistry
- Oral and Maxillofacial Surgery
- Paediatric Dentistry
- Restorative Dentistry and Orthodontics
- Surgical Dentistry
- Medical Ethics
- Medical Statistics and Methodology
- Browse content in Neurology
- Clinical Neurophysiology
- Neuropathology
- Nursing Studies
- Browse content in Obstetrics and Gynaecology
- Gynaecology
- Occupational Medicine
- Ophthalmology
- Otolaryngology (ENT)
- Browse content in Paediatrics
- Neonatology
- Browse content in Pathology
- Chemical Pathology
- Clinical Cytogenetics and Molecular Genetics
- Histopathology
- Medical Microbiology and Virology
- Patient Education and Information
- Browse content in Pharmacology
- Psychopharmacology
- Browse content in Popular Health
- Caring for Others
- Complementary and Alternative Medicine
- Self-help and Personal Development
- Browse content in Preclinical Medicine
- Cell Biology
- Molecular Biology and Genetics
- Reproduction, Growth and Development
- Primary Care
- Professional Development in Medicine
- Browse content in Psychiatry
- Addiction Medicine
- Child and Adolescent Psychiatry
- Forensic Psychiatry
- Learning Disabilities
- Old Age Psychiatry
- Psychotherapy
- Browse content in Public Health and Epidemiology
- Epidemiology
- Public Health
- Browse content in Radiology
- Clinical Radiology
- Interventional Radiology
- Nuclear Medicine
- Radiation Oncology
- Reproductive Medicine
- Browse content in Surgery
- Cardiothoracic Surgery
- Gastro-intestinal and Colorectal Surgery
- General Surgery
- Neurosurgery
- Paediatric Surgery
- Peri-operative Care
- Plastic and Reconstructive Surgery
- Surgical Oncology
- Transplant Surgery
- Trauma and Orthopaedic Surgery
- Vascular Surgery
- Browse content in Science and Mathematics
- Browse content in Biological Sciences
- Aquatic Biology
- Biochemistry
- Bioinformatics and Computational Biology
- Developmental Biology
- Ecology and Conservation
- Evolutionary Biology
- Genetics and Genomics
- Microbiology
- Molecular and Cell Biology
- Natural History
- Plant Sciences and Forestry
- Research Methods in Life Sciences
- Structural Biology
- Systems Biology
- Zoology and Animal Sciences
- Browse content in Chemistry
- Analytical Chemistry
- Computational Chemistry
- Crystallography
- Environmental Chemistry
- Industrial Chemistry
- Inorganic Chemistry
- Materials Chemistry
- Medicinal Chemistry
- Mineralogy and Gems
- Organic Chemistry
- Physical Chemistry
- Polymer Chemistry
- Study and Communication Skills in Chemistry
- Theoretical Chemistry
- Browse content in Computer Science
- Artificial Intelligence
- Computer Architecture and Logic Design
- Game Studies
- Human-Computer Interaction
- Mathematical Theory of Computation
- Programming Languages
- Software Engineering
- Systems Analysis and Design
- Virtual Reality
- Browse content in Computing
- Business Applications
- Computer Security
- Computer Games
- Computer Networking and Communications
- Digital Lifestyle
- Graphical and Digital Media Applications
- Operating Systems
- Browse content in Earth Sciences and Geography
- Atmospheric Sciences
- Environmental Geography
- Geology and the Lithosphere
- Maps and Map-making
- Meteorology and Climatology
- Oceanography and Hydrology
- Palaeontology
- Physical Geography and Topography
- Regional Geography
- Soil Science
- Urban Geography
- Browse content in Engineering and Technology
- Agriculture and Farming
- Biological Engineering
- Civil Engineering, Surveying, and Building
- Electronics and Communications Engineering
- Energy Technology
- Engineering (General)
- Environmental Science, Engineering, and Technology
- History of Engineering and Technology
- Mechanical Engineering and Materials
- Technology of Industrial Chemistry
- Transport Technology and Trades
- Browse content in Environmental Science
- Applied Ecology (Environmental Science)
- Conservation of the Environment (Environmental Science)
- Environmental Sustainability
- Environmentalist Thought and Ideology (Environmental Science)
- Management of Land and Natural Resources (Environmental Science)
- Natural Disasters (Environmental Science)
- Nuclear Issues (Environmental Science)
- Pollution and Threats to the Environment (Environmental Science)
- Social Impact of Environmental Issues (Environmental Science)
- History of Science and Technology
- Browse content in Materials Science
- Ceramics and Glasses
- Composite Materials
- Metals, Alloying, and Corrosion
- Nanotechnology
- Browse content in Mathematics
- Applied Mathematics
- Biomathematics and Statistics
- History of Mathematics
- Mathematical Education
- Mathematical Finance
- Mathematical Analysis
- Numerical and Computational Mathematics
- Probability and Statistics
- Pure Mathematics
- Browse content in Neuroscience
- Cognition and Behavioural Neuroscience
- Development of the Nervous System
- Disorders of the Nervous System
- History of Neuroscience
- Invertebrate Neurobiology
- Molecular and Cellular Systems
- Neuroendocrinology and Autonomic Nervous System
- Neuroscientific Techniques
- Sensory and Motor Systems
- Browse content in Physics
- Astronomy and Astrophysics
- Atomic, Molecular, and Optical Physics
- Biological and Medical Physics
- Classical Mechanics
- Computational Physics
- Condensed Matter Physics
- Electromagnetism, Optics, and Acoustics
- History of Physics
- Mathematical and Statistical Physics
- Measurement Science
- Nuclear Physics
- Particles and Fields
- Plasma Physics
- Quantum Physics
- Relativity and Gravitation
- Semiconductor and Mesoscopic Physics
- Browse content in Psychology
- Affective Sciences
- Clinical Psychology
- Cognitive Psychology
- Cognitive Neuroscience
- Criminal and Forensic Psychology
- Developmental Psychology
- Educational Psychology
- Evolutionary Psychology
- Health Psychology
- History and Systems in Psychology
- Music Psychology
- Neuropsychology
- Organizational Psychology
- Psychological Assessment and Testing
- Psychology of Human-Technology Interaction
- Psychology Professional Development and Training
- Research Methods in Psychology
- Social Psychology
- Browse content in Social Sciences
- Browse content in Anthropology
- Anthropology of Religion
- Human Evolution
- Medical Anthropology
- Physical Anthropology
- Regional Anthropology
- Social and Cultural Anthropology
- Theory and Practice of Anthropology
- Browse content in Business and Management
- Business Ethics
- Business Strategy
- Business History
- Business and Technology
- Business and Government
- Business and the Environment
- Comparative Management
- Corporate Governance
- Corporate Social Responsibility
- Entrepreneurship
- Health Management
- Human Resource Management
- Industrial and Employment Relations
- Industry Studies
- Information and Communication Technologies
- International Business
- Knowledge Management
- Management and Management Techniques
- Operations Management
- Organizational Theory and Behaviour
- Pensions and Pension Management
- Public and Nonprofit Management
- Social Issues in Business and Management
- Strategic Management
- Supply Chain Management
- Browse content in Criminology and Criminal Justice
- Criminal Justice
- Criminology
- Forms of Crime
- International and Comparative Criminology
- Youth Violence and Juvenile Justice
- Development Studies
- Browse content in Economics
- Agricultural, Environmental, and Natural Resource Economics
- Asian Economics
- Behavioural Finance
- Behavioural Economics and Neuroeconomics
- Econometrics and Mathematical Economics
- Economic History
- Economic Systems
- Economic Methodology
- Economic Development and Growth
- Financial Markets
- Financial Institutions and Services
- General Economics and Teaching
- Health, Education, and Welfare
- History of Economic Thought
- International Economics
- Labour and Demographic Economics
- Law and Economics
- Macroeconomics and Monetary Economics
- Microeconomics
- Public Economics
- Urban, Rural, and Regional Economics
- Welfare Economics
- Browse content in Education
- Adult Education and Continuous Learning
- Care and Counselling of Students
- Early Childhood and Elementary Education
- Educational Equipment and Technology
- Educational Strategies and Policy
- Higher and Further Education
- Organization and Management of Education
- Philosophy and Theory of Education
- Schools Studies
- Secondary Education
- Teaching of a Specific Subject
- Teaching of Specific Groups and Special Educational Needs
- Teaching Skills and Techniques
- Browse content in Environment
- Applied Ecology (Social Science)
- Climate Change
- Conservation of the Environment (Social Science)
- Environmentalist Thought and Ideology (Social Science)
- Management of Land and Natural Resources (Social Science)
- Natural Disasters (Environment)
- Pollution and Threats to the Environment (Social Science)
- Social Impact of Environmental Issues (Social Science)
- Sustainability
- Browse content in Human Geography
- Cultural Geography
- Economic Geography
- Political Geography
- Browse content in Interdisciplinary Studies
- Communication Studies
- Museums, Libraries, and Information Sciences
- Browse content in Politics
- African Politics
- Asian Politics
- Chinese Politics
- Comparative Politics
- Conflict Politics
- Elections and Electoral Studies
- Environmental Politics
- Ethnic Politics
- European Union
- Foreign Policy
- Gender and Politics
- Human Rights and Politics
- Indian Politics
- International Relations
- International Organization (Politics)
- Irish Politics
- Latin American Politics
- Middle Eastern Politics
- Political Behaviour
- Political Economy
- Political Institutions
- Political Methodology
- Political Communication
- Political Philosophy
- Political Sociology
- Political Theory
- Politics and Law
- Politics of Development
- Public Policy
- Public Administration
- Qualitative Political Methodology
- Quantitative Political Methodology
- Regional Political Studies
- Russian Politics
- Security Studies
- State and Local Government
- UK Politics
- US Politics
- Browse content in Regional and Area Studies
- African Studies
- Asian Studies
- East Asian Studies
- Japanese Studies
- Latin American Studies
- Middle Eastern Studies
- Native American Studies
- Scottish Studies
- Browse content in Research and Information
- Research Methods
- Browse content in Social Work
- Addictions and Substance Misuse
- Adoption and Fostering
- Care of the Elderly
- Child and Adolescent Social Work
- Couple and Family Social Work
- Direct Practice and Clinical Social Work
- Emergency Services
- Human Behaviour and the Social Environment
- International and Global Issues in Social Work
- Mental and Behavioural Health
- Social Justice and Human Rights
- Social Policy and Advocacy
- Social Work and Crime and Justice
- Social Work Macro Practice
- Social Work Practice Settings
- Social Work Research and Evidence-based Practice
- Welfare and Benefit Systems
- Browse content in Sociology
- Childhood Studies
- Community Development
- Comparative and Historical Sociology
- Disability Studies
- Economic Sociology
- Gender and Sexuality
- Gerontology and Ageing
- Health, Illness, and Medicine
- Marriage and the Family
- Migration Studies
- Occupations, Professions, and Work
- Organizations
- Population and Demography
- Race and Ethnicity
- Social Theory
- Social Movements and Social Change
- Social Research and Statistics
- Social Stratification, Inequality, and Mobility
- Sociology of Religion
- Sociology of Education
- Sport and Leisure
- Urban and Rural Studies
- Browse content in Warfare and Defence
- Defence Strategy, Planning, and Research
- Land Forces and Warfare
- Military Administration
- Military Life and Institutions
- Naval Forces and Warfare
- Other Warfare and Defence Issues
- Peace Studies and Conflict Resolution
- Weapons and Equipment

- < Previous chapter
- Next chapter >
12 The Social Consequences of Online Interaction
Jenna L. Clark, Center for Advanced Hindsight at Duke University
Melanie C. Green, University at Buffalo
- Published: 10 September 2018
- Cite Icon Cite
- Permissions Icon Permissions
This chapter provides an overview of the social consequences of online interaction. Research has provided mixed evidence about whether online interaction is helpful or harmful for well-being and social connectedness. This chapter highlights the factors that moderate the influence of online social interaction on outcomes, with a particular focus on user behaviors. The Interpersonal Connection Behaviors Framework suggests that the positive consequences of any given online interaction depend on the extent to which that interaction serves a relational purpose. Online interactions that promote connection build relationships and increase well-being via increased relational closeness and quality through processes such as self-disclosure and social support. Online interactions that do not promote connection are likely to fall prey to disadvantages like social comparison or loneliness.
Personal account
- Sign in with email/username & password
- Get email alerts
- Save searches
- Purchase content
- Activate your purchase/trial code
- Add your ORCID iD
Institutional access
Sign in with a library card.
- Sign in with username/password
- Recommend to your librarian
- Institutional account management
- Get help with access
Access to content on Oxford Academic is often provided through institutional subscriptions and purchases. If you are a member of an institution with an active account, you may be able to access content in one of the following ways:
IP based access
Typically, access is provided across an institutional network to a range of IP addresses. This authentication occurs automatically, and it is not possible to sign out of an IP authenticated account.
Choose this option to get remote access when outside your institution. Shibboleth/Open Athens technology is used to provide single sign-on between your institution’s website and Oxford Academic.
- Click Sign in through your institution.
- Select your institution from the list provided, which will take you to your institution's website to sign in.
- When on the institution site, please use the credentials provided by your institution. Do not use an Oxford Academic personal account.
- Following successful sign in, you will be returned to Oxford Academic.
If your institution is not listed or you cannot sign in to your institution’s website, please contact your librarian or administrator.
Enter your library card number to sign in. If you cannot sign in, please contact your librarian.
Society Members
Society member access to a journal is achieved in one of the following ways:
Sign in through society site
Many societies offer single sign-on between the society website and Oxford Academic. If you see ‘Sign in through society site’ in the sign in pane within a journal:
- Click Sign in through society site.
- When on the society site, please use the credentials provided by that society. Do not use an Oxford Academic personal account.
If you do not have a society account or have forgotten your username or password, please contact your society.
Sign in using a personal account
Some societies use Oxford Academic personal accounts to provide access to their members. See below.
A personal account can be used to get email alerts, save searches, purchase content, and activate subscriptions.
Some societies use Oxford Academic personal accounts to provide access to their members.
Viewing your signed in accounts
Click the account icon in the top right to:
- View your signed in personal account and access account management features.
- View the institutional accounts that are providing access.
Signed in but can't access content
Oxford Academic is home to a wide variety of products. The institutional subscription may not cover the content that you are trying to access. If you believe you should have access to that content, please contact your librarian.
For librarians and administrators, your personal account also provides access to institutional account management. Here you will find options to view and activate subscriptions, manage institutional settings and access options, access usage statistics, and more.
Our books are available by subscription or purchase to libraries and institutions.
Month: | Total Views: |
---|---|
October 2022 | 7 |
November 2022 | 10 |
December 2022 | 8 |
January 2023 | 16 |
February 2023 | 23 |
March 2023 | 13 |
April 2023 | 14 |
May 2023 | 16 |
June 2023 | 5 |
July 2023 | 12 |
August 2023 | 3 |
September 2023 | 9 |
October 2023 | 19 |
November 2023 | 11 |
December 2023 | 8 |
January 2024 | 1 |
February 2024 | 50 |
March 2024 | 28 |
April 2024 | 10 |
May 2024 | 23 |
June 2024 | 14 |
July 2024 | 7 |
August 2024 | 4 |
- About Oxford Academic
- Publish journals with us
- University press partners
- What we publish
- New features
- Open access
- Rights and permissions
- Accessibility
- Advertising
- Media enquiries
- Oxford University Press
- Oxford Languages
- University of Oxford
Oxford University Press is a department of the University of Oxford. It furthers the University's objective of excellence in research, scholarship, and education by publishing worldwide
- Copyright © 2024 Oxford University Press
- Cookie settings
- Cookie policy
- Privacy policy
- Legal notice
This Feature Is Available To Subscribers Only
Sign In or Create an Account
This PDF is available to Subscribers Only
For full access to this pdf, sign in to an existing account, or purchase an annual subscription.
Thank you for visiting nature.com. You are using a browser version with limited support for CSS. To obtain the best experience, we recommend you use a more up to date browser (or turn off compatibility mode in Internet Explorer). In the meantime, to ensure continued support, we are displaying the site without styles and JavaScript.
- View all journals
- Explore content
- About the journal
- Publish with us
- Sign up for alerts
- Open access
- Published: 10 May 2012
Emotional persistence in online chatting communities
- Antonios Garas 1 ,
- David Garcia 1 ,
- Marcin Skowron 2 &
- Frank Schweitzer 1
Scientific Reports volume 2 , Article number: 402 ( 2012 ) Cite this article
11k Accesses
85 Citations
47 Altmetric
Metrics details
- Applied physics
- Statistical physics, thermodynamics and nonlinear dynamics
- Theoretical physics
How do users behave in online chatrooms, where they instantaneously read and write posts? We analyzed about 2.5 million posts covering various topics in Internet relay channels and found that user activity patterns follow known power-law and stretched exponential distributions, indicating that online chat activity is not different from other forms of communication. Analysing the emotional expressions (positive, negative, neutral) of users, we revealed a remarkable persistence both for individual users and channels. I.e. despite their anonymity, users tend to follow social norms in repeated interactions in online chats, which results in a specific emotional “tone” of the channels. We provide an agent-based model of emotional interaction, which recovers qualitatively both the activity patterns in chatrooms and the emotional persistence of users and channels. While our assumptions about agent's emotional expressions are rooted in psychology, the model allows to test different hypothesis regarding their emotional impact in online communication.
Similar content being viewed by others
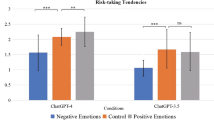
Risk and prosocial behavioural cues elicit human-like response patterns from AI chatbots
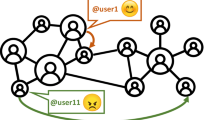
Affective polarization and dynamics of information spread in online networks
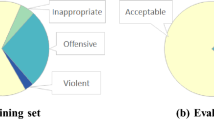
Dynamics of online hate and misinformation
Introduction.
How do human communication patterns change on the Internet? Round the clock activities of Internet users put us into the comfortable situation of having massive data from various sources available at a fine time resolution. But what to look at? Which aggregated measures are most appropriate to capture how new technologies affect our communicative behavior? And then, are we able to match these findings with a dynamic model that is able to generate insights into their origin? In this paper, we provide both: a new way of analysing data from online chats and a model of interacting agents to reproduce the stylized facts of our analysis. In addition to the activity patterns of users, we also analyse and model their emotional expressions that trigger the interactions of users in online chats. Validating our agent-based model against empirical findings allows us to draw conclusions about the role of emotions in this form of communication.
Online communication can be seen as a large-scale social experiment that constantly provides us with data about user activities and interactions. Consequently, time series analyses have already revealed remarkable temporal activity patterns, e.g. in email communication. Such patterns allow conclusions how humans organize their time and give different priorities to their communication tasks 1 , 2 , 3 , 5 , 6 , 7 . One particular quantity to describe these patterns is the distribution P (τ) of the waiting time τ that elapses before a particular user answers e.g. an email. Different studies have confirmed the power-law nature of this distribution, P (τ) ~ τ −α . Its origin was attributed either to the burstiness of events 2 or to circadian activity patterns 3 , while a recent work shows that a combination of both effects is also a plausible scenario 4 . However, the value of the exponent α is still debated. A stochastic priority queue model 6 allows to derive α by comparing two different rates, the average rate λ of messages arriving and the average rate µ of processing messages. If µ ≤ λ, i.e. if messages arrive faster than they can be processed, α = 3/2 was found, which is compatible with most empirical findings and simulation models 1 , 2 , 3 , 8 . However, in the opposite case, µ ≥ λ, i.e. if messages can be processed upon arrival, α = 5/2 was found together with an exponential correction term. The latter regime, also denoted as the “highly attentive regime”, could be verified empirically so far only by using data about donations 7 . So, it is an interesting question to analyze other forms of online communication to see whether there is evidence for the second regime.
In this paper, we analyze data about instant online communication in different chatting communities, specifically Internet Relay Chat (IRC) channels, where each channel covers a particular topic. Prior to the very common social networking sites of today, IRC channels provided a safe and independent way for users to share and discuss information outside traditional media. Different from other types of online communication, such as blogs or fora where entries are posted at a given time (decided by the writer), IRC chats are instantaneous in real time, i.e. users read while the post is written and can react immediately. This type of interaction requires much higher user activity in comparison to persistent communication e.g. in fora. Further, it is more spontaneous, often leading to emotionally-rich communication between involved peers. Consequently, instant communication should require specific tools and models for analysis, that are capable of covering these predominant features.
Nowadays, IRC channels are still one of the most used platforms for collective real-time online communication and are used for various purposes, e.g. organization of open-source project development, Internet activism, dating, etc. Our dataset (described in detail in the data section), consists of 20 IRC channels covering topics as diverse as music, sports, casuals chats, business, politics, or computer related issues – which is important to ensure that there is no topical bias involved in our analysis. For each channel, we have consecutive daily recordings of the open discussion over a period of 42 days, which amounts to more than 2.5 million posts in total generated by more than 20.000 different users.
We process our analysis as follows: first, we look into the communication patterns of instant online discussions, to find out about the average response time of users and its possible dependence on the topics discussed. This shall allow us to identify differences between instantaneous chatting communities and other forms of slower, persistent communication. In a second step, we look more closely into the content of the discussions and how they depend on the emotions expressed by users. Remarkably, we find that most users are very persistent in expressing their positive or negative emotions - which is not expected given the variety of topics and the user anonymity. This leads us to the question in what respect online chats are different from offline discussions which are mostly guided by social norms. We argue that even in instantaneous, anonymous online chats users behave very much like “normal” people. Our quantitative insights into user's activity patters and their emotional expressions are eventually combined to model interacting emotional agents. We demonstrate that the stylised facts of the emotional persistence can be reproduced by our model by only calibrating a small set of agent features. This success indicates that our modeling framework can be used to test further hypothesis about emotional interaction in online communities.
User activity patterns
An IRC channel is always active and enables the real time exchange of posts among users about a specific topic. User interaction is instantaneous, the post written by user u 1 is immediately visible to all other users logged into this channel and user u 2 may reply right away. Fig. 1 illustrates the dynamics in such a channel. As time evolves new users may enter, others may leave or stay quiet until they write follow-up posts at a later time.
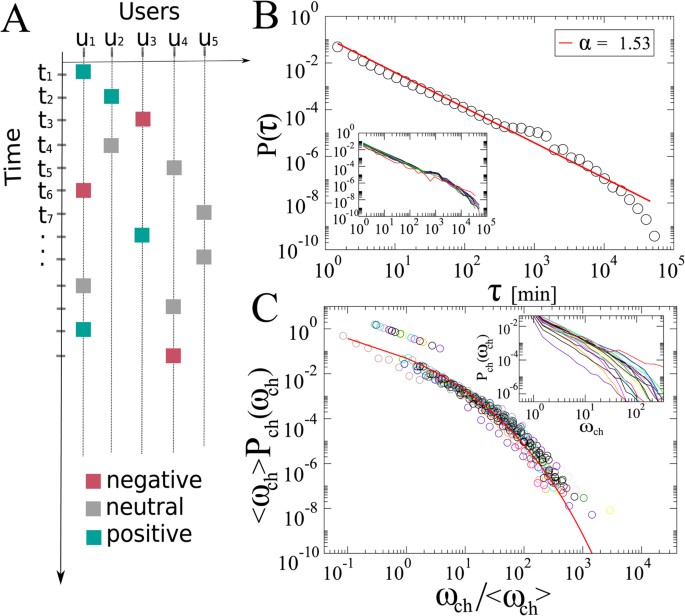
Communication activity over an IRC channel.
A) Schema of the evolution of a conversation in an IRC channel. At every time step, a user enters a post expressing a positive, negative, or neutral emotion. B) Probability distribution of the user activity over all the IRC channels. The activity is expressed as the time interval τ between two consecutive posts of the same user. Inset: Probability distribution of the user activity for individual IRC channels. The time is measured in minutes. C) Scaled probability distribution of the time interval ω ch between consecutive posts entered in all the 20 IRC channels. The solid line represents stretched exponential fit to the data. Inset: Probability distribution of the time interval ω ch between consecutive posts entered in all the 20 IRC channels without rescaling. The time is measured in minutes.
To characterize these activity patterns, we analyzed the waiting-time, or inter-activity time distribution P (τ), where τ refers to the time interval between two consecutive posts of the same user in the same channel and ask about the average response time. We find that τ is power-law distributed P (τ) ~ τ −α with some cut-off ( Fig. 1B ), with an exponent α = 1.53 ± 0.02. The fit is based on the maximum likelihood approach proposed by Clauset et al. 9 and the power-law nature of the distribution could not be rejected ( p = 0.375).
This finding (a) is inline the power-law distribution already found for diverse human activities 1 , 2 , 3 , 5 , 6 , 7 and (b) classifies the communication process as belonging to the regime where posts arrive faster than they can be processed. We note that for α < 2, no average response time is defined (which would have been the case, however, for the highly attentive regime). Further, we observe in the plot of Fig. 1B a slight deviation from the power-law at a time interval of about one day, which shows that some users have an additional regularity in their behavior with respect to the time of the day they enter the online discussion. Such deviations were usually treated as power-laws with an exponential cut-off and can even be explained based on simple entropic arguments 10 , 11 . However, because of the “bump” around the one day time interval, our distribution also seems to provide further evidence to the bi-modality proposed by Wu et al. 12 . We should note, however, that the tail is better fitted by a log-normal distribution (KS = 0.136) rather than an exponential (KS = 0.190) or a Weibull (KS = 0.188) one (again using the maximum likelihood methodology described by Clauset et al. 9 ) as shown in Fig. 1B . Here, KS stands for the Kolmogorov-Smirnov statistical test; the smaller this number, the better the fit.
We now focus on an important difference between online chats and previously studied forms of communication, such as mail or email exchange, which mostly involve two participants. Due to the collective nature of chats, a chatroom automatically aggregates the posts of a much larger amount of users, which allows us to study their collective temporal behavior. If ω denotes the time interval between two consecutive posts in the same channel independent of any user (also denoted as inter-event time and to be distinguished from the inter-activity time characterizing a single user), we find that the distribution P (ω) is is still fat-tailed, but does not follow a power-law. Interestingly, the time interval between posts significantly depends on the topic discussed in the channel (Inset of Fig. 1C ). Some “hot” topics receive posts at a shorter rate than others, which can be traced back to the different number of users involved into these discussions. Specifically, we find that the average inter-event time 〈ω〉 ch depends on the amount of users in the conversation and becomes smaller for more popular channels, as one would expect.
If we rescale the channel dependent inter-event distribution P ch (ω) using the average inter-event time 〈ω〉 ch per channel and plot 〈ω ch 〉 P ch (ω ch ) versus ω ch /〈ω ch 〉, we find that all the curves collapse into one master curve ( Fig. 1C ). The general scaling form that we used is P (ω) = (1/<ω>) F (ω/<ω>), where F(x) is independent of the average activity level of the component and represents a universal characteristic of the particular system. Such scaling behavior was reported previously in the literature describing universal patterns in human activity 13 . We fit this master curve by a stretched exponential 14 , 15 , 16

where the stretched exponent γ is the only fit parameter, while the other two factors a γ and β γ are dependent on γ 14 . A histogram of the γ values across the 20 channels is shown in Supplementary Figure S2 . Using only the regression results with p < 0.001 we find that the mean value of the stretched exponents is 〈γ〉 = 0.21 ± 0.05.
We note that stretched exponentials have been reported to describe the inter-event time distribution in systems as diverse as earthquakes 15 and stock markets 16 . These systems commonly exhibit long range correlations which seem to be the origin of the stretched exponential inter-event time distributions 14 . Long range correlations have also been reported in human interaction activity 5 , 17 and we tested their presence in the temporal activity over IRC communication. As shown in the Supplementary Figure S3 , we verified the existence of long range correlations in the conversation activity. We found that the decay of the autocorrelation function of the inter-event time interval between consecutive posts within a channel is described by a power-law

In conclusion, our analysis of user activities have revealed a universal dynamics in online chatting communities which is moreover similar to other human activities. This regards (a) the temporal activity of individual users (characterized by a power-law distribution with exponent 3/2) and (b) the inter-event dynamics across different channels, if rescaled by the average inter-event time (characterized by a stretched exponential distribution with just one fit parameter). We will use these findings as a point of departure for a more in-depth analysis – because obviously the essence of online communication in chatrooms, as compared to other human activities, is not really covered. From the perspective of activity patters, there is not so much new here, which leads us to ask for other dimensions of human communication that could reveal a difference.
Emotional expression patterns
Human communication, in addition to the mere transmission of information, also serves purposes such as the reinforcement of social bonds. This could be one of the reasons why human languages are found to be biased towards using words with positive emotional charge 20 . Humans, from the early stages of our lives, develop an affective communication system that enables us to express and regulate emotions 21 . But emotions are also the mediators of our consumer responses to advertising 22 and many scientists acknowledge their importance in motivating our cognition and action 23 . However, despite the increasing time we spend online, the way we express our emotions in online communities and its impact on possibly large amounts of people is still to be explored.
Consequently, we are interested in the role of expressed emotions in online chatting communities. Users, by posting text in chatrooms, also reveal their emotions, which in return can influence the emotional response of other users, as illustrated in Fig. 1A . To understand this emotional interaction, we carry out a sentiment analysis of each post which is described in detail in the Methods section. This automatic classification returns the valence v for each post, i.e. a discrete value {−1, 0, +1} that characterizes the emotional charge as either negative, neutral, or positive.
Instead of using the real time stamp of each post as in the analysis of the user activity, we now use an artificial time scale in which at each (discrete) time step one post enters the discussion, so the number of time steps equals the total number of posts. We then monitor how the total emotion expressed in a given channel evolves over time. We use a moving average approach that calculates the mean emotional polarity over different time windows. In Fig. 2A we plot the fraction of neutral, negative and positive posts as a function of time, for different sizes of the time window. While it is obvious that the emotional content largely fluctuates when using a very small time window, we find that for decreasing time resolution (i.e. increasing time window) the fractions of emotional posts settle down to an almost constant value around which they fluctuate. From this, we can make two interesting observations: (i) the emotional content in the online chats does not really change in the long run (one should notice that times of the order 10 3 are still large compared to the time window DT = 50 used), i.e. we observe fluctuations that depend on the time resolution, but no “evolution” towards more positive or negative sentiments. (ii) For the low resolution, the fraction of neutral posts dominates the positive and negative posts at all times. In fact there is a clear ranking where the fraction of negative posts is always the smallest. Both observations become even more pronounced when averaging over the 20 IRC channels, as Fig. 2B shows.
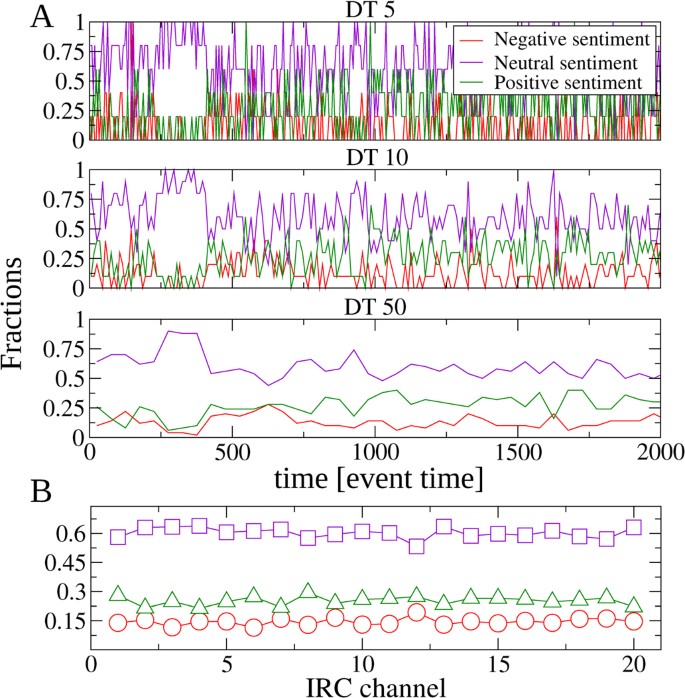
Emotional expressions over different time scales.
A) Fraction of expressions with negative, neutral and positive emotion values under different time scales for one channel. B) Fraction of expressions with negative, neutral and positive emotion values for the 20 IRC channels.
Our findings differ from previous observations of emotional communication in blog posts and forum comments which identified a clear tendency toward negative contributions over time, in particular for periods of intensive user activity 24 , 25 . Such findings suggest that an increased number of negative emotional posts could boost the activity and extend the lifetime of a forum discussion. However, blog communication in general evolves slower than e.g. online chats. Hence, we need to better understand the role of emotions in real time Internet communication, which obviously differs from the persistent and delayed interaction in blogs and fora.
To further approach this goal, we analyse to what extend the rather constant fraction of emotional posts in IRC channels is due to a persistence in the emotional expressions of users. For this, we apply the DFA technique 18 , to the time series of positive, negative and neutral posts. Since our focus is now on the user, we reconstruct for every user a time series that consists of all posts communicated in any channel, where the time stamp is given by the consecutive number at which the post enters the user's record. In order to have reliable statistics, for the further analysis only those users with more than 100 posts are considered (which are nearly 3000 users). As the examples in the Supplementary Figure S4 show, some users are very persistent in their (positive) emotional expressions (even that they occasionally switch to neutral or negative posts), whereas others are really antipersistent in the sense that their expressed emotionality rapidly changes through all three states. The persistence of these users can be characterized by a scalar value, the Hurst exponent H , (see the Material and Methods Section for details) which is 0.5 if users switch randomly between the emotional states, larger than 0.5. if users are rather persistent in their emotional expressions, or smaller than 0.5 if users have strong tendency to switch between opposite states, as the antipersistent time series of Fig. S4 shows.
If we analyse the distribution of the Hurst exponents of all users, shown in the histogram of Fig. 3A , we find (a) that the emotional expression of users is far from being random and (b) that it is clearly skewed towards H > 0.5, which means that the majority of users is quite persistent regarding their positive, negative or neutral emotions. This persistence can be also seen as a kind of memory (or inertia) in changing the emotional expression, i.e. the following post from the same user is more likely to have the same emotional value.
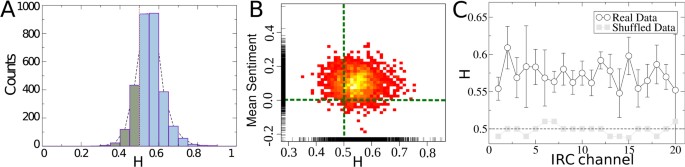
Hurst exponents and emotional persistence.
A) Hurst exponents ( H ) of the emotional expression of individual users, obtained using the DFA method. Only users contributed more than 100 posts were considered and we used the exponents obtained with fitting quality R 2 > 0.98. B) Hurst exponent ( H ) versus the mean emotion polarity expressed by individual users, again only from users who contributed more than 100 posts. C) Hurst exponents ( H ) of the emotions expressed in the 20 IRC channels. The values are averages of the Hurst exponents obtained from 10 different segments of the same channel and the error bars show the standard deviation. The horizontal dashed line shows the expected value for random time series ( H = 0.5) and the gray squares show the value obtained from shuffling the real time series to destroy any correlations. The difference in exponents of the real and the shuffled time series is statistically significant with p < 0.001.
The question whether persistent users express more positive or negative emotions is answered in Fig. 3B , where we show a scatter plot of H versus the mean value of the emotions expressed by each user. Again, we verify that the majority of users has H > 0.5, but we also see that the mean value of emotions expressed by the persistent users is largely positive. This corresponds to the general bias towards positive emotional expression detected in written expression 20 . The lower left quadrant of the scatter plot is almost empty, which means that users expressing on average negative emotions tend to be persistent as well. A possible interpretation for this could be the relation between negative personal experiences and rumination as discussed in psychology 26 . Antipersistent users, on the other hand, mostly switch between positive and neutral emotions.
Are the more active users also the emotionally persistent ones? In Supplementary Figure S6 we show a scatter plot of the Hurst exponent dependent on the total activity of each user. Even though the mean value of H does not show any such dependence, we observe large heterogeneity on the values of H for users with low activity. Furthermore, in Supplementary Figure S7 we show that the Hurst exponent of a very active user varies only slightly if we divide his time series into various segments and apply the DFA method to these segments. Thus we can conclude that active users tend to be emotionally persistent and, as most persistent users express positive emotions, they tend to provide some kind of positive bias to the IRC, whereas users occasionally entering the chat may just try to get rid of some negative emotions.
This leads us to the question how persistent the emotional bias of a whole discussion is. While Fig. 3A has shown the persistence with respect to the different users, Fig. 3C plots the persistence for the different channels, which each feature a very different topic. This persistence holds even even if we analyse only certain segments of the channel, as it is shown in Supplementary Figure S8 . So, we conclude that the persistence of the discussion per se (which is different from the persistence of the users which can leave or enter a arbitrary times) reflects a certain narrative memory. Precisely, for each chat, we observe the emergence of a certain (emotional) ”tone” in the narration which can be positive, negative or neutral, dependent the emotional expressions of the (majority of) persistent users. If we reshuffle these time series such that the same total number of positive, negative and neutral posts is kept, but temporal correlations are destroyed, then the persistence is lost as well as Fig. 3C shows. We note that we could not find evidence of correlations using the autocorrelation function of the emotion time series, while the observed persistence in the fluctuations of user emotional expression, as captured by the Hurst exponent is very robust. This indicates that the chat community assumes an emotional memory locally encoded in the current messages (from the user perspective), while the size of the conversation is too large to detect it through averaging techniques.
An agent-based model for chatroom users
After identifying both the activity patterns and the emotional expression patterns of users in online chats, we setup an agent-based model that is able to reproduce these stylized facts. We start from a general framework 27 , designed to model and explain the emergence of collective emotions in online communities through the evolution of psychological variables that can be measured in experimental setups and psychological studies 28 , 29 . This framework provides a unified approach to create models that capture collective properties of different online communities and allows to compare the different emotional microdynamics present in various types of communication. The case of IRC channel communication is of particular interest because of its fast and ephemeral nature. Thus, we have designed a model for IRC chatrooms, as shown in Fig. 4A . The agents in our model are characterized by two variables, their emotionality, or valence, v which is either positive or negative and their activity, or arousal, which is represented by the time interval τ between two posts s in the chatroom. The valence of an agent i , represented by the internal variable v i , changes in time due to a superposition of stochastic and deterministic influences 27 , 30 :

The stochastic influences are modeled as a random factor A v ξ i normally distributed with zero mean and amplitude A v and represent all changes of the individual emotional state apart from chat communication. The deterministic influences are composed of an internal decay of parameter γ v and an external influence of the conversation. The change in the valence caused by the emotionality of the field ( h + − h − ) is measured in valence change per time unit through the parameter b . Previous models under the same framework 27 , 31 had an additional saturation term in the equation of the valence dynamics. This way the positive feedback between v and h was limited when the field was very large. But, as we show in Fig. 2 , chatrooms do not show the extreme cases of emotional polarization observed in other communities. Thus, we simplify the dynamics of the valence without using any saturation terms, since a large imbalance between h + and h − is unrealistic given our analysis of real IRC data.
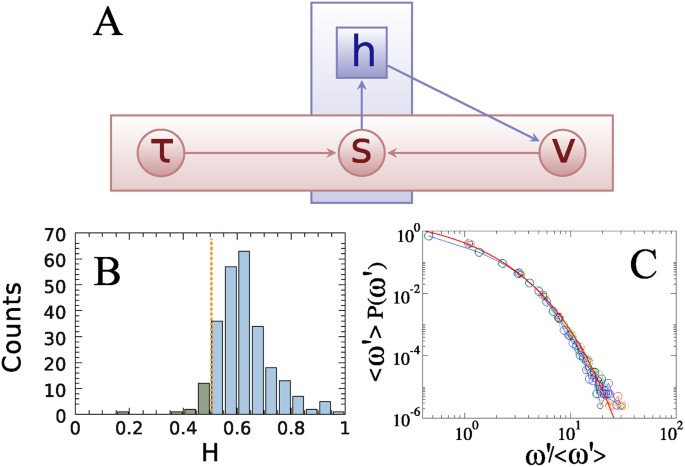
Modeling schema and simulation results.
A) Schematic representation of the model: The horizontal layer represents the agent, the vertical layer the communication in the chatroom where posts are aggregated. After a time lapse τ, which follows the power-law distribution of Fig. 1B , the agents writes a post s which implicitly expresses its emotions, v. Posts read in the chatroom feed back on the emotional state v of the agent. B) Hurst exponents for the individual behavior of agents in isolation with A v ∈ [0.2, 0.5] and γ v ∈ [0.2, 0.5]. Only the exponents derived with fitting quality R 2 > 0.9 are considered. C) Scaled probability distribution of the time interval ω′ between consecutive posts in 10 simulations of the model. Stretched exponential fit shows similar behavior to real IRC channel data.
In general, the level of activity associated with the emotion, known as arousal, can be explicitly modeled by stochastic dynamics as well 31 . Here, the activity of an agent is estimated by the time-delay distribution that triggers the expression of the agent, i.e. by the power-law distribution P (τ) ~ τ −1.53 shown in Fig. 1B . Assuming that an agent becomes active and expresses its emotion at time t , it will become active again after a period τ. The agent then writes a post in the online chat the emotional content of which is determined by its valence (see below). This information is stored in an external field common for all agents, which is composed of two components, h − and h + , for negative and positive information and their difference measures the emotional charge of the communication activity. Since we are interested in emotional communication, we assume that all neutral posts entered, or already present, in a chatroom do not influence the emotions of the agents participating to the conversation. Thus, the dynamics of the field is influenced only by the amount of agents expressing a particular emotion at a given time: N + ( t ) = Σ i (1 − Θ(−1 * s i )) and N − ( t ) = Σ i (1−Θ( s i )), where Θ is the Heaviside step function. Therefore, the time dynamics of the fields can be described as:

These two field components, h + and h − , decay exponentially with a constant factor γ h , i.e. their importance decays very fast as they move further down the screen (posts never disappear, but become less influential). Each field increases by a fixed amount c from every post stored in it. The values of the valence of the agents are changed by the field components, as described by Eq. 3. In contrast with traditional means of communication, online social media can aggregate much larger volumes of user-generated information. This is why h is defined without explicit bounds. Chatrooms pose a special case to this kind of communication, as they can contain large amount of posts but limited amount of users. Most IRC channels have technical limitations for the amount of users that can be connected at once, which in turn is reflected in the total amount of posts present in the general discussion. In our model, h might take any value, but the empirical activity pattern combined with the fixed size of the community dynamically constraints it to limited values.
Whenever an agent creates a new post in an ongoing conversation, the variable, s i , obtain its value in the following way:
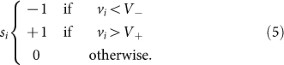
The thresholds V − and V + represent a limit value of the valence that determines the emotional content of each post and in general can be asymmetric, as humans tend to have different thresholds for the triggering of positive and negative emotional expression. Each action contributes to the amount of information stored in the information field of the conversation, increasing h − if s = −1 or h + if s = +1.
We emphasize that the way we model the agent behavior is very much in line with psychological research, where emotional states are represented by valence and arousal, following the dimensional representation of core affect 32 . The valence, v , represents the level of pleasure experienced by the emotional state, while the arousal represents the degree of activity induced by the emotional state and determines the moment when posts are created. Continuously the agent's valence relaxes to a neutral state and is subject to stochastic influences, as show empirically in 33 . The effect of chatroom communication on an agent's emotionality is modeled as an empathy-driven process 34 that influences the valence. In the valence dynamics we propose in Eq. 3, agents perceive a positive influence when their emotional state matches the one of the community and a negative one in the opposite case. When a post is created, its emotional polarity is determined by the valence, as it was suggested by experimental studies on social sharing of emotions 26 , 35 .
All the assumptions of our model are supported by psychological theories. Parameter values and dynamical equations can be tested against experiments in psychology, providing empirical validation for the emotional microdynamics 28 , 29 . Furthermore, our model provides a consistent view of the emotional behavior in chatrooms leading to testable hypotheses that can drive future psychology research.
We performed extensive computer simulations using different parameter sets (see supplementary material for details). By exploring the parameter space, we identified which parameter sets lead to similar conversation patterns as observed in the real data. We used such set to simulate chats in 10 channels and we analysed the agent's activity and their emotional persistence. The results are shown in Fig. 4B, C . Specifically, we find that (a) the distribution of Hurst exponents for individual agents is shifted towards positive values similar to the one observed in real data, this way reproducing the emotional persistence of the conversation without assuming any time dependence between user expressions. Further, we reproduce (b) the empirically observed stretched exponential distribution for the rescaled time delays ω′ between consecutive posts, without any further assumptions.
We do note, however, that the stretched exponent, γ = 0.59 ( p < 0.001), of the simulated distribution is different from real IRC channels where it was γ = 0.21, i.e. there is a faster decay in the simulations. This could be explained by the fact that in the real chat users usually write after they have read the previous post, i.e. there are additional correlations in the times users enter a chat. These, however, are not considered in the simulations, because agents post in the chat at random after a given time interval τ, i.e. there is no additional coupling in posting times. Following the same approach as we did for the real data, we calculated the Hurst exponent of the inter simulated event time-series of the discussions. We found that H ω′ = 0.75, however, we did not observe a power-law decay of the autocorrelation function (see Supplementary Figure S12 ). This suggests that the observed correlations are due to the power-law distributed inter-event times used as input to our model and it is inline with the above discussion about the absence of coupling that also explains the difference in the stretched exponents.
Eventually, we observe (c) the emotional persistence in the simulated conversations. The mean Hurst exponent for the 10 simulated channels is H s = 0.567 ± 0.007, whereas for the real IRC channels H r = 0.572 ± 0.021 was found. These results suggests that our agentbased model reproduces qualitatively the emergence of emotional persistence in the IRC conversation and thus, based on all findings, is able to capture the essence of emotional influence between users in chatrooms.
We started with the question to what extent human communication patterns change on the Internet. To answer this, we used a unique dataset of online chatting communities with about 2.5 million posts on 20 different topics. Our analysis considered two different dimensions of the communication process: (a) activity, expressed by the time intervals τ at which users contribute to the communication and ω at which consecutive posts appear in a chat and (b) the emotional expressions of users. With respect to activity patterns we did not find considerable differences between online chatrooms and other previously studied forms on online and offline communication. Specifically, both the inter-activity distribution of users and the inter-event distribution of posts followed the known distributions. Thus, we may conclude that humans do not really change their activity patterns when they go online. Instead, these patterns seem to be quite robust across online and offline communication.
The picture differs, however, when looking at the emotional expressions of users. While we cannot directly compare our findings on emotional persistence to results about offline communication, we find differences between online chatrooms and other forms online communication, such as blogs, fora. While the latter could be heated up by negative emotional patterns, we observe that online chats, which are instantaneous in time, very much follow a balanced emotional pattern across all topics (shown in the emotional persistence of the channels), but also with respect to individual users, which are in their majority quite persistent in their emotional expressions (mostly positive ones).
This observation is indeed surprising as online chats are mostly anonymous, i.e. users do not reveal their personal identity. However, they still seem to behave according to certain social norms, i.e. there is a clear tendency to express an opinion in a neutral to positive emotional way, avoiding direct confrontations or emotional debates. One of the reasons for such behavior comes from the “repeated interaction” underlying online chats. As the daily “bump” the activity patterns also suggest, most users return to the online chats regularly, to meet other users they may already know. This puts a kind of social pressure on their behavior (even in an unconscious manner) to behave similar to offline conversations. In conclusion, we find that the online communication patters do not differ much from common offline behavior if a repeated interaction could be assumed.
Eventually, we argue that the emotional persistence found is indeed related to the nature of human conversations. After all, the correlations shown in the emotional expressions of different users indicate that there is some form of emotional sharing between participants. This suggests the presence of social bonds among users in the chatroom 26 and confirms similarities between online and offline communication.
The fact that we could reveal patterns of emotional persistence both in users and in topics discussed, does not mean that we also understand their origin. One important step towards this ”microscopic” understanding is provided by our agent-based model of emotional interactions in chatrooms. By using assumptions about the agent's behavior which are rooted in research in psychology, we are able to reproduce the stylized facts of the chatroom conversation, both for the activity in channels and for the emotional persistence. Specifically, our model allows us to test hypotheses about the emotional interaction of agents against their outcome on the systemic level, i.e. for the chatroom simulation. This helps to reveal what kind of rules are underlying the online behavior of users which are hard to access otherwise.
Data collection and classification
The data used in this article is based on a large set of public channels from EFNET Internet Relay Chats ( http://www.efnet.org ), to which any user can connect and participate in the conversation. Based on the assessment of the initially downloaded set of recordings, 20 IRC channels were selected aiming to provide a large number of consecutive daily logs with transcripts of vivid discussions between the channel participants, measured in number of posts. The finally used data set contained consecutive recordings for 42 days spanning the period from 04-04-2006 to 15-05-2006.
The general topics of discussions from the selected channels include: music, sports, casuals chats, business, politics and topics related to computers, operating systems or specific computer programs. The IRC data set contains 2,688,760 posts. The total number of participants to all this channels is 25,166. However, because some people participate to more than one channel, the total number of unique participants is 20,441. On average, the data set provides 3055 posts per day. In the recorded period 15 users created more than 10000 posts. The distribution of the user participation i.e. the number of posts entered by every user, is shown in Supplementary Figure S1 . The mean of the distribution is 97 posts per user and as we can see from Fig. S1 , it is skewed with most of the users contributing only a small number of posts.
The acquired data was anonymized by substituting real user ids to random number references. The text of each post was cleaned by spam detection and substitution of URL links to avoid them from influencing the emotion classification. The emotional content was extracted by using the SentiStrength classifier 36 , which provides two scores for positive and negative content. Each score ranges from 1 to 5 and changes with the appearance of emotion bearing terms from a lexicon of affective word usage, specifically designed for this purpose. Each word of the lexicon has a value on the scale of −5 to 5 which determines the strength of the emotion attached to it. The classifier takes into account syntactic rules like negation, amplification and reduction and detects repetition of letters and exclamation signs as amplifiers. When one of this patterns is detected, SentiStrength applies transformation rules to the contribution of the involved terms to the sentence scores. It has been designed to analyze online data and considers Internet language by detecting emoticons and correcting spelling mistakes.
The perception of emotional expression varies largely across humans and traditional accuracy metrics are not useful when there is lack of an objective space. Human ratings of emotional texts have certain degree of disagreement that needs to be considered by sentiment analysis in order to have a valid quantification of emotions. SentiStrength scores are consistent with the level of disagreement between humans about how they perceive written emotional expressions 37 . This classifier combines an emotion quantization of proved validity with a high accuracy and is considered the state of the art in sentiment detection 38 . Due to the short length of the posts in chatrooms, we calculate a polarity measure by comparing the two different scores of SentiStrength. The sign of the difference of the positive and negative scores provides an approximation to detect positive, negative and neutral posts. The accuracy of this polarity metric was tested against texts tagged by humans and messages including emoticons from MySpace 39 and Twitter 40 , which are of a similar length to the ones in our chatroom data. The data are freely available for research purposes and are provided as Supplementary Material . Detailed information about their structure is provided in the “Data section” of the Supplementary Information text.
Detrended Fluctuation Analysis
The method of Detrended Fluctuation Analysis (DFA) 18 is a useful tool in revealing long-term memory and correlations in time series 5 , 15 , 16 . The method maps the system into a one-dimensional random walk and enable us to compare the properties of the real time series with the time series produced by the random case.

to calculate the root-mean-square fluctuation of the integrated and detrended time series and we characterize the relationship between the average fluctuation F (Δ t ) and the box size Δ t .
Typically, F (Δ t ) will increase with box size as F (Δ t ) ~ (Δ t ) H , which indicates the presence of power-law (fractal) scaling. Therefore, the fluctuations can be characterized only by the scaling exponent H that is analogous to the Hurst exponent 41 and it is calculated from the slope of the line relating log F (Δ t ) to logΔ t . If only short-range correlations (or no correlations) exist in the time series, then it has the statistical properties of a random walk. Therefore F (Δ t ) ~ (Δ t ) 1/2 . However, in the presence of long-range power-law correlations (i.e. no characteristic length scale) H ≠ 1/2. A value H < 1/2 signals the presence of long range anti-correlations, while a value H > 1/2 signals the presence of long range correlations (persistence).
Oliveira, J. G. & Barabási, A. L. Human Dynamics: The Correspondence Patterns of Darwin and Einstein. Nature 437, 1251 (2005).
Article CAS ADS Google Scholar
Barabási, A.-L. The origin of bursts and heavy tails in human dynamics. Nature 435, 207–11 (2005).
Article ADS Google Scholar
Malmgren, R. D., Stouffer, D. B., Motter, A. E. & Amaral, L. a. N. A Poissonian explanation for heavy tails in e-mail communication. Proc. Natl. Acad. Sci. U.S.A 105, 18153–8 (2008).
Jo, H.-H., Karsai, M., Kertész, J. & Kaski, K. Circardian pattern and burstiness in mobile phone communication. New J. Phys. 14, 013055 (2012).
Rybski, D., Buldyrev, S., Havlin, S., Liljeros, F. & Makse, H. Scaling laws of human interaction activity. Proc. Natl. Acad. Sci. U.S.A 106, 12640 (2009).
Grinstein, G. & Linsker, R. Power-law and exponential tails in a stochastic priority-based model queue. Phys. Rev. E 77, 012101 (2008).
Crane, R., Schweitzer, F. & Sornette, D. Power law signature of media exposure in human response waiting time distributions. Phys. Rev. E 81, 56101 (2010).
Vázquez, A. et al. Modeling bursts and heavy tails in human dynamics. Phys. Rev. E 73, 036127 (2006).
Clauset, A., Shalizi, C. R. & Newman, M. E. J. Power-law distributions in empirical data. SIAM Review 51, 661–703 (2009).
Article ADS MathSciNet Google Scholar
Baek, S. K., Bernhardsson, S. & Minnhagen, P. Zipf's law unzipped. New J. Phys. 13, 043004 (2011).
Adamic, L. Unzipping Zipf's law. Nature 474, 164 (2011).
Article CAS Google Scholar
Wu, Y., Zhou, C., Xiao, J., Kurths, J. & Schellnhuber, H. J. Evidence for a bimodal distribution in human communication. Proc. Natl. Acad. Sci. U.S.A 107, 18803–18808 (2010).
Candia, J. et al. Uncovering individual and collective human dynamics from mobile phone records. J. Phys. A 41, 224015 (2011).
Altmann, E. & Kantz, H. Recurrence time analysis, long-term correlations and extreme events. Phys. Rev. E 71, 56106 (2005).
Bunde, A., Eichner, J., Kantelhardt, J. & Havlin, S. Long-Term Memory: A Natural Mechanism for the Clustering of Extreme Events and Anomalous Residual Times in Climate Records. Phys. Rev. Lett. 94, 48701 (2005).
Wang, F., Yamasaki, K., Havlin, S. & Stanley, H. Scaling and memory of intraday volatility return intervals in stock markets. Phys. Rev. E 73, 026117 (2006).
Rybski, D., Buldyrev, S. V., Havlin, S., Liljeros, F., & Makse, H. A. Communication activity in social networks: growth and correlations. Eur. Phys. J. B 84, 147–159 (2011).
Peng, C.-K. et al. Mosaic organization of DNA nucleotides. Phys. Rev. E 49, 1685–1689 (1994).
Kantelhardt, J. W. Fractal and multifractal time series. Encyclopedia of Complexity and Systems Science. (Springer, 2009).
Garcia, D., Garas, A. & Schweitzer, F. Positive words carry less information than negative words. arXiv,1110.4123 (2011).
Tronick, E. Z. Emotions and emotional communication in infants. Am. Psychol. 44, 112–9 (1989).
Holbrook, M. B. & Batra, R. Assessing Mediators to of the Role of Emotions Responses as Consumer Advertising. J. Cons. Res 14, 404–420 (1987).
Article Google Scholar
Izard, C. E. The Many Meanings/Aspects of Emotion: Definitions, Functions, Activation and Regulation. Emotion Rev. 2, 363–370 (2010).
Mitrović, M. & Tadić, B. Bloggers behavior and emergent communities in Blog space. Eur. Phys. J. B 73, 293–301 (2009).
Chmiel, A. et al. Negative emotions boost users activity at BBC Forum. Physica A 390, 2936 (2011).
Rime, B. Emotion Elicits the Social Sharing of Emotion: Theory and Empirical Review. Emotion Review 1, 60–85 (2009).
Schweitzer, F. & Garcia, D. An agent-based model of collective emotions in online communities. Eur. Phys. J. B 77, 533–545 (2010).
Kappas, A., Tsankova, E., Theunis, M. & Küster, D. CyberEmotions: Subjective and physiological responses elicited by contributing to online discussion forums. Poster presented at the 51st Annual Meeting of the Society for Psychophysiological Research, Boston, Massachusetts (2011).
Küster, D., Tsankova, E., Theunis, M. & Kappas, A. Measuring CyberEmotions: How do bodily responses relate to the digital world? Poster presented at the 7th Conference of the Media Psychology Division of the Deutsche Gesellschaft fuer Psychologie, Bremen, Germany (2011).
Schweitzer, F. Brownian Agents and Active Particles. Collective Dynamics in the Natural and Social Sciences, Springer Series in Synergetics. (Springer, Berlin), 1st editio edition, p. 420 (2003).
Garcia, D. & Schweitzer, F. Emotions in product reviews – Empirics and models ed. Randy Bilof. (IEEE Computer Society, Boston, Massachusetts), pp. 483–488 (2011).
Russell, J. A. A circumplex model of affect. J. Pers. Soc. Psychol. 39, 1161–1178 (1980).
Kuppens, P., Oravecz, Z. & Tuerlinckx, F. Feelings change: Accounting for individual differences in the temporal dynamics of affect. J. Pers. Soc. Psychol. 99, 1042–1060 (2010).
Preston, S. D. & de Waal, F. B. M. Empathy: Its ultimate and proximate bases. Behav. Brain Sci. 25, 1–20; discussion 20–71 (2002).
Christophe, V. & Rime, B. Exposure to the social sharing of emotion: Emotional impact, listener responses and secondary social sharing. Eur. J. Soc. Psychol. 27, 37–54 (1997).
Thelwall, M., Buckley, K., Paltoglou, G., Cai, D. & Kappas, A. Sentiment strength detection in short informal text. J. Am. Soc. Inf. Sci. Technol. 61, 2544–2558 (2010).
Thelwall, M., Buckley, K. & Paltoglou, G. Sentiment strength detection for the social web. J. Am. Soc. Inf. Sci. Technol. 63, 163–173 (2012).
Kucuktunc, O., Cambazoglu, B. B., Weber, I. & Ferhatosmanoglu, H. A Large-Scale Sentiment Analysis for Yahoo ! Answers. Proc. of the 5th ACM Int. Conf. on Web Search and Data Mining (2012).
Paltoglou, G., Gobron, S., Skowron, M., Thelwall, M. & Thalmann, D. Sentiment analysis of informal textual communication in cyberspace. (Citeseer), pp. 13–23 (2010).
Thelwall, M., Buckley, K. & Paltoglou, G. Sentiment in Twitter events. J. Am. Soc. Inf. Sci. Technol. 62, 406–418 (2011).
Hurst, H. E. Long-term storage capacity of reservoirs. Am. Soc. Civ. Eng. 116, 770–808 (1951).
Google Scholar
Download references
Acknowledgements
This research has received funding from the European Community's Seventh Framework Programme FP7-ICT-2008-3 under grant agreement no 231323 (CYBEREMOTIONS).
Author information
Authors and affiliations.
Chair of Systems Design, ETH Zurich, Kreuzplatz 5, 8032, Zurich, Switzerland
Antonios Garas, David Garcia & Frank Schweitzer
Austrian Research Institute for Artificial Intelligence, Freyung 6/6, 1010, Vienna, Austria
Marcin Skowron
You can also search for this author in PubMed Google Scholar
Contributions
A.G., D.G. and F.S. designed the research, performed the research, analysed the data and wrote the manuscript. M.S. collected the data and analysed the data.
Ethics declarations
Competing interests.
The authors declare no competing financial interests.
Electronic supplementary material
Supplementary Information
Rights and permissions
This work is licensed under a Creative Commons Attribution-NonCommercial-ShareALike 3.0 Unported License. To view a copy of this license, visit http://creativecommons.org/licenses/by-nc-sa/3.0/
Reprints and permissions
About this article
Cite this article.
Garas, A., Garcia, D., Skowron, M. et al. Emotional persistence in online chatting communities. Sci Rep 2 , 402 (2012). https://doi.org/10.1038/srep00402
Download citation
Received : 21 February 2012
Accepted : 12 April 2012
Published : 10 May 2012
DOI : https://doi.org/10.1038/srep00402
Share this article
Anyone you share the following link with will be able to read this content:
Sorry, a shareable link is not currently available for this article.
Provided by the Springer Nature SharedIt content-sharing initiative
This article is cited by
Classification of endogenous and exogenous bursts in collective emotions based on weibo comments during covid-19.
- Misako Takayasu
Scientific Reports (2022)
Language, demographics, emotions, and the structure of online social networks
- Kristina Lerman
- Luciano G. Marin
- David Garcia
Journal of Computational Social Science (2018)
Generative models of online discussion threads: state of the art and research challenges
- Pablo Aragón
- Vicenç Gómez
- Andreas Kaltenbrunner
Journal of Internet Services and Applications (2017)
By submitting a comment you agree to abide by our Terms and Community Guidelines . If you find something abusive or that does not comply with our terms or guidelines please flag it as inappropriate.
Quick links
- Explore articles by subject
- Guide to authors
- Editorial policies
Sign up for the Nature Briefing newsletter — what matters in science, free to your inbox daily.

Does learning happen? A mixed study of online chat data as an indicator of student participation in an online English course
- Published: 26 February 2022
- Volume 27 , pages 7973–7992, ( 2022 )
Cite this article
- Qiang Huang ORCID: orcid.org/0000-0001-7004-3952 1
3085 Accesses
7 Citations
Explore all metrics
Student participation, as a significant indicator of class learning, has been investigated from various perspectives. The present research seeks to explore student participation by drawing on text data from the chat box of an online learning platform. The two main research questions concern the main types of student participation indicated by the online chat data as well as how extensively and frequently students had participated online in class. The written text messages of 84 university students in the chat box were recorded in an online English course for three months in consecutives. The findings revealed that students’ online chat data generally fell into five major types: students’ responses of factual information (62.77%) social interaction (15.74%), phatic communication (9.95%), tech-related messages (7.5%) and class schedule (4.5%). With 89% of participation concerning meaningful interactions and 11% of participation dealing with simple clarification of tech problems and class schedules, the findings suggest a highly active and meaningful online in-class participation. In addition, further descriptive statistics depicted the level of participation in terms of its frequency and breadth. Results showed that the active and meaningful online participation had been persistent over three months with an average of 74.52% regular participating students and average 410 chat messages sent one day. Implications were discussed in relation to the features of student participation.
Similar content being viewed by others
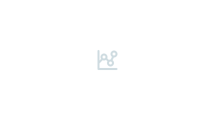
From novice to navigator: Students’ academic help-seeking behaviour, readiness, and perceived usefulness of ChatGPT in learning
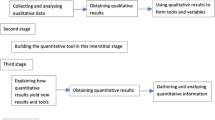
The effectiveness of using chatbot-based environment on learning process, students’ performances and perceptions: A mixed exploratory study
Knowledge-based chatbots: a scale measuring students’ learning experiences in massive open online courses, explore related subjects.
- Artificial Intelligence
- Digital Education and Educational Technology
Avoid common mistakes on your manuscript.
1 Introduction
Student participation, as one of the many variables depicting classroom learning, is believed to be important for both successful learning and effective teaching. Researchers have examined and measured student participation in various ways across different instructional contexts ranging from traditional physical classrooms to online learning modes such as MOOCs, blended learning and online courses (Araka et al, 2020 ; Dewan et al, 2019 ; Khalil & Ebner, 2017 ). Student participation, on the one hand, has been taken as one of the constituents of a broader encompassing concept of student engagement (Dixson, 2015 ; Shi & Tan, 2020 ). These two constructs overlap with each other and sometimes they are used interchangeably (Frymier & Houser, 2016 ). On the other hand, student participation has also been narrowed down to a separate and more specific construct such as in-class online participation in web-based medium (Dewan et al, 2019 ; Rocca, 2010 ). Active participation in class might bring life to the classroom and facilitate lively classroom climate (Rocca, 2010 ). When students actively participate in learning, they tend to learn more deeply, retain knowledge longer, have stronger communication skills, engage in greater critical thinking, and persist better in college especially in online learning experiences (Bigatel & Malizia, 2018 ). Thus, student participation has been an important area that deserves research attention. With the wide disparity of research variables, data types and survey methods in the area, very few studies have looked into students’ online chat data of online courses. Hence, the main purpose of the present study is to take the online chat data of students as an important indicator of student participation to explore and examine online in-class learning.
2 Literature review
Researchers have attempted to define, describe and measure student participation in various ways, which makes up the main challenges in the field. For example, Burchfield and Sappington ( 1999 ) defined student participation as “the number of unsolicited responses volunteered”, which could take up a number of different forms such as students’ questions and comments. Dancer and Kamvounias ( 2005 ) thought that student participation was an active engagement process that could be sorted into five categories: preparation, contribution to discussion, group skills, communication skills and attendance. Rocca ( 2010 ) provided an operational definition that in-class student participation consists of asking questions, raising one’s hand, and making comments. Researchers hold that the meaning of participation may vary depending on the context in which it occurs (Heaslip et al., 2013 ) and it could also differ from teachers’ ideas to students’ understandings. Teachers seem to have more limited perceptions of student participation, which encompasses students’ speaking in class, answering questions, making comments and joining in discussions (Vandrick, 2000 ) or involves “a form of active learning in which students publicly discuss the course material (Heyman & Sailors, 2011 ). In a typical English as foreign language (EFL) context, researchers considered student participation to be playing an active role in all in-class activities ranging from brainstorming, games, quizzes, surveys, group discussions, role plays, simulations, collaborative tasks, presentations, exchanging information (Crosthwaite et al., 2015 ). The present study tends to agree to this interpretation of student participation as students are encouraged to attend extensive learning activities in class rather than being constrained to only asking-answering questions, speaking and discussing.
This holds especially true when it comes to the particular setting of online courses. Researchers state that student participation has a significant impact on student persistence and retention that play an essential role in online environment. With prolific research in the area, few explanations of student participation in the online environment have been provided. This turns out to be one of the main challenges for researchers in the field. Some researchers viewed online participation from the lens of traditional class participation and highlighted asking questions, responding to questions or joining small group discussion (Handelsman et al, 2005 ). Hrastinski ( 2008 ) defined online student participation as “a process of learning by taking part and maintaining relations with others”. This understanding is more relationship-based and emphasizes the significance of connecting with others as the foundation of online participation. Since online learning develops out of traditional face-to-face instructions in physical classrooms (Huang, 2019 ), the researcher holds that online student participation features both traditional class participation and online participation and should thus involve both domains.
Therefore, the present study regards online student participation as “learners attend to learning that might persist in numerous ways and through various media in the online environment”. It may incorporate conventional class participation such as asking & replying questions, making comments, discussions, role-plays, dialogues, presentations that are both typical in physical classroom settings and also conducted online in web-based settings (Frymier & Houser, 2016 ; Hrastinski, 2008 ; Handelsman et al, 2005 ; Tsai et al, 2021 ). Additionally, it also comprises online-specific participation like page views, click-through rate, log data, trace data, online text messages, online posts, winning digital badges and applying student response system, to name just a few. (Cheng & Lei, 2020 ; Chou & He, 2016 ; Heaslip et al, 2013 ).
Once the main construct of student participation is operationally defined, researchers are able to describe and measure student participation in various ways. As a result, these constituent facets have been taken as the major indicators of student participation and make the focal research goals in the literature. A multitude of empirical studies have looked into the indicators in an attempt to disclose the nature and impact of student participation. Researchers have so far examined and measured a wide range of data types in relation to multiple variables by applying different research methods. Table 1 below reviews some latest studies in the area.
Table 1 shows that researchers have taken diverse data types as the indicators to measure and evaluate student participation. These data types range from more web-specific ones like log data, trace data, hits on courses, page views, online posts and comments to more conventional ones like self-report surveys, interviews, field notes and audio/video transcripts of class observations etc. Among them, studies that have examined students’ real-time chat data in the chat box of online learning medium are scant. In fact, real-time chat data from the chat box of online learning medium is able to capture the dynamic nature of learner participation and might reflect the real time conditions of learning and deliver the up-to-the-minute information (Kim et al., 2021 ). In online courses, students tend to spontaneously type in all kinds of text messages in the chat box on the online learning mediums such as ZOOM Meeting or Tencent Meeting. The chat box is an important and essential medium for instructor-learner and learner-learner communication and interaction in online courses. It entails informal and synchronous text messages complementary to video chat and audio chat on the online learning medium. So far studies taking chat data from the chat box of online learning medium are rather scarce. The present study holds that such spontaneous and real-time text messages record the authentic participation in online courses and might indicate in a way the real learning conditions in web-based settings. As a result, the online chat data from the chat box of the online learning medium was taken as the primary data source of the present study and acts as an indicator different from previous literature to examine student in-class participation online in this research.
As to the research methods, Table 1 shows that quantitative, qualitative and mixed research methods are all employed to explore student participation. Depending on different research goals, qualitative methods are utilized to disclose factors impacting student participation or its benefits (Ozkara & Cakir, 2018 ; Sedova, 2017 ; Theriault, 2019 ) while quantitative methods serve to unpack the possible patterns or trends of student participation (Cheng & Lei, 2020 ; Stamm et al, 2017 ) or reveal its relationships with other variables (Crosthwaite et al, 2015 ; Frymier & Houser, 2016 ).
Regarding sample sizes, quantitative studies generally investigate larger sample size and Table 1 shows that it may surge up to over 7000 subjects. In contrast, both qualitative and mixed-method research commonly take much smaller sample sizes and Table 1 shows that they range from 8 to 30 and from 56 to 120 respectively. By investigating 84 university students and collecting their online chat data in the online English course, the present study intends to employ mixed research methods of both qualitative and quantitative means to depict more specific features of student participation in the online English course.
In sum, the present study attempts to take online chat data as an indicator and explore what characterizes students’ participation in the online English course by addressing the following two research questions:
What are the main types of student online participation indicated by the online chat data?
What is the student online participation level? i.e., how frequently and extensively have the students participated in the online English course by engaging in the online chat?
3.1 Study context
The English course in question is a compulsory EGP (English for general purpose) course for English majors of EFL learners (English as foreign language) at a university. The course aims to develop English language knowledge and communication competence of learners. It covers two academic years of four semesters in the first two academic years at university. For each class of students, there are 2 class sessions a day, occurring twice a week for 16 weeks in one semester. Each session is 40-min in length. Due to the COVID 19 pandemic, the English course was changed from traditional face-to-face instruction in physical classrooms to online course during the semester from March to July in 2020. During these four months, four units were instructed ranging from “Health & Medicine”, “Food & Drink”, “Advertisement” to “Sports”.
The online learning platform where the English course was instructed was Tencent Meeting, an online audio and video conferencing platform functioning very similar to Zoom Meeting. Tencent Meeting allows users to set up virtual video and audio meeting, webinars, live chats, screen-sharing, and other collaborative capabilities. On top of the audio/video conferencing and live chats, the chat box of the platform offers one more option other than just speaking to microphones. Teachers and students can thus communicate with each other by writing and sending text messages in the chat box in class. The chat box is used mainly for presenting informal but spontaneous and natural utterances by students especially when they were not called on for any particular questions, exercises or tasks in class. It is these short text messages, free chats and casual utterances online that make up the primary data source of the present study.
3.2 Participants
Participants of this study were three classes of 84 English majors at the university who had learnt English as foreign language (EFL) and had taken the same English course. They were of the same English majors and of the same academic year at university. Thus, the three classes used the same course books and had the same course schedule as well as the same course evaluation of the English course. Their online English courses were instructed by one teacher on the same online learning platform of Tencent Meeting. The three classes were also similar in class sizes of 29, 27 and 28 students respectively. There were only 6 male students but 78 female students. They were of similar ages of around 20 years old.
3.3 Data collection
The present research has taken the written text messages in the chat box of the online learning platform as the primary data to depict the features of student online participation in the English course. To collect the online chat data, the researcher had copied the written text messages that the three classes of students sent in the chat box of the online learning platform each time when they had finished their online lessons. The chat data were gathered for 12 sessions every week from April to July. Finally, a total of 145 sessions of valid chat data had been collected during three consecutive months in the semester. The total text data set consisted of 58,191 words.
4 Data analyses
4.1 coding the online chat data, 4.1.1 coding processes.
To code the 58,191-word online chat data, the researcher and one research assistant first had a briefing of the basic principles of coding. Both coders agreed that one line of written utterance sent in the chat box counted as one complete message. This could be either a word, a phrase, a sentence fragment or a complete sentence in the chat box. Then the two coders coded the chat data respectively. The coding processes included the following steps: summarizing the main features depending on the representative phrases and key words from the chat data; combining similar codes; clustering them into themes; comparing, discussing, modifying, merging and finally reaching consensus.
4.1.2 Validity
To ensure the validity of the survey, the present study had taken several strategies to realize principles specified by researchers (Ozkara & Cakir, 2018 ): collecting long-term information in the natural environment of the online English course, using direct quotations from chat data, gathering detailed and complete information from the chat data, making meanings and explanations based on these data etc. Additionally, the nature of online chat data also added to the validity of the survey in that the on-site recording of the written text messages at the end of each class was unobtrusive and natural, without interrupting either class instruction, participants or the instructor.
4.1.3 Reliability
Regarding the reliability between the two coders, researchers hold that the percentage of reliability between coders must be 70% or more when forming a consensus (Ozkara & Cakir, 2018 ). In the present study, the two coders had first reached a 95.562% consensus and later a full consensus on coding the data after three rounds of discussions. To ensure the reliability, the researcher and the assistant first had a briefing of coding processes, set off coding respectively, compared coding results, discussed the differences together, made modifications and re-merged, then ultimately reached a consensus of a final version of the coded data. All the briefing, discussions and conferencing of the two coders took up to 10 h in total.
4.1.4 Descriptive statistical analyses
Upon finishing data coding, the researcher continued to summarize the data with simple descriptive statistics to reveal any possible trends and patterns. A series of data were calculated either by the entire sample, by each theme group, by each student or by each class session as well as their percentages against the entire sample, their frequencies and their fluctuations over time.
5.1 Findings of coding the online chat data
In this section, findings of the present research will be presented according to the two research questions. To answer research question 1 of “What are the main types of online participation indicated by the online chat data?”, coding the online chat data has generated a total of five major themes, namely, phatic communication, students’ responses of factual information, social interaction, tech-related messages and class schedule. Table 2 lists the five major theme groups as well as their sample chat data. In particular, phatic communication encompassed 939 messages of students’ greetings, farewells, and gratitude to the teacher in the online English course. Typical examples were “ Good morning”, “Good bye”, “Thank you” plus their variants like “morning”, “thanks” or “bye ” etc. These messages all served to establish a mood of sociability and indicated a normal teacher-student relationship in class rather than exchanging concrete information or ideas. Thus, similar messages were coded into the theme of “phatic communication”.
On top of phatic communication, students also attended to the online English class in other ways. For example, replying the teacher’s questions either after being called on or without being called on, responding to the instruction spontaneously, uttering their understandings of the instruction contents and exchanging information briefly with each other in the chat box. All these messages were written and sent in the chat box by students as they kept to the flow of the online instruction in class. They all referred to factual and matter-of-fact knowledge and information that was either being instructed by the teacher or related to the instruction content. Messages in this category were coded as “students’ responses of factual information” and they numbered 5921 in total. Table 2 shows some examples such as “d isease concept, malaria, soup dumplings, carbonated beverage, ad copy, demographic, Petek Phillippe, goal keepers, forwards, shin guard ”.
The second larger theme group was labelled “social interaction” with a total of 1486 messages. In this category, messages revealing students’ feelings, attitudes and opinions towards either the instruction contents, their peers, the teacher or even the course were assembled together. Among them, most of the messages expressed students’ feelings, attitudes and opinions towards the instruction contents. For instance, “ terrible; I thought such pills might taste terrible”; “i love chicken; hah-hah-hah don’t like fish”; “Messi is simply amazing ”. Some messages also revealed student–student interactions such as their emotions and comments on their peers. Examples included.
S1: “ Jasminum nudiflorum” ( students’ responses of factual information )
S2: “Hahahahahah too difficult to remember ” (social interaction)
In this case, one student prompted the words “ Jasminum nudiflorum” to respond to the instructional content and then another student followed immediately by making comment on the difficulty of the vocabulary prompted. Then other messages manifested student–teacher interaction as well where students might express either their appreciations, their affection or their thanks to the teacher. For instance, “ Professor, you have spoken very well the local dialect indeed!”, “Professor, how kind you are!” “Love you soooooo much! ”.
Then the fourth theme group was “Tech-related messages”. Altogether 665 messages concerning technological problems during the online instruction were gathered in this group. Some were about the failed screen-sharing with the students on the online learning platform. Others were related with learning materials of audios or videos that often got stuck online when they were synchronously shared on the learning platform. Or someone’s microphone or camera did not work at the moment. The most exceptional case was found with one student who apologized for being unable to speak on microphone as some guests were visiting his family at that time. Even though it was a rare case, the incident featured the special online learning conditions when learning venue was blended into life venue during COVID-19 pandemic.
Finally, the fifth theme group of “class schedule” collected 423 messages regarding the schedule of class instruction, assignment as well as course examinations etc. Typical examples include “ Good, two weeks (for preparing the assignment) are better”;” where shall we hand in our assignment?”, “Part 3 isn’t finished yet ”.
Overall, a total of 9433 messages in 58,191 words of the online chat data were coded into 5 major themes. These themes, namely, phatic communication, students’ responses of factual information, social interaction, tech-related messages as well as class schedule, indicated both the main contents of student participation and the major ways how they participated through the chat box on the online learning platform.
5.2 Results of descriptive statistical analyses of the online chat data
To answer research question 2 about students’ participation level online, descriptive statistical analyses were conducted to summarize the online chat data after coding, To start with, Fig. 1 below shows the percentages of the five theme groups of online chat data. Among them, students’ responses of factual information accounted for 62.77% of the entire data and made up the majority of student participation in the online English course. Social interaction occupied 15.74% and was the second largest group. The percentages of the rest three groups, i.e., phatic communication, tech-related massages and class schedule were 9.95%, 7.05% and 4.5% respectively .
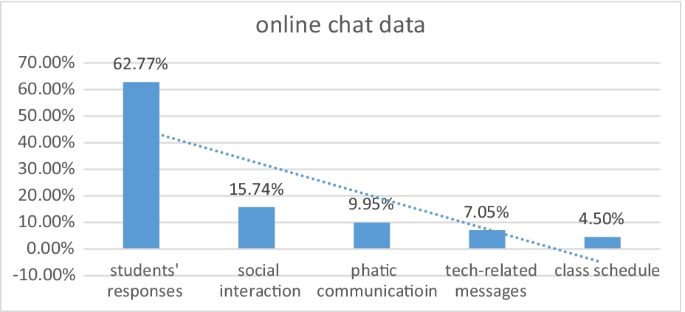
The percentages of the 5 main themes of the online chat data
Apart from the percentages of five theme groups, a longitudinal analysis of the statistics helps to unveil further features of student participation. In particular, Fig. 2 demonstrated the total numbers of messages students sent daily in the chat box over three months. The numbers of messages in the chart fluctuated approximately between 237 and 550 with one exception of 716 at the peak. The average number of messages sent one day was 410. After reviewing the instructor’s teaching plan for the contents of the chat data on that particular date, it turned out that the unit of “Food & Drink” was instructed on that day and a video featuring unusual ingredients for barbecued food was played in class. The surging numbers of messages indicated that students were highly active and lively in that session of online class.
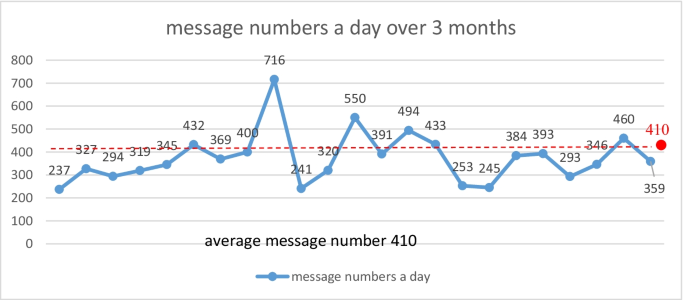
The total numbers of daily messages sent over three months
On the whole, the average daily messages sent in the chat box over three months numbered 410, indicating that students had been actively involved in class learning and had maintained highly frequent participation throughout the semester.
In similar vein, Fig. 3 displayed the percentages of students who sent messages in the chat box over time. The lowest percentage was 65.49% and the highest percentage was 92.85%. With an average of 74.52% students sending text messages in the chat box a day, data in Fig. 3 suggested that the majority of students (74.52%) had regularly participated in the online English class throughout the three months.
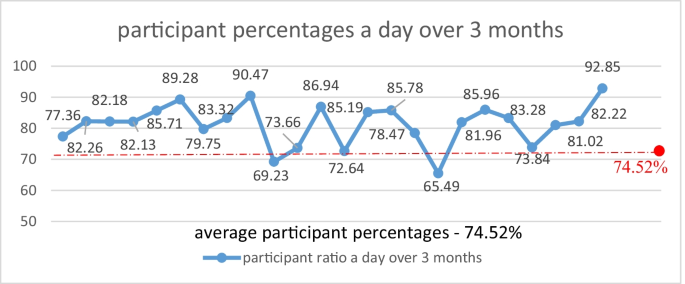
The daily percentages of participating students over 3 months
Figure 2 indicated the frequency of student participation which was measured by the number of messages sent in the chat box. In the meantime, Fig. 3 demonstrated the breadth of student participation that was measured by the percentages of participating students against the sample of 84 participants. The two figures combined together suggested the level of students’ participation online, i.e., how frequently students had participated in the online English class by sending text messages to the online chat box and how extensively students had participated in the class. It helped to depict a more comprehensive picture of student participation in the online English course.
To sum up the results of descriptive statistical analyses, around 89% of the student participation indicated by the online chat data in the online English course were concerned about meaningful communications and interactions. It involved students’ responses of factual information and social interaction and phatic communication. Furthermore, only 11% of student participation dealt with simple clarifications of technical problems and class schedules. Thus, the findings suggest a highly active and also meaningful student participation in the online English course. In addition, with an average 74.52% regular participating students and an average 410 chat messages sent one day, the present study showed that student participation in the online English course had been active, extensive and persistent over three months.
6 Discussion
In this section, the main findings are discussed in relation to the previous literature. First of all, the present study found that student participation in the online English course was largely characterized by five major types. The most salient group was students’ responses of factual information and it indicated the most common way in which students had participated online in the present study. While learning online, participants often sent in the chat box their comprehensions and interpretations of the learning contents such as new words, difficult phrases, complicated sentences as well as other more complex discourse analyses or world knowledge that the teacher was instructing. Messages in this group were largely factual in nature, short in length and closely related to the contents that participants were learning online at the moment. In other words, one major way for students to participate in the online English class and interact with the instruction is to articulate in the chat box their understandings of the subject contents and keep to the flow of cognitive learning in class. In this case, cognitive learning was the major part of the online participation indicated by this group of online chat data.
This finding is quite distinct from previous studies where online dialogue and online discussion are regarded as the most common form of online participation (Hew & Cheung, 2010 ; Hrastinski, 2008 ; Shi & Tan, 2020 ; Tsai et al., 2021 ). The present study has found that cognitive learning of factual information and world knowledge might also play a significant role in engaging students in active participation and class learning. This is supported by students’ responses of factual information that consisted of 5921 messages and took up 62.77% of the total online chat data. This high percentage suggested that students were lively engaged in the cognitive processes of learning in the online English class. Researchers have found that online discussion might not be sufficient to increase student success in online learning and student online participation could also be significantly influenced by content areas (Chou & He, 2016 ). Hence, one possible reason for the active online participation indicated by the high percentages of students’ response might be attributed to students’ interests in the learning contents that could improve their contribution to class. (Rocca, 2010 ). Learning contents and activity design should be meaningful and authentic so as to capture students’ interests and maintain participation (Cheng & Lei, 2020 ; Hew & Cheung, 2010 ). Students’ interests in learning the instruction contents constitute one of the intrinsic factors that are believed to work better in engaging students than extrinsic factors such as offering badges, grades and certificates (Crosthwaite et al., 2015 ; Khalil & Ebner, 2017 ).
Another manifestation for this is the highest number of 716 messages students had sent in the chat box during one session. In this lesson, students learnt the unit of “Food & Drink” which was quite popular with learners. The messages sent in the chat box showed that this lesson began with learning unusual ingredients of food from a documentary featuring barbecue food. A 15-min video clip was shown to students online in class to bring in the topic, familiarize them with the background knowledge and open up discussion of the topic. On watching the video clip and learning unusual food ingredients, students had sent in the chat box the highest number of 716 messages per day during the three months. On the one hand, a large number of messages showed students’ understanding of the topic and their background knowledge, which exemplified the first theme group of students’ response of factual information. Some examples were presented here:
ingredients of food; seasoning; the flavor; food names; recipes; menus; goose liver; fatty liver; snail; French food; insects; bamboo worm; cicada; bird nest; tonic; pork kidney; tonic soup for kidney; barbecue skewers; this whole episode talks about weird food; kangaroo meat; …
On the other hand, numerous messages revealed students’ feelings of and attitudes towards the topic and the video, which exemplified the second theme group of the online chat data, i.e., social interaction. Examples included.
look yummy after cooked; fantastic documentary; The caption (of the documentary) is amazing; best for those who plan to lose weight; some are acceptable others not; (kangaroo) graze too much (in Australia); can’t take insects; hah-hah-hah-hah, gonna bring up my breakfast; professor, please don’t ask any question about it, I simply can’t watch this part; hah-hah-hah-hah-hah-hah-hah-hah;
Researchers hold that the online environment enables boundless access to extensive learning resources and thus makes it possible to relate learning more to the real world. (Cole et al., 2019 ). This is in line with the case of 716-message in which the real-world authentic instructional contents and learning activities helped to facilitate participation by moving students beyond simple sharing and comparing information to greater exploration of ideas, attitudes and feelings (Khalil & Ebner, 2017 ).
Those messages in the chat box that expressed students’ feelings, attitudes and opinions have formed the second theme group of chat data, i.e., social interaction . The fact that social interaction took up 15.74% of the total online chat data and made up the second main theme group indicated that on top of cognitive learning, students in this study were also quite active in exchanging their attitudes and expressing their feelings in the chat box while learning online.
Corroborated with previous research, the present study also found that social interaction consists of three dimensions of learner-content, learner-teacher and learner-learner interaction (Moore, 1989 ). Students were found to respond to instruction content, to the instructor and to each other. They also made comment on peers in triadic interactions or share personal experience and information in classroom participation (Sedova, 2017 ; Theriault, 2019 ). On the one hand, researchers think that well-designed online activities contribute to enhanced online interaction and students’ participation is closely related to course design and course activities (Chou & He, 2016 ; Tsai et al., 2021 ). This is well manifested by the case of 716-message in the present study where the video clip of unusual food had greatly triggered students’ interests and motivated them to interact lively online. On the other hand, online interaction can in turn foster knowledge construction and enhance online participation through engaging with others and getting motivated to post and respond to messages. Although researchers don’t think that extensive faculty-student or student–student interaction would always translate into student success (Crosthwaite et al., 2015 ), connecting with others online through interaction constitutes the foundation of online participation (Chou & He, 2016 ). And lack of interaction with peers and limited social interaction have been identified as the main difficulties and challenges of online learning (Sun, 2014 ). In fact, previous literature has found that conveying feelings and emotions, either positive or negative, could lead to higher participation. For example, engaging and productive comments can evoke joyful feelings which in turn can drive further participation in ongoing communication, conversation, discussion or other forms of learning activities that follow (Kim et al., 2021 ).
The third theme group of the online chat data was phatic communication. With 939 chat messages, phatic communication accounted for 9.95% of the total chat data. Small and trivial as they appeared, phatic communication revealed a distinct kind of in-class participation from other types commonly found in the literature (Araka et al., 2020 ; Dewan et al., 2019 ). In fact, student participation is seldom measured by way of phatic communication and it is thus rarely investigated by researchers (Rocca, 2010 ). Although it is content-free and does not exchange substantial information or ideas, phatic communication is regarded as an important social lubricant to share feelings or establish a mood of sociability in classroom settings. Findings of the present study showed that students had constantly made friendly greetings and farewells to the instructor each class. It implied a positive online class climate where the instructor and students were willing to communicate and collaborate in class (Cole et al., 2019 ; Frishy et al., 2014 ). The present study holds that, combined with social interaction, phatic communication also manifests in a way the class relationship that is safe and comfortable and that is supposed to bring forth more active participation and increase classroom engagement (Tang et al., 2020 ).
The last two theme groups are tech-related messages and class schedule, which occupied 7.05% and 4.5% of the total online chat data. Resembling phatic communication, these two categories, too, reveal a different way for students to participate in class for disparate purposes. In terms of tech-related messages, findings of the present research disclosed that most of them were students’ on-the-spot reports or reminders to the instructor about the technical problems of online learning platform. This is also a common unfavorable feature of online learning that often influences online participation (Chou & He, 2016 ; Ozkara & Cakir, 2018 ). With the small proportion of messages reporting technological problems, the online learning platform employed in the present study had regularly functioned well and had technologically contributed to the overall success of the online English course. Furthermore, the reminders of technical problems also revealed that students were actually following and participating in the ongoing instruction. In similar vein, the group of class schedule also revealed students’ concerns about class timetable and course arrangement. It only accounted for a very small proportion (4.5%) of student participation. Contrary to the present research, previous studies found that in some classes where students were reluctant to participate, 73% of student participation were in reference to simple clarifications of class procedures rather than making meaningful communications or engaging interactions (Rocca, 2010 ). Actually, both domains of tech-related messages and class schedule have been largely left out in the research area of student participation (Araka et al., 2020 ; Dewan et al., 2019 ).
In addition to the five theme groups of online chat data that indicated the major types of students’ online participation, the present study also found high level of online participation in the online English course. Previous literature shows that online participation level could be investigated and gauged in various ways. The most common way to measure the online participation level is by quantity of messages and quality of messages (Hrastinski, 2008 ). In terms of message quality, the present study has found that 89% of the student participation in the online English course centered around meaningful communications and interactions that comprised students’ responses of factual information, social interaction and phatic communication. This great proportion of student participation mainly focused on exchanging factual knowledge and concrete information of the instruction contents and sharing their feelings, attitudes and opinions either among learners or between learners and the instructor. In other words, the majority of online participation indicated by the online chat data was devoted either to meaningful cognitive engagement or to affective interactions in the present study. It suggested high quality of online participation of the online English course that is in sharp contrast to previous studies. For example, Rubio et al ( 2018 ) found that most of the online participation was related to tracing log data, checking posts & comments and clarifying course schedule. In some studies, 73% of students’ participation were in reference to class procedures or clarification of schedule (Rocca, 2010 ). In fact, students’ online participation can come in multiple forms and participating online class through sending messages to the online chat box is one of them. According to Bigatel and Malizia ( 2018 ), indicators of engaged learning online include three dimensions: cognitive engagement, social-emotional engagement and behavioral engagement. It seems that student’s participation through online chat in the online chat box have cognitively, social-emotionally and behaviorally engaged students. It is hoped that such quality online participation will facilitate language learning, knowledge construction, and critical thinking in class.
Regarding the message quantity, the present study reported an average 410 chat messages sent one day along time. In addition, an average of 74.52% students had regularly participated in class by sending meaningful messages to the online chat box. Both the message quantity and the numbers of regular participants contributed to an active, extensive and persistent online participation over time. Nonetheless, many previous studies showed that very often only a handful of students participated orally while the majority mostly listen passively (Theriault, 2019 ). Besides, about 90% of interactions and participation were made by a small number of students but only around 30% were regular participants. In some extreme cases, only 3 questions were recorded per class hour (Rocca, 2010 ). Such limited participation by limited number of participants makes a notable contrast to the active, extensive and persistent participation by much larger percentages of participants in the present study.
Although the importance of student participation is well-recognized, many students do not participate in online courses for multiple reasons. One of the common reasons is the widely-existing participation apprehension that seems to hold students from necessary participation (Rocca, 2010 ). Participation apprehension could be mediated through various strategies (Frishy et al., 2014 ). The high online participation level in the present study suggested that the majority of students had actively and persistently participated in the online chat box and the online chat box seemed to be a safe and comfortable venue for this higher level of online participation.
According to some researchers (Ozkara & Cakir, 2018 ), students tended to prefer online chat to reduce lack of interactivity in the online environment in that it is regarded as less stressful or less face-threatening but more reassuring and face-safer (Frishy et al., 2014 ). In other words, online chat box created a safer and more comfortable online class climate for interaction and communication that help to decrease isolation, facilitate in-class participation and ease participation apprehension (Cole et al., 2019 ). Furthermore, by way of sending short text messages in the chat box, students managed to actively learn the instruction contents, keep to the instruction pace of cognitive learning, stay focused and thus persist better in online class over time (Chou & He, 2016 ).
In all, the five main groups of online chat data revealed different ways for students to participate in the online English course especially through the chat box of the online learning platform. Most of the chat data were free and spontaneous exchange of information, ideas or feelings rather than the intended replies after being called on by the teacher. Basically, they did not always follow the pattern of IRF script that consists of teacher’s initiations, students’ replies and teacher’s follow-up or feedback. Neither did they necessarily occur with particular groups of at least three students over a longer time span of more than 30 s, which featured online open discussion (Sedova, 2017 ). In a way, such text messages were often short utterances that were casual, on-the-spot and unrehearsed. In many cases, the short utterances were not directed at any particular individual or groups. They were more “free chats” among peers than formal speech or public speaking in class that often faces evaluation and judgement from the teacher or the peers (Frishy et al., 2014 ; Rocca, 2010 ).
7 Conclusion and implication
In conclusion, the present research has made a few modest contributions. For example, the researcher has provided a workable operational definition of online student participation that features both traditional class participation and online participation as online learning develops out of face-to-face instructions in physical classrooms (Huang, 2019 ). Consequently, the dimensions and the constituent facets of online student participation are thus extended and more myriad data types could be incorporated as the indicator to examine online student participation. The indicators of online student participation then range from more conventional ones of asking & replying questions and in-class participations to more online-specific participations such as page views, log data, online posts and chat data.
Among them, online chat data has been under-researched. By examining the online chat data, the present study has identified five main types of students’ online participation, i.e., students’ responses of factual information, social interaction, phatic communication, tech-related messages and class schedule in the online English class, which indicates the main contents of online participation. By summarizing the trends and patterns, the researcher has also found that student participation measured by the online chat data turned out to be active, extensive and persistent in class. With active and extensive participation in the online chat box, the findings unravel a third kind of digital participation on top of vocal participation and silent participation in class (Shi & Tan, 2020 ). In other words, students could be digitally “active and verbal” in online contexts but hesitate to speak up orally in class, which creates a unique phenomenon featuring online learning. This echoes the position of going beyond oral participation as well as identifying more indicators of student participation and more dimensions of student learning patterns (Shi & Tan, 2020 ).
Some implications can be drawn from the findings. For example, students might participate in online class in more ways than just online dialogues or discussions and online in-class participation could be measured by different means such as online chat data as online technology develops new learning platforms or instruction tools. Furthermore, researchers think that course-related online chat could help students to increase students’ willingness to participate and thus boost interactivity and reduce isolation while learning online (Heaslip et al., 2013 ). Online chat might relieve students’ worries about negative feedback, decrease pressure of public speaking in front of the whole class, free them from the teacher’s calling-on preferences and ease participation apprehension (Tang et al., 2020 ). The present study holds that the online chat box of the online learning platform might play a beneficial role in facilitating the interaction and participation in class as it offers a handy medium to involve students, make them focused and keep them to the instruction flow. One main advantage of the chat box is that it enables the unobtrusiveness of online interaction and participation without interrupting the regular learning activities and class instruction (Dewan et al., 2019 ). The online chat box might help to create an informal but safe and comfortable venue for students to interact and communicate more freely and spontaneously, which is pertain to the online environment but quite absent in traditional face-to-face instruction settings. Actually, such an encouraging and supportive climate is necessary for both successful learning and effective teaching in both virtual and physical classrooms (Durrington et al., 2006 ).
As to how to encourage students to use online chat box to interact more in class, researchers have found that participation tends to increase when it is overtly included as part of the grading criteria and when a participation score is allocated in class assessment (Crosthwaite et al., 2015 ). On top of similar external stimulus, the researcher holds that students might be more actively motivated to interact in the online chat box when learning contents and instructional pedagogy are more engaging. This is substantiated by the peak message number of 716 in the present study when students were overwhelmingly cheered up by the learning contents in class. The online chat box was then flooded by all exclamations, claims and messages. The combination of both internal and external stimuli will probably work better to spur students to resort to the online chat box for more significant interaction and better class learning.
8 Limitation and future study
Future research is discussed along with the limitations of the research. Due to the nature of the online chat box that is complementary and supplementary to the online learning platform, online chat data carries with itself some restrictions. It is less complete and comprehensive and contributes to only part of the entire learning context for the research. In other words, the inclusion of more diverse sources of data such as other classroom learning activities will help to reveal a more comprehensive picture of online student participation. Additionally, it is also necessary to determine causality and to probe into the complex and dynamic relationships between student participation and other variables like learning achievements, teaching performance and instruction effects especially over a longer period of time. Consequently, these limitations all point to the direction for future studies. For example, how does classroom environment contribute to the patterns of digital participation indicated by online chat data or other types of data, how do teaching behaviors and teacher-student relationship impact student digital participation in class, how do students’ individual characteristics affect participation patterns in online contexts, does students’ online participation show any gender differences and how are digital devices applied in class to influence students’ digital participation. Future studies, thus, will target at more diverse and comprehensive sources of data that can measure student participation and look into the longitudinal and dynamic relationships between student participation and other variables.
Araka, E., Maina, E., Gitonga, R., & Oboko, R. (2020). Research trends in measurement and intervention tools for self-regulated learning for e-learning environments – systematic review (2008–2018). Research and Practice in Technology Enhanced Learning, 15 (6), 1–21.
Google Scholar
Bigatel, P. M., & Malizia, S. E. (2018). Using the “indicators of engaged learning online” framework to evaluate online course quality. TechTrends, 62 , 58–70.
Article Google Scholar
Burchfield, C. M., & Sappington, J. (1999). Participation in classroom discussion. Teaching of Psychology, 26 , 290–291.
Cole, A. W., Lennon, L. & Weber, N. (2019). Student perceptions of online active learning practices and online learning climate predict online course engagement. Interactive Learning Environments , 1–15.
Cheng, J. & Lei, J. (2020). A description of students’ commenting behaviours in an online blogging activity. E-Learning and Digital Media , 1–17.
Chou, C. C., & He, S. (2016). The Effectiveness of digital badges on student online contributions. Journal of Educational Computing Research, 54 (8), 1092–1116.
Crosthwaite, P. R., Bailey, D. R., & Meeker, A. (2015). Assessing in-class participation for EFL: Considerations of effectiveness and fairness for different learning styles. Language Testing in Asia, 5 (9), 1–19.
Dancer, D., & Kamvounias, P. (2005). Student involvement in assessment: A project designed to assess class participation fairly and reliably. Assessment & Evaluation in Higher Education, 30 , 445–454. ES.
Dewan, M. A. A., Murshed, M., & Lin, F. (2019). Engagement detection in online learning: A review. Smart Learning Environments, 6 (1), 1–20.
Dixson, M. D. (2015). Measuring student engagement in the online course: The online student engagement scale (OSE). Online Learning, 19 (4), 1–13.
Durrington, V. A., Berryhill, A., & Swafford, J. (2006). Strategies for enhancing student interactivity in an online environment. College Teaching, 54 (1), 190–193.
Frishy, B. N., Berger, E., Burchett, M., Herovic, E., & Strawser, M. G. (2014). Participation apprehensive students: The influence of Face support and instructor-student rapport on classroom participation. Communication Education, 63 (2), 105–123.
Frymier, A. B., & Houser, M. L. (2016). The role of oral participation in student engagement. Communication Education, 65 (1), 83–104. https://doi.org/10.1080/03634523.2015.1066019
Handelsman, M. M., Briggs, W. L., Sullivan, N., & Towler, A. (2005). A measure of college student course engagement. The Journal of Educational Research, 98 (3), 184–191.
Heaslip, G., Donovan, P., & Cullen, J. G. (2013). Student response systems and learner engagement in large classes. Active Learning in Higher Education, 15 (1), 11–24.
Hew, K. F., & Cheung, W. S. (2010). Possible factors influencing Asian students’ degree of participation in peer-facilitated online discussion forums: A case study. Asia Pacific Journal of Education, 30 (1), 85–104.
Heyman, J. E., & Sailors, J. J. (2011). Peer assessment of class participation: Applying peer nomination to overcome rating inflation. Assessment & Evaluation in Higher Education, 36 (5), 605–618.
Huang, Q. (2019). Comparing teacher’s roles of f2f learning and online learning in a blended English course. Computer Assisted Language Learning, 32 (3), 190–209.
Hrastinski, S. (2008). What is online learner participation? A literature review. Computers and Education, 51 (4), 1755–1765.
Khalil, M., & Ebner, M. (2017). Clustering patterns of engagement in massive open online courses (MOOCs): The use of learning analytics to reveal student categories. Journal of Computing in Higher Education, 29 , 114–132.
Kim, M. K., Lee, I. H., & Kim, S. M. (2021). A longitudinal examination of temporal and iterative relationship among learner engagement dimensions during online discussion. Journal of Computers in Education, 8 (1), 63–86.
Latham, A., & Hill, N. S. (2013). Preference for anonymous classroom participation: Linking student characteristics and reactions to electronic response systems. Journal of Management Education, 38 (2), 192–215.
Liu, C., Yang, C., & Chao, P. (2019). A longitudinal analysis of student participation in a digital collaborative storytelling activity. Educational Technology Research and Development, 67 , 907–929.
Luo, Y., Pan, R., Choi, J. H., & Strobel, J. (2018). Effects of Chronotypes on students’ choice, participation, and performance in online learning. Journal of Educational Computing Research, 55 (8), 1069–1087.
Moore, M. (1989). Editorial: Three types of interaction. The American Journal of Distance Education, 3 (2), 1–5.
Ozkara, O. B., & Cakir, H. (2018). Participation in online courses from the students’ perspective. Interactive Learning Environments, 26 (7), 924–942. https://doi.org/10.1080/10494820.2017.1421562
Rocca, K. A. (2010). Student participation in the college classroom: An extended multidisciplinary literature review. Communication Education, 59 (2), 185–213. https://doi.org/10.1080/03634520903505936
Rubio, F., Thomas, M., & Li, Q. (2018). The role of teaching presence and student participation in Spanish blended courses. Computer-Assisted Language Learning, 31 (3), 226–250.
Sedova, K. (2017). Transforming teacher behaviour to increase student participation in classroom discourse. Teacher Development, 21 (2), 225–242. https://doi.org/10.1080/13664530.2016.1224775
Shi, M., & Tan, C. Y. (2020). Beyond oral participation: A typology of student engagement in classroom discussions. New Zealand Journal of Educational Studies, 55 , 247–265.
Stamm, E. J. P., Zafonte, M., & Palenque, S. M. (2017). The effects of instructor participation and class size on student participation in an online class discussion forum. British Journal of Educational Technology, 48 (6), 1250–1259.
Sun, S. Y. H. (2014). Learner perspectives on fully online language learning. Distance Education, 35 (1), 18–42.
Tang, X., Wang, Y., & Wong, D. (2020). Learning to be silent: Examining Chinese elementary students’ stories about why they do not speak in class. Language, Culture and Curriculum, 33 (4), 384–401.
Theriault, J. C. (2019). Exploring college students’ classroom participation: A case study of a developmental literacy classroom. Journal of College Reading and Learning, 49 (3), 206–222.
Tsai, C., Ku, H. & Campbell, A. (2021). Impacts of course activities on student perceptions of engagement and learning online. Distance Education , 1–20.
Vandrick, S. (2000) Language, culture, class, gender and class participation. Paper presented at TESOL Annual International Convention, Vancouver, Canada
Download references
Author information
Authors and affiliations.
School of English Education, Guangdong University of Foreign Studies, No. 2 North Baiyun Rd. Baiyun District, Guangzhou City, Guangdong Province, China
Qiang Huang
You can also search for this author in PubMed Google Scholar
Corresponding author
Correspondence to Qiang Huang .
Ethics declarations
Conflicts of interest, additional information, publisher's note.
Springer Nature remains neutral with regard to jurisdictional claims in published maps and institutional affiliations.

Rights and permissions
Reprints and permissions
About this article
Huang, Q. Does learning happen? A mixed study of online chat data as an indicator of student participation in an online English course. Educ Inf Technol 27 , 7973–7992 (2022). https://doi.org/10.1007/s10639-022-10963-3
Download citation
Received : 16 December 2021
Accepted : 14 February 2022
Published : 26 February 2022
Issue Date : July 2022
DOI : https://doi.org/10.1007/s10639-022-10963-3
Share this article
Anyone you share the following link with will be able to read this content:
Sorry, a shareable link is not currently available for this article.
Provided by the Springer Nature SharedIt content-sharing initiative
- Student participation
- Online chat data
- Online English course
- Find a journal
- Publish with us
- Track your research

An official website of the United States government
The .gov means it’s official. Federal government websites often end in .gov or .mil. Before sharing sensitive information, make sure you’re on a federal government site.
The site is secure. The https:// ensures that you are connecting to the official website and that any information you provide is encrypted and transmitted securely.
- Publications
- Account settings
- My Bibliography
- Collections
- Citation manager
Save citation to file
Email citation, add to collections.
- Create a new collection
- Add to an existing collection
Add to My Bibliography
Your saved search, create a file for external citation management software, your rss feed.
- Search in PubMed
- Search in NLM Catalog
- Add to Search
Online 'chatting' interviews: An acceptable method for qualitative data collection
Affiliations.
- 1 Faculty of Nursing, Chulalongkorn University, Bangkok, Thailand.
- 2 Belitung Raya Foundation, Manggar, East Belitung, Bangka Belitung, Indonesia.
- 3 The University of Texas at Tyler, School of Nursing, 3900 University Blvd., Tyler, TX 75799, USA.
- PMID: 37546501
- PMCID: PMC10401366
- DOI: 10.33546/bnj.2252
Qualitative research methods allow researchers to understand the experiences of patients, nurses, and other healthcare professionals. Qualitative research also provides scientists with information about how decisions are made and the aspects of existing interventions. However, to get to obtain this important information, qualitative research requires holistic, rich, and nuanced data that can be analyzed to determine themes, categories, or emerging patterns. Generally, offline or in-person interviews, focus group discussions, and observations are three core approaches to data collection. However, geographical barriers, logistic challenges, and emergency conditions, such as the COVID-19 pandemic have necessitated the utilization of online interviews, including chatting as an alternative way of collecting data. This editorial aims to discuss the possibility of online chat interviews as an acceptable design in qualitative data collection.
Keywords: chatting; data collection; messaging; online interview; qualitative research; texting.
© The Author(s) 2022.
PubMed Disclaimer
Conflict of interest statement
The author declares that they have no conflict of interest in this study.
Similar articles
- Online Versus Telephone Methods to Recruit and Interview Older Gay and Bisexual Men Treated for Prostate Cancer: Findings from the Restore Study. Rosser BRS, Capistrant B. Rosser BRS, et al. JMIR Cancer. 2016 Jul 19;2(2):e9. doi: 10.2196/cancer.5578. JMIR Cancer. 2016. PMID: 28410183 Free PMC article.
- Perils and pitfalls for clinicians embarking on qualitative research in physiotherapy. Marshall LR, Edgley A. Marshall LR, et al. Nurse Res. 2015 May;22(5):30-4. doi: 10.7748/nr.22.5.30.e1330. Nurse Res. 2015. PMID: 25976535
- Understanding the Challenges of Intensive Care Staff in Communicating With Patients and Patients' Families During the COVID-19 Crisis: A Qualitative Exploration. Vaz M, D'Silva C, Krishna B, Ramachandran P, D'Souza MC, Mendonca L, Raman P. Vaz M, et al. Cureus. 2023 Jun 26;15(6):e40961. doi: 10.7759/cureus.40961. eCollection 2023 Jun. Cureus. 2023. PMID: 37503489 Free PMC article.
- Selfie consents, remote rapport, and Zoom debriefings: collecting qualitative data amid a pandemic in four resource-constrained settings. Reñosa MDC, Mwamba C, Meghani A, West NS, Hariyani S, Ddaaki W, Sharma A, Beres LK, McMahon S. Reñosa MDC, et al. BMJ Glob Health. 2021 Jan;6(1):e004193. doi: 10.1136/bmjgh-2020-004193. BMJ Glob Health. 2021. PMID: 33419929 Free PMC article. Review.
- Experiences with the implementation of remote monitoring in patients with COVID-19: A qualitative study with patients and healthcare professionals. Van Grootven B, Irusta LA, Christiaens W, Mistiaen P, De Meester C, Cornelis J, Dierckx de Casterlé B, Van Durme T, van Achterberg T. Van Grootven B, et al. J Nurs Scholarsh. 2023 Jan;55(1):67-78. doi: 10.1111/jnu.12814. Epub 2022 Sep 27. J Nurs Scholarsh. 2023. PMID: 36165577 Free PMC article. Review.
- Young adults' experiences of using a young person's mental health peer support app: A qualitative interview study. Cliffe B, Linton MJ, Haime Z, Biddle L. Cliffe B, et al. PLOS Digit Health. 2024 Jul 31;3(7):e0000556. doi: 10.1371/journal.pdig.0000556. eCollection 2024 Jul. PLOS Digit Health. 2024. PMID: 39083458 Free PMC article.
- Barrett, D., & Twycross, A. (2018). Data collection in qualitative research. Evidence Based Nursing, 21(3), 63-64. 10.1136/eb-2018-102939 - DOI - PubMed
- Bryman, A. (2012). Social research methods (4th ed.). Oxford: Oxford university press.
- Dowling, S. (2012). Online asynchronous and face-to-face interviewing: Comparing methods for exploring women’s experiences of breastfeeding long term. Cases in Online Interview Research, 277-296. 10.4135/9781506335155.n11 - DOI
- Free Dictionary . (2022). Chat. https://www.thefreedictionary.com/chatting
- Gunawan, J., Aungsuroch, Y., Fisher, M. L., Marzilli, C., & Sukarna, A. (2022). Identifying and understanding challenges to inform new approaches to improve vaccination rates: A qualitative study in Indonesia. Journal of Nursing Scholarship. 10.1111/jnu.12793 - DOI
Publication types
- Search in MeSH
Related information
Linkout - more resources, full text sources.
- Europe PubMed Central
- PubMed Central
- Citation Manager
NCBI Literature Resources
MeSH PMC Bookshelf Disclaimer
The PubMed wordmark and PubMed logo are registered trademarks of the U.S. Department of Health and Human Services (HHS). Unauthorized use of these marks is strictly prohibited.
share this!
December 23, 2021
Increase in online chatting has adverse effects on reading skills worldwide
by J.g.m. Van Den Elshout (Janneke), University of Twente
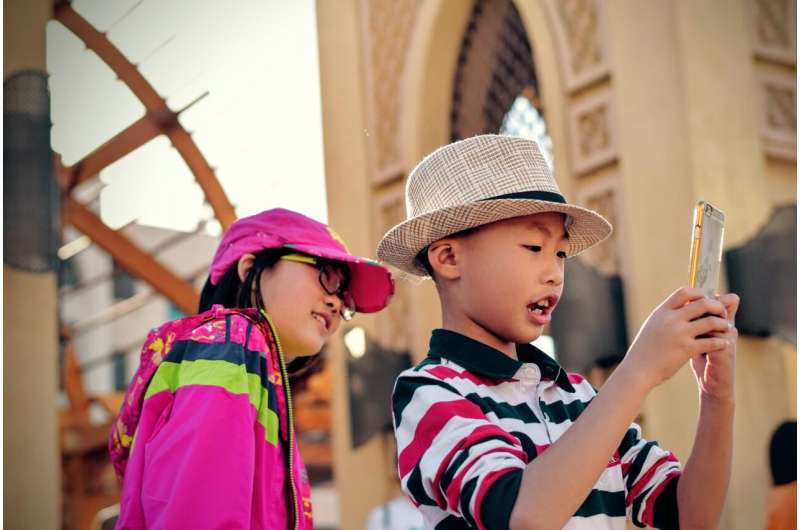
The worldwide deterioration of reading skills due to the increase in online chatting is continuing per country. This is the conclusion of a study by researcher Hans Luyten from the University of Twente. He studied the data from the international PISA-surveys (Programme for International Student Assessment) in 2009 and 2018 in 63 countries. This was part of a large-scale comparative research study conducted under the auspices of the OESO. During that period, online chatting increased significantly in almost every country, but the rate of increase varied considerably from country to country. In Japan, for example, the percentage of students chatting daily increased from 9 percent to 85 percent, whereas the increase in Russia was much lower (from 42 percent to 58 percent).
In recent decades, the rise of information and communication technology (ICT) has greatly influenced the lives of people around the world. Gradually we also started to read more and more on a screen, while a few decades ago we still read everything in paper form. The rise of online chatting was a remarkable development in the first 20 years of the 21st century. There is a risk that digital media can encourage superficial reading strategies, such as scanning, skimming, and browsing, at the expense of more time-consuming strategies that allow for deeper understanding.
The research shows a strong correlation between the country-by-country increase in online chatting among 15-year-olds and the country-by-country decline in both reading skills and awareness of helpful reading strategies. The rise in online chat is the cause of a loss of about 25 score points on the PISA reading scale. Such a drop implies that an average student (who would have previously scored at the 50th percentile) would drop to the 40th percentile.
"While it is important to recognize the adverse effects of online chatting, efforts to reduce this phenomenon seem unrealistic and pointless. Banning online chatting to improve reading skills is just as radical as promoting a return to poverty to rid the world of obesity and other wealth-related health problems," according to Hans Luyten.
The results suggest that a focus on mastering useful reading strategies in reading education is necessary to maintain reading skills. The increased chatting in 2009–2018 has undeniably coincided with a declining awareness of such strategies. It seems that students need more support and stimulation than before to gain their awareness and mastery of useful reading strategies.
Provided by University of Twente
Explore further
Feedback to editors

Women in global fisheries industry are falling through the safety net, study finds
22 minutes ago

In six new rogue worlds, Webb Telescope finds more star birth clues

3D shapes of viral proteins point to previously unknown roles
12 hours ago

Darwin's fear was unjustified: Study suggests fossil record gaps not a major issue
13 hours ago

Using machine learning to speed up simulations of irregularly shaped particles

Closing the RNA loop holds promise for more stable, effective RNA therapies

Scientists use evolution to bioengineer new pathways to sustainable energy and pharmaceuticals

Dual protein analysis approach provides potential way to slow cancer growth

Biomass-based polymer can capture and release CO₂ without high pressure or extreme temperatures

Microscopic fossilized shells reveal ancient climate change patterns
Relevant physicsforums posts, incandescent bulbs in teaching.
Aug 21, 2024
How to explain Bell's theorem to non-scientists
Aug 18, 2024
Free Abstract Algebra curriculum in Urdu and Hindi
Aug 17, 2024
Sources to study basic logic for precocious 10-year old?
Jul 21, 2024
Kumon Math and Similar Programs
Jul 19, 2024
AAPT 2024 Summer Meeting Boston, MA (July 2024) - are you going?
Jul 4, 2024
More from STEM Educators and Teaching
Related Stories

Perceiving sound-letter associations in English can help people learn to read it better
Dec 2, 2021

Study finds boys' dislike for reading fiction is actually fiction
Jul 7, 2021

Girls consistently outperform boys in reading skills—but this could be changing
Feb 12, 2020
Online gaming can boost school scores
Aug 8, 2016

Microsoft introduces new tools for hybrid learning
May 5, 2021

Why do some children read more?
Apr 11, 2018
Recommended for you

More academic freedom leads to more innovation, reports study

Statistical analysis can detect when ChatGPT is used to cheat on multiple-choice chemistry exams
Aug 14, 2024

Larger teams in academic research worsen career prospects, study finds

The 'knowledge curse': More isn't necessarily better
Aug 7, 2024

Visiting an art exhibition can make you think more socially and openly—but for how long?
Aug 6, 2024

Autonomy boosts college student attendance and performance
Jul 31, 2024
Let us know if there is a problem with our content
Use this form if you have come across a typo, inaccuracy or would like to send an edit request for the content on this page. For general inquiries, please use our contact form . For general feedback, use the public comments section below (please adhere to guidelines ).
Please select the most appropriate category to facilitate processing of your request
Thank you for taking time to provide your feedback to the editors.
Your feedback is important to us. However, we do not guarantee individual replies due to the high volume of messages.
E-mail the story
Your email address is used only to let the recipient know who sent the email. Neither your address nor the recipient's address will be used for any other purpose. The information you enter will appear in your e-mail message and is not retained by Phys.org in any form.
Newsletter sign up
Get weekly and/or daily updates delivered to your inbox. You can unsubscribe at any time and we'll never share your details to third parties.
More information Privacy policy
Donate and enjoy an ad-free experience
We keep our content available to everyone. Consider supporting Science X's mission by getting a premium account.
E-mail newsletter
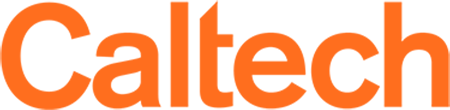
Understanding Online Toxicity
In 2022, Professor of Cognitive Neuroscience Dean Mobbs began to investigate the relationship between social media use and mental health and well-being. As his research program ramps up to test brain activity and physiological markers of stress during social media use, Mobbs and his colleagues, postdocs Swati Pandita, Ketika Garg, and Jiajin Zhang, have constructed a theoretical model to highlight key differences between online and face-to-face communications.
Mobbs, who is also the director and Allen V.C. Davis and Lenabelle Davis Leadership Chair of the Caltech Brain Imaging Center , and an affiliated faculty member of the Tianqiao and Chrissy Chen Institute for Neuroscience at Caltech, calls this model the "DAD" framework. "What is it about the environment of social media platforms that causes more toxicity during social communication than we find in face-to-face communication?" Mobbs asks. The answer is DAD—Disembodiment, lack of Accountability, and Disinhibition—all of which make it more likely that social interactions online skew toward a level of nastiness and misinformation exceeding what we experience when interacting with others in person.
First, disembodiment: In most social media exchanges, people have no direct sensory experience of one another. "When I'm speaking to somebody online," Mobbs explains, "the conversation I'm having is all in my head; it's completely disembodied." Without cues from another's facial expressions or body position, these interactions, though they may feel external, are transacted entirely within one's internal world. It is here, Mobbs says, that the problem lies: "Your internal world is your playground not just for ideas, or for what you want to say, but sometimes for things you shouldn't say. And when you are communicating in this disembodied state, having a conversation in your mind, you begin to forget that you're having a conversation with a real person."
The second factor is lack of accountability: "I can say something via text on social media, and often I don't have to pay the consequences of saying it," Mobbs explains. "I don't get social disapproval at the same level I would as if I were with someone in person, and, frequently, I either am or believe myself to be completely anonymous." Social and cultural norms that operate in person to provide checks on interpersonal communication are absent, and with anonymity, even the fear of criminal punishment is gone.
Lack of accountability and embodied cues leads to the third factor: disinhibition. "It is disinhibition that allows you to say whatever you think, whatever you want," Mobbs says. "All of those nasty thoughts that you have in your head can just come out your fingertips without interference."
The DAD framework is based in an evolutionary perspective on emotion that is at the foundation of Mobbs's research. "We need to understand the conditions in which our emotions and survival strategies evolved, and what the contingencies were within this environment, so that we can then understand how these psychological and behavioral mechanisms are likely to manifest themselves in the significantly altered environment of online communication," Mobbs explains.
Mobbs notes that there are two tools humans rely on extensively to deal with threats. "The first is that with our imagination, we can simulate the world in our minds," Mobbs says. "I can imagine a threat and think about its behavior and my possible reactions before I even encounter the threat. The second is vicarious learning. I watch the news, I listen to people tell stories, or I watch my friend being eaten by a predator, and I learn vicariously. Other animals have different strategies, such as camouflage or enhanced senses that detect threats, but we typically rely on avoiding predators before actually encountering them."
In short, "We have not evolved for a social media environment," Mobbs says. "The sensory systems and theory of mind systems we have evolved in previous millennia do not translate well into an online domain." This leads to the "impaired interactions" that create online toxicity.
The DAD framework suggests that toxic social interactions online can be ameliorated by taking steps to reduce the elements of disembodiment, lack of accountability, and disinhibition on social media platforms. For example, Mobbs and his co-authors suggest that accountability can be strengthened by forcing users to register their accounts under their legal names, by slowing down the rate of interactions, or by introducing AI content moderators to provide more time for users to think about the consequences of their actions. Even the simple use of emoticons and avatars can help to mitigate the effects of disembodiment on our online behavior as they help to make others appear more real and their feelings more apparent.
" Three roots of online toxicity: disembodiment, accountability, and disinhibition " appears in Trends in Cognitive Sciences. The co-authors, Pandita, Garg, Zhang, and Mobbs, are all members of the Division of Humanities and Social Sciences at Caltech, and their work was funded by a gift from Sonja and William Davidow.
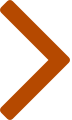
ORIGINAL RESEARCH article
The naturalness of chinese online chatting: organization and recontextualization.
- 1 China Research Center for Language Studies, Nanjing University, Nanjing, China
- 2 Institute of Education, University College London, London, United Kingdom
Two-party or multi-party typed chatting on social media platforms is becoming a popular object of study in pragmatic research nowadays. Apparently, such chatting is very often non-synchronous and non-spontaneous and thus is arguably not so naturally occurring. However, based on a close examination of some details of WeChat typed talk (WTT) among Chinese, the present study seeks to demonstrate that in terms of organization and recontextualization, WTT is naturally occurring in some common as well as distinctive ways and thus amenable to digital conversation analysis (CA). It is hoped that this study may contribute to the understanding of online typed chatting and provide further justification for adopting digital conversation analysis in the study of online typed chatting for performing social actions.
1. Introduction
In the field of pragmatic research, conversational data used for analysis can be authentic or naturally occurring (e.g., those coming from daily face-to-face communication, telephone communication, radio-mediated communication, TV-broadcast face-to-face communication), elicited (such as those collected via the use of questionnaire or discourse complete test), or representational/fictional (such as characters' conversation in novels, plays, or TV series) ( Chen, 2019 ). In the first case, the data reflects what people actually say and how they actually say it in real life; in the second case, the data only stand for what people might or probably say in the projected or imagined situations; in the third case, however, the data only represent what people in the fictional world are designed to say, and how they are designed to say it. Practically, in pragmatics literature, there seems to be an explicit or implicit preference for the use of naturally occurring data over non-natural data (i.e., elicited or representational data) ( Chen, 2019 ), although literary data have their plausible parallel in the real world.
While often explicitly claiming their data to be “naturally occurring,” scholars (e.g., Yu and Li, 2009 ; Hyeri Kim, 2017 ; Yu and Drew, 2017 ) may sometimes take them for granted, without offering or citing any definition of the term. The assumption becomes problematic when we assess the naturalness of the data from non-oral online chatting 1 , such as WeChat typed talk (WTT) and QQ typed talk (QTT) among Chinese. Apparently, the form of talk sometimes lacks spontaneity and synchrony, two essential features of face-to-face naturally occurring conversation. For this reason, can we simply disqualify online chatting as “non-naturally occurring,” given that traditional written communication is basically neither spontaneous nor synchronous, either? Alternatively, can we argue that it is still naturally occurring in its own right, given that it represents the natural and genuine way WeChat users communicate with features of both face-to-face conversation and non-face-to-face written communication? This study favors the latter position and seeks to illustrate the “naturalness” of online chatting such as WTT from two perspectives, namely its organization and recontextualization. We incline to argue that while Conversation Analysis (CA) was designed for spoken conversation, it is not inadequate for analyzing WTT interaction as a form of performing social actions, particularly when it takes on some adaptations (as suggested in digital CA). It is hoped that this study may contribute to the understanding of online chatting on the one hand and provide methodological implications for doing online chat research via digital conversation analysis (CA) on the other.
2. Research background
2.1. defining “naturally occurring”.
Loosely speaking, data used in pragmatics research that are not contrived or elicited (as in simulation contexts, such as those collected by means of questionnaire) can be called natural or naturally occurring. However, in CA, what can be called natural/naturally-occurring data generally need to possess at least the following array of defining features, by the standard of the “primordial kind of human verbal communication” ( Gruber, 2019 , p. 59), i.e., oral, face-to-face conversation:
– Oral (involving paralinguistic features such as pitch, intonation, and voice characteristics).
– Spontaneous (in terms of production).
– Synchronous and immediate (in terms of response).
– Sequentially linear (overlaps kept at a minimum).
– Backchanneled (involving various kinds of immediate mutual feedback). 2
– Multimodal (involving the use of non-verbal aspects such as body movements, facial expressions, gestures, etc.)
– Co-presence of communicators in real-life world ( Gruber, 2019 , p. 59).
With the advent of communication tools such as telephone, radio, Skype, WeChat and QQ, some “affordances” ( Hutchby, 2001 ) of oral face-to-face communication become inactive. For example, in non-visual, non-prepared, non-edited telephone talk, radio-mediated talk, Skype oral talk, WeChat oral talk, and QQ oral talk, 3 body movements, facial expressions and gestures are not visible to one's interlocutor(s) and thus cease to function. Also, the communicators are no longer co-present, such that the turn-taking system may be partly disrupted in the absence of mutual monitoring of speaking. Nevertheless, non-face-to-face, non-prepared telephone talk is still generally assumed to be naturally occurring thanks to its remaining features, especially orality, synchrony, and spontaneity. In existing related research adopting CA, the data are often taken from telephone calls and radio-meditated calls, to the neglect of calls supported by Skype, WeChat, and QQ. 4
By contrast, conversational data from sources such as TV series and novels are generally considered non-natural or not natural enough, although the characters as communicators involved are co-present and the talk is oral, synchronous, immediate, sequential, multi-modal, and backchanneled. The claimed non-naturalness of the kind of conversational data arises from the fact that they are not spontaneous but prepared, edited, and rehearsed. Moreover, conversational data from novels are not oral or multimodal, although the writers may describe some multimodal features such as manners of speaking and emotion as a complement.
Then, how about quasi-conversational forms of Internet-mediated communication such as WTT or QTT? 5 Although they are not oral, they seem to be a lot more natural than fictional data because they are very much spontaneous and very often synchronous, as we shall demonstrate in section 4.
2.2. Research on features and affordances of online chatting
With the development of Internet pragmatics or cyberpragmatics ( Yus, 2011 ), a growing number of scholars show interest in the study of Internet communication such as online chatting, focusing on the effect of its new affordances.
Previous scholars are primarily concerned with the differences between online chatting and face-to-face conversation. For example, Shang (2001) finds that unlike real-world face-to-face chatting, online chatting does not afford the possibility of using body language, facial expressions, and the like. Jiang (2006) notes that online chatting has integrated and subverted traditional/ordinary spoken and written language, as it involves limited contextual constraints, hybridity of style, novel and even bizarre expressions, and volatile and unstable language. Adopting CA, Xu and Li (2012) find that the turn-taking features of online chatting in the virtual world are different from those of face-to-face conversation (e.g., one speaker often starts to type and send an utterance before waiting for the other to finish his or her turn, thus resulting in more “chaotic” turn-taking). Dynel (2014) discovers a distinctive participatory framework underlying multi-party interaction on YouTube, a type of asynchronous computer-mediated interaction, involving changes of the users' participatory statuses at both the production and reception ends. Sandel et al. (2018) point out that WeChat interaction platform affords users new methods for creating messages and online interaction. Wang et al. (2016) study the language exchange via WeChat between students in Australia and the U.S. They claim that WeChat affords a “semi-synchronous” interaction, a term they used to describe the speed of the interaction as falling between synchronous and asynchronous. They demonstrate some of the affordances of this technology, including the ability of users to send messages that use text, audio files, photos, and/or emoticons and stickers. They present examples of data, including screenshots of messages, photos, stickers, and audio messages.
By comparison, very few scholars have addressed the commonality between online chatting and oral, face-to-face communication. As an exception, Sun and He (2006) compare face-to-face English conversation with conversation in online chatroom in terms of turn taking. They find that despite some new and distinctive features (e.g., the initiation of talk with “hello” or “Can i talk to u?” and more frequent occurrence of overlaps), online conversation is not fundamentally different from traditional oral communication.
It is worthy of note that online chatting apps are evolving all the time, making available not only some affordances of oral, face-to-face communication but also new possibilities of meaning making, as we shall see in section 4. For example, the use of audio message, which Shang (2001) mentions as lacking, is now partly afforded in WeChat.
2.3. Use of CA to analyze online chatting
Methodologically, the (non-)naturalness of the conversational data may determine whether they are amenable to “pure” or “real” conversation analysis (CA) (Giles, etc., 2015), which supposedly works on naturally occurring conversational data only ( Yu and Li, 2009 ; Wu and Yu, 2017 ; Yu and Wu, 2020 ). Given that online chats largely lack some important features of natural conversation such as orality and spontaneity but involve unconventional features such as occasional sequential disruption ( Herring, 1999 ) and asynchrony ( Yus, 2011 ), it has been cautioned that they are not suitable for the use of CA approach. As Giles et al. (2015) note:
We were all, to a greater or lesser extent, successfully using conversation analysis (CA) to conduct analyses of online data and publishing this work; yet, we had all encountered some skepticism from journal reviewers and others, to the effect that what we were doing was not “real” CA. Moreover, none of us was aware of any systematic attempt to develop a methodology for the microanalysis of conversation-like data encountered so frequently in online environments, most commonly in the form of asynchronous discussion forum threads, message feeds in social media, or Gmail chat” ( Giles et al., 2015 , p. 45).
Despite some doubt about its suitability, recent years have witnessed the growth in the use of conversation analysis (CA) as a method for analyzing interactional practices in online communication (e.g., Gonzalez-Lloret, 2011 ; Giles et al., 2015 ; for a comprehensive review of related literature, refer to Paulus et al., 2016 ). After exploring the participants' methods of interaction, Housley et al. (2017) pay more attention to the primacy of the online interaction phenomenon and demonstrate how useful CA and MCA (multimodal CA) are in exploring these methods in Twitter posts.
To accommodate the special features of online interactions, Meredith (2017) suggests combining CA and the concept of affordances ( Hutchby, 2001 ) to enable analysis of not only the online interaction, but also the effect of its technological context. On the one hand, by analyzing turn adjacency and openings of online interaction, two key interactional practices in light of CA, we can derive an in-depth understanding of it. On the other hand, depending on technological affordances, we get a clear idea of its distinctiveness. Meredith (2017) argues that this kind of so-called “digital CA” (DCA) as opposed to “spoken CA” is a kind of “refracted” CA due to the lens of technological affordances, which can develop a better understanding of interactional patterns across different online platforms. Indeed, some researchers have attempted to adapt CA into DCA in order to analyze online conversation. For example, Meredith (2017) has developed a transcription system for the screen-capture data on the basis of the Jefferson system ( Meredith, 2016 ).
By and large, there is still much to do with the development of DCA with regard to various methodological questions such as to what extent spoken CA findings apply to the analysis of online interaction ( Meredith, 2017 ). On the conceptual side, however, an even more significant question is whether we should confine our attention to them at all, especially as online interaction platforms are evolving so fast. We should not only look for different or new manifestations of spoken CA features, but also embrace new, natural mechanisms of digital conversational organization and recontextualization. The latter, while discussed in discourse analysis (DA) (e.g., van Leeuwen, 2008 ; Culpeper and Haugh, 2014 ; Gruber, 2019 ), has been underexplored in CA. In this direction, the present study on WTT, which has been underexplored, serves as an illustration. Specifically, it will address the following research questions:
a. How is WTT organized for performing social actions in terms of turn taking, adjacency, repairing, etc.?
b. How is recontextualization realized in WTT?
3. Data sources and analysis
For convenience sake and from an emic perspective, all the data of this study were excerpted from the recent WeChat interaction that all involved the first author (10 one-on-one private chats and 15 group chats) but only occasionally the second author (5 group chats). The chats varied in length of turns (from 4 to 16). Of the 25 chats, 18 were more or less synchronous and continuous, whereas the remaining 7 chats involved long breaks that significantly affected the continuity of the chatting. Also, 23 of the chats were interpersonally oriented, whereas 2 chats related to work.
Apart from the first author (and the second author), other people involved in the WeChat groups included family members, colleagues, students, or friends. 6 The version of WeChat app used was the latest one at the time of writing this paper (i.e., 8.0.22) for mobile phone and for computer (3.6.0.1000), both launched in April, 2022. WTT 7 varies in its composition of modalities. It can be purely typed language; it also can be a mixture of typed language and emojis, or a mixture of typed words and voice message(s), or a mixture of typed language, emojis and voice message(s). This study only concerned typed talk with or without emojis.
To answer the two research questions, the first author looked into how WTT interactants organized and recontextualized information, bearing in mind the notions of CA (such as turn taking, adjacency pairing, and repairing) and recontextualization (notably features of intertextuality such as quoting). In recognizing spontaneity and continuity of turn taking, we depended on the technological 5-min threshold of WeChat beyond which breaks will be automatically marked by a new time message. Meanwhile, he paid close attention to finding whether any new manners of organization and recontextualization have emerged or occurred. As no quantitative analyses would be attempted, he just detected varied instances of WTT organization and recontextualization. He would take screenshots whenever he ran across relevant cases such as spontaneity, (a) synchrony and mechanism of turn-taking, as well as modes of recontextualization.
In the presentation of the data for analysis and discussion below, the authors removed personal data to comply with ethical requirements. The data were not transcribed with a strict CA format (because features such as pauses, pitch, and overlaps were not recognizable) but presented in the same manner and sequence as they actually showed on the screen, with emojis retained where they were, in a way very much similar to the use of screenshots neatly practiced by Sandel et al. (2018) . Yet the dialogues on the screen were converted into the format of exchange we see in regularly transcribed conversation.
4. Findings
This section presents the findings in relation to the two research questions.
4.1. The organizational features of WTT
Very often, WTT as found in our data is a synchronous, jointly and continuously completed chat. This occurs especially when the communicators are performing a particular task. Asynchronous WTT occurs often when one or two sides are occupied or distracted by some other task(s). Occasionally, an asynchronous response is ascribable to a negative attitude or a reluctance to reply immediately. Yet, sometimes, it is hard to tell which. For this reason, WTT, like Facebook chat, is quasi-synchronous ( Garcia and Jacobs, 1999 ). In other words, for WTT, sometimes, “synchronicity is not a fixed feature but rather is something that participants negotiate through the course of the interaction.” ( Meredith, 2017 , p. 53). Most explicitly, the synchrony is technically marked by the signaling of “对方正在输入…” (XX is inputting …) on the top line of the screen, as demonstrated in Ex. 1:
1 陈: 忙啥呢?
2 吴: 你猜呢?
3 陈: 我猜你在写文章吧?
6 吴: 呃
7 陈: ? ?
[对方正在输入…]
8 吴: 好吧
1 Chen: What are you busy with?
2 Wu: Guess?
3 Chen: You're writing the paper, I guess?
7 Chen: ? ?
[Wu is inputting …]
8 Wu: Oaky.
In Ex. 1, after Chen shows puzzlement (line 7) over Wu's use of a hesitation marker “uh” (line 6), Wu is shown to be working out a response, technologically indicated by “Wu is inputting ….” The signal does not occur anytime when somebody is offering a response. Rather, it occurs only when the recipient responds by beginning to type in the input space within 10 s after receiving the speaker's message. It immediately disappears after the message being typed is sent out. The affordance of the app can be shut down manually, though.
In WeChat platform, a response is technologically recognized as synchronous as long as it follows a previous message within 5 min. Thus, the notion of synchrony is very much stretched, although responses often are almost equally immediate. Most often, WTT is sequentially well-organized in the turn-taking format, very much similar to face-to-face conversation is, as shown in Ex. 2:
1 陈: 你好!周五下午有课吗?
2 李: 老师您好!没有课的, 一定来听讲座。
3 陈: 想请你去南京农业大学翰苑宾馆接下黄老师, 下午2点到那儿接。
4 李: 好的老师!
5 陈: 136xxxx0530
6 陈: 他的电话
7 李: 好的, 谢谢老师!我和黄老师联系。
1 Chen: Hi, do you have class this Friday afternoon?
2 Li: Hi, professor. No, I'll have no class. I'll come to attend the lecture!
3 Chen: I'd like you to pick up Professor Huang from Hanyuan Hotel of Nanjing University of Agriculture. Two o'clock.
4 Li: Alright, professor.
5 Chen: 136xxxx0530
6 Chen: His phone number
7 Li: Ok. Thank you, professor. I'll contact him.
In Ex. 2, Chen and Li speak alternately in the first four lines, resulting in a joint construction of the conversation. For both the fifth and sixth lines, Chen apparently occupies two turns. He could have typed the two messages in one single turn. In reality, he sends the two messages one after another. This has happened perhaps as an afterthought or an increment because he wants to clarify that the phone number is that of Professor Huang, which partly evidences the spontaneity of WTT. Yet, more often than not, sending a series of broken or fragmental messages in continuous turns rather than a complete message in one single turn occurs in very relaxing chatting between familiar or intimate interlocutors (it can also be an idiosyncratic practice, though), as shown in Ex. 3:
1 妈妈:@Peter 你的胃好些了吗
2 儿子: 吃了药以后连续两天不疼了
3 儿子: 很有效果
4 妈妈: 养胃是一生的事
5 妈妈: 咖啡、可乐等不能多喝
6 妈妈: 牛奶、稀饭养胃
7 妈妈: 白开水是最好的饮料
8 妈妈: 烟酒都不能沾
9 妈妈: 作息有规律
10 儿子: 是的是的
11 儿子: 马上就睡了
12 儿子: 嗯嗯
1 Mom: @Peter Does your stomach feel any better?
2 Son: It does not pain after I've taken medicine for 2 days on end.
3 Son: Very good effects.
4 Mom: Nourishing the stomach is a lifelong thing.
5 Mom: Can't drink much coffee, kola and the like.
6 Mom: Milk and porridge nourish the stomach.
7 Mom: Boiled water is the best drink.
8 Mom: Can't smoke or drink alcohol.
9 Mom: Work and rest need to be regular.
10 Son: Yes, yes.
11 Son: Going to bed soon.
12 Son: Okay, okay.
In Ex. 3, the son could have finished his first utterances (lines 2–3) in one single shot (i.e., sent out with one single press or click of the button “send”). The same applies to the mom's utterances in lines 4–9. There is another possible reason for the mom not to finish her utterances at one shot: she is adding up new points as she is thinking. Since she is chatting with her son, she may find it more comfortable and natural to talk like this than produce a long message after it is completely thought over and ready for articulation. This is just another manifestation of the widely and often distributed spontaneity of WTT (the production of these utterances is completed within a short span of time and, apparently in fast succession; after all, there is the 5-min threshold for technological recognition of it as continuous chatting on the WeChat platform). 8
Owing to non-co-presence, participants to WTT cannot monitor each other's speaking time in the operation of turn-taking. This inability may lead to apparently messy turn taking in WTT. 9 For example, in Ex. 3, the son's response “Yes, yes” (line 10) is a response to his mother's views (lines 4–9) on how to keep a healthy stomach; his response of “Going to bed soon” is targeted specifically at her point “Work and rest need to be regular” (line 9); and his last utterance “Okay, okay” shows the son's compliance with his mother's advice, including “Can't smoke or drink alcohol” (line 8).
Yet, despite the occurrence of such disrupted synchrony, we should not refute the basic fact that people are still doing turn taking in their interaction and that the resultant WTT is still sequentially organized. Example 3 involved what Herring (2013) termed “loosened relevance” of adjacency pairs in CMC. The notion may be evoked to explain how interactants make sense of apparent non-adjacency. After all, participants can read back and forth and make sense of the apparently disorderly messages. In other words, we can still identify adjacency pairs from sometimes disorderly exchange of utterances.
As in face-to-face conversation, participants to WTT can repair their messages immediately, which is another important signal of spontaneity. Consider Ex. 4:
2 陈: 不用 keq
3 陈: 客气
4 曹: 应该的啊, 老师千万莫推辞哦。
2 Chen: No need to keq
3 Chen: stand on courtesy ( keqi )
4 Cao: It's what we should do. Please do not decline.
In Ex. 4, Chen makes a mistake (line 2) when using Chinese pinyin to type the expression “客气.” Actually, he is using the English input mode to type the characters.
Another sign of spontaneity in terms of WTT organization is the recalling of messages. Technologically, WeChat app affords the opportunity to recall one's message within 2 min, as indicated in Ex. 5.
1 蔡: 各位好, 晚上有人打牌吗?
2 徐: 我
3 蔡: 呵呵 还要两人呀
4 游: @sky 如果缺人, 我可以参加
5 刘: 我可以补缺
6 蔡: 好啊 五点结合!
7 刘: 啥地方
8 游: 都是响应会长的号召啊
sky 撤回了一条消息
9 蔡: 会长定的地点
10 刘: 可以啊
1 Cai: Hi, all. Wanna play cards this evening?
3 Cai: Hehe, we need two more
4 You: @sky I can if needed
5 Liu: I can fill a vacancy
6 Cai: Okay. Let's meet at five.
7 Liu: Where
8 You: We're all responding to the president's call
sky has recalled a message
9 Cai: The place fixed by the president
10 Liu: Alright
When recalling occurs, the platform will leave a trace of recalling, as shown by the shadowed part in Ex. 5. Sometimes, we do not just recall a message, but go on to repair it and resend a revised message. In this case, the platform will leave the trace “你撤回了一条消息 重新编辑” (You have recalled a message re-edit) on the sender's screen above the resent message. Note that repairs, as well as restarts and reruns, although frequently done, are not visible in WTT unless the repaired message has been sent and then recalled within 2 min. This might undermine the degree of our claim about the spontaneity of WTT and thus its suitability for CA analysis, as some important information inferable from the repairs, reruns and restarts is lost.
One remarkable aspect of turn-internal organization in WTT signaling spontaneous “monitoring” is bracket annotating (Song and Chen, in preparation). Consider Ex. 6:
1 A: 你们有无看好的馆子
2 A: 一直想去的
3 B: 我好像不知道有啥
4 C: 唯一一家看好的是澡堂
4 C: (bushi)
5 A: 哈哈哈哈哈哈
6 A: 快分享一下
1 A: Do you have any good restaurant to recommend?
2 A: One you always want to choose
3 B: I'm afraid I don't know any
4 C: The only one I favor is a bathhouse
6 A: Hahhhh
7: A: Share it right now
In Ex. 6, in response to A's request to recommend a good restaurant, C jokingly recommends a bathhouse instead (line 4). Then, she immediately follows up with a clarification “No” within the brackets. Such form of interactional practice in turn-internal organization, which is frequent among young Chinese netizens, serves to clarify the speaker's jocular intent (as in Ex. 6) or perform some other pragmatic functions (Song and Chen, in preparation). 10
Another function worthy of special mention is tickling. Specifically, a member of a chat group can tickle someone else during a chat, without saying anything. For example,
1 A: 今晚约球
3 C拍了拍B
4 B: 今晚有事, 改天约
1 A: Let's meet this evening and play table tennis
3 C tickles B
4 B: I've got an appointment today. Let's meet some other day.
Other organizational features of face-to-face conversation such as the occurrence of insertion sequence and back sequence ( Levinson, 1983 ) are also found in WTT. As they are easily recognizable and identical with those in face-to-face interaction, we choose not to spare any space to illustrate them here.
4.2. Recontextualization features of WTT
As in the case of discourse organization, WTT also demonstrates both common and distinctive features in terms of discourse representation or, more generally, recontextualization in discourse.
By recontextualization, we mean “the insertion of a discourse element from a source context into a target context” ( Gruber, 2019 , p. 61). As a ubiquitous discursive practice ( van Leeuwen, 2008 ), it may take on various forms. In traditional spoken and written communication, it may be realized as either direct or indirect reported speech. In written communication, direct reported speech, generally a verbatim representation of a chunk of the source text, is marked by the use of quotation marks, whereas indirect reported speech is a paraphrase of the represented content (usually coupled with the source information in both cases). In oral communication, we may mark our direct 11 or indirect quoting of others' utterance by metapragmatic expressions ( Culpeper and Haugh, 2014 ; Chen, 2020 , 2022 ) such as “as you said,” “somebody once said,” and “in somebody's words.” In both written and oral communication, we can quote our speech or cite our own text by using metapragmatic expressions such as “as I said earlier” and “as I mentioned in Section 2”.
In online communication, Internet communicator tools (ICTs) provide built-in program features that enable users to insert an entire previous message (or parts of it) automatically into a current message ( Gruber, 2019 , p. 57). The built-in quoting and sharing features make exact quoting possible (for a relatively complete review of research on different forms and practices of discourse representation in various media and genres, refer to Gruber, 2019 ).
Like other apps, WeChat app provides its users with effective affordances for doing recontextualization or discourse representation. One affordance is the citing function. It was introduced into WeChat (Edition 7. 0.9) in 2019. By long pressing the target message (or photo) or right-click the message (or photo) on the mobile phone, 12 the citing function will appear along with other functions on the screen. Communicatively speaking, the function is particularly necessary and useful, because it is sometimes difficult to respond to an earlier utterance without repeating it verbally. Since it has been intervened by pieces of more recent utterances, understanding based on adjacency or coherent pairing sometimes becomes difficult or even impossible. With the affordance of the citing function, the earlier utterance or coherence is selected and then “inserted” in the current context in a marked way as shown in Ex. 8. In so doing, adjacency is recovered so that the new utterance could be comprehended in the context of this inserted former utterance. Consider the shadowed part of Ex. 8, which indicates the citing function:
1 陈: 请写一个本次会议的报道
2 孙: 好的, 我知道了, 写完后发在公众号吗?
3 孙: 后面可能还是要弄个网站。网站和公众号还是不一样的
4 陈: 应该有网站吧
5 陈: 报道写好后让我看一下
6 孙: 好的
7 孙: 学院主页上中心有个链接, 现在打不开
陈: 应该有网站吧
8 陈: 问一下
9 孙: 好的
1 Chen: Please write a report on the meeting
2 Sun: Okay. Got it. Publish it on our official accounts after finishing it?
3 Sun: Perhaps we need to build a website afterwards. Websites and official accounts are different
4 Chen: I guess we've already got one
5 Chen: Let me have a look after you finish the report
6 Sun: Alright
7 Sun: There's a link to the Center on the School's website, but it can't be accessed
Chen: I guess we've already got one
8 Chen: Ask someone
9 Sun: Okay.
In Ex. 8, Chen not only mentions that there should be a website for the Center (line 4), but also asks Sun to let him have a look at the report when it is ready (line 5). Sun first responds to Chen's request. Then, with the citing function, she quotes his guessing utterance in line 4 and responds to it with what she has found in relation to the Center's website.
Unlike the case of the citing function, which enables verbatim representation of the cited content and form, WTT enables partial representation or paraphrase of the source message. Consider Ex. 9:
(Context: Chen shared a lecture poster)
1 曹: @陈 老师早!谢谢分享
1 Cao: @Chen Morning, professor. Thanks for sharing
In Ex. 9, after Cao greets Professor Chen and thanks him for sharing the lecture poster (line 1), several former doctoral students of Professor Chen follow suit (lines 2–5). It is quite evident that Yuan, Yang and Cheng have more or less duplicated or reworded Cao's utterance (including the emojis) in one way or another. Shen not only borrows exactly what Cheng says but also goes a step further by adding that she is looking forward to the lecture (line 5).
A less explicit way of recontextualization in WTT is to resend or forward a post or utterance(s) from one chat group to another or other groups. The outcome is that the forwarded post or utterance(s) extracted from the source context has acquired a new context and may be processed similarly or differently in terms of its meaning or function in the new context. Note that Gruber (2019) calls such online practice “decontextualizing” (p. 75) rather than recontextualizing, in the sense that the post or utterance(s) is removed out of its original context but resent to someone else or members of other group(s).
It is worth mentioning that recontextualization by way of traditional means such as the use of metapragmatic expressions of citations (e.g., “as you said,” “sb. once said”) is still applicable in WTT.
5. Discussion
From the illustrations of the organization and recontextualization of WTT, we can find that it is a form of digital conversation that is naturally occurring in its own way. Notably, we mean that it is not only similar to face-to-face interaction in many ways, but also different in several ways.
On the one hand, WTT is an immediate, spontaneous, synchronous, sequentially completed chat 13 to a significant extent, with more or less frequent exceptions. In WTT, we find a variety of face-to-face conversational features and mechanisms of organization, such as immediate response, turn taking, repairs, fragmental speech, overlapped speech, and reported speech. Yet, we need to interpret the notions of immediacy and synchrony not as purely temporal notions but rather sequential ones. Although sequence in WTT equals immediacy in time on many occasions, it does not always denote real immediacy. As mentioned earlier, on WeChat platform, a follow-up message is displayed on the screen as an immediate one as long as it is sent within 5 min since the preceding message by oneself or the other interactant was sent.
On the other hand, WTT is natural in many unique ways. To start with, the spontaneity of WTT is technologically afforded in various ways. For example, it is sometimes indicated by “对方正在输入…” (XX is inputting …) on the top line of the screen. It is sometimes shown by the practice of recalling a message marked by “XX has recalled a message” at the turn where it takes place. Also, it is sometimes manifested by the use of bracket annotation for various purposes, which has little or even no equivalent in face-to-face interaction or written communication (but sometimes in drama scripts). 14 Secondly, WeChat platform affords the chance to “listen” back in WTT, thanks to the persistence and storability of interactions ( Gruber, 2019 , p. 55). As the interlocutors are not co-present, overlapped speech seems to occur more frequently than in face-to-face interaction. This may more or less disrupt the turn-taking system characterized by sequential organization. Yet the change to “listen back” reduces the risk of missing messages and enhances the chances of “deep” reading. Finally, in terms of recontextualization, WeChat platform affords quick and accurate reported speech owing to the embedded copying function, and convenient text quoting thanks to the embedded citing function.
With the invention and application of various social media, our way of communication has undergone significant changes. We communicate not only in face-to-face physical world but also in non-face-to-face, non-co-present virtual world. Communication in the latter world has made us gradually but fundamentally accustomed to interaction involving varying degrees of non-immediacy, non-synchrony and spontaneity. Partly because we often perform multi-tasks today and partly we try not to engage others who we reckon may be occupied at the moment, we take occasional or even frequent short-term delayed responses to be normal and natural and respond to them as if they were synchronous. WTT reflects the new natural form of interaction. Neither the reception of messages nor subsequent feedback is or needs to be immediate or synchronous. It can be synchronous and non-synchronous alike. In cases of little or no urgency, we choose to exchange short-text messages, synchronously or non-synchronously, instead of making audio/visual phone calls, or going offline to interact. In the new form of interpersonal interaction, when we cannot or even need not immediately capture the thinking process of others manifested in restarts, reruns or repairs, we do not always care about it (although it significantly helps us capture our interlocutor's intention and attitude). Rather, we care more about what he or she has actually and eventually “said” and how it is “said.” Although delayed response sometimes suggests a passive attitude (e.g., a refusal, a disagreement) in face-to-face interaction, we generally do not take the delay to be negative but rather to be a consequence of non-availability of the interlocutor at the moment. To avoid potential misunderstanding, we do sometimes provide an explanation to excuse ourselves from making a long delayed response. When it comes to recontextualization, the affordances of WTT enable novel recontextualization formats for representing extracts from previous utterances in their actual utterances ( Gruber, 2019 , p. 55–56). They satisfy the need of economy and sometimes that of alignment ( Lee and Tanaka, 2016 ). For one thing, when following other group members in responding to a post sharing good or important news, directly copying a prior response more or less verbatim and then posting it saves time and improves efficiency. At the same time, the practice contributes to alignment among group members by way of complete or partial identical response. In addition, the citing function facilitates intertextuality and can maximally rescue the disruption of turn-taking system caused by non-co-presence-induced non-synchrony, while guaranteeing the degree of accuracy of discourse representation ( Gruber, 2019 , p. 55).
All the unique features of WTT, functionally motivated and technologically afforded, are “genre constitutive practices” ( Gruber, 2019 , p. 55). Based on these features, we may conclude that it constitutes a distinctive sub-genre of cyber discourse. Given the primacy of phenomenon, we need to “recognize some of the wider potential for reading and applying Sacksian principles in ways that move beyond a sole focus on sequential matters within talk and interaction” ( Housley et al., 2017 , p. 15). Instead of strictly following the hard-line of pure CA analysis, “a wider interpretation of Sacks' work can be mobilized and in ways that transcend any “narcissism of small differences” amongst the ethnomethodological, conversation analytic and wider interactionist Communities” ( Housley et al., 2017 ). After all, Sacks used to take an interest in the phenomena per se , observing, describing and documenting how members do social life in “any possible places and ways” ( Fitzgerald, 2018 , p. 7) it occurs. Actually, as he focused on social actions, he could engage in whatever data he had available, including social media data, in ways that enable systematic, repeatable and inspectable analyses ( Fitzgerald, 2018 ). This means that we can, sometimes at least, use CA to directly handle online conversation, which, like traditional face-to-face conversation, falls within the “scope of humans activities as methodical” ( Fitzgerald, 2018 , p. 8). We are not supposed to doubt about the suitability of subjecting WTT to CA analysis, only because some important information inferable from the reruns and restarts is lost. Instead of rejecting CA for online conversation, we can extend, enrich and modify the current version of CA to capture the new affordances made possible by the new platform of interaction, as suggested by Giles et al. (2017) :
“As more researchers in the social sciences and humanities are turning to digital phenomena as their substantive objects of interest, it is becoming increasingly clear that traditional methods of inquiry need considerable adjustment to fully understand the kinds of interaction that are taking place in online environments.” ( Giles et al., 2017 , p. 37)
As a genre variant of digital communication, WTT exhibits the combined effect of “pull factors” and “push factors” ( Gruber, 2019 , p. 57). The former have to do all kinds of Internet Communication Tools (ICTs) as new medium affordances; the latter have to do with the communicative needs of a social group faced with a communicative problem ( Gruber, 2017 ). Thus, digital CA needs to embrace the idea that online chatting like WTT is “digitally” naturally occurring, and take into account all the technological affordances of organization and recontextualization when analyzing collected typed talk from social media platforms.
Moreover, digital CA can still be improved, at least by benefitting from the use of input log software. Clearly, one disadvantage of digital CA is its inability to capture the speaker's languaging ( Li, 2011 ) process. Although we find conversational repairs in WTT, they probably occur with a lesser frequency in face-to-face interaction, as observed from the perspective of the interlocutor. Actually, our own experience of WTT is that as “speakers,” we repair very frequently. However, in WTT, pauses, reruns and restarts are not visible. 15 Although this is still not technologically shown, the record of our “languaging” process via an input log software would tell the whole story. If that is done, we will be able to record and analyze the spontaneous and significant features of WTT even though the process and result of reruns and repairs are not shown on the screen of the interlocutor's computer or smart phone.
6. Conclusion
The goal of this paper has been to demonstrate the naturalness of WTT as a form of digital conversation in terms of interactional organization and recontextualization in particular. Based on a close examination of some details of WTT, we have concluded that WTT is natural in both common and distinctive ways. On the one hand, its naturalness consists in partial or even predominant spontaneity and synchrony characteristic of face-to-face conversation. On the other hand, it is technologically marked in some noticeable ways, such as the recalling of a message, the act of “X is inputting …,” the act of bracket annotating, the citing of a prior message, and the act of copying. These features shown on the screen may impress us as if we could feel the co-presence of our interlocutors. Although interactants occasionally overlap in some disorder, owing to the non-co-presence of the interactants, WeChat platform allows them to read back to sort out the adjacency issue. In view of the above-mentioned features of WTT, which make it a unique sub-genre of digital conversation, it is clear that while we can apply canonical CA in some cases, we can enact a modified version of CA in other cases. For this reason, the assumption that CA is not applicable to social media data, while cautious and helpful, may risk oversimplifying the picture of online chatting and overlook its ubiquitous and sometimes distinctively “natural” features. Instead, it is sensible that we adopt an alternative view of the notion of “(non-) naturalness.” Specifically, we need to bear in mind the “natural” features of online chatting made possible by various technological affordances. In so doing, we are truly embracing Sacks' insistence on the primary focus of the phenomena, and have great chances of understanding online interaction “members' social action and their methods in undertaking that social action” ( Fitzgerald, 2018 , p. 9).
The present study, first of its kind to relatively systematically examines patterns of WeChat organization and recontextualization, adds to the existing literature on messaging on other platforms, mostly in English. Some issues to be addressed in the future regarding WeChat talk may include the possible reasons and interpersonal consequences of delayed response as opposed to immediate response, message recalling and emoji/emoticon use, the manifestations of interactional style choice between involvement vs. detachment (such as occurrence of overlaps, use of backchanneling, use of emoji, and short, fragmental turns) and the like. The issue of emoji literacy is worthy of special mention, as discrepancy in understanding the emojis may mean possible digital “generation gap” and “gender gap” and lead to severe interpersonal consequences. Finally, the issue of inadequate contextual support in WTT due to its being non-situated calls for the reconceptualization of context in a different way so that the analysis of WTT could benefit from it.
This study suffers various limitations. First, in terms of data collection, the data size and extent were limited. Second, the selection of instances of “spontaneity,” “mechanism of turning taking” and “modes of recontextualization” might not be systematic in the absence of a principled scheme. Third, the issue of recontextualization only receives limited discussion in this study although it could be much broader/more complex. Finally, we only addressed the organization and recontextualization in WTT, to the neglect of other social media. Different platforms vary in their technological affordances and thus may enable different forms of organization and recontextualization. Different ICTs provide quite different possibilities for representing and integrating reported utterances into a report utterance ( Gruber, 2019 ). The differences and variations may constitute a key challenge for analyzing digital communication ( Meredith, 2017 ). How to capture the naturalness of other digital communication remains to be explored.
Data availability statement
The raw data supporting the conclusions of this article will be made available by the authors, without undue reservation.
Author contributions
XC provides the general structure and basic ideas of the paper. TC helps to develop the draft of the paper and supply needed references. All authors contributed to the article and approved the submitted version.
This work was funded by National Social Science Fund of China (20&ZD299).
Acknowledgments
The early version of the study was presented at the First International Conference on Internet Pragmatics held in Fujian Normal University (FNU) on September 21-23, 2018.
Conflict of interest
The authors declare that the research was conducted in the absence of any commercial or financial relationships that could be construed as a potential conflict of interest.
Publisher's note
All claims expressed in this article are solely those of the authors and do not necessarily represent those of their affiliated organizations, or those of the publisher, the editors and the reviewers. Any product that may be evaluated in this article, or claim that may be made by its manufacturer, is not guaranteed or endorsed by the publisher.
1. ^ Online chatting can be oral as well; for example, WeChat and QQ apps both allow phone-like calling and afford voice messaging. Sometimes, online chatting is a mixture of oral and typed production. In this study, we only focus on written chatting implemented by typing either on the mobile phone or the computer.
2. ^ Backchanneling also occurs in WTT ( Hu and Chen, 2017 ; Zhong, 2017 ), though to a much less frequent and explicit extent.
3. ^ Actually, visual talk is also afforded on these platforms.
4. ^ Intermittent voice messages sent from WeChat, Skype, QQ, etc. are different from calls mediated by the apps, because synchrony is not always ensured.
5. ^ WTT and QTT are also very often/very much multimodal through the use of emojis.
6. ^ One reviewer pointed out that our data were mostly based on the chats of a single person (with various other persons), which may affect the possibility of finding more patterns of organization and recontextualization. To make up for this, we shared our findings with friends and students around us and asked them to report if they could think of new possibilities. This was rewarding, as we found a new pattern, as reported in Ex. 7, which came from earlier WeChat interaction the first author participated in.
7. ^ WTT sometimes involves the use of punctuation marks at the end of an utterance, sometimes not, very much depending on personal habits.
8. ^ We do not deny, as one reviewer commented, that there may be much more complexity there (e.g., see Baron, 2010 ).
9. ^ In face-to-face conversation, overlapped utterances will concur in time. In contrast, in WTT, the overlapped utterances still occur sequentially on the screen, resulting in “messy” turns. Thus, we need to sort them out as overlapped speech first on the basis of their directionality, i. e., to which prior utterance the current utterance is directed.
10. ^ Apart from ( ), Chinese young netizens may also use ≪≫ to emphasize something put within the sign.
11. ^ Very often, a verbatim oral report of a previous utterance is hard to provide and thus not be expected ( Tannen, 1989 ).
12. ^ On the computer, we right-click the target message or photo to pop out the citing function of WeChat.
13. ^ This occurs especially when the communicators are performing a particular task. Asynchronous WTT occurs often when one or two sides are occupied or distracted by some other task(s). Occasionally, an asynchronous response is ascribable to a negative attitude or a reluctance to reply immediately. Yet, sometimes, it is hard to tell which. It seems that a person in a superior position is more likely to delay or even refrain from a response, something worthy of verification on the basis of large-size data. For this reason, WTT, like Facebook chat, is quasi-synchronous ( Garcia and Jacobs, 1999 ). In other words, for WTT, sometimes, “synchronicity is not a fixed feature but rather is something that participants negotiate through the course of the interaction.” ( Meredith, 2017 , p. 53).
14. ^ The technological affordance, based on input convenience (cf. Song and Chen, in preparation), makes WTT a distinctive genre resembling that of drama scripting.
15. ^ In social media apps, such as iMessage, Facebook Chat, or WhatsApp, the “…” symbol appears when another person is typing. However, WeChat does not have this function. Therefore, the content or form of a message is not known until it is sent and appears on the recipient's screen.
Baron, N. S. (2010). Discourse structures in instant messaging: the case of utterance breaks. Language@Internet 7, 1–25. Retrieved at: www.languageatinternet.de
Google Scholar
Chen, X. (2019). You're a nuisance! Patch-up jocular abuse in Chinese fiction. J. Pragmatics 139, 52–63. doi: 10.1016/j.pragma.2018.10.015
CrossRef Full Text | Google Scholar
Chen, X. (2020). A new taxonomy of metadiscourse based on metapragmatics. Foreign Lang. Teach. Learn. 1–10. doi: 10.13458/j.cnki.flatt.004692
Chen, X. (2022). Metapragmatics and the Chinese Language . Newcastle upon Tyne: Cambridge Scholars Publishing.
PubMed Abstract | Google Scholar
Culpeper, J., and Haugh, M. (2014). Pragmatics and the English Language . Basingstoke: Palgrave McMillan.
Dynel, M. (2014). Participation framework underlying YouTube interaction. J. Pragmat. 73, 37–52. doi: 10.1016/j.pragma.2014.04.001
Fitzgerald, R. (2018). The data and methodology of Harvey Sacks: lessons from the archive. J. Pragmat. 143, 205–214. doi: 10.1016/j.pragma.2018.04.005
Garcia, A. C., and Jacobs, J. B. (1999). The eyes of the beholder: understanding the turn-taking system in quasi-synchronous computer-mediated communication. Res. Lang. Soc. Interact . 32, 337–367. doi: 10.1207/S15327973rls3204_2
Giles, D., Stommel, W., and Paulus, T. (2017). The microanalysis of online data: the next stage. J. Pragmat. 115, 37–41. doi: 10.1016/j.pragma.2017.02.007
Giles, D., Stommel, W., Paulus, T., Lester, J., and Reed, D. (2015). Microanalysis of online data: the methodological development of “digital CA”. Discourse Context Media 7, 45–51. doi: 10.1016/j.dcm.2014.12.002
Gonzalez-Lloret, M. (2011). Conversation analysis of computer-mediated communication. CALICO J 28, 308–325. doi: 10.11139/cj.28.2.308-325
Gruber, H. (2017). Quoting and retweeting as communicative practices in computer mediated discourse. Discour. Context Media . doi: 10.1016/j.dcm.2017.06.005
Gruber, H. (2019). Genres, media, and recontextualization practices: re-considering basic concepts of genre theory in the age of social media. Int. Pragmat. 2, 54–82. doi: 10.1075/ip.00023.gru
Herring, S. C. (1999). Interactional coherence in CMC. J. Comput. Med. Commun. doi: 10.1109/HICSS.1999.772674
Herring, S. C. (2013). “Relevance in computer-mediated conversation,” in Pragmatics of Computer Mediated Communication , eds S. Herring, D. Stern, and T. Virtanen (Berlin: Mouton de Gruyter), 245–268.
Housley, W., Webb, H., Edwards, A., Proctor, R., and Jirotka, M. (2017). Digitizing sacks? approaching social media as data. Qual. Res . 17, 627–644. doi: 10.1177/1468794117715063
Hu, C., and Chen, R. (2017). “Backchanneling for positive politeness in Chinese TV interviews,” in Politeness Phenomena across Chinese Genres , eds X. Chen (Sheffield: Equinox), 47–70.
Hutchby, I. (2001). Technologies, texts and affordances. Sociology 35, 441–456. doi: 10.1177/S0038038501000219
Hyeri Kim, S. (2017). Enacting surprise with ani ‘no'-prefaced questions. East Asian Pragmatics 2, 141–161. doi: 10.1558/eap.34617
Jiang, Y. (2006). The pragmatics and culture of online chatting. J. Beijing Norm. Univ. 63–68. doi: 10.3969/j.issn.1002-0209.2006.02.009
Lee, S. H., and Tanaka, H. (2016). Affiliation and alignment in responding actions. J. Pragmat. 100, 1–7. doi: 10.1016/j.pragma.2016.05.008
Levinson, S. (1983). Pragmatics . Cambridge: Cambridge University Press.
Li, W. (2011). Moment Analysis and translanguaging space: discursive construction of identities by multilingual Chinese youth in Britain. J. Pragmat. 43, 1222–1235. doi: 10.1016/j.pragma.2010.07.035
Meredith, J. (2016). Transcribing screen-capture data: the process of developing a transcription system for multi-modal text-based data. Int. J. Soc. Res. Methodo. 19, 663–676. doi: 10.1080/13645579.2015.1082291
Meredith, J. (2017). Analysing technological affordances of online interactions using conversation analysis. J. Pragmat. 115, 42–55. doi: 10.1016/j.pragma.2017.03.001
Paulus, T., Warren, A., and Lester, J. N. (2016). Applying conversation analysis methods to online talk: a literature review. J. Pragmat. 12, 1–10. doi: 10.1016/j.dcm.2016.04.001
Sandel, T., Ou, C., Wangchuk, D., Ju, B., and Duque, M. (2018). Unpacking and describing interaction on Chinese WeChat: a methodological approach. J. Pragmatics . doi: 10.1016/j.pragma.2018.08.011
Shang, C. (2001). The style of online chatting. Contemp. Rhetoric. 32–33. doi: 10.16027/j.cnki.cn31-2043/h.2001.05.021
Song, C., and Chen, X. (in preparation). Bracket annotating in Chinese online communication: Communicative orientations pragmatic functions.
Sun, Y., and He, R. (2006). A comparative study of English natural conversation and online chat room conversation from the perspectives of turn and turn taking. Foreign Lang. Teach. Learn. 13–16. doi: 10.3969/j.issn.1004-6038.2006.02.004
Tannen, D. (1989). Talking Voices: Repetition, Dialogue, and Imagery in Conversational Discourse . Cambridge: Cambridge University Press.
van Leeuwen, T. (2008). Discourse and Practice . Oxford: Oxford University Press.
Wang, Y., Fang, W.-C., Han, J., and Chen, N.-S. (2016). Exploring the affordances of WeChat for facilitating teaching, social and cognitive presence in semi-synchronous language exchange. Australas. J. Educ. Technol . 32, 18–37. doi: 10.14742/ajet.2640
Wu, Y., and Yu, G. (2017). Putting conversation analysis in the right perspective. J. Shanxi Univ. 40, 85–90. doi: 10.13451/j.cnki.shanxi.univ(phil.soc.).2017.01.011
Xu, T., and Li, B. (2012). The conversation analysis of online chatting language from the pragmatic perspective. J. Shanxi Univ. 100–102.
Yu, G., and Drew, P. (2017). The role of búshì (不是) in talk about everyday troubles and difficulties. East Asian Pragmat. 2, 195–227. doi: 10.1558/eap.34673
Yu, G., and Li, F. (2009). Conversation analysis: a sociological research methodology in favor of language facts. Sci-Tech Phil. Res. 26, 14–17.
Yu, G., and Wu, Y. (2020). Special issue: social actions in Mandarin conversations. East Asian Pragmat. 5, 289–294. doi: 10.1558/eap.39905
Yus, F. (2011). Cyberpragmatics: Internet-Mediated Communication in Context . Amsterdam and Philadelphia: John Benjamins.
Zhong, X. (2017). “Backchanneling in Internet interactions: implications on netiquette, in Politeness Phenomena across Chinese Genre , eds X. Chen (Sheffield: Equinox), 47–70.
Keywords: online typed chatting, WeChat, conversation analysis, internet pragmatics, cyberpragmatics
Citation: Chen X and Chen T (2023) The naturalness of Chinese online chatting: Organization and recontextualization. Front. Commun. 8:994192. doi: 10.3389/fcomm.2023.994192
Received: 14 July 2022; Accepted: 09 January 2023; Published: 23 February 2023.
Reviewed by:
Copyright © 2023 Chen and Chen. This is an open-access article distributed under the terms of the Creative Commons Attribution License (CC BY) . The use, distribution or reproduction in other forums is permitted, provided the original author(s) and the copyright owner(s) are credited and that the original publication in this journal is cited, in accordance with accepted academic practice. No use, distribution or reproduction is permitted which does not comply with these terms.
Disclaimer: All claims expressed in this article are solely those of the authors and do not necessarily represent those of their affiliated organizations, or those of the publisher, the editors and the reviewers. Any product that may be evaluated in this article or claim that may be made by its manufacturer is not guaranteed or endorsed by the publisher.
ScholarChat
GPT-4o mini
Claude 3 Haiku
ScholarChat is a state-of-the-art AI academic research assistant. This tool allows you to access over 200M+ scholarly papers and gather science-based answers to any query with accurate citations also included. It covers countless topics and subjects such as business, history, chemistry, physics, biology, mathematics, and more.

IMAGES
COMMENTS
By a correlational and cross-sectional research design, the current study testified the mediating role of two factors—social connectedness and perceived social support in the relationship between online chatting and three indicators of psychological well-being (happiness, self-esteem, and loneliness) for adolescents.
While in the online chatting method, all conversations, chats, voices, and attached documents are recorded automatically in the mobile app. Researchers can access the stored archive and re-read the content. ... Cases in Online Interview Research, 277-296. 10.4135/9781506335155.n11 [Google Scholar] Free Dictionary . (2022). Chat. https://www ...
More specifically, much of this research has focused on the features and affordances of different online platforms. For example, in the early days of online interaction, popular platforms such as chat rooms were often anonymous. Online anonymity has been linked to both positive and negative consequences.
The reported correlation of increased online chatting with declining reading literacy and awareness of reading strategies provides empirical support for concerns raised by scholars such as Carr (2010) and Wolf (2018). Research thus far on the impact of ICT use has not revealed clear-cut effects in large-scale surveys.
3.1. Theme 1: Features and affordances of online contexts. Broadly, this theme outlines perceived differences in young adults' interactions and self-expressions across online and offline contexts as they relate to known features and affordances of online settings (Walther, 1996).The Features and Affordances theme was the smaller of the two themes. . Young adults in this study who identified ...
The current study aimed to provide more insight into the role of online chatting in young adults' emotional adjustment. A model was tested that takes into account (a) extraversion of individuals who communicate online, (b) the kind of peers these individuals communicate with online (i.e., online-exclusive peers vs. friends), and (c) the extent to which effects of online chatting on emotional ...
In this experiment, which was designed to study the acquaintance process, we randomly assigned 417 participants into three conditions: face-to-face (FTF) chat with a human, online chat with a human, and online chat with a commercially-available, emotionally-responsive chatbot, Replika. After a 20-min getting-acquainted chat, participants ...
The current study aimed to provide more insight into the role of online chatting in young adults' emotional adjustment. A model was tested that takes into account (a) extraversion of individuals who communicate online, (b) the kind of peers these individuals communicate with online (i.e., online-exclusive peers vs. friends), and (c) the extent to which effects of online chatting on emotional ...
How do users behave in online chatrooms, where they instantaneously read and write posts? We analyzed about 2.5 million posts covering various topics in Internet relay channels and found that user ...
Student participation, as a significant indicator of class learning, has been investigated from various perspectives. The present research seeks to explore student participation by drawing on text data from the chat box of an online learning platform. The two main research questions concern the main types of student participation indicated by the online chat data as well as how extensively and ...
Online interviews and chatting were conducted among 17 clinical nurses who were purposively selected from March to June 2020. Data were audio-recorded, transcribed, and validated among researchers.
Considerable research on computer-mediated communication has examined online communication between strangers, but little is known about the emotional experience of connectedness between friends in digital environments. ... in-person, video chat, audio chat, and instant messaging (IM). Bonding in each condition was measured through both self ...
effect of online chat counseling, of which two found that individual online synchronous chat was equivalent to face-to-face help (Cohen and Kerr , 1998; Murphy et al., 2009); one found that it was ...
Qualitative research methods allow researchers to understand the experiences of patients, nurses, and other healthcare professionals. Qualitative research also provides scientists with information about how decisions are made and the aspects of existing interventions. However, to get to obtain this …
Abstract. This article looks at online Internet chatting as a means to resolve simulation tasks in lieu of face-to-face negotiation. It is suggested here that online chatting extends linguistic opportunities for participation in small-group settings in a much more egalitarian manner, while retaining the interactive qualities of spoken conversation.
In most western countries, online chat platforms and chatbots are increasingly being used in HE to boost student engagement (Studente et al., Citation 2020) ... Considering the paucity of research on the impact of using chatbots and creating student-led online communities in HE, this paper contributes to the literature of mature students in HE ...
This research examines the motivations individuals give for Web-based chatting and attempts to understand the breakdown of barriers to online chatting. In this article, we view Web-based chatting as an online tool that allows for interactive consumer communications, both socially and commercially. We focus on Web-based chatting as a human ...
The research shows a strong correlation between the country-by-country increase in online chatting among 15-year-olds and the country-by-country decline in both reading skills and awareness of ...
This application provides users with the ability to create. accounts, join chat rooms, and send mess ages to other users in real-time. With the use of web sockets and Angular's two-way binding ...
Still, academic research on attitudes about online dating at the turn of the millennium found that college students, for example, had more negative than positive attitudes about online dating. ... They use voice and text chat while engaging in dating activities and conversation stimulators to get a better sense of each other." ...
In 2022, Professor of Cognitive Neuroscience Dean Mobbs began to investigate the relationship between social media use and mental health and well-being. As his research program ramps up to test brain activity and physiological markers of stress during social media use, Mobbs and his colleagues, postdocs Swati Pandita, Ketika Garg, and Jiajin Zhang, have constructed a theoretical model to ...
Qualitative research methods allow researchers to understand the experiences of patients, nurses, and other healthcare professionals. Qualitative research also provides scientists with information about how decisions are made and the aspects of existing interventions. However, to get to obtain this important information, qualitative research requires holistic, rich, and nuanced data that can ...
1 China Research Center for Language Studies, Nanjing University, Nanjing, China; 2 Institute of Education, University College London, London, United Kingdom; Two-party or multi-party typed chatting on social media platforms is becoming a popular object of study in pragmatic research nowadays. Apparently, such chatting is very often non-synchronous and non-spontaneous and thus is arguably not ...
ScholarChat. ScholarChat is a state-of-the-art AI academic research assistant. This tool allows you to access over 200M+ scholarly papers and gather science-based answers to any query with accurate citations also included. It covers countless topics and subjects such as business, history, chemistry, physics, biology, mathematics, and more.